- 1Department of Surgical Sciences, School of Veterinary Medicine, University of Wisconsin-Madison, Madison, WI, United States
- 2Bioinformatics Resource Center, Biotechnology Center, University of Wisconsin-Madison, Madison, WI, United States
Anterior cruciate ligament (ACL) rupture is a common condition that disproportionately affects young people, 50% of whom will develop knee osteoarthritis (OA) within 10 years of rupture. ACL rupture exhibits both hereditary and environmental risk factors, but the genetic basis of the disease remains unexplained. Spontaneous ACL rupture in the dog has a similar disease presentation and progression, making it a valuable genomic model for ACL rupture. We leveraged the dog model with Bayesian mixture model (BMM) analysis (BayesRC) to identify novel and relevant genetic variants associated with ACL rupture. We performed RNA sequencing of ACL and synovial tissue and assigned single nucleotide polymorphisms (SNPs) within differentially expressed genes to biological prior classes. SNPs with the largest effects were on chromosomes 3, 5, 7, 9, and 24. Selection signature analysis identified several regions under selection in ACL rupture cases compared to controls. These selection signatures overlapped with genome-wide associations with ACL rupture as well as morphological traits. Notable findings include differentially expressed ACSF3 with MC1R (coat color) and an association on chromosome 7 that overlaps the boundaries of SMAD2 (weight and body size). Smaller effect associations were within or near genes associated with regulation of the actin cytoskeleton and the extracellular matrix, including several collagen genes. The results of the current analysis are consistent with previous work published by our laboratory and others, and also highlight new genes in biological pathways that have not previously been associated with ACL rupture. The genetic associations identified in this study mirror those found in human beings, which lays the groundwork for development of disease-modifying therapies for both species.
Introduction
The anterior cruciate ligament (ACL) is a ligament spanning from the lateral femoral condyle to the proximal tibia that provides crucial stability to the knee joint, counteracting anterior translation, hyperextension, and internal rotation of the tibia (Noyes, 2009). ACL rupture is most often a midsubstance failure of this ligament (van der List et al., 2017), which occurs for multiple and complex reasons including genetic predisposition (Smith et al., 2012a,b). Standard of care includes physical therapy alone or after surgical reconstruction. Unfortunately, neither treatment prevents the long-term development of post-traumatic osteoarthritis (OA) (Lohmander et al., 2007) and disease-modifying therapies are critically needed. The key to disease-modifying therapy may lie within the underlying genetic predisposition to ACL rupture. Multiple studies have been performed in search of genetic drivers of disease, but discoveries have been limited, mostly due to inadequate sample composition (e.g., male-only samples) and size (John et al., 2016).
Anterior cruciate ligament rupture in the dog is a useful genomic model for human ACL rupture. The onset and progression of the condition is remarkably similar between humans and dogs (Baker et al., 2018). There are several advantages to the dog as a genomic model for ACL rupture that have been discussed previously (Baker et al., 2017, 2018), including higher disease prevalence (Witsberger et al., 2008), established heritability of the disease (Nielen et al., 2003; Wilke et al., 2006; Baker et al., 2017), and within breed homogeneity and extensive linkage disequilibrium (LD) (Karlsson and Lindblad-Toh, 2008). While genome-wide association studies (GWAS) have been performed (Wilke et al., 2009; Baird et al., 2014a,b; Hayward et al., 2016, 2019; Baker et al., 2017, 2018; Huang et al., 2017), the associations identified have not been repeatable from one study to the next. Our previous work on the genetic basis of ACL rupture in the Labrador Retriever (Baker et al., 2017, 2018) supports the hypothesis that ACL rupture is highly polygenic, and that most, if not all, single nucleotide polymorphisms (SNP) effects are relatively small. While we have identified some reasonable candidate genes, the majority of the identified associations do not have clear relevance to ACL rupture.
In the present study, we embrace the polygenicity of ACL rupture with a Bayesian approach to GWAS. In contrast to traditional single-marker models [e.g., linear mixed models (LMM)], Bayesian models for GWAS estimate the combined effect of all SNPs in the dataset. A Bayesian approach could treat all SNP associations as random effects drawn from a normal distribution which allows for an unbiased estimate of variance explained by the SNPs (Moser et al., 2015). This approach can be tailored further to GWAS of complex phenotypes by treating SNP effects as drawn from a mixture of normal distributions corresponding to differing SNP effect sizes, including a distribution for SNPs with zero effect. BayesR (Erbe et al., 2012; Moser et al., 2015) is one such implementation that models SNP effects using four normal distributions with variance ranging from zero to 1% of total genetic variance, which more accurately models the effect size distribution expected from a complex phenotype. BayesR has been shown to be equal or superior to the LMM for both prediction modeling and QTL mapping (Moser et al., 2015; Kemper et al., 2015).
Another advantage of the Bayesian approach to GWAS is the ease with which prior biological information can be incorporated into the model (Stephens and Balding, 2009). Most single marker models, including the LMM, assume each SNP has an equal probability of having an effect on the phenotype of interest. However, SNPs that are within or near candidate genes may have a higher probability of being associated with the phenotype. Bayesian models allow the user to set a higher prior probability of effect to these SNPs. While there is some subjectivity to assigning prior probabilities, this is an improvement from the arguably arbitrary way biological knowledge is used to interpret results after GWAS analysis (Stephens and Balding, 2009; MacLeod et al., 2016; Gallagher and Chen-Plotkin, 2018). BayesRC (MacLeod et al., 2016) is a modification to BayesR that can incorporate prior biological knowledge as a part of the analysis. To do this, SNPs are assigned to separate classes, defined by the user, based on whether the classes differ in the prior likelihood that they contain variants that are associated with the phenotype. This method improves the power and precision to detect associated variants when compared to BayesR (MacLeod et al., 2016).
The purpose of this study was to incorporate knowledge of ACL rupture candidate genes with BayesRC GWAS to identify and prioritize genetic variants with clear relevance to the disease process and evaluate the repeatability of associations previously reported in the literature. We defined candidate genes through differential gene expression analysis of RNA sequencing data and published literature. SNPs within candidate genes were assigned to biological priors. We discovered associations in genes from molecular pathways that were not previously implicated in ACL rupture pathogenesis and replicated associations that have been previously reported in studies of ACL rupture in both human beings and dogs. Many of these associations are within haplotypes that are under selection in the Labrador Retriever.
Materials and Methods
Data Collection and Phenotyping
All procedures were performed in accordance with the recommendations in the Guide for the Care and Use of Laboratory Animals of the National Institutes of Health and the American Veterinary Medical Association and with approval from the Animal Care Committee of the University of Wisconsin-Madison (protocols V1070 and V5463). Informed consent of each owner was obtained before participation in the study. Recruitment and quality control have been reported in previous publications by our laboratory (Baker et al., 2017, 2018). Client-owned Labrador Retriever dogs (Canis lupus familiaris) were recruited from the University of Wisconsin-Madison UW Veterinary Care teaching hospital, online advertising, and through local and national breed clubs. If available, a pedigree was collected from each dog to confirm purebred status. A single ACL rupture was sufficient to consider a dog a case. All cases were diagnosed by a veterinarian. In the vast majority of cases, a ruptured ACL was confirmed during knee stabilization surgery. Control dogs were over the age of 8 years (Reif and Probst, 2003) with a normal orthopaedic exam and knee radiographs with no evidence of ACL rupture (joint effusion or osteophytosis) (Chuang et al., 2014). Age, weight, and whether the dog was spayed or neutered were recorded. DNA was extracted from blood or saliva. Dogs were genotyped using the Illumina Canine HD BeadChip (220,000 SNPs).
Imputation
Dogs genotyped on the Illumina HD BeadChip were imputed to the higher density Thermo Fisher Scientific Axiom Canine HD array (710,000 SNPs) using Beagle 5.0 (Browning et al., 2018) and a previously described method (Friedenberg and Meurs, 2016). Our reference panel consisted of N = 646 dogs that were genotyped on a pre-commercial version of the Axiom array, including 96 Labrador Retrievers. These data were obtained from the laboratory of Dr. Brian Davis of Texas A&M University and are the subject of an unpublished research project and are therefore not currently available to the public. To validate the imputation method in our population, we used whole-genome sequencing (WGS) data from n = 22 Labrador Retrievers that were sequenced for an unrelated project in our laboratory. 173,662 SNPs, present on the Illumina Canine HD BeadChip, were extracted from WGS data to create a test set for the imputation method. The test dataset was imputed with the multibreed reference, a window size of 3 cm with a 1 cm overlap, and effective population size of 100. The effective population size of the Labrador Retriever was based on results from two studies, one that states the effective population size is 114 (Calboli et al., 2008), and another more recent study that states the effective population size is 82 (Wiener et al., 2017). To evaluate accuracy, bi-allelic genotypes at imputed SNPs from the 22 Labrador Retrievers with WGS data were compared to the genotypes at the same SNP locations extracted from WGS data. If the complete imputed genotype matched the complete WGS genotype, the SNP genotype was scored as correct. Accuracy of imputation was calculated per chromosome as number of genotypes imputed correctly divided by the number of genotypes compared. Overall, accuracy exceeded 90% for all autosomes, and the vast majority of autosomes (36/38) achieved accuracy of 96% or higher (Supplementary Table 1). Given these acceptable results, we moved forward with imputation of our study dataset using Beagle 5.0 and the aforementioned parameters.
Our final dataset included 397 (156 ACL rupture affected and 241 unaffected control) purebred Labrador Retriever dogs. Of these, 55 were intact males, 30 were intact females, 161 were castrated males, and 151 were ovariohysterectomized females. A total of 237 dogs were part of a previously published GWAS analysis (Baker et al., 2017). Quality control on the imputed data was performed using PLINK v1.9 (Chang et al., 2015). SNPs were removed from the dataset if they had minor allele frequency (MAF) < 0.01, genotyping call rate <90%, or did not conform to Hardy–Weinberg proportions at a P-value less than 1E-07. Because BayesRC does not tolerate missing genotypes, SNPs with any missing genotypes were also removed from the dataset. After quality control 443,227 SNPs remained for analysis.
RNA Sequencing and Differential Gene Expression Analysis
Anterior cruciate ligament and knee synovial tissue biopsies were collected from four ACL rupture affected cases and four unaffected control dogs. These dogs were recruited under the same phenotyping criteria that was established for genotyping. It was important to examine both ACL and synovium, as synovitis is known to precede ACL rupture in the dog (Bleedorn et al., 2011) and may play a role in disease progression and development of OA (Comerford et al., 2011). Cases and controls were matched as closely as possible based on breed, sex, neutered status, age, and weight. Medications that the dogs were taking at the time of sample collection were also considered. We prioritized sample size and quality above all other variables, therefore, two matched pairs of Golden Retrievers were chosen with two matched pairs of Labrador Retrievers for this analysis (Table 1). In phylogenetic terms, the Golden Retriever is closely related to the Labrador Retriever (Parker et al., 2017). Tissues from cases were collected during knee stabilization surgery. Tissues from unaffected control dogs were collected from dogs undergoing pelvic limb amputation or euthanasia for reasons unrelated to this study. Library preparation and sequencing was performed at the University of Wisconsin-Madison Biotechnology Center (Madison, WI, United States). Illumina TruSeq RNA libraries were constructed and 150 bp paired-end sequencing was performed using the Illumina Hi-Seq 2500 platform. Read quality was evaluated using FastQC (Andrews, 2010).

Table 1. Breed, sex, age, and weight of matched case and control pairs chosen for RNA sequencing analysis.
Bioinformatic analysis of RNASeq reads adhered to ENCODE guidelines and best practices for RNASeq (Encode Consortium,, 2016). Briefly, alignment of adapter-trimmed (Skewer v0.1.123) (Jiang et al., 2014) 2 × 150 bp paired-end strand-specific Illumina reads to the canFam3.1 genome (assembly accession: GCA_000002285.2) was achieved with the Spliced Transcripts Alignment to a Reference (STAR v2.5.3a) software (Dobin et al., 2013), and a splice-junction aware aligner using Ensembl annotation (Aken et al., 2016). Expression estimation was conducted using RSEM v1.3.0 (RNASeq by Expectation Maximization) (Li and Dewey, 2011). To test for differential expression among individual group contrasts, expected read counts were used as input into edgeR v3.16.5 (Robinson et al., 2010). Significance of the negative-binomial test was adjusted with a Benjamini–Hochberg false discovery rate (FDR) correction at the 5% level (Reiner et al., 2003). Before statistical analysis with edgeR, independent filtering was performed, requiring a threshold of at least 1 read per million in two or more samples. The validity of the Benjamini–Hochberg FDR multiple testing procedure was evaluated by inspection of the uncorrected P-value distribution. Lists of differentially expressed genes (DEGs) were submitted for pathway analysis using the PANTHER classification system v15.0 (Mi et al., 2013; Mi et al., 2019) to analyze for statistical overrepresentation using the Fisher’s Exact test. Significance was defined as P < 0.05 after correction for FDR.
Association Analysis and Assignment of Biological Priors
We used the BayesRC algorithm (MacLeod et al., 2016) to perform a genome-wide association analysis that incorporated prior biological knowledge. A copy of the software was obtained electronically via Dr. Iona MacLeod (MacLeod et al., 2016). BayesRC is an extension of the Bayesian mixture model (BMM) BayesR (Erbe et al., 2012; Moser et al., 2015). The BayesR algorithm assumes that SNP effects are derived from a mixture of four normal distributions including a zero-effect distribution. The three effect distributions are N(0, ), N(0, ), and N(0, ), with representing the additive genetic variance explained by the SNPs. This mixture of distributions approximates the various SNP effect sizes that would typically describe the underlying genetic architecture of complex traits (Moser et al., 2015). A Markov Chain Monte Carlo (MCMC) approach is used to estimate SNP effects from the four distributions. As the algorithm runs, it uses the data to estimate the probability that each SNP belongs within distribution 1, 2, 3, and 4, and updates the proportions each iteration.
To incorporate biological information, BayesRC directs the user to assign each SNP to a class (of 2 or more classes) where each class represents some biological information. For example, SNPs within the boundaries of candidate genes could be assigned to one class, and all other SNPs would be assigned to separate class. SNPs that receive the same class assignment are analyzed together, and each class is analyzed separately from other classes. The BayesRC algorithm updates the distribution of SNP effects within each class and separate from other classes, which is an advantage if any one class is enriched for associated loci. A uniform prior is applied across all classes to ensure that biological information only influences the analysis if the data supports it (MacLeod et al., 2016). We used a mostly uninformative Dirichlet prior [α = (1,1,1,1)]across classes to define the prior proportion of SNPs in each distribution (MacLeod et al., 2016).
We defined a total of five biological prior classes using the results of our RNASeq analysis and peer-reviewed literature (Table 2 and Supplementary Figure 1). Three biological prior classes were defined using candidate genes identified through RNASeq and differential gene expression analysis: DEGs in ACL (“ACL”), DEGs in knee synovium (“SYN”), and DEGs identified in both tissues (“A&S”). A fourth class was defined using candidate genes that have been reported in peer-reviewed literature as associated with ACL rupture or tendinopathy in humans and/or dogs (“LIT,” Table 3). SNPs were assigned to a class if they were within the boundaries of a candidate gene ±25 kb. The size of the flanking region was conservatively defined by calculating the average haplotype block size in our data using PLINK, which was 19.43 kb with a maximum haplotype block size of 200 kb. Gene boundaries were based on canFam3.1 from Ensembl release 97 using the python package PyEnsembl v1.7.5.
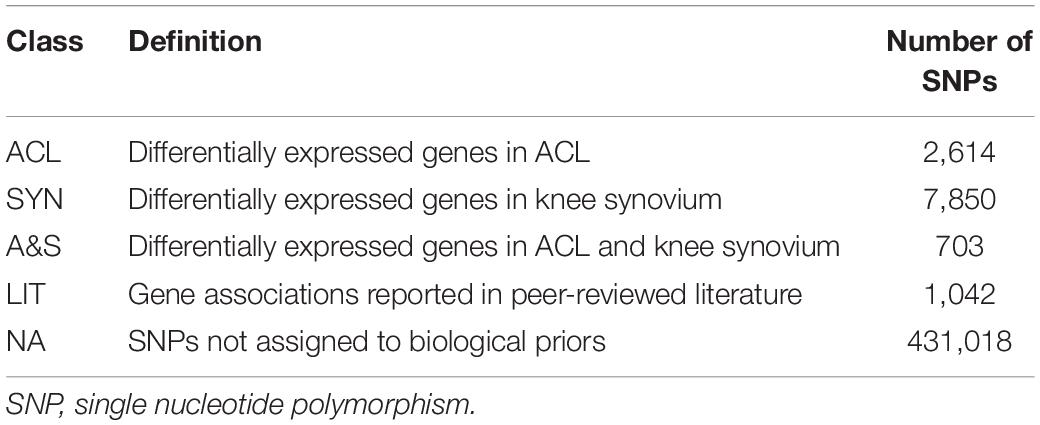
Table 2. The number of SNPs assigned to biological priors defined by differential gene expression analysis and candidate genes reported in the literature.
Because Labrador Retrievers in the current dataset were present in the datasets of our previously published work [Baker et al., 2017 (N = 237 dogs), 2018 (N = 222 dogs)], candidate genes identified through significant associations from our previous studies were not included in the peer-reviewed literature class to avoid introducing bias. These genes included PPP1R16B (Baker et al., 2017), DOCK2 (Baker et al., 2018), and ROR2 (Baker et al., 2018). We have previously reported a weak association with the gene ACAN (Baker et al., 2017). We chose to include ACAN as a part of our peer-reviewed literature class because our previously reported association did not meet genome-wide significance, and it is an especially interesting candidate gene for degenerative ligament disease that has been reported in human (Mannion et al., 2014; Johnson et al., 2015), horse (Plaas et al., 2011), and dog genetic research, including work that was independent of our research group (Wilke, 2010). SNPs that were not within or near candidate genes were assigned to a separate class. Ultimately, 12,209 SNPs were assigned to a biological prior.
We ran the BayesRC algorithm for a total of 200,000 iterations with a burn-in period of 100,000 iterations. The model analysis was repeated five times to assess model convergence. Fixed effects included in the analysis were dog sex, age, weight, and neuter status (Whitehair et al., 1993; Witsberger et al., 2008). To account for population structure in the dataset, the top five principal components derived from eigen decomposition of the variance-standardized genetic relationship matrix were also included as fixed effects in the model. Principal components analysis was performed using PLINK v1.9. Final mean SNP effects were evaluated based on the absolute value of the reported SNP effect. SNP effects were assigned to genes if they were within a gene boundary ±25 kb. For the purpose of comparing results with and without assignment of biological priors, we performed an analysis with all of the above parameters, but assigned all SNPs to a single prior class, which is effectively equivalent to a BayesR analysis.
Selection Signature Analysis
Anterior cruciate ligament rupture in dogs has a marked breed predisposition, with reported breed prevalence in the Labrador Retriever of 5.79% (Witsberger et al., 2008). Artificial selection is a necessary part of breed creation, and genetic risk of ACL rupture in the Labrador Retriever may be the result of unintentional selection due to linkage between ACL rupture risk variants and desirable traits. Regions of the genome that have been under selection have reduced heterozygosity which is identifiable through selection signature analysis. ACL rupture risk variants that are also within regions of the genome that are under selection may be especially important to defining breed predisposition to ACL rupture. We performed selection signature analysis to detect regions that show preferential selection in the genomes of case versus control subpopulations. We performed whole genome scans for signatures of selection based on the concept of extended haplotype homozygosity (EHH) as formulated by Sabeti et al. (2002). In EHH analysis, reduction in haplotype diversity is computed as the probability that two extended haplotypes around a given locus are the same, given that they have the same allele at the locus.
We defined haplotypes for case and control subpopulations using fastPHASE software (Scheet and Stephens, 2006) with the number of random starts set to 10 (-T10) and the number of iterations set to 20 (-C20). The fastPHASE model is based on the idea that, over short regions of the genome, haplotypes in a population tend to cluster into groups of similar haplotypes. The number of clusters, K, is an essential hyperparameter that must be computed. To define K, a portion of the data is set to missing, and for several values of K, fastPHASE makes a best guess for the missing genotypes. This process is repeated multiple times, each time choosing a different portion of the observed data to set to missing. The chosen value for K is the one that produced the lowest overall error rate. We assigned the upper limit for the number of clusters equal to 40 (-Ku40) and the lower limit to 10 (-Kl10), with an interval of 5 (-Ki5). The masking procedure was repeated 100 times (-Ks100), randomly selecting 500 SNP loci (-Ks500), and 5% of observed genotypes among individuals (-Kp0.05) to be masked.
To define selection signatures, we calculated the cross-population extended haplotype heterozygosity test (XP-EHH) using the R package “rehh” v.3.1.0 (Gautier and Vitalis, 2012). XP-EHH compares the integrated EHH between two populations at the same SNP. Selection signatures are identified based on overrepresented haplotypes in one population compared to the other (Sabeti et al., 2007). We evaluated case and control populations to assess whether selection pressures have affected individuals in the case category relative to the founder population (unaffected control dogs) (Voight et al., 2006). Candidate SNPs were defined using a threshold of −log10(P ≤ 1E-05). Genome-wide significance was defined at −log10(P ≤ 1E-08). We used the “calc_haplen” function within “rehh” to define the length of the average haplotype around each significant marker, and each haplotype was evaluated for genes that may be driving selection using the canFam 3.1 gene annotation.
Results
RNA Sequencing
FastQC analysis determined that all samples were of good quality. Overall, average coverage and mapping were excellent across samples. There were 98,214,398 average reads per sample. The average primary and secondary alignment percentages were 90.18 and 8.21%, respectively. The average proportion of properly paired reads was 99.97%. After adjustment for multiple testing and without imposing a threshold for log fold change, we identified 200 genes from ACL tissue and 444 genes from synovium tissue that were significantly differentially expressed between case and control dogs (Supplementary Tables 2, 3). To ease interpretation of results, only transcript ID’s that could be matched to a known gene were included in the assignment of biological priors. This left a total of 181 DEGs from ACL and 373 DEGs from synovium for prior assignment.
Pathway analysis using the PANTHER classification system did not identify overrepresented pathways among DEGs identified in ligament. There were two overrepresented pathways among DEGs from case and control synovium (Table 4).
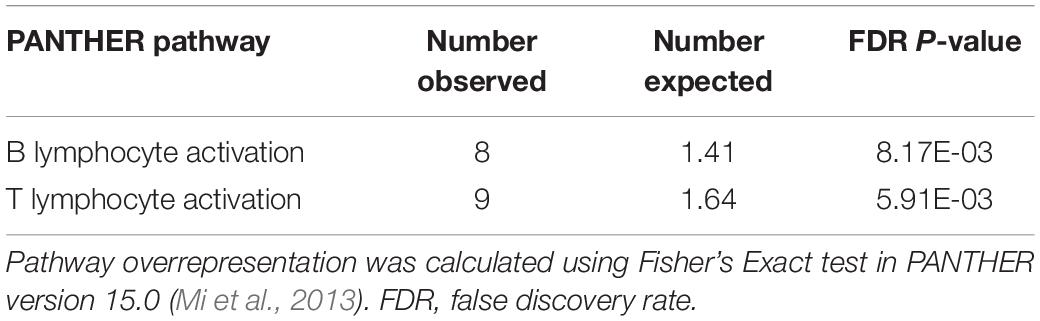
Table 4. Overrepresented pathways identified from differentially expressed genes (DEGs) derived from synovium tissue collected from ACL rupture cases and matched controls.
Association Analysis
Single nucleotide polymorphism effects were averaged across five BayesRC runs. Overall, an average of 3,728 SNPs (0.8%) had some estimated effect, with the remainder of SNPs assigned to the zero-effect distribution. On average, 37 SNPs were assigned to the distribution, 361 SNPs were assigned to the distribution, and 3,330 SNPs were assigned to the distribution. GWAS results from analysis with and without biological priors are visually represented in a Manhattan plot (Figure 1), showing five regions with largest effects on chromosomes 3, 5, 7, 9, and 24 (Supplementary Figure 2). The 50 largest SNP effects and their distance to genes are reported in Table 5. Full results are reported in Supplementary Table 4.
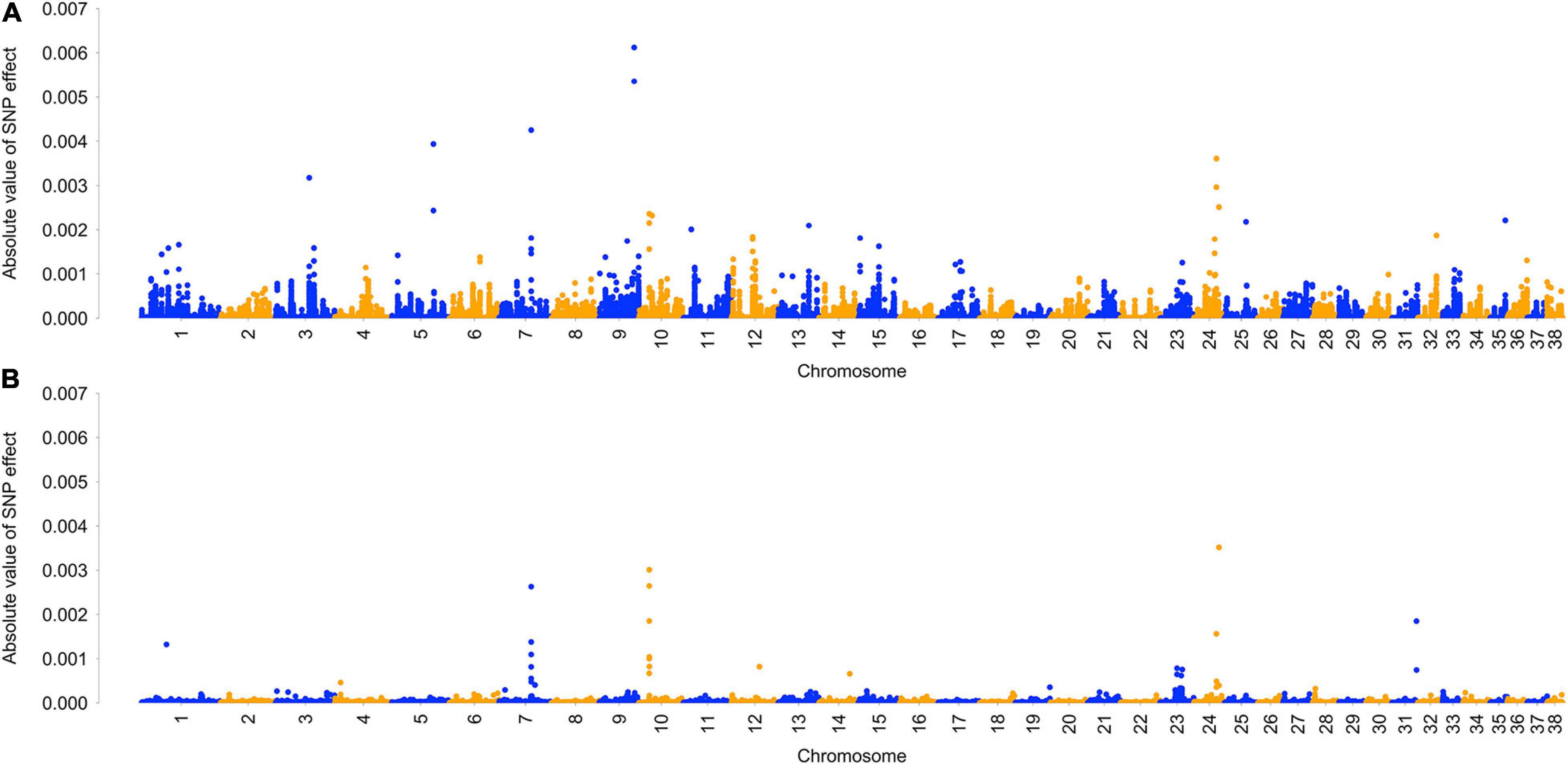
Figure 1. Manhattan plot of genome-wide association analysis of ACL rupture case and control dogs with and without biological priors. Plot shows average SNP effect across five reps of BayesRC algorithm (A) and BayesR algorithm (B).
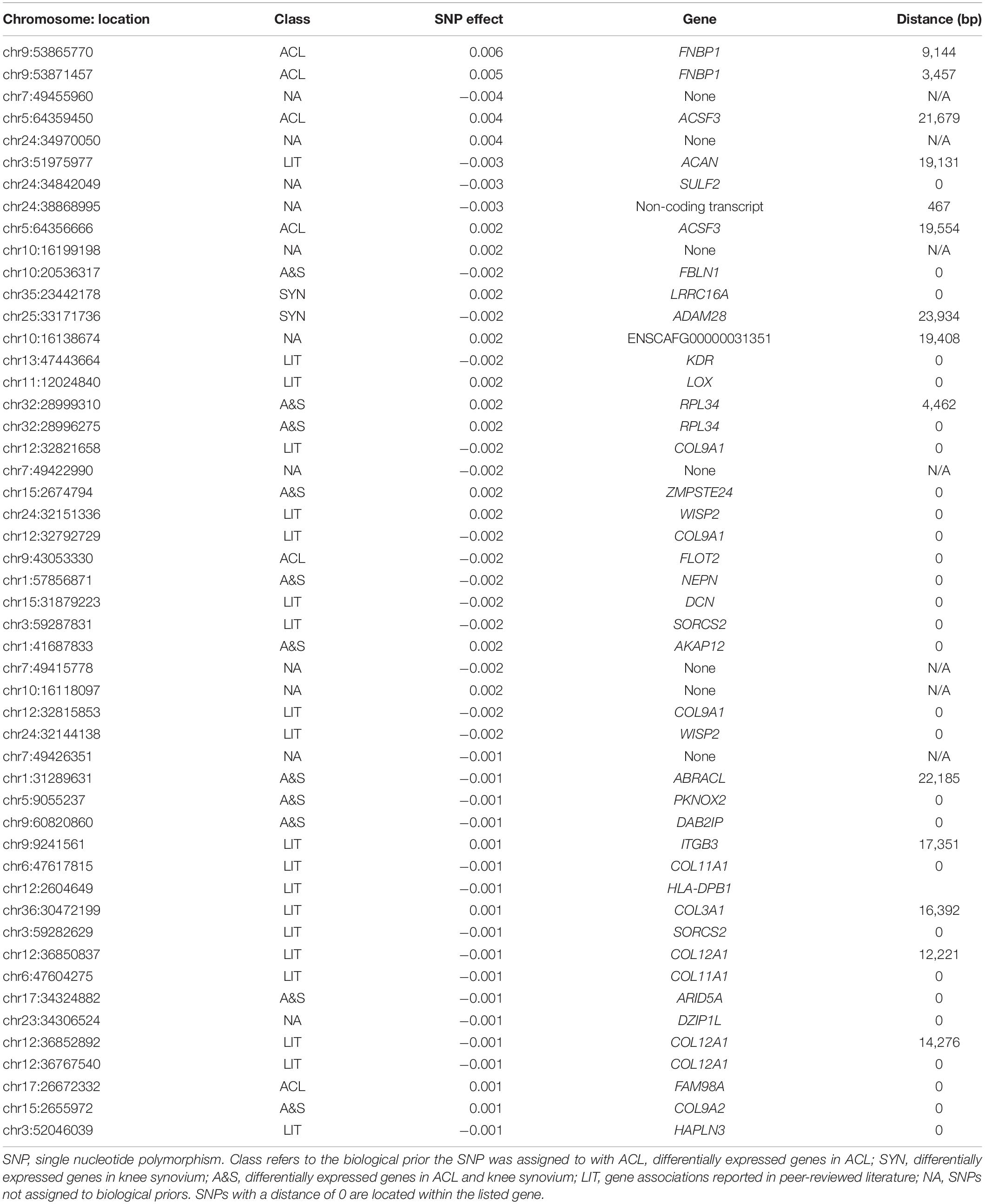
Table 5. The 50 largest SNP effects from Bayesian mixture model (BayesRC) association analysis that included biological priors.
Selection Signature Analysis
A Manhattan plot of XP-EHH results is presented in Figure 2. Overall, 11 regions of the genome showed high levels of differentiation between case and control populations (Table 6). Significant selection signatures were identified on chromosomes 4, 5, 9, and 27. In multiple cases, haplotype boundaries overlapped associations from the GWAS analysis and/or genes that may be relevant to selection in the Labrador Retriever.
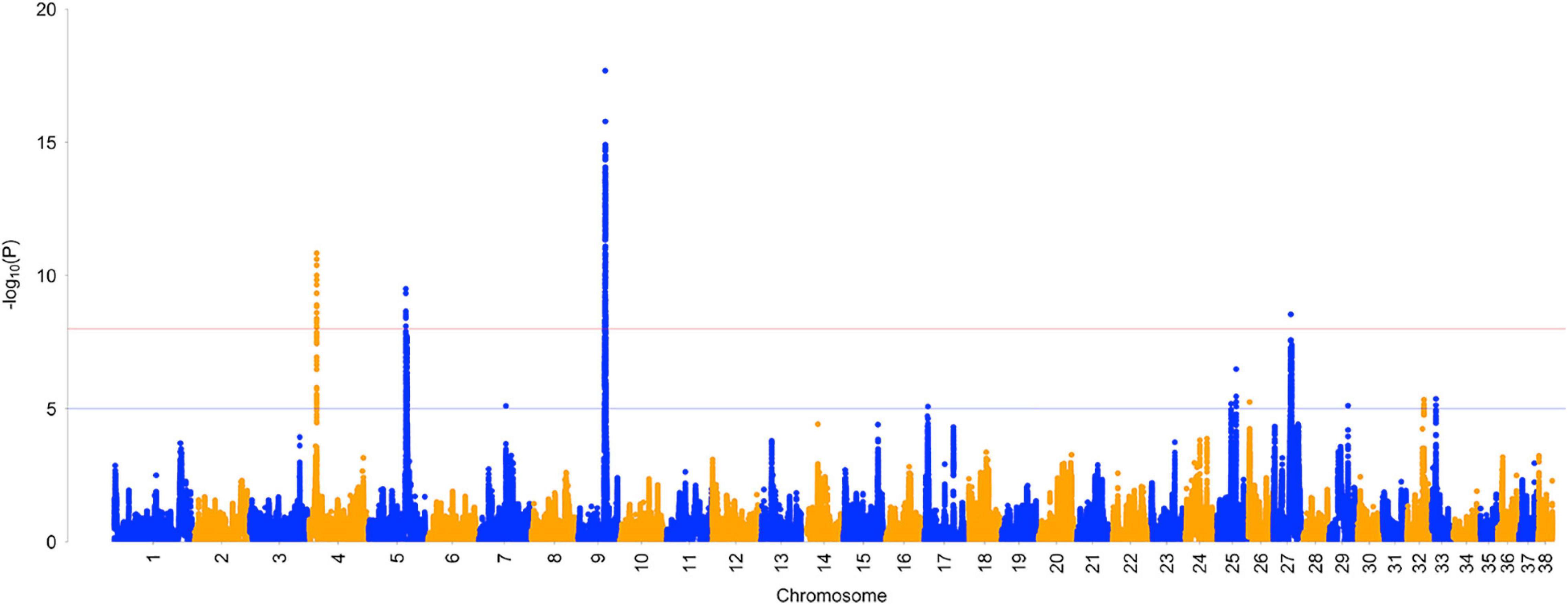
Figure 2. Manhattan plot of –log10 P-values from selection signature analysis using the XP-EHH test between ACL rupture case and control populations in the Labrador Retriever model. The red line denotes genome-wide significance (P < 1E-08). The blue line denotes suggestive significance (P < 1E-05).
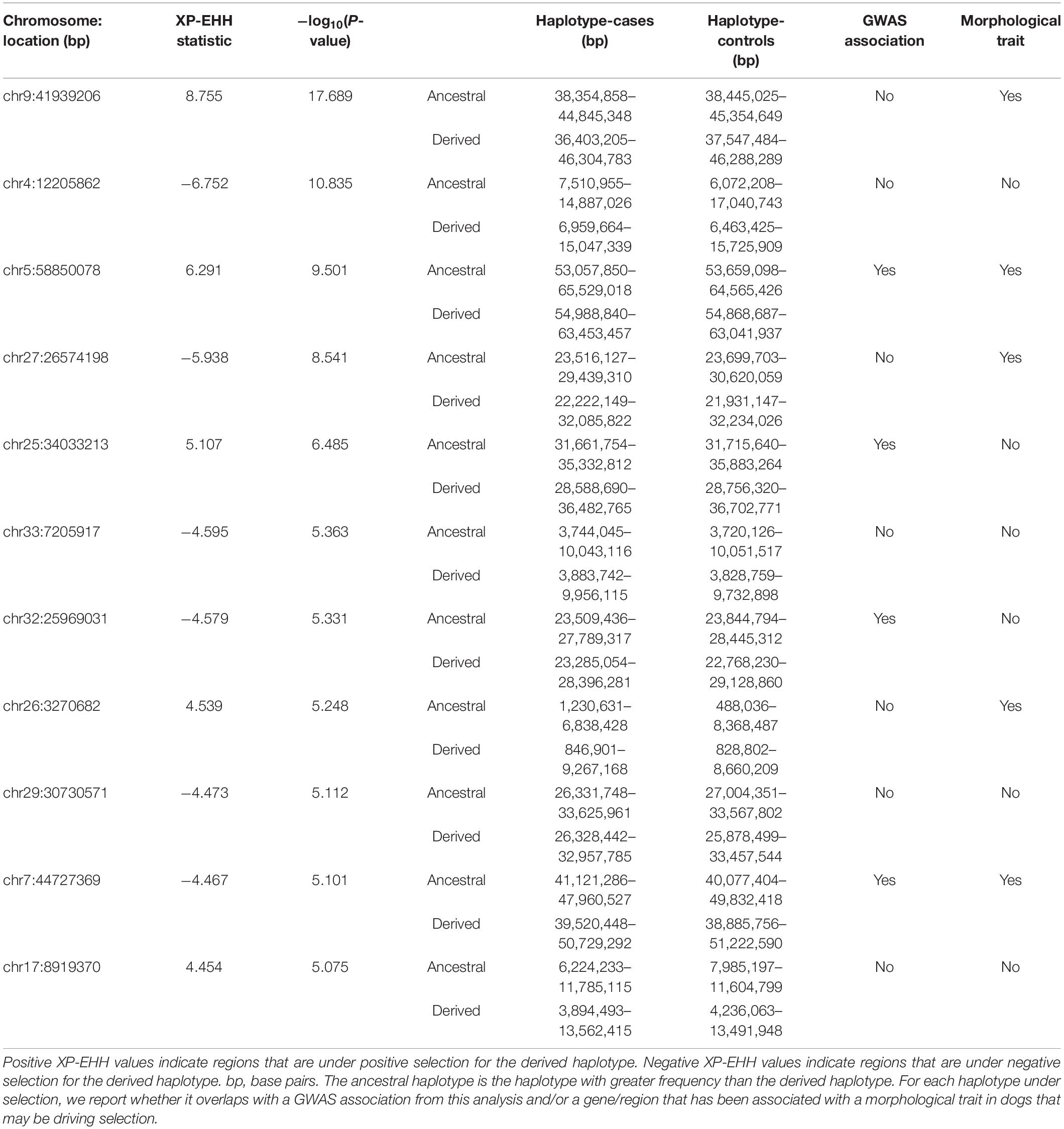
Table 6. SNPs from selection signature analysis using the XP-EHH test between ACL rupture case and control populations with P ≤ 1E-05.
Discussion
Incorporation of prior biological information using the BMM algorithm BayesRC allows the user to prioritize SNPs based on biological probability of effect in GWAS analysis. This is in contrast to the more subjective decisions that are often made when evaluating GWAS results (Thompson et al., 2013). Here, we were able to identify associations within or near many relevant candidate genes for ACL rupture. Many of the largest effect SNPs were within or near genes that were either differentially expressed between ACL rupture case and control dogs or were candidate genes that have been reported previously in both human and canine studies of the genetic predisposition to ACL rupture.
To assign biological priors, we first performed RNA sequencing in ACL and synovium tissues from ACL rupture affected and matched control dogs to identify DEGs, and DEG lists were submitted for pathway enrichment analysis. While there were no overrepresented pathways identified in ACL, there were two overrepresented pathways in synovium representing genes that are expressed in B and T lymphocyte activation, clearly indicating that inflammatory response is an important differentiator between cases and controls. This is perhaps unsurprising, as ACL rupture is associated with marked lymphocytic synovitis in dogs (Little et al., 2014). It is unclear whether ACL rupture-associated synovitis is a cause or effect of ligament rupture, as signs of synovial effusion are often present on radiographs and synovitis can also be seen arthroscopically before development of complete ACL rupture and associated joint instability (Muir et al., 2011; Little et al., 2014), and these signs are predictive of future ACL rupture (Chuang et al., 2014). In humans, synovitis is associated with development and progression of OA (Hügle and Geurts, 2017). Future research warrants further investigation into the details of the genes that were significantly differentially expressed between ACL rupture cases and controls, and whether their differential expression may be related to a response unique to ACL rupture patients.
BayesRC analysis identified several regions of the genome that show association with ACL rupture in the dog model. A Manhattan plot of GWAS results shows five regions with the largest effects on chromosomes 3, 5, 7, 9, and 24. When BayesRC results are compared to a similar analysis without the inclusion of biological priors (BayesR), associations on chromosomes 7, 10 (10th largest effect in BayesRC results), and 24 remain, but we can see that many more associations are identified when biological priors are included as part of the analysis.
Intense artificial selection during breed creation may be partially responsible for the high prevalence of ACL rupture in the Labrador Retriever population. Associations that are identified in both GWAS and selection signature analysis may be more biologically significant to ACL rupture risk than regions that were identified in a single analysis. We identified 11 regions of the genome that showed evidence of selection. Some of these regions lend themselves to hypotheses over what may be driving selection in a direction that increases risk of ACL rupture. The signature on chromosome 5 is most notable. This region overlaps our GWAS association on chromosome 5, which is near the gene ACSF3, which was also differentially expressed in ligament. This region also overlaps the boundaries of MC1R, the gene responsible for black versus yellow coat color in the Labrador Retriever (Everts et al., 2000), a clear target for artificial selection. We have observed in previous work that Labrador Retrievers with yellow coat color are overrepresented among cases (Terhaar et al., 2020). ACSF3 has also been shown to be under selection in sporting breeds (which includes the Labrador Retriever) compared to other breeds (Kim et al., 2018). In humans, ACSF3 has been reported as a risk gene in patients with rheumatoid arthritis (RA) (Julià et al., 2017). Within the cell, mitochondria maintain a pathway for fatty acid synthesis (FAS) that is distinct from cytosolic FAS. ACSF3 encodes the enzyme responsible for the first step of mitochondrial FAS. T-cells from patients with RA have undergone a metabolic shift to a pro-invasive, proinflammatory state that is marked by impaired glycolysis and increased cytosolic FAS (Shen et al., 2017). In our study, ACSF3 was expressed more highly in cases, which indicates that cells in the sample were highly metabolically active (Bowman and Wolfgang, 2018). In vitro research suggests that increased activity of mitochondrial FAS leads to reduced glucose utilization and increased cytosolic FAS (Clay et al., 2016), which may support a proinflammatory state. Since MC1R and ACSF3 are within the same haplotype in our population, selecting for coat color may select for a haplotype that affects ACSF3 activity, or through MC1R itself (or both). MC1-signaling is not limited to melanin production, it also plays a role in the inflammatory system and has been shown to protect against cartilage degeneration and subchondral bone sclerosis in OA (Montero-Melendez et al., 2020). MC1 agonists are being investigated as disease-modifying treatments for a range of inflammatory diseases (Spana et al., 2019; Montero-Melendez et al., 2020). Yellow Labrador Retrievers lack functional MC1 receptors, which means that they may be predisposed to inflammation as well, and this could affect their risk of ACL rupture. Though certain people with red hair also lack functional MC1 receptors, research on the effect of MC1 signaling on the risk of inflammatory disease in people or dogs is limited. Given the findings in the present study and clear biological significance of this region, it will be important to follow up on these findings in future work.
Other signatures also illuminate hypotheses for biological mechanisms underlying ACL rupture risk. The signature on chromosome 7, which overlaps with our GWAS association, is a well-known region that contains the gene SMAD2, which has repeatedly been associated with weight and body size in dogs (Chase et al., 2009; Rimbault et al., 2013; Hayward et al., 2019; Plassais et al., 2019; Bannasch et al., 2020). The signature on chromosome 26 also overlaps with a region that has been associated with weight and height in dogs (Hayward et al., 2019). Multiple epidemiological studies of ACL rupture in dogs, including our own, have identified weight as a risk factor (Whitehair et al., 1993; Duval et al., 1999; Adams et al., 2011; Baker et al., 2018), though in most cases it is unclear if this is a function of obesity or body size. The signature on chromosome 9 has been identified previously in the Labrador Retriever (Wiener et al., 2017) as a region that is under selection in dogs bred for sport (hunting) versus show. Labrador Retrievers that are bred for hunting are morphologically distinct from those that are bred for show, and it is possible that these morphological differences may be contributing to risk of ACL rupture. The region on chromosome 27 overlaps the boundaries of KRT71, which is responsible for curled-coat in dogs (Cadieu et al., 2009). Labrador Retrievers may have a wavy coat, though this is a less desirable feature according to AKC standard.
There were several associations from GWAS analysis that did not overlap with the selection signature analysis. These associations are also important for understanding the underlying pathophysiology of ACL rupture. For example, the top association on chromosome 9 was within the gene FNBP1, which was also differentially expressed in ligament. FNBP1, which is also known as FBP17, encodes a protein essential for clathrin-mediated endocytosis (Kamioka et al., 2004). It is also involved in regulation of the actin filament assembly for the actin cytoskeleton, which is important for cellular migration and the maintenance of cell shape (Higgs, 2005; Aspenström, 2010). ACL fibroblasts are known to undergo cytoskeletal reorganization after a strain event to align in longitudinal orientation with the strain (Lee et al., 2005). In this study, FNBP1 was expressed more highly in cases than controls, which may have been associated with actin reorganization in response to ACL injury. GWAS results highlighted several other genes that also have a role in actin cytoskeleton homeostasis, including LRRC16A (also known as CARMIL1) (Edwards et al., 2013), KDR (Luykenaar et al., 2009), LOX (Payne et al., 2006), FLOT2 (Langhorst et al., 2007), AKAP12 (Benz et al., 2019), ABRACL (Wang et al., 2019), PKNOX2 (also known as PREP2) (Haller et al., 2004), ITGB3 (Urbinati et al., 2012), and SORCS2 (Deinhardt et al., 2011), which has also been reported as associated with ACL rupture in Newfoundland dogs (Baird et al., 2014b). This pattern in the association results suggests that variable actin dynamics may play a role in genetic predisposition to ACL rupture. These observations indicate there could be a heritable difference in response to injury between dogs that rupture their ACL and those that do not.
Other associations within the top 50 SNP effects point to genes involved in the extracellular matrix. Aggrecan (ACAN) was within the top five GWAS associations. Aggrecan plays a vital role in maintaining hydration in the extracellular matrix of collagenous tissues, including ligamentous tissue. An association between ACAN and ACL rupture has been reported multiple times in humans (Mannion et al., 2014; Johnson et al., 2015) and dogs (Wilke, 2010). It has also been associated with degenerative ligament disease in horses (Plaas et al., 2011). While an association between aggrecan and ACL rupture has been reported before in a GWAS from a subset of this dataset (Baker et al., 2017), we chose to keep ACAN in the list of candidate genes because of its connection to degenerative ligament disease across species, and because the previously reported association in the Labrador Retriever was weak (P = 1.07E-04). Because of this, care should be taken not to overinterpret this association. However, the strength of the association in this study as well as the body of evidence that exists to support it leads us to consider this association as additional evidence of a role for aggrecan in the pathobiology of ACL rupture. SULF2 was also among the top five associations. SULF2 encodes an enzyme that is important for regulation of overall balance of cartilage matrix synthesis and degradation (Otsuki et al., 2010). SULF2 was not assigned to a biological prior, and therefore this association is derived from genetic data only. SULF2 knockout mice develop early-onset OA in their knee joints at 6 months of age with reduced glycosaminoglycan content and lower cellularity in articular cartilage (Otsuki et al., 2008). Dogs with ACL rupture also develop early osteoarthritic changes that are typically present at the time of diagnosis (Chuang et al., 2014), and early-onset OA is common in human ACL rupture patients (Lohmander et al., 2007). An association between SULF2 and ACL rupture has not been previously reported. Other notable extracellular matrix genes include several collagen genes COL9A1, COL11A1, COL12A1, COL3A1, and COL9A2. All of these genes were included in the candidate genes from peer-reviewed literature class, as various associations have been reported previously in human and dogs. These associations have not been previously validated in either species. COL9A2 was also differentially expressed in both ACL and knee synovium. COL9A2 encodes the alpha-2 chain of type IX collagen, which is crucial to the maintenance of articular cartilage. Reduced levels of type IX collagen may contribute to OA pathogenesis (Luo et al., 2017).
With the exception of the associations on chromosome 3 with ACAN (Wilke, 2010; Baker et al., 2017) and SORCS2 (Baird et al., 2014a), most the associations identified in this study did not overlap with associations identified in previous GWAS for ACL rupture in dogs (Baker et al., 2017, 2018; Huang et al., 2017; Hayward et al., 2019). The use of biological priors in the present work was performed, in part, due to the inconsistencies in GWAS results across studies. We consider the current study an extension of our former work that differs in many ways. Baker et al. (2017) analyzed considerably fewer dogs (N = 237), used a different method for statistical analysis, and did not correct for weight or age as fixed effects, and any of these factors could explain different results. Baker et al. (2018) was a multivariate analysis aimed at answering how genetics may impact tibial morphology, and whether this has an effect on risk of ACL rupture. We expect that the effects discovered in this analysis would have smaller effects on ACL rupture as a whole compared to their effects on tibial morphology combined with ACL rupture risk (Baker et al., 2018), so it is not surprising that these results were not among the top SNP effects in the present analysis. The research that has come from Cornell University (Hayward et al., 2016, 2019; Huang et al., 2017) used a dataset that contains many dog breeds. The SNP associations identified in these studies may be reflective of ACL rupture risk that are either weaker in the Labrador Retriever, or specific to risk in other breeds. We believe that the overlap of significant GWAS results with DEGs and selection signatures speaks to the strength of the present work.
In MacLeod et al. (2016), it is noted that the Dirichlet prior in BayesRC may have greater influence on the posterior if the number of variants in one class is low relative to the rest of the dataset. The authors suggested that classes should have 1,000 variants or greater to allow the data to have strong influence on the posterior parameters, especially when there is greater uncertainty, for example, when candidate genes from reported literature are used for prior assignment. To avoid this, we made sure that >1,000 SNPs were assigned to the peer-reviewed literature class used in this study (Class LIT, Table 2). However, there were fewer than 1,000 SNPs in Class A&S, which represented genes that were differentially expressed in both ligament and synovium tissues. This was known before BayesRC analysis, and the choice was made to maintain this class for two reasons: (1) these genes were differentially expressed in both ACL and knee synovium tissues, so there is inherently less uncertainty around their candidacy and (2) because they were differentially expressed in both tissues, it seemed important to designate them separately from genes that were differentially expressed in only one tissue. Ten of the top 50 SNP effects were assigned to Class A&S, which is a relatively high number given 703/433,227 = 0.16% of SNPs were assigned to Class A&S, and these SNPs represent 20% of the top SNP effects. It is not clear whether the effect of these SNPs is due to true association with the disease, or potential bias from prior assignment, and these results should be interpreted with this in mind.
Genomic prediction is widely used in production animal populations to select individuals for breeding based on their estimated breeding value (EBV) for a desirable trait such as milk production or meat quality. Genomic prediction for complex diseases in human populations, referred to as a polygenic risk score (PRS), has received considerable attention in recent research (Chatterjee et al., 2016). Though the calculation is generally the same, the goal in human research is focused on individual risk management and personalized medicine rather than breeding decisions (Wray et al., 2019). In companion animal populations such as the dog, the EBV/PRS could be used for both breeding decisions and individual medical management. ACL rupture, in particular, is an acquired trait in dogs that may not present itself until well after a dog has given birth to or sired many litters (Witsberger et al., 2008). High-risk individuals would become candidates for a weight management program (Witsberger et al., 2008), possible delayed neutering (Torres de la Riva et al., 2013), and radiographic screening (Chuang et al., 2014). Development of a PRS for ACL rupture in the dog model is an important goal of our research (Baker et al., 2017, 2020). Incorporating biological knowledge using the BayesRC algorithm has been reported to improve accuracy of genomic prediction in livestock populations (MacLeod et al., 2016). The accuracy of genomic prediction is heavily affected by the size of the reference population, with most estimates using sample sizes well into the thousands (Van Raden et al., 2009; Liu et al., 2011; Dudbridge, 2013). We did not attempt genomic prediction as a part of this study due to the size of our reference dataset. Recruitment of additional Labrador Retrievers is underway with the intention to develop a PRS for ACL rupture in the dog model using BayesRC and/or other algorithms.
Conclusion
Incorporation of a priori biological information into BMM analysis using BayesRC in a dog model of ACL rupture was able to replicate associations that were previously reported in human and dog studies, especially in collagen genes, and also identify novel genetic associations with ACL rupture. Several associations reported in human studies have been identified here, in the dog, which highlights the value of One Health medicine (Gyles, 2016), and the dog in particular as a valuable model for genomic research. The actin cytoskeleton is the basis for cellular organization and shape and is integral for a cell’s capacity to migrate. This is the first study to suggest a role for the actin cytoskeleton in risk of ACL rupture. Additionally, while SULF2 has been implicated in onset and progression of OA, which is typically associated with ACL rupture, this is the first publication to report an association between SULF2 and ACL rupture itself. Many of the associations we identified in this study overlap with regions of the genome that are under selection in the Labrador Retriever. These findings begin to provide an explanation for the high prevalence of ACL rupture in this breed and highlight the unintended consequences of artificial selection.
Data Availability Statement
The RNASeq data presented in the study are deposited in the ArrayExpress repository, accession number E-MTAB-10119. The SNP data presented in this study are deposited in the European Variation Archive (EVA), project number PRJEB43243 and analysis number ERZ1743079.
Ethics Statement
The animal study was reviewed and approved by the Animal Care Committee of the University of Wisconsin-Madison (protocols V1070 and V5463). Written informed consent was obtained from the owners for the participation of their animals in this study.
Author Contributions
LB carried out the study and wrote the first draft of the manuscript. MM performed selection signature analysis and contributed to writing and editing of the manuscript. RM contributed to initial data analysis and editing the manuscript. MB consulted on study design, performed the RNA sequencing analysis for the experiment, and contributed to the writing and editing of the manuscript. EB and SS contributed to dog recruitment and sample collection, maintenance of the data, interpretation of the results, and reviewed the manuscript. PM designed the experiment, obtained funding for the experiment, supervised the study, and revised the manuscript. All authors have read and approved the final version of the manuscript.
Conflict of Interest
The authors of this manuscript have the following competing interests: PM, LB and MM are named on US Patent US20160222451A1 “Method to predict heritable canine non-contact cruciate ligament rupture.” This does not alter our adherence to the journal’s policies on data sharing and materials.
The remaining authors declare that the research was conducted in the absence of any commercial or financial relationships that could be construed as a potential conflict of interest.
Funding
LB received support from a National Library of Medicine training grant to the Computation and Informatics in Biology and Medicine Training Program (NLMT15M007359). SS received support from the National Institutes of Health (K01OD019743-01A1). EB also received support from the National Institutes of Health (T32OD010423). Funding for this project was partially provided by the Morris Animal Foundation (D13CA-020, www.morrisanimalfoundation.org) and the Melita Grunow Family Professorship in Companion Animal Health. The funders had no role in study design, data collection and analysis, decision to publish, or preparation of the manuscript.
Acknowledgments
The authors gratefully acknowledge Iona MacLeod for her advice and assistance with interpretation of BayesRC analysis. The authors thank Brian Davis for providing the multi-breed dataset used for imputation. The authors would also like to thank the faculty, residents, and students throughout the University of Wisconsin-Madison UW Veterinary Care hospital and the many individual breeders and pet owners for their help in recruitment of Labrador Retrievers for this study.
Supplementary Material
The Supplementary Material for this article can be found online at: https://www.frontiersin.org/articles/10.3389/fgene.2021.593515/full#supplementary-material
References
Adams, P., Bolus, R., Middleton, S., Moores, A. P., and Grierson, J. (2011). Influence of signalment on developing cranial cruciate rupture in dogs in the UK. J. Small Anim. Pract. 52, 347–352. doi: 10.1111/j.1748-5827.2011.01073.x
Aken, B. L., Ayling, S., Barrell, D., Clarke, L., Curwen, V., Fairley, S., et al. (2016). The ensembl gene annotation system. Database 2016:baw093. doi: 10.1093/database/baw093
Andrews, S. (2010). FastQC: A Quality Control Tool for High Throughput Sequence Data. Available online at: http://www.bioinformatics.babraham.ac.uk/projects/fastqc (accessed December 1, 2017).
Aspenström, P. (2010). Formin-binding proteins: modulators of formin-dependent actin polymerization. BBA Mol. Cell Res. 1803, 174–182. doi: 10.1016/j.bbamcr.2009.06.002
Baird, A. E., Carter, S. D., Innes, J. F., Ollier, W., and Short, A. (2014a). Genome-wide association study identifies genomic regions of association for cruciate ligament rupture in Newfoundland dogs. Anim. Genet. 45, 542–549. doi: 10.1111/age.12162
Baird, A. E., Carter, S. D., Innes, J. F., Ollier, W. E., and Short, A. D. (2014b). Genetic basis of cranial cruciate ligament rupture (CCLR) in dogs. Connect. Tissue Res. 55, 275–281. doi: 10.3109/03008207.2014.910199
Baker, L. A., Kirkpatrick, B., Rosa, G. J., Gianola, D., Valente, B., Sumner, J. P., et al. (2017). Genome-wide association analysis in dogs implicates 99 loci as risk variants for anterior cruciate ligament rupture. PLoS One 12:e0173810. doi: 10.1371/journal.pone.0173810
Baker, L. A., Momen, M., Chan, K., Bollig, N., Brito Lopes, F., Rosa, G. J. M., et al. (2020). Bayesian and machine learning models for genomic prediction of anterior cruciate ligament rupture in the canine model. G3 (Bethesda) 10, 2619–2628. doi: 10.1534/g3.120.401244
Baker, L. A., Rosa, G. J., Hao, Z., Piazza, A., Hoffman, C., Binversie, E. E., et al. (2018). Multivariate genome-wide association analysis identifies novel and relevant variants associated with anterior cruciate ligament rupture risk in the dog model. BMC Genet. 19:39. doi: 10.1186/s12863-018-0626-7
Bannasch, D. L., Baes, C. F., and Leeb, T. (2020). Genetic variants affecting skeletal morphology in domestic dogs. Trends Genet. 36, 598–609. doi: 10.1016/j.tig.2020.05.005
Benz, P. M., Ding, Y., Stingl, H., Loot, A. E., Zink, J., Wittig, I., et al. (2019). AKAP12 deficiency impairs VEGF -induced endothelial cell migration and sprouting. Acta Physiol. 228:e13325. doi: 10.1111/apha.13325
Bleedorn, J. A., Greuel, E. N., Manley, P. A., Schaefer, S. L., Markel, M. D., Holzman, G., et al. (2011). Synovitis in dogs with stable stifle joints and incipient cranial cruciate ligament rupture: a cross-sectional study. Vet. Surg. 40, 531–543. doi: 10.1111/j.1532-950x.2011.00841.x
Bowman, C. E., and Wolfgang, M. J. (2018). Role of the malonyl-CoA synthetase ACSF3 in mitochondrial metabolism. Adv. Biol. Regul. 71, 34–40. doi: 10.1016/j.jbior.2018.09.002
Browning, B. L., Zhou, Y., and Browning, S. R. (2018). A one-penny imputed genome from next-generation reference panels. Am. J. Hum. Genet. 3, 338–348. doi: 10.1016/j.ajhg.2018.07.015
Cadieu, E., Neff, M. W., Quignon, P., Walsh, K., Chase, K., Parker, H. G., et al. (2009). Coat variation in the domestic dog is governed by variants in three genes. Science 326, 150–153. doi: 10.1126/science.1177808
Calboli, F. C., Sampson, J., Fretwell, N., and Balding, D. J. (2008). Population structure and inbreeding from pedigree analysis of purebred dogs. Genetics 179, 593–601. doi: 10.1534/genetics.107.084954
Chang, C. C., Chow, C. C., Tellier, L. C., Vattikuti, S., Purcell, S. M., and Lee, J. J. (2015). Second-generation PLINK: rising to the challenge of larger and richer datasets. GigaScience 4:7. doi: 10.1186/s13742-015-0047-8
Chase, K., Jones, P., Martin, A., Ostrander, E. A., and Lark, K. G. (2009). Genetic mapping of fixed phenotypes: diseases frequency as a breed characteristic. J. Hered. 100, S37–S41. doi: 10.1093/jhered/esp011
Chatterjee, N., Shi, J., and García-Closas, M. (2016). Developing and evaluating polygenic risk prediction models for stratified disease prevention. Nat. Rev. Genet. 17, 392–406. doi: 10.1038/nrg.2016.27
Chuang, C., Ramaker, M. A., Kaur, S., Csomos, R. A., Kroner, K. T., Bleedorn, J. A., et al. (2014). Radiographic risk factors for contralateral rupture in dogs with unilateral cranial cruciate ligament rupture. PLoS One 9:e106389. doi: 10.1371/journal.pone.0106389
Clay, H. B., Parl, A. K., Mitchell, S. L., Singh, L., Bell, L. N., and Murdock, D. G. (2016). Altering the mitochondrial fatty acid synthesis (mtFASII) pathway modulates cellular metabolic states and bioactive lipid profiles as srevealed by metabolomic profiling. PLoS One 11:e0151171. doi: 10.1371/journal.pone.0151171
Collins, M., and Raleigh, S. M. (2009). “Genetic risk factors for musculoskeletal soft tissue injuries,” in Genetics and Sports, ed. M. Collins, (Basel: Karger Publishers), 136–149. doi: 10.1159/000235701
Comerford, E. J., Smith, K., and Hayashi, K. (2011). Update on the aetiopathogenesis of canine cranial cruciate ligament disease. Vet. Comp. Orthop. Traumatol. 24, 91–98. doi: 10.3415/vcot-10-04-0055
Deinhardt, K., Kim, T., Spellman, D. S., Mains, R. E., Eipper, B. A., Neubert, T. A., et al. (2011). Neuronal growth cone retraction relies on proneurotrophin receptor signaling through Rac. Sci. Signal. 4:ra82. doi: 10.1126/scisignal.2002060
Dobin, A., Davis, C. A., Schlesinger, F., Drenkow, J., Zaleski, C., Jha, S., et al. (2013). STAR: ultrafast universal RNA-seq aligner. Bioinformatics 29, 15–21. doi: 10.1093/bioinformatics/bts635
Dudbridge, F. (2013). Power and predictive accuracy of polygenic risk scores. PLoS Genet. 9:e1003348. doi: 10.1371/journal.pgen.1003348
Duval, J. M., Budsberg, S. C., Flo, G. L., and Sammarco, J. L. (1999). Breed, sex, and body weight as risk factors for rupture of the cranial cruciate ligament in young dogs. J. Am. Vet. Med. Assoc. 215, 811–814.
Edwards, M., Liang, Y., Kim, T., and Cooper, J. A. (2013). Physiological role of the interaction between CARMIL1 and capping protein. Mol. Biol. Cell 24, 3047–3055. doi: 10.1091/mbc.e13-05-0270
El Khoury, L., Posthumus, M., Collins, M., van der Merwe, W., Handley, C., Cook, J., et al. (2015). ELN and FBN2 gene variants as risk factors for two sports-related musculoskeletal injuries. Int. J. Sports Med. 36, 333–337. doi: 10.1055/s-0034-1390492
Encode Consortium, (2016). ENCODE Guidelines and Best Practices for RNA-Seq: Revised December 2016. ENCODE Project. Available online at: https://www.encodeproject.org/documents/cede0cbe-d324-4ce7-ace4-f0c3eddf5972/@@download/attachment/ENCODE%20Best%20Practices%20for%20RNA_v2.pdf (accessed December 1, 2017).
Erbe, M., Hayes, B. J., Matukumalli, L. K., Goswami, S., Bowman, P. J., Reich, C. M., et al. (2012). Improving accuracy of genomic predictions within and between dairy cattle breeds with imputed high-density single nucleotide polymorphism panels. J. Dairy Sci. 95, 4114–4129. doi: 10.3168/jds.2011-5019
Everts, R. E., Rothuizen, J., and van Oost, B. A. (2000). Identification of a premature stop codon in the melanocyte stimulating hormone receptor gene (MC1R) in Labrador and Golden retrievers with yellow coat colour. Anim. Genet. 31, 194–199. doi: 10.1046/j.1365-2052.2000.00639.x
Ficek, K., Cieszczyk, P., Kaczmarczyk, M., Maciejewska-Karłowska, A., Sawczuk, M., Cholewinski, J., et al. (2013). Gene variants within the COL1A1 gene are associated with reduced anterior cruciate ligament injury in professional soccer players. J. Sci. Med. Sport 16, 396–400. doi: 10.1016/j.jsams.2012.10.004
Friedenberg, S. G., and Meurs, K. M. (2016). Genotype imputation in the domestic dog. Mamm. Genome 27, 485–494. doi: 10.1007/s00335-016-9636-9
Gallagher, M. D., and Chen-Plotkin, A. S. (2018). The post-GWAS era: from association to function. Am. J. Hum. Genet. 102, 717–730. doi: 10.1016/j.ajhg.2018.04.002
Gautier, M., and Vitalis, R. (2012). rehh: an R package to detect footprints of selection in genome-wide SNP data from haplotype structure. Bioinformatics 28, 1176–1177. doi: 10.1093/bioinformatics/bts115
Gibbon, A., Saunders, C. J., Collins, M., Gamieldien, J., and September, A. V. (2018). Defining the molecular signatures of Achilles tendinopathy and anterior cruciate ligament ruptures: a whole-exome sequencing approach. PLoS One 13:e0205860. doi: 10.1371/journal.pone.0205860
Haller, K., Rambaldi, I., Daniels, E., and Featherstone, M. (2004). Subcellular localization of multiple PREP2 isoforms is regulated by actin, tubulin, and nuclear export. J. Biol. Chem. 279, 49384–49394. doi: 10.1074/jbc.m406046200
Hayward, J. J., Castelhano, M. G., Oliveira, K. C., Corey, E., Balkman, C., Baxter, T. L., et al. (2016). Complex disease and phenotype mapping in the domestic dog. Nat. Commun. 7:10460. doi: 10.1038/ncomms10460
Hayward, J. J., White, M. E., Boyle, M., Shannon, L. M., Casal, M. L., Castelhano, M. G., et al. (2019). Imputation of canine genotype array data using 365 whole-genome sequences improves power of genome-wide association studies. PLoS Genet. 15:e1008003. doi: 10.1371/journal.pgen.1008003
Higgs, H. N. (2005). Formin proteins: a domain-based approach. Trends Biochem. Sci. 30, 342–353. doi: 10.1016/j.tibs.2005.04.014
Huang, M., Hayward, J. J., Corey, E., Garrison, S. J., Wagner, G. R., Krotscheck, U., et al. (2017). A novel iterative mixed model to remap three complex orthopedic traits in dogs. PLoS One 12:e0176932. doi: 10.1371/journal.pone.0176932
Hügle, T., and Geurts, J. (2017). What drives osteoarthritis?—Synovial versus subchondral bone pathology. Rheumatology 56, 1461–1471.
Jiang, H., Lei, R., Ding, S. W., and Zhu, S. (2014). Skewer: a fast and accurate adapter trimmer for next-generation sequencing paired-end reads. BMC Bioinformatics 15:182. doi: 10.1186/1471-2105-15-182
John, R., Dhillon, M. S., Sharma, S., Prabhakar, S., and Bhandari, M. (2016). Is there a genetic predisposition to anterior cruciate ligament tear? A systematic review. Am. J. Sports Med. 44, 3262–3269. doi: 10.1177/0363546515624467
Johnson, J. S., Morscher, M. A., Jones, K. C., Moen, S. M., Klonk, C. J., Jacquet, R., et al. (2015). Gene expression differences between ruptured anterior cruciate ligaments in young male and female subjects. J. Bone Joint Surg. 97, 71–79. doi: 10.2106/jbjs.n.00246
Julià, A., Absher, D., López-Lasanta, M., Palau, N., Pluma, A., Jones, L. W., et al. (2017). Epigenome-wide association study of rheumatoid arthritis identifies differentially methylated loci in B cells. Hum. Mol. Genet. 26, 2803–2811. doi: 10.1093/hmg/ddx177
Kamioka, Y., Fukuhara, S., Sawa, H., Nagashima, K., Masuda, M., Matsuda, M., et al. (2004). A novel dynamin-associating molecule, formin-binding protein 17, induces tubular membrane invaginations and participates in endocytosis. J. Biol. Chem. 279, 40091–40099. doi: 10.1074/jbc.m404899200
Karlsson, E. K., and Lindblad-Toh, K. (2008). Leader of the pack: gene mapping in dogs and other model organisms. Nat. Rev. Genet. 9, 713–725. doi: 10.1038/nrg2382
Kemper, K. E., Reich, C. M., Bowman, P. J., Vander Jagt, C. J., Chamberlain, A. J., Mason, B. A., et al. (2015). Improved precision of QTL mapping using a nonlinear Bayesian method in a multi-breed population leads to greater accuracy of across-breed genomic predictions. Genet. Sel. Evol. 47:29. doi: 10.1186/s12711-014-0074-4
Khoschnau, S., Melhus, H., Jacobson, A., Rahme, H., Bengtsson, H., Ribom, E., et al. (2008). Type I collagen α1 Sp1 polymorphism and the risk of cruciate ligament ruptures or shoulder dislocations. Am. J. Sports Med. 36, 2432–2436. doi: 10.1177/0363546508320805
Kim, J., Williams, F. J., Dreger, D. L., Plassais, J., Davis, B. W., Parker, H. G., et al. (2018). Genetic selection of athletic success in sport-hunting dogs. Proc. Natl. Acad. Sci. U.S.A. 115, E7212–E7221. doi: 10.1073/pnas.180045515
Kim, S. K., Roos, T. R., Roos, A. K., Kleimeyer, J. P., Ahmed, M. A., Goodlin, G. T., et al. (2017). Genome-wide association screens for Achilles tendon and ACL tears and tendinopathy. PLoS One 12:e0170422. doi: 10.1371/journal.pone.0170422
Langhorst, M. F., Solis, G. P., Hannbeck, S., Plattner, H., and Stuermer, C. A. (2007). Linking membrane microdomains to the cytoskeleton: regulation of the lateral mobility of reggie-1/flotillin-2 by interaction with actin. FEBS Lett. 581, 4697–4703. doi: 10.1016/j.febslet.2007.08.074
Lee, C. H., Shin, H. J., Cho, I. H., Kang, Y. M., Kim, I. A., Park, K. D., et al. (2005). Nanofiber alignment and direction of mechanical strain affect the ECM production of human ACL fibroblast. Biomaterials 26, 1261–1270. doi: 10.1016/j.biomaterials.2004.04.037
Li, B., and Dewey, C. N. (2011). RSEM: accurate transcript quantification from RNA-Seq data with or without a reference genome. BMC Bioinformatics 12:323. doi: 10.1186/1471-2105-12-323
Little, J. P., Bleedorn, J. A., Sutherland, B. J., Sullivan, R., Kalscheur, V. L., Ramaker, M. A., et al. (2014). Arthroscopic assessment of stifle synovitis in dogs with cranial cruciate ligament rupture. PLoS One 9:e97329. doi: 10.1371/journal.pone.0097329
Liu, Z., Seefried, F. R., Reinhardt, F., Rensing, S., Thaller, G., and Reents, R. (2011). Impacts of both reference population size and inclusion of a residual polygenic effect on the accuracy of genomic prediction. Gen. Sel. Evol. 43:19. doi: 10.1186/1297-9686-43-19
Lohmander, L. S., Englund, P. M., Dahl, L. L., and Roos, E. M. (2007). The long-term consequence of anterior cruciate ligament and meniscus injuries: osteoarthritis. Am. J. Sports Med. 35, 1756–1769. doi: 10.1177/0363546507307396
Lulińska-Kuklik, E., Maculewicz, E., Moska, W., Ficek, K., Kaczmarczyk, M., Michałowska-Sawczyn, M., et al. (2019). Are IL1B, IL6 and IL6R gene variants associated with anterior cruciate ligament rupture susceptibility? J. Sports Sci. Med. 18, 137–145.
Luo, Y., Sinkeviciute, D., He, Y., Karsdal, M., Henroitin, Y., Mobasheri, A., et al. (2017). The minor collagens in articular cartilage. Protein Cell 8, 560–572. doi: 10.1007/s13238-017-0377-7
Luykenaar, K. D., El-Rahman, R. A., Walsh, M. P., and Welsh, D. G. (2009). Rho-kinase-mediated suppression of KDR current in cerebral arteries requires an intact actin cytoskeleton. Am. J. Physiol. Heart Circ. Physiol. 296, H917–H926.
MacLeod, I. M., Bowman, P. J., Vander Jagt, C. J., Haile-Mariam, M., Kemper, K. E., Chamberlain, A. J., et al. (2016). Exploiting biological priors and sequence variants enhances QTL discovery and genomic prediction of complex traits. BMC Genomics 17:144. doi: 10.1186/s12864-016-2443-6
Mannion, S., Mtintsilana, A., Posthumus, M., van der Merwe, W., Hobbs, H., Collins, M., et al. (2014). Genes encoding proteoglycans are associated with the risk of anterior cruciate ligament ruptures. Br. J. Sports Med. 48, 1640–1646. doi: 10.1136/bjsports-2013-093201
Mi, H., Muruganujan, A., Casagrande, J., and Thomas, P. D. (2013). Large-scale gene function analysis with the PANTHER classification system. Nat. Protoc. 8, 1551–1566. doi: 10.1038/nprot.2013.092
Mi, H., Muruganujan, A., Ebert, D., Huang, X., and Thomas, P. D. (2019). PANTHER version 14: more genomes, a new PANTHER GO-slim and improvements in enrichment analysis tools. Nuc. Acids. Res. 47, D419–D426. doi: 10.1093/nar/gky1038
Montero-Melendez, T., Nagano, A., Chelala, C., Filer, A., Buckley, C. D., and Perretti, M. (2020). Therapeutic senescence via GPCR activation in synovial fibroblasts facilitates resolution of arthritis. Nat. Comm. 11:745. doi: 10.1038/s41467-020-14421
Moser, G., Lee, S. H., Hayes, B. J., Goddard, M. E., Wray, N. R., and Visscher, P. M. (2015). Simultaneous discovery, estimation and prediction analysis of complex traits using a Bayesian mixture model. PLoS Genet. 11:e1004969. doi: 10.1371/journal.pgen.1004969
Muir, P., Schwartz, Z., Malek, S., Kreines, A., Cabrera, S. Y., Buote, N. J., et al. (2011). Contralateral cruciate survival in dogs with unilateral non-contact cranial cruciate ligament rupture. PLoS One 6:e25331. doi: 10.1371/journal.pone.0025331
Nielen, A. L., Knol, B. W., van Hagen, M. A., and van der Gaag, I. (2003). Genetic and epidemiological investigation of a birth cohort of boxers. Tijdschr. Diergeneeskd. 128, 586–590.
Noyes, F. R. (2009). The function of the human anterior cruciate ligament and analysis of single and double-bundle graft reconstructions. Sports Health 1, 66–75. doi: 10.1177/1941738108326980
O’Connell, K., Knight, H., Ficek, K., Leonska-Duniec, A., Maciejewska-Karlowska, A., Sawczuk, M., et al. (2015). Interactions between collagen gene variants and risk of anterior cruciate ligament rupture. Eur. J. Sport Sci. 15, 341–350. doi: 10.1080/17461391.2014.936324
Otsuki, S., Hanson, S. R., Miyaki, S., Grogan, S. P., Kinoshita, M., Asahara, H., et al. (2010). Extracellular sulfatases support cartilage homeostasis by regulating BMP and FGF signaling pathways. Proc. Nat. Acad. Sci.U.S.A. 22, 10202–10207. doi: 10.1073/pnas.0913897107
Otsuki, S., Taniguchi, N., Grogan, S. P., D’Lima, D., Kinoshita, M., and Lotz, M. (2008). Expression of novel extracellular sulfatases Sulf-1 and Sulf-2 in normal and osteoarthritic articular cartilage. Arthritis Res. Ther. 10:R61. doi: 10.1186/ar2432
Parker, H. G., Dreger, D. L., Rimbault, M., Davis, B. W., Mullen, A. B., Carpintero-Ramirez, G., et al. (2017). Genomic analyses reveal the influence of genographic origin, migration, and hybridization on modern dog breed development. Cell Rep. 19, 697–708. doi: 10.1016/j.celrep.2017.03.079
Payne, S. L., Hendrix, M. J., and Kirschmann, D. A. (2006). Lysyl oxidase regulates actin filament formation through the p130Cas/Crk/DOCK180 signaling complex. J. Cell. Biochem. 98, 827–837. doi: 10.1002/jcb.20792
Plaas, A., Sandy, J. D., Liu, H., Diaz, M. A., Schenkman, D., Magnus, R. P., et al. (2011). Biochemical identification and immunolocalizaton of aggrecan, ADAMTS5 and inter-alpha-trypsin–inhibitor in equine degenerative suspensory ligament desmitis. J. Orthop. Res. 29, 900–906. doi: 10.1002/jor.21332
Plassais, J., Kim, J., Davis, B. W., Karyadi, D. M., Hogan, A. N., Harris, A. C., et al. (2019). Whole genome sequencing of canids reveals genomic regions under selection and variants influencing morphology. Nat. Commun. 10:1489. doi: 10.1038/s41467-019-09373-w
Posthumus, M., Collins, M., Van Der Merwe, L., O’Cuinneagain, D., Van Der Merwe, W., Ribbans, W. J., et al. (2012). Matrix metalloproteinase genes on chromosome 11q22 and the risk of anterior cruciate ligament (ACL) rupture. Scand. J. Med. Sci. Sports 22, 523–533. doi: 10.1111/j.1600-0838.2010.01270.x
Posthumus, M., September, A. V., Keegan, M., O’Cuinneagain, D., Van der Merwe, W., Schwellnus, M. P., et al. (2009a). Genetic risk factors for anterior cruciate ligament ruptures: COL1A1 gene variant. Br. J. Sports Med. 43, 352–356. doi: 10.1136/bjsm.2008.056150
Posthumus, M., September, A. V., O’Cuinneagain, D., van der Merwe, W., Schwellnus, M. P., and Collins, M. (2009b). The COL5A1 gene is associated with increased risk of anterior cruciate ligament ruptures in female participants. Am. J. Sports Med. 37, 2234–2240. doi: 10.1177/0363546509338266
Posthumus, M., September, A. V., O’Cuinneagain, D., van der Merwe, W., Schwellnus, M. P., and Collins, M. (2010). The association between the COL12A1 gene and anterior cruciate ligament ruptures. Br. J. Sports Med. 44, 1160–1165. doi: 10.1136/bjsm.2009.060756
Rahim, M., Gibbon, A., Hobbs, H., van der Merwe, W., Posthumus, M., Collins, M., et al. (2014). The association of genes involved in the angiogenesis-associated signaling pathway with risk of anterior cruciate ligament rupture. J. Orthop. Res. 32, 1612–1618. doi: 10.1002/jor.22705
Rahim, M., Mannion, S., Klug, B., Hobbs, H., van der Merwe, W., Posthumus, M., et al. (2017). Modulators of the extracellular matrix and risk of anterior cruciate ligament ruptures. J. Sci. Med. Sport 20, 152–158. doi: 10.1016/j.jsams.2016.07.003
Raleigh, S. M., Posthumus, M., O’Cuinneagain, D., van der Merwe, W., and Collins, M. (2013). The GDF5 gene and anterior cruciate ligament rupture. Int. J. Sports Med. 34, 364–367. doi: 10.1055/s-0032-1316361
Reif, U., and Probst, C. W. (2003). Comparison of tibial plateau angles in normal and cranial cruciate deficient stifles of Labrador retrievers. Vet. Surg. 32, 385–389. doi: 10.1053/jvet.2003.50047
Reiner, A., Yekutieli, D., and Benjamini, Y. (2003). Identifying differentially expressed genes using false discovery rate controlling procedures. Bioinformatics 19, 368–375. doi: 10.1093/bioinformatics/btf877
Rimbault, M., Beale, H. C., Schoenebeck, J. J., Hoopes, B. C., Allen, J. J., Kilroy-Glynn, P., et al. (2013). Derived variants at six genes explain nearly half of size reduction in dog breeds. Genome Res. 23, 1985–1995. doi: 10.1101/gr.157339.113
Robinson, M. D., McCarthy, D. J., and Smyth, G. K. (2010). EdgeR: a Bioconductor package for differential expression analysis of digital gene expression data. Bioinformatics 26, 139–140. doi: 10.1093/bioinformatics/btp616
Sabeti, P. C., Reich, D. E., Higgins, J. M., Levine, H. Z., Richter, D. J., Schaffner, S. F., et al. (2002). Detecting recent positive selection in the human genome from haplotype structure. Nature 419, 832–837. doi: 10.1038/nature01140
Sabeti, P. C., Varilly, P., Fry, B., Lohmueller, J., Hostetter, E., Cotsapas, C., et al. (2007). Genome-wide detection and characterization of positive selection in human populations. Nature 449, 913–918. doi: 10.1038/nature06250
Saunders, C. J., Dashti, M. J., and Gamieldien, J. (2016). Semantic interrogation of a multi knowledge domain ontological model of tendinopathy identifies four strong candidate risk genes. Sci Rep. 6:19820. doi: 10.1038/srep19820
Scheet, P., and Stephens, M. (2006). A fast and flexible statistical model for large-scale population genotype data: applications to inferring missing genotypes and haplotypic phase. Am. J. Hum. Genet. 78, 629–644. doi: 10.1086/502802
Shen, Y., Wen, Z., Li, Y., Matteson, E. L., Hong, J., Goronzy, J. J., et al. (2017). Metabolic control of the scaffold protein TKS5 in tissue-invasive, pro-inflammatory T cells. Nat. Immun. 18, 1025–1034. doi: 10.1038/ni.3808
Smith, H. C., Vacek, P., Johnson, R. J., Slaterbeck, J. R., Hashemi, J., Shultz, S., et al. (2012a). Risk factors for anterior cruciate ligament injury: a review of the literature-part 1: neuromuscular and anatomic risk. Sports Health 4, 69–78. doi: 10.1177/1941738111428281
Smith, H. C., Vacek, P., Johnson, R. J., Slaterbeck, J. R., Hashemi, J., Shultz, S., et al. (2012b). Risk factors for anterior cruciate ligament injury: a review of the literature-part 2: hormonal, genetic, cognitive function, previous injury, and extrinsic risk factors. Sports Health 4, 155–161. doi: 10.1177/1941738111428282
Spana, C., Taylor, A. W., Yee, D. G., Makhlina, M., Yang, W., and Dodd, J. (2019). Probing the role of melanocortin type 1 receptor agonists in diverse immunological diseases. Front. Pharmacol. 9:1535. doi: 10.3389/fphar.2018.01535
Stephens, M., and Balding, D. J. (2009). Bayesian statistical methods for genetic association studies. Nat. Rev. Genet. 10, 681–690. doi: 10.1038/nrg2615
Stȩpień-Słodkowska, M., Ficek, K., Eider, J., Leońska-Duniec, A., Maciejewska-Karłowska, A., Sawczuk, M., et al. (2013). The +1245g/t polymorphisms in the collagen type I alpha 1 (COL1A1) gene in polish skiers with anterior cruciate ligament injury. Biol. Sport 30, 57–60. doi: 10.5604/20831862.1029823
Stȩpień-Słodkowska, M., Ficek, K., Kaczmarczyk, M., Maciejewska-Karłowska, A., Sawczuk, M., Leoñska-Duniec, A., et al. (2015a). The variants within the COL5A1 gene are associated with reduced risk of anterior cruciate ligament injury in skiers. J. Hum. Genet. 45, 103–111. doi: 10.1515/hukin-2015-0011
Stȩpień-Słodkowska, M., Ficek, K., Maciejewska-Karłowska, A., Sawczuk, M., Ziêtek, P., Król, P., et al. (2015b). Overrepresentation of the COL3A1 AA genotype in Polish skiers with anterior cruciate ligament injury. Biol. Sport 32, 143–147. doi: 10.5604/20831862.1144416
Terhaar, H. M., Muir, P., Baker, L. A., Binversie, E. E., Chi, J., and Sample, S. J. (2020). Contribution of habitual activity to cruciate ligament rupture in Labrador Retrievers. Vet. Comp. Orthop. Traumatol. 33, 82–88.
Thompson, J. R., Gögele, M., Weichenberger, C. X., Modenese, M., Attia, J., Barrett, J. H., et al. (2013). SNP prioritization using a Bayesian probability of association. Genet. Epidemiol. 37, 214–221. doi: 10.1002/gepi.21704
Torres de la Riva, G., Hart, B. L., Farver, T. B., Oberbauer, A. M., McV Messam, L. L., Willits, N., et al. (2013). Neutering dogs: effects on joint disorders and cancers in golden retrievers. PLoS One 8:e55937. doi: 10.1371/journal.pone.0055937
Urbinati, C., Ravelli, C., Tanghetti, E., Belleri, M., Giacopuzzi, E., Monti, E., et al. (2012). Substrate-Immobilized HIV-1 tat drives VEGFR2/αvβ3–integrin complex formation and polarization in endothelial cells. Arterioscler. Thromb. Vac. Biol. 32, e25–e34. doi: 10.1161/ATVBAHA.111.242396
van der List, J. P., Mintz, D. N., and DiFelice, G. S. (2017). The location of anterior cruciate ligament tears. Orthop. J. Sports Med. 5, 2325967117709966. doi: 10.1177/2325967117709966
Van Raden, P. M., Van Tassell, C. P., Wiggans, G. R., Sonstegard, T. S., Schnabel, R. D., Taylor, J. F., et al. (2009). Invited review: reliability of genomic predictions for North American Holstein bulls. J. Dairy Sci. 92, 16–24. doi: 10.3168/jds.2008-1514
Voight, B. F., Kudaravalli, S., Wen, X., and Pritchard, J. K. (2006). A map of recent positive selection in the human genome. PLoS Biol. 4:e72. doi: 10.1371/journal.pbio.0040072
Wang, D., Liu, H., Ren, C., and Wang, L. (2019). High expression of ABRACL is associated with tumorigenesis and affects clinical outcome in gastric cancer. Genet. Test Mol. Biomarkers 23, 91–97. doi: 10.1089/gtmb.2018.0195
Whitehair, J. G., Vasseur, P. B., and Willits, N. H. (1993). Epidemiology of cranial cruciate ligament rupture in dogs. J. Am. Vet. Med. Assoc. 203, 1016–1019.
Wiener, P., Sánchez-Molano, E., Clements, D. N., Woolliams, J. A., Haskell, M. J., and Blott, S. C. (2017). Genomic data illuminates demography, genetic structure and selection of a popular dog breed. BMC Genomics. 18:609. doi: 10.1186/s12864-017-3933-x
Wilke, V. L. (2010). “Genetics of cruciate ligament rupture,” in Advances in The Canine Cranial Cruciate Ligament, ed. P. Muir, (Hoboken, NJ: Wiley Blackwell), 53–58. doi: 10.1002/9781118786796.ch7
Wilke, V. L., Conzemius, M. C., and Rothschild, M. F. (2005). SNP detection and association analyses of candidate genes for rupture of the cranial cruciate ligament in the dog. Anim. Genet. 36, 519–521. doi: 10.1111/j.1365-2052.2005.01355.x
Wilke, V. L., Conzemius, M. G., Kinghorn, B. P., Macrossan, P. E., Cai, W., and Rothschild, M. F. (2006). Inheritance of rupture of the cranial cruciate ligament in Newfoundlands. J. Am. Vet. Med. Assoc. 228, 61–64. doi: 10.2460/javma.228.1.61
Wilke, V. L., Zhang, S., Evans, R. B., Conzemius, M. G., and Rothschild, M. F. (2009). Identification of chromosomal regions associated with cranial cruciate ligament rupture in a population of Newfoundlands. Am. J. Vet. Res. 70, 1013–1017. doi: 10.2460/ajvr.70.8.1013
Witsberger, T. H., Villamil, J. A., Schultz, L. G., Hahn, A. W., and Cook, J. L. (2008). Prevalence of and risk factors for hip dysplasia and cranial cruciate ligament deficiency in dogs. J. Am. Vet. Med. Assoc. 232, 1818–1824. doi: 10.2460/javma.232.12.1818
Keywords: ACL rupture, ACL, cruciate rupture, dog model, anterior cruciate ligament, cruciate ligament, GWAS, dog GWAS
Citation: Baker LA, Momen M, McNally R, Berres ME, Binversie EE, Sample SJ and Muir P (2021) Biologically Enhanced Genome-Wide Association Study Provides Further Evidence for Candidate Loci and Discovers Novel Loci That Influence Risk of Anterior Cruciate Ligament Rupture in a Dog Model. Front. Genet. 12:593515. doi: 10.3389/fgene.2021.593515
Received: 14 August 2020; Accepted: 01 February 2021;
Published: 05 March 2021.
Edited by:
Dana C. Crawford, Case Western Reserve University, United StatesReviewed by:
Jessica J. Hayward, Cornell University, United StatesPaul B. Higgins, Philips Research China, China
Elizabeth Hare, Dog Genetics, LLC., United States
Copyright © 2021 Baker, Momen, McNally, Berres, Binversie, Sample and Muir. This is an open-access article distributed under the terms of the Creative Commons Attribution License (CC BY). The use, distribution or reproduction in other forums is permitted, provided the original author(s) and the copyright owner(s) are credited and that the original publication in this journal is cited, in accordance with accepted academic practice. No use, distribution or reproduction is permitted which does not comply with these terms.
*Correspondence: Peter Muir, cGV0ZXIubXVpckB3aXNjLmVkdQ==; Lauren A. Baker, bGF1cmVuLmEuYmFrZXJAd2lzYy5lZHU=