- Department of Biology, Brigham Young University, Provo, UT, United States
Compound heterozygous (CH) variants occur when two recessive alleles are inherited and the variants are located at different loci within the same gene in a given individual. CH variants are important contributors to many different types of recessively inherited diseases. However, many studies overlook CH variants because identification of this type of variant requires knowing the parent of origin for each nucleotide. Using computational methods, haplotypes can be inferred using a process called “phasing,” which estimates the chromosomal origin of most nucleotides. In this paper, we used germline, phased, whole-genome sequencing (WGS) data to identify CH variants across seven pediatric diseases (adolescent idiopathic scoliosis: n = 16, congenital heart defects: n = 709, disorders of sex development: n = 79, ewing sarcoma: n = 287, neuroblastoma: n = 259, orofacial cleft: n = 107, and syndromic cranial dysinnervation: n = 172), available as parent-child trios in the Gabriella Miller Kids First Data Resource Center. Relatively little is understood about the genetic underpinnings of these diseases. We classified CH variants as “potentially damaging” based on minor allele frequencies (MAF), Combined Annotation Dependent Depletion scores, variant impact on transcription or translation, and gene-level frequencies in the disease group compared to a healthy population. For comparison, we also identified homozygous alternate (HA) variants, which affect both gene copies at a single locus; HA variants represent an alternative mechanism of recessive disease development and do not require phasing. Across all diseases, 2.6% of the samples had a potentially damaging CH variant and 16.2% had a potentially damaging HA variant. Of these samples with potentially damaging variants, the average number of genes per sample was 1 with a CH variant and 1.25 with a HA variant. Across all samples, 5.1 genes per disease had a CH variant, while 35.6 genes per disease had a HA variant; on average, only 4.3% of these variants affected common genes. Therefore, when seeking to identify potentially damaging variants of a putatively recessive disease, CH variants should be considered as potential contributors to disease development. If CH variants are excluded from analysis, important candidate genes may be overlooked.
Introduction
Each year in the United States, ∼3.0% of babies are born with a structural defect, and ∼11,050 children under the age of 15 are diagnosed with pediatric cancer (Lupo et al., 2019; American Cancer Society, 2020). Researchers are working to understand the genetic causes of these diseases, now often via whole-genome sequencing (WGS) (Zhang et al., 2015; Gröbner et al., 2018; Lupo et al., 2019). When researchers analyze DNA sequencing data, the parent of origin for each nucleotide is often unknown (Choi et al., 2018). While this is not an issue when looking at heterozygous variants in dominantly inherited diseases or when identifying homozygous alternate (HA) variants in recessively inherited diseases, it is problematic when looking at more complex inheritance patterns, such as compound heterozygous (CH) variants (Sanjak et al., 2017). Under the assumptions of recessive disease inheritance, in the majority of cases, a single heterozygous variant in a gene does not result in decreased fitness of an organism (Morrill and Amon, 2019). However, CH variants—which consist of alternate alleles positioned at different loci within a gene on opposite homologous chromosomes—may result in no functional copies of the associated proteins and a decrease in overall fitness. CH variants have been observed in many pediatric diseases. For example, pathogenic CH variants in the ASXL3 gene can lead to a disruption in ASXL3 protein expression, potentially contributing to the development of congenital heart disease (Fu et al., 2020). Additionally, potentially damaging CH variants have been identified in many cancer predisposition genes, across various pediatric cancer types (Miller and Piccolo, 2020a). Although the importance of assessing CH variants in pediatric diseases has been established, researchers may ignore these types of variants in genome-wide studies due to additional steps required to identify and interpret them; one of these steps is haplotype estimation via computational phasing. It has been shown that using a population-based, haplotype reference panel and/or trio-based samples can improve phasing as much as 10-fold (Choi et al., 2018); however, this process is computationally expensive, and current phasing algorithms require specific file formats or that reads be aligned to a specific reference genome and be free of multi-allelic positions. These requirements may deter some researchers from performing phasing—and thus identifying CH variants.
Prior studies involving CH variant identification have focused primarily on individual diseases, one or a few samples, or specific genes (Miller and Piccolo, 2020a). Furthermore, little is known about how to filter and classify CH variants in genome-wide studies. Common practice in genetic studies is to filter variants based on population-level MAF; however, it is unclear how this applies to CH variants because it would be infeasible to compile a database that estimates the frequency of all possible combinations of in trans alleles in a control population. Many combinations are extremely rare, and others are private to a single individual. Furthermore, the number of possible combinations will differ by gene—many more combinations can occur in longer genes than shorter ones.
In this study, we surveyed germline CH variants in a total of 1,629 affected samples across 7 pediatric diseases: adolescent idiopathic scoliosis, congenital heart defects, disorders of sex development, ewing sarcoma, neuroblastoma, orofacial cleft, and syndromic cranial dysinnervation. Little is understood about the genetic underpinnings of these diseases (Beaty et al., 2016; Eggers et al., 2016; Grauers et al., 2016; Brohl et al., 2017; Singh et al., 2017; Tolbert et al., 2017; Pierpont et al., 2018). We used WGS data from the Gabriella Miller Kids First initiative, which has a mission of generating high-quality sequencing data for large cohorts of pediatric-disease patients and making those data available for broad use (Heath et al., 2019). For most probands, sequencing data were available for the affected child and both of her/his parents. Thus, we were able to estimate haplotypes using the best-available computational-phasing approach (Delaneau et al., 2013; Choi et al., 2018). We used computational tools to estimate pathogenicity of individual alleles within a given gene and then identified genes with multiple, potentially pathogenic alleles on homologous chromosomes. To better understand the frequency of CH variants in the general population, we estimated gene-level frequencies of CH variants in healthy controls and used these as a comparison group against our probands. Finally, we compared the observed frequencies of CH variants in each disease against the frequencies of HA variants. The resulting observations yielded novel insights about how often CH variants occur in diverse pediatric diseases, how one disease compares to another, and genes that may play a role in recessive inheritance of these pediatric diseases. This study is the first to report multi-cohort, genome-wide evaluations of CH variants in pediatric diseases.
Materials and Methods
Disease Cohorts
We analyzed trio data stored in the Gabriella Miller Kids First Data Resource Center (Heath et al., 2019). These data were generated via Illumina-based, WGS of non-disease cells (Gabriella Miller Kids First Pediatric Research Program, 2019) from each proband and their parents. The diseases we studied are adolescent idiopathic scoliosis (16 trios), congenital heart defects (709 trios), disorders of sex development (79 trios), Ewing’s sarcoma (287 trios), neuroblastoma (259 trios), orofacial cleft (107 trios), and syndromic cranial dysinnervation (172 trios). Specific diagnoses for each disease are in Supplementary Table 1. Trios were selected based on whether or not they were labeled as a trio in the database and DNA variant data were available for all three members of the trio. In addition, we filtered patients based on research use consent status. For all diseases except syndromic cranial dysinnervation, we used trios that had been consented for general research use or health/medical/biomedical use. For syndromic cranial dysinnervation, we used trios that were consented for disease-specific use (in accordance with data-use restrictions).
Variant Identification for Disease Cohorts
In the Gabriella Miller Kids First Data Resource Center, germline variant data are provided as gVCF files (Poplin et al., 2017). Using these files as input, we identified CH and HA variants in a series of steps including file aggregation, liftover, phasing, annotation, and filtering (described below and in Figure 1). We completed these steps using scripts adapted from the CompoundHetVIP pipeline (Miller and Piccolo, 2020b). These and other scripts are available in an open-source repository at https://github.com/dmiller903/CompoundHetVIP-GMKF. This repository contains Python scripts1 with custom code for parsing metadata and handling the gVCF files in a way that is specific to the Gabriella Miller Kids First Data Resource Center. All of these scripts are stored within a Docker image, which is available at: https://hub.docker.com/r/dmill903/compound-het-vip-gmkf. A detailed example of how data from each disease was processed can be viewed at: https://github.com/dmiller903/CompoundHetVIP-GMKF/blob/master/usage_example.pdf.
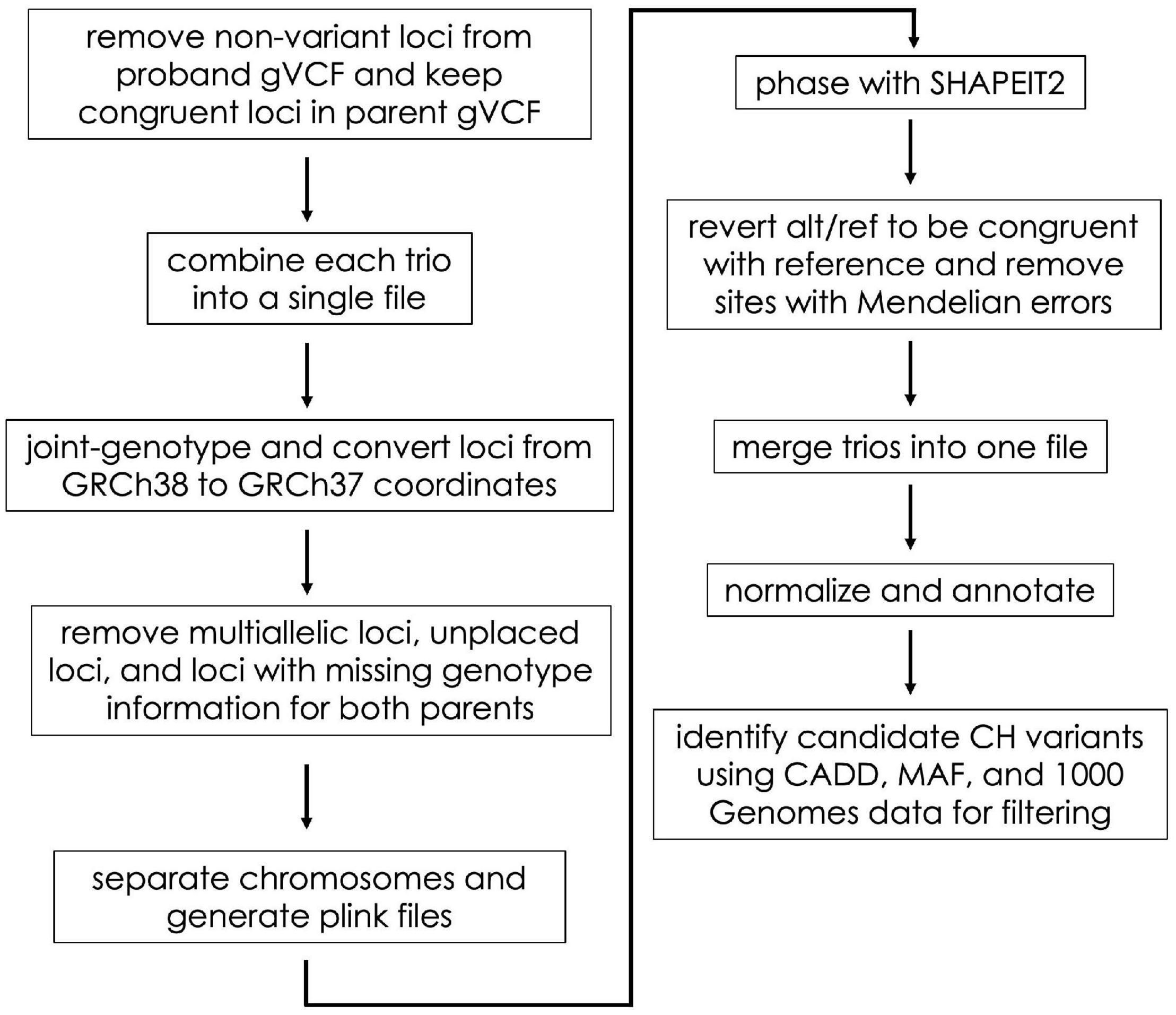
Figure 1. Flow diagram of the gVCF processing steps. These steps were taken prior to HA and CH variant identification.
For each disease, gVCF, clinical, and manifest files were downloaded from the Gabriella Miller Kids First Data Resource Center. These files were downloaded in September 2019 (adolescent idiopathic scoliosis), January 2020 (disorders sex development), February 2020 (Ewing’s sarcoma), March 2020 (congenital heart defects, orofacial cleft, syndromic cranial dysinnervation), and April 2020 (orofacial cleft). The clinical and manifest files contain information about file names, sample ID’s, family relationships, disease status, etc. The information from the clinical and manifest files were combined using the “kids_first_meta.py” script. The resulting combined file was used as input to many of the subsequent scripts to keep track of file names, family relationships, probands, etc. Unlike VCF files (Danecek et al., 2011), gVCF files contain information for variant sites as well as non-variant positions (Poplin et al., 2017). These files are large and would take much longer to process if non-variant positions were included throughout the whole pipeline. Therefore, using the “keep_variant_sites.py” script, we removed all non-variant positions for each proband (Figure 1). Using the variant positions of the proband as a reference, the script then retained the same positions in each parent’s gVCF file (regardless of whether the position was variant or non-variant).
The “combine_trios.py” script was used for each trio to combine data from all family members into a single file; we used Genome Analysis Toolkit (GATK) (version 4.0.5.1) for this step (Poplin et al., 2017; Figure 1). In addition, the script created a “.fam” file to be used later in processing. As part of its data-processing pipeline, the KFDRC had aligned reads to build GRCh38 of the human reference genome (Schneider et al., 2016). Alignment to this build was an issue because subsequent tools in our pipeline required the files to be aligned to GRCh37. Therefore, our “liftover.py” script joint-genotyped the combined trio files using GATK and converted the coordinates to GRCh37 using Picard’s (Picard Tools, 2019) Liftover tool. During liftover, some sites with unknown positions in the GRCh37 build were included in the VCF file; we created a “remove_unplaced_multiallelic.py” script to remove these positions. In addition, the script removed multiallelic or duplicate positions because programs such as PLINK2 (Purcell and Chang, 2020; Chang et al., 2015) (used next) and SHAPEIT2 (Delaneau et al., 2013) (used later) cannot handle these types of sites. For each trio, sites containing missing genotype information (i.e., “./.”) for both parents were removed to improve phasing accuracy.
SHAPEIT2 requires chromosomes to be phased separately. PLINK files are also needed by SHAPEIT2 during phasing. Our “separate_chr_generate_plink.py” script separated the data for each trio file into chromosome files (autosomes only) and executed PLINK to generate the files needed for phasing (.bed,.bim,.fam). Next, we used “phase_with_shapeit.py” to phase each chromosome. The parameters for phasing were set so that SHAPEIT2 used family relationship genotype information and also used the 1000 Genomes Project (1000GP) phase 3 haplotype reference panel. Default parameters were used. The files used for phasing can be found at https://mathgen.stats.ox.ac.uk/impute/1000GP_Phase3.html and through the 1000GP online database (1000 Genomes Project Consortium, Auton et al., 2015).
In some cases, SHAPEIT2 switches the REF and ALT alleles during phasing. Our “alt_ref_revert.py” script ensured that the REF/ALT alleles of the phased VCF files were congruent with the REF/ALT order of the reference genome. In addition, it removed sites with Mendelian errors. To make subsequent analysis of the phased files easier, “concat_merge_phased_vcf.py” executed bcftools (Li, 2011) (version 1.9) to concatenate all phased chromosomes for each trio into a single trio file. Finally, using bcftools, each concatenated trio file was merged into a single VCF file containing all trios for a particular disease, and a.fam file was created for this merged file.
As a preprocessing step, GEMINI (Paila et al., 2013) recommends left trimming and normalizing VCF files using vt tools (Tan et al., 2015). Our “vt_split_trim_left_align.py” script used vt tools (version 2015.11.10) to trim and normalize the phased VCF file. Next, “annotate.py” executed snpEff (Cingolani et al., 2012) to annotate the variants with information about whether or not a variant is in a gene, the type of variant, the predicted effect the variant may have on protein coding (i.e., variant impact severity, and whether or not the variant causes loss of protein function), etc.
GEMINI (version 0.30.2) allowed us to provide additional annotations and then filter and summarize the annotated genetic variants. The “gemini_load.py” script loaded the VCF file into a GEMINI database, which provided information such as Combined Annotation Dependent Depletion (CADD) scores (Rentzsch et al., 2019) and MAF from the 1000GP (1000 Genomes Project Consortium, Auton et al., 2015) or gnomAD (Karczewski et al., 2020) (version 2.1). The “identify_CH_variants.py” script first exports the GEMINI database to a.tsv file and then uses that file to identify CH variants. CH variants were identified based on five criteria: (1) whether snpEff classified each of the alleles contributing to the CH variant as “HIGH” impact, (2) whether each allele had a CADD score ≥ 15, (3) neither parent was HA at either allele as a HA allele would unlikely be damaging given that it is present in a healthy parent, (4) neither parent had the exact same alleles making up a CH variant as found in the child, and (5) at least one allele of the CH variant had a MAF ≤ 5% (gnomAD MAF used as priority over 1000GP). We allowed one of the alleles to have a MAF > 5% to include scenarios where a single, damaging, common allele does not have a phenotypic effect when found to be heterozygously inherited, but may have a phenotypic effect when paired with a different, damaging, rare allele found on the opposite chromosome at a different position within the same gene. The genes in the final output are referred to as having “potentially damaging” variants.
To identify HA variants, the “identify_HA_variants.py” script was used. This script used the same GEMINI database and selected variants that were of “HIGH” impact, had a MAF ≤ 5%, and a CADD score ≥ 15. HA variants for which either parent had a HA variant at the same position as the child were excluded from the final output file.
Identification of CH and HA Variants in 1000 Genomes Samples
Data from the 1000GP (1000 Genomes Project Consortium, Auton et al., 2015) were used as a baseline to better understand the frequency of potentially damaging CH and HA variants in the general population. Phased VCF data for the 1000GP were obtained from ftp://ftp.1000genomes.ebi.ac.uk/vol1/ftp/release/20130502/. This repository contains genotypes/haplotypes for 2,504 unrelated individuals. Because these files had previously been phased using a combination of Beagle (Browning and Browning, 2007) and SHAPEIT2, many of the steps required for the GMKF data were unnecessary. We processed chromosome-level VCF files (autosomes only) starting with the “concat_merge_phased_vcf.py” script and progressing until the “add_GDI_and_gene_lengths.py” script in the CompoundHetVIP pipeline (Miller and Piccolo, 2020b), a generalized version of the pipeline that was used to identify variants in the GMKF data. Because the 1000GP data does not contain trios or other family relationships, the “identify_CH_variants.py” script was not able to exclude variants based on observations in parents. However, we used the same CADD, MAF, and impact filtering criteria for the 1000 Genomes data as what we used for the GMKF variants.
Filtering CH Variants Using 1000 Genomes Data as Reference
Using RStudio RStudio Team (2020) (version 1.2.5042), R (R Core Team, 2020) (version 3.6.3) and the tidyverse package (Wickham et al., 2019) (version 1.3.0), we further filtered the genes using potentially damaging CH variants from 1000GP as a reference. We identified genes in which a CH variant was present in more than 1% of the 1000 Genome samples and excluded these genes from the GMKF analysis. This same logic was used to filter the HA variants.
Known Tumor Suppressor and Developmental Genes
Because inherited DNA variations in germline tissue can contribute to the inactivation of tumor suppressor genes and result in subsequent cancer development (Wang et al., 2018), we used R to identify which of the genes with potentially damaging CH and HA variants were in known tumor suppressor genes. In addition, given that both structural birth defects and pediatric cancers tend to occur at an early age, we identified which of the potentially damaged genes were in known developmental biology genes. During the identification process, a list of known tumor suppressor genes from Cancer Gene Census and a list of known developmental genes from Reactome (Reactome Developmental Biology pathway: R-HSA-1266738) were used (Sondka et al., 2018; Jassal et al., 2020). For these genes and all genes identified with potentially damaging CH variants, we used DisGeNET to determine whether any gene-disease associations had been identified in previous studies (Piñero et al., 2020). DisGeNET uses many different databases (e.g., UniProt, ClinVar, etc.) to establish gene-disease associations. One metric DisGeNET provides is a gene-disease association (GDA) score which ranges from 0 to 1. The more sources and publications that support a gene-disease association, the higher the value. We considered GDA values greater than or equal to 0.5 as strong evidence for a gene-disease association. In addition to GDA scores, we used DisGeNET to produce variant-disease association (VDA) scores for each potentially damaging variant contributing to a CH variant. VDA scores follow the same scale as GDA scores.
Pathway Analysis of Genes Containing Potentially Pathogenic CH Variants
Pathway enrichment analysis can help determine whether specific biological pathways are significantly enriched based on a list of candidate genes (Reimand et al., 2019). We used the R and the ReactomePA (Yu and He, 2016) package (version 1.30.0), to perform a pathway enrichment analysis for each disease. Default parameters were used. For each disease, the genes with CH variants that were retained after 1000GP data filtering were used as input. Pathways that had an adjusted p-value (adjusted using the Benjamini-Hochberg False Discovery Rate method; Benjamini and Hochberg, 1995) less than or equal to 0.05 and a q-value less than or equal to 0.2 were retained.
Results
In this study, we focus on scenarios in which at least two alternate alleles occurred on different chromosomes in the same gene and/or locus; thus, we assume the diseases follow an autosomal-recessive inheritance pattern in some cases and that these traits are subject to Mendelian inheritance. Autosomal-recessive and Mendelian inheritance have been associated with some pediatric cancer types and structural birth defects (Webber et al., 2015; Zhang et al., 2015; Miller and Piccolo, 2020a). For recessively inherited diseases, CH or HA variants may play a role if both alleles lead to loss of protein function. Therefore, we also identified HA variants, in which two non-reference alleles occurred at the same locus within a gene. We included these variants in the analysis as a way to show how the number of samples and genes with potentially damaging HA variants compares to the number of samples and genes with potentially damaging CH variants.
Across all analyzed pediatric diseases, the median number of unphased variants per sample was 5,509,545 prior to any data processing and variant-type classifications (Figure 2). After joint-genotyping, liftover, removing unplaced and multiallelic sites, phasing, and removing sites with Mendelian errors, the median number of unphased variants per sample was 3,894,315 (Figure 2). Therefore, on average, 70.7% of the original variants were available for CH and HA identification after these preprocessing steps. Joint-genotyping (which includes quality filtering) and phasing led to the greatest reductions in the number of unphased variants: 613,225 and 602,443 variants removed on average, respectively (Figure 2). Of the variants available for phasing, ∼86.7% were successfully phased on average. Unphased sites included those that were incompatible with the reference panel (e.g., SNP missing in reference haplotype panel), monomorphic or singleton SNPs (these sites are uninformative for phasing), and those with Mendelian inheritance errors (Delaneau et al., 2013).
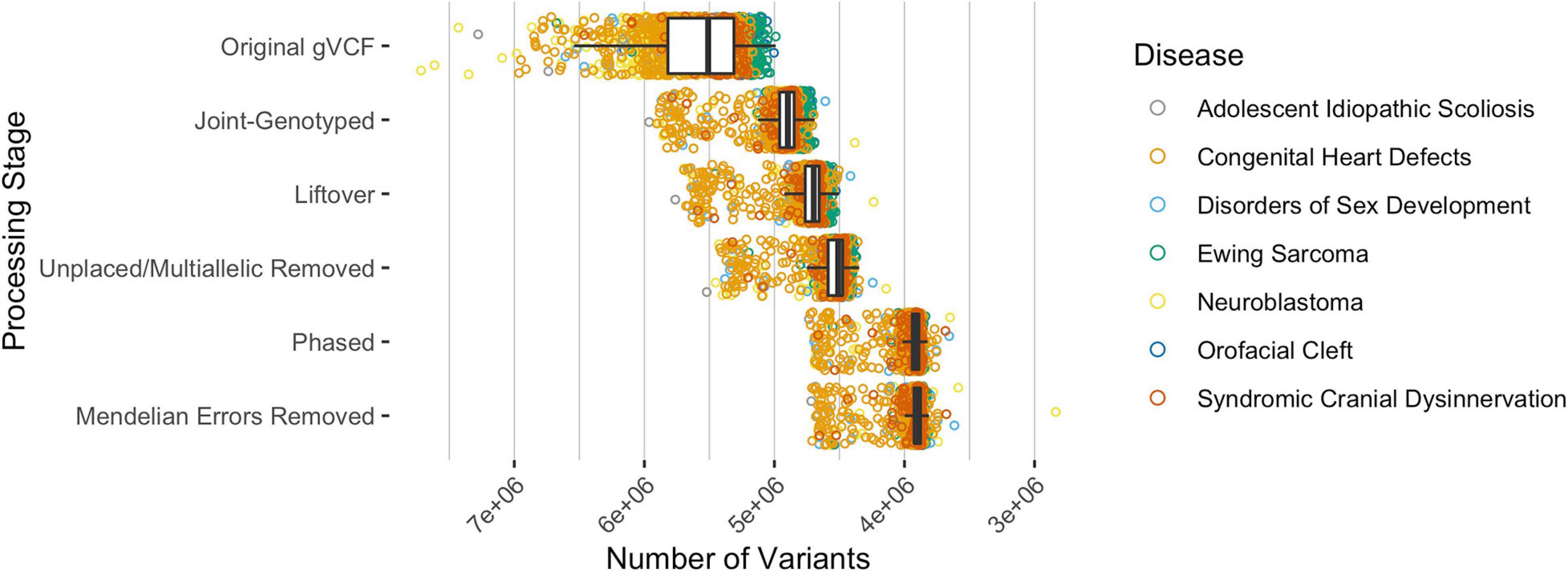
Figure 2. The median number of variants per sample, across all disease types, after each processing step where variants were excluded. The original gVCF files had a median of 5,509,545 unphased variants across all samples and diseases. Approximately 70.7% of the variants were available for CH and HA identification after processing the original gVCF files. Of the available variants, ∼86.7% were phased on average.
Across all disease types, the initial query for CH variants resulted in 146 probands and 41 unique genes with potentially damaging CH variants that met our minimum CADD, MAF, and gene-impact thresholds during variant identification (see section “Materials and Methods”). Across all diseases, based on our initial query, an average of 9.5% of the samples had at least one CH variant and there were an average of 10.9 genes per disease with potentially damaging CH variants. To further filter these potentially damaging variants, we used 1000GP data as a baseline of what to expect, in terms of CH variation, in a seemingly healthy population. Potentially damaging CH variants were identified in 151 genes and 883 samples. Of the samples with a potentially damaging CH variant, 26 of the genes were present in more than 1% of the samples (Figure 3). For each disease population, we retained genes in which a CH variant occurred in less than 1% of the 883 1000GP samples. After this filtering step, the number of samples and genes with potentially damaging CH variants was reduced (Table 1); across all diseases, the average percentage of samples with potentially damaging CH variants decreased to 3.3% and the average number of genes per disease with potentially damaging CH variants was 5.1 (25 total unique genes, 17 of which were not identified as having HA variants). We observed similar results for HA variants before and after 1000GP filtering (Table 2). For example, across all diseases, the average number of samples with potentially damaging HA variants was 22.2% per disease and there were an average of 45 genes per disease with potentially damaging HA variants. After 1000GP filtering, the average percentage of samples with potentially damaging HA variants was 17.2% per disease, and the average number of genes with potentially damaging HA variants was 35.6 per disease.
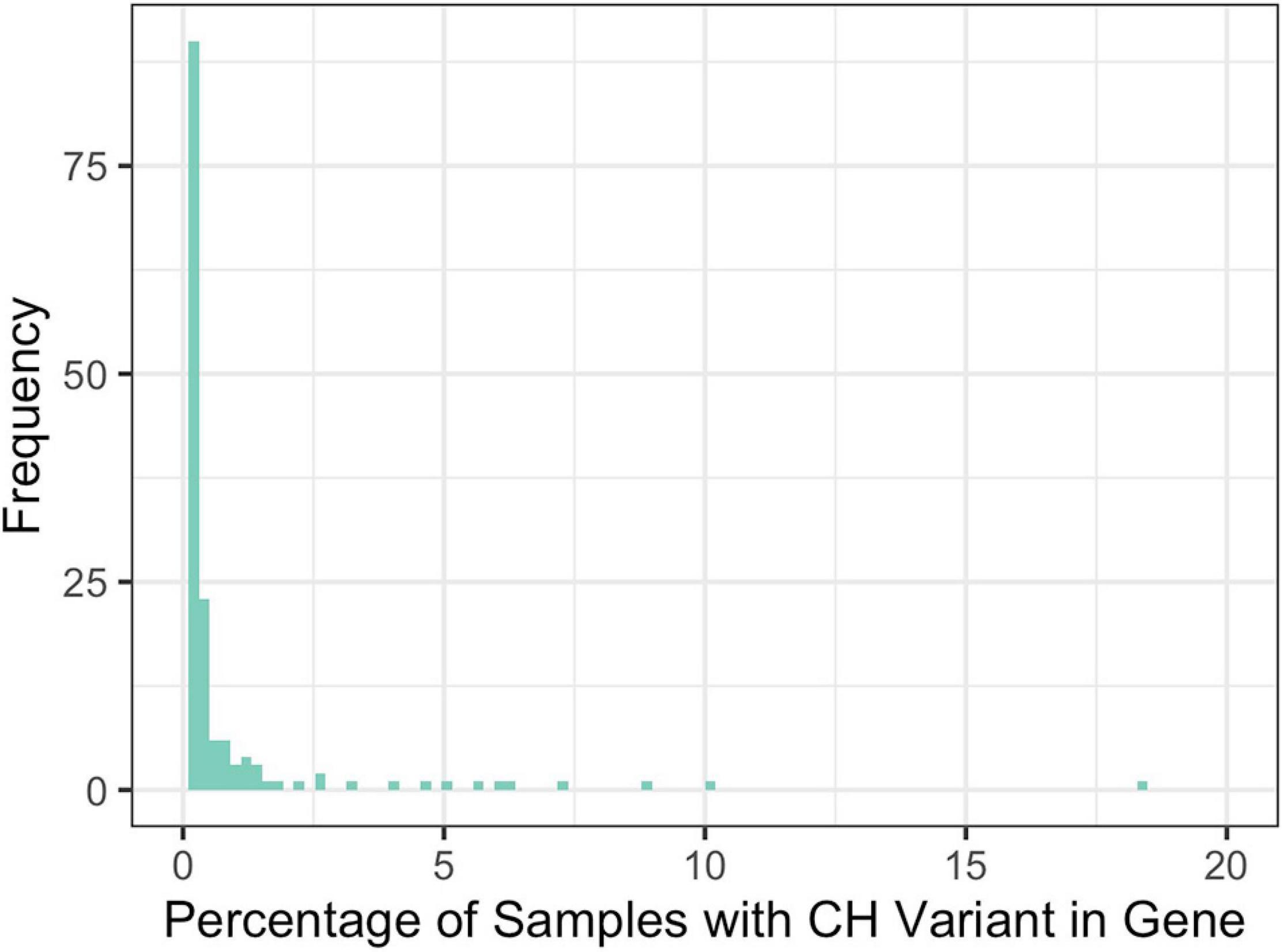
Figure 3. Percentage of 1000GP samples with a CH variant in a gene. Frequency represents the number of genes that were observed at a specific percentage.
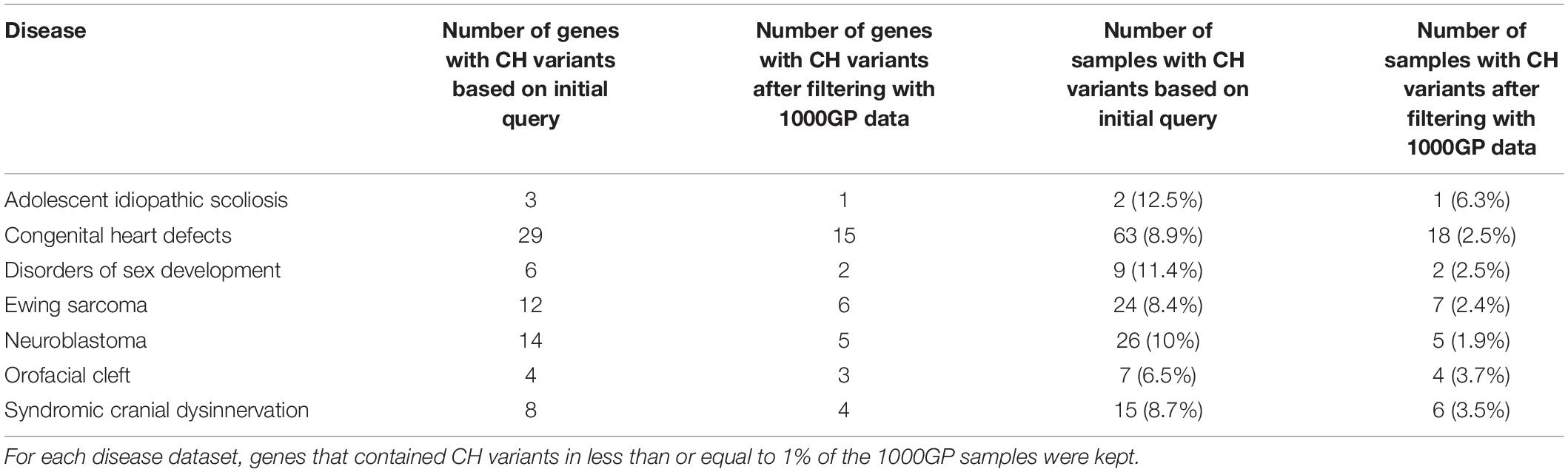
Table 1. The number of genes and samples with potentially damaging CH variants before and after filtering with the 1000GP data.
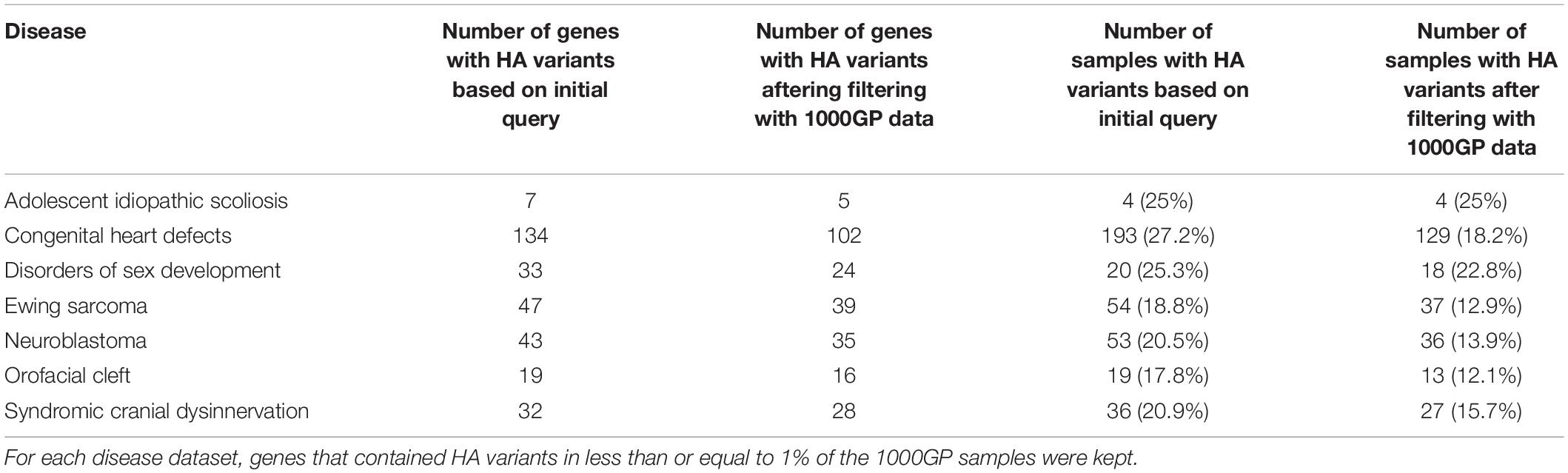
Table 2. The number of genes and samples with potentially damaging HA variants before and after filtering with the 1000GP data.
CH variants and HA variants sometimes affect common genes, generally they occur in different genes. When researchers do not consider CH variants in genotype-phenotype studies, they may overlook important candidate genes. In turn, overlooking candidate genes may cause the investigator to overlook some samples by assuming that they have no potentially pathogenic variants when, in fact, they do. Although not our focus, this is an important factor to consider when a clinician is trying to understand what genes have contributed to a patient’s disease. Using the genes retained after filtering with 1000GP data, potentially damaging CH variants are observed in samples and genes that HA variants are not identified in. For example, all diseases showed a percent increase in the number of samples with potentially damaging CH variants that did not have potentially damaging HA variants (Table 3). In addition, all diseases experienced a percent increase in the number of genes with potentially damaging CH variants that did not have potentially damaging HA variants (Table 4).
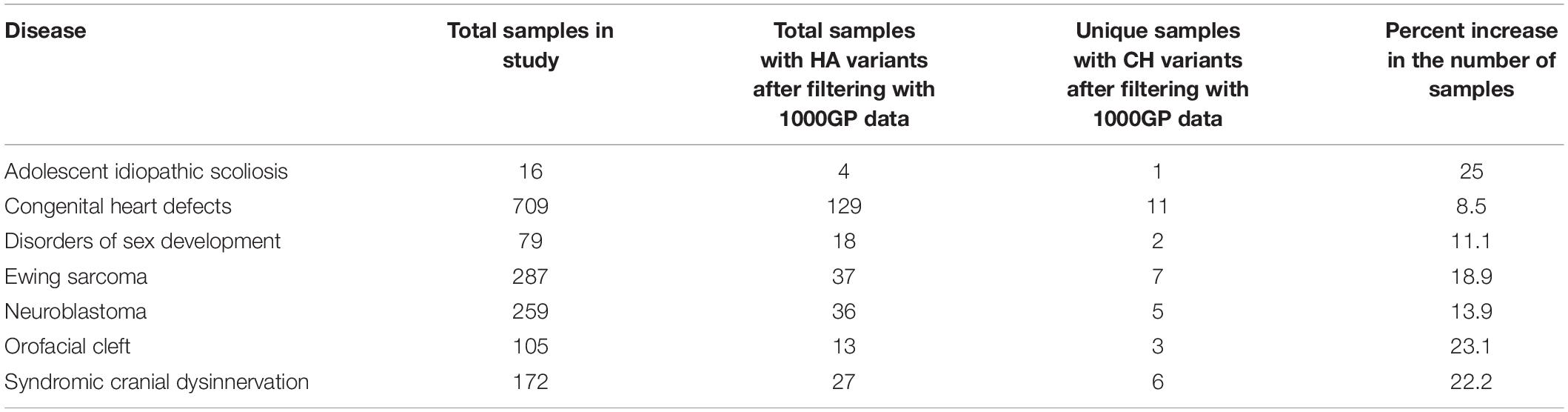
Table 3. The number of samples with potentially damaging HA variants and the number of unique samples (not seen with potentially damaging HA variants) with potentially damaging CH variants after filtering with 1000GP data.
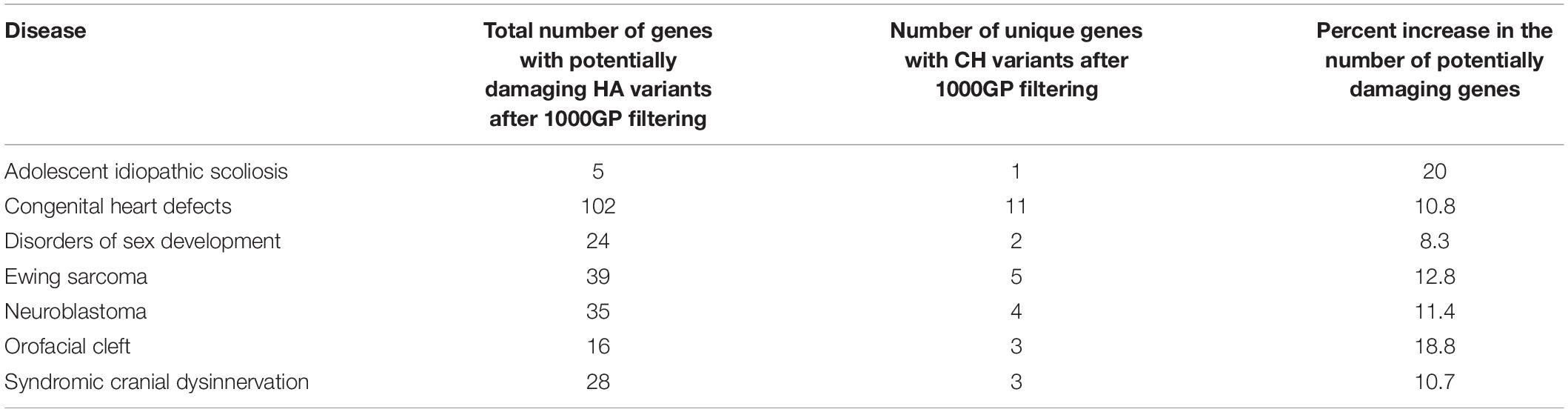
Table 4. The number of genes with potentially damaging HA variants and the number of unique genes (potentially damaging HA variants not identified in gene) with potentially damaging CH variants after filtering with 1000GP data.
Given that non-functional tumor suppressor genes can lead to tumor development and given that pediatric cancers and structural birth defects occur early in a child’s life, we sought to determine whether any of the potentially damaged genes (after 1000GP filtering) occurred in tumor suppressor or developmental biology genes (see section “Materials and Methods”). Congenital heart defects was the only disease where a CH variant was identified in a developmental biology gene (KRTAP4-7: n = 1) (Figures 4, 5). However, KRTAP4-7 and the variants contributing to the CH variant identified in this gene were not associated with congenital heart defects or any of the other diseases in this study based on a GDA or VDA score greater than or equal to 0.5 (Piñero et al., 2020). All diseases except adolescent idiopathic scoliosis and orofacial cleft had at least one sample with a HA variant in a gene known to be involved in developmental biology (Figure 4). No genes associated with tumor suppression were identified for either disease. Variants in the developmental biology genes ACTG1, CCND3, COL9A2, KRTAP4-7, and KRTAP4-8 were seen in more than one disease. However, using DisGeNET, we did not identify any previous gene-disease associations between the developmental biology genes and the disease(s) they were identified in. To expand the list of candidate genes for gene-disease associations, we also analyzed all genes that contained CH variants after 1000GP filtering (Figure 5). These genes and the CH variants identified in these genes also resulted in no GDA or VDA scores greater than or equal to 0.5 for the diseases that they were identified in. Supplementary Table 2 provides a complete list of heterozygous variants that were identified as part of a CH variant, the genes and disease(s) they were identified in, and the number of samples with each heterozygous variant. Supplementary Table 3 provides a complete list of HA variants, the genes and disease(s) they were identified in, and the number of samples with each HA variant.
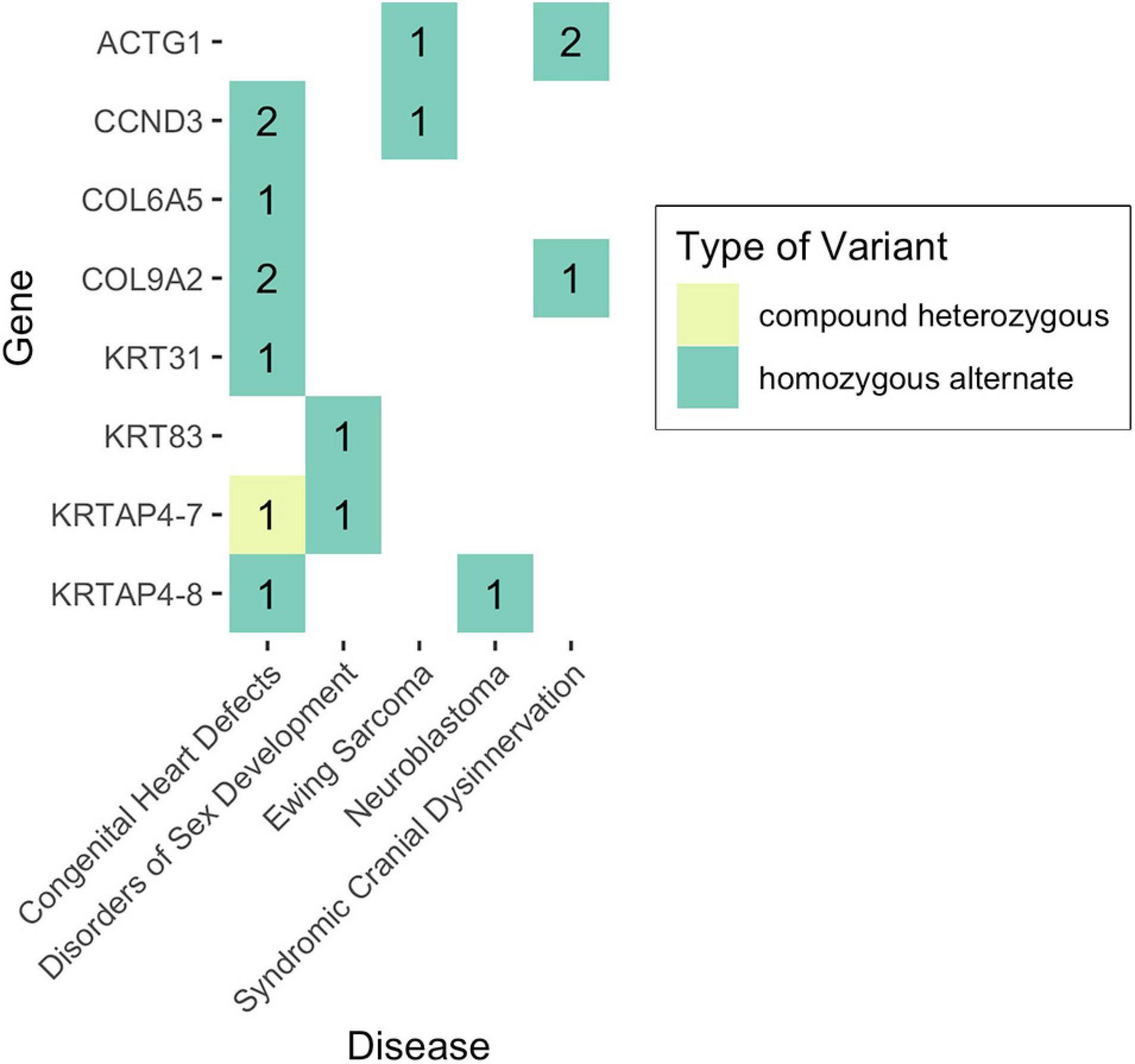
Figure 4. Number of samples with potentially damaging CH or HA variants in genes involved in developmental biology. No potentially damaging CH or HA variants were identified in tumor suppressor genes.
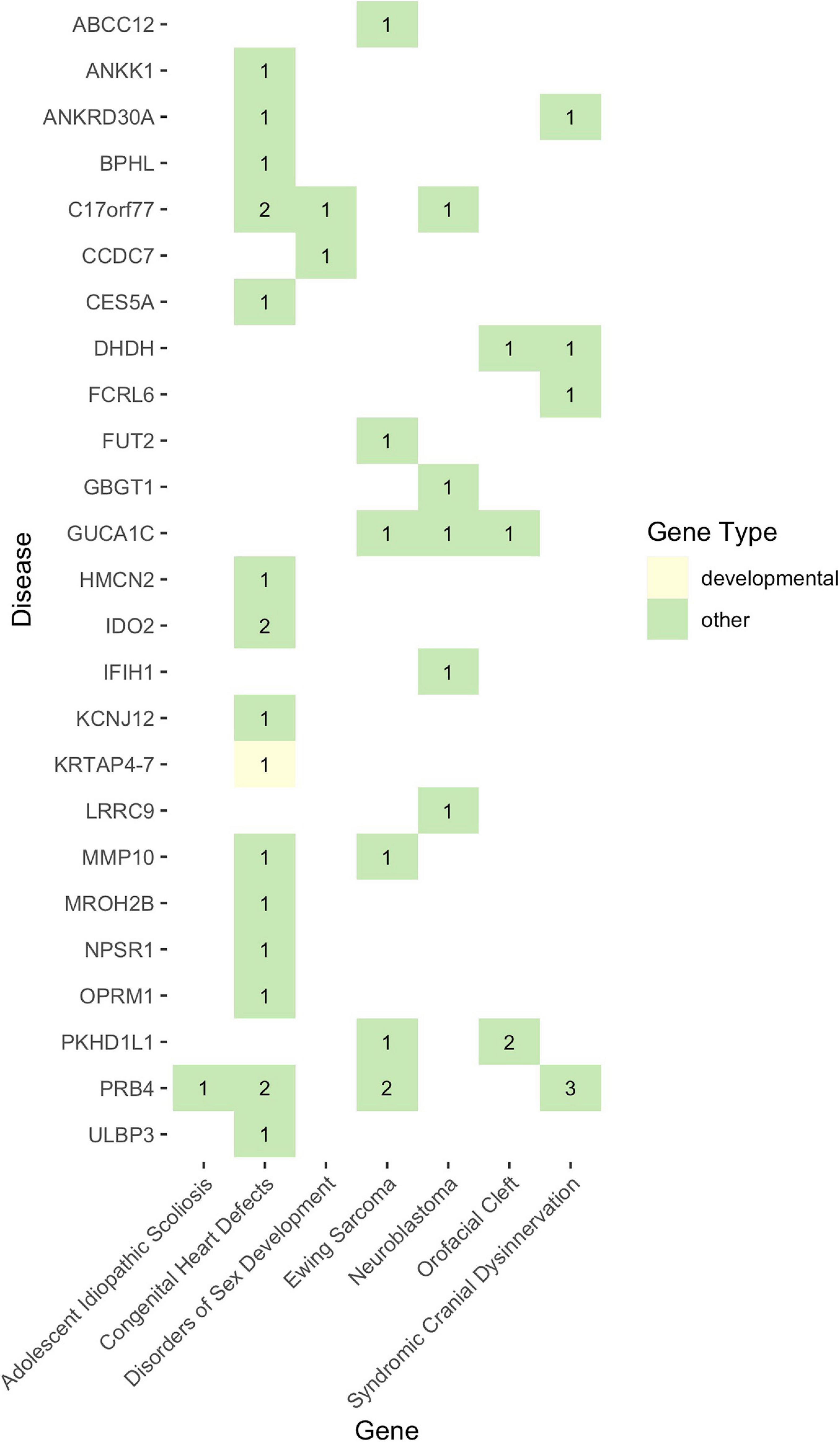
Figure 5. The landscape of potentially damaging CH variants. Each colored-box represents a gene type (see legend). The values within each colored-box indicate how many samples for that disease had a CH variant in that gene.
For each disease, we performed a pathway analysis to determine if any disease pathways were enriched with genes containing potentially damaging CH variants. Using the genes retained after 1000GP filtering of the CH variant gene data, we identified three diseases as having enriched pathways based on an adjusted p-value less than or equal to 0.05 and a q-value less than or equal to 0.2 (Table 5): Ewing sarcoma, neuroblastoma, and orofacial cleft. Each of the enriched pathways, across all diseases, had a single gene identified as being part of the pathway. All three diseases had two pathways in common: the inactivation, recovery and regulation of the phototransduction cascade, and the phototransduction cascade. These two pathways involve the GUCA1C gene. However, no diseases were found to have a strong association with GUCA1C based on a GDA search through DisGeNET. Other genes identified as being part of an enriched pathway included FUT2, MMP10, and IFIH1. These genes are not found in the Cancer Gene Census, nor are they part of a developmental biology pathway. Supplementary Table 4 provides all pathways identified for each disease, regardless of adjusted p- and q-values.
Discussion
The importance of studying CH variants in pediatric diseases has been established, but to date, the number and scope of studies that have focused on CH variants are limited. For example, most studies on CH variants in pediatric cancers have focused on a single disease type, one or a few different gene targets, and have not accounted for background rates of CH variation in diverse, healthy populations (Miller and Piccolo, 2020a). A systematic review showed that since the advent of next-generation sequencing (NGS) approximately 20 years ago, only 10 studies have used NGS to identify CH variants in pediatric cancers (Miller and Piccolo, 2020a). Of these 10 studies, seven used trios and patterns of Mendelian inheritance to infer CH variants (Valentine et al., 2014; Spinella et al., 2015; Diets et al., 2018; Diness et al., 2018; Zhang et al., 2018; Maciaszek et al., 2019; Schieffer et al., 2019), two used computational phasing without the use of trio data (Gröbner et al., 2018; Waszak et al., 2018), and one used patient RNA-seq data to confirm the presence of a single CH variant (Zhang et al., 2015). In total, these 10 prior studies examined 1,279 samples across nine different cancer types, and identified CH variants in 23 unique genes. While no comprehensive review has been published on the role of CH variation in structural birth defects to date, a search on Google Scholar for “‘structural birth defect’ AND ‘compound heterozygous’ AND ‘next generation,”’ limited to the last 20 years, uncovered four studies that used trio data and Mendelian inheritance to infer CH variants (Li et al., 2015, 2020; Takeda et al., 2017; Aarabi et al., 2018), and one study that used computational phasing without trio data (Jiang et al., 2018). These five studies included 1,192 samples across numerous structural birth defect types, and identified CH variants in eight genes likely playing a role in the formation of a defect. The computational pipelines used in these studies were inconsistent with each other, and none took background rates of CH variation in healthy populations into consideration. In this study, we analyzed two pediatric cancer types and five structural birth defect diseases for CH variants across 1,629 affected individuals using a consistent, reproducible, computational pipeline. WGS data for these individuals were phased using trio data and a haplotype reference panel. After accounting for background rates of CH variation in the 1000GP control population, we identified 25 unique genes with at least one potentially damaging CH variant.
During the variant-identification process for both CH and HA variants, we focused on variants in which both alleles were classified as having “HIGH” impact severity and thus were among the most likely to be pathogenic. Across all diseases, we identified high-impact variants that included splice-acceptor, splice-donor, start-loss, stop-gain, stop-loss, and structural-interaction variants. We focused on variants of high impact to help control for false positives. However, by excluding alleles of lower impact (which may have included missense variants, in-frame insertions or deletions, transcription-factor binding site variants, etc.), we likely excluded some true positives. Using a healthy population as a baseline may help reduce the number of false positives, as well as the number of candidate genes that must be considered in genotype-phenotype studies. Therefore, in addition to variant-level filtering, we performed gene-level filtering using 1000GP data. We observed potentially damaging CH variants in 883 out of 2,504 of these seemingly healthy individuals. For each disease that we studied, we identified genes affected with CH variants in more than 1% of 1000GP individuals and excluded these from consideration as candidate disease genes because it is unlikely that these genes are disease-causing, given their prevalence in this seemingly healthy population.
Due to the stringent variant-level and gene-level filtering that we used, this study erred on the side of specificity rather than sensitivity. However, one of our goals was to estimate a lower bound on the number of biallelic gene variants that would be missed or mischaracterized without genome phasing. Future efforts will be necessary to refine the ability to estimate pathogenicity of varying combinations of “HIGH,” “MODERATE,” and “LOW” impact alleles in the context of CH variant identification. Although we expect that the genes presented in this paper as having potentially pathogenic CH variants are among the most likely to play a role in these diseases, we likely missed other genes that should be considered. Among the genes that our filtering process excluded are MUC19 and multiple HLA genes (HLA-DRB5, HLA-B, and HLA-DRB1). Mucin genes are known to be highly variant and HLA genes are among the most polymorphic genes (Akle et al., 2015; Wu et al., 2019).
Across all diseases, our analyses revealed potentially damaging CH variants in genes involved in developmental biology (Figures 4, 5), but no statistically significant gene-disease associations were identified. That is not to say that these genes have no effect on disease development. For example, IFIH1, for which a potentially pathogenic CH variant was identified in a single neuroblastoma patient, has been associated with lupus erythematosus (GDA score: 0.7), Aicardi-Goutieres syndrome (GDA score: 0.7), and Singleton-Merten syndrome (GDA score: 0.69) (Piñero et al., 2020). Of the genes identified in this study, further investigation into their biological role through wet lab experiments, such as gene knockdown, could reveal novel possible mechanisms of disease development.
Phasing is computationally expensive and requires additional analysis steps. However, our analysis shows that it is common to observe CH variants in diverse diseases, thus providing evidence that phasing is justified. Our CH variant identification process enabled us to identify a considerable number of potentially disease-causing variants that would not have been identified without phasing. For example, for the pediatric diseases that we studied, the number of samples with potentially damaging CH variants increased by 8.5–25.0%, and the number of genes in which these variants occurred increased by 8.3–20.0% when compared to assessing HA variants alone (Tables 3, 4). Without phased data, across all studies, 17 genes with potentially damaging variants would have been overlooked and 35 samples would have been overlooked as having potentially damaging variants.
The process that we used to identify CH variants in these cohorts has various limitations. Despite using the best available methods and tools throughout the CH variant identification process, many variant positions were removed during preprocessing steps (Figure 2). Because of this loss, additional high impact alleles may be unaccounted for, so our results may underestimate the number of genes with potentially damaging CH variants for these pediatric diseases. Much of the data loss is due to current limitations of software programs or due to data-quality issues. For example, when converting chromosomal positions from one genome build to another, the exact position must be known in the target genome (Lowy-Gallego et al., 2019). If the position is unknown in the target genome, the position will be excluded in the final output. Another software limitation that leads to data loss occurs during phasing. Phasing relies on several factors, such as the number of high-quality variant calls and completeness of a haplotype reference panel (Choi et al., 2018). Phasing completeness is also not guaranteed when using family information during phasing. For example, if both parents and the child are heterozygous at a position, or inheritance of a variant does not follow Mendelian inheritance patterns, phase may be undetermined due to these ambiguities. Across all diseases, on average, there were 14,130 variant positions per sample removed due to Mendelian inheritance inconsistencies (Figure 2). Despite these limitations, we identified many CH variants that could be explored further.
In addition to the software limitations that are part of the phasing process, phasing can be time consuming and computationally demanding. For example, phasing a single trio can take ∼4 h if only one CPU core is being used. However, using 22 CPU cores simultaneously, we reduced the duration of this process to ∼21 min per trio. Our recently developed program, CompoundHetVIP, can help facilitate the phasing process and assist in CH variant identification; it uses commonly used programs such as SHAPEIT2 (for phasing) and GEMINI (to assist with CH variant identification) (Delaneau et al., 2013; Paila et al., 2013; Miller and Piccolo, 2020b). CompoundHetVIP requires minimal command-line experience. This is one example in which phasing and CH variant identification is becoming more easily accessible to researchers of all computational skill levels.
Conclusion
Although much insight has been gained from recent studies focusing on the role of germline variants in pediatric cancer and structural birth defect diseases, many studies overlook CH variants because identification of these variants requires sequencing data from parents as well as additional time and computational resources. However, embracing these limitations is worth the additional knowledge that is gained when studying diseases that are putatively inherited in a recessive manner. Using trio data from the GMKF, across seven pediatric diseases with unknown etiologies, we showed that the number of samples and genes with potentially damaging variants increases when compared to the number of samples and genes with HA variants, alone. We used 1000GP data as a baseline of what to expect in terms of CH variation across a genome and then used this information as a filter to reduce the number of candidate genes with potentially damaging CH variants in each disease dataset. Across all seven diseases, we observed 17 genes with potentially damaging CH variants that would have been overlooked if CH variants were not considered. In addition to research applications, the identification of CH variants may be beneficial to clinicians when trying to understand what genes may be contributing to a patient’s disease.
Data Availability Statement
Publicly available datasets were analyzed in this study. This data can be found here: Data were downloaded from https://kidsfirstdrc.org/after gaining access through dbGaP. Information on data access requests can be found for each disease as follows: Congenital Heart Data (phs001194.v2.p2); https://www.ncbi.nlm.nih.gov/projects/gap/cgi-bin/study.cgi?study_id=phs001194.v2.p2, Neuroblastoma Data (phs001436.v1.p1); https://www.ncbi.nlm.nih.gov/projects/gap/cgi-bin/study.cgi?study_id=phs001436.v1.p1, Cranial dysinnervation (phs001247.v1.p1); https://www.ncbi.nlm.nih.gov/projects/gap/cgi-bin/study.cgi?study_id=phs001247.v1.p1, Orofacial Cleft Data (phs001168.v2.p2); https://www.ncbi.nlm.nih.gov/projects/gap/cgi-bin/study.cgi?study_id=phs001168.v2.p2, Idiopathic Scoliosis Data (phs001410.v1.p1); https://www.ncbi.nlm.nih.gov/projects/gap/cgi-bin/study.cgi?study_id=phs001410.v1.p1, Disorders of Sex Development Data (phs001178.v1.p1); https://www.ncbi.nlm.nih.gov/projects/gap/cgi-bin/study.cgi?study_id=phs001178.v1.p1, and Ewing Sarcoma Data (phs001228.v1.p1); https://www.ncbi.nlm.nih.gov/projects/gap/cgi-bin/study.cgi?study_id=phs001228.v1.p1.
Ethics Statement
Ethical review and approval was not required for the study on human participants in accordance with the local legislation and institutional requirements. Written informed consent from the participants’ legal guardian/next of kin was not required to participate in this study in accordance with the national legislation and the institutional requirements.
Author Contributions
DM wrote the data processing scripts, processed the data, performed critical analysis, and wrote the manuscript. SP oversaw the project, and provided edits and critical feedback. Both authors contributed to the article and approved the submitted version.
Conflict of Interest
The authors declare that the research was conducted in the absence of any commercial or financial relationships that could be construed as a potential conflict of interest.
Acknowledgments
The results analyzed and published here are based in whole or in part upon data generated by the Gabriella Miller Kids First Pediatric Research Program projects phs001436, phs001138, phs001168, phs001228, phs001247, phs001410, phs001178, and were accessed from the Kids First Data Resource Portal (kidsfirstdrc.org and/or dbGaP; www.ncbi.nlm.nih.gov/gap).
Supplementary Material
The Supplementary Material for this article can be found online at: https://www.frontiersin.org/articles/10.3389/fgene.2021.640242/full#supplementary-material
Footnote
References
1000 Genomes Project Consortium, Auton, A., Brooks, L. D., Durbin, R. M., Garrison, E. P., Kang, H. M., et al. (2015). A global reference for human genetic variation. Nature 526, 68–74. doi: 10.1038/nature15393
Aarabi, M., Sniezek, O., Jiang, H., Saller, D. N., Bellissimo, D., Yatsenko, S. A., et al. (2018). Importance of complete phenotyping in prenatal whole exome sequencing. Hum. Genet. 137, 175–181. doi: 10.1007/s00439-017-1860-1
Akle, S., Chun, S., Jordan, D. M., and Cassa, C. A. (2015). Mitigating false-positive associations in rare disease gene discovery. Hum. Mutat. 36, 998–1003. doi: 10.1002/humu.22847
American Cancer Society (2020). Key Statistics for Childhood Cancers. Atlanta, GA: American Cancer Society.
Beaty, T. H., Marazita, M. L., and Leslie, E. J. (2016). Genetic factors influencing risk to orofacial clefts: today’s challenges and tomorrow’s opportunities. F1000Res 5:2800. doi: 10.12688/f1000research.9503.1
Benjamini, Y., and Hochberg, Y. (1995). Controlling the false discovery rate: a practical and powerful approach to multiple testing. J. R. Stat. Soc. Series B Stat. Methodol. 57, 289–300. doi: 10.1111/j.2517-6161.1995.tb02031.x
Brohl, A. S., Patidar, R., Turner, C. E., Wen, X., Song, Y. K., Wei, J. S., et al. (2017). Frequent inactivating germline mutations in DNA repair genes in patients with Ewing sarcoma. Genet. Med. 19, 955–958. doi: 10.1038/gim.2016.206
Browning, S. R., and Browning, B. L. (2007). Rapid and accurate haplotype phasing and missing-data inference for whole-genome association studies by use of localized haplotype clustering. Am. J. Hum. Genet. 81, 1084–1097. doi: 10.1086/521987
Chang, C. C., Chow, C. C., Tellier, L. C., Vattikuti, S., Purcell, S. M., and Lee, J. J. (2015). Second-generation PLINK: rising to the challenge of larger and richer datasets. Gigascience 4:7. doi: 10.1186/s13742-015-0047-8
Choi, Y., Chan, A. P., Kirkness, E., Telenti, A., and Schork, N. J. (2018). Comparison of phasing strategies for whole human genomes. PLoS Genet. 14:e1007308. doi: 10.1371/journal.pgen.1007308
Cingolani, P., Platts, A., Wang, L. L., Coon, M., Nguyen, T., Wang, L., et al. (2012). A program for annotating and predicting the effects of single nucleotide polymorphisms, SnpEff: SNPs in the genome of Drosophila melanogaster strain w1118; iso-2; iso-3. Fly 6, 80–92. doi: 10.4161/fly.19695
Danecek, P., Auton, A., Abecasis, G., Albers, C. A., Banks, E., DePristo, M. A., et al. (2011). The variant call format and VCFtools. Bioinformatics 27, 2156–2158. doi: 10.1093/bioinformatics/btr330
Delaneau, O., Howie, B., Cox, A. J., Zagury, J.-F., and Marchini, J. (2013). Haplotype estimation using sequencing reads. Am. J. Hum. Genet. 93, 687–696. doi: 10.1016/j.ajhg.2013.09.002
Diets, I. J., Waanders, E., Ligtenberg, M. J., van Bladel, D. A. G., Kamping, E. J., Hoogerbrugge, P. M., et al. (2018). High yield of pathogenic germline mutations causative or likely causative of the cancer phenotype in selected children with cancer. Clin. Cancer Res. 24, 1594–1603. doi: 10.1158/1078-0432.CCR-17-1725
Diness, B. R., Risom, L., Frandsen, T. L., Hansen, B., Andersen, M. K., Schmiegelow, K., et al. (2018). Putative new childhood leukemia cancer predisposition syndrome caused by germline bi-allelic missense mutations in DDX41. Genes Chromosomes Cancer 57, 670–674. doi: 10.1002/gcc.22680
Eggers, S., Sadedin, S., van den Bergen, J. A., Robevska, G., Ohnesorg, T., Hewitt, J., et al. (2016). Disorders of sex development: insights from targeted gene sequencing of a large international patient cohort. Genome Biol. 17:243. doi: 10.1186/s13059-016-1105-y
Fu, F., Li, R., Lei, T.-Y., Wang, D., Yang, X., Han, J., et al. (2020). Compound heterozygous mutation of the ASXL3 gene causes autosomal recessive congenital heart disease. Hum. Genet. 140, 333–348. doi: 10.1007/s00439-020-02200-z
Gabriella Miller Kids First Pediatric Research Program (2019). National Institutes of Health Office of Strategic Coordination – The Common Fund. Available online at: https://commonfund.nih.gov/kidsfirst (accessed March 26, 2019).
Grauers, A., Einarsdottir, E., and Gerdhem, P. (2016). Genetics and pathogenesis of idiopathic scoliosis. Scoliosis Spinal Disord 11:45. doi: 10.1186/s13013-016-0105-8
Gröbner, S. N., Worst, B. C., Weischenfeldt, J., Buchhalter, I., Kleinheinz, K., Rudneva, V. A., et al. (2018). The landscape of genomic alterations across childhood cancers. Nature 555, 321–327. doi: 10.1038/nature25480
Heath, A. P., Taylor, D. M., Zhu, Y., Raman, P., Lilly, J., Storm, P., et al. (2019). Abstract 2464: Gabriella Miller Kids First Data Resource Center: harmonizing clinical and genomic data to support childhood cancer and structural birth defect research. Cancer Res. 79, 2464–2464. doi: 10.1158/1538-7445.AM2019-2464
Jassal, B., Matthews, L., Viteri, G., Gong, C., Lorente, P., Fabregat, A., et al. (2020). The reactome pathway knowledgebase. Nucleic Acids Res. 48, D498–D503. doi: 10.1093/nar/gkz1031
Jiang, T., Huang, M., Jiang, T., Gu, Y., Wang, Y., Wu, Y., et al. (2018). Genome-wide compound heterozygosity analysis highlighted 4 novel susceptibility loci for congenital heart disease in Chinese population. Clin. Genet. 94, 296–302. doi: 10.1111/cge.13384
Karczewski, K. J., Francioli, L. C., Tiao, G., Cummings, B. B., Alföldi, J., Wang, Q., et al. (2020). The mutational constraint spectrum quantified from variation in 141,456 humans. Nature 581, 434–443. doi: 10.1038/s41586-020-2308-7
Li, H. (2011). A statistical framework for SNP calling, mutation discovery, association mapping and population genetical parameter estimation from sequencing data. Bioinformatics 27, 2987–2993. doi: 10.1093/bioinformatics/btr509
Li, N., Zhou, P., Tang, H., He, L., Fang, X., Zhao, J., et al. (2020). In-depth analysis reveals complex molecular etiology of idiopathic cerebral palsy. bioRxiv [Preprint]. doi: 10.1101/2020.08.17.255158
Li, Y., Garrod, A. S., Madan-Khetarpal, S., Sreedher, G., McGuire, M., Yagi, H., et al. (2015). Respiratory motile cilia dysfunction in a patient with cranioectodermal dysplasia. Am. J. Med. Genet. A 167A, 2188–2196. doi: 10.1002/ajmg.a.37133
Lowy-Gallego, E., Fairley, S., Zheng-Bradley, X., Ruffier, M., Clarke, L., Flicek, P., et al. (2019). Variant calling on the GRCh38 assembly with the data from phase three of the 1000 Genomes Project. Wellcome Open Res. 4:50. doi: 10.12688/wellcomeopenres.15126.2
Lupo, P. J., Mitchell, L. E., and Jenkins, M. M. (2019). Genome-wide association studies of structural birth defects: a review and commentary. Birth Defects Res. 111, 1329–1342. doi: 10.1002/bdr2.1606
Maciaszek, J. L., Oak, N., Chen, W., Hamilton, K. V., McGee, R. B., Nuccio, R., et al. (2019). Enrichment of heterozygous germline RECQL4 loss-of-function variants in pediatric osteosarcoma. Cold Spring Harb. Mol. Case Stud. 5:a004218. doi: 10.1101/mcs.a004218
Miller, D. B., and Piccolo, S. R. (2020a). Compound heterozygous variants in pediatric cancers: a systematic review. Front. Genet. 11:493. doi: 10.3389/fgene.2020.00493
Miller, D. B., and Piccolo, S. R. (2020b). CompoundHetVIP: compound heterozygous variant identification pipeline. F1000Res 9:1211. doi: 10.12688/f1000research.26848.1
Morrill, S. A., and Amon, A. (2019). Why haploinsufficiency persists. Proc. Natl. Acad. Sci. U. S. A. 116, 11866–11871. doi: 10.1073/pnas.1900437116
Paila, U., Chapman, B. A., Kirchner, R., and Quinlan, A. R. (2013). GEMINI: integrative exploration of genetic variation and genome annotations. PLoS Comput. Biol. 9:e1003153. doi: 10.1371/journal.pcbi.1003153
Picard Tools (2019). Broad Institute, GitHub Repository. Available online at: http://broadinstitute.github.io/picard/ (accessed April 17, 2020).
Pierpont, M. E., Brueckner, M., Chung, W. K., Garg, V., Lacro, R. V., McGuire, A. L., et al. (2018). Genetic basis for congenital heart disease: revisited: a scientific statement from the American Heart Association. Circulation 138, e653–e711. doi: 10.1161/CIR.0000000000000606
Piñero, J., Ramírez-Anguita, J. M., Saüch-Pitarch, J., Ronzano, F., Centeno, E., Sanz, F., et al. (2020). The DisGeNET knowledge platform for disease genomics: 2019 update. Nucleic Acids Res. 48, D845–D855. doi: 10.1093/nar/gkz1021
Poplin, R., Ruano-Rubio, V., DePristo, M. A., Fennell, T. J., Carneiro, M. O., Van der Auwera, G. A., et al. (2017). Scaling accurate genetic variant discovery to tens of thousands of samples. bioRxiv [Preprint]. doi: 10.1101/201178
Purcell, S., and Chang, C. (2020). PLINK 2.0. Available online at: www.cog-genomics.org/plink/2.0/ (accessed October 10, 2020).
R Core Team (2020). R: A Language and Environment for Statistical Computing. Vienna Available online at: www.R-project.org/ (accessed October 10, 2020).
Reimand, J., Isserlin, R., Voisin, V., Kucera, M., Tannus-Lopes, C., Rostamianfar, A., et al. (2019). Pathway enrichment analysis and visualization of omics data using g:Profiler, GSEA, Cytoscape and EnrichmentMap. Nat. Protoc. 14, 482–517. doi: 10.1038/s41596-018-0103-9
Rentzsch, P., Witten, D., Cooper, G. M., Shendure, J., and Kircher, M. (2019). CADD: predicting the deleteriousness of variants throughout the human genome. Nucleic Acids Res. 47, D886–D894. doi: 10.1093/nar/gky1016
Sanjak, J. S., Long, A. D., and Thornton, K. R. (2017). A model of compound heterozygous, loss-of-function alleles is broadly consistent with observations from complex-disease GWAS datasets. PLoS Genet. 13:e1006573. doi: 10.1371/journal.pgen.1006573
Schieffer, K. M., Varga, E., Miller, K. E., Agarwal, V., Koboldt, D. C., Brennan, P., et al. (2019). Expanding the clinical history associated with syndromic Klippel-Feil: a unique case of comorbidity with medulloblastoma. Eur. J. Med. Genet. 62:103701. doi: 10.1016/j.ejmg.2019.103701
Schneider, V. A., Graves-Lindsay, T., Howe, K., Bouk, N., Chen, H.-C., Kitts, P. A., et al. (2016). Evaluation of GRCh38 and de novo haploid genome assemblies demonstrates the enduring quality of the reference assembly. Genome Res. 27, 849–864. doi: 10.1101/072116
Singh, A., Pandey, P. K., Agrawal, A., Mittal, S. K., Rana, K. M., and Bahuguna, C. (2017). Congenital cranial dysinnervation disorders. Int. Ophthalmol. 37, 1369–1381. doi: 10.1007/s10792-016-0388-z
Sondka, Z., Bamford, S., Cole, C. G., Ward, S. A., Dunham, I., and Forbes, S. A. (2018). The COSMIC Cancer Gene Census: describing genetic dysfunction across all human cancers. Nat. Rev. Cancer 18, 696–705. doi: 10.1038/s41568-018-0060-1
Spinella, J.-F., Healy, J., Saillour, V., Richer, C., Cassart, P., Ouimet, M., et al. (2015). Whole-exome sequencing of a rare case of familial childhood acute lymphoblastic leukemia reveals putative predisposing mutations in Fanconi anemia genes. BMC Cancer 15:539. doi: 10.1186/s12885-015-1549-6
Takeda, K., Kou, I., Kawakami, N., Iida, A., Nakajima, M., Ogura, Y., et al. (2017). Compound heterozygosity for null mutations and a common hypomorphic risk haplotype in TBX6 causes congenital scoliosis. Hum. Mutat. 38, 317–323. doi: 10.1002/humu.23168
Tan, A., Abecasis, G. R., and Kang, H. M. (2015). Unified representation of genetic variants. Bioinformatics 31, 2202–2204. doi: 10.1093/bioinformatics/btv112
Tolbert, V. P., Coggins, G. E., and Maris, J. M. (2017). Genetic susceptibility to neuroblastoma. Curr. Opin. Genet. Dev. 42, 81–90. doi: 10.1016/j.gde.2017.03.008
Valentine, M. C., Linabery, A. M., Chasnoff, S., Hughes, A. E. O., Mallaney, C., Sanchez, N., et al. (2014). Excess congenital non-synonymous variation in leukemia-associated genes in MLL- infant leukemia: a Children’s Oncology Group report. Leukemia 28, 1235–1241.
Wang, L.-H., Wu, C.-F., Rajasekaran, N., and Shin, Y. K. (2018). Loss of tumor suppressor gene function in human cancer: an overview. Cell. Physiol. Biochem. 51, 2647–2693.
Waszak, S. M., Northcott, P. A., Buchhalter, I., Robinson, G. W., Sutter, C., Groebner, S., et al. (2018). Spectrum and prevalence of genetic predisposition in medulloblastoma: a retrospective genetic study and prospective validation in a clinical trial cohort. Lancet Oncol. 19, 785–798.
Webber, D. M., MacLeod, S. L., Bamshad, M. J., Shaw, G. M., Finnell, R. H., Shete, S. S., et al. (2015). Developments in our understanding of the genetic basis of birth defects. Birth Defects Res. A Clin. Mol. Teratol. 103, 680–691.
Wickham, H., Averick, M., Bryan, J., Chang, W., François, R., Grolemund, G., et al. (2019). Welcome to the Tidyverse. J. Open Source Softw. 4:1686.
Wu, R., Li, H., Peng, D., Li, R., Zhang, Y., Hao, B., et al. (2019). Revisiting the potential power of human leukocyte antigen (HLA) genes on relationship testing by massively parallel sequencing-based HLA typing in an extended family. J. Hum. Genet. 64, 29–38.
Yu, G., and He, Q.-Y. (2016). ReactomePA: an R/Bioconductor package for reactome pathway analysis and visualization. Mol. Biosyst. 12, 477–479.
Zhang, J., Walsh, M. F., Wu, G., Edmonson, M. N., Gruber, T. A., Easton, J., et al. (2015). Germline mutations in predisposition genes in pediatric cancer. N. Engl. J. Med. 373, 2336–2346.
Keywords: pediatric cancer, structural birth defect, germline variants, compound heterozygous variants, genetic analysis of complex diseases, trios
Citation: Miller DB and Piccolo SR (2021) A Survey of Compound Heterozygous Variants in Pediatric Cancers and Structural Birth Defects. Front. Genet. 12:640242. doi: 10.3389/fgene.2021.640242
Received: 10 December 2020; Accepted: 24 February 2021;
Published: 22 March 2021.
Edited by:
Charlotte L. Alston, Wellcome Trust Centre for Mitochondrial Research (WT), United KingdomReviewed by:
Bao Chi Bui, Vietnam National University, VietnamAline L. Petrin, The University of Iowa, United States
Copyright © 2021 Miller and Piccolo. This is an open-access article distributed under the terms of the Creative Commons Attribution License (CC BY). The use, distribution or reproduction in other forums is permitted, provided the original author(s) and the copyright owner(s) are credited and that the original publication in this journal is cited, in accordance with accepted academic practice. No use, distribution or reproduction is permitted which does not comply with these terms.
*Correspondence: Stephen R. Piccolo, c3RlcGhlbl9waWNjb2xvQGJ5dS5lZHU=