- 1Department of Bioinformatics and Biosciences, Capital University of Science and Technology, Islamabad, Pakistan
- 2Department of Biological Sciences, National University of Medical Sciences, Rawalpindi, Pakistan
- 3Department of Biochemistry, Bahauddin Zakariya University, Multan, Pakistan
- 4Department of Pharmaceutical Chemistry, College of Pharmacy, King Saud University, Riyadh, Saudi Arabia
- 5Department of Pharmacology, College of Pharmacy, King Saud University, Riyadh, Saudi Arabia
- 6Department of Soil Science, College of Food and Agriculture Sciences, King Saud University, Riyadh, Saudi Arabia
- 7Department of Pharmacognosy (MAPPRC), College of Pharmacy, King Saud University, Riyadh, Saudi Arabia
Streptococcus gallolysticus (Sg) is an opportunistic Gram-positive, non-motile bacterium, which causes infective endocarditis, an inflammation of the inner lining of the heart. As Sg has acquired resistance with the available antibiotics, therefore, there is a dire need to find new therapeutic targets and potent drugs to prevent and treat this disease. In the current study, an in silico approach is utilized to link genomic data of Sg species with its proteome to identify putative therapeutic targets. A total of 1,138 core proteins have been identified using pan genomic approach. Further, using subtractive proteomic analysis, a set of 18 proteins, essential for bacteria and non-homologous to host (human), is identified. Out of these 18 proteins, 12 cytoplasmic proteins were selected as potential drug targets. These selected proteins were subjected to molecular docking against drug-like compounds retrieved from ZINC database. Furthermore, the top docked compounds with lower binding energy were identified. In this work, we have identified novel drug and vaccine targets against Sg, of which some have already been reported and validated in other species. Owing to the experimental validation, we believe our methodology and result are significant contribution for drug/vaccine target identification against Sg-caused infective endocarditis.
Introduction
Streptococcus gallolyticus (Sg) is Gram-positive, non-motile bacteria previously referred as Streptococcus bovis. It is phenotypically diverse bacteria belonging to the Lancefield Group D Streptococci (Pasquereau-Kotula et al., 2018; Arjun et al., 2020). This bacterium grows in chain or pairs and is non-γ-hemolytic or slightly γ-hemolytic but sometimes shows alpha-hemolytic activity on ovine blood agar plates (Rusniok et al., 2010; Hensler, 2011). Although commonly present in microflora, approximately 2.5–15% is present in the gastrointestinal tract of a healthy individual (Hinse et al., 2011) and become an opportunistic pathogen causing various diseases, including infective endocarditis, colon cancer, meningitis, and septicemia.
This opportunistic pathogenesis of Sg is dependent on genes involved in polysaccharide production, glucan mucopolysaccharide, a putative component of biofilm produced by this species, and three types of pili and collagen-binding protein (Takamura et al., 2014). These genes provide protection from host immune system and help in adherence to the epithelial lining of the heart (Rusniok et al., 2010), causing infection and resulting in endocarditis (Millar and Moore, 2004).
For the last two decades, a significant rise in incidence of infective endocarditis were observed worldwide (Tripodi et al., 2005; Marmolin et al., 2016; Shahid et al., 2018; Arregle et al., 2019; Chamat-Hedemand et al., 2020). Among 100,000 population, 2.6–7 cases of endocarditis have been reported per year, a significant proportion of which was contributed by streptococcal infections: with incidence of 17% in North America, 31% in other European countries, 39% in the South America, and 32% in rest of the world (Holland et al., 2016). This disease mostly occurs in elderly patients (Firstenberg, 2016), and the median age of patients is ≥58 (Vilcant and Hai, 2018). The risk of developing Sg endocarditis rises with the consumption of uncooked meat or fresh dairy products, weakened immune system, history of hepatic diseases, and comorbidities such as diabetes mellitus and rheumatic disorders (Cãruntu et al., 2014).
In the presence of primary infection, metabolic disorder, or immune-compromised state, Sg tries to cause endocardial injury. This injury then triggers the thrombus formation by the removal of fibrin and platelets. After thrombus formation, the bacteria enters into the bloodstream through the thrombus. As Sg has virulence properties, it can enter into the bloodstream in a paracellular manner without inducing major immune response and adheres to the damaged collagen-rich surface of the cardiac valve (endocardium). Once it is attached to the endocardium, this bacterium proliferates and forms a biofilm, which causes the inflammation in the lining of the heart and causes endocarditis (McDonald, 2009; Hensler, 2011).
Antibacterial drugs such as Penicillin G along with Gentamycin and estreptomicin are preferred medical treatments against infective endocarditis. Other options include Gentamicin-related Ceftriaxone and vancomycin in patients allergic to penicillin (Satué-Bartolomé and Alonso-Sanz, 2009). For patients with persistent fever and resistance to medical therapy, an expensive surgical intervention may be needed (Grubitzsch et al., 2016). Sg is resistant to penicillin, and one of the strains of Sg is also found to be resistant to tetracycline (Hinse et al., 2011). Therefore, development of an efficient treatment strategy against endocarditis, novel therapeutic targets, and potent drugs are urgently required.
For the rapid identification, many computational methods have been established such as core genome and subtractive genomic approaches that allow us to identify the core essential genomes and which do not possess any homology with the human genome (Caputo et al., 2019). These approaches has been used in a number of human pathogens such as Corynebacterium diphtheria (Jamal et al., 2017), Corynebacterium pseudotuberculosis (Tiwari et al., 2014), and Treponema pallidium (Jaiswal et al., 2017). This study is designed with a goal to exploit in silico approaches to link Sg species genomic data with its proteome and to identify the putative therapeutic targets. It can be used to classify potent inhibitors that may contribute to the discovery of compounds that can inhibit pathogenic developments (Jamal et al., 2017). The proteomes from the seven genomes of Sg were compared using a pan genome approach, from which only those genes were selected that were present in all the strains of Sg (Hinse et al., 2011). Then, the predicted core genome was further filtered out on the basis of essentiality for the bacteria, from which only 18 proteins were found to be essential, and all these proteins were non-homologous to the host (human). Out of these 18 proteins, 12 cytoplasmic proteins were identified as drug targets. These essential and non-host homologous protein targets were subjected to virtual screening using a library of 11,993 compounds. The identified putative targets might be used to design peptide vaccines and suggest novel lead druggable compounds that could bind to the proposed target proteins (Barh et al., 2011; Jamal et al., 2017; Uddin et al., 2019).
Materials and Methods
Genome Selection
In the current study, all available strains of Sg with available complete genome were considered for the pan genome analysis. A total of seven strains of Sg were selected; gene and protein sequences were retrieved from NCBI1.
Identification of Core Genomes
The core genome of Sg was identified from pan genome analysis using EDGAR software (Blom et al., 2016). Only those genes that were common in all the strains of Sg were selected. The selection criteria in EDGAR software were as follows: one strain is selected as a reference strain, and rest of all the strains were compared with the reference strains and from which the core genomes were selected that were common in all the strains. The algorithm that it used was protein Basic Local Alignment Search Tool (BLASTp) with the standard scoring matrix BLOSUM62 and cutoff value of E = 1 × 10–5 (Blom et al., 2016).
Identification of Non-host Homologous Proteins
The identified core genome of Sg was then subjected to BLASTp against the human proteome to find out the proteins non-homologous to human host using default parameters e-value = 0.0001, bit score ≥ 100, scoring matrix BLOSUM62 and identity ≥ 25%. Only those proteins that showed no hit against human proteome database were selected (Jamal et al., 2017).
Identification of Essential Genes
The non-host homologous proteins were subjected to BLASTp against Database of Essential Genes (DEG) with the standard scoring matrix BLOSUM62, e-value = 0.001 and identity ≥25% to find out essential proteins that are indispensable for the survival of pathogen. The database of essential genes consist of experimentally validated data from eukaryotes, archaea, and prokaryotes, and it covers a large number of essential genes for 31 bacteria containing more than 12,000 bacterial essential genes (Luo et al., 2014).
Drug Target Prioritization
For the determination of potential therapeutics, several factors are used like molecular weight, molecular function, cellular localization, pathway analysis, and virulence (Agüero et al., 2008). Molecular weight (MW) was determined by ProtParam tool2. Targets whose MW is <100 kDa are considered as best therapeutic target (Mondal et al., 2015). Molecular functions and biological process for target proteins were determined by Uniprot3. Subcellular localization of pathogen was performed by CELLO4. The cellular localization of bacteria determines the environment in which proteins operate. It affects the function of protein by controlling accessibility and availability of all types of molecular interaction partners. The knowledge of protein localization often plays an important role in characterizing the cellular function of hypothetical and newly discovered proteins (Scott et al., 2005). For pathway analysis, the Kyoto Encyclopedia of Genes and Genomes (KEGG) web tool5 was used to determine the role of protein targets in different cellular and metabolic pathways (Kanehisa and Sato, 2020). To identify virulence of protein targets, Virulence Factor Database (VFDB)6 was used, which determines the pathogenic virulence of the target proteins.
Catalytic Pocket Detection
The shortlisted potential druggable proteins were further screened to detect the possible binding pockets by calculating the druggable score using DoGSiteScorer (Volkamer et al., 2012). It is an automated pocket detection tool that is used for the calculation of druggability of protein cavities. This tool needs sequence of interest in 3D structure format; therefore, SwissModel was used for the prediction of the 3D structure. SwissModel web tool predicts the 3D structures of protein targets (Nielsen et al., 2010). After obtaining 3D structures, the druggability evaluation was performed by DoGSiteScorer. This tool returns the pocket residue and druggability score, which ranges from 0 to 1. The score closer to 1 is considered as a highly druggable protein cavity (Jamal et al., 2017).
Retrieval of Ligands
Eleven thousand nine hundred ninety-three druggable molecules with Tonimoto cutoff level of 60% were retrieved from the ZINC database (Sterling and Irwin, 2015). Then, partial charges were calculated, and energies of these compounds were minimized using energy minimization algorithm with default parameters. All minimized structures were saved in.mdb file. Then, these prepared ligands were used as an input file for molecular docking (Wadood et al., 2014).
Validation of 3D Structures
All the 3D structures quality was further validated using RAMPAGE and ERRAT tool. RAMPAGE stands for RNA Annotation and Mapping of Promoters for the Analysis of Gene Expression. This tool does Ramachandran plot analysis and provides validity score for the 3D structure of target proteins. The score ≥80 were considered good (Batut and Gingeras, 2013). For further validation, ERRAT, an online tool, was used, which provides information about the protein structure with bad regions. The quality factor of the 3D structure ≥37% were considered good (Saddala and Adi, 2018).
Preparation of Protein for Docking
The predicted 3D structures were further prepared for docking using the Molecular Operating Environment (MOE) tool. This tool is quite robust along with the meticulous algorithm. It not only predicts the top ranking poses but also prognosticate the root mean-square deviation (RMSD) along with the calculated energies of docked molecule (Pagadala et al., 2017). The 3D protonation and energy minimization of these 3D structures was done (Vilar et al., 2008); then, these minimized structures were further used as template for molecular docking.
Molecular Docking of Drug Targets
The prepared minimized structures of targeted proteins and ligands were further subjected to molecular docking carried out in MOE using the MOE Dock (Figure 1). It predicted the favorable binding possess of selected ligands active sites of drug targets. Default parameters were selected for molecular docking. After the docking, we analyzed the best poses for hydrogen bonding/π–π interactions, and then, RMSD was calculated in MOE (Wadood et al., 2014). The orientation of the best dock molecules was further analyzed in chimera.
Results and Discussion
Genome Selection
The seven strains of Sg were retrieved from the National Center for Biotechnology Information (NCBI)7. The selection was based on the availability of their complete genome to have accuracy in our result. The details of the selected strains are summarized in Table 1.
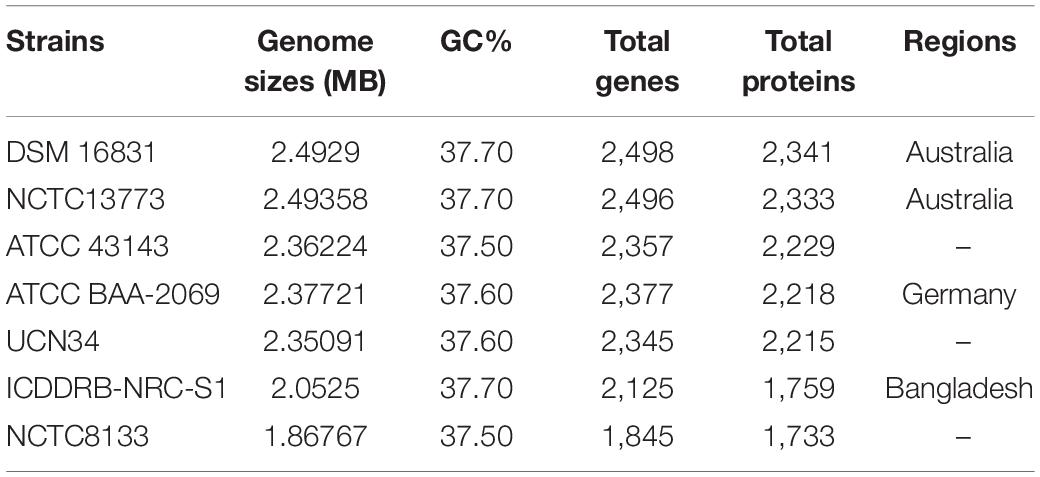
Table 1. Strains of Streptococcus gallolyticus with information on genome statistics and regions of isolation.
Identification of Core Genomes
Core genome was identified to find drug targets that are homologous to all strains. Basically, only those core genomes that were defined as genes persistently present in all population of an organism were extracted (Uddin and Jamil, 2018). Core genomes were identified from pan genome analysis using EDGAR software. UCN34 strain was selected as reference genome, and the rest of the strains were compared to the reference strain. The total genes identified in pan genome were 3,242, out of which 1,138 were core genes.
Identification of Non-host Homologous Proteins
The file generated by NCBI-BLASTp of Sg core genomes against human was parsed. Amidst 1,138 of core genomes, 1,115 proteins showed no hit and hence selected as non-homologous to the human proteome to avoid the aftereffect.
Identification of Essential Genes
The core 1,115 non-host homologous proteins were subjected to BLASTp against essential proteins present in DEG (Luo et al., 2014). The number of non-homologous proteins that is essential for the survival of the pathogen was 176. Among these, 18 proteins were selected as potential drug targets whose percent identity was >25, shown in Table 2. Out of these 18 proteins, 12 cytoplasmic proteins were selected as potential drug targets. The selection of final set of drug targets was kept strict to percentage identity to host, essentiality, and cutoff values.
Drug Target Prioritization
To determine the potential therapeutic targets, various factors were considered, including molecular weight from ProtParam (ExPASy, 2020; ProtParam documentation) of all 12 proteins was <100 kDa; therefore, these molecules featured as “druggable” molecule (Hughes et al., 2011). All these druggable molecules were analyzed using BLASTp against virulence factor database of VFDB (Chen et al., 2005), which predicts all therapeutics targets as virulent. Subcellular localization is a key factors in determining the function of protein. The CELLO (Yu et al., 2006) was used to predict the subcellular localization of 12 query proteins. These query proteins were further subjected for pathway analysis using KEGG database (Kanehisa et al., 2017). It appeared that most of the proteins are involved in metabolic pathways like enzymes, glycosyltransferases, peptidoglycan biosynthesis, degradation of proteins, and lipids biosynthesis proteins. Whereas a few of them are involved in cell signaling and cell processing such as secretion system and two-component system, very few proteins were involved in genetic information processing and resistance pathway such as transcription factor, ribosomes, DNA replication protein, mitochondrial biogenesis, β-lactam pathway, and vancomycin resistance pathway. The details about the drug target prioritization parameters and functional annotation of 12 essential non-host homologous proteins are shown in Table 3.
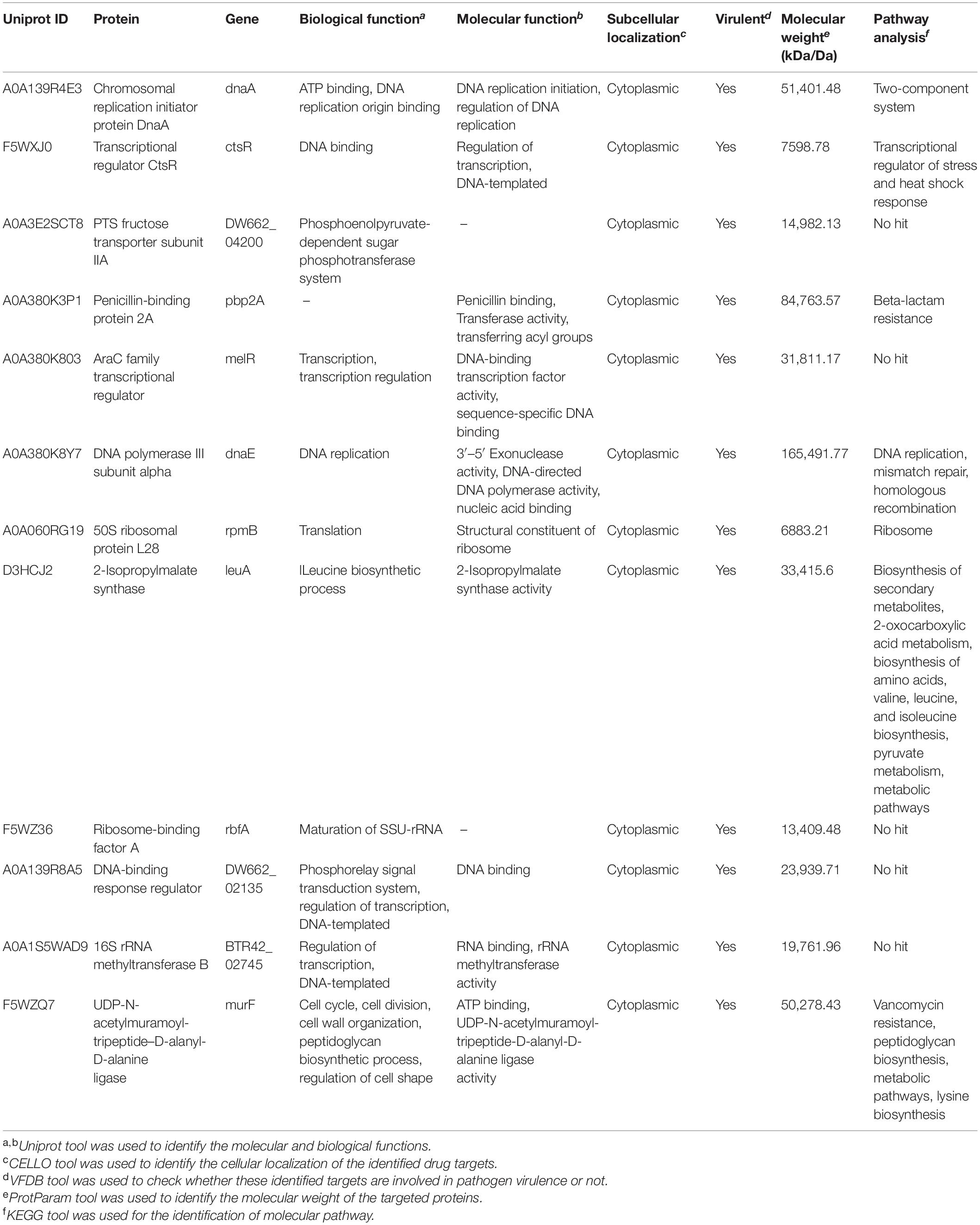
Table 3. Drug and vaccine target prioritization parameters and functional annotation of 12 essential non-host homologous proteins.
Quality factor of 3D structures of druggable proteins were further validated through RAMPAGE and ERRAT. Quality factor predicted by both tool was ≥80 and ≥37%, respectively, as shown in Table 4. This score shows that our protein 3D structures are good and could be prepared for docking.
Docking
Docking was performed against 12 drug targets with 11,993 ZINC druggable compounds via MOE tool. Top 100 molecules were redocked into the binding pocket of target proteins, and finally, a set of top 10 molecules was selected. The orientation of best docked molecule was analyzed in Chimera.
Validation of docking
In order to validate the MOE Dock program, the cocrystallized ligand was removed from the active site and redocked within the inhibitor binding cavity of penicillin-binding protein (PDB ID: 3vsl). In this study, RMSD value (Figure 2) was found as 1.0968 Å, showing that our docking method is valid for the studied druggable molecules, and MOE Dock method, therefore, is reliable for docking of these compounds.
The analysis and biological significance of each of the predicted protein–ligand interaction are described as follows.
16S rRNA methyltransferase B (BTR42_02745) is a protein that plays an important role in methylation of cytosine at position 967 of 16S rRNA. The structure of this protein consists of active sites in which two conserved cysteine residues are present. These cysteine residues are located near the activated methyl of cofactor. One of the cysteine residues act as a catalytic nucleophile and other play an important role in methyl transferase mechanism (Foster et al., 2003; Shen et al., 2020). The top 10 best confirmations are shown in Table 5 along with their ZINC ID, number of interactions, interacting residues, dock score, and minimized energy. The residues Lys 285, Lys 339, and Cys330 were found to interact with active ligand (ZINC01532584). The interaction of 16S rRNA methyltransferase B with ZINC01532584 is shown in Figure 3.
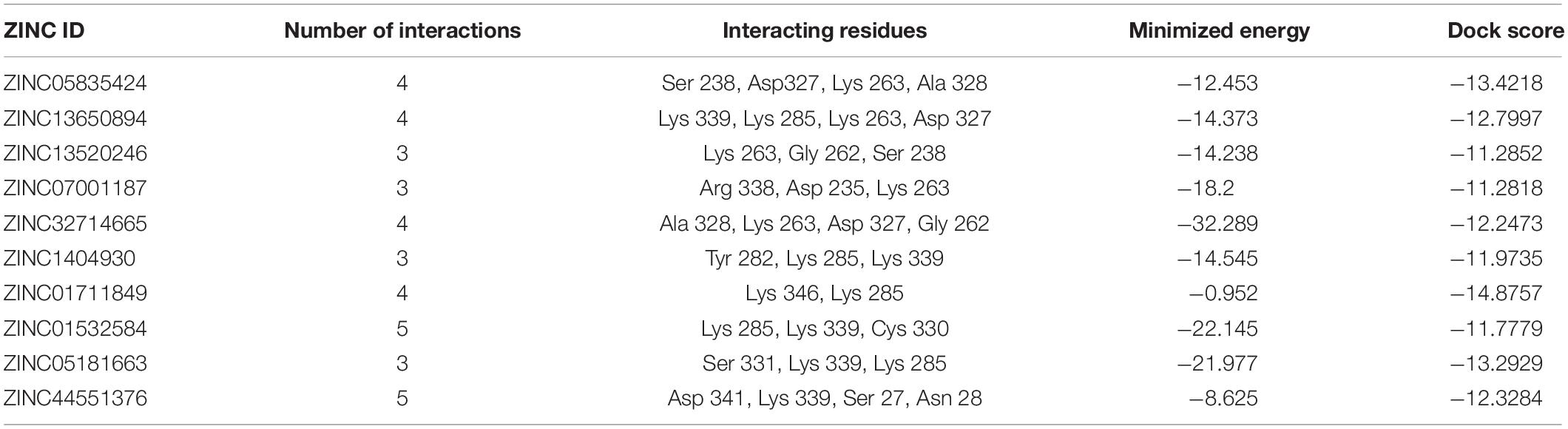
Table 5. 16S rRNA methyltransferase B and its interaction profile with docked compounds, their ZINC ID, minimized energy, number of interactions, dock score, and interacting residues.
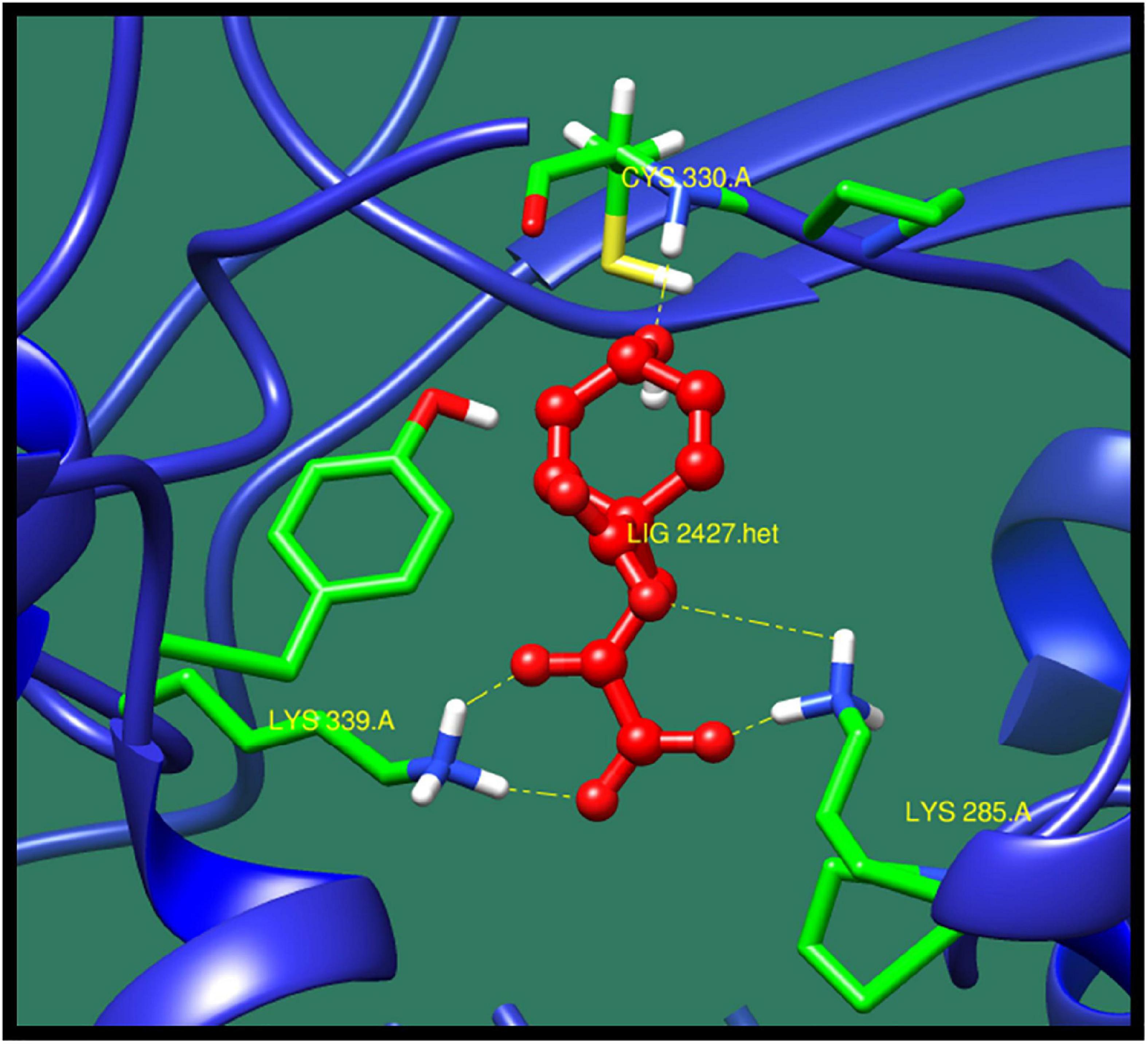
Figure 3. Interaction of 16S rRNA methyltransferase B with ZINC01532584 (colored in red). The interacting residues (green) are shown bonding (dotted lines) with the ligand.
Chromosomal replication initiator protein DnaA (dnaA) is a protein that plays a significant role in initiation and regulation of chromosomal replication. In DNA regulation, the initiation process is the key event in the cell cycle of all organism. The initiation of replication starts at the site of origin, which is recognized and processed by the initiator protein. The structure of this protein consist of nucleotide binding folds with the long helical connector to all-helical DNA binding domain. The conserved motif of this protein provide information about two most important steps in origin processing, which are binding of DNA and homo-oligomerization (Erzberger et al., 2002). Table 6 presents top 10 protein–ligand interaction with ZINC ID, minimized energy, number of interactions, Dock score, and interactive residues. ZINC71782058 was predicted as the most active lead compound against chromosomal replication initiator protein DnaA (dnaA). The protein–ligand interaction is shown in Figure 4.
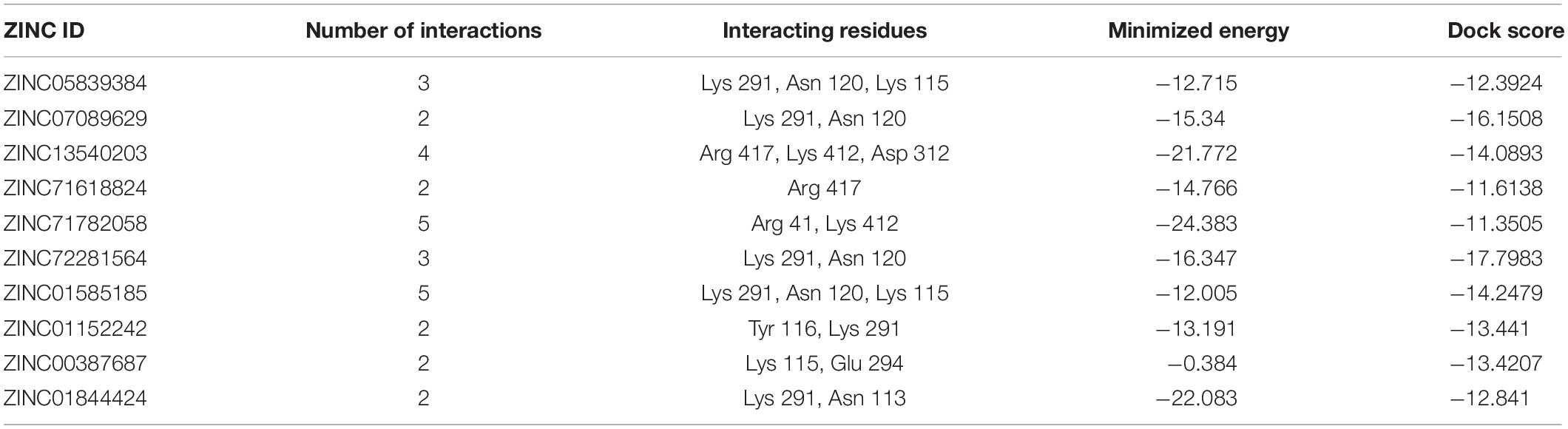
Table 6. Chromosomal replication initiator protein DnaA and its interaction profile with docked compounds, their ZINC ID, minimized energy, number of interactions, dock score, and interactive residues.
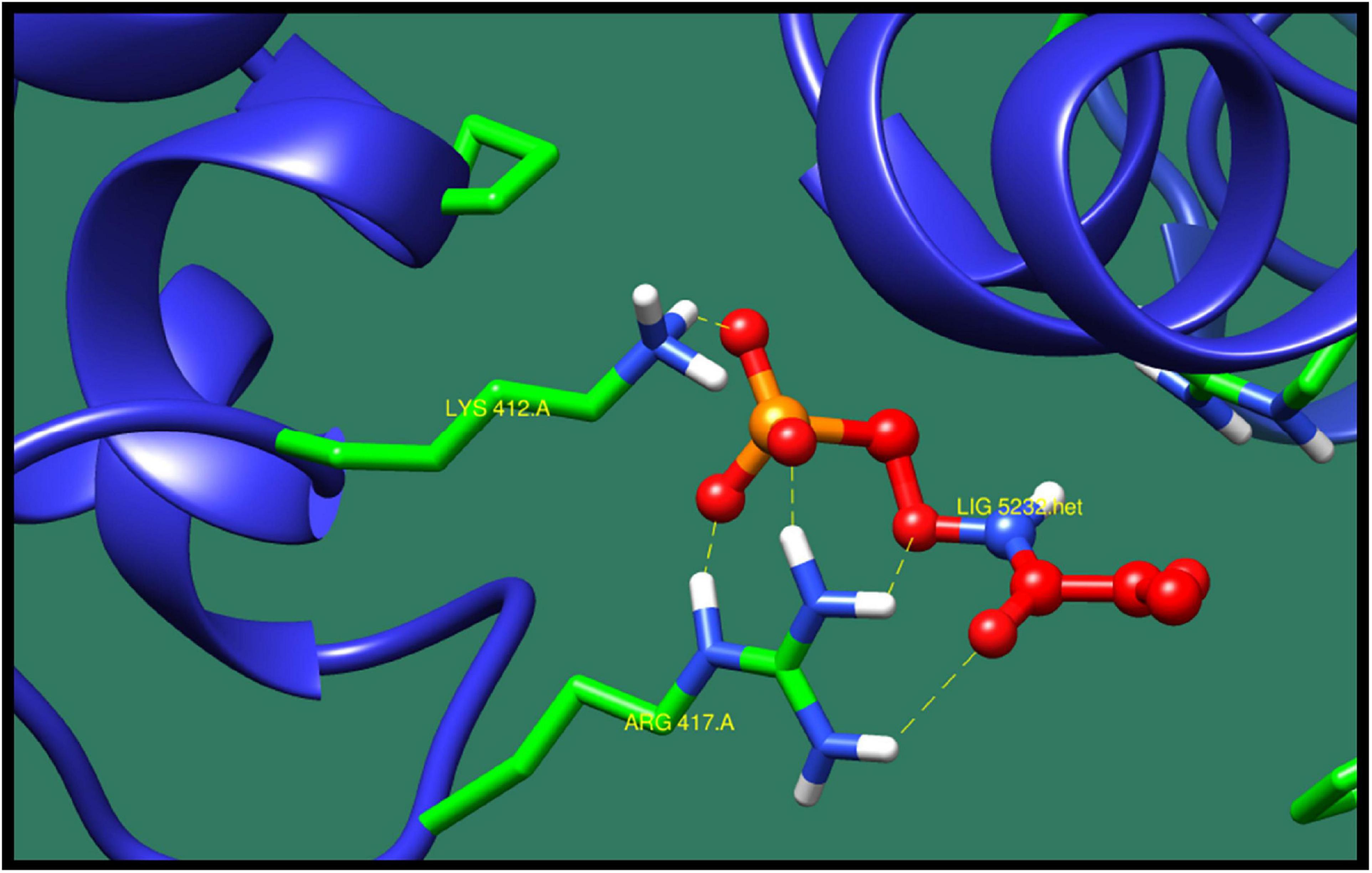
Figure 4. Interaction of chromosomal replication initiator protein DnaA with ZINC71782058 (colored in red). The interacting residues (green) are shown bonding (dotted lines) with the ligand.
Transcriptional regulator CtsR (ctsR) is an important repressor that regulates the transcription of class III stress genes in Gram-positive bacteria. CtsR controls the expression of genes encoding for chaperons and proteases. These genes play an important role in protein quality control system of bacteria. The structure of this protein consist of N-terminal DNA binding domain and C-terminal dimerization domain. N-Terminal DNA binding domain consists of helix-turn-helix (HTH) folds, and C-terminal dimerization domain consist of α-helices organized in four helix bundle. This protein also play an important role in pathogenicity, as it provides benefit to the bacteria during its stress condition and improves the survival chances for bacteria (Fuhrmann et al., 2009). Top 10 lead molecules against this protein are shown in Table 7 consisting ZINC ID, minimized energy, dock score, numbers of interactions, and interacting residues. The best interaction was shown by ZINC79090716 as shown in Figure 5.
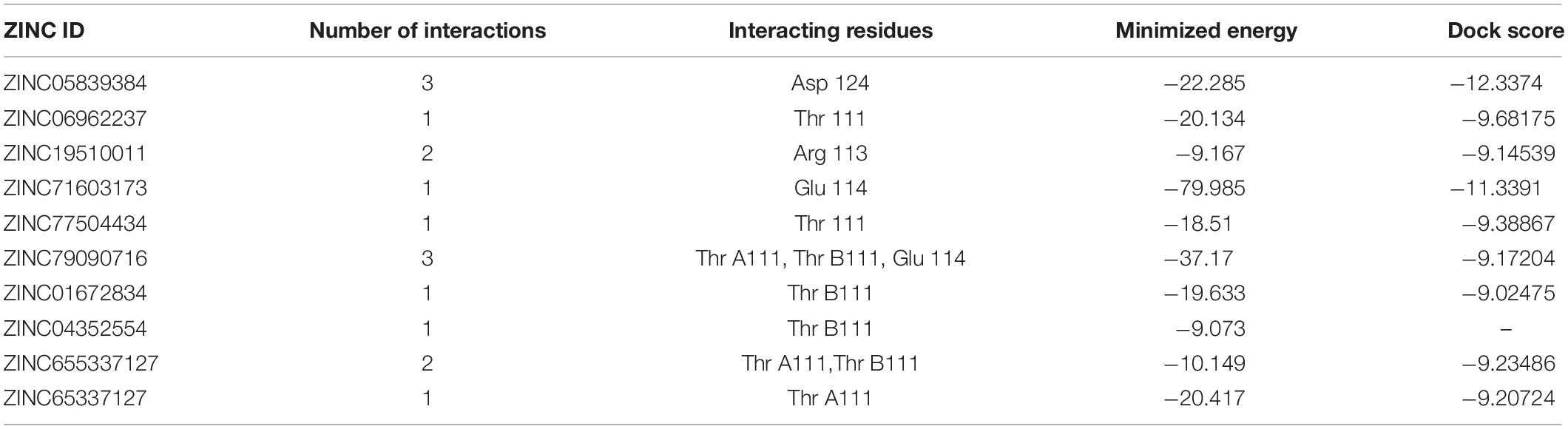
Table 7. Transcriptional regulator CtsR and its interaction profile with docked compounds their ZINC ID, minimized energy, number of interactions, dock score, and interactive residues.
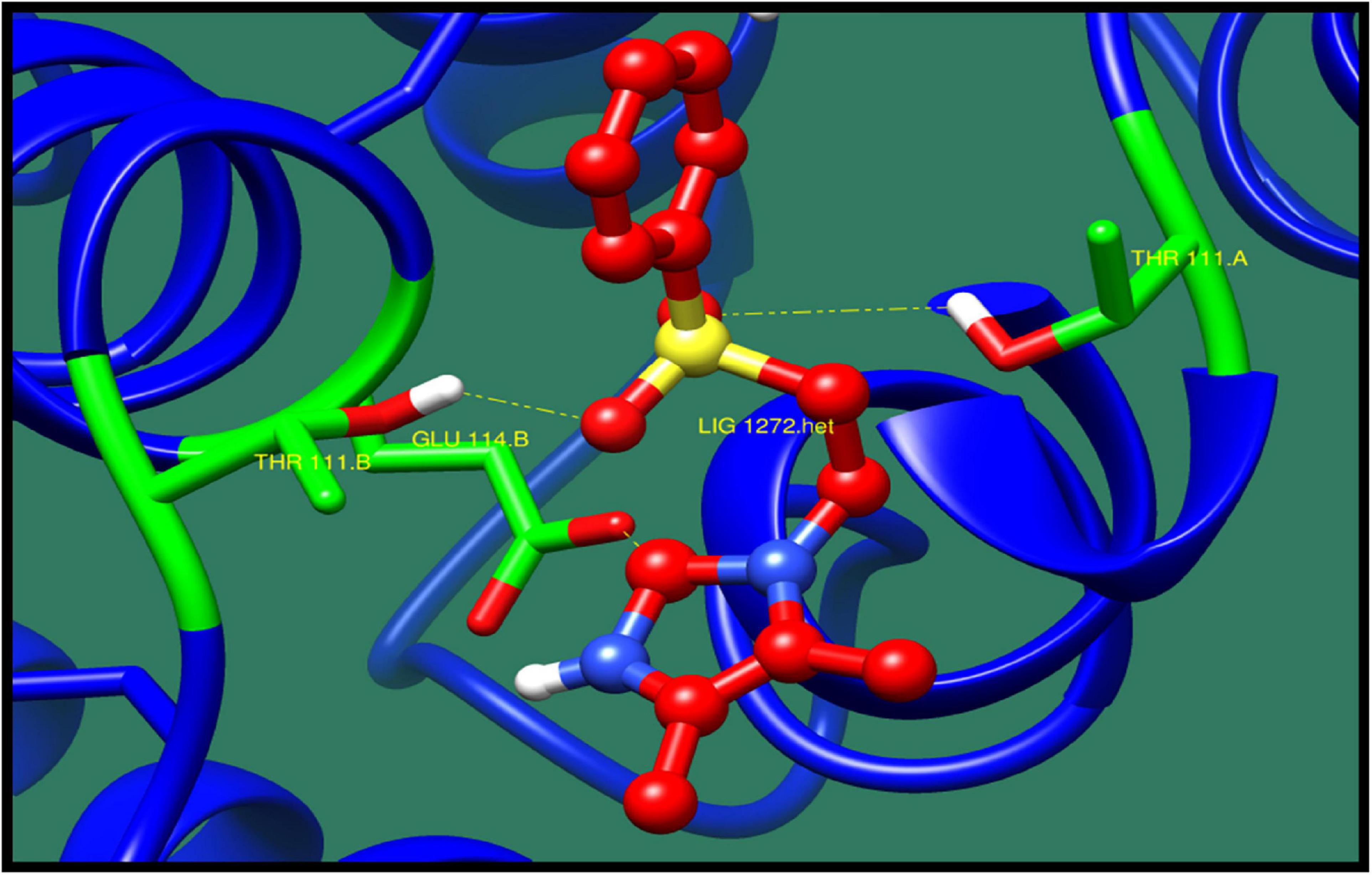
Figure 5. Interaction of transcriptional regulator CtsR with ZINC79090716 (colored in red). The interacting residues (green) are shown bonding (dotted lines) with the ligand.
Phosphotransferase system (PTS) fructose transporter subunit IIA (DW662_04200) is a protein that is involved in phosphoenolpyruvate-dependent sugar PTS. In bacteria, it is a major carbohydrate transport system. PTS catalyzes the translocation with naturally occurring phenomenon of phosphorylation of sugar and hexitols, and it also regulates the metabolism in response to the availability of carbohydrates. It consists of two proteins HPr and enzyme I protein. These are the cytoplasmic proteins, in which first enzyme I transfers phosphoryl groups from phosphoenolpyruvate to phosphoryl carrying protein HPr. Then, this HPr further transfers the phosphoryl group to different transport complexes. PTS fructose transporter subunit IIA belongs to the fructose–mannitol family. This is a large and complex family that consists of several sequenced fructose and mannitol-specific permeases and putative permeases of unknown specificities. This family have three domains, IIA, IIB, and IIC, from which the most specific domain is IIA for the fructose PTS transporters (Siebold et al., 2001). The top 10 protein–ligand interaction is shown in Table 8, and the best interaction is shown in Figure 6 with ZINC01638334.
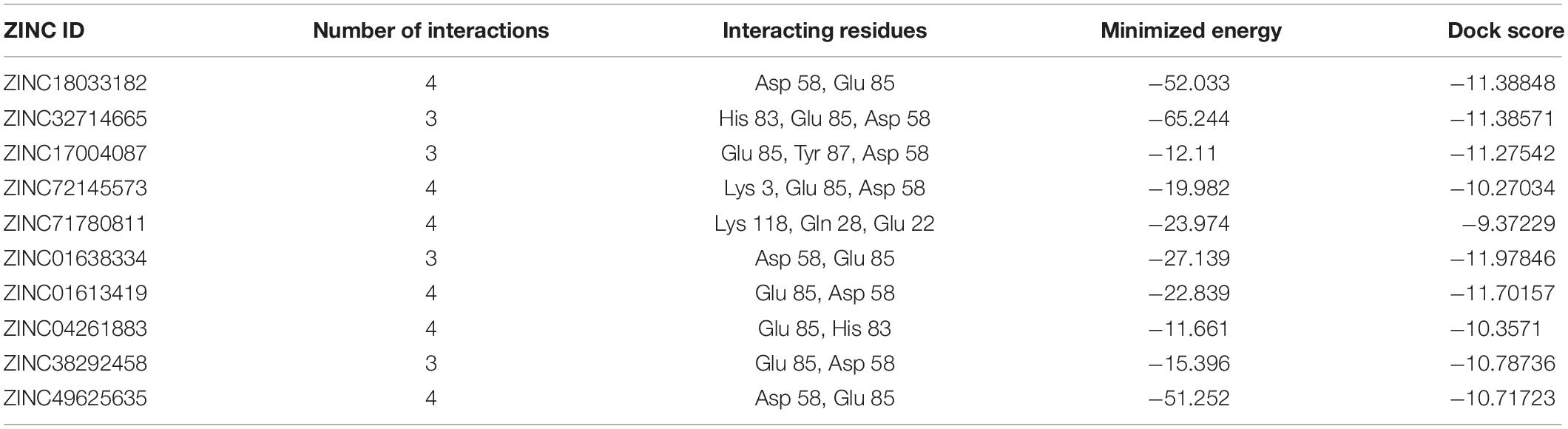
Table 8. Phosphotransferase system (PTS) fructose transporter subunit IIA and its interaction profile with docked compounds, their ZINC ID, minimized energy, number of interactions, dock score, and interactive residues.
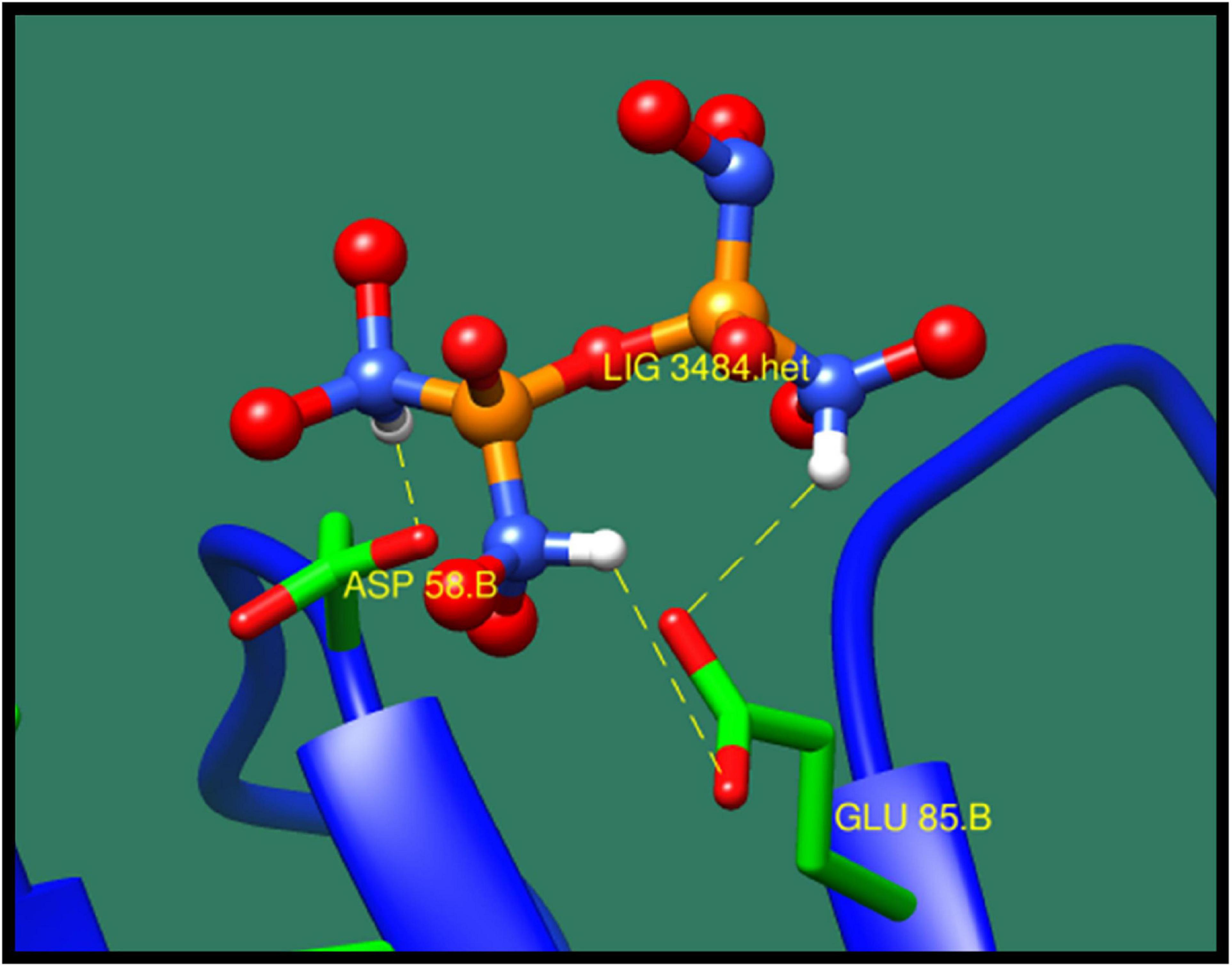
Figure 6. Interaction of phosphotransferase system (PTS) fructose transporter subunit IIA with ZINC01638334 (colored in red). The interacting residues (green) are shown bonding (dotted lines) with the ligand.
Penicillin-binding protein 2A (pbp2A) is a transpeptidase that catalyzes the cell wall crosslinking, which is quite essential for the growth and survival of bacteria. This protein activation is regulated by active site at which the crosslinking take place (Fishovitz et al., 2014). Through pathway analysis, it is clear that it is involved in β-lactam resistance pathway. β-Lactam antibiotic is the most used group of antibiotics, which exerts its effect by interfering with the bacterial cell wall by structural crosslinking of peptidoglycan. This protein has already been reported as β-lactam resistant. This antibiotic resistance is due to the inactivation of the enzymes, change in β-lactam targets of pbp, change in porins, and use of efflux pump (Kocaoglu and Carlson, 2015). The top-ranked lead compounds are given in Table 9 where compound ZINC16942644 was predicted as best on the basis of minimized energy, dock score, and number of interactions made (Figure 7).
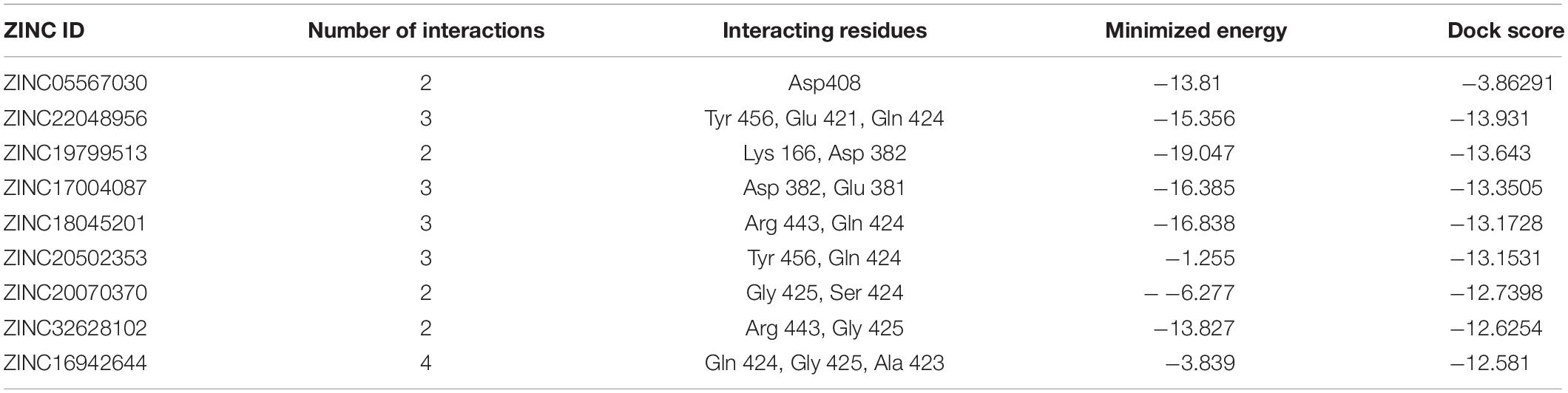
Table 9. Penicillin-binding protein 2A and its interaction profile with docked compounds, their ZINC ID, minimized energy, number of interactions, dock score, and interactive residues.
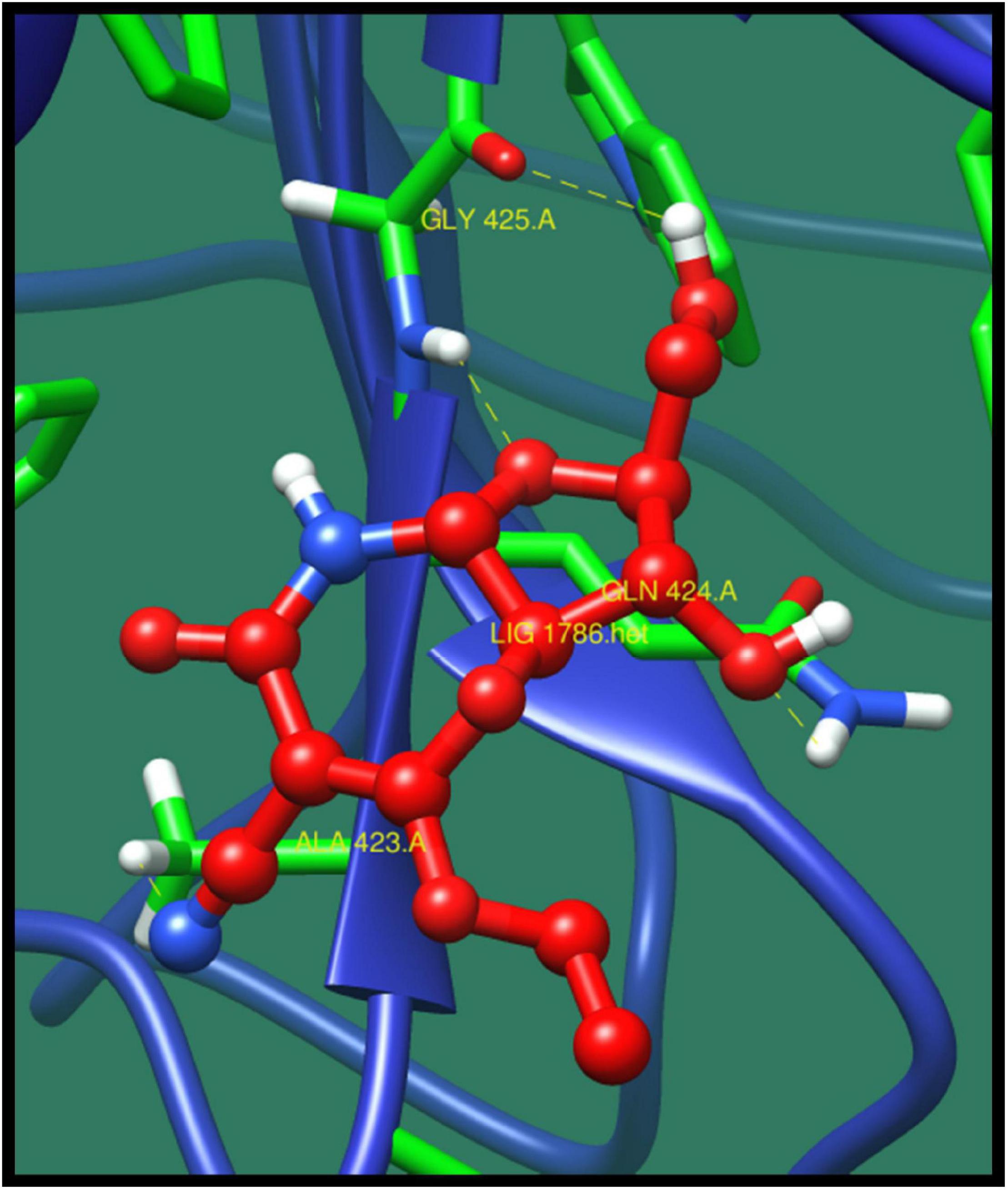
Figure 7. Interaction of penicillin-binding protein 2A with ZINC16942644 (colored in red). The interacting residues (green) are shown bonding (dotted lines) with the ligand.
UDP-N-acetylmuramoyl-tripeptide-D-alanyl-D-alanine ligase (murF) is a protein involved in the biosynthesis of peptidoglycan. Peptidoglycan is the important component of bacterial cell wall, and enzymes involved in its synthesis could represent as potential drug target. MurF catalyzes the final step in the biosynthesis of the peptidoglycan in which it adds the D-Ala–D-Ala to the nucleotide precursor UDP-MurNAc-L-Ala-γ-D-Glu-meso-DAP (Hrast et al., 2013). The protein–ligand interaction of the top 10 molecules is shown in Table 10, and among these molecules, the best interaction was with ZINC14681317 as shown in Figure 8.
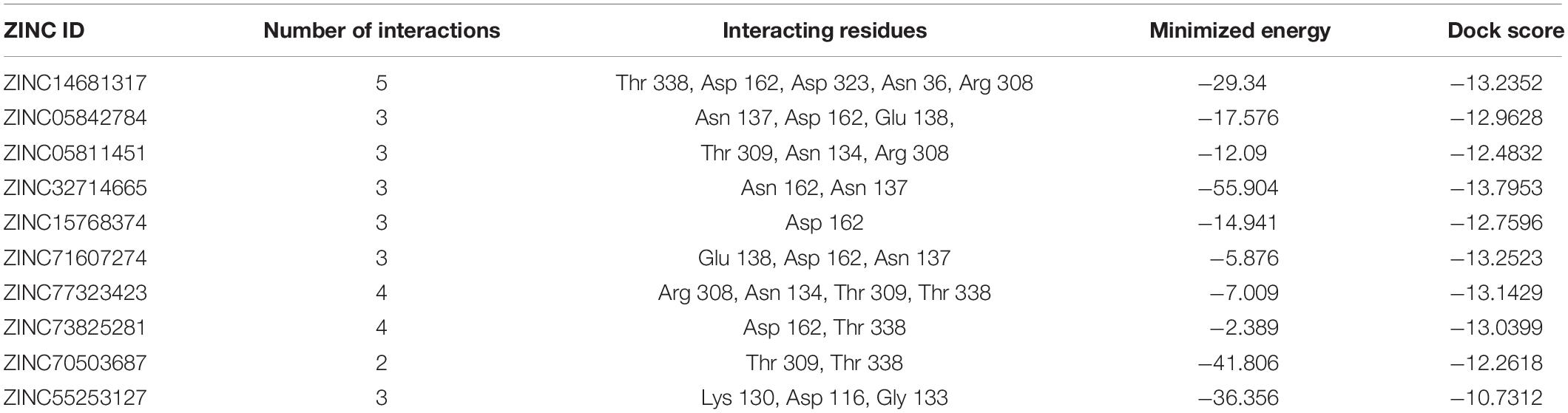
Table 10. UDP-N-acetylmuramoyl-tripeptide–D-alanyl-D-alanine ligase and its interaction profile with docked compounds, their ZINC ID, minimized energy, number of interactions, dock score, and interactive residues.
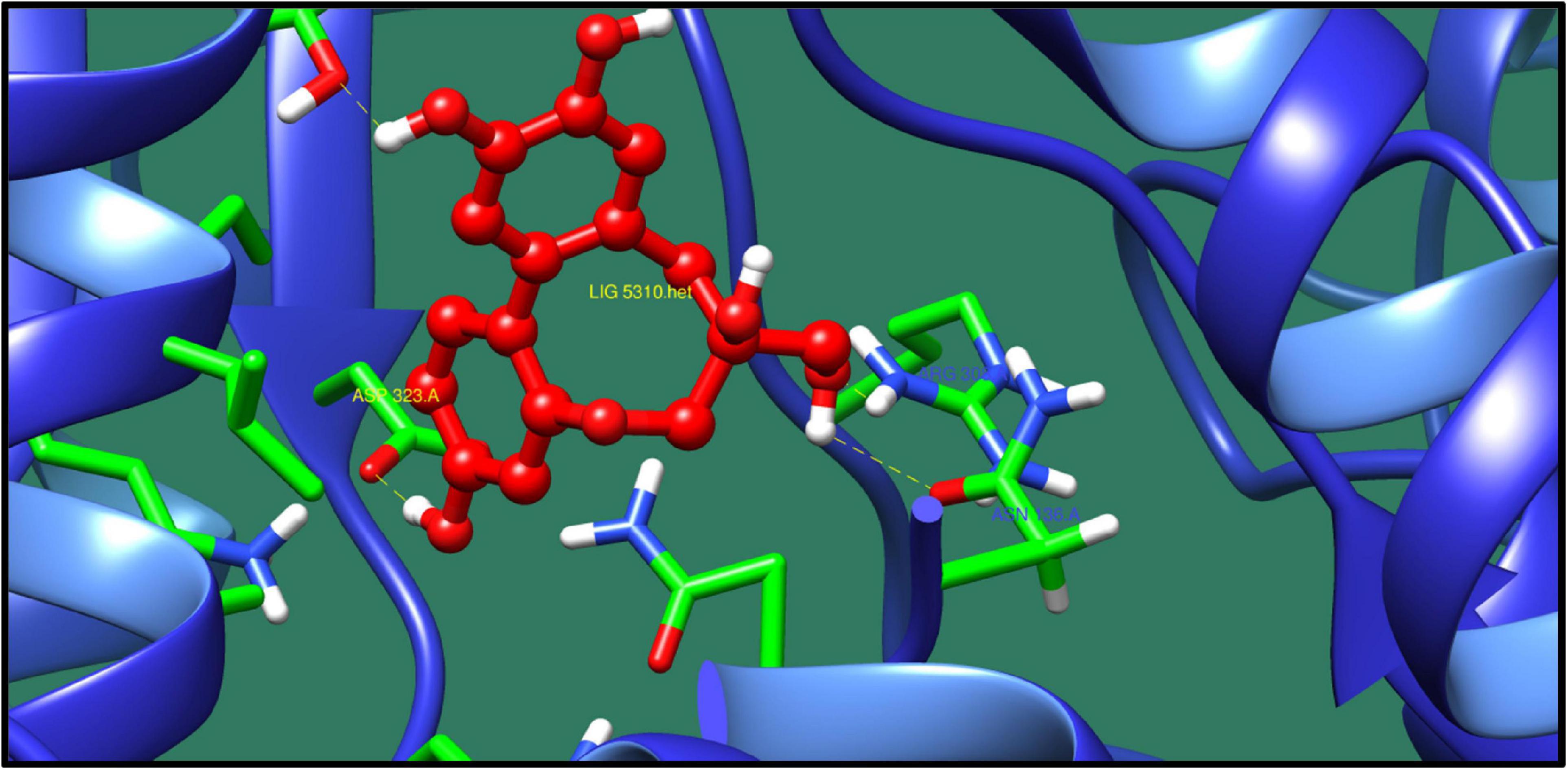
Figure 8. Interaction of UDP-N-acetylmuramoyl-tripeptide–D-alanyl-D-alanine ligase with ZINC14681317 (colored in red). The interacting residues (green) are shown bonding (dotted lines) with the ligand.
AraC family transcriptional regulator (melR) protein belongs to Arac/XylS family. This is a family of transcription regulators and is widely distributed in bacteria. This protein regulates the transcription of several genes and operons that are involved in arabinose catabolism and transport. This protein coregulates with another transcription regulator that is also involved in degradation of I-arabinose. By binding together, these regulators activate the transcription of five operons that are involved in transport, catabolism, and autoregulation of I-arabinose. Its structure is composed of C-terminal DNA binding domain and N-terminal domain. C-Terminal DNA binding domain consists of two HTHs that are connected with α-helix, and N-terminal domain is responsible for dimerization and binding of I-arabinose. The structure of this reveal that the N-terminal of this protein plays an important role in regulation of arabinose (Rodgers and Schleif, 2009; Fernandez-López et al., 2015; Malaga et al., 2016). Table 11 presents the best results against AraC family transcriptional regulator (melR) where ZINC71781167 was predicted as top lead compound as shown in Figure 9.
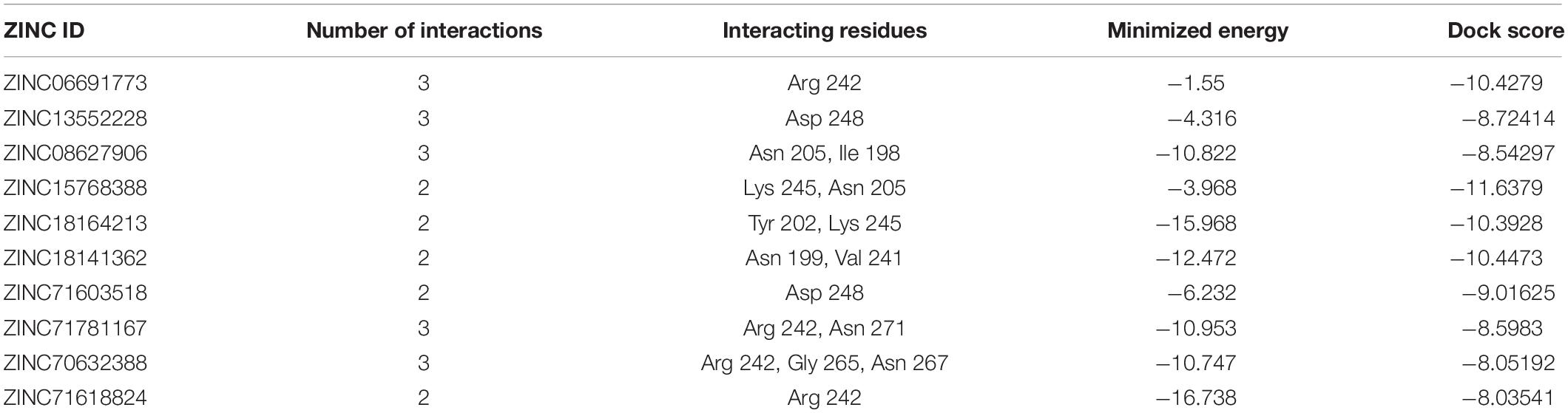
Table 11. AraC family transcriptional regulator and its interaction profile with docked compounds, their ZINC ID, minimized energy, number of interactions, dock score, and interactive residues.
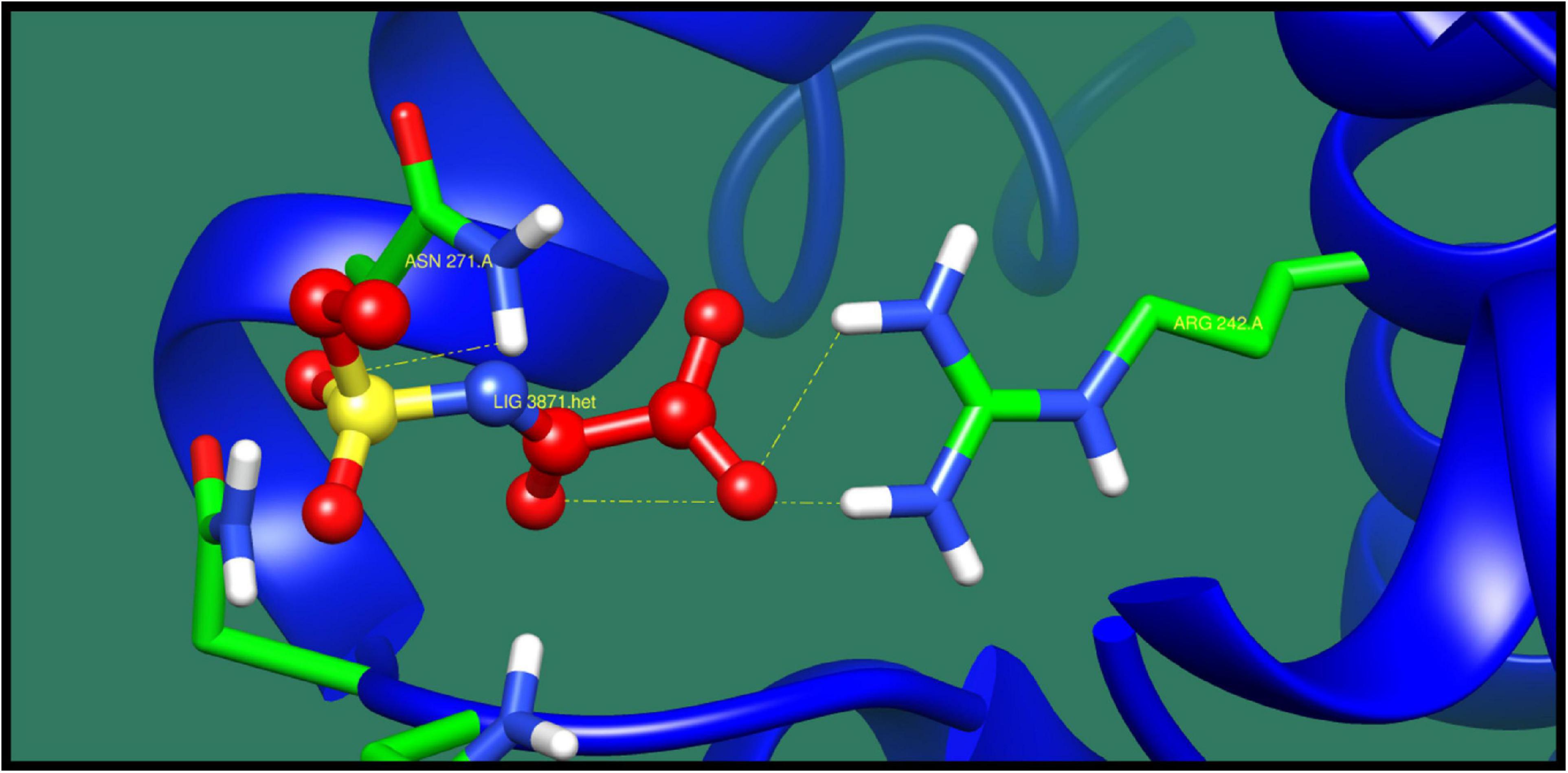
Figure 9. Interaction of AraC family transcriptional regulator with ZINC71781167 (colored in red). The interacting residues (green) are shown making bonding (dotted lines) with the ligand.
DNA polymerase III subunit alpha (dnaE) is responsible for the replication in bacterial genome. This protein function as tripartite assembly consisting two core polymerases. In Escherichia coli, the core polymerases contain the catalytic α-subunit also known as PolIIIα, the 3′–5′ exonuclease ε-subunit and the θ subunit whose function is essentially unknown (Wing et al., 2008). From the function and pathway analysis, this protein is involved in DNA replication, mismatch repair pathway, and homologous recombination. It is located in the cytoplasm, which means it could act as drug target. The top 10 interaction of this protein with ligands is shown in Table 12 along with their ZINC ID, minimized energy, number of interactions, dock score, and interactive residues. The binding pocket residues Arg955, Lys553, Gln556, and Arg554 were predicted to contribute in the interaction with lead molecule ZINC38653615 as shown in Figure 10.
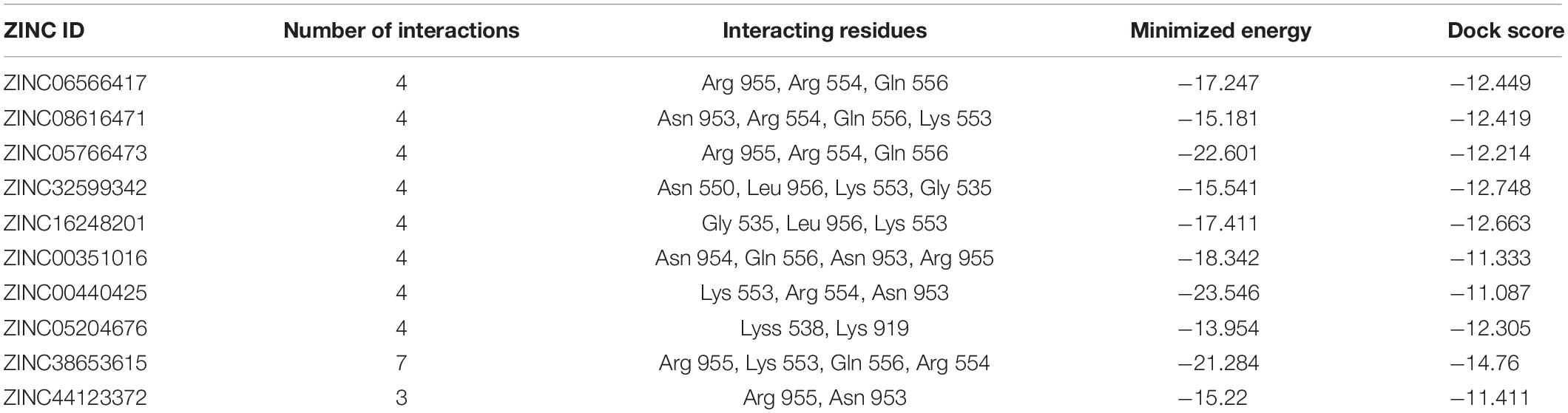
Table 12. DNA polymerase III subunit alpha and its interaction profile with docked compounds, their ZINC ID, minimized energy, number of interactions, dock score, and interactive residues.
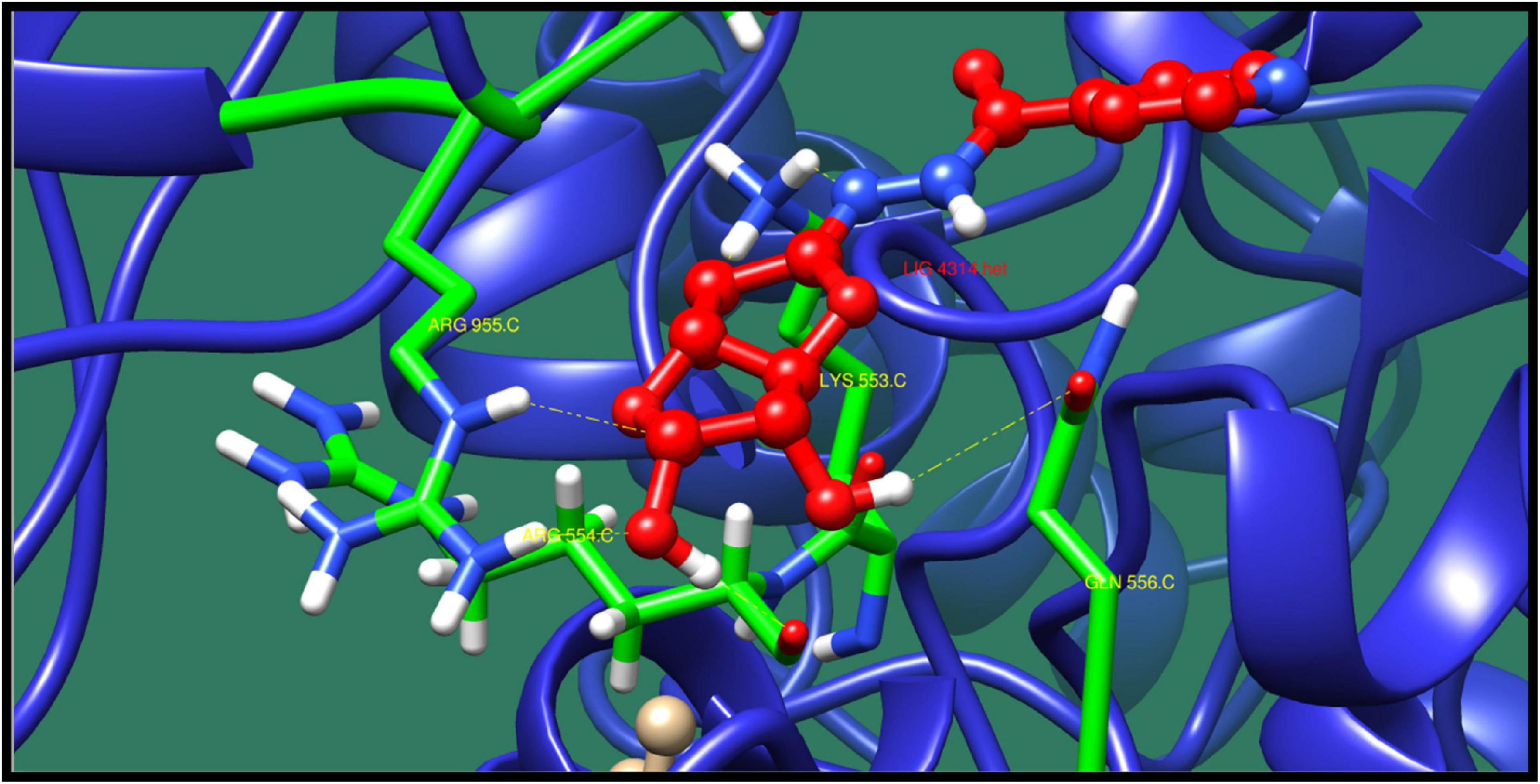
Figure 10. Interaction of DNA polymerase III subunit alpha with ZINC38653615 (colored in red). The interacting residues (green) are shown bonding (dotted lines) with the ligand.
50S ribosomal protein L28 (rpmB) protein plays an important role in the assembly of ribosome. This protein is encoded by rpmB operon. This protein could act as potential drug target as its role in ribosome assembly and functioning (Aseev et al., 2016). The functional analysis also showed its role in translation and structural constituent in ribosomes, which makes it a good drug target. The top 10 results of 50S ribosomal protein L28 protein is shown in Table 13 along with their ZINC ID, minimized energy, dock score, number of interactions, and interactive residues, and the best interaction was observed with ZINC03872713 shown in Figure 11.
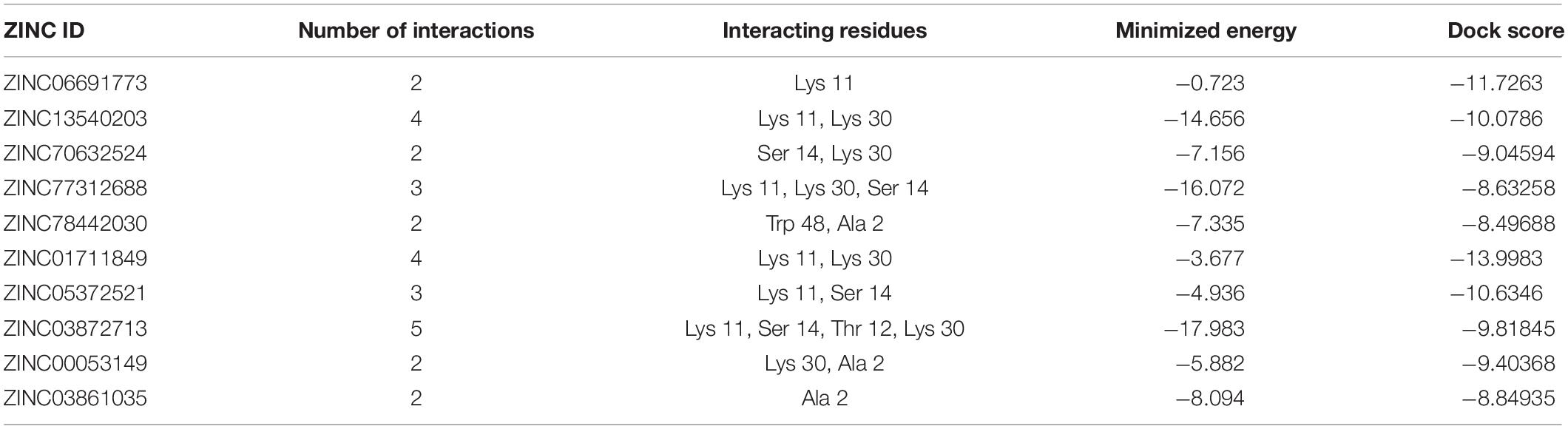
Table 13. 50S ribosomal protein L28 and its interaction profile with docked compounds, their ZINC ID, minimized energy, number of interactions, dock score, and interactive residues.
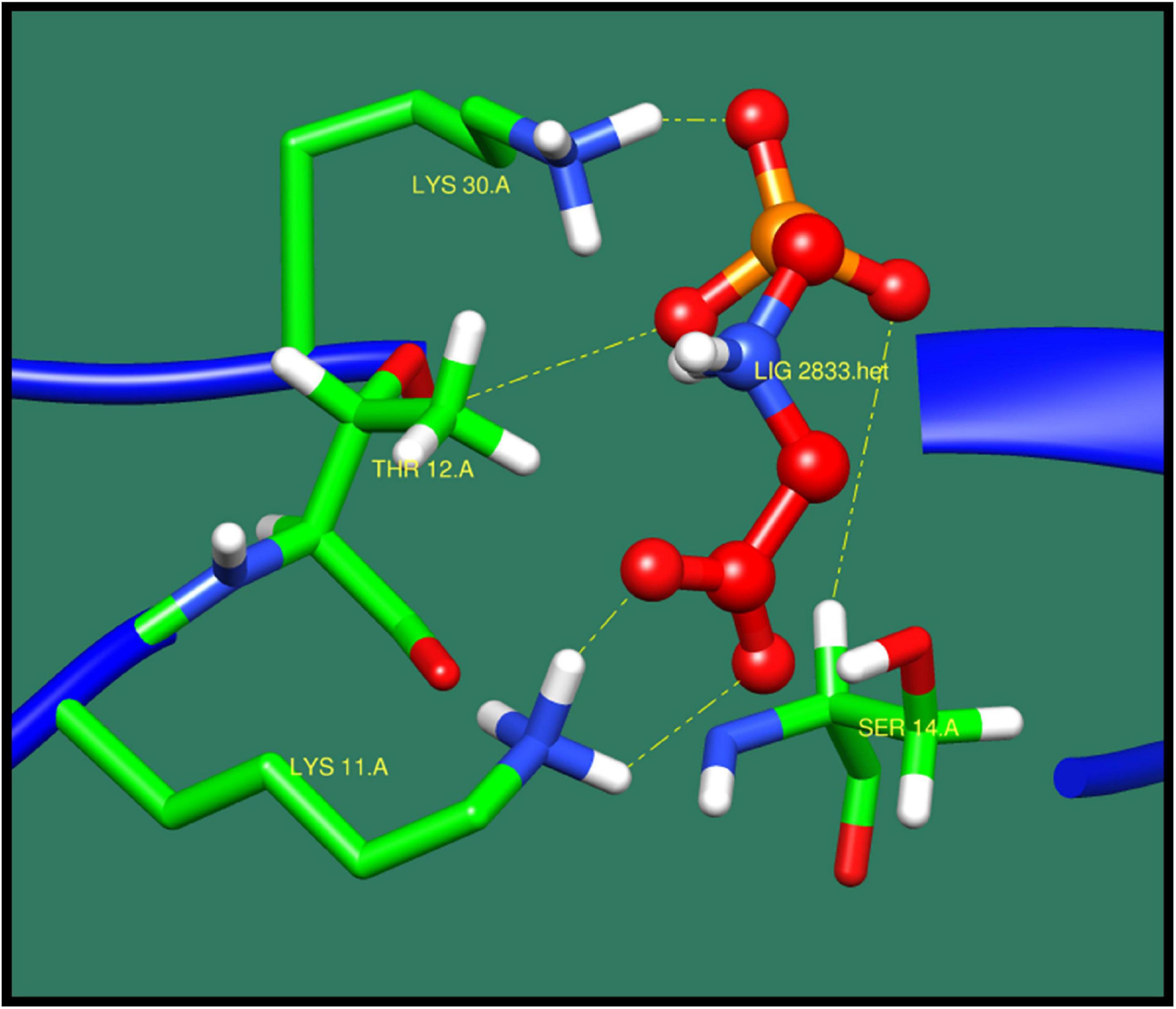
Figure 11. Interaction of 50S ribosomal protein L28 with ZINC03872713 (colored in red). The interacting residues (green) are shown bonding (dotted lines) with the ligand.
2-Isopropylmalate synthase (leuA) protein catalyzes to form 2-isopropylmalate by the condensation of acetyl group of acetyl-CoA with 2-oxoisovalerate. It is also involved in biosynthesis of leucine, by synthesizing L-leucine from 3-methly-2-oxobutanate (De Carvalho and Blanchard, 2006). In Mycobacterium tuberculosis, biosynthesis of leucine plays an essential role, which is important for the growth of bacteria, and so it could act as a potential drug target. The structure of this protein consist two domains N- and C-terminal. N-Terminal consist of triosephosphate isomerase (TIM) barrel catalytic domain, and C-terminal is a regulatory domain (Koon et al., 2004). The top 10 ligands against 2-isopropylmalate synthase (leuA) protein are shown in Table 14 along with ZINC ID, minimized energy, number of interactions, dock score, and interactive residue, and the best interacting protein–ligand confirmation is shown in Figure 12.
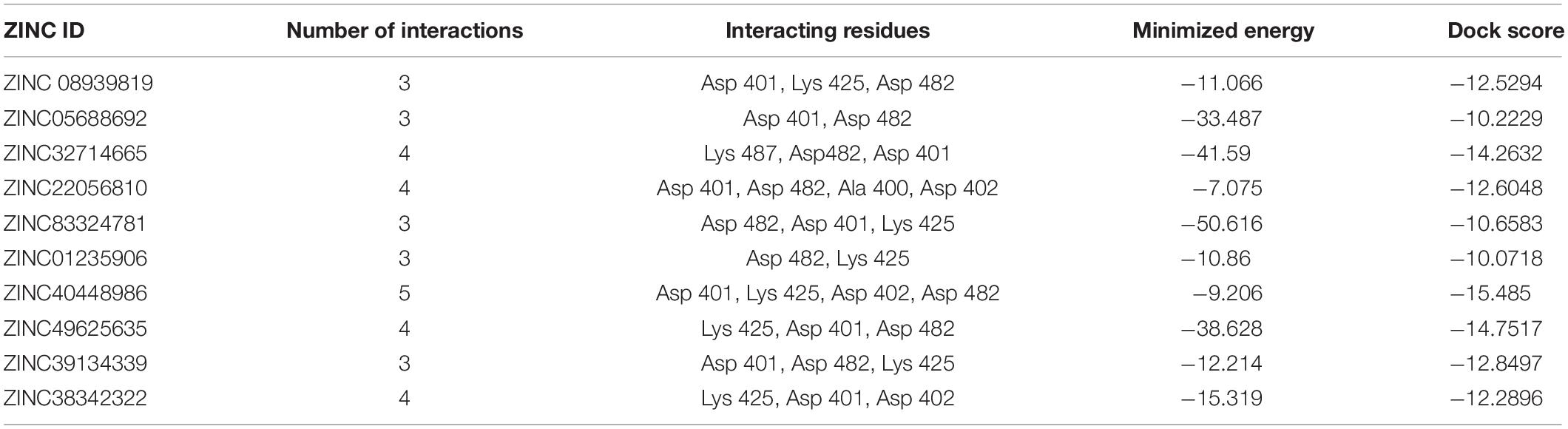
Table 14. 2-Isopropylmalate synthase and its interaction profile with docked compounds their ZINC ID, minimized energy, number of interactions, dock score, and interactive residues.
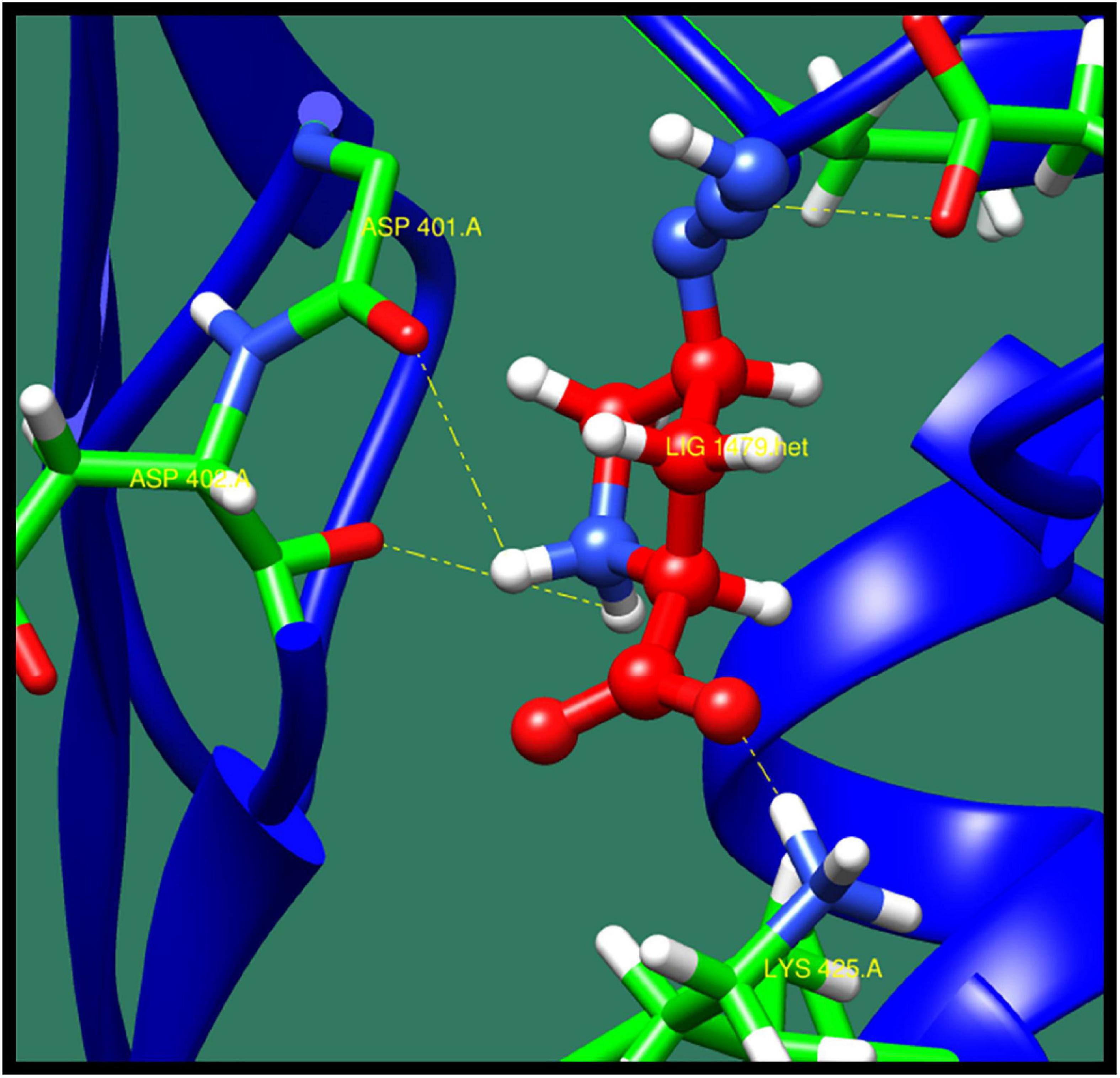
Figure 12. Interaction of 2-isopropylmalate synthase with ZINC40448986 (colored in red). The interacting residues (green) are shown bonding (dotted lines) with the ligand.
Ribosome-binding factor A (rbfA) is cold shock adaptation protein that helps bacteria to grow at low temperature (10–20°C). This protein associates with 30S ribosomal subunit but do not associate with 70S ribosomes or polysomes. It also interacts with 5′-terminal helix of 16S rRNA. During the cold shock adaptation, several cold shock proteins are synthesized, which allow the efficient translation processing of the messenger RNAs (mRNAs), which facilitates the ribosome assembly that is required for the growth of bacteria (Huang et al., 2003). This protein is found to be virulent and quite essential for bacteria so that it could act as potential drug target. The best interacting lead molecules are shown in Table 15 along with ZINC ID, minimized energy, dock score, number of interactions, and interacting residues. ZINC01235906 was predicted as top ranked molecule interacting with binding site residues lys24 and Arg77 (Figure 13).
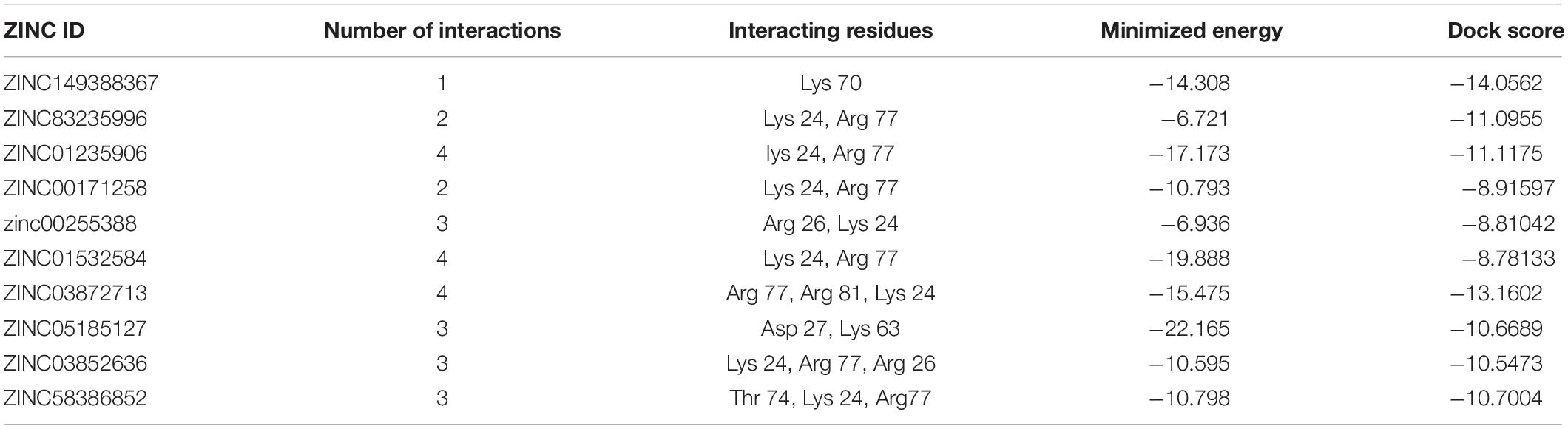
Table 15. Ribosome-binding factor A and its interaction profile with docked compounds, their ZINC ID, minimized energy, number of interactions, dock score, and interactive residues.
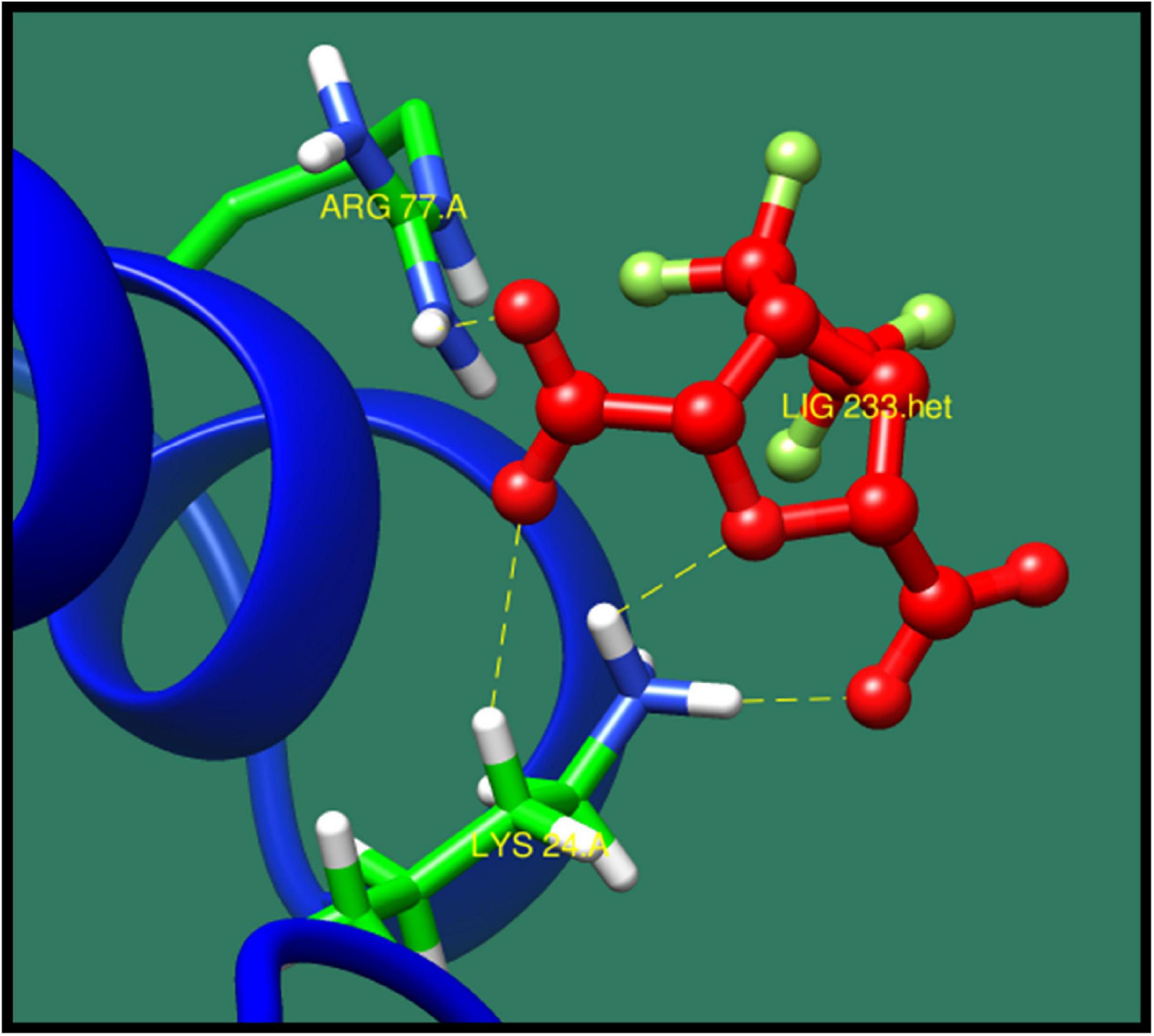
Figure 13. Interaction of ribosome-binding factor A with ZINC01235906 (colored in red). The interacting residues (green) are shown making bonding (dotted lines) with the ligand.
DNA-binding response regulator (DW662_02135) is a protein that mediates the change in cell according to the response in the environment. This protein is a part of a two-component regulatory system (TCS). Bacteria tend to change its environment according to different levels of regulation and expression of genes, expression of multiple operons and stress response and sporulation and cellular motility, cell aggregation, and biofilm formation. All these levels are controlled by TCS from primarily through transcription, translations, and posttranslation of regulation of genes and also through different types of protein–protein interaction and also its virulence. TCS consists of histidine kinases, which sense the environmental signal and generate the response regulator. This process is phosphorylated by the cognate histidine kinase, and it also sometimes function as transcription regulator to regulate the expression of genes (Wang et al., 2007; Galperin, 2010). As this protein is non-homolog to human and also found to be essential and virulent, this protein could be a potential drug target against Sg. Table 16 presents best interacting lead molecules along with their ZINC ID, interacting residues, number of interactions, dock score, and minimized energy. Binding site residues His74, Ser114, Arg117, Lys156, and Lys153 were predicted to interact with ZINC38140720 as shown in Figure 14.
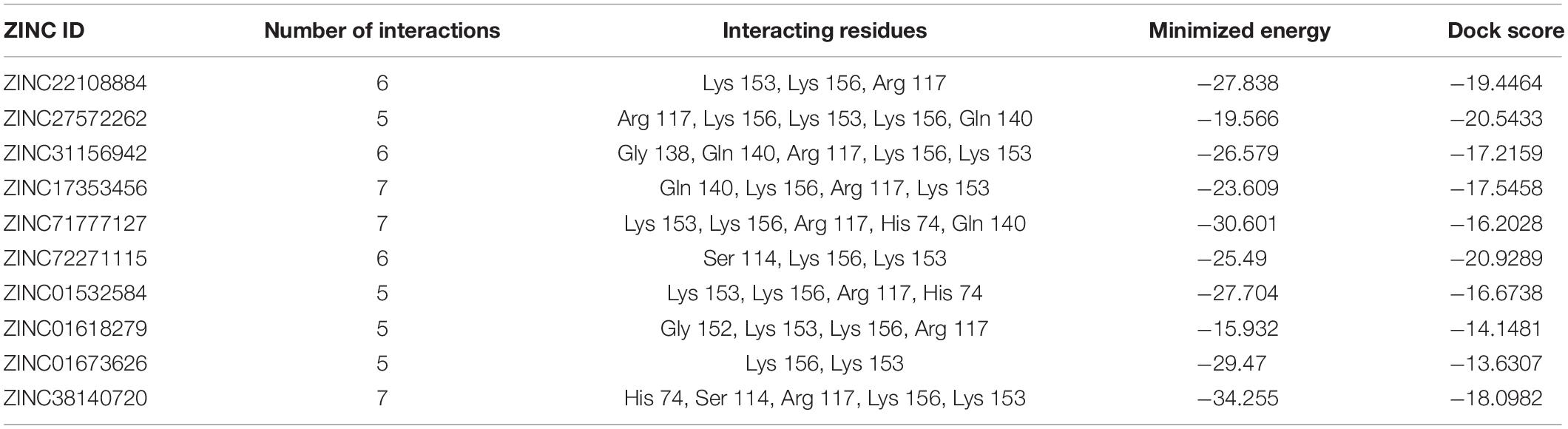
Table 16. DNA-binding response regulator and its interaction profile with docked compounds, their ZINC ID, minimized energy, number of interactions, dock score, and interactive residues.
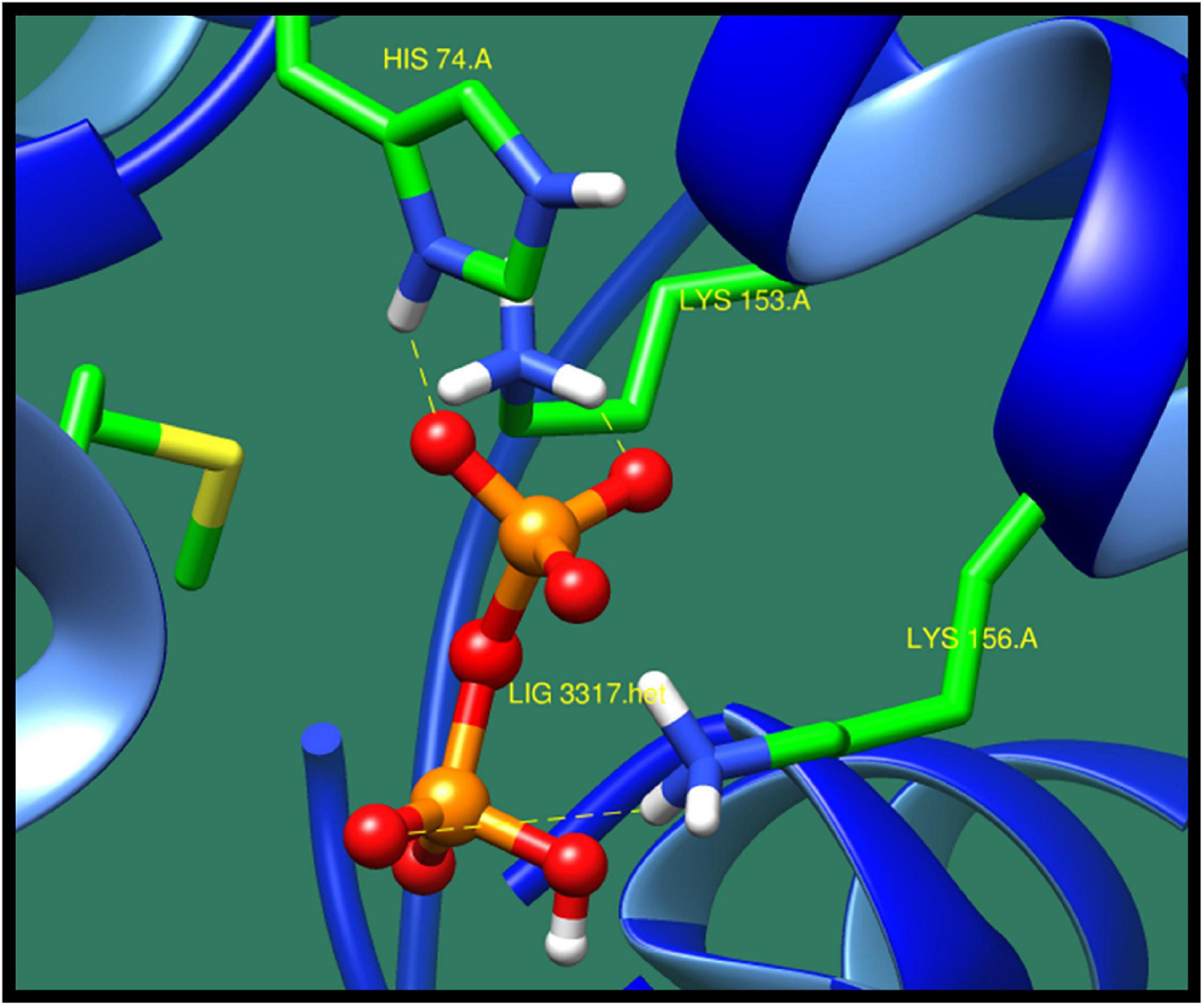
Figure 14. Interaction of DNA-binding response regulator with ZINC38140720 (colored in red). The interacting residues (green) are shown bonding (dotted lines) with the ligand.
For each target protein, we were able to shortlist 10 lead molecules out of which 1 molecule was ranked on top. It would be appropriate to translate these in silico findings into in vitro and finally in vivo to channelize the computational findings toward experimental validation.
Conclusion
In the current study, we have used an in silico approach in which 1,138 core proteins of 7 strains of Sg were determined from pan genome analysis. Subtractive genomic and identification of essential genes further reduced the number of selected targets to 18. The exploitation of 3D structural information and drug prioritization of these proteins enabled to prioritize 12 putative drug targets. All of the identified drug targets are playing an essential role in the bacterial growth, survival, and virulence, which could act as potential therapeutic targets. Furthermore, molecular docking analysis allowed us to shortlist 10 active molecules from which the best active molecule was selected on the basis of drug score, number of interactions, and binding free energy. Thus, this study provides a significant breakthrough in designing new and potent compounds against Sg. For the future work, the experimental validation of these targets is suggested to validate its role in survival and virulence of Sg.
Data Availability Statement
The raw data supporting the conclusions of this article will be made available by the authors, without undue reservation.
Author Contributions
NQ, RU, and SJ conceived the idea and performed the experimental work. NQ, MF, MSh, AB, HM, MSo, and SJ performed all the analysis. NQ and RM drafted the manuscript. SJ, SB, and RU critically reviewed the manuscript and provided intellectual support. All authors contributed to the article and approved the submitted version.
Funding
This study was funded by the Deanship of Scientific Research at King Saud University through research group no. RG-1440-100, King Saud University, Riyadh, Saudi Arabia.
Conflict of Interest
The authors declare that the research was conducted in the absence of any commercial or financial relationships that could be construed as a potential conflict of interest.
Acknowledgments
This work is a part of a mutual collaboration among the three leading research/academic institutions of Pakistan and Saudi Arabia: Department of Bioinformatics and Biosciences, Capital University of Science and Technology (CUST), Department of Biological Sciences, National University of Medical Sciences (NUMS), and King Saud University, Riyadh, Saudi Arabia. We extend their appreciation to the Deanship of Scientific Research at King Saud University for funding this work through research group no. RG-1440-100, King Saud University, Riyadh, Saudi Arabia for financial support. We acknowledge the support of all coauthors who have worked and collaborated in accomplishing this project.
Footnotes
- ^ https://www.ncbi.nlm.nih.gov/genome/
- ^ http://web.expasy.org/protparam/
- ^ https://www.uniprot.org/
- ^ http://cello.life.nctu.edu.tw/
- ^ https://www.genome.jp/kegg/
- ^ http://www.mgc.ac.cn/VFs/
- ^ https://www.ncbi.nlm.nih.gov/
References
Agüero, F., Al-Lazikani, B., Aslett, M., Berriman, M., Buckner, F. S., Campbell, R. K., et al. (2008). Genomic-scale prioritization of drug targets: the TDR Targets database. Nat. Rev. Drug Discov. 7, 900–907. doi: 10.1038/nrd2684
Arjun, R., Niyas, V. K., Sasidharan, A., and Palakunnath, G. A. (2020). Streptococcus gallolyticus bacteremia: experience from a tertiary center in South India. Indian J. Crit. Care Med. 24, 943–945. doi: 10.5005/jp-journals-10071-23569
Arregle, F., Gouriet, F., Amphoux, B., Edouard, S., Chaudet, H., Casalta, J.-P., et al. (2019). Western immunoblotting for the diagnosis of Enterococcus faecalis and Streptococcus gallolyticus infective endocarditis. Front. Cell. Infect. Microbiol. 9:314. doi: 10.3389/fcimb.2019.00314
Aseev, L. V., Koledinskaya, L. S., and Boni, I. V. (2016). Regulation of ribosomal protein operons rplM-rpsI, rpmB-rpmG, and rplU-rpmA at the transcriptional and translational levels. J. Bacteriol. 198, 2494–2502. doi: 10.1128/JB.00187-16
Barh, D., Jain, N., Tiwari, S., Parida, B. P., D’Afonseca, V., Li, L., et al. (2011). A novel comparative genomics analysis for common drug and vaccine targets in Corynebacterium pseudotuberculosis and other CMN group of human pathogens. Chem. Biol. Drug Des. 78, 73–84. doi: 10.1111/j.1747-0285.2011.01118.x
Batut, P., and Gingeras, T. R. (2013). RAMPAGE: promoter activity profiling by paired-end sequencing of 5’-complete cDNAs. Curr. Protoc. Mol. Biol. 104, Unit 25B.11. doi: 10.1002/0471142727.mb25b11s104
Blom, J., Kreis, J., Spänig, S., Juhre, T., Bertelli, C., Ernst, C., et al. (2016). EDGAR 2.0: an enhanced software platform for comparative gene content analyses. Nucleic Acids Res. 44, W22–W28. doi: 10.1093/nar/gkw255
Caputo, A., Fournier, P. E., and Raoult, D. (2019). Genome and pan-genome analysis to classify emerging bacteria. Biol. Direct 14:5. doi: 10.1186/s13062-019-0234-0
Cãruntu, F., Andor, M., Streian, C., Tomescu, M., and Marincu, I. (2014). Streptococcus gallolyticus spontaneous infective endocarditis on native valves, in a diabetic patient. Med. Evol. 3, 323–328.
Chamat-Hedemand, S., Dahl, A., Østergaard, L., Arpi, M., Fosbøl, E., Boel, J., et al. (2020). Prevalence of infective endocarditis in Streptococcal bloodstream infections is dependent on Streptococcal species. Circulation 142, 720–730. doi: 10.1161/CIRCULATIONAHA.120.046723
Chen, L., Yang, J., Yu, J., Yao, Z., Sun, L., Shen, Y., et al. (2005). VFDB: a reference database for bacterial virulence factors. Nucleic Acids Res. 33, D325–D328. doi: 10.1093/nar/gki008
De Carvalho, L. P. S., and Blanchard, J. S. (2006). Kinetic and chemical mechanism of α-isopropylmalate synthase from Mycobacterium tuberculosis. Biochemistry 45, 8988–8999. doi: 10.1021/bi0606602
Erzberger, J. P., Pirruccello, M. M., and Berger, J. M. (2002). The structure of bacterial DnaA: implications for general mechanisms underlying DNA replication initiation. EMBO J. 21, 4763–4773. doi: 10.1093/emboj/cdf496
ExPASy (2020). ProtParam Documentation. Available online at: https://web.expasy.org/protparam/protparam-doc.html (accessed 8 February 2020).
Fernandez-López, R., Ruiz, R., de la Cruz, F., and Moncalián, G. (2015). Transcription factor-based biosensors enlightened by the analyte. Front. Microbiol. 6:648. doi: 10.3389/fmicb.2015.00648
Firstenberg, M. S. (2016). Contemporary Challenges in Endocarditis. Norderstedt: BoD – Books on Demand.
Fishovitz, J., Hermoso, J. A., Chang, M., and Mobashery, S. (2014). Penicillin-binding protein 2a of methicillin-resistant Staphylococcus aureus. IUBMB Life 66, 572–577. doi: 10.1002/iub.1289
Foster, P. G., Nunes, C. R., Greene, P., Moustakas, D., and Stroud, R. M. (2003). The first structure of an RNA m5C Methyltransferase, Fmu, provides insight into catalytic mechanism and specific binding of RNA substrate. Structure 11, 1609–1620. doi: 10.1016/j.str.2003.10.014
Fuhrmann, J., Schmidt, A., Spiess, S., Lehner, A., Turgay, K., Mechtler, K., et al. (2009). McsB is a protein arginine kinase that phosphorylates and inhibits the heat-shock regulator ctsr. Science 324, 1323–1327. doi: 10.1126/science.1170088
Galperin, M. Y. (2010). Diversity of structure and function of response regulator output domains. Curr. Opin. Microbiol. 13, 150–159. doi: 10.1016/j.mib.2010.01.005
Grubitzsch, H., Christ, T., Melzer, C., Kastrup, M., Treskatsch, S., and Konertz, W. (2016). Surgery for prosthetic valve endocarditis: associations between morbidity, mortality and costs. Interact. Cardiovasc. Thorac. Surg. 22, 784–791. doi: 10.1093/icvts/ivw035
Hensler, M. E. (2011). Streptococcus gallolyticus, infective endocarditis, and colon carcinoma: new light on an intriguing coincidence. J. Infect. Dis. 203, 1040–1042. doi: 10.1093/infdis/jiq170
Hinse, D., Vollmer, T., Rückert, C., Blom, J., Kalinowski, J., Knabbe, C., et al. (2011). Complete genome and comparative analysis of Streptococcus gallolyticus subsp. gallolyticus, an emerging pathogen of infective endocarditis. BMC Genomics 12:400. doi: 10.1186/1471-2164-12-400
Holland, T. L., Baddour, L. M., Bayer, A. S., Hoen, B., Miro, J. M., and Fowler, V. G. (2016). Infective endocarditis. Nat. Rev. Dis. Prim. 2:16059. doi: 10.1038/nrdp.2016.59
Hrast, M., Turk, S., Sosič, I., Knez, D., Randall, C. P., Barreteau, H., et al. (2013). Structure-activity relationships of new cyanothiophene inhibitors of the essential peptidoglycan biosynthesis enzyme MurF. Eur. J. Med. Chem. 66, 32–45. doi: 10.1016/j.ejmech.2013.05.013
Huang, Y. J., Swapna, G. V. T., Rajan, P. K., Ke, H., Xia, B., Shukla, K., et al. (2003). Solution NMR structure of ribosome-binding factor A (RbfA), a cold-shock adaptation protein from Escherichia coli. J. Mol. Biol. 327, 521–536. doi: 10.1016/S0022-2836(03)00061-5
Hughes, J. P., Rees, S. S., Kalindjian, S. B., and Philpott, K. L. (2011). Principles of early drug discovery. Br. J. Pharmacol. 162, 1239–1249. doi: 10.1111/j.1476-5381.2010.01127.x
Jaiswal, A. K., Tiwari, S., Jamal, S. B., Barh, D., Azevedo, V., and Soares, S. C. (2017). An in silico identification of common putative vaccine candidates against treponema pallidum: a reverse vaccinology and subtractive genomics based approach. Int. J. Mol. Sci. 18:402. doi: 10.3390/ijms18020402
Jamal, S. B., Hassan, S. S., Tiwari, S., Viana, M. V., Benevides, L. J., Ullah, A., et al. (2017). An integrative in-silico approach for therapeutic target identification in the human pathogen Corynebacterium diphtheriae. PLoS One 12:e0186401. doi: 10.1371/journal.pone.0186401
Kanehisa, M., Furumichi, M., Tanabe, M., Sato, Y., and Morishima, K. (2017). KEGG: new perspectives on genomes, pathways, diseases and drugs. Nucleic Acids Res. 45, D353–D361. doi: 10.1093/nar/gkw1092
Kanehisa, M., and Sato, Y. (2020). KEGG Mapper for inferring cellular functions from protein sequences. Protein Sci. 29, 28–35. doi: 10.1002/pro.3711
Kocaoglu, O., and Carlson, E. E. (2015). Profiling of β-lactam selectivity for penicillin-binding proteins in Escherichia coli strain DC2. Antimicrob. Agents Chemother. 59, 2785–2790. doi: 10.1128/AAC.04552-14
Koon, N., Squire, C. J., and Baker, E. N. (2004). Crystal structure of LeuA from Mycobacterium tuberculosis, a key enzyme in leucine biosynthesis. Proc. Natl. Acad. Sci. U.S.A. 101, 8295–8300. doi: 10.1073/pnas.0400820101
Luo, H., Lin, Y., Gao, F., Zhang, C. T., and Zhang, R. (2014). DEG 10, an update of the database of essential genes that includes both protein-coding genes and noncoding genomic elements. Nucleic Acids Res. 42, D574–D580. doi: 10.1093/nar/gkt1131
Malaga, F., Mayberry, O., Park, D. J., Rodgers, M. E., Toptygin, D., and Schleif, R. F. (2016). A genetic and physical study of the interdomain linker of E. Coli AraC protein-a trans-subunit communication pathway. Proteins Struct. Funct. Bioinform. 84, 448–460. doi: 10.1002/prot.24990
Marmolin, E. S., Hartmeyer, G. N., Christensen, J. J., Nielsen, X. C., Dargis, R., Skov, M. N., et al. (2016). Bacteremia with the bovis group streptococci: species identification and association with infective endocarditis and with gastrointestinal disease. Diagn. Microbiol. Infect. Dis. 85, 239–242. doi: 10.1016/j.diagmicrobio.2016.02.019
McDonald, J. R. (2009). Acute infective endocarditis. Infect. Dis. Clin. North Am. 23, 643–664. doi: 10.1016/j.idc.2009.04.013
Millar, B. C., and Moore, J. E. (2004). Emerging issues in infective endocarditis. Emerg. Infect. Dis. J. 10, 1110–1116. doi: 10.3201/EID1006.030848
Mondal, S. I., Ferdous, S., Jewel, N. A., Akter, A., Mahmud, Z., Islam, M. M., et al. (2015). Identification of potential drug targets by subtractive genome analysis of Escherichia coli O157:H7: an in silico approach. Adv. Appl. Bioinform. Chem. 8, 49–63. doi: 10.2147/AABC.S88522
Nielsen, M., Lundegaard, C., Lund, O., and Petersen, T. N. (2010). CPHmodels-3.0—remote homology modeling using structure-guided sequence profiles. Nucleic Acids Res. 38, W576–W581. doi: 10.1093/nar/gkq535
Pagadala, N. S., Syed, K., and Tuszynski, J. (2017). Software for molecular docking: a review. Biophys. Rev. 9, 91–102. doi: 10.1007/s12551-016-0247-1
Pasquereau-Kotula, E., Martins, M., Aymeric, L., and Dramsi, S. (2018). Significance of Streptococcus gallolyticus subsp. gallolyticus association with colorectal cancer. Front. Microbiol. 9:614. doi: 10.3389/fmicb.2018.00614
Rodgers, M. E., and Schleif, R. (2009). Solution structure of the DNA binding domain of AraC protein. Proteins Struct. Funct. Bioinform. 77, 202–208. doi: 10.1002/prot.22431
Rusniok, C., Couvé, E., Da Cunha, V., Gana, R., El, Zidane, N., et al. (2010). Genome sequence of Streptococcus gallolyticus: insights into its adaptation to the bovine rumen and its ability to cause endocarditis †. J. Bacteriol. 192, 2266–2276. doi: 10.1128/JB.01659-09
Saddala, M. S., and Adi, P. J. (2018). Discovery of small molecules through pharmacophore modeling, docking and molecular dynamics simulation against Plasmodium vivax Vivapain-3 (VP-3). Heliyon 4:e00612. doi: 10.1016/j.heliyon.2018.e00612
Satué-Bartolomé, J. A., and Alonso-Sanz, M. (2009). Streptococcus gallolyticus: a new name for an old organism. Arch. Med. 5, 2–4. doi: 10.3823/020
Scott, M. S., Calafell, S. J., Thomas, D. Y., and Hallett, M. T. (2005). Refining protein subcellular localization. PLoS Comput. Biol. 1:e66. doi: 10.1371/journal.pcbi.0010066
Shahid, U., Sharif, H., Farooqi, J., Jamil, B., and Khan, E. (2018). Microbiological and clinical profile of infective endocarditis patients: an observational study experience from tertiary care center Karachi Pakistan. J. Cardiothorac. Surg. 13, 1–9. doi: 10.1186/s13019-018-0781-y
Shen, X., Liu, L., Yu, J., Ai, W., Cao, X., Zhan, Q., et al. (2020). High prevalence of 16s rRNA methyltransferase genes in carbapenem-resistant klebsiella pneumoniae clinical isolates associated with bloodstream infections in 11 Chinese teaching hospitals. Infect. Drug Resist. 13, 2189–2197. doi: 10.2147/IDR.S254479
Siebold, C., Flükiger, K., Beutler, R., and Erni, B. (2001). Carbohydrate transporters of the bacterial phosphoenolpyruvate: sugar phosphotransferase system (PTS). FEBS Lett. 504, 104–111. doi: 10.1016/S0014-5793(01)02705-3
Sterling, T., and Irwin, J. J. (2015). ZINC 15 - ligand discovery for everyone. J. Chem. Inf. Model. 55, 2324–2337. doi: 10.1021/acs.jcim.5b00559
Takamura, N., Kenzaka, T., Minami, K., and Matsumura, M. (2014). Infective endocarditis caused by Streptococcus gallolyticus subspecies pasteurianus and colon cancer. BMJ Case Rep. 2014:bcr2013203476. doi: 10.1136/bcr-2013-203476
Tiwari, S., Da Costa, M. P., Almeida, S., Hassan, S. S., Jamal, S. B., Oliveira, A., et al. (2014). C. pseudotuberculosis Phop confers virulence and may be targeted by natural compounds. Integr. Biol. 6, 1088–1099. doi: 10.1039/c4ib00140k
Tripodi, M. F., Fortunato, R., Utili, R., Triassi, M., and Zarrilli, R. (2005). Molecular epidemiology of Streptococcus bovis causing endocarditis and bacteraemia in Italian patients. Clin. Microbiol. Infect. 11, 814–819. doi: 10.1111/j.1469-0691.2005.01248.x
Uddin, R., and Jamil, F. (2018). Prioritization of potential drug targets against P. aeruginosa by core proteomic analysis using computational subtractive genomics and Protein-Protein interaction network. Comput. Biol. Chem. 74, 115–122. doi: 10.1016/j.compbiolchem.2018.02.017
Uddin, R., Masood, F., Azam, S. S., and Wadood, A. (2019). Identification of putative non-host essential genes and novel drug targets against Acinetobacter baumannii by in silico comparative genome analysis. Microb. Pathog. 128, 28–35. doi: 10.1016/j.micpath.2018.12.015
Vilar, S., Cozza, G., and Moro, S. (2008). Medicinal chemistry and the molecular operating environment (MOE): application of QSAR and molecular docking to drug discovery. Curr. Top. Med. Chem. 8, 1555–1572. doi: 10.2174/156802608786786624
Vilcant, V., and Hai, O. (2018). Endocarditis, Bacterial. Available online at: http://www.ncbi.nlm.nih.gov/pubmed/29262218 (accessed 31 October 2019)
Volkamer, A., Kuhn, D., Rippmann, F., and Rarey, M. (2012). DoGSiteScorer: a web server for automatic binding site prediction, analysis and druggability assessment. Bioinformatics 28, 2074–2075. doi: 10.1093/bioinformatics/bts310
Wadood, A., Jamal, S. B., Riaz, M., and Mir, A. (2014). Computational analysis of benzofuran-2-carboxlic acids as potent Pim-1 kinase inhibitors. Pharm. Biol. 52, 1170–1178. doi: 10.3109/13880209.2014.880488
Wang, S., Engohang-Ndong, J., and Smith, I. (2007). Structure of the DNA-binding domain of the response regulator PhoP from Mycobacterium tuberculosis. Biochemistry 46, 14751–14761. doi: 10.1021/bi700970a
Wing, R. A., Bailey, S., and Steitz, T. A. (2008). Insights into the replisome from the structure of a ternary complex of the DNA polymerase III α-Subunit. J. Mol. Biol. 382, 859–869. doi: 10.1016/j.jmb.2008.07.058
Keywords: Streptococcus gallollyticus, infective endocarditis, pan-genome, subtractive proteomics, drug prioritization
Citation: Qureshi NA, Bakhtiar SM, Faheem M, Shah M, Bari A, Mahmood HM, Sohaib M, Mothana RA, Ullah R and Jamal SB (2021) Genome-Based Drug Target Identification in Human Pathogen Streptococcus gallolyticus. Front. Genet. 12:564056. doi: 10.3389/fgene.2021.564056
Received: 20 May 2020; Accepted: 16 February 2021;
Published: 25 March 2021.
Edited by:
Debmalya Barh, Institute of Integrative Omics and Applied Biotechnology (IIOAB), IndiaReviewed by:
Ashutosh Mani, Motilal Nehru National Institute of Technology Allahabad, IndiaMuhammad Tariq, University of Tabuk, Saudi Arabia
Nurnabi Azad Jewel, Shahjalal University of Science and Technology, Bangladesh
Muhammad Ilyas, Islamia College University, Pakistan
Copyright © 2021 Qureshi, Bakhtiar, Faheem, Shah, Bari, Mahmood, Sohaib, Mothana, Ullah and Jamal. This is an open-access article distributed under the terms of the Creative Commons Attribution License (CC BY). The use, distribution or reproduction in other forums is permitted, provided the original author(s) and the copyright owner(s) are credited and that the original publication in this journal is cited, in accordance with accepted academic practice. No use, distribution or reproduction is permitted which does not comply with these terms.
*Correspondence: Riaz Ullah, cnVsbGFoQGtzdS5lZHUuc2E=; Syed Babar Jamal, YmFiYXIuamFtYWxAbnVtc3Bhay5lZHUucGs=; c3llZGJhYmFyLmphbWFsQGdtYWlsLmNvbQ==