- Department of Obstetrics and Gynecology, The Third Affiliated Hospital of Chongqing Medical University, Chongqing, China
Ovarian cancer is the most deadly gynecologic malignancy worldwide and it is warranted to dissect the critical gene regulatory network in ovarian cancer. N6-methyladenosine (m6A) RNA methylation, as the most prevalent RNA modification, is orchestrated by the m6A RNA methylation regulators and has been implicated in malignant progression of various cancers. In this study, we investigated the genetic landscape and expression profile of the m6A RNA methylation regulators in ovarian cancer and found that several m6A RNA methylation regulators were frequently amplified and up-regulated in ovarian cancer. Utilizing consensus cluster analysis, we stratified ovarian cancer samples into four clusters with distinct m6A methylation patterns and patients in these subgroups displayed the different clinical outcomes. Moreover, multivariate Cox proportional hazard model was used to screen the key m6A regulators associated with the prognosis of ovarian cancer and the last absolute shrinkage and selection operator (LASSO) Cox regression was used to construct the gene signature for prognosis prediction. The survival analysis exhibited the risk-gene signature could be used as independent prognostic markers for ovarian cancer. In conclusion, m6A RNA methylation regulators are associated with the malignant progression of ovarian cancer and could be a potential in prognostic prediction for ovarian cancer.
Introduction
Ovarian cancer is a gynecologic malignancy with the most deaths worldwide (Narod, 2016). Nearly 90% of all ovarian cancer is epithelial ovarian cancer, which contributes to 70% of ovarian cancer deaths (Jayson et al., 2014). Despite large efforts toward better prognosis of ovarian cancer patients, the 5-year overall survival rate is lower than 40% because of the high relapse rate and drug resistance (Doherty et al., 2017; Reid et al., 2017). Thus, ovarian cancer remains a threat to women and enhancing understanding of underlying mechanisms and identification of critical gene regulatory network in ovarian cancer progression are conducive to prediction and developing new therapies for ovarian cancer.
m6A RNA methylation is the most abundant RNA epigenetic modification in mammals (Roundtree et al., 2017). m6A RNA methylation is a reversible and dynamic process mediated by methylation transferases and demethylation transferases (Jia et al., 2011; Zheng et al., 2013; Liu et al., 2014). To date, the complex including methyltransferase-like 3/14 (METTL3/14), and Wilms’ tumor 1-associating protein (WTAP) was identified as the core m6A methylation transferases and acts as m6A methylation “writers” (Schöller et al., 2018). Other factors including Vir like m6A methyltransferase associated (VIRMA, also named as KIAA1429), RNA binding motif protein 15/15B (RBM15/15B), and zinc finger CCCH domain-containing protein 13 (ZC3H13) were also demonstrated to be involved in m6A methylation deposition (Schwartz et al., 2014; Meyer and Jaffrey, 2017; Wen et al., 2018). Regarding demethylation transferases, obesity-associated protein (FTO) and alkB homolog 5 (ALKBH5) act as m6A methylation “erasers” and could decrease the m6A modification in RNA (Jia et al., 2011; Zheng et al., 2013). Besides m6A methylation “writers” and “erasers,” a group of RNA binding proteins were found to specifically recognize m6A modified RNAs and decide the fate of RNA through regulating RNA splicing, turnover, export and translation (Haussmann et al., 2016; Patil et al., 2018; Zhao et al., 2018). m6A methylation “readers” are consisted of YTH domain family YTHDF1-3, YTHDC1-2, insulin-like growth factor 2 mRNA-binding proteins IGF2BP1-3, heterogeneous nuclear ribonucleoprotein A2B1 (HNRNPA2B1), heterogeneous nuclear ribonucleoprotein C (HNRNPC) and embryonic lethal abnormal vision Drosophila like 1 (ELAVL1) (Wang et al., 2014, 2015; Wojtas et al., 2017; Sun et al., 2019). m6A RNA methylation is an important RNA epigenetic regulation mode and leads to a complex gene regulatory network through the posttranscriptional control. Recent studies revealed that dysregulation of m6A RNA methylation has been significantly implicated in various diseases especially in development of cancers (Geula et al., 2015; Yoon et al., 2017; Chen et al., 2019; Esteve-Puig et al., 2020). For example, endometrial cancer is associated with a reduced level of m6A mRNA methylation because of decreased expression of METTL3 and METTL14 and reduced m6A methylation promotes the proliferation of endometrial cancer cell (Liu et al., 2018). METTL3 has been also reported to inhibit myeloid differentiation of normal hematopoietic and leukemia cells (Vu et al., 2017). On the contrary, METTL3 was up-regulated in human hepatocellular carcinoma and lung cancer (Chen et al., 2018; Choe et al., 2018). METTL3 directed m6A modification of tumor suppressor gene SOCS2 and silenced its expression depending on YTHDF2-mediated degradation pathway, which promoted the progression of hepatocellular cancer (Chen et al., 2018). In lung cancer, METTL3 enhanced circularization and translation of m6A modified mRNAs, and thus promoted oncogenesis (Choe et al., 2018). FTO was revealed as the first RNA m6A demethylase which is highly expressed in several AML subtypes (Barbieri et al., 2017). FTO plays an oncogenic role through facilitating cell proliferation and leukemogenesis, and inhibiting all-trans-retinoic acid-mediated differentiation of leukemia cells. R-2HG, a specific small molecule inhibitor of FTO, displays anti-leukemia activity by suppressing FTO/m6A/MYC signaling (Su et al., 2018). In our previous study, we found that the m6A “reader” YTHDF1 aggravated ovarian cancer progression by enhancing EIF3C translation in an m6A-dependent manner (Liu et al., 2020). However, the role of m6A RNA methylation-mediated gene regulatory network in diagnosis and treatment of ovarian cancer is largely unexplored.
In this study, we examined the genetic variations and gene expression of m6A RNA methylation regulators. We found several m6A RNA methylation regulators were amplified and up-regulated in ovarian cancer. We also identified the hub genes by protein interaction analysis and a signature gene for prognostic predication of ovarian cancer. Moreover, we classified the ovarian cancer patients into four subgroups with distinct overall survivals based on the expression of 20 m6A RNA methylation regulators. Our study demonstrated that m6A RNA methylation regulators have an important value in prognostic prediction for ovarian cancer.
Materials and Methods
m6A Regulators
According to the mRNA expression detected by the Cancer Genome Atlas (TCGA) database, 20 m6A regulators including 7 “writers” (KIAA1429, METTL3, METTL14, RBM15, RBM15B, WTAP, ZC3H13), 2 “erasers” (ALKBH5, FTO) and 11 “readers” (YTHDC1-2, YTHDF1-3, IGF2BP1-3, HNRNPA2B1, HNRNPC, ELAVL1) were analyzed in this study.
m6A Regulators Mutation and Copy Number Variation Analysis
The workflow of our study was shown in Supplementary Figure 1. DNA mutation and copy number variation data were downloaded from the Genomic Data Commons (GDC)1. The R bioconductor package maftools was used for somatic mutation investigation of the m6A regulators (Mayakonda et al., 2018). The Pan cancer project of TCGA-OV was used in our study.
Microarray Datasets and Differentially Expressed Genes Analysis
Gene Expression Omnibus (Choe et al., 2018) database was selected to study the differential expression of 20 m6A regulators between normal tissues and tumor tissues. Datasets containing 4 normal samples with expression of all the 20 m6A regulators were selected in this study. 4 normalized matrix files (GSE27651, GSE52037, GSE54388, and GSE66957) were selected and downloaded from GEO. Batch effects were corrected by sva package (Leek and Storey, 2008) and differential expression was calculated by limma package (Ritchie et al., 2015).
Interaction Between 20 m6A Regulators
Protein-Proterin interaction was constructed using the STRING 11.0b website2. The RNA expression correlation among the m6A regulators was conducted by R package corrplot. RNA expression data (FPKM) was downloaded from GDC.
Clustering Analysis of 20 m6A Regulators
ConsensusClusterPlus package (Wilkerson and Hayes, 2010) was used to classify the TCGA patients to identify distinct m6A phenotype based on the expression of 20 m6A regulators and 1,000 times repetitions were conducted to make sure our classification is stable.
Tumor Microenvironment Cell Infiltration Estimate
The TCGA ovarian cancer immune cell type information predicted by deconvolution algorithm was downloaded from the CIBERSORT website3. ESTIMATE was conducted by estimate package to quantify the overall stromal cells, immune cells, and tumor purity of individual TCGA patients. To further qualify the relative levels of different activated or naïve immune cell types infiltration among the distinct m6A clusters, the enrichment score of 23 immune cell types which reported in pancancer (Charoentong et al., 2017) were calculated by ssGSEA (single-sample gene-set enrichment analysis) algorithm from gsva package (Hänzelmann et al., 2013).
Cluster Function Annotation
First of all, R package GSVA was used to study the KEGG pathway enrichment among different m6A clusters. Secondly, functional annotation of each m6A clusters was performed by R package ClusterProfiler (Yu et al., 2012) among the top 500 expressed genes. Thirdly, differential gene expression analysis was conducted using limma package between each cluster and the rest patients, and then used overexpressed analysis by ClusterProfiler package to identify the individual cluster.
Survival Analysis
Overall survival analysis was conducted using the integrated microarray datasets4 through Kaplan-Meier method. Survival and survminer packages (Scrucca et al., 2007) were used to establish the univariate Cox proportional hazards model and overall survival plot. Genes with the p < 0.1 were selected to lasso regression. Receiver operating characteristic curve (ROC) and area under curve (AUC) were calculated by R package survivalROC (Heagetry and Zheng, 2005). Patients with survival information were randomly divided into two subgroups (75% in training group and 25% in test group) by createDataPartition function from caret package. Four gene risk signature and their corresponding coefficient were determined in the training group by glmnet function. Risk score was calculated for each patient using prediction function. The best cutoff value for our model was selected as follow: true positive (TP) and false positive (FP) of every patient in training group was calculated through survivalROC function, Risk score of the patients with the minimum value of the formula (TP-1)2 + FP2 was determined as the best cutoff value. This cutoff value was used in training group, test group and external validation set to divide the sample into high-risk group and low-risk group. R package forestplot and survminer were used for visualized the Cox results and survival curves, respectively.
Statistical Analysis
Co-occurrence of CNV and mRNA expression correlation among different m6A regulators were calculated by Spearman correlation analyses by corrplot package. Kruskal-Wallis test was employed to compare gene expression among different samples. R 4.0.3 was used for all the statistical analysis in this study. p < 0.05 is the significance threshold for all the data.
Results
Landscape of Genetic Variation and Expression Patterns of m6A Regulators in Ovarian Cancer
We first analyzed the mutation status and copy number variation of 20 m6A regulator genes including m6A “writers,” “erasers” and “readers” in TCGA ovarian cancer database. These genes displayed different copy number variations in ovarian cancer but low frequency of mutations occurred in all these genes (Figure 1A). IGF2BP2, KIAA1429 and YTHDF1 genes were highly amplified with amplification frequencies of 18%, 7% and 6%, respectively. HNRNPC, YTHDC2 and ZC3H13 genes were depleted in ovarian cancer (Figure 1B). Moreover, we analyzed the co-occurrence of DNA mutation and amplification among the m6A regulators respectively. Co-occurrence of DNA mutation is rarely and only 5 of 8 pair of genes significantly co-exist in the same patients (Figure 1C). DNA copy number variation is rather pervasive and all the co-exist copy number variation are positive related (Figure 1D). Additionally, we selected four GSE datasets to examine the expression of m6A-related genes and found that these genes were usually up-regulated in ovarian cancer compared to normal tissues (Figures 1E,F).
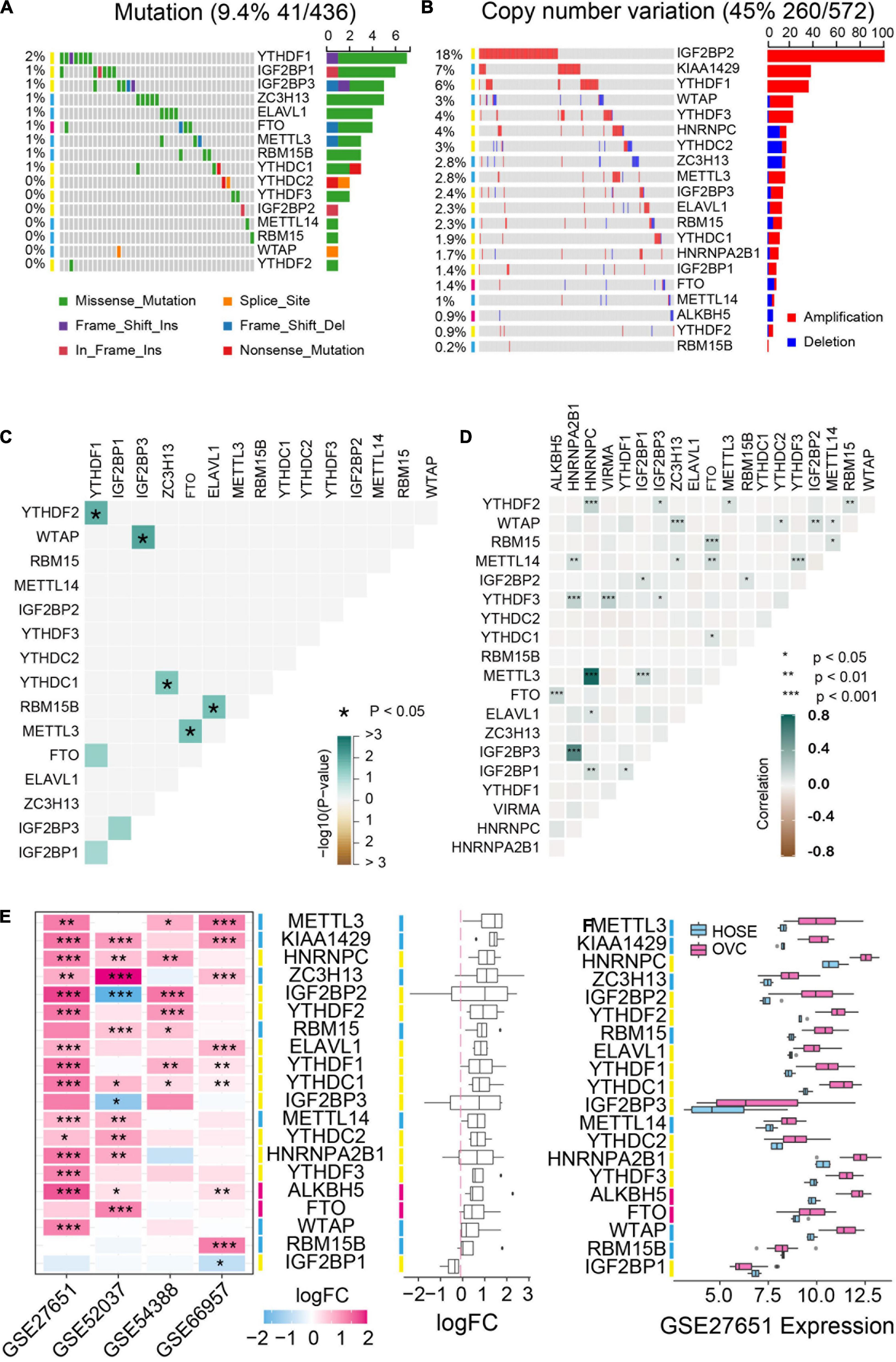
Figure 1. Genetic variation and expression of m6A regulators in ovarian cancer. (A) The mutation frequency of m6A regulators in ovarian cancer available at TCGA database by using maftools package. (B) Copy number variations of m6A regulators in ovarian cancer available at TCGA database by using cBioPortal (http://cbioportal.org). (C) The mutation co-occurrence and exclusion analysis for m6A regulators. (D) The copy number variation co-occurrence and exclusion analysis for m6A regulators. (E) The mRNA expression alterations of m6A regulators in four independent GEO microarray datasets. (F) The mRNA expression of m6A regulators in GSE27651 cohort. HOSE, human ovarian surface epithelium, OVC, ovarian cancer. *P < 0.05, **P < 0.01, ***P < 0.001.
Interaction and Correlation Analysis Between m6A RNA Methylation Regulators
To understand the mutual interaction of 20 m6A RNA methylation regulators, a protein-protein interaction (PPI) network using Cytoscape was constructed based on the STRING 11.0b database. As shown in Figure 2A, the 20 m6A RNA methylation regulators displayed the complex interactions. The writers including METTL14 and WATP interacted with each other and were the hub genes. Moreover, the correlation analysis was conducted to analyze the correlation among these regulators in ovarian cancer. Part of the different m6A RNA methylation regulators showed weakly to moderately positive correlation (Figure 2B). Among 20 m6A RNA methylation regulators, YTHDF2 was positively correlated with all of the m6A RNA methylation regulators except YTHDC2 (Figure 2B). We also found that tumors with a high expression of writer genes (METTL14, RBM5B, RBM15, and KIAA1429) co-expressed with a high expression of “eraser” genes FTO and ALKBH5, whereas a high expression of writer gene WTAP had no correlation with the expression of FTO and ALKBH5 (Figures 2C-I). Considering the high amplification frequency of KIAA1429, we analyzed the differential expression of “eraser” genes in tumors with the distinct copy number variations. We found that both of “eraser” genes were down-regulated in KIAA1429-amplified tumors compared to wide-type tumors (Figure 2J). These results demonstrated that m6A RNA methylation regulators formed a complex regulatory network which contributed to the dynamics of m6A RNA methylation in ovarian cancer.
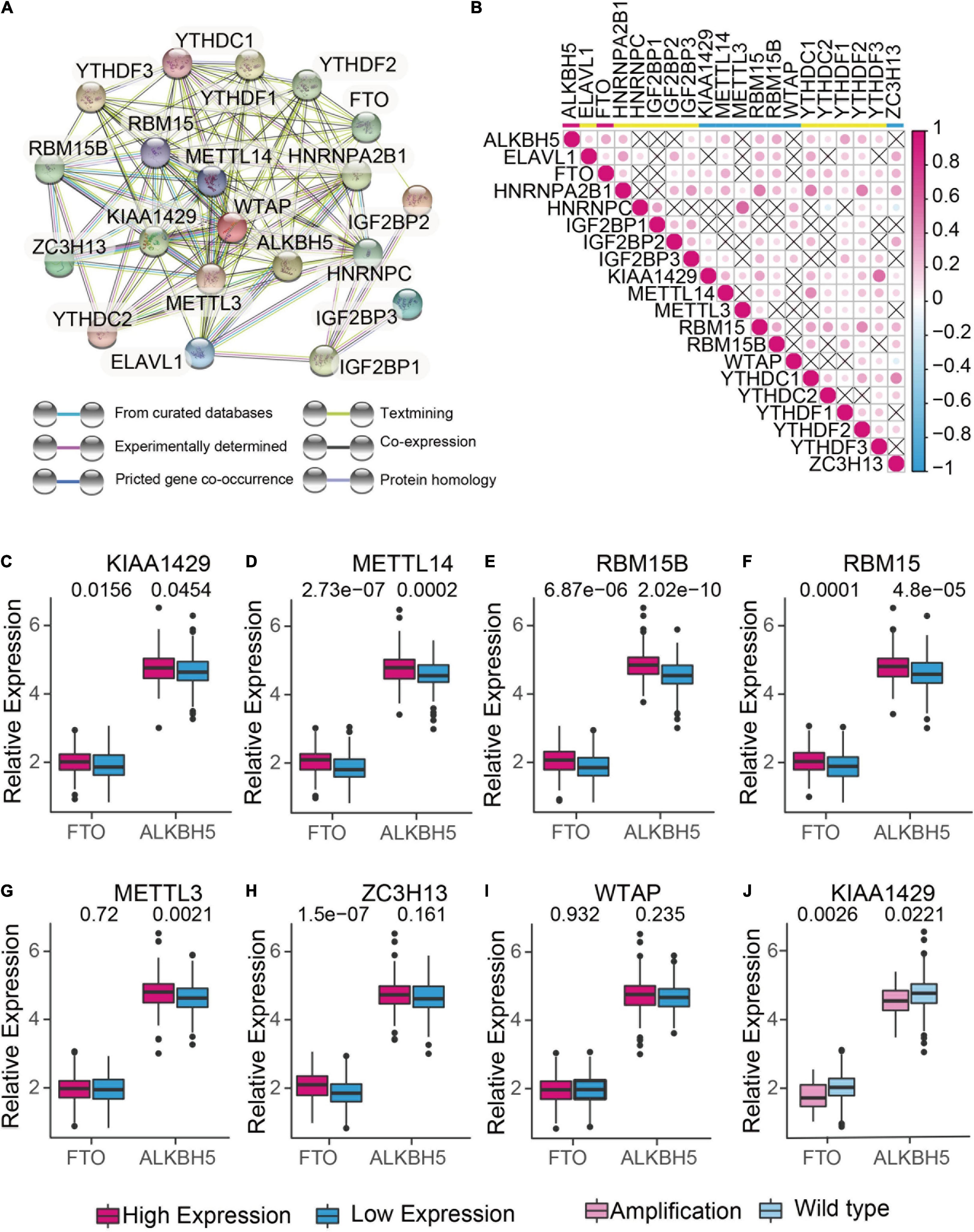
Figure 2. The interaction and correlation analysis between m6A regulators in ovarian cancer. (A) Protein-Protein Interactions among the 20 m6A RNA methylation regulators using STRING 11.b. (B) Spearman correlation analysis of 20 m6A RNA methylation regulators in TCGA cohorts using R. (C-I) Correlation analysis between the expression of “erasers” (FTO and ALKBH5) and “writers” (KIAA1429, METTL14, RBM15, RBM15B, METTL3, ZC3H13, and WTAP). Median expression value was used to divide the patients into high and low expression groups. (J) Difference in the gene expression of “erasers” between KIAA1420-amplified and wild types.
Consensus Clustering of m6A RNA Methylation Regulators Identified Four Clusters of Ovarian Cancer With Distinct Clinical Outcomes
To examine the clinical relevance of m6A RNA methylation regulators in ovarian cancer, the ConsensusClusterPlus tool was used to separately cluster the TCGA ovarian cancer samples into subgroups according to the gene expression patterns of m6A RNA methylation regulators. Four distinct modification patterns (cluster 1-4) were identified using unsupervised clustering while k = 4 (Figures 3A-C). Significant differences were found among these four subgroups regarding tumor grade and FIGO stage (Figure 3D). Furthermore, prognostic analysis for the four main m6A modification patterns in Figure 3E revealed that cluster 1 (C1) and cluster 3 (C3) had a better overall survival compared to cluster 2 (C2) and cluster 4 (C4).
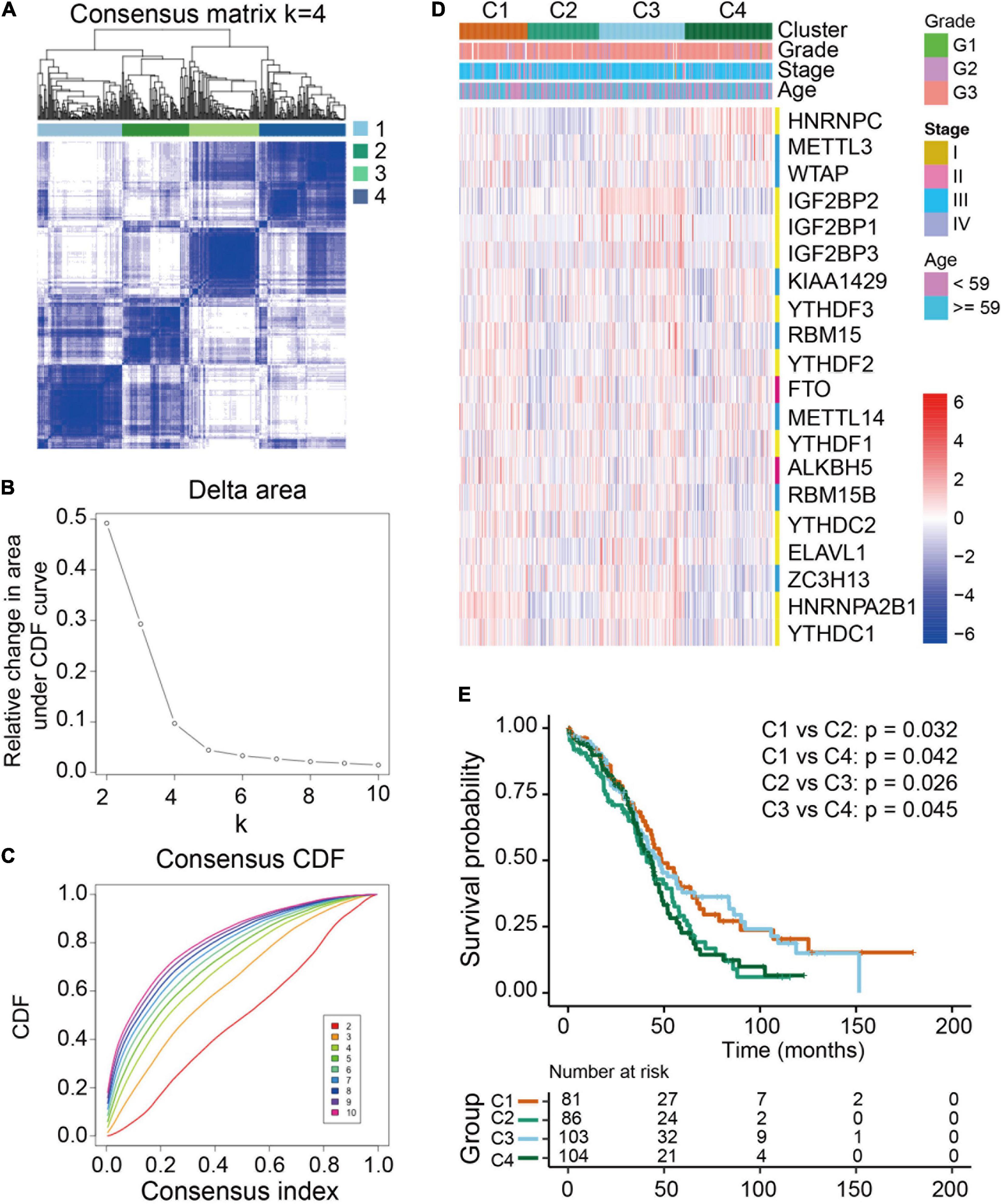
Figure 3. Differential clinicopathological features and overall survival of ovarian cancer in four clusters with distinct m6A RNA methylation regulator features. (A) Consensus clustering cumulative distribution function (CDF) for k = 2-10. (B) Relative changes in the area under the CDF curve at k = 2-10. (C) Consensus clustering matrix for k = 4. (D) Heatmap with the clinical and pathological characterizations for clusters according to m6A RNA methylation regulator consensus clustering. (E) Kaplan-Meier overall survival curves of the four clusters.
Evaluation of TME Cell Infiltration in Distinct m6A Modification Patterns
Then we evaluated the landscape of TME cells in 4 subgroups with distinct m6A modification patterns. As shown in Figures 4A,B, we found 23 TME cells presented different changes in infiltration among subgroups. For most immune cells, the relative quantity of immune cells in the C2 group and the C4 group was significantly higher than that in the C1 group and C3 group (Figure 4B). The correlation between the m6A RNA methylation regulators and 23 immune cells was analyzed. We found that the expression levels of most m6A RNA methylation regulators were highly related to the abundances of multiple immune cells (Figure 4C). Unexpectedly, activated B cells, eosinophil cells and natural killer cells were significantly enriched in the C2 subgroup, but patients in the C2 group did not present an advantaged prognosis (Figure 4B). Consistently, we used ESTIMATE algorithm to evaluate the immune activity in distinct m6A modification subgroups and found that the C2 or C4 subgroups exhibited a higher immune score than the C3 subgroup (Figure 4D). Stroma surrounding tumor cell nests was demonstrated to contribute to the immune excluded phenotype of tumors and thus the stroma activity of m6A modification subgroups was evaluated (Chen and Mellman, 2017). Figure 4E showed that the stroma activity in the C2 or C4 subgroups was also higher than that in the C3 subgroup.
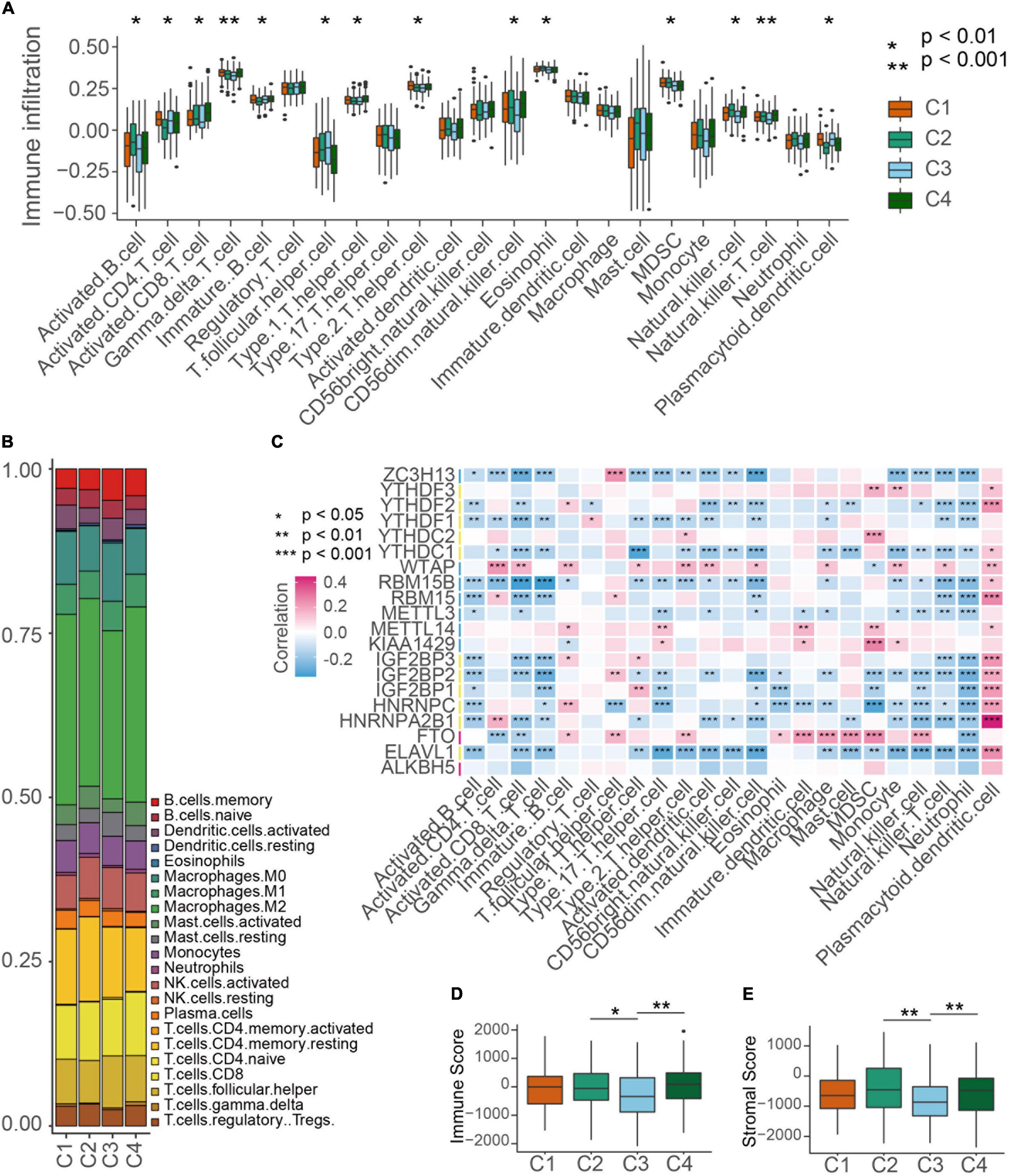
Figure 4. TME cell infiltration characteristics in distinct m6A modification patterns. (A) The abundance of each TME infiltrating cell type in four m6A modification patterns. The TME infiltrating cell types were defined by ssGSEA methods. The upper and lower ends of the boxes represented interquartile range of values. Kruskal-Wallis test was used to calculate the significance between poor prognosis groups and good prognosis groups. *P < 0.01, **P < 0.001. (B) The component differences of immune cells among the four m6A modification patterns analyzed by CIBERSORT. (C) The correlation between each m6A regulator mRNA levels and each TME infiltration cell type ssGSEA scores using spearman analyses. Red color means positive correlated and blue color means negative correlated. *P < 0.05, **P < 0.01, ***P < 0.001. (D,E) Differences of immuneScore and stromaScore in four subgroups by ESTIMATE. *P < 0.05, **P < 0.01.
Characteristics of Transcriptome Traits in Clustering Subgroups
To further explore the transcriptomic characterization of these m6A modification phenotypes, the top 500 expressed genes in each cluster were collected for gene ontology (GO) enrichment analysis using R ClusterProfiler packages. Consistent with the TME immune cell infiltration patterns, all the four m6A clusters top 500 expressed genes enriched in immune associated pathways (Figures 5A,B and Supplementary Figure 2A), which demonstrated that m6A modification is implicated in ovarian cancer TME. We also determined phenotype-related differential expression genes (DEGs) in different m6A clusters and performed GO enrichment analysis. The results showed that cell proliferation associated genes were enriched in Cluster 1 (Figure 5C). And both the canonical and non-canonical Wnt pathways were up-regulated in Cluster 2 (Figure 5D). For Cluster 3, genes involved in DNA repair pathways including homologous recombination and mismatch repair were significant up-regulated (Figure 5E). And energy metabolism pathways such as oxidative phosphorylation and aerobic respiration were highly expressed in Cluster 4 (Figure 5F). Then GSVA was conducted to investigate the potential KEGG pathways mediated by m6A regulators. We compared each m6A cluster with the other clusters and determined 168 phenotype-associated KEGG pathways with the threshold of p < 0.05. As expected, DNA repair pathways were enriched in Cluster 1, and pathways associated with energy metabolism exhibited remarkably high expression in m6A Cluster 4 (Supplementary Figure 2B).
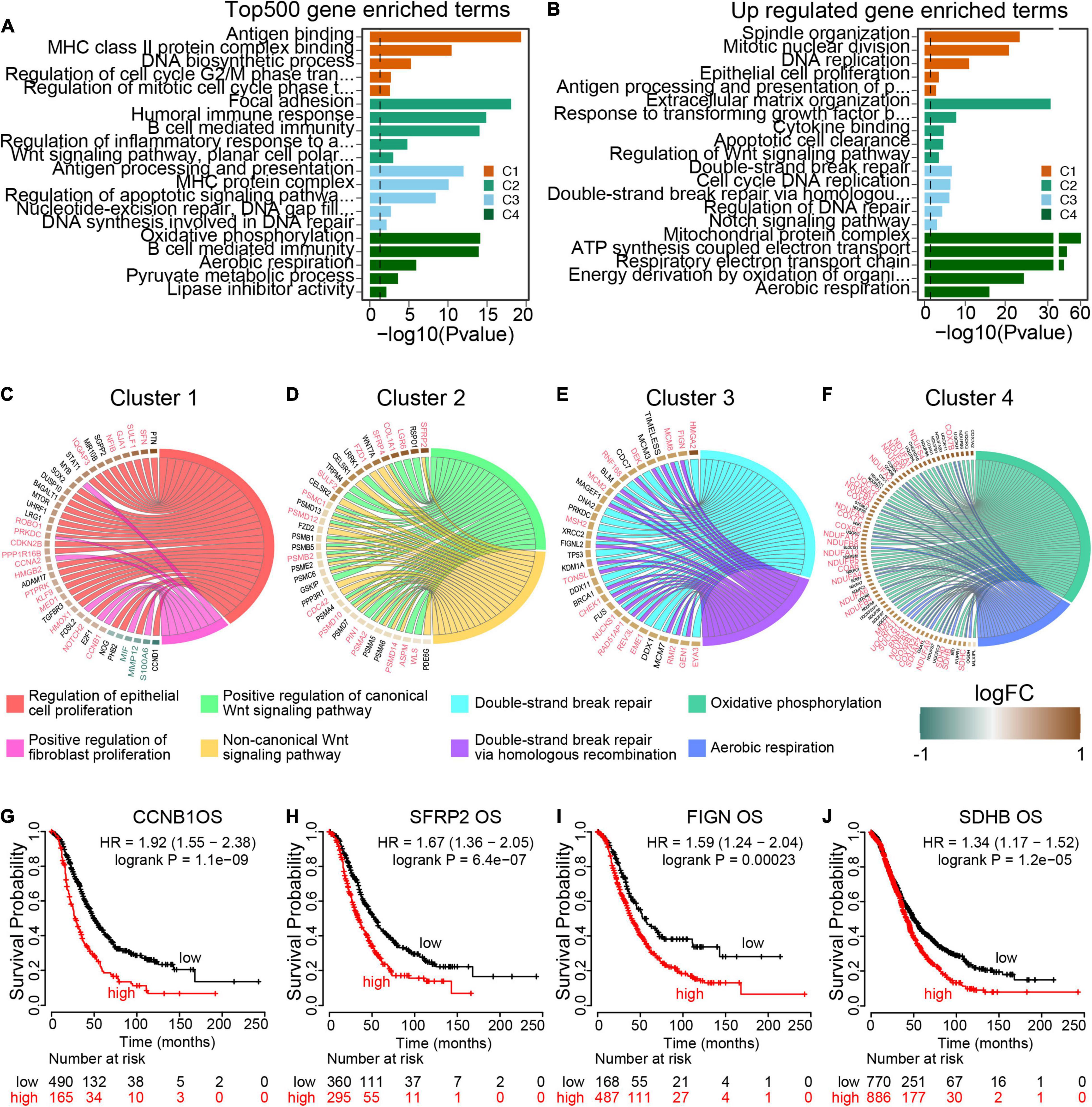
Figure 5. Transcriptome traits in distinct m6A modification patterns. (A) GO terms enrichment using the top 500 expressed genes in each m6A modification patterns. (B) GO terms enrichment using the upregulated genes from each cluster compared to the rest cases. (C–F) Circos plot exhibits the essential cancer associated GO term clusters of the four m6A modification patterns with the differential expressed genes (fold change >1.5 and p value <0.05). Genes with good prognosis in high grade serous ovarian cancer are highlighted by red color, and the green for good prognosis genes. Each square represents the fold change of genes between each m6A modification patterns and the rest cases. Brown color for upregulated genes and green color for downregulated genes. (G–J) Overall survival curves of representative genes enriched in each m6A modification patterns.
To identify target genes regulated by m6A regulators, we visualized the typical pathways of the four m6A modification phenotype clusters. Of 38 DEGs expressed in Cluster 1 specifically, 20 genes were markedly associated with ovarian cancer overall survival, including 17 up-regulated genes with poor prognosis and 3 down-regulated genes with good prognosis (Figure 5G and Supplementary Figure 2C). All the 35 DEGs within the Wnt pathway were up-regulated and out of 16 (45.71%) genes were oncogenes in ovarian cancer (Figure 5H and Supplementary Figure 2D). In Cluster 3, 32 homologous recombination repair associated genes were up-regulated, of which 16 genes predicted poor prognosis in ovarian cancer (Figure 5I and Supplementary Figure 2E). Among the 72 aerobic respiration related DGEs in Cluster 4, there were 33 (45.83%) genes highly associated with ovarian cancer survival (Figure 5J and Supplementary Figure 2F). All these results demonstrated that the m6A regulators were implicated in the prognosis of ovarian cancer, and more importantly, different pathways were activated to regulate tumor progression in different m6A modification phenotype clusters with distinct prognosis states.
Construction and Validation of a Risk Signature With Four Selected m6A Methylation Regulators
We next investigated the prognostic value of m6A RNA methylation regulators in ovarian cancer. A univariate Cox regression analysis was performed in training set concerning the expression levels of m6A RNA methylation regulators to identify the regulators associated with overall survival in TCGA ovarian cancer cohort. We found that 3 out of 20 regulators were significantly associated with overall survival, among which KIAA1429 and IGF2BP2 belonged to risky genes with HR > 1 while HNRNPA2B1 was the only protective gene with HR < 1 (Figure 6A). To more precisely predict the prognosis of ovarian cancer with m6A RNA methylation regulators, we applied the LASSO Cox regression algorithm to develop a risk signature in the training set. According to the minimum criteria, the survival risk score model was established as follow: risk score = −0.0158 ELAVL1 −0.00763 HNRNPA2B1 + 0.12218 IGF2BP1 + 0.0687 KIAA1429 (Figures 6B,C). The ROC curves displayed that prognosis prediction using the risk signature had an area under the ROC curve (AUC) value of 0.662 (1 year), 0.598 (3 years) and 0.602 (5 years) in training set (Figure 6D). To detect the prognostic role of the four-gene risk signature, we divided the ovarian cancer patients in both training set and test set into low-risk and high-risk group based on the lasso cutoff risk score calculated above and compared the overall survival of patients in different subgroups. Results indicated patients in high-risk group exhibited a worse overall survival than low-risk patients in both sets (Figures 6E,F). The distributions of four-gene signature-based risk scores as well as its corresponding expression profiles were shown in Figures 6G,H. Collectively, these results demonstrated that this risk signature could identify high-risk ovarian cancer patients with poor prognosis. Moreover, we confirm the prognostic role of the four-gene risk signature in an independent ovarian cancer dataset in UCSC database (Supplementary Figure 3). Collectively, our results demonstrated that the m6A regulators contributed to the progression and prognosis of ovarian cancer.
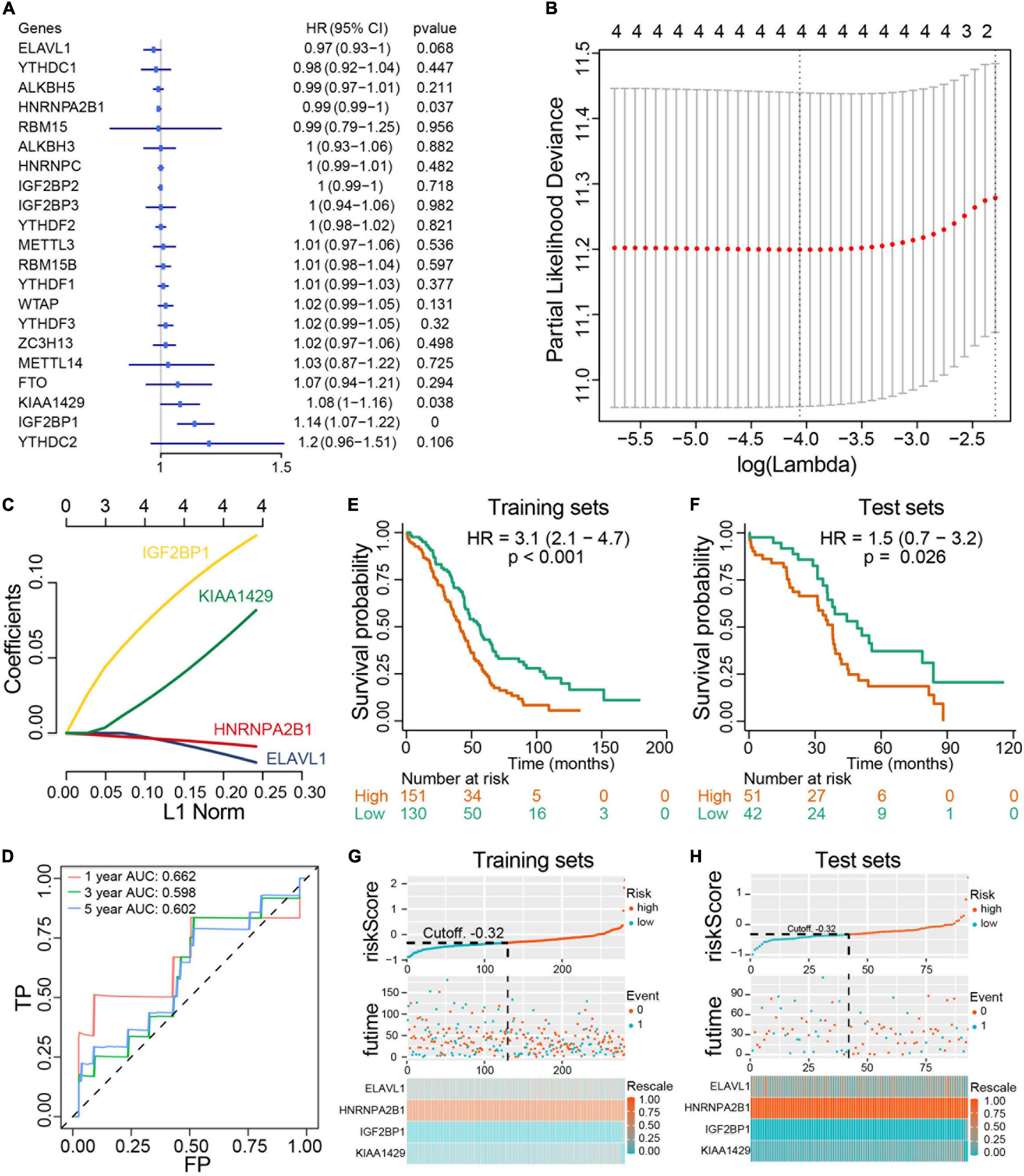
Figure 6. Construction and evaluation of prognostic risk signature with four m6A RNA methylation regulators in ovarian cancer cohorts. (A) Univariate Cox regression analysis results show the p values and hazard ratios (Liu et al.) with confidence intervals (CI) of the 14 differentially expressed m6A RNA methylation regulatory genes. (B,C) LASSO Cox regression analysis results show the identification of the 4 prognostic risk signature genes, and the risk score model is: risk score = 0.12218 × IGF2BP1 + 0.0687 × KIAA1429 - 0.0158 × ELAVL1 - 0.00763 × HNRNPA2B1. (D) The ROC curve for evaluating the prediction efficiency of the prognostic signature. (E,F) The survival analysis of training set and test set. Both two set were divided by the cutoff value according risk score calculated by LASSO multivariate Cox model. (G,H) The distributions of prognostic signature-based risk scores and its corresponding expression profiles in the training set and test set. The red dots represent high-risk patients and green dots represent low-risk patients.
Discussioin
Recent studies have demonstrated that m6A RNA methylation was implicated in tumorigenesis of various cancers (Esteve-Puig et al., 2020). Previously we explored the role of the specific m6A regulator YTHDF1 in ovarian cancer progression and found that YTHDF1 as the m6A “reader” could promote protein synthesis in an m6A -dependent manner, indicating that m6A RNA methylation might have a key value of prognostic prediction for ovarian cancer patients (Liu et al., 2020). In this study, we analyzed genetic variations and gene expression of the 20 m6A methylation regulators in TCGA ovarian cancer cohort as well as GSE ovarian cancer datasets. These m6A RNA methylation regulators exhibited the low frequency of mutations but the high frequency of copy number variation, which is consistent with previous notion that high grade serous ovarian cancer is driven by genomic copy number changes rather than point mutations (Cancer Genome Atlas Research Network, 2011). Despite that TP53 was highly mutated in ovarian cancer according to genomic analysis, few genes other than TP53 are mutated at a high level. Extensive copy number change in more than half of ovarian cancer contributed to tumorigenesis of ovarian cancer (Cancer Genome Atlas Research Network, 2011). In our study, we found that multiple m6A RNA methylation regulator genes were amplified in ovarian cancer. Especially, the m6A “reader” IGF2BP2 gene was predominantly amplified with a frequency of 18% in ovarian cancer and the high amplification rate of IGF2BP2 was also reported in other cancers. IGF2BP2 was involved in the development of various cancers including colorectal carcinoma, liver cancer and cervical cancer through recognizing m6A modified RNAs and regulating RNA stability and translation. Further investigations are warranted to confirm the role of IGF2BP2-mediated fate regulation of m6A modified RNA in ovarian cancer. Besides gene amplification of multiple m6A RNA methylation regulators in ovarian cancer, most of the m6A RNA methylation regulators exhibited upregulation at the RNA level in ovarian cancer such as METTL3, KIAA1429, HNRNPC, ZC3H13 as well as IGF2BP2, suggesting the critical unexplored functions of m6A RNA methylation in ovarian cancer.
Notably, the m6A writers METTL3, KIAA1429, METTL14, and WTAP constructed the hub genes in m6A RNA methylation regulator interaction network according to PPI in ovarian cancer. Though acting as m6A writers, these m6A RNA methylation regulators exerted distinct effects on the progression of different cancers. For instance, METTL3 initiated m6A mRNA methylation to promote drug resistance and metastasis of non-small-cell lung cancer by enhancing the translation and activity of YAP (Jin et al., 2019). On the contrary, reduced METTL3 expression followed by reductions in m6A methylation increased AKT activity and thus promoted the proliferation and tumorigenesis of endometrial cancer (Liu et al., 2018). Upregulation of METTL14 induced PERP elevation and promoted the growth and metastasis of pancreatic cancer (Wang et al., 2020). However, METTL14 mediated the N6-methyladenosine modification of SOX4 mRNA and suppressed the metastasis of colorectal cancer (Chen et al., 2020). In terms of WTAP, it acted as an oncogene in hepatocellular carcinoma and high-grade serous ovarian carcinoma (Yu et al., 2019). KIAA1429 contributed to the progression of liver cancer and breast cancer (Lan et al., 2019; Qian et al., 2019). These studies suggest that regulatory network formed by m6A RNA methylation is complex and depends on cellular contexts. Thus we constructed an m6A RNA methylation regulators-based signature for predicting the prognosis of ovarian cancer. According to the four- m6A RNA methylation regulator signature, the ovarian cancer patients in both training set and test set could be stratified into high-risk group and low-risk group, and patients in high-risk group had a worse prognosis than that in low-risk group, suggesting its good performance for prognostic prediction. The signature genes included IGF2BP1, KIAA1429, HNRNPA2B1, and ELAVL1, among which HNRNPA2B1 acts as a protective gene. Although our previous study demonstrated that loss of HNRNPA2B1 inhibited the growth and metastasis of ovarian cancer, this oncogenic role of HNRNPA2B1 is likely independent of m6A RNA methylation (Yang et al., 2020).
By an unsupervised clustering based on 20 m6A RNA methylation regulators, patents in TCGA ovarian cancer cohort were divided into four clusters and different clusters showed the distinct m6A RNA methylation patterns and overall survival. Intriguingly, we found higher TME immune cell infiltration as well as higher stroma score in clusters with worse prognostic patients, suggesting that immune cells might be retained in the stroma and were suppressive in these clusters of ovarian cancer patients as the previous study reported (Chen and Mellman, 2017). Subsequently, GSVA enrichment analysis was conducted to comprehensively understand the characterization in clusters with different m6A RNA methylation patterns. The results showed that each cluster enriched distinct patterns of key genes and regulatory pathways.
In conclusion, our study explored genetic variation and the prognostic value of m6A RNA methylation regulators in ovarian cancer, and a four-gene signature was found to predict the prognosis of ovarian cancer. We also demonstrated the key regulatory pathways associated with m6A RNA methylation and more investigation might be required to decode the precise role of specific m6A RNA methylation regulators as well as their related genes or regulatory pathways.
Data Availability Statement
The original contributions presented in the study are included in the article/Supplementary Material, further inquiries can be directed to the corresponding author/s.
Ethics Statement
In accordance with the local legislation and institutional requirements, ethical review and approval was not required for the study on human participants.
Author Contributions
PY and TL designed the research study. QW, DY, XL, HZ, YY, and JX analyzed the data. QW, DY, and XL wrote the manuscript and interpreted the data. All authors read and approved the final manuscript.
Funding
This work was sponsored by the Natural Science Foundation of Chongqing, China (cstc2018jcyjAX0576 and cstc2020jcyj-msxmX0344), the Postdoctoral Science Foundation of Chongqing, China (cstc2019jcyj-bshX0129), the National Natural Science Foundation of China (81902668), and the Social Undertakings and People’s Livelihood Security Innovation of Science and Technology Special Project of Chongqing Yubei District Science and Technology Bureau, China (KY19036).
Conflict of Interest
The authors declare that the research was conducted in the absence of any commercial or financial relationships that could be construed as a potential conflict of interest.
Acknowledgments
The authors acknowledge contributions from TCGA databases.
Supplementary Material
The Supplementary Material for this article can be found online at: https://www.frontiersin.org/articles/10.3389/fgene.2021.650554/full#supplementary-material
Footnotes
- ^ https://portal.gdc.cancer.gov
- ^ https://string-db.org
- ^ http://cibersort.stanford.edu/
- ^ http://kmplot.com/analysis/index.php?p=background
References
Barbieri, I., Tzelepis, K., Pandolfini, L., Shi, J., Millán-Zambrano, G., Robson, S. C., et al. (2017). Promoter-bound METTL3 maintains myeloid leukaemia by m(6)A-dependent translation control. Nature 552, 126–131. doi: 10.1038/nature24678
Cancer Genome Atlas Research Network (2011). Integrated genomic analyses of ovarian carcinoma. Nature 474, 609–615. doi: 10.1038/nature10166
Charoentong, P., Finotello, F., Angelova, M., Mayer, C., Efremova, M., Rieder, D., et al. (2017). Pan-cancer immunogenomic analyses reveal genotype-immunophenotype relationships and predictors of response to checkpoint blockade. Cell Rep. 18, 248–262. doi: 10.1016/j.celrep.2016.12.019
Chen, D. S., and Mellman, I. (2017). Elements of cancer immunity and the cancer-immune set point. Nature 541, 321–330. doi: 10.1038/nature21349
Chen, M., Wei, L., Law, C. T., Tsang, F. H., Shen, J., Cheng, C. L., et al. (2018). RNA N6-methyladenosine methyltransferase-like 3 promotes liver cancer progression through YTHDF2-dependent posttranscriptional silencing of SOCS2. Hepatology 67, 2254–2270. doi: 10.1002/hep.29683
Chen, X., Xu, M., Xu, X., Zeng, K., Liu, X., Pan, B., et al. (2020). METTL14-mediated N6-methyladenosine modification of SOX4 mRNA inhibits tumor metastasis in colorectal cancer. Mol. Cancer 19:106. doi: 10.1186/s12943-020-01220-7
Chen, X., Yu, C., Guo, M., Zheng, X., Ali, S., Huang, H., et al. (2019). Down-regulation of m6A mRNA methylation is involved in dopaminergic neuronal death. ACS Chem. Neurosci. 10, 2355–2363. doi: 10.1021/acschemneuro.8b00657
Choe, J., Lin, S., Zhang, W., Liu, Q., Wang, L., Ramirez-Moya, J., et al. (2018). mRNA circularization by METTL3-eIF3h enhances translation and promotes oncogenesis. Nature 561, 556–560. doi: 10.1038/s41586-018-0538-8
Doherty, J. A., Peres, L. C., Wang, C., Way, G. P., Greene, C. S., and Schildkraut, J. M. (2017). Challenges and opportunities in studying the epidemiology of ovarian cancer subtypes. Curr. Epidemiol. Rep. 4, 211–220. doi: 10.1007/s40471-017-0115-y
Esteve-Puig, R., Bueno-Costa, A., and Esteller, M. (2020). Writers, readers and erasers of RNA modifications in cancer. Cancer Lett. 474, 127–137. doi: 10.1016/j.canlet.2020.01.021
Geula, S., Moshitch-Moshkovitz, S., Dominissini, D., Mansour, A. A., Kol, N., Salmon-Divon, M., et al. (2015). Stem cells. m6A mRNA methylation facilitates resolution of naïve pluripotency toward differentiation. Science 347, 1002–1006. doi: 10.1126/science.1261417
Hänzelmann, S., Castelo, R., and Guinney, J. (2013). GSVA: gene set variation analysis for microarray and RNA-seq data. BMC Bioinformatics 14:7. doi: 10.1186/1471-2105-14-7
Haussmann, I. U., Bodi, Z., Sanchez-Moran, E., Mongan, N. P., Archer, N., Fray, R. G., et al. (2016). m(6)A potentiates Sxl alternative pre-mRNA splicing for robust Drosophila sex determination. Nature 540, 301–304. doi: 10.1038/nature20577
Heagetry, P. J., and Zheng, Y. (2005). Survival model predictive accuracy and ROC curves. Biometrics 61, 92–105. doi: 10.1111/j.0006-341X.2005.030814.x
Jayson, G. C., Kohn, E. C., Kitchener, H. C., and Ledermann, J. A. (2014). Ovarian cancer. Lancet 384, 1376–1388. doi: 10.1016/s0140-6736(13)62146-7
Jia, G., Fu, Y., Zhao, X., Dai, Q., Zheng, G., Yang, Y., et al. (2011). N6-methyladenosine in nuclear RNA is a major substrate of the obesity-associated FTO. Nat. Chem. Biol. 7, 885–887. doi: 10.1038/nchembio.687
Jin, D., Guo, J., Wu, Y., Du, J., Yang, L., Wang, X., et al. (2019). m(6)A mRNA methylation initiated by METTL3 directly promotes YAP translation and increases YAP activity by regulating the MALAT1-miR-1914-3p-YAP axis to induce NSCLC drug resistance and metastasis. J. Hematol. Oncol. 12:135. doi: 10.1186/s13045-019-0830-6
Lan, T., Li, H., Zhang, D., Xu, L., Liu, H., Hao, X., et al. (2019). KIAA1429 contributes to liver cancer progression through N6-methyladenosine-dependent post-transcriptional modification of GATA3. Mol. Cancer 18:186. doi: 10.1186/s12943-019-1106-z
Leek, J. T., and Storey, J. D. (2008). A general framework for multiple testing dependence. Proc. Natl. Acad. Sci. U. S. A. 105, 18718–18723. doi: 10.1073/pnas.0808709105
Liu, J., Eckert, M. A., Harada, B. T., Liu, S. M., Lu, Z., Yu, K., et al. (2018). m(6)A mRNA methylation regulates AKT activity to promote the proliferation and tumorigenicity of endometrial cancer. Nat. Cell Biol. 20, 1074–1083. doi: 10.1038/s41556-018-0174-4
Liu, J., Yue, Y., Han, D., Wang, X., Fu, Y., Zhang, L., et al. (2014). A METTL3-METTL14 complex mediates mammalian nuclear RNA N6-adenosine methylation. Nat. Chem. Biol. 10, 93–95. doi: 10.1038/nchembio.1432
Liu, T., Wei, Q., Jin, J., Luo, Q., Liu, Y., Yang, Y., et al. (2020). The m6A reader YTHDF1 promotes ovarian cancer progression via augmenting EIF3C translation. Nucleic Acids Res. 48, 3816–3831. doi: 10.1093/nar/gkaa048
Mayakonda, A., Lin, D. C., Assenov, Y., Plass, C., and Koeffler, H. P. (2018). Maftools: efficient and comprehensive analysis of somatic variants in cancer. Genome Res. 28, 1747–1756. doi: 10.1101/gr.239244.118.
Meyer, K. D., and Jaffrey, S. R. (2017). Rethinking m(6)A Readers. Writers, and Erasers. Annu. Rev. Cell Dev. Biol. 33, 319–342. doi: 10.1146/annurev-cellbio-100616-060758
Narod, S. (2016). Can advanced-stage ovarian cancer be cured? Nat. Rev. Clin. Oncol. 13, 255–261. doi: 10.1038/nrclinonc.2015.224
Patil, D. P., Pickering, B. F., and Jaffrey, S. R. (2018). Reading m(6)A in the transcriptome: m(6)A-Binding proteins. Trends Cell Biol. 28, 113–127. doi: 10.1016/j.tcb.2017.10.001
Qian, J. Y., Gao, J., Sun, X., Cao, M. D., Shi, L., Xia, T. S., et al. (2019). KIAA1429 acts as an oncogenic factor in breast cancer by regulating CDK1 in an N6-methyladenosine-independent manner. Oncogene 38, 6123–6141. doi: 10.1038/s41388-019-0861-z
Reid, B. M., Permuth, J. B., and Sellers, T. A. (2017). Epidemiology of ovarian cancer: a review. Cancer Biol. Med. 14, 9–32. doi: 10.20892/j.issn.2095-3941.2016.0084
Ritchie, M. E., Phipson, B., Wu, D., Hu, Y., Law, C. W., Shi, W., et al. (2015). Limma powers differential expression analyses for RNA-sequencing and microarray studies. Nucleic Acids Res. 43:e47. doi: 10.1093/nar/gkv007
Roundtree, I. A., Evans, M. E., Pan, T., and He, C. (2017). Dynamic RNA modifications in gene expression regulation. Cell 169, 1187–1200. doi: 10.1016/j.cell.2017.05.045
Schöller, E., Weichmann, F., Treiber, T., Ringle, S., Treiber, N., Flatley, A., et al. (2018). Interactions, localization, and phosphorylation of the m(6)A generating METTL3-METTL14-WTAP complex. Rna 24, 499–512. doi: 10.1261/rna.064063.117
Schwartz, S., Mumbach, M. R., Jovanovic, M., Wang, T., Maciag, K., Bushkin, G. G., et al. (2014). Perturbation of m6A writers reveals two distinct classes of mRNA methylation at internal and 5′ sites. Cell Rep. 8, 284–296. doi: 10.1016/j.celrep.2014.05.048
Scrucca, L., Santucci, A., and Aversa, F. (2007). Competting risk analysis using R: an easy guide for clinicians. Bone Marrow Transplant. 40, 381–387. doi: 10.1038/sj.bmt.1705727
Su, R., Dong, L., Li, C., Nachtergaele, S., Wunderlich, M., Qing, Y., et al. (2018). R-2HG exhibits anti-tumor activity by targeting FTO/m(6)A/MYC/CEBPA Signaling. Cell 172, 90.e23–105.e23. doi: 10.1016/j.cell.2017.11.031
Sun, T., Wu, R., and Ming, L. (2019). The role of m6A RNA methylation in cancer. Biomed. Pharmacother. 112:108613. doi: 10.1016/j.biopha.2019.108613
Vu, L. P., Pickering, B. F., Cheng, Y., Zaccara, S., Nguyen, D., Minuesa, G., et al. (2017). The N(6)-methyladenosine (m(6)A)-forming enzyme METTL3 controls myeloid differentiation of normal hematopoietic and leukemia cells. Nat. Med. 23, 1369–1376. doi: 10.1038/nm.4416
Wang, M., Liu, J., Zhao, Y., He, R., Xu, X., Guo, X., et al. (2020). Upregulation of METTL14 mediates the elevation of PERP mRNA N(6) adenosine methylation promoting the growth and metastasis of pancreatic cancer. Mol. Cancer 19:130. doi: 10.1186/s12943-020-01249-8
Wang, X., Lu, Z., Gomez, A., Hon, G. C., Yue, Y., Han, D., et al. (2014). N6-methyladenosine-dependent regulation of messenger RNA stability. Nature 505, 117–120. doi: 10.1038/nature12730
Wang, X., Zhao, B. S., Roundtree, I. A., Lu, Z., Han, D., Ma, H., et al. (2015). N(6)-methyladenosine Modulates Messenger RNA Translation Efficiency. Cell 161, 1388–1399. doi: 10.1016/j.cell.2015.05.014
Wen, J., Lv, R., Ma, H., Shen, H., He, C., Wang, J., et al. (2018). zc3h13 regulates nuclear RNA m(6)A methylation and mouse embryonic stem cell self-renewal. Mol. Cell 69, 1028.e6–1038.e6. doi: 10.1016/j.molcel.2018.02.015
Wilkerson, M. D., and Hayes, D. N. (2010). ConsensusClusterPlus: a class discovery tool with confidence assessments and item tracking. Bioinfomatics 26, 1572–1573. doi: 10.1093/bioinformatics/btq170
Wojtas, M. N., Pandey, R. R., Mendel, M., Homolka, D., Sachidanandam, R., and Pillai, R. S. (2017). Regulation of m(6)A Transcripts by the 3′→5′ RNA Helicase YTHDC2 is essential for a successful meiotic program in the mammalian germline. Mol. Cell 68, 374.e12–387.e12. doi: 10.1016/j.molcel.2017.09.021
Yang, Y., Wei, Q., Tang, Y., Yuanyuan, W., Luo, Q., Zhao, H., et al. (2020). Loss of hnRNPA2B1 inhibits malignant capability and promotes apoptosis via down-regulating Lin28B expression in ovarian cancer. Cancer Lett. 475, 43–52. doi: 10.1016/j.canlet.2020.01.029
Yoon, K. J., Ringeling, F. R., Vissers, C., Jacob, F., Pokrass, M., Jimenez-Cyrus, D., et al. (2017). Temporal control of mammalian cortical neurogenesis by m(6)A methylation. Cell 171, 877.e17–889.e17. doi: 10.1016/j.cell.2017.09.003
Yu, G., Wang, L. G., Han, Y., and He, Q. Y. (2012). clusterProfiler: an R package for comparing biological themes among gene clusters. Omics 16, 284–287. doi: 10.1089/omi.2011.0118.
Yu, H. L., Ma, X. D., Tong, J. F., Li, J. Q., Guan, X. J., and Yang, J. H. (2019). WTAP is a prognostic marker of high-grade serous ovarian cancer and regulates the progression of ovarian cancer cells. Onco Targets Ther. 12, 6191–6201. doi: 10.2147/ott.S205730
Zhao, B. S., Roundtree, I. A., and He, C. (2018). Publisher correction: post-transcriptional gene regulation by mRNA modifications. Nat. Rev. Mol. Cell Biol. 19:808. doi: 10.1038/s41580-018-0075-1
Keywords: ovarian cancer, m6A, TCGA, TME, prognosis, LASSO Cox regression, consensus clustering
Citation: Wei Q, Yang D, Liu X, Zhao H, Yang Y, Xu J, Liu T and Yi P (2021) Exploration of the Role of m6 A RNA Methylation Regulators in Malignant Progression and Clinical Prognosis of Ovarian Cancer. Front. Genet. 12:650554. doi: 10.3389/fgene.2021.650554
Received: 07 January 2021; Accepted: 06 April 2021;
Published: 03 June 2021.
Edited by:
Tianbao Li, Geneis (Beijing) Co., Ltd., ChinaReviewed by:
Zhang Shaowu, Northwestern Polytechnical University, ChinaDongqiang Zeng, Southern Medical University, China
Copyright © 2021 Wei, Yang, Liu, Zhao, Yang, Xu, Liu and Yi. This is an open-access article distributed under the terms of the Creative Commons Attribution License (CC BY). The use, distribution or reproduction in other forums is permitted, provided the original author(s) and the copyright owner(s) are credited and that the original publication in this journal is cited, in accordance with accepted academic practice. No use, distribution or reproduction is permitted which does not comply with these terms.
*Correspondence: Tao Liu, anti1988@163.com; Ping Yi, yiping@cqmu.edu.cn
†These authors have contributed equally to this work