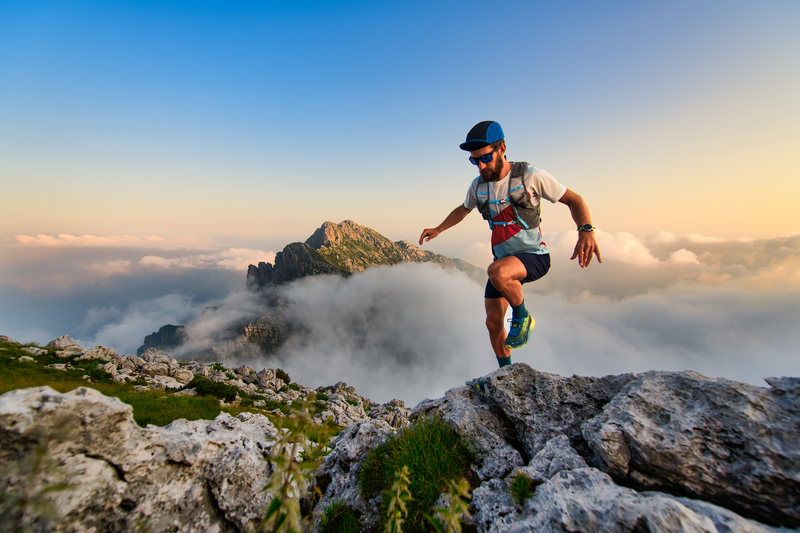
94% of researchers rate our articles as excellent or good
Learn more about the work of our research integrity team to safeguard the quality of each article we publish.
Find out more
ORIGINAL RESEARCH article
Front. Genet. , 23 February 2021
Sec. Systems Biology Archive
Volume 12 - 2021 | https://doi.org/10.3389/fgene.2021.633963
This article is part of the Research Topic Dynamics and Complexity of Molecular Networks Controlling Cell Fate Decisions View all 17 articles
The highly controlled migration of neutrophils toward the site of an infection can be altered when they are trained with lipopolysaccharides (LPS), with high dose LPS enhancing neutrophil migratory pattern toward the bacterial derived source signal and super-low dose LPS inducing either migration toward an intermediary signal or dysregulation and oscillatory movement. Empirical studies that use microfluidic chemotaxis-chip devices with two opposing chemoattractants showed differential neutrophil migration after challenge with different LPS doses. The epigenetic alterations responsible for changes in neutrophil migratory behavior are unknown. We developed two mathematical models that evaluate the mechanistic interactions responsible for neutrophil migratory decision-making when exposed to competing chemoattractants and challenged with LPS. The first model, which considers the interactions between the receptor densities of two competing chemoattractants, their kinases, and LPS, displayed bistability between high and low ratios of primary to intermediary chemoattractant receptor densities. In particular, at equilibrium, we observe equal receptor densities for low LPS (< 15ng/mL); and dominance of receptors for the primary chemoattractant for high LPS (> 15ng/mL). The second model, which included additional interactions with an extracellular signal-regulated kinase in both phosphorylated and non-phosphorylated forms, has an additional dynamic outcome, oscillatory dynamics for both receptors, as seen in the data. In particular, it found equal receptor densities in the absence of oscillation for super-low and high LPS challenge (< 0.4 and 1.1 <LPS< 375 ng/mL); equal receptor densities with oscillatory receptor dynamics for super-low LPS (0.5 < LPS< 1.1ng/mL); and dominance of receptors for the primary chemoattractant for super-high LPS (>376 ng/mL). Predicting the mechanisms and the type of external LPS challenge responsible for neutrophils migration toward pro-inflammatory chemoattractants, migration toward pro-tolerant chemoattractants, or oscillatory movement is necessary knowledge in designing interventions against immune diseases, such as sepsis.
Researchers have recently challenged the dogma that innate immunity is the same at every challenge. It has been shown that macrophages are able to develop different kinds of memory depending on the type of priming they encounter via epigenetic reprogramming (Yuan et al., 2016a,b). For instance, they can develop a memory phenotype that leads them to be less reactive or even tolerant to a challenge, or they can develop a memory phenotype that leads them to have an enhanced response to a challenge. This same concept has recently been shown by us for neutrophil migratory decision-making, and it is thought that the response is influencing the outcomes of infectious diseases. For example, in sepsis or COVID-19 infection, the immune system overreacts because of underlying low-grade inflammation that primes neutrophils into choosing between tolerant and inflammatory migratory phenotypes (Alves-Filho et al., 2005, 2010). As a result, neutrophils can migrate to healthy organs and unleash their anti-microbial arsenal in healthy tissue, leading to organ failure in the lungs, kidney, or heart. The mechanisms underlying trained innate immunity have not been fully elucidated, with epigenetic modifications playing a key role in the induction of innate memory or training (Pillay et al., 2010; Demaret et al., 2015). In this study we investigate innate memory in the context of neutropil migratory decision-making.
The ability of neutrophils to migrate plays a pivotal role in a cell's ability to clear infections and resolve inflammation. During infection and inflammation, chemoattractants are released, signaling and activating neutrophils in the bloodstream. Neutrophils must be able to precisely migrate within the tissue to the specific site of infection, without being diverted toward other locations, in a process called chemotaxis. Chemotaxis is a highly regulated process that involves activation of various pathways and downstream polarization of the cell (Kolaczkowska and Kubes, 2013). The first step in chemotaxis is recognition of chemoattractants by the cell. Cells have specific receptors on their surface for various chemoattractants. These chemoattractant receptors are G protein-coupled receptors (GPCRs), which are regulated by a variety of G protein-coupled receptor kinases (GRKs) (Murphy, 1994; Dianqing, 2005). When bound by a specific agonist, in this case a chemoattractant, the GPCRs undergo phosphorylation, which unbinds the G proteins and desensitizes the receptor. This leads to internalization of the receptor, activation of downstream signaling pathways, and activation of cellular responses, such as cell polarization and chemotaxis (Murphy, 1994; Dianqing, 2005; Futosi et al., 2013). After internalization, receptors can be recycled back to the cell surface, where they can again be bound by the receptor's agonist. This process is crucial in chemotaxis, as it allows the cell to continue sensing the chemoattractant and migrate in its direction (Neel et al., 2005). Most chemoattractant receptors are similar in their response to ligand-binding; however there are slight differences in the activated signaling pathways (Heit et al., 2002, 2008). Within the tissue, neutrophils are exposed to several chemoattractants at once, originating from pathogens, cells within the tissue, the endothelium, and several other sources (Kolaczkowska and Kubes, 2013). Cells must prioritize these signals to properly clear the pathogen. It has been hypothesized that neutrophils have an internal hierarchy, where chemoattractants derived from bacterial sources and the complement system, such as fMLP and C5a (Heit et al., 2002; Petri and Sanz, 2018), take precedent over intermediary chemoattractants, such as LTB4 and IL-8, which are secreted by other immune cells. This leads to neutrophils migrating toward end-target chemoattractants over intermediary chemottractants in a competitive environment (Heit et al., 2002, 2008; Wang et al., 2016b), allowing neutrophils to prioritize an invading pathogen. This hierarchy is thought to occur through the activation of differing signaling pathways, where end-target chemoattractants signal through p38 MAPK and intermediary chemoattractants signal through PI3K (Heit et al., 2002, 2008).
The highly controlled migration of neutrophils toward the site of an infection, as well as their dynamic interaction with pathogens, can be altered when they are pre-conditioned with Lipopolysaccharides (LPS) to induce endotoxin priming. In previous work, we showed that training with high dose LPS (100 ng/mL) enhances neutrophil migration toward the end-target, bacterial derived, source signal fMLP. By contrast, training with super-low dose LPS (1 ng/mL) alters neutrophil migratory phenotypes, which either migrate toward the intermediary signal LTB4 or become dysregulated and exhibit oscillatory migratory patterns (Jones et al., 2016; Boribong et al., 2019). While the empirical data shows that neutrophils trained with LPS change migratory phenotype, it does not give information on the molecular mechanisms responsible for the difference in behavior. The migratory decision-making process is finely governed by complex signaling networks that dynamically receive and interpret molecular and cellular signals from outside and within. The intrinsic complexity of immune cell decision-making processes has created difficulty for experimental immunologists to determine the mechanisms of disease, in spite of expansive experimental studies with conventional reductionist cellular and molecular approaches. It is increasingly recognized that cross-disciplinary studies combining experimental and mathematical modeling approaches are critically required.
In this study, we investigate the molecular mechanisms of neutrophil migratory decision-making in the presence of competing chemoattractants and external challenge with LPS, by building deterministic mathematical models of interaction between two chemoattractant receptors, Formyl Peptide Receptor 1 (FPR1) and Leukotriene B4 Receptor 1 (BLT1), and key molecules involved in their regulation. We are interested in determining the relationship between the receptor dynamics and migration pattern, and in quantifying the LPS dose resulting in neutrophils migration toward a pro-inflammatory chemoattractant, toward a pro-resolution chemoattractant, or in neutrophils dysregulation and oscillation (Fan and Malik, 2003; Liu et al., 2012; Byrne et al., 2014). The model will qualitatively match the experimental results of our previous work, where stimulation with a super-low concentration of LPS will result in greater BLT1 over FPR1, and stimulation with a high concentration of LPS will result in greater FPR1 over BLT1 (Boribong et al., 2019). We construct a model with bistable behavior, with the motif for bistability coming from the non-linear mutual inhibition of GRK2 and GRK5 (see Figure 1). The dual inhibition leads to the activation of different signaling pathways (p38/JNK vs. ERK), leading to differences in functional neutrophil migration (Davenport et al., 2020). Both GRK2 and GRK5 have been demonstrated to be critical mediators of the molecular alterations that occur in the inflammatory disorders, but the complex mutual inhibition interaction has largely been ignored (Philipp et al., 2014). Mathematical models have been used before to model cellular decision-making (Day et al., 2006; Kadelka et al., 2019), neutrophil chemotaxis (Ionides et al., 2004; Postma and van Haastert, 2016; Bayani et al., 2020), immune responses (Reynolds et al., 2006; Fischer, 2008; Nelson et al., 2009; Vodovotz et al., 2009) and bistable dynamics (Ciupe et al., 2007, 2018; Leber et al., 2016).
We developed a novel system of differential equations based on diagram in Figure 2, which describes the interactions between [LPS], kinases [GRK2] and [GRK5], the receptor for end-target chemoattractant fMLP, [FPR1], and the receptor for intermediary chemoattractant LTB4, [BLT1]. Priming by LPS occurs through activation of both GRK2 and GRK5 (Prossnitz et al., 1995; Arraes et al., 2006; Sorriento et al., 2008; Wang et al., 2016a). For simplicity, we model linear effects of LPS on the kinases' activity. In particular, we assume that the GRK2 activation occurs at rate cw+aw[LPS], with cw and aw being the LPS-independent and LPS-dependent activation rates. Similarly, GRK5 activation occurs at rate cf+af[LPS], with cf and af being the LPS-independent and LPS-dependent activation rates. The two kinases mutually inhibit one another. We model inhibition of GRK2 via GRK5 at rate and inhibition of GRK5 via GRK2 at rate 1/(bwf+[GRK2]), where bfw and bwf are the mutual inhibition rates of GRK2 by GRK5 and GRK5 by GRK1, respectively. n is the cooperativity coefficient. We assumed increased cooperativity in GRK2 inhibition by GRK5, but not the inhibition of GRK5 by GRK2. The results are preserved if the same cooperativity is included in the GRK5 inhibition by GRK2 (not shown). We assume GRK2 and GRK5 decay at per capita rates dw and df, respectively, with GRK5 decay being modeled in a density dependent manner, with the GRK5 value where the decay is half-maximal being given by parameter bf.
The chemoattractant receptors FPR1 and BLT1 internalize from the plasma membrane into the cell via phosphorylation (Magalhaes et al., 2012; Mócsai et al., 2015). We assume that the number of receptors on a cell is conserved and, through the process of dephosphorylation, the receptors are recycled and brought back to the surface of the cell. Thus, we have conservation laws of the total number of the receptor equalling the sum of the non-phosphrylated and phosphorylated receptor, [FPR1]total = [FPR1]+[FPR1p] and [BLT1]total = [BLT1]+[BLT1p]. The process of receptor phosphorylation and dephosphorylation is modeled using Hill-type functions. In particular, FPR1 is produced through dephosphorylation, modeled by a Michaelis-Menten term a1([FPR1]total − [FPR1])/(JF1+[FPR1]total − [FPR1]), where a1 is maximal production and JF1 is the receptor quantity where dephosphorylation is half-maximal. Similarly, FPR1 is lost through phosphorylation, which is enhanced in the presence of GRK2 (Wang et al., 2016a). We model this by a Hill-type function a2[FPR1][GRK2]/(JF2+[FPR1]), where a2 is the maximal rate and JF2 is the receptor quantity where phosphorylation is half-maximal.
BLT1 is produced through dephosphorylation, modeled by a Michaelis-Menten term b1([BLT1]total − [BLT1])/(JB1+[BLT1]total − [BLT1]), where b1 is the maximal production rate and JB1 is the receptor quantity where dephosphorylation is half-maximal. BLT1 is lost through phosphorylation, which is enhanced in the presence of both GRK2 and GRK5 (Gaudreau et al., 2002; Chen et al., 2004). We model this by a Hill-type function [BLT1](b2[GRK2]+b3[GRK5])/(JB2+[BLT1]), where b2 are b3 are maximal decay rates and JB2 is the receptor quantity where phosphorylation is half-maximal. We assume a single LPS dose, after which LPS decays exponentially at a rate dL (Kadelka et al., 2019). The dynamical system describing these interactions is given by:
We are interested in determining the ratio between the cells that migrate toward the primary and those that migrate toward the intermediary chemoattractants given, as a proxy, by the ratio of their receptors FPR1/BLT1, when initial LPS is varied.
In previous research, we used a microfluidic competitive chemotaxis-chip device to measure the migratory decision-making process of dHL-60 cells, a model neutrophil cell line, 5 h after they were pre-challenged with super-low-dose (1 ng/mL) and high-dose (100 ng/mL) of LPS in the presence of two competing chemoattractants, LTB4 and fMLP (Boribong et al., 2019). Challenging the cells with a super-low dose of LPS resulted in fMLP/LTB4 ratio of 0.8672. Challenging the cells with a high dose of LPS (100 ng/mL) resulted in fMLP/LTB4 ratio of 10.2646 (see Figure 3A).
Figure 3. Empirical data: (A) Ratio of fMLP/LTB4 cell migration, and (B) number of cells that oscillate (change direction at least three times) while migrating toward fMLP (green) and LTB4 (red) vs. LPS concentration in ng/mL. Data reproduced from Boribong et al. (2019).
There are approximately 40, 000 FPR1 and 13, 333 BLT1 receptors on each neutrophil (Schneider et al., 2012). We therefore set initial conditions to [FPR1](0) = 40, 000 and [BLT1](0) = 13, 333. The reported GRK2/GRK5 ratio is 1.5 (Arraes et al., 2006). We choose initial conditions [GRK2](0) = 0.75 and [GRK5](0) = 0.5, to preserve this ratio. The reported GRK2 half-life varies between 60 min in HEK, COS-7, Jurkat, C6 glioma cells (Penela et al., 1998) and 20–24 h in undifferentiated HL-60 cells (Luo and Benovic, 2003). We choose a shorter half-life of 1 h, which corresponds to the GRK decay rate dw = log(2)/1 = 0.69 per hour. The reported GRK5 life-span is 3 h (Wu et al., 2012), which corresponds to the GRK5 decay rate df = 1/3 = 0.33 per hour. The FPR1's phosphorylation half-life is 15 s (Leoni et al., 2015). We choose both the phosphorylation and dephosphorylation rates based on this value, a1 = a2 = log(2) × 3, 600/15 = 166 per hour. BLT1 phosphorylation's half-life is 120 s (Gaudreault et al., 2005). We choose both the phosphorylation and dephosphorylation rates based on this value, b1 = b2 = b3 = log(2) × 3, 600/120 = 20 per hour. As in our previous work (Kadelka et al., 2019), the LPS degradation rate is dL = 0.1 per day. For simplicity, we fix most unknown parameters at one, aw = cf = af = bf = JF1 = Jf2 = JB1 = JB2 = 1. Moreover, cw = 15, , bwf = 0.13 and n = 3. The parameter values are summarized in Table 1.
We coupled system (1) with an oscillator describing the dynamics of non-phosphorylated and phosphorylated extracellular signal-regulated kinases (ERK), [ERK] and [ERKp], that are participating in an autocatalytic reaction with the help of intermediate non-phosphorylated and phosphorylated enzymes, [E] and [Ep] (see Figure 7). We assume that [ERK] activation is LPS-dependent and occurs at rate k1[LPS]. The phosphorylated [ERKp] decays at rate k2. The phosphorylated enzyme [Ep] follows the following reaction:
where kEi are the dephosphorylation and phosphorilation rates and JEi are the phosphorylation and dephosphorylation half-maximal rates, i = {1, 2}. If we assume chemical equilibrium, [Ep]+[E] = 1, and k3 = kE2/kE1, we obtain that:
where X = k3 − [ERKp] + k3JE1 + JE2[ERKp] and G([ERKp], k3, JE1, JE2)) is the Goldbeter-Koshland function (Goldbeter and Koshland, 1981). Hence, the phosphorylation of [ERK] occurs at rate (k0s + k0G([ERKp], k3, JE1, JE2))[ERK]. Lastly, the LPS is constant at all times LPS=[LPS](0), to account for positive long-term [ERK] levels. The model is given by the system:
Experimental results reported that neutrophils treated overnight with LPS may lose their ability to move up the chemoattractant gradient, become disoriented, and display oscillatory behavior (Boribong et al., 2019). Moreover, the highest number of cells to display such oscillatory behavior occurs following LPS exposure with super-low dose (1 ng/mL) (see Figure 3B) (Boribong et al., 2019).
We assume that initially [ERK](0) = 5 and [ERKp](0) = 0.1. Kinase [ERK] is produced at rate k1 = 0.3 and phosphorylated at rate k0s = 0.01. Kinase [ERKp] is lost at rate k2 = 1. Enzyme [E] is phosphorylated, in the presence of [ERK], at rate k0 = 0.4 and dephosphorylated at rate k3 = 0.3. The processes are modeled using Michaelis-Menten terms, with densities where phosphorylation/ dephosphorylation are half-maximal being set to JE1 = JE2 = 0.005. All other parameters and initial conditions are as in model (1). The new parameter values are summarized in Table 2.
We evaluated neutrophil migration between end-target chemoattractant fMLP and intermediary chemoattractant LTB4 by developing model (1), which considers the interaction between the chemoattractants' receptors, [FPR1] and [BLT1], the receptors' kinases, [GRK2] and [GRK5], and [LPS]. We quantified the [FPR1]/[BLT1] ratio for different [LPS] doses under the dynamics of system (1), and parameters/initial conditions given in Table 1. Since the experimental data has collected ratios of cell migration 5 h after LPS challenge, we first quantified [FPR1]/[BLT1] at time t = 5.
Model (1) exhibits bistable behavior between high and low [GRK2] concentrations (low and high [GRK5] concentrations), with low [LPS] priming leading to high [GRK2] production and high [LPS] priming leading to low [GRK2] production (see Figure 4B). Five hours following challenge with super-low-dose (1 ng/mL) LPS, model (1) predicts the presence of a small number of receptors, which are distributed equally among FPR1 and BLT1, [FPR1]/[BLT1](5) = 1 (see Figure 4A, solid lines). Under our abstraction this means that, following challenge with 1 ng/mL LPS, an equal number of neutrophils migrated toward the fMLP and LTB4 gradients. Conversely, 5 h following high-dose challenge (100 ng/mL) LPS, model (1) predicts the presence of a large number of receptors of both types, with [FPR1] exceeding [BLT1] by one fold, i.e, [FPR1]/[BLT1](5) = 10 (see Figure 4A, solid lines). Under our abstraction this means that a large number of neutrophils have migrated in both directions, with ten times more neutrophils migrating toward fMLP than LTB4. These [FPR1]/[BLT1] ratios are similar to the fMLP/LTB4 ratios observed in the experimental data (Boribong et al., 2019) (see Figure 3A). We further quantified the [FPR1]/[BLT1] ratio past the 5 h in the experiment. For the [LPS](0) = 1 ng/mL LPS challenge, [FPR1]/[BLT1](t) = 1 for all t ≥ 5. By contrast, for the [LPS](0) = 100 ng/mL LPS challenge, the [FPR1]/[BLT1](t) ratio becomes larger and larger as t increases, with the majority of cells favoring the primary fMLP gradient (not shown).
Figure 4. Theoretical results: Dynamics of (A) [FPR1] (green) and [BLT1] (red) and, (B) [GRK2] (purple) and [GRK5] (pink) for [LPS](0) = 1 ng/mL (solid lines) and [LPS](0) = 100 ng/mL (dashed lines) as given by model (1). Parameters and initial conditions are given in Table 1.
To determine the relationship between the LPS challenge dose and the FPR1/BLT1 ratio, we derived a graph that quantifies [FPR1]/[BLT1](5), 5 h following cell priming, as a function of the [LPS] dose, predicted by model (1) and parameter values/initial conditions in Table 1. We found that the experimental observation for the super-low-dose (1 ng/mL) LPS, [FPR1]/[BLT1](5) = 1, is preserved for all challenges with LPS values lower than 3.9 ng/mL. For 4 − 6.7 ng/mL LPS challenge, [BLT1] exceeds [FPR1] at t = 5 h, but the two receptors will eventually reach identical levels at equilibrium. Lastly, the [FPR1]/[BLT1](5) ratio grows larger than one and keeps increasing for LPS dose >6.7 ng/mL, eventually reaching the experimental prediction of ten, [FPR1]/[BLT1](5) = 10, for high-dose LPS challenge (100 ng/mL) (see Figure 5) and increasing further as time passes or for higher challenge (not shown).
We have chosen the parameters in model (1) such that the [FPR1]/[BLT1](5) ratio matches the observed fMLP/LTB4 data (Boribong et al., 2019). We are interested in determining how this balance can be broken and which interactions are responsible for the bimodal switch between equal [FPR1] and [BLT1] values and dominant [FPR1] values. The results presented at t = 5 h are transient results. At equilibrium, the [FPR1]/[BLT1] ratio is 1 for LPS < 15 ng/mL and as large as 107 for LPS = 100 ng/mL. This indicates that all [BLT1] molecules have been down regulated, and only [FPR1] molecules remain on the surface of neutrophils. This is due to the large non-LPS activation rate of [GRK2] protein, cw = 15. If we either increase the cw value to cw = 28 or decrease it to cw = 5, we maintain the [FRP1]/[BLT1] ratio 5 h after super-low-dose (1 ng/mL) and high-dose (100 ng/mL), [FPR1]/[BLT1](5), if we simultaneously decrease the inhibition rate of [GRK5] to 5 × 10−4 or increase it to 0.25, respectively (see Figure 6). The range of LPS initial conditions that lead to identical [FPR1] and [BLT1] distribution decrease as cw decreases, with the [FPR1]/[BLT1](5) = 1 prediction being lost for cw = 5 (see Figure 6, red curves). The results are insensitive to the LPS decay rate, or to [FPR1] and [BLT1] values where phosphorylation and dephosphorylation levels are half-maximal (not shown). These results suggests that the bimodal switch between the [FPR1]/[BLT1] levels is due to mutual inhibition of GRK2-GRK5 kinases. To confirm this, we removed the inhibition factors, by replacing with (cw + aw[LPS])/bfw and (cf + af[LPS])/(bwf + [GRK2]) with (cf + af[LPS])/bwf. When the mutual inhibition is removed, the equilibrium [FPR1]/[BLT1] levels are constant, and equal to 1,500, regardless of the size of LPS stimulus.
Figure 6. FPR1/BLT1 at time t = 5 h, as given by model (1), for cw = 28, (black stars); cw = 15, bwf = 0.13 (blue stars); and cw = 5, bwf = 0.245 (red stars).
Experimental results reported that neutrophils treated overnight with LPS may loose their ability to move up the chemoattractant gradient, become disoriented, and display oscillatory behavior (Boribong et al., 2019). Moreover, the highest number of cells to display such oscillatory behavior occurs following LPS exposure with low dose of 1 mg/ml (see Figure 3B) (Boribong et al., 2019). To determine the molecular mechanisms responsible for the oscillations, we extended the bistable system (1), by coupling it with an activator-inhibitor oscillatory model for the dynamics of non-phosphorylated and phosphorylated extracellular signal-regulated kinases, [ERK] and [ERKp], and two auxiliary enzymes, [E] and [Ep] based on diagram (7), model (4) and parameter values/initial conditions in Tables 1, 2. Moreover LPS is fixed at its initial condition. Under the chosen parameters, we obtain long-term oscillatory movement for all populations, for constant super-low-challenge LPS (1 ng/mL), as predicted by the data (Boribong et al., 2019) (see Figures 8A–C). Interestingly, the oscillatory behavior is maintained for a short LPS range, (0.5-1.1) ng/mL; and corresponds to equal distribution of [FPR1] and [BLT1] receptors. If we either lower the constant LPS challenge to < 0.4ng/mL or increase it to 1.2 − 375ng/mL, we obtain equal density of [FPR1] and [BLT1] receptors, but no oscillations (see Figures 8D–F). If the LPS constant challenge is increased further, to > 376ng/mL, [FPR1] receptors dominate the outcome (see Figures 8G–I). While the switch between low and high [FPR1]/[BLT1] ratio observed in the constant high dose LPS challenge is due to mutual inhibitions of [GRK2] and [GRK5] kinases, as observed in model (1), the oscillatory dynamics are due to the oscillatory dynamics of the [ERK] and [ERKp] kinases. This oscillatory behavior can be broken by either increasing or decreasing the constant LPS challenge. Such information can inform interventions, as dysoriented neutrophil movement is not desirable and has been shown to have negative effects during pathogenic infections. Dysregulated neutrophil response to infection can lead to sepsis and end-organ failure and is a leading cause of death worldwide (Reddy and Standiford, 2010; Shen et al., 2017).
Figure 8. Theoretical results: Dynamics of [ERK] and [ERKp] (A,D,G); [GRK2] and [GRK5] (B,E,H); and [FPR1] and [BLT1] (C,F,I), as given by model (4) for [LPS](0) = 1 ng/mL (A–C); [LPS](0) = 100 ng/mL (D–F); and [LPS](0) = 400 ng/mL (G–I).
In this study, we developed compartmental mathematical models of molecular interactions that govern neutrophil migratory patterns when exposed to competing chemoattractants and challenged with external stimuli. When the models were restricted to the interactions between the chemoattractants' receptors, their kinases, and LPS, we predicted a bistable switch between two states: one in which the densities of the two chemoattractant receptors, FPR1 and BLT1, are equal and one in which the receptors for the primary chemoattractant, FPR1, dominate. We hypothesized that the two states correspond to two states observed experimentally: equal migration toward the primary and intermediary chemoattractants, fMLP and LTB4, and predominant migration toward the primary chemoattractant, fMLP (Boribong et al., 2019). The experimental data connected the differential migratory outcomes with the magnitude of the external LPS challenge, with super-low 1 ng/mL LPS leading to equal migration toward both chemoattractants and high 100 ng/mL LPS leading to ten times higher migration toward fMLP, 5 h after challenge. In the mathematical model, the external signal corresponds to the initial condition for variable LPS. Our model was calibrated to match the experimental data 5 h after stimuli, with [FPR1]/[BLT1](5) = 1 for [LPS](0) = 1 and [FPR1]/[BLT1](5) = 10 for [LPS](0) = 100. Furthermore, 5 h after LPS challenge, we obtain equal levels of FPR1 and BLT1 receptors for all initial conditions [LPS](0) < 3.7 ng/mL and increasingly more FPR1 than BLT1 receptors when the initial condition for LPS increases, with ten times more FPR1 than BLT1 receptors when [LPS](0) = 100 ng/mL. When we run the model to equilibrium, however, we obtain equal FPR1 and BLT1 receptors for an even larger range of LPS initial conditions, < 15 ng/ml; and dominant FPR1 levels for LPS> 16 ng/mL. This implies that not just the challenge dose, but the duration of the experiment may influence the quantitative outcomes.
We investigated the molecular interactions that are responsible for the bistable outcomes and found that when the mutual inhibition of GRK2 and GRK5 kinases is either removed, or the balance is broken, the model is no longer bistable. Instead, when run to equilibrium, it settles into a state where FPR1 receptors dominate the outcome, indicative of predominant migration toward the primary chemoattractant.
When the models were expanded to add the interaction with the ERK signaling pathways under constant LPS challenge, we obtained a third dynamical state, where we have equal FPR1/BLT1=1, but the equilibrium is lost, and the FPR1 and BLT1 receptors oscillate between two values. We assume this to be indicative of neutrophil oscillation which, in the experimental setup, is equivalent to cells changing direction (Boribong et al., 2019). We investigated how changes in the constant LPS dose affect the outcomes and found that, at equilibrium, FPR1 and BLT1 receptors are equal and non-oscillating for both super-super-low (< 0.5 ng/mL) and high dose (1.1, 375 ng/mL) LPS; are equal and oscillating for super-low dose (0.5–1.1 ng/mL) LPS; and FPR1 outnumbers BLT1 for super-high dose (> 376 ng/mL) LPS. The non-asymptotic dynamics are due to the oscillatory behavior of phosphorylated and non-phosphorylated ERK molecules, who undergo an auto-catalytic interaction with two undefined enzymes. We have modeled the interaction using an activation-inhibition motif, with similar dynamics being obtained if the oscillations are induced by a substrate depletion motif (Tyson et al., 2003). Further work is needed to determine the nature of enzyme or their regulation. We are currently working to validate the model by retrieving neutrophils from our microfluidic device post-migration and quantifying FPR1, BLT1, GRK2, and GRK 5 levels by Droplet DigitalTM PCR (ddPCRTM).
Our models are limited by the presence of many unknown parameters. While we strived to match the empirical data, the results are mostly qualitative. Similar results can be obtained with many different parameters sets. For example, cw only slightly influences the FPR1/BLT1 ratio at t = 5 h (see Figure 9, left panel), while the cooperativity coefficient n and the LPS-dependent GRK5 production af have drastic effects on the size of the ration (see Figure 9, middle and right panels). The unchanging factors, however, are the motifs of bistability, which are induced by the dual inhibition of the G-protein kinases; the oscillatory motifs, which are induced by the oscillatory ERK dynamics; and the influence of external stimuli on outcomes.
Figure 9. FPR1/BLT1 at time t = 5 h, as given by model (1), for (Left) cw = 10 (blue stars), cw = 15 (black stars), cw = 20 (red stars); (Middle) n = 1 (blue stars), n = 3 (black stars), n = 5 (red stars); and (Right) af = 0.1 (blue stars), af = 1 (black stars), af = 3 (red stars).
In conclusion, we developed mathematical models for the molecular interactions responsible for neutrophils migratory phenotypes, calibrated them against empirical data, and used their dynamics to determine the external stimuli ranges that account for neutrophils migration toward a pro-inflammatory chemoattractant, a pro-tolerant chemoattractant, or oscillatory dynamics indicative of dysorientation and loss of function. Understanding the relationship between neutrophils' dynamics and the mechanisms responsible for their movement is important for preventing and predicting immune disorders. Activation markers in neutrophils are potential biomarkers for the diagnosis and prognosis of sepsis. Septic patients can be screened for neutrophil markers, such as GRK 2/5, FPR1, and BLT1, and this predictive model can guide patient treatment. In the future, we plan to expand this model to include more complex signaling from the microenvironment and aim to predict not only dysfunctional migration in neutrophils, but even the probability of cells accumulating in specific organs, such as the lung or kidney. We can use these predictive models to define optimal patient treatment and to identify immunotherapeutic targets (i.e., small molecule inhibition, microRNAs, gene therapy) to promote directional neutrophil migration.
The original contributions presented in the study are included in the article/supplementary material, further inquiries can be directed to the corresponding author/s.
SC, SK, and BB wrote the code. SC wrote the manuscript. All authors reviewed and revised the manuscript and conceived the study.
The authors declare that the research was conducted in the absence of any commercial or financial relationships that could be construed as a potential conflict of interest.
SK and SC acknowledge funding from National Science Foundation grant No. 1813011. BB and CJ acknowledge funding from The National Institute of General Medical Sciences of the National Institutes of Health under award number R35GM133610.
Alves-Filho, J. C., Benjamim, C., Tavares-Murta, B. M., and Cunha, F. Q. (2005). Failure of neutrophil migration toward infectious focus in severe sepsis: a critical event for the outcome of this syndrome. Memorias do Instituto Oswaldo Cruz 100, 223–226. doi: 10.1590/S0074-02762005000900038
Alves-Filho, J. C., Spiller, F., and Cunha, F. Q. (2010). Neutrophil paralysis in sepsis. Shock 34, 15–21. doi: 10.1097/SHK.0b013e3181e7e61b
Arraes, S. M. A., Freitas, M. S., da Silva, S. V., de Paula Neto, H. A., Alves-Filho, J. C., Martins, M. A., et al. (2006). Impaired neutrophil chemotaxis in sepsis associates with grk expression and inhibition of actin assembly and tyrosine phosphorylation. Blood 108, 2906–2913. doi: 10.1182/blood-2006-05-024638
Bayani, A., Dunster, J., Crofts, J., and Nelson, M. (2020). Mechanisms and points of control in the spread of inflammation: a mathematical investigation. Bull. Math. Biol. 82, 1–22. doi: 10.1007/s11538-020-00709-y
Boribong, B. P., Lenzi, M. J., Li, L., and Jones, C. N. (2019). Super-low dose lipopolysaccharide dysregulates neutrophil migratory decision-making. Front. Immunol. 10:359. doi: 10.3389/fimmu.2019.00359
Byrne, M. B., Kimura, Y., Kapoor, A., He, Y., Mattam, K. S., Hasan, K. M., et al. (2014). Oscillatory behavior of neutrophils under opposing chemoattractant gradients supports a winner-take-all mechanism. PLoS ONE 9:e85726. doi: 10.1371/journal.pone.0085726
Chen, Z., Gaudreau, R., Le Gouill, C., Rola-Pleszczynski, M., and Stanková, J. (2004). Agonist-induced internalization of leukotriene B4 receptor 1 requires G-protein-coupled receptor kinase 2 but not arrestins. Mol. Pharmacol. 66, 377–386. doi: 10.1124/mol.66.3
Ciupe, S. M., Miller, C. J., and Forde, J. E. (2018). A bistable switch in virus dynamics can explain the differences in disease outcome following SIV infections in rhesus macaques. Front. Microbiol. 9:1216. doi: 10.3389/fmicb.2018.01216
Ciupe, S. M., Ribeiro, R. M., Nelson, P. W., and Perelson, A. S. (2007). Modeling the mechanisms of acute hepatitis B virus infection. J. Theor. Biol. 247, 23–35. doi: 10.1016/j.jtbi.2007.02.017
Davenport, A. P., Scully, C. C., de Graaf, C., Brown, A. J., and Maguire, J. J. (2020). Advances in therapeutic peptides targeting G protein-coupled receptors. Nat. Rev. Drug Discov. 19, 389–413. doi: 10.1038/s41573-020-0062-z
Day, J., Rubin, J., Vodovotz, Y., Chow, C. C., Reynolds, A., and Clermont, G. (2006). A reduced mathematical model of the acute inflammatory response II. Capturing scenarios of repeated endotoxin administration. J. Theor. Biol. 242, 237–256. doi: 10.1016/j.jtbi.2006.02.015
Demaret, J., Venet, F., Friggeri, A., Cazalis, M.-A., Plassais, J., Jallades, L., et al. (2015). Marked alterations of neutrophil functions during sepsis-induced immunosuppression. J. Leukocyte Biol. 98, 1081–1090. doi: 10.1189/jlb.4A0415-168RR
Dianqing, W. (2005). Signaling mechanisms for regulation of chemotaxis. Cell Res. 15, 52–56. doi: 10.1038/sj.cr.7290265
Fan, J., and Malik, A. B. (2003). Toll-like receptor-4 (TLR4) signaling augments chemokine-induced neutrophil migration by modulating cell surface expression of chemokine receptors. Nat. Med. 9, 315–321. doi: 10.1038/nm832
Fischer, H. P. (2008). Mathematical modeling of complex biological systems: from parts lists to understanding systems behavior. Alcohol Res. Health 31:49.
Futosi, K., Fodor, S., and Mócsai, A. (2013). Neutrophil cell surface receptors and their intracellular signal transduction pathways. Int. Immunopharmacol. 17, 1185–1197. doi: 10.1016/j.intimp.2013.11.010
Gaudreau, R., Le Gouill, C., Venne, M.-H., Stankova, J., and Rola-Pleszczynski, M. (2002). Threonine 308 within a putative casein kinase 2 site of the cytoplasmic tail of leukotriene B4 receptor (BLT1) is crucial for ligand-induced, G-protein-coupled receptor-specific kinase 6-mediated desensitization. J. Biol. Chem. 277, 31567–31576. doi: 10.1074/jbc.M202723200
Gaudreault, E., Thompson, C., Stankova, J., and Rola-Pleszczynski, M. (2005). Involvement of BLT1 endocytosis and yes kinase activation in leukotriene b4-induced neutrophil degranulation. J. Immunol. 174, 3617–3625. doi: 10.4049/jimmunol.174.6.3617
Goldbeter, A., and Koshland, D. E. (1981). An amplified sensitivity arising from covalent modification in biological systems. Proc. Natl. Acad. Sci. U.S.A. 78, 6840–6844. doi: 10.1073/pnas.78.11.6840
Heit, B., Robbins, S. M., Downey, C. M., Guan, Z., Colarusso, P., Miller, B. J., et al. (2008). Pten functions to'prioritize'chemotactic cues and prevent'distraction'in migrating neutrophils. Nat. Immunol. 9, 743. doi: 10.1038/ni.1623
Heit, B., Tavener, S., Raharjo, E., and Kubes, P. (2002). An intracellular signaling hierarchy determines direction of migration in opposing chemotactic gradients. J. Cell Biol. 159, 91–102. doi: 10.1083/jcb.200202114
Ionides, E. L., Fang, K. S., Isseroff, R. R., and Oster, G. F. (2004). Stochastic models for cell motion and taxis. J. Math. Biol. 48, 23–37. doi: 10.1007/s00285-003-0220-z
Jones, C. N., Hoang, A. N., Martel, J. M., Dimisko, L., Mikkola, A., Inoue, Y., et al. (2016). Microfluidic assay for precise measurements of mouse, rat, and human neutrophil chemotaxis in whole-blood droplets. J. Leukocyte Biol. 100, 241–247. doi: 10.1189/jlb.5TA0715-310RR
Kadelka, S., Boribong, B. P., Li, L., and Ciupe, S. M. (2019). Modeling the bistable dynamics of the innate immune system. Bull. Math. Biol. 81, 256–276. doi: 10.1007/s11538-018-0527-y
Kolaczkowska, E., and Kubes, P. (2013). Neutrophil recruitment and function in health and inflammation. Nat. Rev. Immunol. 13, 159–175. doi: 10.1038/nri3399
Leber, A., Abedi, V., Hontecillas, R., Viladomiu, M., Hoops, S., Ciupe, S., et al. (2016). Bistability analyses of CD4+ T follicular helper and regulatory cells during Helicobacter pylori infection. J. Theor. Biol. 398, 74–84. doi: 10.1016/j.jtbi.2016.02.036
Leoni, G., Gripentrog, J., Lord, C., Riesselman, M., Sumagin, R., Parkos, C. A., et al. (2015). Human neutrophil formyl peptide receptor phosphorylation and the mucosal inflammatory response. J. Leukocyte Biol. 97, 87–101. doi: 10.1189/jlb.4A0314-153R
Liu, X., Ma, B., Malik, A. B., Tang, H., Yang, T., Sun, B., et al. (2012). Bidirectional regulation of neutrophil migration by mitogen-activated protein kinases. Nat. Immunol. 13:457. doi: 10.1038/ni.2258
Luo, J., and Benovic, J. L. (2003). G protein-coupled receptor kinase interaction with Hsp90 mediates kinase maturation. J. Biol. Chem. 278, 50908–50914. doi: 10.1074/jbc.M307637200
Magalhaes, A. C., Dunn, H., and Ferguson, S. S. (2012). Regulation of GPCR activity, trafficking and localization by GPCR-interacting proteins. Brit. J. Pharmacol. 165, 1717–1736. doi: 10.1111/j.1476-5381.2011.01552.x
Mócsai, A., Walzog, B., and Lowell, C. A. (2015). Intracellular signalling during neutrophil recruitment. Cardiovasc. Res. 107, 373–385. doi: 10.1093/cvr/cvv159
Murphy, P. M. (1994). The molecular biology of leukocyte chemoattractant receptors. Annu. Rev. Immunol. 12, 593–633. doi: 10.1146/annurev.iy.12.040194.003113
Neel, N. F., Schutyser, E., Sai, J., Fan, G.-H., and Richmond, A. (2005). Chemokine receptor internalization and intracellular trafficking. Cytokine Growth Factor Rev. 16, 637–658. doi: 10.1016/j.cytogfr.2005.05.008
Nelson, P., Smith, N., Ciupe, S., Zou, W., Omenn, G. S., and Pietropaolo, M. (2009). Modeling dynamic fluctuations in type 1 diabetes progression: quantifying β-cell variation after the appearance of islet-specific autoimmune responses. Math. Biosci. Eng. 6, 753. doi: 10.3934/mbe.2009.6.753
Penela, P., Ruiz-Gómez, A., Casta no, J. G., and Mayor, F. (1998). Degradation of the G protein-coupled receptor kinase 2 by the proteasome pathway. J. Biol. Chem. 273, 35238–35244. doi: 10.1074/jbc.273.52.35238
Petri, B., and Sanz, M.-J. (2018). Neutrophil chemotaxis. Cell Tissue Res. 371, 425–436. doi: 10.1007/s00441-017-2776-8
Philipp, M., Berger, I. M., Just, S., and Caron, M. G. (2014). Overlapping and opposing functions of G protein-coupled receptor kinase 2 (GRK2) and GRK5 during heart development. J. Biol. Chem. 289, 26119–26130. doi: 10.1074/jbc.M114.551952
Pillay, J., Ramakers, B. P., Kamp, V. M., Loi, A. L. T., Lam, S. W., Hietbrink, F., et al. (2010). Functional heterogeneity and differential priming of circulating neutrophils in human experimental endotoxemia. J. Leukocyte Biol. 88, 211–220. doi: 10.1189/jlb.1209793
Postma, M., and van Haastert, P. J. (2016). Mathematics of experimentally generated chemoattractant gradients. Methods Mol Biol. 1407, 381–396. doi: 10.1007/978-1-4939-3480-5_26
Prossnitz, E. R., Kim, C. M., Benovic, J. L., and Richard, D. Y. (1995). Phosphorylation of the N-formyl peptide receptor carboxyl terminus by the G protein-coupled receptor kinase, GRK2. J. Biol. Chem. 270, 1130–1137. doi: 10.1074/jbc.270.3.1130
Reddy, R. C., and Standiford, T. J. (2010). Effects of sepsis on neutrophil chemotaxis. Curr. Opin. Hematol. 17, 18–24. doi: 10.1097/MOH.0b013e32833338f3
Reynolds, A., Rubin, J., Clermont, G., Day, J., Vodovotz, Y., and Ermentrout, G. B. (2006). A reduced mathematical model of the acute inflammatory response: I. Derivation of model and analysis of anti-inflammation. J. Theor. Biol. 242, 220–236. doi: 10.1016/j.jtbi.2006.02.016
Schneider, E. H., Weaver, J. D., Gaur, S. S., Tripathi, B. K., Jesaitis, A. J., Zelenka, P. S., et al. (2012). The leukocyte chemotactic receptor FPR1 is functionally expressed on human lens epithelial cells. J. Biol. Chem. 287, 40779–40792. doi: 10.1074/jbc.M112.411181
Shen, X.-F., Cao, K., Jiang, J. P., Guan, W.-X., and Du, J.-F. (2017). Neutrophil dysregulation during sepsis: an overview and update. J. Cell. Mol. Med. 21, 1687–1697. doi: 10.1111/jcmm.13112
Sorriento, D., Ciccarelli, M., Santulli, G., Campanile, A., Altobelli, G. G., Cimini, V., et al. (2008). The G-protein-coupled receptor kinase 5 inhibits NFκB transcriptional activity by inducing nuclear accumulation of iκbα. Proc. Natl. Acad. Sci. U.S.A. 105, 17818–17823. doi: 10.1073/pnas.0804446105
Tyson, J. J., Chen, K. C., and Novak, B. (2003). Sniffers, buzzers, toggles and blinkers: dynamics of regulatory and signaling pathways in the cell. Curr. Opin. Cell Biol. 15, 221–231. doi: 10.1016/S0955-0674(03)00017-6
Vodovotz, Y., Constantine, G., Rubin, J., Csete, M., Voit, E. O., and An, G. (2009). Mechanistic simulations of inflammation: current state and future prospects. Math. Biosci. 217, 1–10. doi: 10.1016/j.mbs.2008.07.013
Wang, X., Qin, W., Song, M., Zhang, Y., and Sun, B. (2016a). Exogenous carbon monoxide inhibits neutrophil infiltration in LPS-induced sepsis by interfering with FPR1 via p38 MAPK but not GRK2. Oncotarget 7:34250. doi: 10.18632/oncotarget.9084
Wang, X., Qin, W., Zhang, Y., Zhang, H., and Sun, B. (2016b). Endotoxin promotes neutrophil hierarchical chemotaxis via the p38-membrane receptor pathway. Oncotarget 7:74247. doi: 10.18632/oncotarget.12093
Wu, Z., Chen, Y., Yang, T., Gao, Q., Yuan, M., Ma, L., et al. (2012). Targeted ubiquitination and degradation of G-protein-coupled receptor kinase 5 by the DDB1-CUL4 ubiquitin ligase complex. PLoS ONE 7:e43997. doi: 10.1371/journal.pone.0043997
Yuan, R., Geng, S., Chen, K., Diao, N., Chu, H. W., and Li, L. (2016a). Low-grade inflammatory polarization of monocytes impairs wound healing. J. Pathol. 238, 571–583. doi: 10.1002/path.4680
Keywords: neutrophil migration, mathematical model, lipopolysaccharide (LPS), bistability, cellular decision-making
Citation: Ciupe SM, Boribong BP, Kadelka S and Jones CN (2021) Bistable Mathematical Model of Neutrophil Migratory Patterns After LPS-Induced Epigenetic Reprogramming. Front. Genet. 12:633963. doi: 10.3389/fgene.2021.633963
Received: 26 November 2020; Accepted: 27 January 2021;
Published: 23 February 2021.
Edited by:
Tian Hong, The University of Tennessee, Knoxville, United StatesReviewed by:
Stephanie Evans, University of Michigan, United StatesCopyright © 2021 Ciupe, Boribong, Kadelka and Jones. This is an open-access article distributed under the terms of the Creative Commons Attribution License (CC BY). The use, distribution or reproduction in other forums is permitted, provided the original author(s) and the copyright owner(s) are credited and that the original publication in this journal is cited, in accordance with accepted academic practice. No use, distribution or reproduction is permitted which does not comply with these terms.
*Correspondence: Stanca M. Ciupe, c3RhbmNhQHZ0LmVkdQ==; Caroline N. Jones, Y2Fyb2xpbmUuSm9uZXNAdXRkYWxsYXMuZWR1
Disclaimer: All claims expressed in this article are solely those of the authors and do not necessarily represent those of their affiliated organizations, or those of the publisher, the editors and the reviewers. Any product that may be evaluated in this article or claim that may be made by its manufacturer is not guaranteed or endorsed by the publisher.
Research integrity at Frontiers
Learn more about the work of our research integrity team to safeguard the quality of each article we publish.