- 1Biological Resources and Post-Harvest Division, Japan International Research Center for Agricultural Sciences, Tsukuba, Japan
- 2Crop Improvement Section, ICAR-Indian Institute of Soybean Research, Indore, India
Seed size and shape traits are important determinants of seed yield and appearance quality in soybean [Glycine max (L.) Merr.]. Understanding the genetic architecture of these traits is important to enable their genetic improvement through efficient and targeted selection in soybean breeding, and for the identification of underlying causal genes. To map seed size and shape traits in soybean, a recombinant inbred line (RIL) population developed from K099 (small seed size) × Fendou 16 (large seed size), was phenotyped in three growing seasons. A genetic map of the RIL population was developed using 1,485 genotyping by random amplicon sequencing-direct (GRAS-Di) and 177 SSR markers. Quantitative trait locus (QTL) mapping was conducted by inclusive composite interval mapping. As a result, 53 significant QTLs for seed size traits and 27 significant QTLs for seed shape traits were identified. Six of these QTLs (qSW8.1, qSW16.1, qSLW2.1, qSLT2.1, qSWT1.2, and qSWT4.3) were identified with LOD scores of 3.80–14.0 and R2 of 2.36%–39.49% in at least two growing seasons. Among the above significant QTLs, 24 QTLs were grouped into 11 QTL clusters, such as, three major QTLs (qSL2.3, qSLW2.1, and qSLT2.1) were clustered into a major QTL on Chr.02, named as qSS2. The effect of qSS2 was validated in a pair of near isogenic lines, and its candidate genes (Glyma.02G269400, Glyma.02G272100, Glyma.02G274900, Glyma.02G277200, and Glyma.02G277600) were mined. The results of this study will assist in the breeding programs aiming at improvement of seed size and shape traits in soybean.
Introduction
Soybean [Glycine max (L.) Merr.] is one of the world’s most economically important food and oil crops. It is a rich source of edible oil and protein, and provides substrate for several food and industrially important products (Lam et al., 2010; Kumawat et al., 2016). Increasing yield and appearance quality of soybean seed are primary breeding objectives. Seed yield per unit area is the product of the number of seeds per unit area and seed weight. Seed size is highly correlated with seed weight, and thus a primary breeding target to improve seed yield (Xu et al., 2011; Li et al., 2019). Seed size traits such as length (SL), width (SW), thickness (ST), and single seed weight (SSW), and seed shape traits such as length-to-width (SLW), length-to-thickness (SLT) and width-to-thickness (SWT) ratios, are important determinants of soybean seed appearance quality and yield (Xu et al., 2011; Hina et al., 2020).
Seed size and shape traits in soybean are not only major components of seed yield, but also important morphological traits in determining commercial value of soybean seed in international trade (Wilson, 1995; Liang et al., 2005; Cui and Xuan, 2007). Different types of soy-based food products also require a specific type of soybean seed shape and size (Cui et al., 2004). For example, large-seeded soybean varieties are used for the preparation of green soybean (edamame), boiled soybean (nimame), soymilk, soy nuts, and soybean curd (tofu), while small seeded ones are used for natto and soy sprouts (Liang et al., 2016; Teng et al., 2017; Wu et al., 2018).
Soybean seed size and shape traits are quantitatively inherited and influenced by genetic and environmental factors (Hu et al., 2013; Wang et al., 2015; Cui et al., 2020), making it difficult and time-consuming to improve these traits using traditional breeding methods (Hao et al., 2012). The genetic control of quantitatively inherited traits, such as seed size and shape traits, can be dissected and genomic positions of the controlling loci can be identified using molecular markers (Tanksley, 1993; Sun et al., 2012). These quantitative trait loci (QTLs) can be used in soybean breeding for introgression and selection of favorable alleles of various seed size and shape traits to facilitate the development of new soybean varieties with seed size and appearance tailored for each distinct endeavor (Allen, 1994; Cao et al., 2017; Cui et al., 2020).
In soybean, QTLs for seed size and shape traits have been identified using simple sequence repeats (SSRs) and single nucleotide polymorphisms (SNPs) markers (Hoeck et al., 2003; Salas et al., 2006; Xu et al., 2011; Hu et al., 2013; Niu et al., 2013; Kato et al., 2014; Liang et al., 2016; Fang et al., 2017; Yang et al., 2017; Fujii et al., 2018; Li et al., 2019, 2020; Cui et al., 2020; Hina et al., 2020). Many QTLs have been identified for seed size and shape traits in soybean, but our understanding of their genetic architecture for practical application in soybean breeding is still limited because most of the QTLs are population and environment specific (Hina et al., 2020). Although association mapping uses diverse natural populations, yet the identified loci must be characterized and validated for their allelic effect and environmental stability in the potential genetic sources used in the breeding program. This study further explored the genetic architecture of seed size and shape traits in soybean using a recombinant inbred line (RIL) population and identified six stable QTLs for seed size and shape traits with large effect phenotypic contribution across multiple years. A major genomic locus harboring three major QTLs was validated in residual heterozygous line (RHL)-derived near isogenic lines (NILs).
Materials and Methods
Plant Material and Phenotypic Evaluation
A RIL population of 94 RILs was used to map QTLs for seed size and shape. The RIL population was derived from a cross between “K099” cultivar having small and round seed shape and “Fendou 16” cultivar having large and oblong seed shape. K099 is a Korean cultivar and Fendou 16 (PI574476A) is a cultivar from Shanxi, China. K099 was provided by the National BioResource Project (Lotus japonicus and G. max)1, and Fendou 16 was provided by the US National Plant Germplasm System (NPGS)2. The RIL population (F6) was developed from the F2 generation by the single-seed descent method without selection during the generation advancement.
The RIL population was grown in 2012, 2016, and 2017 at the farm of Japan International Research Center for Agricultural Sciences, Tsukuba, Ibaraki, Japan. Each RIL was grown in a 3-m single row plot spaced 60 cm between rows and 20 cm between plants. Seeds were harvested as plot bulk and a total of 20 seeds randomly selected from each RIL, were used for the trait measurement from three growing environments. The RIL population was phenotyped for four seed size traits namely SSW (g), SL, SW, and ST (mm) using a digital Vernier caliper, as described in Niu et al. (2013). Three seed shape traits namely SLW, SLT, and SWT, were calculated from the seed size traits for each RIL (Niu et al., 2013).
DNA Marker Analysis and Linkage Map Construction
Amplicon sequence-based genotyping was performed using genotyping by random amplicon sequencing-direct (GRAS-Di) technology at Eurofins Genomics, Tokyo (Enoki and Takeuchi, 2018). Libraries were constructed for GRAS-Di, according to the protocol described in Hosoya et al. (2019). Sequencing of the libraries was conducted using the Illumina HiSeq4000. Marker identification was performed using GRAS-Di software version 1.0.5 (Toyota, Aichi, Japan). The software evaluates marker quality according to the empirical criteria of genotyping reproducibility identified based on the number of reads and reproducibility of genotyping (presence and absence of reads) over samples (Patent ID P2018-42548A). Genotype of a RIL sample was determined based on the number of reads, and presence or absence of respective read compared to the reference sample (Fendou 16). The identified GRAS-Di markers were tested for 1:1 segregation ratio using the Chi-square test. Markers (reads) identified in this way were mapped to soybean genome Wm82.a2.v1, using BWA-MEM version 0.7.12 (Li, 2013).
Other than GRAS-Di markers, classical SSR markers were also genotyped in the RIL population. Publically available soybean SSRs on SoyBase3 were amplified (∼600) for polymorphism analysis between the parents of RILs as per methods given in Liu et al. (2018). PCR amplification was performed in a final volume of 20 μl with 10 ng of template DNA, 10 pmol forward and reverse primers, and 10 μl Quick TaqTM HS DyeMix (Toyobo, Tokyo, Japan). The PCR reaction was performed over 35 cycles of 30 s at 94°C, 30 s at 56°C, and 30 s at 72°C followed by an extension of 5 min at 72°C. Amplified products were separated on 8.0% polyacrylamide gel and stained with ethidium bromide. The banding pattern was documented using a Pharos FX Molecular Imager (Bio-Rad, Tokyo, Japan). A total of 229 polymorphic SSR markers were genotyped in the RILs.
Redundant markers with high collinearity were filtered out using the bin functionality of QTL IciMapping software (Meng et al., 2015). Non-redundant SSR and GRAS-Di markers were used for linkage map construction using MapDisto version 2.0 software (Heffelfinger et al., 2017). A logarithm of odds (LOD) score of 6.0 and maximum recombination fraction of 0.2 estimated using the classical method was used to group of markers. Kosambi mapping function and the seriation II method were used for ordering markers.
Data Analysis and QTL Mapping
Phenotypic data were analyzed in Excel 2016 software (Microsoft, Redmond, WA, United States). Correlations among traits were assessed using Pearson’s correlation coefficient and visualized using the “corrplot” package in R (Wei and Simko, 2017). Trait values between genotype pairs were compared for significance by Student’s t-test. The inclusive composite interval mapping (ICIM) method was used to identify seed size and shape QTLs, using the QTL IciMapping software (Meng et al., 2015). The significance for the declaration of QTL was estimated from 1,000 permutation tests. A LOD score corresponding to an experiment-wise threshold of P = 0.05 was used to declare a QTL as significant. Composite interval mapping in Windows QTL Cartographer v2.5 (Wang et al., 2012) was used to confirm the QTL results. Confidence interval of QTLs were determined using a 1-LOD support interval as a 95% confidence interval (Lander and Botstein, 1989). Neighboring QTLs of different seed size and shape related traits with overlapping confidence intervals were grouped into QTL clusters. The map positions of QTLs in the QTL clusters were depicted on the linkage map using MapChart software (Voorrips, 2002).
Development of NILs and qSS2 Validation
Two NILs, NILs-F, and NILs-K, were selected from the progenies of a self-pollinated F6 RIL, RIL98, which was heterozygous at QTL cluster qSS2. Homozygous plants with the Fendou 16 and K099 genotypes at qSS2 were selected from the RIL98 progenies based on SSR markers BARCSOYSSR_02_1667 and Sat_415. The genomic background difference between NIL pair was analyzed using 122 SSR markers selected from 20 soybean chromosomes. These contrasting NILs, NILs-F, and NILs-K, were used to confirm the effect of qSS2. The NILs were grown in 2018 and 2019 under similar agronomic conditions as the RIL population. Each NIL was phenotyped for seven seed size and shape traits (SL, SW, ST, SSW, SLW, SLT, and SWT) in three replicates of 20 seeds each.
Candidate Gene Mining in the QTL Cluster qSS2
Predicted genes in the physical genomic interval of qSS2 and their annotations information were downloaded from SoyBase (see text footnote 3). A freely available RNA-Seq data set on SoyBase, which includes seven seed development stages (seed_10DAF, seed_14DAF, seed_21DAF, seed_25DAF, seed_28DAF, seed_35DAF, and seed_42DAF, where DAF stands for days after flowering), was used to download expression data for the predicted candidate genes to analyze the expression of these genes among different tissue samples and developmental stages (Severin et al., 2010). A heatmap was constructed by using TBtools software to visualize the relative expression patterns of the predicted candidate genes in different tissue samples (Chen et al., 2020). Candidate genes were mined based on the gene annotations, expression data, and available literature.
Results
Phenotypic Variation for Seed Size and Shape Traits
The two parental genotypes of the RIL population, K099 and Fendou 16, significantly differ for all seed size and seed shape traits (P < 0.01, Supplementary Table 1). Fendou 16 seeds are larger with trait values 21.5% (SSW), 29.3% (SL), 30.7% (SLW), 36.7% (SLT), and 8.7% (SWT) higher compared to K099, which has an ST value 11.3% higher than Fendou 16. The absolute values of kurtosis and skewness were <1 for most traits in all years, except for SLT and SWT in 2016 and ST in 2017, indicating typical quantitative inheritance of seed size and shape traits in RILs (Supplementary Table 2). Higher and lower values than means of the parental genotypes was observed for most of the evaluated traits indicating transgressive segregation (Figure 1). Correlation analysis identified significant positive correlations between SL and all other seed size and shape traits, except ST and SWT in 2017 and 2016, respectively (Figure 2). SSW was positively correlated with all seed size and shape traits in 2017 and combined environments data, but was not significant for three seed shape traits in 2012 and 2016. Significant negative correlations were observed between SW and SLW, and for ST with respect to SLW, SLT and SWT (Figure 2).
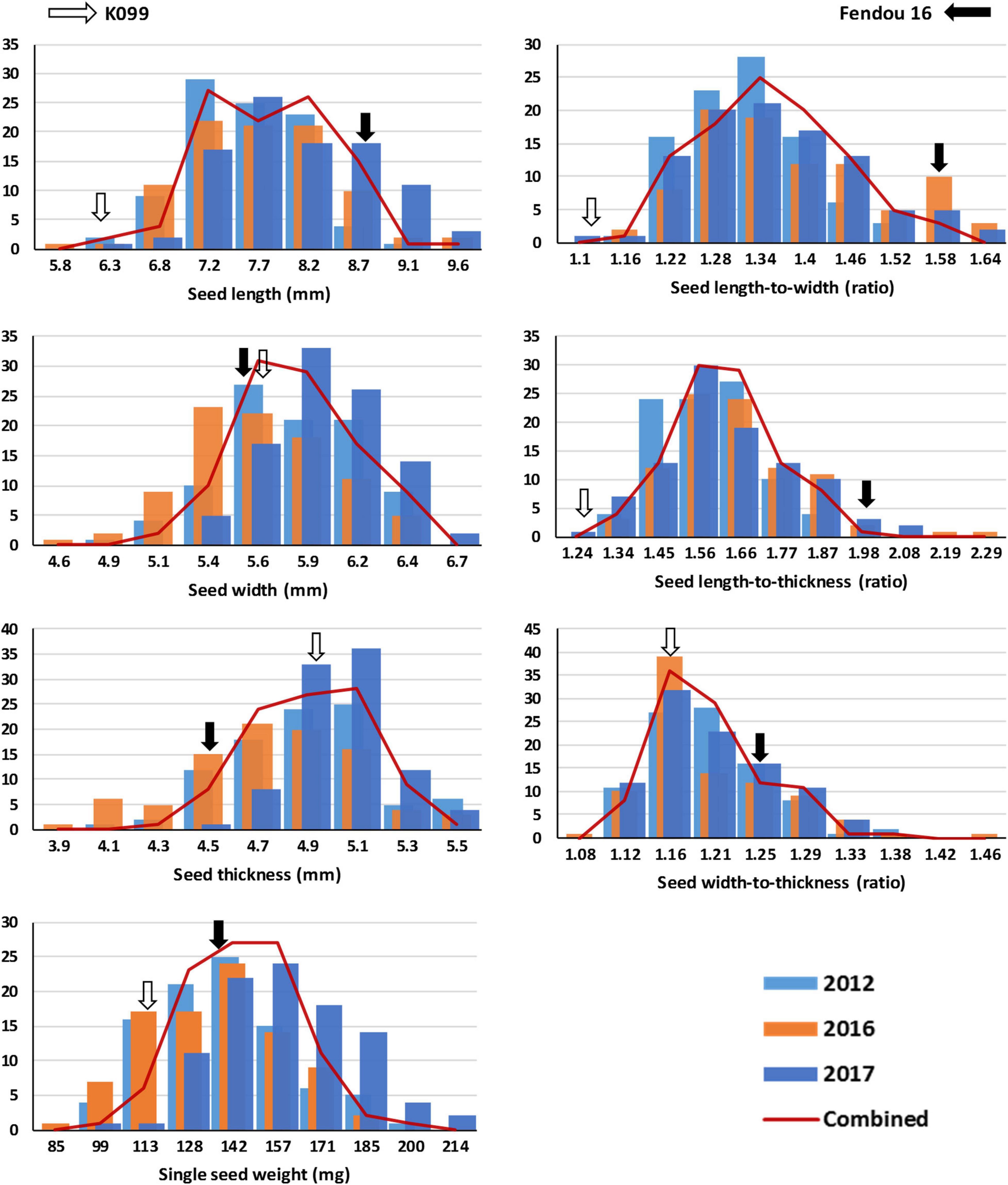
Figure 1. Frequency distribution of seed length (SL), seed width (SW), seed thickness (ST), single seed weight (SSW), and ratios of seed length-to-width (SLW), seed length-to-thickness (SLT), and seed width-to-thickness (SWT) in K099 × Fendou 16 recombinant inbred line (RIL) population (n = 94) grown across multiple years (2012, 2016, and 2017). Trend lines show the average of 3-year environments data. Arrows represent the mean value of the corresponding parent genotype. The horizontal and vertical axes represent the trait value and number of RILs, respectively.
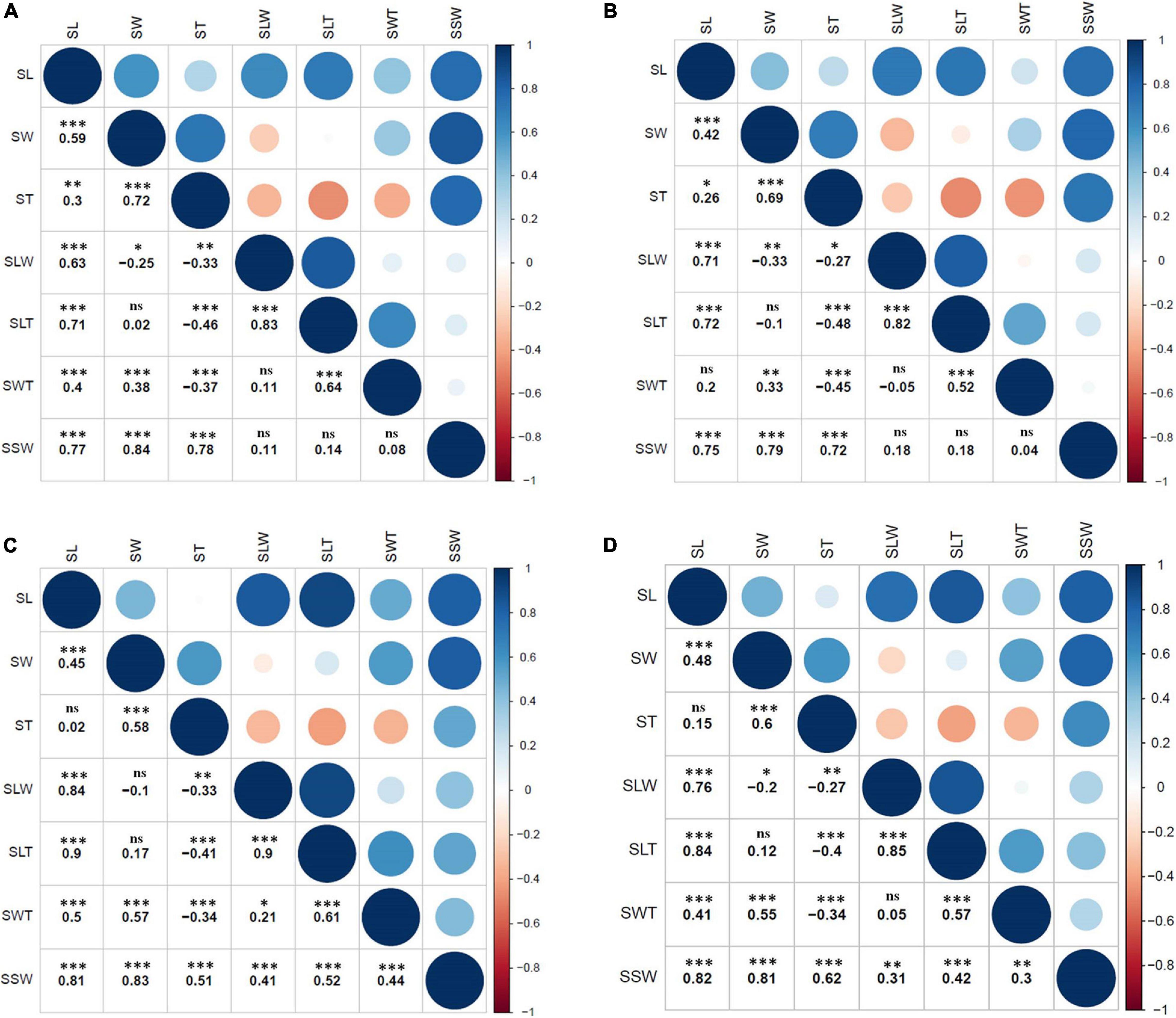
Figure 2. Pearson’s correlation analysis of seven seed size and shape traits among RILs (n = 94) within or across environments; (A) 2012, (B) 2016, (C) 2017, and (D) combined (mean values of 3 years). ∗, ∗∗, and ∗∗∗ indicate significant correlations at the 5%, 1%, and 0.1% level, respectively; ns indicates no significant correlation at the 5% level.
Marker Identification and Linkage Map Construction in 94 RILs
Genotyping by random amplicon sequencing, direct of 94 RILs and two parents yielded an average of 6,050,345 paired-end reads for each line generating 611 Mb sequence data per line. After filtering markers with low quality and segregation distortion (1:1, P > 0.01), 4,366 high quality polymorphic markers (reads) were obtained. These 4,366 GRAS-Di markers and 229 SSR markers were used for binning to remove redundant markers with high collinearity, resulting in 2,010 unique markers. These 2,010 unique markers were used for linkage map construction. Of these, 1,662 markers (1,485 GRAS-Di and 177 SSRs) were correctly assigned to 20 soybean chromosomes (Supplementary Table 3). Chromosome 12 had the fewest markers (n = 50), and Chromosome 9 had the most (n = 119). Most markers showed collinearity with the physical map, with exception of a few segments on Chr.01, Chr.09, Chr.18, and Chr.20 (Supplementary Table 3). The total map length spans 4,464 cM with an average of 2.68 cM distance per marker (Supplementary Table 4). The longest linkage group was for Chr.13 with a 304.2 cM genetic map distance, and the shortest was for Chr.14 with a 154.2 cM map distance.
QTLs for Seed Size Traits
Inclusive composite interval mapping identified 53 QTLs, distributed on 17 chromosomes, for four seed size traits in RILs (Table 1). Of these, 19 QTLs were identified for SL, 15 for SW, 7 for ST, and 12 for SSW. The 19 QTLs identified for SL explained 4.39–20.86% of phenotypic variance (PV), 10 of which were major QTLs accounting for >10% phenotypic variation (Table 1). Two major QTLs for SL, qSL2.2 and qSL2.3, were identified in the nearby genomic regions for the growing environments of 2017 and 2016, respectively, while qSL2.3 was also detected in the combined environments data. The qSL2.3 was detected with a LOD score of 14.1 between BARCSOYSSR_02_1686 and BARCSOYSSR_02_1697 for the combined environments data and explained 19.85% of phenotypic variance (PV) for SL in the RIL population. The additive allele of Fendou 16 at qSL2.3 increased SL by 0.26 mm in the combined environments data. One QTL, qSL10.1, was identified in 2016, as well as for the combined environments data, explaining 20.86% and 14.06% of PV, respectively. Two QTLs, qSL11.1, and qSL11.2, identified for combined environments data and 2012, respectively, are present in close proximity and seem to be the same QTL. Similarly, qSL13.2 and qSL13.3 were also detected in close proximity for combined environments data and 2017, respectively.
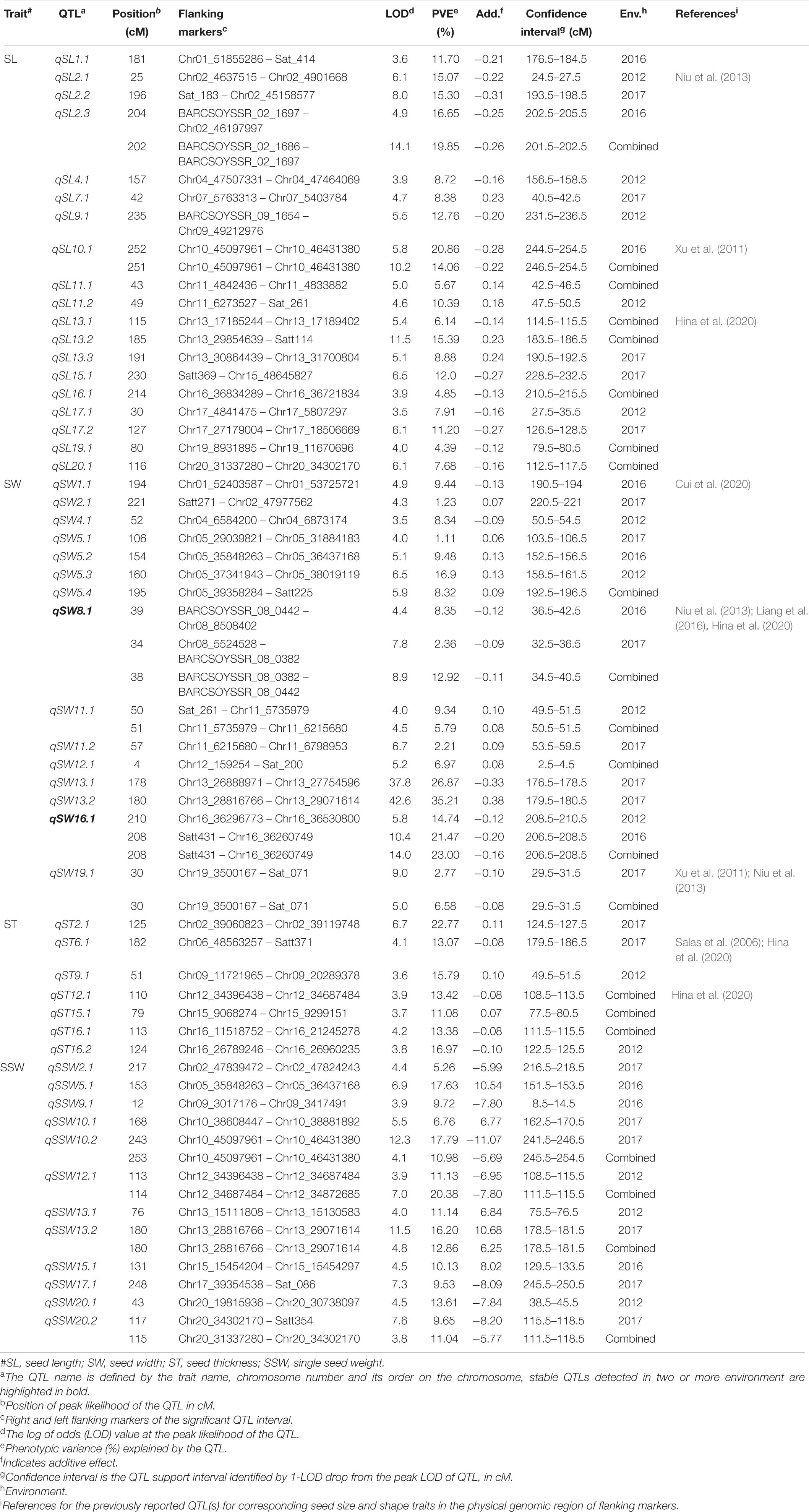
Table 1. QTLs identified for seed-size traits in K099 × Fendou 16 RIL population across multiple years.
Fifteen QTLs were identified for the SW trait explaining 1.11% to 35.21% of PV across three environments (Table 1). Among them, two QTLs, qSW8.1, and qSW16.1, were stably expressed. Stable QTL, qSW8.1 was identified in 2016 and 2017, as well as for combined environments data. Positive allele for SW at this locus was contributed by Fendou 16. Stable QTL qSW16.1 was detected between 206.5 cM to 2010.5 cM, for 2012, 2016 and the combined environments data. PV explained by this stable QTL ranged from 14.74% to 23%, and positive allele for SW at this QTL was also contributed by Fendou 16. Interestingly two SW QTLs, qSW13.1, and qSW13.2, were detected in nearby genomic regions with large PV explained (26.87 and 35.21%, respectively) in 2017, but the QTL effect was found to be in the opposite direction (Table 1). The positive allele at qSW13.1 was contributed by Fendou 16, whereas qSW13.2, is from K099.
Of the seven QTLs identified for ST, three (qST12.1, qST15.1, and qST16.1) were identified for combined environments data (Table 1). The major QTL qST2.1 was detected in 2017, explaining 22.77% of PV. K099 contributed favorable allele at this major. QTLs qST16.1 and qST16.2 were in close proximity explaining 13.38% and 16.97% of PV for combined environments data and 2012, respectively. The positive alleles at both of these ST QTLs were contributed by Fendou 16.
Twelve QTLs were identified for SSW, explaining 5.26% to 20.38% of PV. Among them, qSSW10.2, qSSW12.1, qSSW13.2, and qSSW20.2 were identified in one of the environments and combined environments data. Highest PV of 20.38%, was explained by qSSW12.1 for combined environments data. Fendou 16 contributed positive alleles at seven SSW QTLs and K099 contributed positive alleles at five QTLs (Table 1).
Composite interval mapping of seed size traits identified 54 QTLs, 36 of which were shared with the QTLs identified by ICIM (Supplementary Tables 5, 6). Stable QTLs qSW8.1 and qSW16.1 were also detected at the same genomic position in CIM.
QTLs for Seed Shape Traits
A total of 27 QTLs were identified for three seed shape traits which were distributed on 12 chromosomes (Table 2). Of these, four QTLs were identified for SLW, 13 for SLT and 10 for SWT. The four QTLs identified for SLW explained 6.91 to 39.49% of PV across various environments. One major and stable QTL qSLW2.1, was identified consistently at the same genomic region between map position 199.5 cM to 205.5 cM on Chr.02, in all of the 3 years growing environments as well as for combined environments data. qSLW2.1 explained 29.04 to 37% of PV in the RILs across 3 years’ growing environments, and 39.49% of PV for the combined environments data. Positive allele at qSLW2.1 was contributed by Fendou 16. Among three environment-specific QTLs, qSLW8.1 and qSLW9.1 were detected in the growing environment of 2012, and qSLW10.1 was identified in 2016.
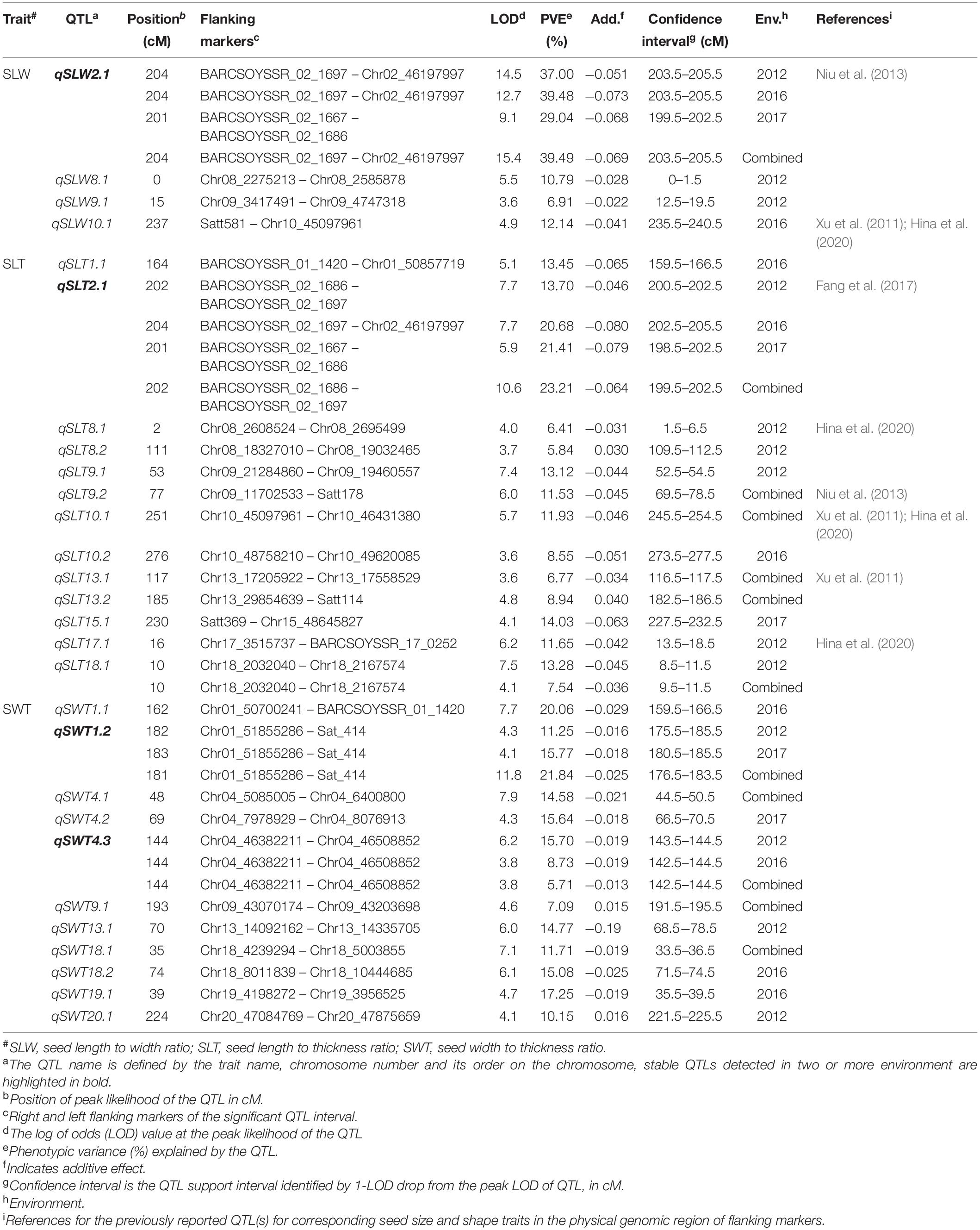
Table 2. QTLs identified for seed-shape traits in K099 × Fendou 16 RIL population across multiple years.
Thirteen QTLs were detected for SLT explaining 5.84 to 23.21% of PV (Table 2). A major and stable QTL qSLT2.1, was identified at a similar genomic region between map position 199.5 cM and 205.5 cM on Chr.02, in all 3 years’ growing environments and combined environments data. qSLT2.1 explained 13.70 to 21.41% of the PV across the 3 years’ growing environments, and 23.21% of PV for the combined environments data. Positive allele at qSLT2.1 was contributed by Fendou 16. qSLT2.1 was identified in the same genomic region as qSL2.3 and qSLW2.1, indicating pleiotropic control of these traits by this locus. qSLT18.1 was detected in 2012 and for the combined environments data, explaining 13.28 and 7.54% of PV, respectively. Fendou 16 contributed favorable alleles at all SLT QTLs, except at qSLT8.2 and qSLT13.2.
Ten QTLs were detected for SWT explaining 5.71 to 21.84% of PV (Table 2). One major and stable QTL qSWT1.2, was detected between markers Chr01_51855286 and Sat_414, in 2012 and 2017, and for the combined environments data. The qSWT1.2 explained 11.25 and 15.77% of PV in RILs for years 2012 and 2017, respectively, and 21.84% of PV for combined environments data. In 2016, a major QTL qSWT1.1 was detected near qSWT1.2 at 159.5–166.5 cM, explaining 20% of PV. Stable QTL qSWT4.3 was identified between markers Chr04_46382211 and Chr04_46508852, in 2012 and 2016, as well as for combined environments data with PV 5.71%–15.70%. Three QTLs, qSWT4.1, qSWT9.1, and qSWT18.1, were identified for combined environments data and the remainder were environment specific. Positive alleles for SWT were contributed by Fendou 16 at all QTLs detected for SWT, except qSWT9.1 and qSWT20.1.
Composite interval mapping of seed shape traits identified 47 QTLs, 20 of which were shared with the QTLs identified by ICIM (Supplementary Tables 5, 6). All four stable QTLs, qSLW2.1, qSLT2.1, qSWT1.2, and qSWT4.3, were detected in CIM. This further confirmed the QTL mapping results.
QTL Clusters for Seed Size and Shape Traits
Most of the seed size and shape traits are positively or negatively correlated with each other perhaps because a QTL for one seed size and shape trait may be present in the same chromosomal region as QTL(s) of other trait(s). These QTLs for different seed size and shape traits may be either different or same locus due to random shifting and are referred to as integrated QTLs or QTL clusters. Of the total 80 QTLs identified for seven seed size and shape traits, 24 were located on 11 QTL clusters, distributed on nine chromosomes (Table 3 and Figure 3). These QTL clusters were designated as “qSS” (seed size and shape locus followed by chromosome number and their order), and were named qSS1a, qSS1b, qSS2, qSS5, qSS9, qSS10, qSS11, qSS12, qSS13a, qSS13b, and qSS16. Two QTL clusters, qSS2 and qSS10, were composed of three QTLs each, while the other nine QTL clusters were composed of two QTLs each (Table 3). QTL cluster, qSS2, located between 198.5 cM and 205.5 cM on Chr.02 harbored two stable QTLs, qSLW2.1, and qSLT2.1, as well as a major SL QTL qSL2.3. QTL cluster qSS10 located between 241.5 cM and 254.5 cM on Chr.10 harbored qSL10.1, qSSW10.2, and qSLT10.1, but none of them had shown consistent expression in the three growing environments.
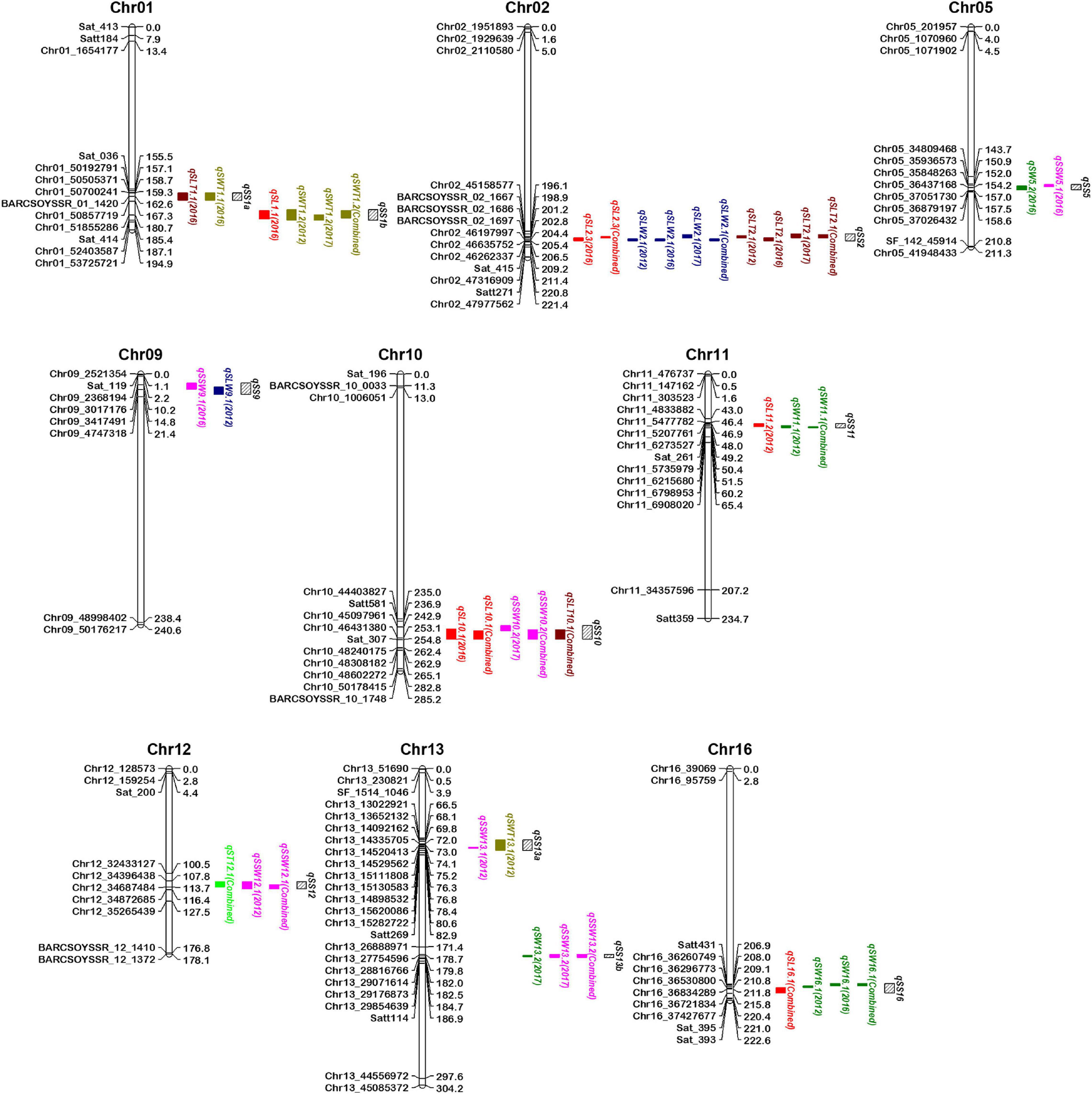
Figure 3. Map positions of quantitative trait loci (QTLs) for seed size and shape traits present in 11 QTL clusters on the genetic linkage map of the K099 × Fendou 16 RIL population. Distances in cM are indicated to the right of the linkage groups and names of markers are shown on the left. Markers of only in and around identified QTLs are shown on chromosomes. Positions of different QTLs are represented by colored bars on the right of chromosomes. QTL names are followed by the environment (year) in parentheses depicting year of QTL expression. QTL cluster positions are depicted by black bars with slanting lines on the right of chromosomes.
Validation of Allelic Effect of Stable Locus qSS2
The effect of QTL cluster qSS2 on seed size and shape traits was validated using RHL-derived NILs, NILs-F and NILs-K. The NILs-F had the Fendou 16 homozygous genotype, and NILs-K had the K099 homozygous genotype at the qSS2. Genomic background difference analysis using 122 SSR markers identified 14 SSR markers as polymorphic, of which three were from the qSS2 locus. Thus, other than the qSS2 locus, the two NILs were differed at 9.01% of the background genome. Of the 11 QTL clusters, the NILs were differing at qSS2 and qSS10. These two contrasting NILs were evaluated for all seven seed size and shape traits for 2 years, 2018 and 2019. NILs-F and NILs-K showed significant differences for SL and SLW in both years (P < 0.01) and for SLT in 2019 (P < 0.001) (Figure 4). They also showed significant differences in SSW, ST and SWT in both years (P < 0.01), and for SW in 2018 (P < 0.01; Supplementary Table 7). Although, qSSW10.2 of QTL cluster qSS10 was not stably detected in QTL analysis, the significant differences observed in SSW of NILs in both the years suggest that qSSW10.2 was also stably expressed.
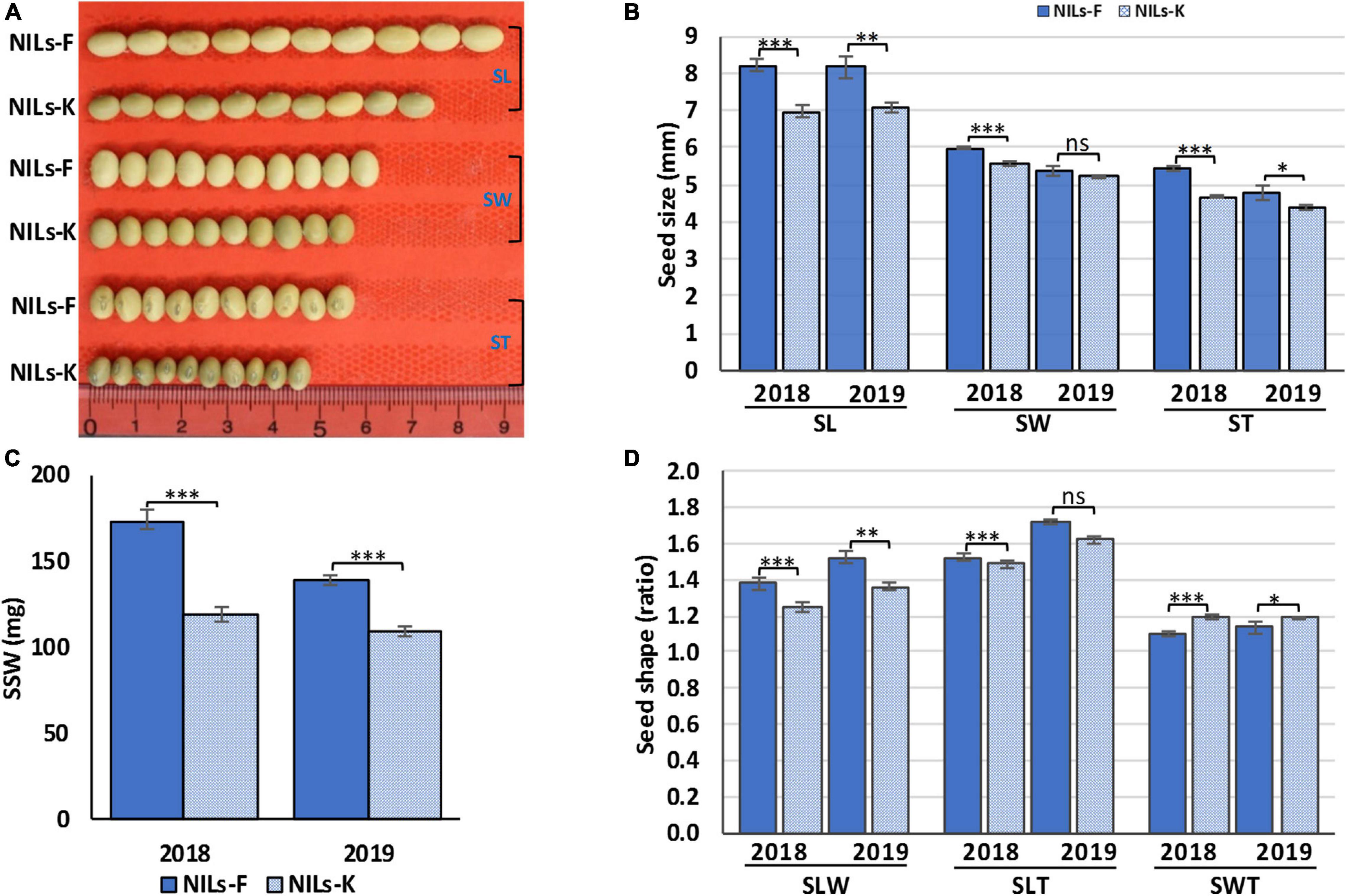
Figure 4. Seed size and shape phenotypes of near isogenic lines, NILs-F and NILs-K in 2018 and 2019, (A) phenotypic appearance, (B) SL, seed length; SW, seed width; ST, seed thickness, (C) single seed weight (SSW), and (D) seed shape traits (SLW, ratios of seed length-to-width; SLT, seed length-to-thickness; and SWT, seed width-to-thickness). Error bars represent means ± SD of three replicates. Asterisks indicate significant differences between NILs-F and NILs-K at 5% (∗), 1% (∗∗), and 0.1% (∗∗∗); ns indicates no significant difference at the 5% level in Student’s t-test.
Mining of Candidate Genes in qSS2
Quantitative trait locus cluster qSS2 is flanked by BARCSOYSSR_02_1667 and Chr02_46197997. These markers are separated by a physical interval of ∼887 Kb. Based on the Glyma 2.0 assembly of Williams 82, 110 protein coding genes are predicted to be present in the genomic sequence of this physical interval (Supplementary Table 8). A total of 22 protein coding genes with gene function annotations for cell proliferation, differentiation, tissue morphogenesis and seed metabolism were selected as candidate genes (Supplementary Table 9). Twenty-one of the 22 predicted candidate genes are represented in the RNA-Seq atlas available in SoyBase. Expression analysis using RNA-Seq data for different soybean tissues showed that five of them have high expression specifically in pods and seed tissues at various developmental stages (Figure 5). Two Glycosyl hydrolase family members, Glyma.02G269400 and Glyma.02G272100, were highly expressed in seed tissues and are associated with carbohydrate metabolism. Glyma.02G274900, a translation initiation factor 2C gene, was annotated with cytokinesis and several tissue differentiation terms. A cyclin-dependent protein serine/threonine kinase regulator activity gene, Glyma.02G277600, was highly expressed in seed tissues. Glyma.02G277200, encoding an ATP synthase regulator, was annotated with several organ morphogenesis terms, and was highly expressed in seed tissue 10 days after flowering. Glyma.02G276000, encoding cyclin-dependent kinase C2, was annotated for the vegetative to reproductive phase transition. Two late embryogenesis abundant protein genes (Glyma.02G274400 and Glyma.02G277300), two pentatricopeptide repeat (PPR) protein genes (Glyma.02G271600 and Glyma.02G276200) and a protein phosphatase 2C gene (Glyma.02G278100) are other potential candidates associated with seed development and morphogenesis, controlling various seed size and shape traits.
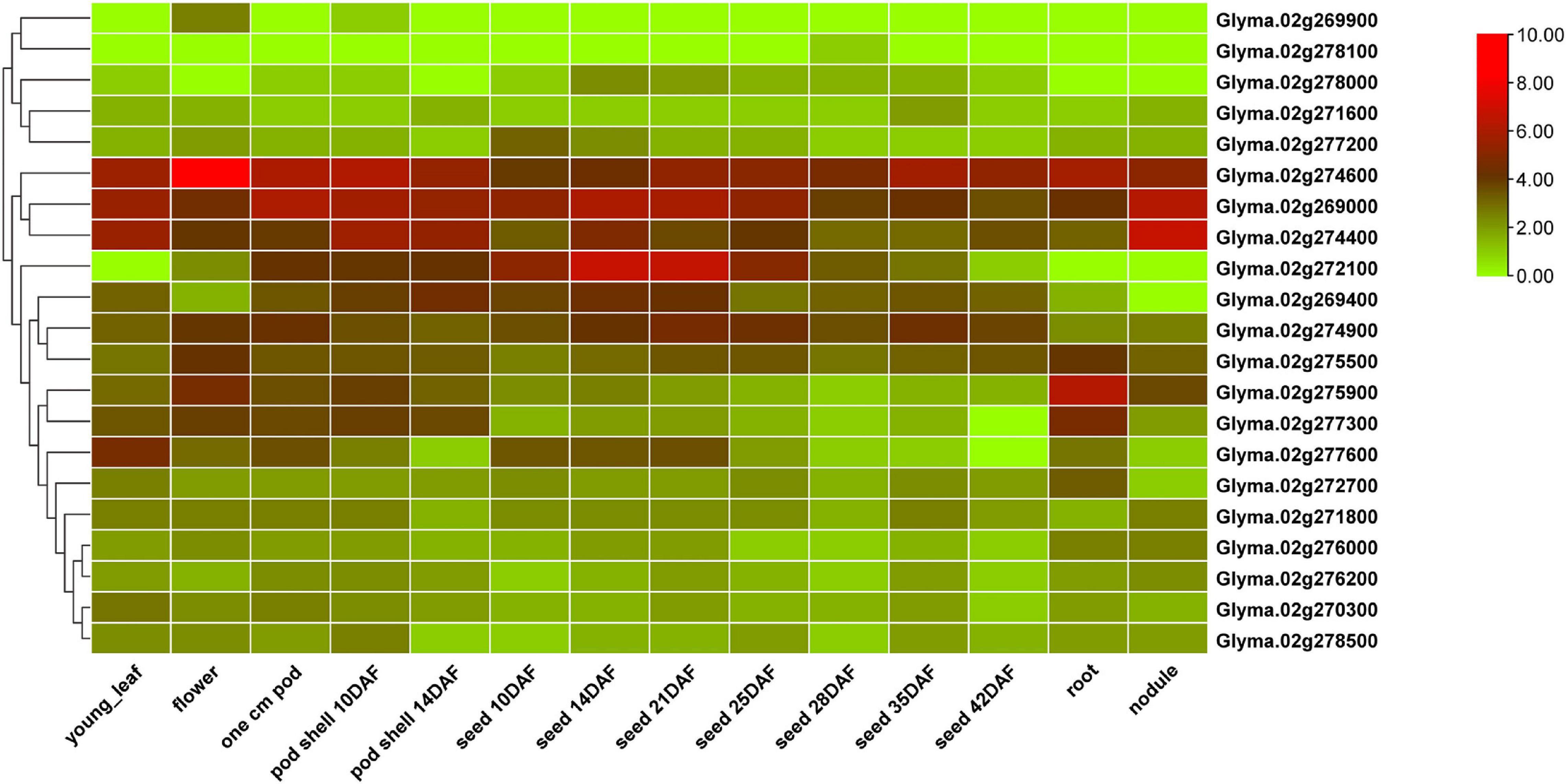
Figure 5. Heatmap visualization of relative gene expression in different tissues and developmental stages, for the candidate genes predicted in the qSS2 physical interval. Normalized FPKM values are depicted on the log2 scale.
Discussion
Seed size is one of the most important traits selected during domestication of soybean. In order to continuously improve seed yield and appearance in soybean, dissection of the genetic architecture of seed size and shape traits is imperative. Identification of genomic loci controlling seed size and shape traits and further characterization of genes underlying these loci can help in molecular designing of seed size and shape traits in soybean. Seed size and shape traits in soybean are highly complex and controlled by many loci as demonstrated in the earlier QTL mapping studies (Xu et al., 2011; Hu et al., 2013; Niu et al., 2013; Hina et al., 2020). Several QTLs for seed size and shape traits have been previously identified in soybean using bi-parental mapping (Hoeck et al., 2003; Salas et al., 2006; Xu et al., 2011; Hu et al., 2013; Che et al., 2014; Kato et al., 2014; Liang et al., 2016; Fang et al., 2017; Yang et al., 2017; Fujii et al., 2018; Cui et al., 2020; Hina et al., 2020; Li et al., 2020). Genome-wide association studies were also attempted to identify QTLs for seed size and shape traits in soybean (Hu et al., 2013; Niu et al., 2013; Fang et al., 2017; Li et al., 2019). Although, many QTLs were identified for seed size and shape traits in soybean, only a few were stable in different genetic backgrounds and environments (Hina et al., 2020). Further, looking at the vast diversity and complexity of these traits, additional characterization of these traits’ genetic underpinning in novel genetic resources is warranted. Fendou 16, the larger seed size parent used in our study, showed significant variation in SSW, SL, SLW, and SLT, by showing 21.5, 29.3, 30.7, and 36.7% higher values than small-seeded parent K099, whereas K099 showed 11.3% higher values of ST. Longer seed length with higher SLW and SLT are characteristics of wild species Glycine soja, while the opposite is the case for cultivated soybean. However, seed size and shape traits have evolved during domestication and it would be interesting to know whether the higher SLW and SLT ratio in Fendou 16 is related to wild soybean alleles or are newly acquired.
Genotyping by random amplicon sequencing-direct is a recently developed method for genotyping by sequencing using random primers for PCR amplification and next-generation sequencing, for identifying many highly reproducible genetic markers (Enoki and Takeuchi, 2018; Hosoya et al., 2019; Miki et al., 2020). Using GRAS-Di and SSR markers, a dense genetic map of K099 × Fendou 16 RIL population was developed. Using ICIM, we identified a large number of additive QTLs for seven seed size and shape traits. QTLs identified in two or more environments with PVE > 10% are considered as stable QTLs. In this study, we identified two stable QTL for seed size traits (qSW8.1 and qSW16.1) and four stable QTLs for seed shape traits (qSWT1.2, qSLW2.1, qSLT2.1, and qSWT4.3), explaining a considerable phenotypic variation in these traits across multiple years. CIM further confirmed these stable QTLs including all QTLs of QTL cluster qSS2. Although, majority of seed size and shape trait QTLs were detected in CIM, the differences observed in the position of other QTLs may be due to small population size used in this study, as well as differences in the method of selecting background markers as cofactors and minimum map distance used to declare a new QTL in CIM compared to ICIM (Meng et al., 2015). The large effect and stable QTL qSLW2.1 was earlier reported for the association with SLW (Niu et al., 2013), and ST and SLT (Fang et al., 2017), in association mapping studies. In the present study, we found that qSLW2.1 also control SL and SLT, as the major QTLs qSL2.3 and qSLT2.1, were co-localized in the same genomic region of qSLW2.1. Thus a major gene in this genomic region affects SL, SLW and SLT, which is also evident from their high positive correlation. This major locus, a QTL cluster for seed size and shape traits in soybean, has been named as qSS2. The NILs contrasting for qSS2 showed significant differences for various seed size and shape traits evaluated for 2 years’ growing environments, confirming qSS2 as a major and stable locus. In the present study, no stable QTL was identified for SSW at qSS2 but a SSW QTL qSSW2.1 was identified near qSS2. The two NILs also differed at qSSW2.1 and qSSW10.2, perhaps explaining significant difference for SSW. The interaction of both these QTLs with qSS2, might be contributing to significantly large effects on seed size and shape traits, therefore further studies are required to dissect the effect of individual and multiple QTL clusters on seed size and shape traits.
Clustering of QTLs for seed size and shape traits has been frequently reported elsewhere. Xu et al. (2011) reported co-localization of several seed size and shape QTLs on Chr.06 and Chr.10, in the direct and reciprocal cross of Lishuizhongzihuang × Nannong 493-1. QTL cluster identified on Chr.06 was fine mapped and eight candidate genes were predicted (Xie et al., 2014). Hina et al. (2020) reported co-localization of several seed size and shape QTLs in four QTL hotspots on Chr.06, Chr.10, Chr.13, and Chr.20, in two RIL populations developed from the crossing of higher seed size genotype Nannong1138-2 (N) with cultivated soybean varieties Zhengxiaodou (Z) and Kefeng35 (K3). Recently, Cui et al. (2020) identified 30 significant QTLs for six seed size and shape traits in a RIL population of BX10 × BD2. Among these QTLs, co-localization of seven QTLs for five traits was found at one locus, named qSS3. The regular reports on co-localization of QTLs for seed size and shape traits are probably due to high correlation among various seed size and shape traits.
An SW QTL, qSW19.1, was identified in the nearby genomic region earlier reported by Xu et al. (2011) and Niu et al. (2013). Two stable QTLs for SWT, qSWT1.2 and qSWT4.3, were not reported earlier and are newly identified. Using high-density mapping in two RIL populations, Hina et al. (2020) identified three stable QTLs for SL on Chr.04, Chr.09, and Chr.13, one for SW on Chr.13 and two for ST on Chr.06 and Chr.13. Among the seed shape traits, Hina et al. (2020) identified three stable QTLs for SLW on Chr.06, Chr.19, and Chr.20, two for SLT on Chr.10 and Chr.20, and two for SWT on Chr.02 and Chr.08, but none of them was present in the overlapping genomic regions of stable QTLs identified in the present study. Notably, only three stable QTLs (qSL-9-1ZY,K3N, qSLW-19-1K3N,ZY, and qSWT-2-1K3N,ZY) were common between two RILs evaluated by Hina et al. (2020). Cui et al. (2020) also identified one stable QTL named qSS3, on Chr.03 explaining 7.3 to 26.2% of PV for five seed size and shape traits, in a RIL population tested in three growing locations. The qSS3 was validated in RHL-derived NILs (Cui et al., 2020). These stable QTLs with significantly large effect phenotypic variance contribution are suitable for practical application in soybean breeding to improve seed size and appearance traits. The Fendou 16 will serve as a vital genetic resource for the introgression of favorable alleles of SL, SLW, and SLT traits in soybean.
The identified stable QTLs will pave the way for positional cloning of genes regulating seed size and shape traits in soybean. Several QTLs and genes controlling seed size traits have been cloned in other crop species, but similar studies are lagging in soybean (Mao et al., 2010; Li et al., 2011; Ma et al., 2015; Xu et al., 2015; Lu et al., 2017; Nguyen et al., 2020). The physical interval of the qSS2, spans ∼887 Kb and harbors 110 protein coding genes. Among them, 22 genes were annotated with cell differentiation, tissue morphogenesis, signal transduction, carbohydrate metabolic process and fatty acid biosynthetic process, and might be involved in regulating various seed size and shape traits. Candidate genes were further prioritized based on the RNA-Seq gene expression data of seven seed tissues. Glycosyl hydrolase family members are involved in lignin and cellulose degradation pathway in microbes, but not characterized in plants (Davison and Blaxter, 2005). Three glycosyl hydrolase family genes were present in qSS2, of which two had shown high expression in seed tissues. Glyma.02G275500 encodes a 5′-AMP-activated protein kinase beta subunit. The gene encoding SNF1-related protein kinase-1 is plant homolog of mammalian 5′-AMP-activated protein kinase beta-2 subunit protein, which controls cell proliferation in Arabidopsis (Guérinier et al., 2013). Glyma.02G276000 encodes a cyclin-dependent kinase C2. Cyclin-dependent kinases play an important role in tissue differentiation. Loss of a cyclin-dependent kinase C showed an altered size of leaves and stomatal patterning in Arabidopsis (Zhao et al., 2017). Two PPR protein genes (Glyma.02G271600 and Glyma.02G276200) were present in qSS2. Genes encoding PPR have been shown to affect kernel size in maize (Huang et al., 2020) and seed development in maize and rice (Li et al., 2014). A protein phosphatase 2C gene in soybean controls seed weight in soybean (Lu et al., 2017). Glyma.02G278100, encoding a protein phosphatase 2C, is a potential candidate gene for seed size and shape at qSS2. Cui et al. (2020) delimited a QTL hotspot, qSS3, to a ∼1,126 kb region, and 123 genes were predicted in the candidate genomic region. Hina et al. (2020) predicted 88 candidate genes for seed size and shape traits in 4 QTL hotspots clustered on Chr.06, Chr.10, Chr.13, and Chr.20. These candidate genes are stepping stones for identifying causal genes for seed size and shape traits in soybean.
Conclusion
We have identified six stable QTLs for seed size and shape traits accounting for significant phenotypic contributions across multiple years, besides identifying several other environment-specific QTLs. QTL clusters identified for various seed size and shape traits indicate many strong inter-relationships among these traits. Major genomic locus qSS2, harboring three major QTLs was validated in NILs. Information on predicted candidate genes for qSS2 is useful for identifying causal genes determining seed size and shape traits in soybean. This study will assist in breeding programs aiming to improve seed size and shape traits in soybean.
Data Availability Statement
All datasets presented in this study are available in the article and associated Supplementary Material.
Author Contributions
DX designed the experiments. GK and DX carried out the experiments and analysis, and wrote the manuscript. Both authors contributed to the article and approved the submitted version.
Funding
This work was supported in-part by the Japan International Research Center for Agricultural Sciences (JIRCAS) under a research project “Environmental Stress-Tolerant Crops.”
Conflict of Interest
The authors declare that the research was conducted in the absence of any commercial or financial relationships that could be construed as a potential conflict of interest.
Acknowledgments
We thank Ms. Nozawa, Ms. Sato, and Ms. Kawashima for their assistance in the experiments.
Supplementary Material
The Supplementary Material for this article can be found online at: https://www.frontiersin.org/articles/10.3389/fgene.2021.646102/full#supplementary-material
Supplementary Table 1 | Phenotypic differences between two parents of recombinant inbred lines (RIL) population, K099 and Fendou 16.
Supplementary Table 2 | Descriptive statistics of seed size and shape traits evaluated in recombinant inbred line (n = 94) population developed from cross K099 × Fendou 16.
Supplementary Table 3 | Linkage map information of 1662 markers mapped in K099 × Fendou 16 RILs.
Supplementary Table 4 | Statistical details for linkage map of K099 × Fendou 16 RILs.
Supplementary Table 5 | Seed size and shape related QTLs identified in RILs by composite interval mapping (CIM).
Supplementary Table 6 | Comparisons of QTLs for seed size and shape related traits identified by inclusive composite interval mapping (ICIM) and composite interval mapping (CIM).
Supplementary Table 7 | Phenotypic differences for seed size and shape traits between two NILs for qSS2, NILs-K and NILs-F.
Supplementary Table 8 | Predicted protein coding genes in the physical interval of qSS2 and their annotation information according to Williams 82 assembly Gmax version 2.0 in SoyBase.
Supplementary Table 9 | Predictive candidate genes in qSS2.
Footnotes
- ^ https://www.legumebase.brc.miyazaki-u.ac.jp/
- ^ https://www.ars-grin.gov/npgs/
- ^ https://www.soybase.org/
References
Allen, F. L. (1994). “Usefulness of plant genome mapping to plant breeding,” in Plant Genome Analysis, ed. P. Gresshoff (Boca Raton, FL: CRC Press), 11–18. doi: 10.1201/9781003068907-2
Cao, Y., Li, S., He, X., Chang, F., Kong, J., Gai, J., et al. (2017). Mapping QTLs for plant height and flowering time in a Chinese summer planting soybean RIL population. Euphytica 213:39. doi: 10.1007/s10681-016-1834
Che, J., Ding, J., Liu, C., Xin, D., Jiang, H., Hu, G., et al. (2014). Quantitative trait loci of seed traits for soybean in multiple environments. Genet. Mol. Res. 13, 4000–4012. doi: 10.4238/2014.may.23.11
Chen, C., Chen, H., Zhang, Y., Thomas, H. R., Frank, M. H., He, Y., et al. (2020). TBtools: an integrative toolkit developed for interactive analyses of big biological data. Mol. Plant 13, 1194–1202. doi: 10.1016/j.molp.2020.06.009
Cui, B., Chen, L., Yang, Y., and Liao, H. (2020). Genetic analysis and map-based delimitation of a major locus qSS3 for seed size in soybean. Plant Breed. 139, 1145–1157. doi: 10.1111/pbr.12853
Cui, C. X., and Xuan, Y. N. (2007). Analysis of the factors affecting soybean trade in China and suggestions of strategies. World Agric. 29, 7–10.
Cui, Z., James, A., Miyazaki, S., Wilson, R. F., and Carter, T. (2004). “Breeding specialty soybeans for traditional and new soyfoods,” in Soybeans as Functional Foods and Ingredients, ed. K. Liu (Champaign, IL: AOSC Press), 274–332.
Davison, A., and Blaxter, M. (2005). Ancient origin of glycosyl hydrolase family 9 cellulase genes. Mol. Biol. Evol. 22, 1273–1284. doi: 10.1093/molbev/msi107
Enoki, H., and Takeuchi, Y. (2018). “New genotyping technology, GRAS-Di, using next generation sequencer,” in Proceedings of the Plant and Animal Genome Conference XXVI, (San Diego, CA).
Fang, C., Ma, Y., Wu, S., Liu, Z., Wang, Z., Yang, R., et al. (2017). Genome-wide association studies dissect the genetic networks underlying agronomical traits in soybean. Genome Biol. 18:161. doi: 10.1186/s13059-017-1289-9
Fujii, K., Sayama, T., Takagi, K., Kosuge, K., Okano, K., Kaga, A., et al. (2018). Identification and dissection of single seed weight QTLs by analysis of seed yield components in soybean. Breed Sci. 68, 177–187. doi: 10.1270/jsbbs.17098
Guérinier, T., Millan, L., Crozet, P., Oury, C., Rey, F., Valot, B., et al. (2013). Phosphorylation of p27(KIP1) homologs KRP6 and 7 by SNF1-related protein kinase-1 links plant energy homeostasis and cell proliferation. Plant J. 75, 515–525. doi: 10.1111/tpj.12218
Hao, D., Cheng, H., Yin, Z., Cui, S., Zhang, D., Wang, H., et al. (2012). Identification of single nucleotide polymorphisms and haplotypes associated with yield and yield components in soybean (Glycine max) landraces across multiple environments. Theor. Appl. Genet. 124, 447–458. doi: 10.1007/s00122-011-1719-0
Heffelfinger, C., Fragoso, C. A., and Lorieux, M. (2017). Constructing linkage maps in the genomics era with MapDisto 2.0. Bioinformatics 33, 2224–2225. doi: 10.1093/bioinformatics/btx177
Hina, A., Cao, Y., Song, S., Li, S., Sharmin, R. A., Elattar, M. A., et al. (2020). High-Resolution mapping in two RIL populations refines major “QTL Hotspot” regions for seed size and shape in soybean (Glycine max L.). Int. J. Mol. Sci. 21:1040. doi: 10.3390/ijms21031040
Hoeck, J. A., Fehr, W. R., Shoemaker, R. C., Welke, G. A., Johnson, S. L., and Cianzio, S. R. (2003). Molecular marker analysis of seed size in soybean. Crop Sci. 43, 68–74. doi: 10.2135/cropsci2003.6800
Hosoya, S., Hirase, S., Kikuchi, K., et al. (2019). Random PCR-based genotyping by sequencing technology GRAS-Di (genotyping by random amplicon sequencing, direct) reveals genetic structure of mangrove fishes. Mol. Ecol. Resour. 19, 1153–1163. doi: 10.1111/1755-0998.13025
Hu, Z., Zhang, H., Kan, G., Ma, D., Zhang, D., Shi, G., et al. (2013). Determination of the genetic architecture of seed size and shape via linkage and association analysis in soybean (Glycine max L. Merr.). Genetica 141, 247–254. doi: 10.1007/s10709-013-9723-8
Huang, J., Lu, G., Liu, L., Raihan, M. S., Xu, J., Jian, L., et al. (2020). The kernel size-related quantitative trait locus qKW9 encodes a pentatricopeptide repeat protein that affects photosynthesis and grain filling. Plant Physiol. 183, 1696–1709. doi: 10.1104/pp.20.00374
Kato, S., Sayama, T., Fujii, K., Yumoto, S., Kono, Y., Hwang, T.-Y., et al. (2014). A major and stable QTL associated with seed weight in soybean across multiple environments and genetic backgrounds. Theor. Appl. Genet. 127, 1365–1374. doi: 10.1007/s00122-014-2304-0
Kumawat, G., Gupta, S., Ratnaparkhe, M. B., Maranna, S., and Satpute, G. K. (2016). QTLomics in soybean: a way forward for translational genomics and breeding. Front. Plant Sci. 7:1852. doi: 10.3389/fpls.2016.01852
Lam, H. M., Xu, X., Liu, X., Chen, W., Yang, G., Wong, F. L., et al. (2010). Resequencing of 31 wild and cultivated soybean genomes identifies patterns of genetic diversity and selection. Nature Genet. 42, 1053–1059. doi: 10.1038/ng.715
Lander, E. S., and Botstein, D. (1989). Mapping mendelian factors underlying quantitative traits using RFLP linkage maps. Genetics 121, 185–199. doi: 10.1093/genetics/121.1.185
Li, H. (2013). Aligning sequence reads, clone sequences and assembly contigs with BWA-MEM. arXiv [preprint]. https://arxiv.org/abs/1303.3997 (accessed May 26, 2013).
Li, J., Zhao, J., Li, Y., Gao, Y., Hua, S., Nadeem, M., et al. (2019). Identification of a novel seed size associated locus SW9-1 in soybean. Crop J. 7, 548–559. doi: 10.1016/j.cj.2018.12.010
Li, M., Chen, L., Zeng, J., Razzaq, M. K., Xu, X., Xu, Y., et al. (2020). Identification of additive–epistatic QTLs conferring seed traits in soybean using recombinant inbred lines. Front. Plant Sci. 11:566056. doi: 10.3389/fpls.2020.566056
Li, X. J., Zhang, Y. F., Hou, M., Sun, F., Shen, Y., Xiu, Z. H., et al. (2014). Small kernel 1 encodes a pentatricopeptide repeat protein required for mitochondrial nad7 transcript editing and seed development in maize (Zea mays) and rice (Oryza sativa). Plant J. 79, 797–809. doi: 10.1111/tpj.12584
Li, Y., Fan, C., Xing, Y., Jiang, Y., Luo, L., Sun, L., et al. (2011). Natural variation in GS5 plays an important role in regulating grain size and yield in rice. Nat. Genet. 43, 1266–1269. doi: 10.1038/ng.977
Liang, H., Xu, L., Yu, Y., Yang, H., Dong, W., and Zhang, H. (2016). Identification of QTLs with main, epistatic and QTL by environment interaction e?ects for seed shape and hundred-seed weight in soybean across multiple years. J. Genet. 95, 475–477. doi: 10.1007/s12041-016-0648-8
Liang, H. Z., Li, W. D., Wang, H., and Fang, X. J. (2005). Genetic effects on seed traits in soybean. Acta Genetica Sinica 32, 1199–1204.
Liu, D., Yan, Y., Fujita, Y., and Xu, D. (2018). Identification and validation of QTLs for 100-seed weight using chromosome segment substitution lines in soybean. Breed Sci. 68, 442–448. doi: 10.1270/jsbbs.17127
Lu, X., Xiong, Q., Cheng, T., Li, Q. T., Liu, X. L., Bi, Y. D., et al. (2017). A PP2C-1 allele underlying a quantitative trait locus enhances soybean 100-seed weight. Mol. Plant 10, 670–684. doi: 10.1016/j.molp.2017.03.006
Ma, M., Wang, Q., Li, Z. J., Cheng, H. H., Li, Z. J., Liu, X. L., et al. (2015). Expression of TaCYP78A3, a gene encoding cytochrome P450 CYP78A3 protein in wheat (Triticum aestivum L.), affects seed size. Plant J. 83, 312–325. doi: 10.1111/tpj.12896
Mao, H., Sun, S., Yao, J., Wang, C., Yu, S., Xu, C., et al. (2010). Linking differential domain functions of the GS3 protein to natural variation of grain size in rice. Proc. Natl. Acad. Sci. U S A. 107, 19579–19584. doi: 10.1073/pnas.1014419107
Meng, L., Li, H., Zhang, L., and Wang, J. (2015). QTL IciMapping: integrated software for genetic linkage map construction and quantitative trait locus mapping in biparental populations. Crop J. 3, 269–283. doi: 10.1016/j.cj.2015.01.001
Miki, Y., Yoshida, K., Enoki, H., et al. (2020). GRAS-Di system facilitates high-density genetic map construction and QTL identification in recombinant inbred lines of the wheat progenitor Aegilops tauschii. Sci Rep. 10:21455. doi: 10.1038/s41598-020-78589-4
Nguyen, C. X., Paddock, K. J., Zhang, Z., and Stacey, M. G. (2020). GmKIX8-1 regulates organ size in soybean and is the causative gene for the major seed weight QTL qSw17-1. New Phytol. 229, 920–934. doi: 10.1111/nph.16928
Niu, Y., Xu, Y., Liu, X. F., Yang, S. X., Wei, S. P., Xie, F. T., et al. (2013). Association mapping for seed size and shape traits in soybean cultivars. Mol. Breed. 31, 785–794. doi: 10.1007/s11032-012-9833-5
Salas, P., Oyarzo-Llaipen, J. C., Wang, D., Chase, K., and Mansur, L. (2006). Genetic mapping of seed shape in three populations of recombinant inbred lines of soybean (Glycine max L. Merr.). Theor. Appl. Genet. 113, 1459–1466. doi: 10.1007/s00122-006-0392-1
Severin, A. J., Woody, J. L., Bolon, Y.-T., Joseph, B., Diers, B. W., Farmer, A. D., et al. (2010). RNA-Seq atlas of glycine max: a guide to the soybean transcriptome. BMC Plant Biol. 10:160. doi: 10.1186/1471-2229-10-160
Sun, Y. N., Pan, J. B., Shi, X. L., Du, X. Y., Wu, Q., Qi, Z. M., et al. (2012). Multi-environment mapping and meta-analysis of 100-seed weight in soybean. Mol. Biol. Rep. 39, 9435–9443. doi: 10.1007/s11033-012-1808-4
Tanksley, S. D. (1993). Mapping polygenes. Annu. Rev. Genet. 27, 205–233. doi: 10.1146/annurev.ge.27.120193.001225
Teng, W., Feng, L., Li, W., Wu, D., Zhao, X., Han, Y., et al. (2017). Dissection of the genetic architecture for soybean seed weight across multiple environments. Crop Pasture Sci. 68, 358–365. doi: 10.1071/cp16462
Voorrips, R. E. (2002). MapChart: software for the graphical presentation of linkage maps and QTLs. J. Hered. 93, 77–78. doi: 10.1093/jhered/93.1.77
Wang, S., Basten, C. J., and Zeng, Z.-B. (2012). Windows QTL Cartographer 2.5. Raleigh, NC: Department of Statistics, North Carolina State University.
Wang, X., Li, Y., Zhang, H., Sun, H. G., Zhang, W., and Qiu, L. (2015). Evolution and association analysis of GmCYP78A10 gene with seed size/weight and pod number in soybean. Mol. Biol. Rep. 42, 489–496. doi: 10.1007/s11033-014-3792-3
Wei, T., and Simko, V. (2017). R Package “Corrplot”: Visualization of a Correlation Matrix (Version 0.84).
Wilson, D. O. Jr. (1995). “Storage of orthodox seeds,” in Seed Quality: Basic Mechanisms Agricultural Implications, ed. A. S. Basra (New York, NY: Food Products Press), 173–208. doi: 10.4324/9781003075226-6
Wu, D., Zhan, Y., Sun, Q., Xu, L., Lian, M., Zhao, X., et al. (2018). Identification of quantitative trait loci underlying soybean (Glycine max [L.] Merr.) seed weight including main, epistatic and QTL×environment efiects in difierent regions of Northeast China. Plant Breed. 137, 194–202. doi: 10.1111/pbr.12574
Xie, F. T., Niu, Y., Zhang, J., Bu, S. H., Zhang, H. Z., Geng, Q. C., et al. (2014). Fine mapping of quantitative trait loci for seed size traits in soybean. Mol. Breed. 34, 2165–2178. doi: 10.1007/s11032-014-0171-7
Xu, F., Fang, J., Ou, S., Gao, S., Zhang, F., Du, L., et al. (2015). Variations in CYP78A13 coding region influence grain size and yield in rice. Plant Cell Environ. 38, 800–811. doi: 10.1111/pce.12452
Xu, Y., Li, H. N., Li, G. J., Wang, X., Cheng, L. G., and Zhang, Y. M. (2011). Mapping quantitative trait loci for seed size traits in soybean (Glycine max L. Merr.). Theor. Appl. Genet. 122, 581–594. doi: 10.1007/s00122-010-1471-x
Yang, H., Wang, W., He, Q., Xiang, S., Tian, D., Zhao, T., et al. (2017). Chromosome segment detection for seed size and shape traits using an improved population of wild soybean chromosome segment substitution lines. Physiol. Mol. Biol. Plants 23, 877–889. doi: 10.1007/s12298-017-0468-1
Keywords: soybean, seed size, seed shape, seed yield, quantitative trait loci, stable QTLs, qSS2
Citation: Kumawat G and Xu D (2021) A Major and Stable Quantitative Trait Locus qSS2 for Seed Size and Shape Traits in a Soybean RIL Population. Front. Genet. 12:646102. doi: 10.3389/fgene.2021.646102
Received: 25 December 2020; Accepted: 23 March 2021;
Published: 16 April 2021.
Edited by:
Yuan-Ming Zhang, Huazhong Agricultural University, ChinaReviewed by:
Tuanjie Zhao, Nanjing Agricultural University, ChinaJia Wen, University of North Carolina at Chapel Hill, United States
Youlu Yuan, Cotton Research Institude, Chinese Academy of Agricultural Sciences (CAAS), China
Copyright © 2021 Kumawat and Xu. This is an open-access article distributed under the terms of the Creative Commons Attribution License (CC BY). The use, distribution or reproduction in other forums is permitted, provided the original author(s) and the copyright owner(s) are credited and that the original publication in this journal is cited, in accordance with accepted academic practice. No use, distribution or reproduction is permitted which does not comply with these terms.
*Correspondence: Donghe Xu, xudh@jircas.affrc.go.jp
†ORCID: Giriraj Kumawat, orcid.org/0000-0003-3380-1173; Donghe Xu, orcid.org/0000-0002-2447-795X