- 1Department of Neurosurgery/Neuro-oncology, State Key Laboratory of Oncology in South China, Collaborative Innovation Center for Cancer Medicine, Sun Yat-sen University Cancer, Guangzhou, China
- 2The First Clinical Medical College of Yunnan University of Traditional Chinese Medicine, Kunming, China
- 3Department of Cerebrovascular Surgery, The Third Affiliated Hospital, Sun Yat-sen University, Guangzhou, China
- 4Department of Neurosurgy, Jiangmen Central Hospital, Jiangmen, China
Enhancer RNAs, a type of long non-coding RNAs (lncRNAs), play a critical role in the occurrence and development of glioma. RNA-seq data from 161 glioblastoma multiforme (GBM) samples were acquired from The Cancer Genome Atlas database. Then, 70 eRNAs were identified as prognosis-related genes, which had significant relations with overall survival (log-rank test, p < 0.05). AC003092.1 was demonstrated as an immune-related eRNA by functional enrichment analysis. We divided samples into two groups based on AC003092.1 expression: AC003092.1 High (AC003092.1_H) and AC003092.1 Low (AC003092.1_L) and systematically analyzed the influence of AC003092.1 on the immune microenvironment by single-sample gene-set enrichment analysis and CIBERSORTx. We quantified AC003092.1 and TFPI2 levels in 11 high-grade gliomas, 5 low-grade gliomas, and 7 GBM cell lines. Our study indicates that AC003092.1 is related to glioma-immunosuppressive microenvironment, and these results offer innovative sights into GBM immune therapy.
Introduction
In the human central nervous system, gliomas are the most common primary tumor. Among gliomas, glioblastoma multiforme (GBM) is the most malignant and has the worst prognosis. Despite that surgery, radiotherapy, and chemotherapy were applied, the treatment effect of patients with glioblastoma is still worse (Cuddapah et al., 2014). So far, the median survival time for patients with glioblastoma is only 14 months (Weller et al., 2015).
Long non-coding RNAs (lncRNAs) are a type of non-coding RNAs longer than 200 nucleotides (Wapinski and Chang, 2011; Spizzo et al., 2012). LncRNAs have multiple functions such as chromatin topology, scaffolding and modulating the activity of proteins and RNA, regulators of neighboring transcription, and encoding functional micropeptides (Ransohoff et al., 2018), and part of lncRNAs has been shown to encode functional peptides or proteins (Matsumoto et al., 2017; Rion and Ruegg, 2017; Lu et al., 2019; Cardon et al., 2020). With the identification of more and more functional lncRNAs, lncRNAs have become a critical factor in the occurrence and development of gliomas (Peng et al., 2018).
Enhancer RNAs (eRNAs), a subclass of lncRNAs, are transcribed from enhancers and regulate biological process by adjusting target gene expression (Long et al., 2016). Thousands of eRNAs have been determined to play an important role in the transcription of human cells (Li et al., 2016). During the development of malignant tumors, eRNA can participate in the expression of oncogenes and the activation of oncogenic pathways. In prostate cancer, KLK3e, an androgen-induced eRNA, scaffolds the androgen receptor (AR)–associated protein complex could modulate chromosomal architecture and enhance AR-dependent KLK3 expression to promote prostate carcinogenesis (Hsieh et al., 2014). And in regulation of the immune response, eRNA (IL1β-eRNA) attenuates messenger RNA transcription and release of the proinflammatory mediators to influence human innate immune response (IIott et al., 2014). As far as we know, the researches of immune-related eRNA in GBM have not been reported.
In our study, we identified prognosis-related eRNAs and their predicted target genes in GBM. According to our analysis, lncRNA AC003092.1 was an immune-related eRNA with significant correlation to survival in patients with GBM and upregulated target gene, TFPI2 (tissue factor pathway inhibitor 2), expression. Immunogenomic analysis showed that the expression of AC003092.1 is related to the immune cell fractions, function, and pathways. We validated the positive correlation between the expression of AC003092.1 and TFPI2 in high-grade gliomas and glioblastoma cell lines.
Materials and Methods
Gene Expression Datasets and Data Processing
RNA-seq data of 161 GBM patients were obtained from The Cancer Genome Atlas (TCGA) datasets. All patients had accurate follow-up data. For further analysis, RNA transcriptome profiling was then performed using log2-based transformation of FPKM values by R Studio software (version 1.2.5001). Figure 1 shows the paths of the data analysis.
Identification of Prognosis-Related eRNA in GBM
PreSTIGE, an algorithm that could predict tissue-specific enhancers based on the H3K4me1 mark and tissue-specific expression of mRNAs, was applied to predict lncRNAs expressed from active tissue-specific enhancers and their target genes (Corradin et al., 2014; Vucicevic et al., 2015). Ensembl transcript ID and gene symbol were annotated by “biomaRt” R package (human GRCh38.p13) (Durinck et al., 2009; Smedley et al., 2009). The log-rank test was used to calculate the differences between eRNA expression (cutoff value = 50%) and survival time in GBM patients using a threshold of p < 0.05. Based on the result of log-rank test, Kaplan–Meier curves were plotted to indicate the survival time differences. According to the list of eRNAs and target genes, Spearman correlation test was used to evaluate correlations and Spearman rank correlation coefficient r > 0.4, p < 0.001 as the threshold.
Functional Enrichment Analysis
All transcripts with significant correlation (Spearman rank correlation coefficient r > 0.4, p < 0.001) with prognosis-related eRNAs were identified as eRNA-related genes by Spearman correlation test. To investigate gene ontology and signaling pathway enrichment, eRNA-related genes were uploaded to online tool Metascape1 website for gene annotation, functional enrichment, and interactome analysis (Ashburner et al., 2000; Lebrec et al., 2009; Gene Ontology Consortium, 2015; Zhou et al., 2019). The terms were considered as significant and grouped into clusters when p < 0.01 and the numbers of enriched genes ≥3 based on their membership similarities. According to results of functional enrichment analysis, we confirmed the immune-related eRNA for further research.
Immunogenomic Analysis of AC003092.1
One hundred sixty-one GBM patients were divided into two groups, 80 AC003092.1_H patients and 81 AC003092.1_L patients, according to the expression of AC003092.1 (cutoff value = 50%). In every GBM patient, the single-sample gene-set enrichment analysis (ssGSEA) score was used to measure the enrichment levels of the 29 immune signatures (Supplementary Table 3) (Barbie et al., 2009; Hanzelmann et al., 2013). The infiltration of immune cell (immune score), stromal content (stromal score), and tumor purity were quantified by Estimation of STromal and Immune cells in MAlignant Tumors using Expression data (ESTIMATE) (Yoshihara et al., 2013). The immunogenomic analysis was executed by R Studio software (version 1.2.5001).
Assessment of Immune Cell–Type Fractions
The CIBERSORTx and the LM22 gene signature were used to estimate the fraction of immune cell in the GBM patients (Newman et al., 2019). CIBERSORTx2 is an analytical tool from the Alizadeh Lab and Newman Lab to impute gene expression profiles and provide an estimation of the abundances of member cell types in a mixed cell population, using gene expression data. We uploaded RNA-seq data as the Mixture file and choose LM22 (22 immune cell types) as Signature matrix file. The successful deconvolution of a sample should be satisfied with the criteria of 1,000 permutations and p < 0.05. Mann–Whitney U test was used to compare the proportions of the immune cell phenotypes between AC003092.1_H and AC003092.1_L.
Quantification of AC003092.1 and TFPI2 Transcript Levels in Glioma Samples and GBM Cell Lines
To confirm the RNA-sequencing data, we validated AC003092.1 and TFPI2 transcript levels in glioma samples and GBM cell lines by reverse transcription quantitative polymerase chain reaction (RT-qPCR). The isolated total RNA was sourced in 11 HGG patients and 5 LGG patients who were diagnosed at our institution in Sun Yat-sen University Cancer Center (SYSUCC) from February 1, 2019, to July 1, 2019 (Supplementary Table 4), and 7 glioblastoma cell lines (U251, U138, T98G, SKMG4, U87, U118, and LN229). U251, U138, T98G, SKMG4, U87, U118, and LN229 were obtained from the State Key Laboratory of Oncology in South China and were maintained in Dulbecco modified eagle medium supplemented with 10% fetal bovine serum. All cells were maintained in a humidified incubator at 37°C and 5% CO2. The Ethics Committee of Sun Yat-sen University Cancer Center approved the procedures (no. GZR2018-244). Total RNA was isolated from tissue samples or cultured cells using RNA-Quick Purification Kit (ESscience, Shanghai, China). The cDNA was synthesized from 1,000 ng total RNA by Fast All-in-One RT Kit (ESscience, Shanghai, China). RT-qPCR was performed by Bio-Rad CFX96 Real-Time PCR System (Bio-Rad Laboratories, Inc., Hercules, CA, United States) and LightCycler 480 II Real-Time PCR System (Roche, Basel, Switzerland) with PowerUp SYBR Green Master Mix (Thermo Fisher Scientific, Waltham, MA, United States). Custom primers for AC003092.1 (forward: TTAGCAGCAAACCCAGAAC, reve rse: TGCTGAGGATACATGACGAA) and TFPI2 (forward: GCCTGAGAACTTTGAATGATGCTG, reverse: GGCCCTGT GTTTCTTATGTATCCTG) were obtained from Tianyi Huiyuan Bioscience and Technology Inc., Wuhan, China. Each sample was measured in triplicate, and the results were normalized to the housekeeping gene U6 (forward: CTCGCTTCGGCAGCACA, reverse: AACGCTTCACGAATTTGCGT) and GAPDH (for ward: GGAGCGAGATCCCTCCAAAAT, reverse: GGCTGTT GTCATACTTCTCATGG).
Results
Analysis of Prognosis-Related eRNA in GBM
According to previous study, there are 2,695 lncRNA transcripts, and 2,303 predicted target genes have been identified by the PreSTIGE algorithm (Vucicevic et al., 2015). The eRNA-target genes pairs were confirmed by this eRNA transcripts database. For further analysis, we conversed transcript ID to gene ID by “biomaRt” R package (Durinck et al., 2009). After conversion, the 2,695 eRNA transcripts were mapped to 1,288 genes. Then log-rank test was used to analyze the relationship of eRNAs and over survival in RNA-sequence data from 161 GBM samples of TCGA database. Next, we identified that 70 eRNAs were significantly associated with overall survival (Supplementary Table 1, log-rank test, p < 0.05).
Finally, the correlation between eRNAs and their predicted target genes was evaluated, and only 28 eRNAs were included in the next study (Spearman rank correlation coefficient r > 0.4, p < 0.001; Table 1).
LncRNA AC003092.1 Is an Immune-Related eRNA in GBM
To further evaluate biological process that eRNAs may regulate, we uploaded the eRNA-related genes to the website Metascape to identify Gene Ontology (GO) terms and Kyoto Encyclopedia of Genes and Genomes (KEGG) pathways. GO terms and KEGG pathways functional enrichment analysis showed that 420 genes that were significantly correlated to lncRNA AC003092.1 enriched notably in immune-related GO terms (Supplementary Table 2 and Figure 2A). The terms were considered as significant and grouped into clusters when p < 0.01 and the numbers of enriched genes were ≥ 3 based on their membership similarities. Each node represents an enriched term and is first colored by its cluster ID (Figure 2B) and then by its p value (Figure 2C). In glioblastoma patients, the AC003092.1 showed a positive correlation with the gene expression of its predicted target gene TFPI2 (tissue factor pathway inhibitor 2) (Figure 2D, Spearman r = 0.6, p < 0.001). A previous study had shown that AC003092.1 could upregulate the expression of TFPI2 by inhibiting the function of miR-195 (Xu et al., 2018). According to the expression of AC003092.1, the patients were divided into two groups: AC003092.1 High (AC003092.1_H) and AC003092.1 Low (AC003092.1_L) (cutoff value = 50%). Survival analyses showed that the AC003092.1_L had a better prognosis than the AC003092.1_H (Figure 2E, log-rank test, p = 0.006).
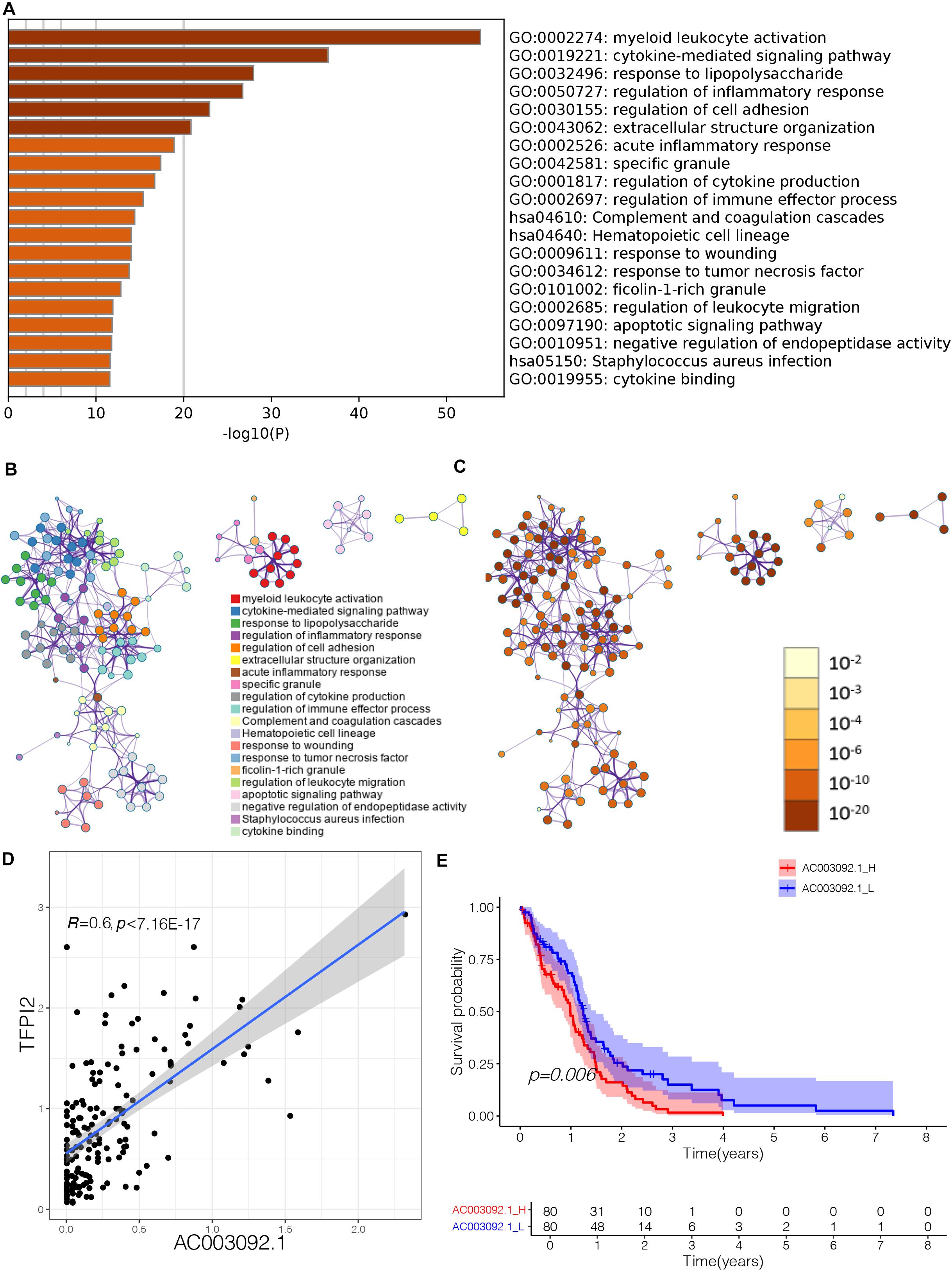
Figure 2. Effect of lncRNA AC003092.1, as an immune-related gene, in GBM. (A) Heatmap of enriched terms across input gene lists, colored by p values. (B) Enriched terms colored by cluster ID, where nodes that share the same cluster ID are typically close to each other in AC003092.1-related genes. (C) Enriched terms colored by p value, where terms containing more genes tend to have a more significant p value in AC003092.1-related genes. (D) Scatterplot showing the correlation between AC003092.1 and TFPI2 levels. (E) Comparison of overall survival between AC003092.1_H and AC003092.1_L by log-rank test.
Immunogenomic Analysis Between AC003092.1_H and AC003092.1_L
To confirm the immune-related feature between AC003092.1_H and AC003092.1_L, we analyzed 29 sets of immune-associated genes (Supplementary Table 3) representing different immune cell types, functions, and pathways in each GBM patient by ssGSEA analysis (Barbie et al., 2009; Hanzelmann et al., 2013). The results of ssGSEA indicated that immune cell types, functions, and pathways were enriched in AC003092.1_H (Figure 3A). Although the AC003092.1_H had a stronger immune function, the overall survival is better in AC003092.1_L. So, we further confirm the expression of immune checkpoints. We found that PD-L1, ICOS, and TIM-3 expressed highly in AC003092.1_H [analysis of variance (ANOVA) test, p < 0.05] (Figure 3B). Therefore, CD8+ T cells in AC003092.1_H were exhausted, and AC003092.1_H patients might get more benefit from immune checkpoint inhibitors.
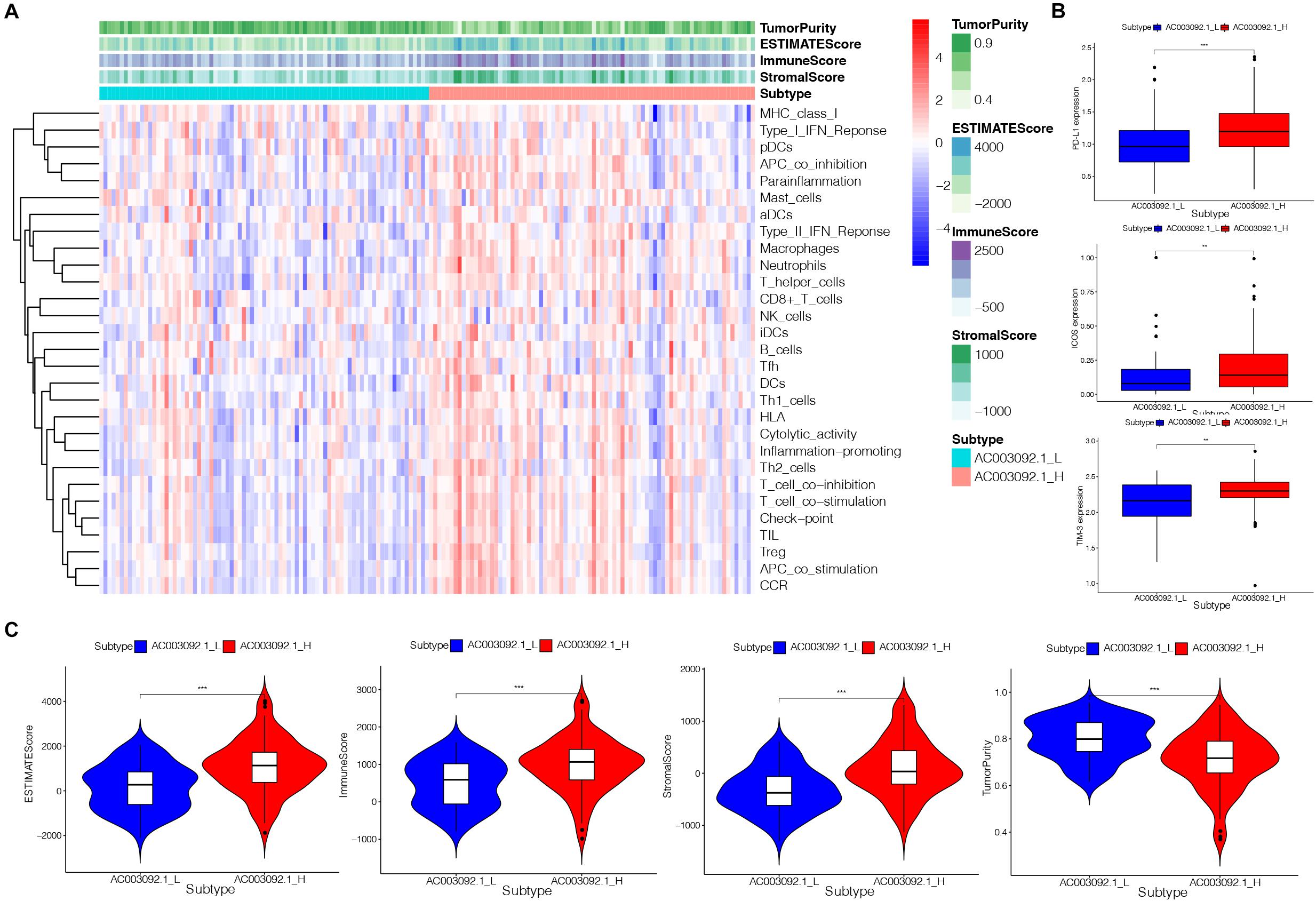
Figure 3. Immunogenomic analysis of AC003092.1_H and AC003092.1_L from TCGA. (A) The enrichment levels of the 29 immune signatures by ssGSEA score in each GBM sample. (B) Comparison of immune checkpoint gene expression levels between AC003092.1_H and AC003092.1_L (ANOVA test). (C) Comparison of the immune score, stromal score, ESTIMATE score, and tumor purity between AC003092.1_H and AC003092.1_L (Kruskal–Wallis test). *p < 0.05, **p < 0.01, ***p < 0.001.
Moreover, the immune scores, stromal scores, ESTIMATE scores, and tumor purity were compared between AC003092.1_H and AC003092.1_L by ESTIMATE. The results showed that the immune scores (Kruskal–Wallis test, p < 0.001), ESTIMATE scores (Kruskal–Wallis test, p < 0.001), and stromal scores (Kruskal–Wallis test, p < 0.001) were higher in the AC003092.1_H group. However, the tumor purity was higher in the AC003092.1_L group (Kruskal–Wallis test, p < 0.001) (Figure 3C). From the above, there were more immune and stromal cells in AC003092.1_H, whereas there were more tumor cells in AC003092.1_L.
The Landscape of Infiltrating Immune Cells in AC003092.1_H and AC003092.1_L
In order to analyze the difference of immune cell proportion between AC003092.1_H and AC003092.1_L, we applied CIBERSORTx, which is a deconvolution algorithm based on gene expression (Newman et al., 2019). CIBERSORTx was combined with LM22, which have 547 gene expression characteristics to distinguish 22 immune cell subsets and define p value of the proportions of immune cell subsets. One of the GBM patients in AC003092.1_L was excluded because of p > 0.05. According to the result of CIBERSORTx, the proportion of immune cells between AC003092.1_H and AC003092.1_L varies widely (Figure 4A). Monocytes and neutrophils had a higher proportion in the AC003092.1_H, whereas the percentages of naive B cell, T cells, follicular helper cells, and macrophages M1 were higher in AC003092.1_L (Mann–Whitney U test, p < 0.05) (Figure 4B).
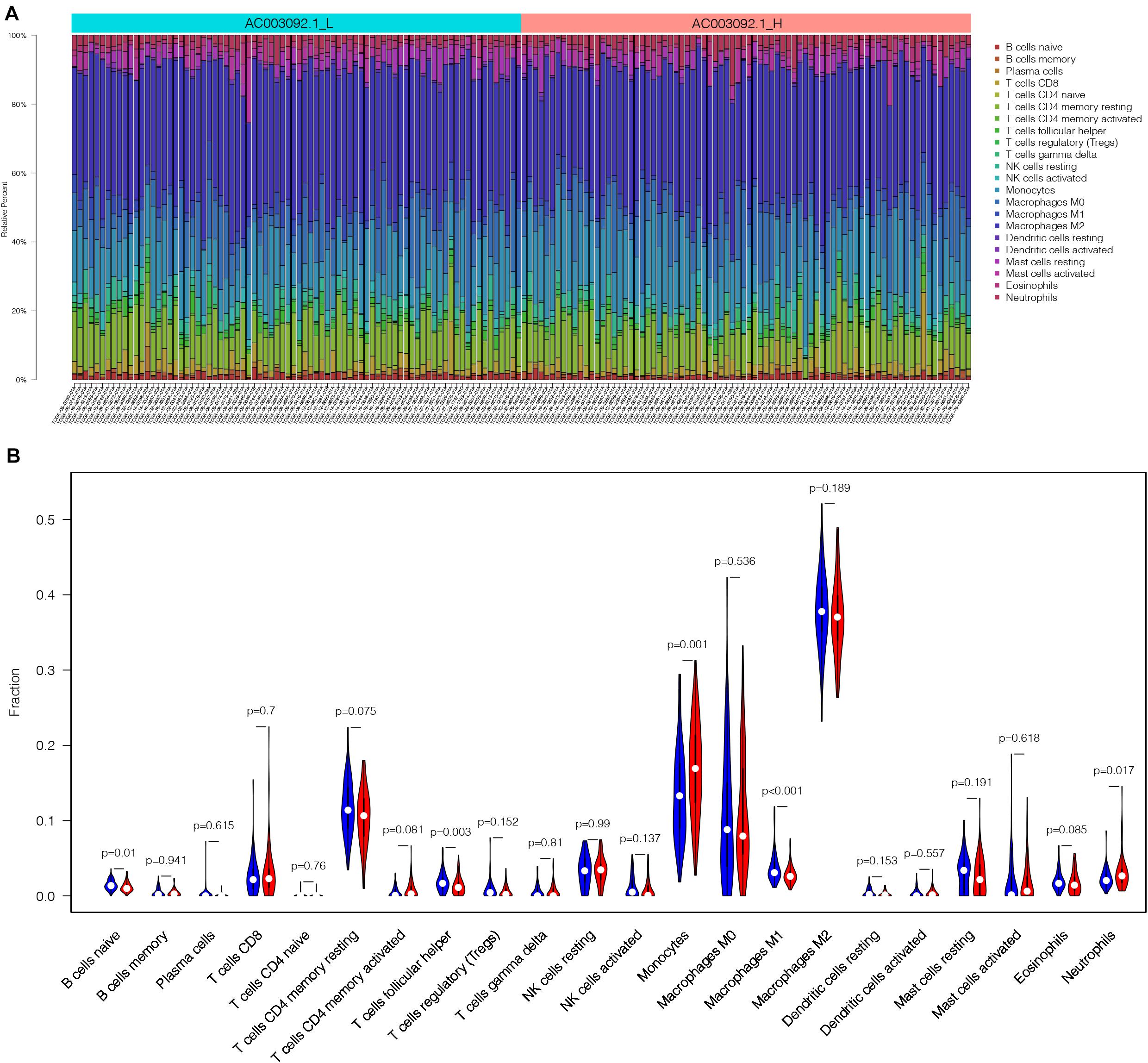
Figure 4. The landscape of infiltrating immune cells in AC003092.1_H and AC003092.1_L. (A) The bar plot of immune infiltration between AC003092.1_L and AC003092.1_H. (B) Comparison of the immune cell proportion between AC003092.1_H and AC003092.1_L by CIBERSORTx (Mann–Whitney U test).
Validation of AC003092.1 and TFPI2 Levels by RT-qPCR
The correlation between AC003092.1 and TFPI2 in 11 patients with high-grade gliomas, 5 patients with low-grade gliomas, and 7 glioblastoma cell lines was investigated using RT-qPCR. In high-grade gliomas and glioblastoma cell lines, there was a significantly positive correlation between AC003092.1 and TFPI2 (high-grade gliomas: Spearman rank correlation coefficient r = 0.8614, p < 0.001; glioblastoma cell lines: Spearman rank correlation coefficient r = 0.8200, p < 0.05) (Figures 5A,B and Supplementary Figure 1). There was no significant correlation between the two RNAs in low-grade gliomas, the same as the result in 529 low-grade gliomas of TCGA (Spearman rank correlation coefficient r = 0.8646, p > 0.05) (Figures 5C,D).
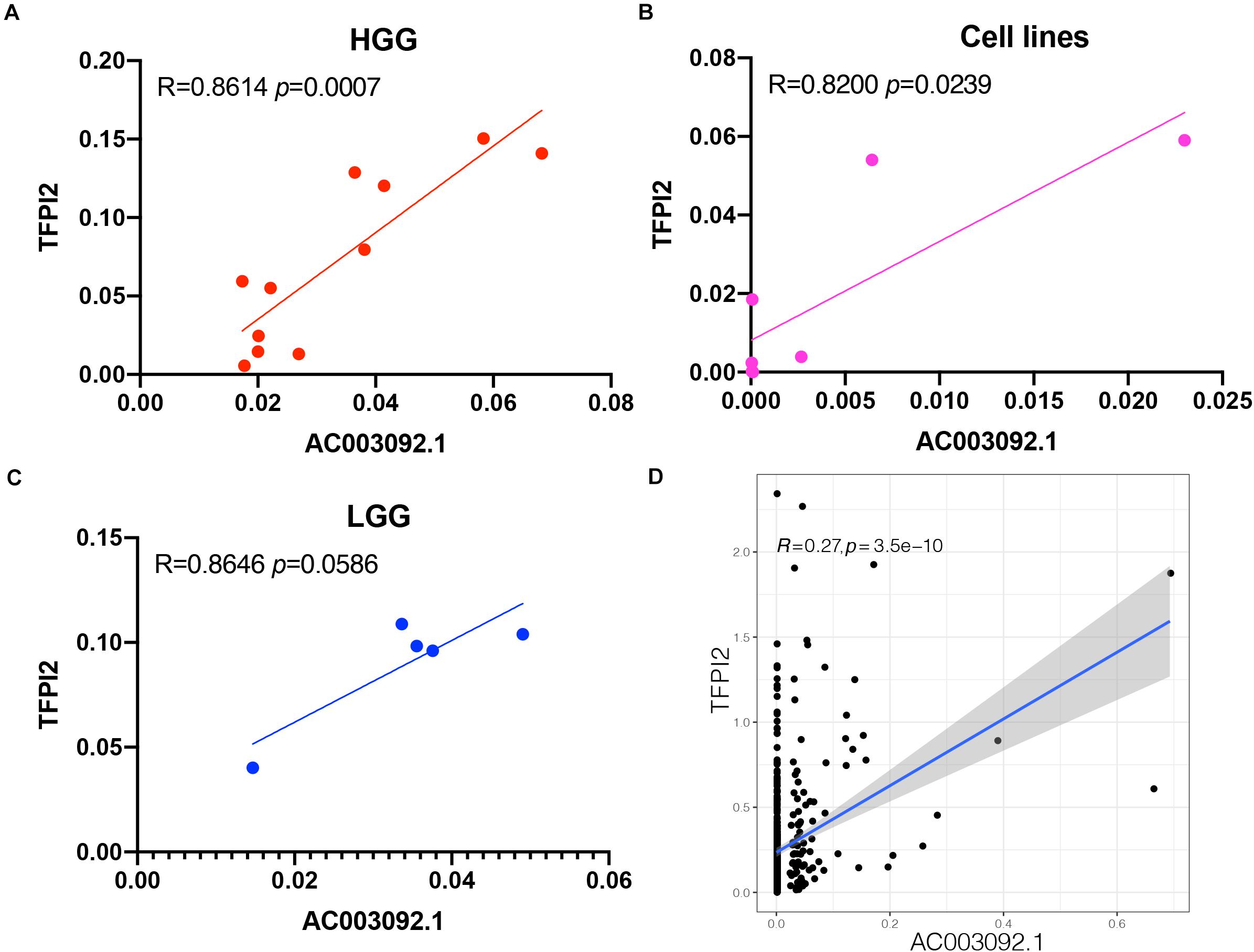
Figure 5. Validation of AC003092.1 and TFPI2 levels by RT-qPCR. Scatterplot showing correlation between AC003092.1 and TFPI2 levels in HGG (A), GBM cell lines (B), LGG (C), and patients with LGG in TCGA (D).
Discussion
eRNA as an emerging class of lncRNAs that could regulate target gene expression to influence biological process has received especially high levels of attention. In our study, we first screened prognosis-related eRNAs from glioblastoma transcriptome data through bioinformatics. Then, we identified AC003092.1 as an immune-related eRNA by GO and KEGG enrichment analysis. Moreover, we quantified the immune signature by ssGSEA and ESTIMATE. And we depicted the landscape of immune cell fractions in different groups. Finally, we validated the AC003092.1 and TFPI2 transcript levels in high-grade gliomas, low-grade gliomas, and GBM cell lines.
Instead of proliferation and differentiation, lncRNA had the ability to activate immune cells, including T cells, B cells, macrophages, monocytes, and so on (Satpathy and Chang, 2015). Lnc-Lsm3b could bind to retinoic acid–inducible gene-I to terminate type I interferon production to restrict innate immune response (Jiang et al., 2018). Moreover, lncRNA could affect tumor immune. LncRNA NKILA promoted tumor immune evasion by inhibiting nuclear factor κB activity to regulate T-cell sensitivity to activation-induced cell death (Huang et al., 2018). Recently, many studies focus on the function of lncRNAs in glioma. And some immune-related lncRNAs had been identified as genes that could regulate glioma microenvironment (Wang et al., 2018; Zhou et al., 2018; Li and Meng, 2019).
AC003092.1 as a functional lncRNA was reported by Xu et al. (2018). They found AC003092.1 located at about 32 kb from TFPI2 and revealed that TFPI2 was the target gene of AC003092.1 in temozolomide-resistant glioma cells. In subsequent study, Xu et al. demonstrated that AC003092.1 could inhibit the function of miR-195 to upregulate expression of TFPI2 and promote TMZ-induced apoptosis. In the present study, instead of chemosensitivity, AC003092.1 was potentially associated with the formation of glioblastoma immunosuppressive microenvironment. In function enrichment analysis, we found that AC003092.1-related genes were mainly enriched in immune-associated terms and pathways. And the immune-related feature showed that immune cell types, functions, and pathways were enriched in AC003092.1_H. Even so, CD8+ T cells in AC003092.1_H were exhausted because the PD-L1 and TIM-3 were expressed highly. And in previous study of transcriptional landscape of eRNA, the authors found that six checkpoints were correlated with eRNAs in at least five cancer types (Zhang et al., 2019). It had been demonstrated that colorectal cancer–associated transcript 1-long isoform (CCAT1-L) as a super-enhancer RNA (a cluster of eRNA) could bind at MYC to induce many pro-proliferative genes and maintain cancer cell survival by exempting from immune surveillance and the antitumor immune response (Cotterman et al., 2008; Kim et al., 2017). Thus, eRNA had putative interaction with immune checkpoints and could be a potential therapeutic target in malignancy treatment.
GO:0002274: myeloid leukocyte activation was the most significant terms that AC003092.1-related genes enriched. At the same time, we found that the proportions of monocytes, macrophages, and neutrophils were notably different between AC003092.1_H and AC003092.1_L.
Tissue factor pathway inhibitor 2, a 32-kDa matrix-associated Kunitz-type serine protease inhibitor, was encoded by the TFPI2 gene. The expression of TFPI2 was negatively correlated with tumor invasion and proliferation (Sierko et al., 2007). Thus, TFPI2 was regarded as a tumor suppressor gene. TFPI2 was also related to the occurrence and development of glioma. In glioblastoma, AGAP2-AS1 could epigenetically silence TFPI2 expression by binding to EZH2 and LSD1 to promote GBM (Luo et al., 2019). TFPI2 had conserved C-terminal region. Kasetty et al. (2016) demonstrated that the C-terminal TFPI-2–derived peptides from different vertebrates could exert antibacterial effects through the complement activation. The neutrophil elastase could generate a C-terminal TFPI-2 fragment, which interacted with bacteria to inhibit microorganism growth (Papareddy et al., 2012). The expression of TFPI2 in human umbilical vein endothelial cell was significantly upregulated after stimulation with inflammatory mediators such as Phorbol-12-myristate-13-acetate (PMA), lipopolysaccharide, and tumor necrosis factor α (Iino et al., 1998). Research had shown that recombinant TFPI-2 could promote U937-derived macrophage apoptosis by upregulation of Fas/FsaL (Pan et al., 2011). Papareddy et al. (2012) demonstrated that TFPI2 existed in skin and would be upregulated during the wounding. These researches revealed that TFPI2 could participate in the immune response in different ways. So, we hypothesized that although AC003092.1 was a non-coding RNA, it could upregulate TFPI2 expression to affect GBM immune response.
Conclusion
According to our study, we found that AC003092.1 was an immune-related gene in glioblastoma patients, and the genes that correlated to AC003092.1 are enriched in immune-associated terms and pathways especially in myeloid leukocyte activation. Immune cell types, functions, and pathways were enriched in AC003092.1_H by ssGSEA. And the present study indicated that AC003092.1 had a close relationship with monocytes, macrophages, and neutrophils. The results of qRT-PCR validated the AC003092.1 and TFPI2 levels in high-grade glioma and glioblastoma cell lines. This provides a new idea for studying the immunosuppressive microenvironment of glioblastoma.
Data Availability Statement
Publicly available datasets were analyzed in this study. This data can be found here: https://portal.gdc.cancer.gov/.
Ethics Statement
The studies involving human participants were reviewed and approved by the Ethics Committee of Sun Yat-sen University Cancer Center. The patients/participants provided their written informed consent to participate in this study.
Author Contributions
X-YG, SZ, and Z-NW participated in the design of the study, performed the statistical analysis, interpretation of data, and drafted the manuscript. HD, YW, and J-JD collected and organized the data. TX, J-YZ, and G-HZ participated in the design of the study and performed the statistical analysis. LL, RC, H-RH, and JL carried out the qRT-PCR. Z-QH and Y-GM conceived of the study, and revised and approved the final manuscript. All authors read and approved the final manuscript.
Funding
This work was supported by the National Natural Science Foundation of China (grant number 81872324), Guangdong Basic and Applied Basic Research Foundation (2020A1515110203), Fundamental Research Funds for Junior Teachers in Sun Yat-sen University, China (20ykpy163), Science and Technology Program of Guangzhou, China (grant number 201704020133), Science and Technology Program of Jiangmen, China (grant number 2018630100110019805), Medical Scientific Research Foundation of Guangdong Province of China (A2020149), and Science and Technology Planning Project of Guangdong Province, China (grant number 2015B010125003).
Conflict of Interest
The authors declare that the research was conducted in the absence of any commercial or financial relationships that could be construed as a potential conflict of interest.
Supplementary Material
The Supplementary Material for this article can be found online at: https://www.frontiersin.org/articles/10.3389/fgene.2021.633812/full#supplementary-material
Supplementary Figure 1 | The level of expression of AC003092.1 and TFPI2 in each single cell line.
Footnotes
References
Ashburner, M., Ball, C. A., Blake, J. A., Botstein, D., Butler, H., Cherry, J. M., et al. (2000). Gene ontology: tool for the unification of biology. the gene ontology consortium. Nat. Genet. 25, 25–29. doi: 10.1038/75556
Barbie, D. A., Tamayo, P., Boehm, J. S., Kim, S. Y., Moody, S. E., Dunn, I. F., et al. (2009). Systematic RNA interference reveals that oncogenic KRAS-driven cancers require TBK1. Nature 462, 108–112. doi: 10.1038/nature08460
Cardon, T., Franck, J., Coyaud, E., Laurent, E. M. N., Damato, M., Maffia, M., et al. (2020). Alternative proteins are functional regulators in cell reprogramming by PKA activation. Nucleic Acids Res. 48, 7864–7882. doi: 10.1093/nar/gkaa277
Corradin, O., Saiakhova, A., Akhtar-Zaidi, B., Myeroff, L., Willis, J., Cowper-Sal lari, R., et al. (2014). Combinatorial effects of multiple enhancer variants in linkage disequilibrium dictate levels of gene expression to confer susceptibility to common traits. Genome Res. 24, 1–13. doi: 10.1101/gr.164079.113
Cotterman, R., Jin, V. X., Krig, S. R., Lemen, J. M., Wey, A., Farnham, P. J., et al. (2008). N-Myc regulates a widespread euchromatic program in the human genome partially independent of its role as a classical transcription factor. Cancer Res. 68, 9654–9662. doi: 10.1158/0008-5472.CAN-08-1961
Cuddapah, V. A., Robel, S., Watkins, S., and Sontheimer, H. (2014). A neurocentric perspective on glioma invasion. Nat. Rev. Neurosci. 15, 455–465. doi: 10.1038/nrn3765
Durinck, S., Spellman, P. T., Birney, E., and Huber, W. (2009). Mapping identifiers for the integration of genomic datasets with the R/Bioconductor package biomaRt. Nat. Protoc. 4, 1184–1191. doi: 10.1038/nprot.2009.97
Gene Ontology Consortium (2015). Gene ontology consortium: going forward. Nucleic Acids Res. 43, D1049–D1056. doi: 10.1093/nar/gku1179
Hanzelmann, S., Castelo, R., and Guinney, J. (2013). GSVA: gene set variation analysis for microarray and RNA-seq data. BMC Bioinformatics 14:7. doi: 10.1186/1471-2105-14-7
Hsieh, C. L., Fei, T., Chen, Y., Li, T., Gao, Y., Wang, X., et al. (2014). Enhancer RNAs participate in androgen receptor-driven looping that selectively enhances gene activation. Proc. Natl. Acad. Sci. U.S.A. 111, 7319–7324. doi: 10.1073/pnas.1324151111
Huang, D., Chen, J., Yang, L., Ouyang, Q., Li, J., Lao, L., et al. (2018). NKILA lncRNA promotes tumor immune evasion by sensitizing T cells to activation-induced cell death. Nat. Immunol. 19, 1112–1125. doi: 10.1038/s41590-018-0207-y
Iino, M., Foster, D. C., and Kisiel, W. (1998). Quantification and characterization of human endothelial cell-derived tissue factor pathway inhibitor-2. Arterioscler. Thromb. Vasc. Biol. 18, 40–46. doi: 10.1161/01.atv.18.1.40
IIott, N. E., Heward, J. A., Roux, B., Tsitsiou, E., Fenwick, P. S., Lenzi, L., et al. (2014). Long non-coding RNAs and enhancer RNAs regulate the lipopolysaccharide-induced inflammatory response in human monocytes. Nat. Commun. 5:3979. doi: 10.1038/ncomms4979
Jiang, M., Zhang, S., Yang, Z., Lin, H., Zhu, J., Liu, L., et al. (2018). Self-Recognition of an inducible host lncRNA by RIG-I feedback restricts innate immune response. Cell 173, 906–919.e13. doi: 10.1016/j.cell.2018.03.064
Kasetty, G., Smeds, E., Holmberg, E., Wrange, L., Adikesavan, S., Papareddy, P., et al. (2016). Vertebrate TFPI-2 C-terminal peptides exert therapeutic applications against Gram-negative infections. BMC Microbiol. 16:129. doi: 10.1186/s12866-016-0750-3
Kim, E. Y., Kim, A., Kim, S. K., and Chang, Y. S. (2017). MYC expression correlates with PD-L1 expression in non-small cell lung cancer. Lung Cancer 110, 63–67. doi: 10.1016/j.lungcan.2017.06.006
Lebrec, J. J., Huizinga, T. W., Toes, R. E., Houwing-Duistermaat, J. J., and van Houwelingen, H. C. (2009). Integration of gene ontology pathways with North American Rheumatoid Arthritis Consortium genome-wide association data via linear modeling. BMC Proc. 3 (Suppl 7):S94. doi: 10.1186/1753-6561-3-s7-s94
Li, W., Notani, D., and Rosenfeld, M. G. (2016). Enhancers as non-coding RNA transcription units: recent insights and future perspectives. Nat. Rev. Genet. 17, 207–223. doi: 10.1038/nrg.2016.4
Li, X., and Meng, Y. (2019). Survival analysis of immune-related lncRNA in low-grade glioma. BMC Cancer 19:813. doi: 10.1186/s12885-019-6032-3
Long, H. K., Prescott, S. L., and Wysocka, J. (2016). Ever-Changing landscapes: transcriptional enhancers in development and evolution. Cell 167, 1170–1187. doi: 10.1016/j.cell.2016.09.018
Lu, S., Zhang, J., Lian, X., Sun, L., Meng, K., Chen, Y., et al. (2019). A hidden human proteome encoded by ‘non-coding’ genes. Nucleic Acids Res. 47, 8111–8125. doi: 10.1093/nar/gkz646
Luo, W., Li, X., Song, Z., Zhu, X., and Zhao, S. (2019). Long non-coding RNA AGAP2-AS1 exerts oncogenic properties in glioblastoma by epigenetically silencing TFPI2 through EZH2 and LSD1. Aging 11, 3811–3823. doi: 10.18632/aging.102018
Matsumoto, A., Pasut, A., Matsumoto, M., Yamashita, R., Fung, J., Monteleone, E., et al. (2017). mTORC1 and muscle regeneration are regulated by the LINC00961-encoded SPAR polypeptide. Nature 541, 228–232. doi: 10.1038/nature21034
Newman, A. M., Steen, C. B., Liu, C. L., Gentles, A. J., Chaudhuri, A. A., Scherer, F., et al. (2019). Determining cell type abundance and expression from bulk tissues with digital cytometry. Nat. Biotechnol. 37, 773–782. doi: 10.1038/s41587-019-0114-2
Pan, J. J., Shi, H. M., Luo, X. P., Ma, D., Li, Y., Zhu, J., et al. (2011). Recombinant TFPI-2 enhances macrophage apoptosis through upregulation of Fas/FasL. Eur. J. Pharmacol. 654, 135–141. doi: 10.1016/j.ejphar.2010.12.015
Papareddy, P., Kalle, M., Sørensen, O. E., Lundqvist, K., Mörgelin, M., Malmsten, M., et al. (2012). Tissue factor pathway inhibitor 2 is found in skin and its C-terminal region encodes for antibacterial activity. PLoS One 7:e52772. doi: 10.1371/journal.pone.0052772
Peng, Z., Liu, C., and Wu, M. (2018). New insights into long noncoding RNAs and their roles in glioma. Mol. Cancer 17:61. doi: 10.1186/s12943-018-0812-2
Ransohoff, J. D., Wei, Y., and Khavari, P. A. (2018). The functions and unique features of long intergenic non-coding RNA. Nat. Rev. Mol. Cell Biol. 19, 143–157. doi: 10.1038/nrm.2017.104
Rion, N., and Ruegg, M. A. (2017). LncRNA-encoded peptides: More than translational noise? Cell Res. 27, 604–605. doi: 10.1038/cr.2017.35
Satpathy, A. T., and Chang, H. Y. (2015). Long noncoding RNA in hematopoiesis and immunity. Immunity 42, 792–804. doi: 10.1016/j.immuni.2015.05.004
Sierko, E., Wojtukiewicz, M. Z., and Kisiel, W. (2007). The role of tissue factor pathway inhibitor-2 in cancer biology. Semin. Thromb. Hemost. 33, 653–659. doi: 10.1055/s-2007-991532
Smedley, D., Haider, S., Ballester, B., Holland, R., London, D., Thorisson, G., et al. (2009). BioMart–biological queries made easy. BMC Genomics 10:22. doi: 10.1186/1471-2164-10-22
Spizzo, R., Almeida, M. I., Colombatti, A., and Calin, G. A. (2012). Long non-coding RNAs and cancer: a new frontier of translational research? Oncogene 31, 4577–4587. doi: 10.1038/onc.2011.621
Vucicevic, D., Corradin, O., Ntini, E., Scacheri, P. C., and Orom, U. A. (2015). Long ncRNA expression associates with tissue-specific enhancers. Cell Cycle 14, 253–260. doi: 10.4161/15384101.2014.977641
Wang, W., Zhao, Z., Yang, F., Wang, H., Wu, F., Liang, T., et al. (2018). An immune-related lncRNA signature for patients with anaplastic gliomas. J. Neurooncol. 136, 263–271. doi: 10.1007/s11060-017-2667-6
Wapinski, O., and Chang, H. Y. (2011). Long noncoding RNAs and human disease. Trends Cell Biol. 21, 354–361. doi: 10.1016/j.tcb.2011.04.001
Weller, M., Wick, W., Aldape, K., Brada, M., Berger, M., Pfister, S. M., et al. (2015). Glioma. Nat. Rev. Dis. Primers 1:15017. doi: 10.1038/nrdp.2015.17
Xu, N., Liu, B., Lian, C., Doycheva, D. M., Fu, Z., Liu, Y., et al. (2018). Long noncoding RNA AC003092.1 promotes temozolomide chemosensitivity through miR-195/TFPI-2 signaling modulation in glioblastoma. Cell Death Dis. 9:1139. doi: 10.1038/s41419-018-1183-8
Yoshihara, K., Shahmoradgoli, M., Martínez, E., Vegesna, R., Kim, H., Torres-Garcia, W., et al. (2013). Inferring tumour purity and stromal and immune cell admixture from expression data. Nat. Commun. 4:2612. doi: 10.1038/ncomms3612
Zhang, Z., Lee, J. H., Ruan, H., Ye, Y., Krakowiak, J., Hu, Q., et al. (2019). Transcriptional landscape and clinical utility of enhancer RNAs for eRNA-targeted therapy in cancer. Nat. Commun. 10:4562. doi: 10.1038/s41467-019-12543-5
Zhou, M., Zhang, Z., Zhao, H., Bao, S., Cheng, L., Sun, J., et al. (2018). An Immune-Related Six-lncRNA Signature to Improve Prognosis Prediction of Glioblastoma Multiforme. Mol. Neurobiol. 55, 3684–3697. doi: 10.1007/s12035-017-0572-9
Keywords: lncRNA, eRNA, glioblastoma multiforme, AC003092.1, TFPI2, immunogenomic
Citation: Guo X-Y, Zhong S, Wang Z-N, Xie T, Duan H, Zhang J-Y, Zhang G-H, Liang L, Cui R, Hu H-R, Lu J, Wu Y, Dong J-J, He Z-Q and Mou Y-G (2021) Immunogenomic Profiling Demonstrate AC003092.1 as an Immune-Related eRNA in Glioblastoma Multiforme. Front. Genet. 12:633812. doi: 10.3389/fgene.2021.633812
Received: 26 November 2020; Accepted: 16 February 2021;
Published: 18 March 2021.
Edited by:
Daniele Vergara, University of Salento, ItalyReviewed by:
Tristan Cardon, Institut National de la Santé et de la Recherche Médicale (INSERM), FranceMarina Damato, University of Salento, Italy
Copyright © 2021 Guo, Zhong, Wang, Xie, Duan, Zhang, Zhang, Liang, Cui, Hu, Lu, Wu, Dong, He and Mou. This is an open-access article distributed under the terms of the Creative Commons Attribution License (CC BY). The use, distribution or reproduction in other forums is permitted, provided the original author(s) and the copyright owner(s) are credited and that the original publication in this journal is cited, in accordance with accepted academic practice. No use, distribution or reproduction is permitted which does not comply with these terms.
*Correspondence: Yong-Gao Mou, bW91eWdAc3lzdWNjLm9yZy5jbg==; Zhen-Qiang He, aGV6aGVucUBzeXN1Y2Mub3JnLmNu
†These authors have contributed equally to this work