- Liver Disease Center, The Affiliated Hospital of Qingdao University, Qingdao, China
Recently, growing evidence has revealed the significant effect of reprogrammed metabolism on pancreatic cancer in relation to carcinogenesis, progression, and treatment. However, the prognostic value of metabolism-related genes in pancreatic cancer has not been fully revealed. We identified 379 differentially expressed metabolic-related genes (DEMRGs) by comparing 178 pancreatic cancer tissues with 171 normal pancreatic tissues in The Cancer Genome Atlas (TCGA) and the Genotype-Tissue Expression project (GTEx) databases. Then, we used univariate Cox regression analysis together with Lasso regression for constructing a prognostic model consisting of 15 metabolic genes. The unified risk score formula and cutoff value were taken into account to divide patients into two groups: high risk and low risk, with the former exhibiting a worse prognosis compared with the latter. The external validation results of the International Cancer Genome Consortium (IGCC) cohort and the Gene Expression Omnibus (GEO) cohort further confirm the effectiveness of this prognostic model. As shown in the receiver operating characteristic (ROC) curve, the area under curve (AUC) values of the risk score for overall survival (OS), disease-specific survival (DSS), and progression-free survival (PFS) were 0.871, 0.885, and 0.886, respectively. Based on the Gene Set Enrichment Analysis (GSEA), the 15-gene signature can affect some important biological processes and pathways of pancreatic cancer. In addition, the prognostic model was significantly correlated with the tumor immune microenvironment (immune cell infiltration, and immune checkpoint expression, etc.) and clinicopathological features (pathological stage, lymph node, and metastasis, etc.). We also built a nomogram based on three independent prognostic predictors (including individual neoplasm status, lymph node metastasis, and risk score) for the prediction of 1-, 3-, and 5-year OS of pancreatic cancer, which may help to further improve the treatment strategy of pancreatic cancer.
Introduction
Despite the great progress made in treating pancreatic cancer over the last few decades, the prognosis has not been effectively improved (Neoptolemos et al., 2018). Genetic concepts and tools are increasingly being applied to clinical practice, especially in precision medicine (Lomberk et al., 2019). However, the biomarkers related to the prognosis of pancreatic cancer are still limited.
Recently, more and more evidence has revealed the significant effect of the reprogrammed metabolism on pancreatic cancer in terms of carcinogenesis, progression, treatment, and prognosis (Qin et al., 2020). The so-called metabolic reprogramming refers to the significant changes in metabolic patterns during cell carcinogenesis, which involves glycolysis, tricarboxylic acid cycle, oxidative phosphorylation, as well as metabolism of amino acid, fatty acid, and nucleic acid (Ward and Thompson, 2012; Huo et al., 2021a,b). During proliferation, tumor cells rely on metabolic reprogramming to provide nutrition, energy, and biosynthetic activity (Pavlova and Thompson, 2016; Neoptolemos et al., 2018). Pancreatic cancer is a malignant tumor with metabolic heterogeneity. Changes in glucose, lipid metabolism as well as amino acid in pancreatic tumors, from cells to microenvironment, and even at the systemic level, can significantly impact tumor progression (Daemen et al., 2015; Qin et al., 2020). Even for the same patients with pancreatic cancer, the metabolic gene expression of the primary focus and the metastatic focus were relatively different (Chaika et al., 2012; Qin et al., 2020). Although the metabolic targeted therapy for pancreatic cancer is not mature at present (Biancur and Kimmelman, 2018), successive clinical trials have shown that metabolic treatment of pancreatic cancer may improve the prognosis of patients (Zachar et al., 2011; Raez et al., 2013; Alistar et al., 2017). Hence, more metabolic biomarkers related to pancreatic cancer prognosis need to be identified. Considering that the effective clinical treatment of pancreatic cancer is still limited, it is urgent to explore new treatment strategies.
The microenvironment around pancreatic cancer cells is composed of immune cells, stellate cells/fibroblasts, and extracellular matrix (ECM). The rapid proliferation of tumor cells leads to a lack of nutrients in the microenvironment, increased release of lactic acid and other metabolites, and metabolic remodeling such as hypoxia and oxidative stress imbalance. Pancreatic cancer cells rely on metabolic reprogramming to adapt to the lack of energy and nutrition in the tumor microenvironment, abnormal oxidative stress, and so on (Bapat et al., 2011). Therefore, it is necessary to deeply understand the impact of metabolic reprogramming on the occurrence and development of pancreatic cancer, so as to provide new ideas for the targeted intervention of metabolic characteristics for the treatment of pancreatic cancer.
In this study, we identified metabolic genes with different expressions between pancreatic cancer and normal tissues through the TCGA and GTEx databases and explored their prognostic value. The prognostic model, composed of 15 metabolic genes, can accurately predict the survival rate of pancreatic cancer and is an independent predictor related to prognosis. In addition, we integrate the GEO database and ICGC database to verify the model and build a survival predictive nomogram.
Materials and Methods
Data Collection
We obtained the mRNA sequencing data from The Cancer Genome Atlas (TCGA)1 as well as the Genotype-Tissue Expression project (GTEx) (including 178 cancer samples and 171 normal samples). Corresponding clinical data (including the age, gender, survival time, survival status, histological grade, AJCC–TNM stage, presence of new tumors after initial treatment, number of lymph node metastasis, and individual tumor status) were downloaded from UCSC Xena2. The mRNA sequencing data together with the corresponding clinical data were downloaded from the International Cancer Genome Consortium (ICGC) (including PACA-AU and PACA-CA, n = 273)3 and the Gene Expression Omnibus (GEO) (including GSE62452 and GSE57495, n = 128)4. The work flow chart is shown in Figure 1. R package “sva” was employed to remove batch effects between different datasets; the “sva” package supports surrogate variable estimation with the “sva” function, direct adjustment for known batch effects with the “ComBat” function, and adjustment for batch and latent variables in prediction problems with the “fsva” function (Leek et al., 2012). The study excluded patients whose survival time was less than 1 month and included a total of 572 patients with pancreatic cancer. The acquisition of the above data follows the regulations and permissions of the corresponding database, and does not need to be approved by the local ethics committee.
Identification of Differentially Expressed Metabolic-Related Genes
We extracted 2,752 metabolism-related genes from mRNA sequencing data of TCGA and GTEx database, which encoded all known human metabolic enzymes and transporters (Possemato et al., 2011). Differential expression of metabolic genes was identified by R package “limma”; false discovery rate (FDR) < 0.05 and log fold change (FC) absolute value >1 were set as the criteria. We also used R package “clusterProfiler” to annotate the gene ontology (GO) and the Kyoto Encyclopedia of Genes and Genomes (KEGG) functions of DEMRGs. The items were recognized with a p-value threshold less than 0.05.
Identification of Prognostic-Associated Metabolic Genes
We used univariate Cox regression analysis to identify DEMRGs related to prognosis. p < 0.001 was considered to have a significant effect on prognosis.
Construction of Prognostic Model in the Cancer Genome Atlas Cohort
One hundred seventy-one samples with completed prognostic information in the TCGA cohort were used for prognostic model construction. We used Lasso regression to narrow the range of prognostic genes, remove overfitting between genes, and calculated risk scores according to Lasso regression coefficients. The risk score is equal to the sum of Lasso regression coefficient of each mRNA multiplied by the normalized expression levels of each mRNA. The median risk score was taken into account to divide patients into two groups: high risk and low risk. Lasso regression analysis was carried out by using R-package “glmnet”; Kaplan–Meier (KM) survival curve was drawn with the R-package “survminer.” Log-rank test evaluated if the survival curve was different, a p-value of less than 0.05 was considered to be statistically significant, using R-package “survivalROC” to access the accuracy of risk score. A higher AUC (area under the ROC curve) value generally represents a higher prediction accuracy.
Assess Whether the Risk Score Could Predict Prognosis Independently
We used univariate and multivariate Cox regression analysis for determining if the risk score was an independent predictor of the prognosis of pancreatic cancer. p < 0.05 was considered with statistical significance.
Analysis of the Association Between the Risk Score and the Clinical Characteristics
We used Wilcoxon signed-rank test (two groups) or Kruskal–Wallis (≥ two groups) for analyzing how risk score affected the clinicopathology. p < 0.05 was considered with statistical significance. Boxplot was generated using the “beeswarm” package in the R software.
External Validation of the Prognostic Model in International Cancer Genome Consortium and Gene Expression Omnibus Cohort
For testing the universality exhibited by the risk score, we integrated 401 pancreatic cancer patients from the ICGC database and GEO database as an external testing cohort. The risk score exhibited by each patient was calculated following the formula and was classified according to the uniform risk group cutoff value. The R package “survminer” was used to generate the Kaplan–Meier survival curve between the two groups, and log-rank assisted in confirming if the survival curve was significantly different (Huo et al., 2020).
Gene Set Enrichment Analysis Between Different Risk Groups
We conducted GSEA in the populations of the two groups, exploring the potential mechanism of prognostic models affecting prognosis, selecting an annotated gene set file (c2.cp.v7.1.symbols.gmt) as the reference gene set. We set the threshold at nom p-value < 0.05.
Analysis of the Association Between the Risk Score and the Tumor Immune Cell Infiltration
We used TIMER [TumorImmune Estimation Resource, which provided the levels of six tumor-infiltrating immune cells in 10,897 cancer samples (32 types of cancer) from the TCGA database] and CIBERSORT algorithms (using microarray data and a predefined immune signal matrix, estimated the proportion of 22 tumor-infiltrating immune cells in a given sample) to quantify the proportion of immune cell infiltration in tumor tissue (Li et al., 2017; Chen et al., 2018).
Building a Survival Predictive Nomogram
We incorporated independent prognostic factors into a nomogram to construct a combined model for predicting the OS of pancreatic cancer. The advantage of a nomogram is that each patient can get his or her own specific total score and find the corresponding survival rate on the nomogram, which makes the prognosis assessment more personalized, and we also used calibration curve, concordance index, and ROC curve for verifying the precision exhibited by the combined model. The abscissa of the calibration chart is the predicted survival rate, and the ordinate is the actual survival rate. The closer the predicted survival rate is to the actual survival rate, the higher the overlap between the calibration curve and the reference line. The nomogram was built with R package “rms”.
Results
Function Annotation of Differentially Expressed Metabolic-Related Genes
Among the 379 differential genes, there were 169 and 210 upregulated genes in normal tissues and tumor tissues, respectively (Figures 2A,B). They are mainly involved in a variety of metabolic processes, such as small molecular catabolism, coenzyme metabolism, carbon metabolism, oxidative phosphorylation, and so on (Figures 2C,D).
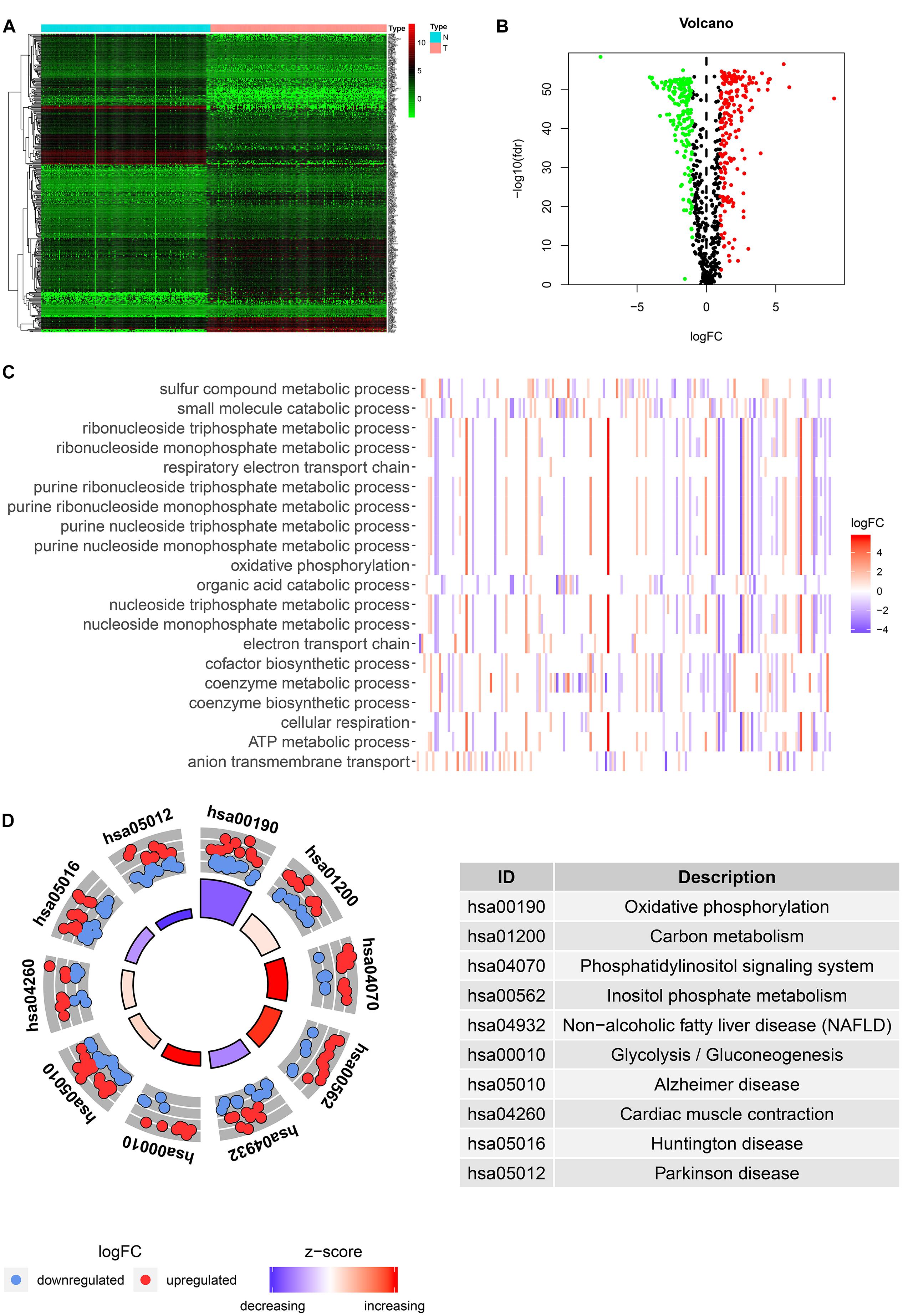
Figure 2. Identification and functional enrichment analysis of differentially expressed metabolic-related genes (DEMRGs). (A) Heat map, volcano map, and boxplot of DMRGs. (B) Gene Ontology (GO) enrichment analysis of DMRGs. (C,D) Kyoto Encyclopedia of Genes and Genomes (KEGG) enrichment analysis of DMRGs.
Identification of Prognostic Differentially Expressed Metabolic-Related Genes
Through univariate Cox regression analysis, we screened 18 genes most significantly related to prognosis (p < 0.001) from the 379 DEMRGs, of which four genes were protective factors of prognosis and 14 genes were risk factors (Figure 3A).
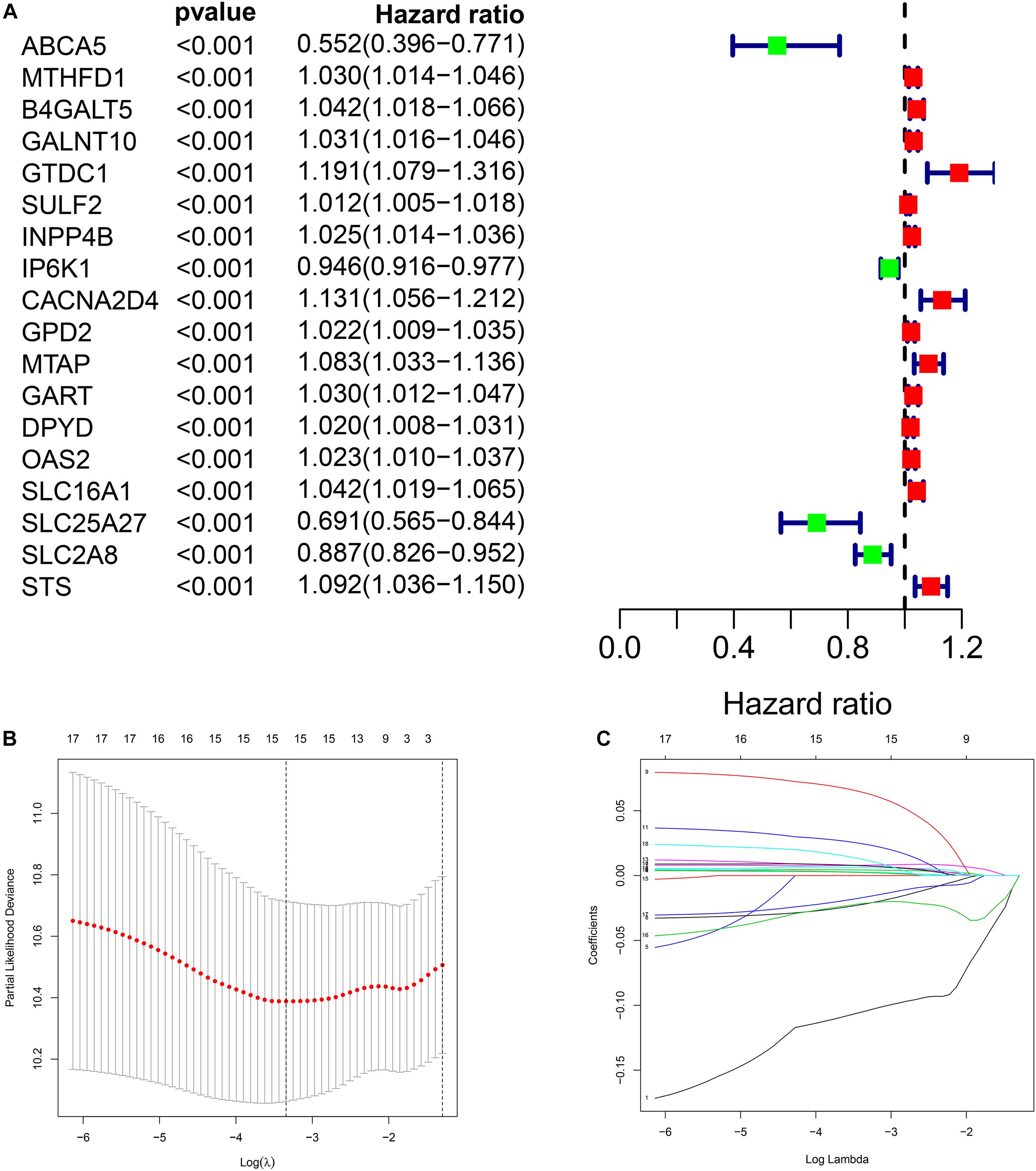
Figure 3. Construction of metabolic prognostic model in The Cancer Genome Atlas (TCGA) cohort. (A) Forest plot of prognostic DMRGs. (B,C) Lasso regression analysis.
Prognostic Model Construction in the Cancer Genome Atlas Cohort
We performed Lasso regression analysis on the above prognostic genes, and after 1,000 cross-validations, the error of a prognostic model containing 15 genes is the smallest (Figures 3B,C). The risk score is equal to the sum of Lasso regression coefficient of each mRNA multiplied by the normalized expression levels of each mRNA. Table 1 lists the calculation coefficient of the risk score. The median risk score (0.655) was taken into account to divide patients into two groups. The group with a high risk exhibited a significantly lower overall survival rate (OS), disease-specific survival rate (DSS), and progression-free survival rate (PFS) compared with the group with a low risk (Figures 4A,C,E). The AUC for 1-year OS was 0.766, for 3-year OS, 0.768, and for 5-year OS, 0.871 (Figure 4B); The AUC for 1-year DSS was 0.805, for 3-year DSS, 0.775, and for 5-year DSS, 0.885 (Figure 4D); The AUC for 1-year PFS was 0.651, for 3-year PFS, 0.808, and for 5-year PFS, 0.886 (Figure 4F). The risk score distribution is shown in Supplementary Material 1. Accordingly, the risk score can be reliably applied for predicting pancreatic cancer patients’ prognosis.
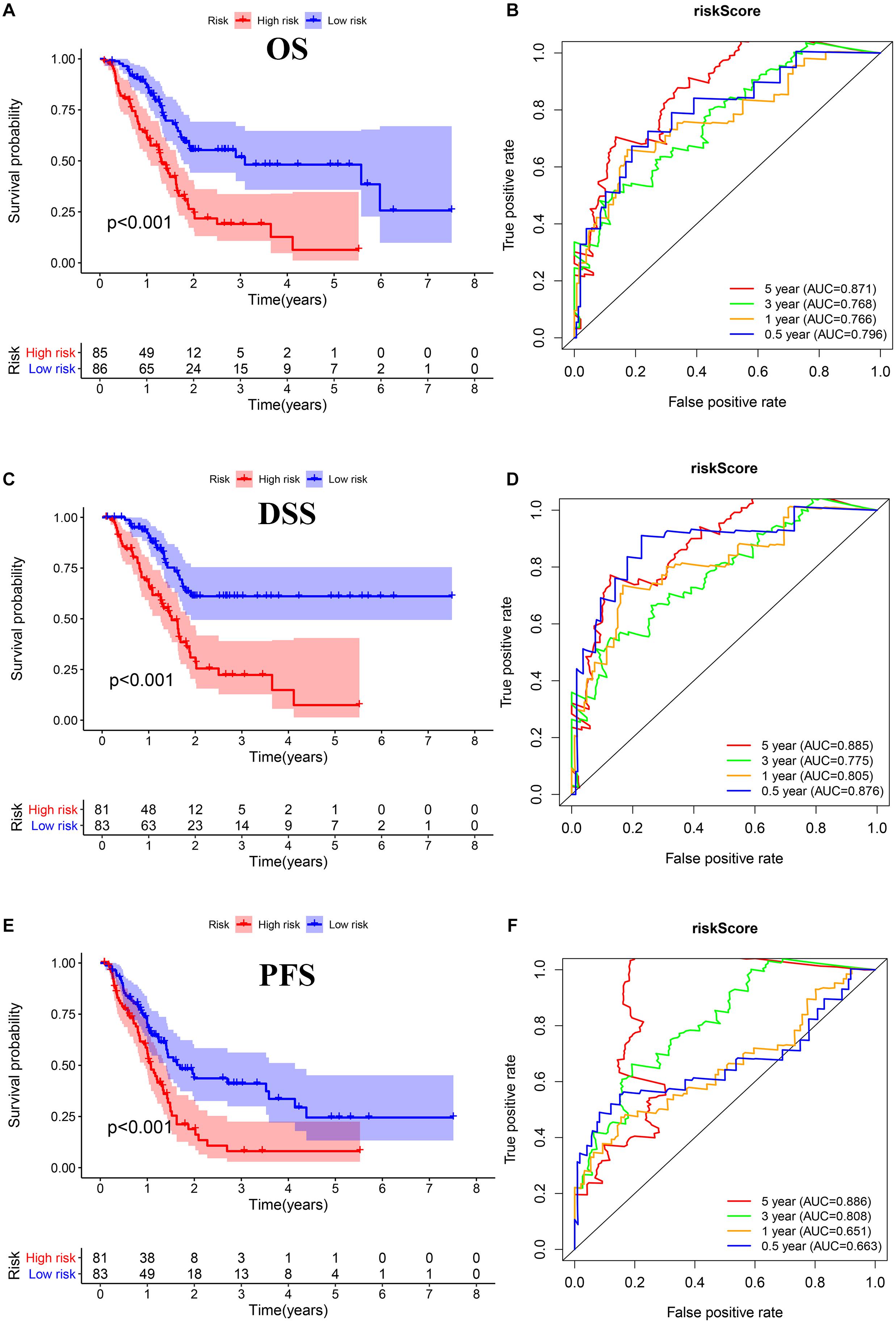
Figure 4. Survival assessment of the prognostic model in TCGA cohort. (A,B) Kaplan–Meier survival analysis and time-dependent receiver operating characteristic (ROC) analysis of overall survival (OS). (C,D) Kaplan–Meier survival analysis and time-dependent ROC analysis of disease special survival (DSS). (E,F) Kaplan–Meier survival analysis and time-dependent ROC analysis of progression-free survival (PFS).
Independence Validation of the Prognostic Model
Through univariate and multivariate Cox regression analyses, we found three independent prognostic factors, including risk score, lymph nodes metastasis, and individual neoplasm status (Figures 5A,B).
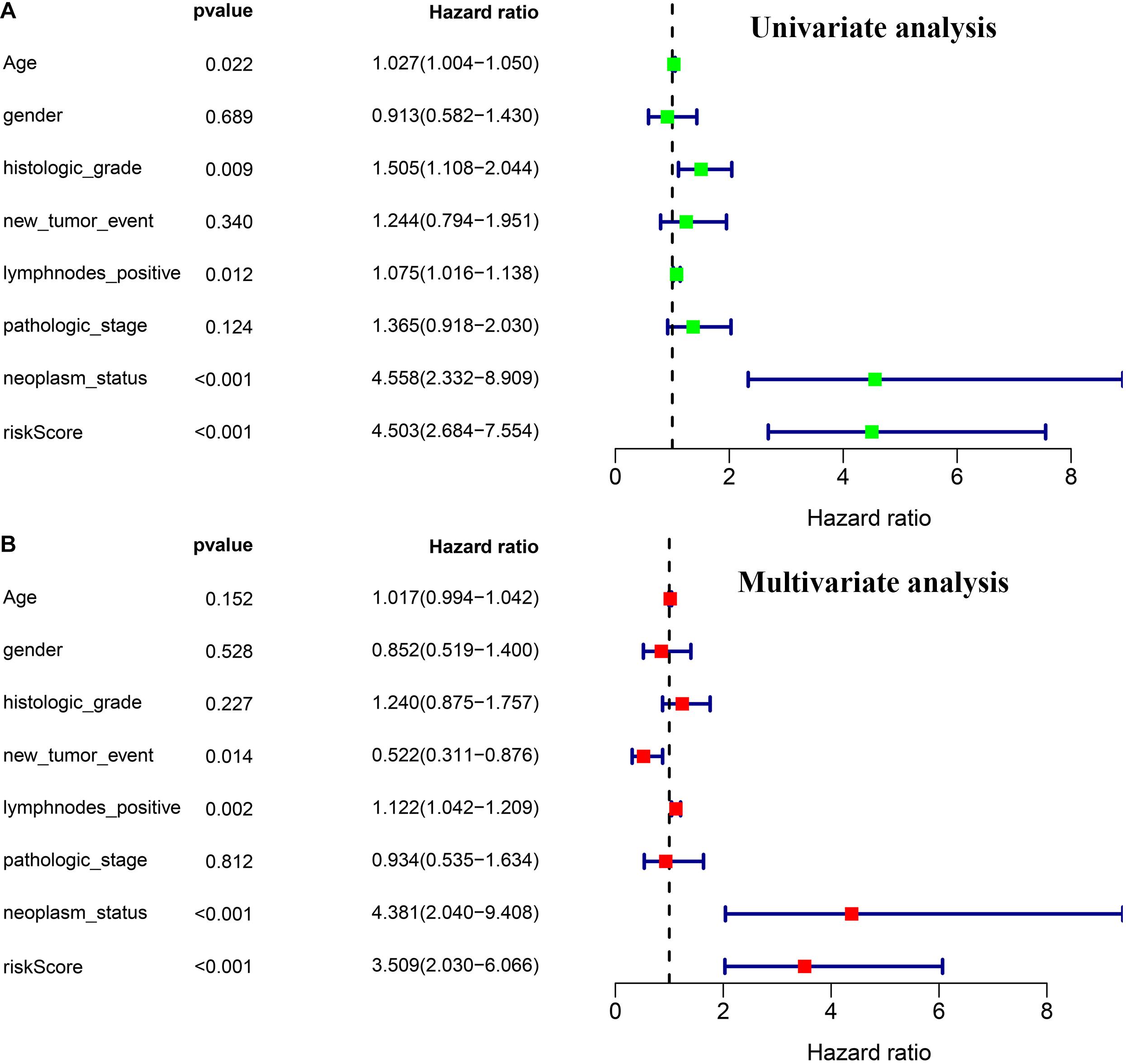
Figure 5. Independence validation of the prognostic model and other clinical features. (A) Univariate Cox regression analysis. (B) Multivariate Cox regression analysis.
Analysis of the Association Between the Risk Score and the Clinical Characteristics
The risk score exhibited an obvious association with histological grade, lymph node metastasis, new tumor after initial treatment, pathologic stage, and neoplasm status (Figures 6A–E). We also performed chi-square test on the TCGA cohort for analysis of clinical features with different risk groups, the results showed that there were significant differences in lymph node metastasis (p = 0.002), personal tumor status (p = 0.002), and survival status (p < 0.001) among the different risk groups (Table 2).
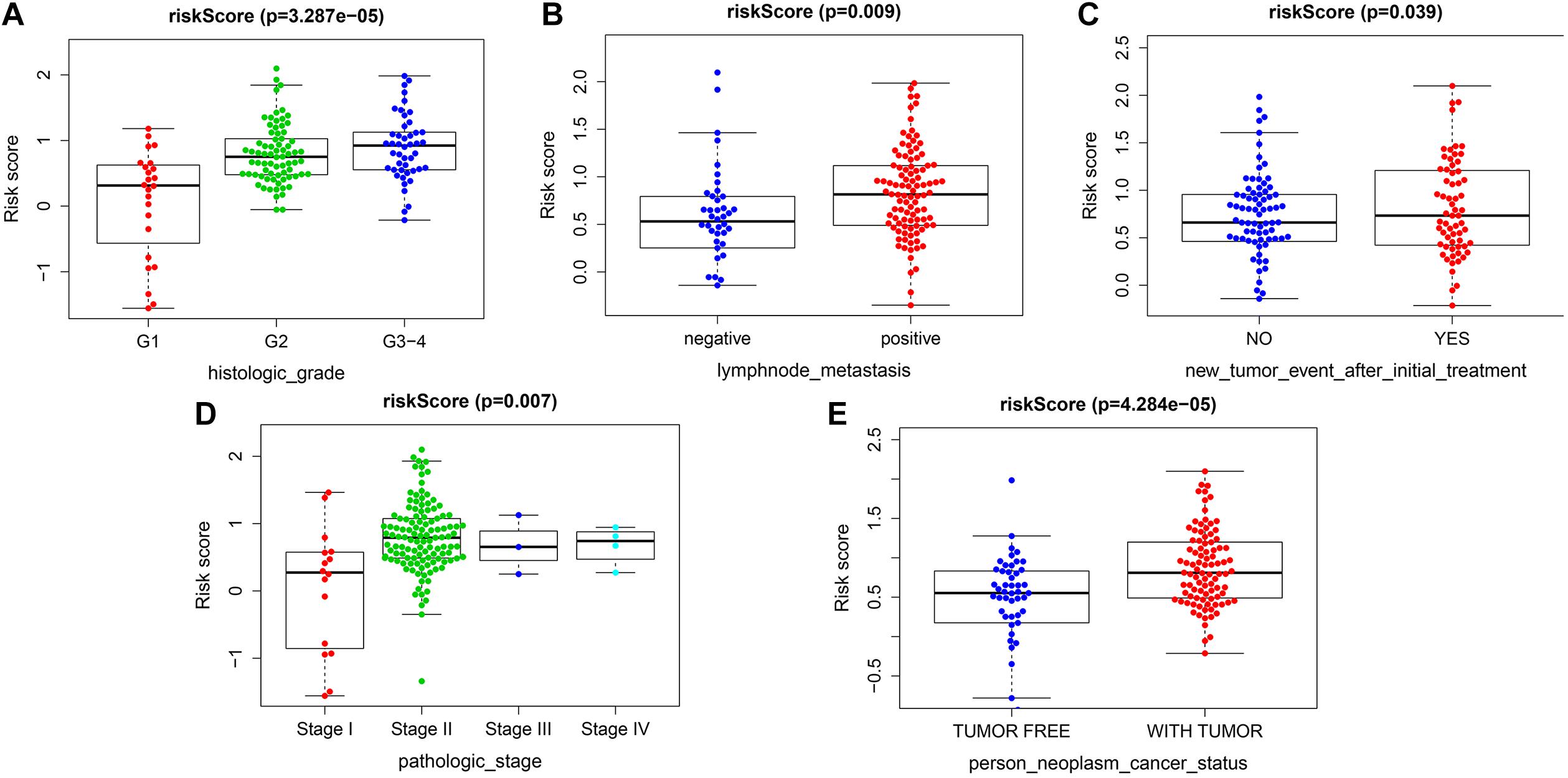
Figure 6. Correlation analysis between risk score and clinicopathological characteristics. (A) Histopathological grade. (B) Lymph node metastasis. (C) New tumor event after initiate treatment. (D) Pathological stage. (E) Individual neoplasm status.
External Validation of the Prognostic Model Combined International Cancer Genome Consortium and Gene Expression Omnibus Database
The validation cohort included 401 pancreatic cancer patients from ICGC (PACA-AU and PACA-CA) and GEO databases (GSE62452 and GSE57495). Based on the uniform cutoff value obtained in the TCGA cohort, the group with a high risk included 74 patients, and the group with a low risk included 327 patients. KM survival curve showed that the group with a high risk had a significantly lower OS compared with the group with a low risk (p < 0.001) (Figures 7A–C). The AUC values for the risk score predicting OS at 1, 3, and 5 years were 0.589, 0.560, and 0.586, respectively (Supplementary Material 2).
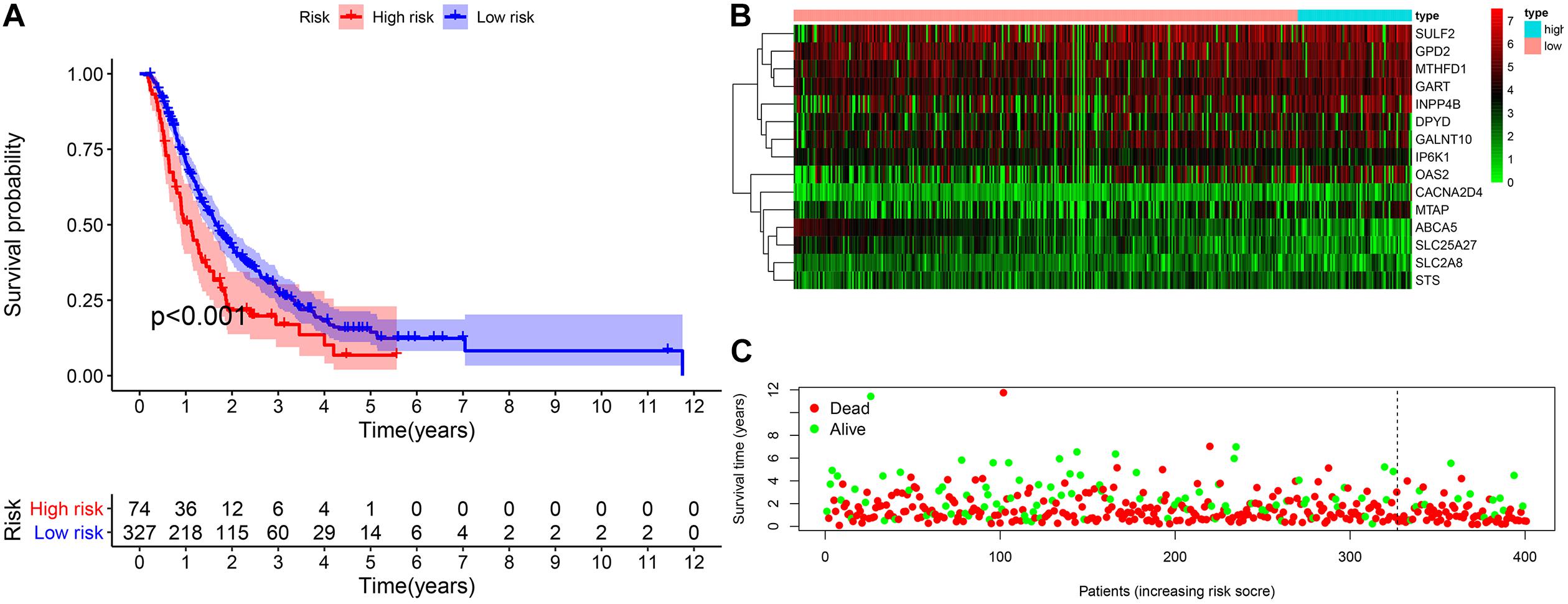
Figure 7. External validation of the prognostic model. (A) The Kaplan–Meier curve of overall survival. (B,C) The heatmap of the 15 genes and the survival status of patients.
Gene Set Enrichment Analysis Between Different Risk Groups
We identified five oncogenic gene sets with significant enrichment in the group with a high risk: p53 signaling pathway (NES = 1.99, NOM p-value < 0.001), pathways in cancer (NES = 1.87, NOM p-value < 0.001), cell cycle (NES = 1.92, NOM p-value < 0.001), pancreatic cancer (NES = 1.85, NOM p-value < 0.001), and small cell lung cancer (NES = 1.83, NOM p-value = 0.002) (Figure 8A), while the enriched gene set in the low-risk group was significantly related to metabolism (Figure 8B), indicating that the metabolic activity of the high-risk group was significantly different from that of the low-risk group.
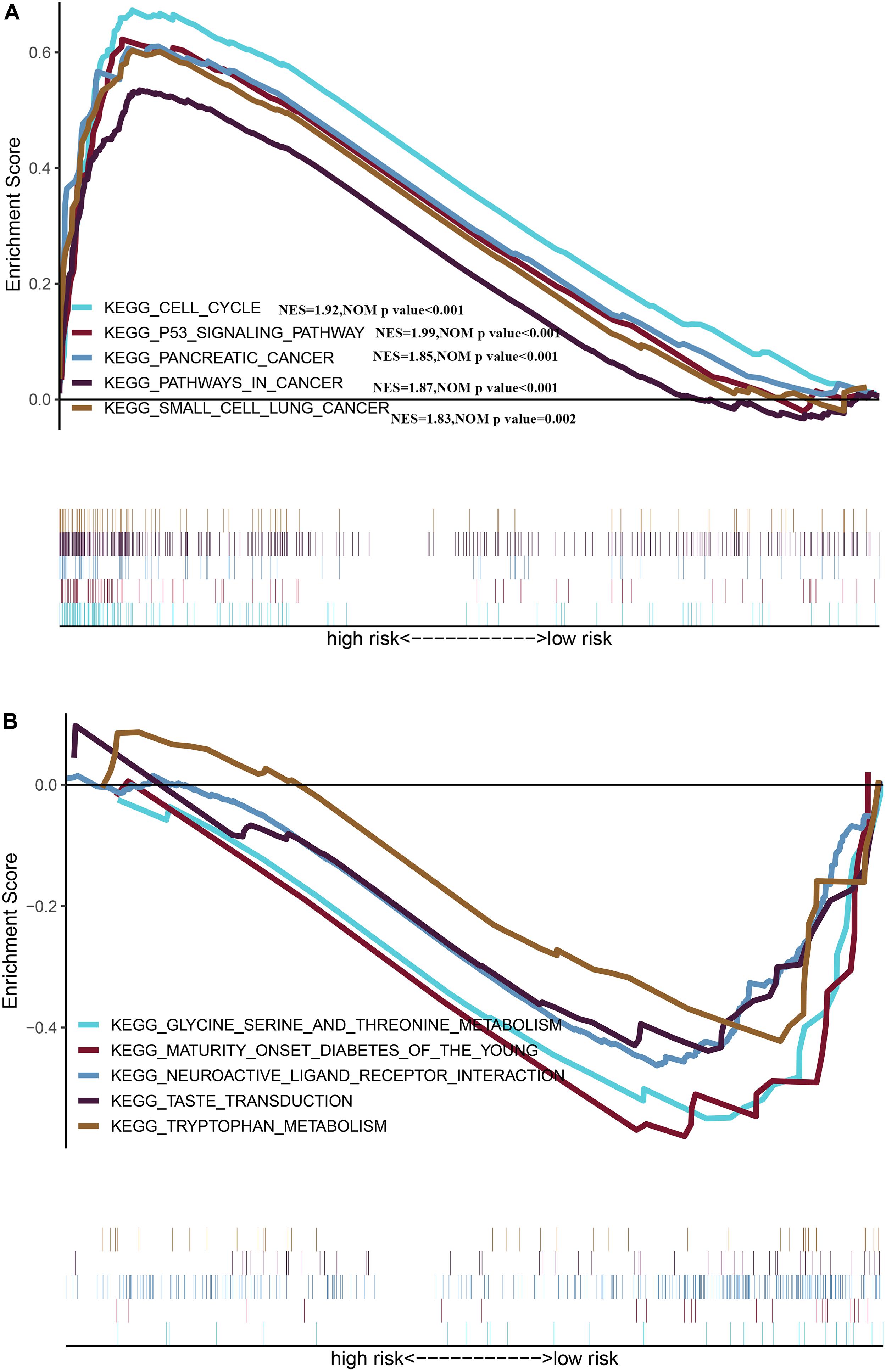
Figure 8. Gene Set Enrichment Analysis between different risk groups (A) Multiple GSEA plot of the KEGG pathways enriched for the high-risk group. (B) Multiple GSEA plot of the KEGG pathways enriched for the low-risk group.
Tumor-Infiltrating Immune Cells Between Different Risk Groups
The results of the TIMER database showed that there was a negative correlation between risk score and CD4T cell infiltration (Figure 9B). The group with a high risk exhibited an obviously higher infiltration level of macrophage M0 compared with the group with a low risk, while the group with a low risk exhibited an obviously higher infiltration level of B cells and CD8T lymphocytes (Figures 9A,C). There was a negative correlation between macrophage M0, and B cells and CD8T cells (Figure 9D). Besides, the risk score was positively associated with the expression level of CD274 (PDL1) (r = 0.369, p < 0.001) (Figures 9E,F).
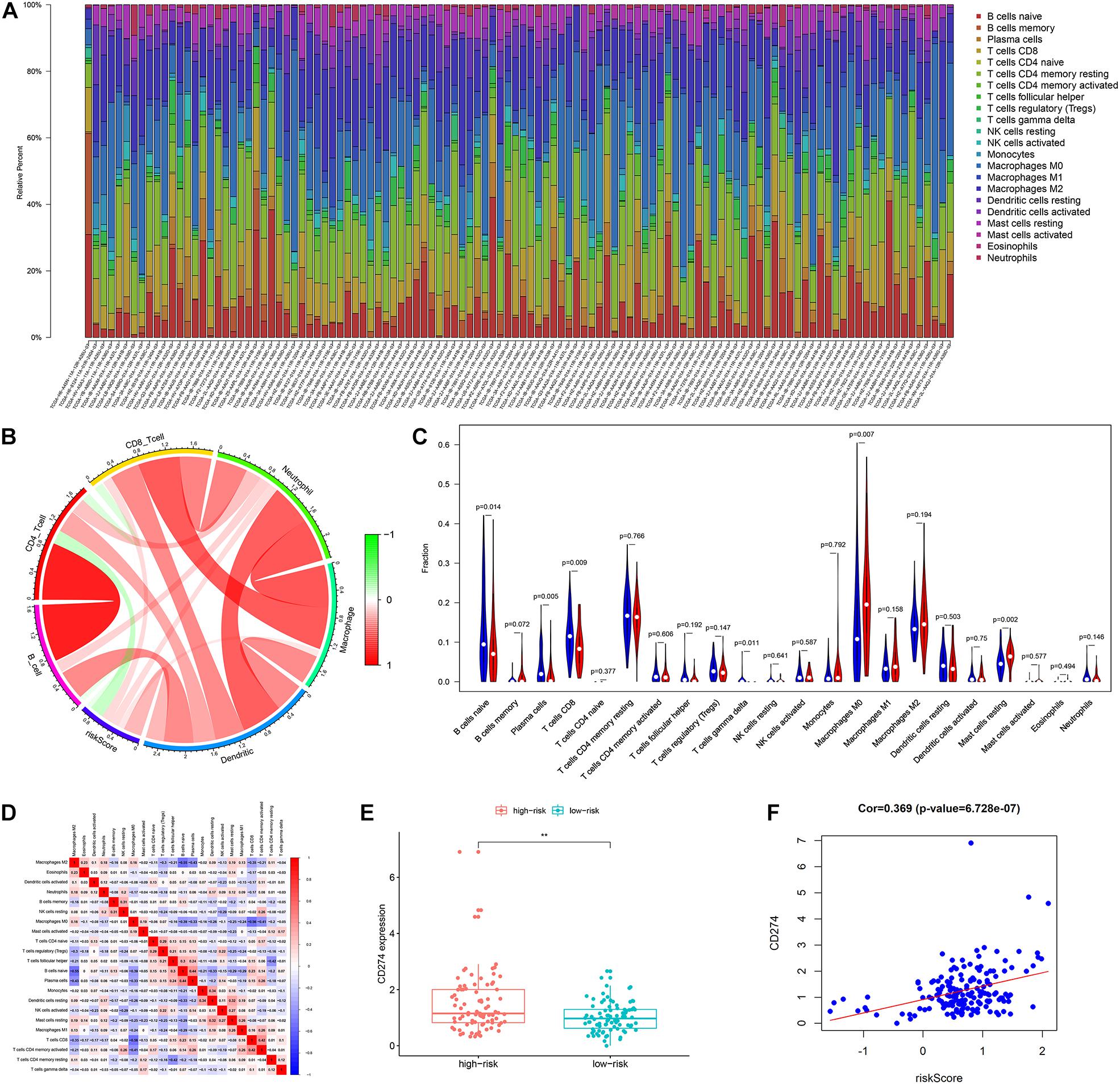
Figure 9. The landscape of immune infiltration in high- and low-risk HCC patients. (A) The bar plot of immune infiltration in high- and low-risk HCC patients (B) Correlation of the risk score with the immune infiltration of six kinds of immune cells (TIMER method). (C) Violin plots visualizing significantly different immune cells between high-risk and low-risk patients (CIBERSORT method, red represents the high-risk group, blue represents the low-risk group). (D) Correlation matrix of all 22 immune cells proportions. (E) Boxplot plot of the expression level of CD274 between high-risk and low-risk patients. (F) Correlation of the risk score with the expression of CD274 (p-value significant codes: 0 ≤ ∗∗∗ < 0.001 ≤ ∗∗ < 0.01 ≤ ∗ < 0.05).
Building a Survival Predictive Nomogram
The nomogram we constructed consists of tumor status, lymph node metastasis, and risk score. Each index is an independent factor affecting prognosis. We can estimate patients’ 1-, 3-, and 5-year survival rates based on the cumulative scores of the three indicators (Figure 10A). We used two methods to evaluate the accuracy of the nomogram. The large overlap between the calibration curve and the reference line indicated that the predicted survival rate is close to the actual survival rate, especially in the prediction of patients’ 3- and 5-year survival rate (Figure 10B). The ROC curve demonstrates a better prediction performance exhibited by the combined model compared with a single prediction index (Figure 10C). The concordance index was 0.71, which indicated that the probability of the predicted results consistent with the observed results was high (Supplementary Material 3). Therefore, the combination of risk score and clinical factors can reliably assist in evaluating pancreatic cancer patients’ prognosis.
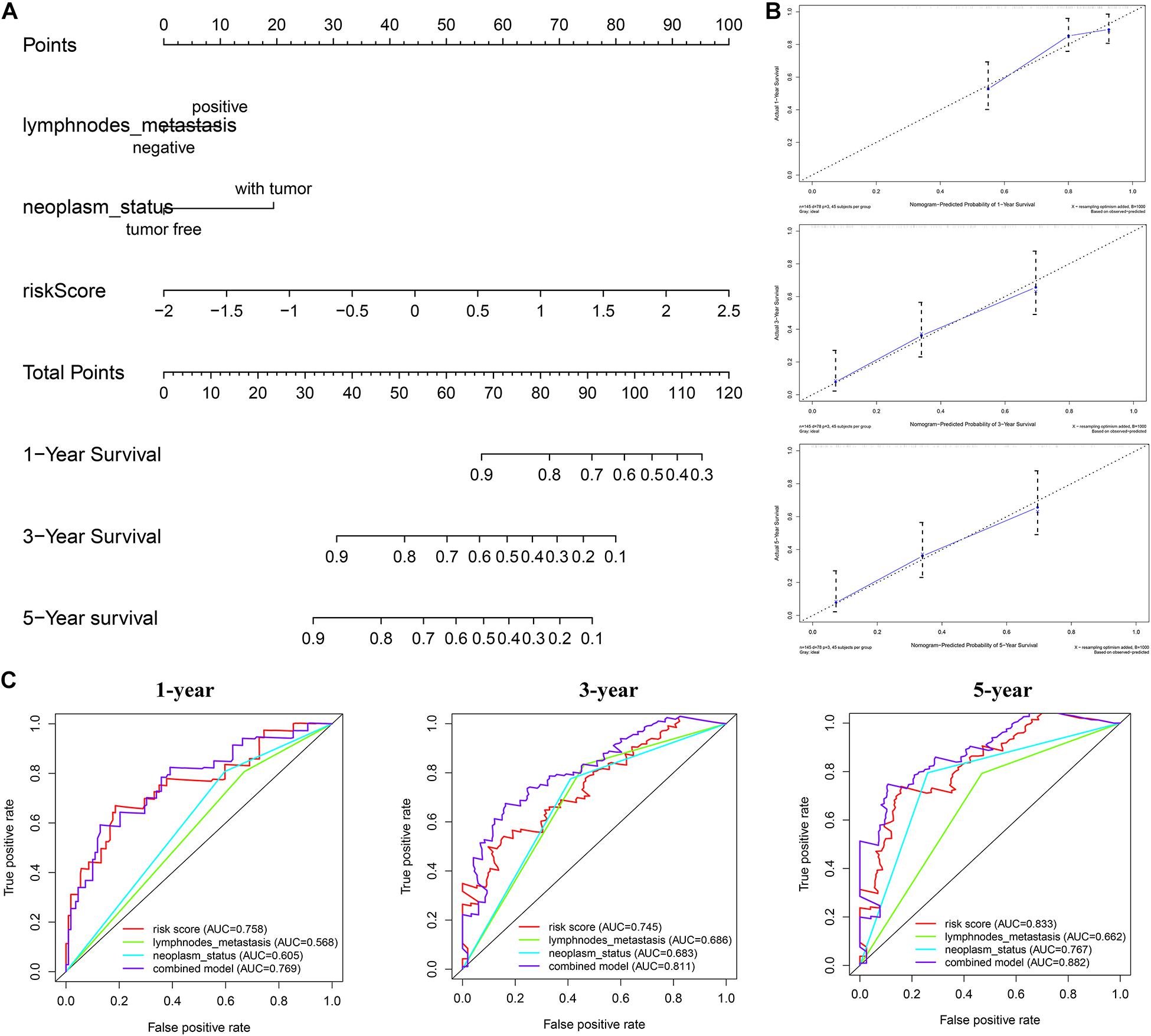
Figure 10. Construction of combined prognostic model in TCGA cohort. (A) Nomogram for predicting the probability of 1-, 3-, and 5-year overall survival (OS) for pancreatic cancer patients. (B) Calibration plot of the nomogram for predicting the probability of OS at 1, 3, and 5 years. (C) Time-dependent ROC curve analyses of the combined prognostic model.
Discussion
Pancreatic cancer is a highly malignant digestive tract tumor. Because of its concealed early symptoms, rapid disease progression, low resection rate, and low effective rate of chemotherapy, patients have a very poor prognosis (Ilic and Ilic, 2016). With the accumulation of high-throughput sequencing data, more and more biomarkers have been developed for diagnosing and treating pancreatic cancer. These prognostic signatures involve m6A methylation, autophagy, immunity, and many other aspects (Zheng et al., 2018; Wu et al., 2019; Zhou et al., 2019; Tian et al., 2020; Yue et al., 2020). In recent years, more and more evidence shows that reprogramming metabolism could greatly affect pancreatic cancer in terms of the occurrence, the development, as well as the treatment (Qin et al., 2020). However, the prognostic signatures related to metabolic reprogramming in pancreatic cancer are far from fully cleared.
Patients (572) with complete prognostic information were included in this study. First, we compared 178 pancreatic cancer tissues with 171 normal pancreatic tissues in TCGA and GTEx databases, and identified 379 DEMRGs. Then univariate Cox regression analysis together with the Lasso regression assisted in constructing a novel prognostic model. The unified risk score formula together with the cutoff value were considered to divide patients into a group with a high risk and a group with a low risk. The ROC curve showed the prognostic model with high accuracy in predicting OS, DSS, and PFS of patients. There were 15 genes included into our signature. Among them, ABCA5 is a member of the ATP binding cassette (ABC) transporters, which play a variety of roles in cancer biology and drug resistance. Low expression of ABCA5 is associated with poor prognosis of serous ovarian cancer (Hedditch et al., 2014). Irene Aksoy and others (Aksoy et al., 2017) combined sequencing technology with IPSC technology to identify that GTDC1 is related to neurodevelopmental disorders. Ema et al. (2015) found that SLC25A27 was amplified in advanced gastric cancer with lymph node metastasis. Sulfate endonuclease SULF2 regulates heparan sulfate protein polysaccharide 6-O-sulfation. Alhasan reported that the increase in SULF2 in PDAC is related to advanced tumor stage, vascular invasion, short interval between imaging progression, and short OS (Alhasan et al., 2016). GPD2 is a component of glycerol phosphate shuttle, which can promote the oxidation of glucose, thus, promoting the production of acetyl-CoA. Langston found that GPD2 is involved in the regulation of macrophage inflammation (Langston et al., 2019). MTHFD1 is an enzyme that provides tetrahydrofolic acid-carbon derivatives. Yu found that the high expression of MTHFD1 in hepatocellular carcinoma is associated with a lower survival rate and higher recurrence rate (Yu et al., 2019). Type II inositol polyphosphate 4-phosphatase (INPP4B) is a member of the PI3K/Akt signaling pathway. Zhai found that the overexpression of INPP4B in pancreatic cancer could lead to poor OS and DFS (Zhai et al., 2019). Glycosylation can remarkably affect tumor invasion and immune escape. Zhang found that the high expression of GALNT10 in high-grade ovarian serous cancer (HGSC) is related to immunosuppressive microenvironment, thus promoting tumor progression (Zhang et al., 2020). No reports focus on studying the effect of the remaining genes on cancer.
The group with a high risk presented a worse prognosis compared with the group with a low risk. The external validation results of the ICGC and the GEO cohorts further confirm the effectiveness of this prognostic model. GSEA revealed the oncological characteristics with significant enrichment in the group with a high risk, and pancreatic cancer is one of them, while the group with a low risk was associated with multiple metabolic pathways, indicating that the imbalance of tumor metabolic microenvironment may affect the progression of pancreatic cancer. The tumor microenvironment is a hot topic in the field of tumor research in recent years. Multiple studies have shown that metabolic reprogramming can have a significant impact on the tumor microenvironment (Lyssiotis and Kimmelman, 2017; Reina-Campos et al., 2017). Immune cells are an important component of the tumor microenvironment, which has been proved to be valuable in predicting the prognosis of tumors (Gentles et al., 2015). YIno found that tumor-infiltrating CD8T cells can be used to independently predict the prognosis of pancreatic cancer, and the high infiltration of CD8T cells is associated with longer survival (Ino et al., 2013). In this study, we also found that the proportion of CD8T cell infiltration in the group with a low risk was higher than the group with a high risk, further confirming the prognostic value owned by tumor-infiltrating CD8T cells in pancreatic cancer. Programmed cell death ligand 1 is one protein encoded by the CD274 gene. When it binds to PD1, it transmits a negative regulatory signal to T-cells, induces T-cells to enter a resting state, reduces the proliferation of CD8T cells in lymph nodes, making them unable to recognize cancer cells, reduces T-cell proliferation or apoptosis, effectively relieves the immune response of the body, and promotes further proliferation of cancer cells (Chen and Han, 2015; Naidoo et al., 2015). This study found that the risk score was positively related to the expression level of CD274 (PDL1), so the group with a high risk exhibited a poor prognosis possibly caused by the mechanism of immune escape. Besides, we can also predict the degree of tumor differentiation, clinicopathological stage, and lymph node metastasis according to the risk score, which has important reference value for clinical decision making. As revealed by the univariate and multivariate Cox regression analyses, individual neoplasm status, lymph node metastasis, as well as risk score were independent predictors of prognosis. We combined three indicators to construct one nomogram for the prediction of 1-, 3-, and 5-year OS of pancreatic cancer. The nomogram further enriches the prognosis evaluation system of pancreatic cancer, and the predictive ability of the risk score is further improved. The nomogram has a better prediction effect than a single predictor.
The study integrated as well as analyzed high-throughput sequencing data from multiple databases, and a personalized nomogram for survival prediction was gradually created. However, due to the lack of corresponding clinical data in the validation queue, we only performed internal validation on nomogram. Metabolic genes in the model may be potential targets for diagnosis or treatment of pancreatic cancer, and their detailed mechanisms need to be explored with the help of in vivo and in vitro verification experiments. This study is only a retrospective study, and further prospective results are needed to support each other.
Conclusion
The study focused on constructing a signature and a nomogram associated with metabolic reprogramming for predicting the prognosis of pancreatic cancer, which may help to further improve the treatment strategy of pancreatic cancer.
Data Availability Statement
The original contributions presented in the study are included in the article/Supplementary Material, further inquiries can be directed to the corresponding author/s.
Author Contributions
JH and LW designed this study. JH analyzed the data in this study, interpreted the findings, and drafted the manuscript. LW and YZ carried out data management and revised the manuscript. All authors reviewed the final version of the manuscript.
Conflict of Interest
The authors declare that the research was conducted in the absence of any commercial or financial relationships that could be construed as a potential conflict of interest.
Supplementary Material
The Supplementary Material for this article can be found online at: https://www.frontiersin.org/articles/10.3389/fgene.2021.561254/full#supplementary-material
Supplementary Material 1 | The risk score distribution of TCGA.
Supplementary Material 2 | The time-dependent ROC curve for external validation.
Supplementary Material 3 | Concordance index for the nomogram.
Abbreviations
TCGA, The Cancer Genome Atlas; ICGC, International Cancer Genome Consortium; GEO, Gene Expression Ominibus; GTEx, genotype-tissue expression comprehensive database; DEMRGs, differentially expressed metabolic-related genes; GO, Gene Ontology; KEGG, Kyoto Encyclopedia of Genes and Genomes; GSEA, gene set enrichment analysis; RS, risk score; HR, high risk; LR, low risk; KM, Kaplan–Meier; ROC, receiver operating characteristic; AUC, the area under the curve; OS, overall survival; DSS, disease special survival; DFS, disease-free survival; PFS, progression-free survival; FDR, false discovery rate.
Footnotes
- ^ https://portal.gdc.cancer.gov/
- ^ https://xenabrowser.net/
- ^ https://icgc.org/
- ^ https://www.ncbi.nlm.nih.gov/geo/
References
Aksoy, I., Utami, K. H., Winata, C. L., Hillmer, A. M., Rouam, S. L., Briault, S., et al. (2017). Personalized genome sequencing coupled with iPSC technology identifies GTDC1 as a gene involved in neurodevelopmental disorders. Hum. Mol. Genet. 26, 367–382. doi: 10.1093/hmg/ddw393
Alhasan, S. F., Haugk, B., Ogle, L. F., Beale, G. S., Long, A., Burt, A. D., et al. (2016). Sulfatase-2: a prognostic biomarker and candidate therapeutic target in patients with pancreatic ductal adenocarcinoma. Br. J. Cancer 115, 797–804. doi: 10.1038/bjc.2016.264
Alistar, A., Morris, B. B., Desnoyer, R., Klepin, H. D., Hosseinzadeh, K., Clark, C., et al. (2017). Safety and tolerability of the first-in-class agent CPI-613 in combination with modified FOLFIRINOX in patients with metastatic pancreatic cancer: a single-centre, open-label, dose-escalation, phase 1 trial. Lancet Oncol. 18, 770–778. doi: 10.1016/s1470-2045(17)30314-5
Bapat, A. A., Hostetter, G., Von Hoff, D. D., and Han, H. J. N. R. C. (2011). Perineural invasion and associated pain in pancreatic cancer. Nat. Rev. Cancer 11, 695–707. doi: 10.1038/nrc3131
Biancur, D. E., and Kimmelman, A. C. J. (2018). The plasticity of pancreatic cancer metabolism in tumor progression and therapeutic resistance. Biochim. Biophys. Acta Rev. Cancer 1870, 67–75. doi: 10.1016/j.bbcan.2018.04.011
Chaika, N. V., Yu, F., Purohit, V., Mehla, K., Lazenby, A. J., DiMaio, D., et al. (2012). Differential expression of metabolic genes in tumor and stromal components of primary and metastatic loci in pancreatic adenocarcinoma. PLoS One 7:e32996. doi: 10.1371/journal.pone.0032996
Chen, B., Khodadoust, M. S., Liu, C. L., Newman, A. M., and Alizadeh, A. A. (2018). “Profiling tumor infiltrating immune cells with CIBERSORT,” in Cancer Systems Biology, ed. L. von Stechow (New York, NY: Humana Press), 243–259.
Chen, L., and Han, X. (2015). Anti–PD-1/PD-L1 therapy of human cancer: past, present, and future. J. Clin. Invest. 125, 3384–3391. doi: 10.1172/jci80011
Daemen, A., Peterson, D., Sahu, N., McCord, R., Du, X., Liu, B., et al. (2015). Metabolite profiling stratifies pancreatic ductal adenocarcinomas into subtypes with distinct sensitivities to metabolic inhibitors. Proc. Natl. Acad. Sci. U.S.A. 112, E4410–E4417.
Ema, A., Waraya, M., Yamashita, K., Kokubo, K., Kobayashi, H., Hoshi, K., et al. (2015). Identification of EGFR expression status association with metastatic lymph node density (ND) by expression microarray analysis of advanced gastric cancer. Cancer Med. 4, 90–100. doi: 10.1002/cam4.311
Gentles, A. J., Newman, A. M., Liu, C. L., Bratman, S. V., Feng, W., Kim, D., et al. (2015). The prognostic landscape of genes and infiltrating immune cells across human cancers. Nat. Med. 21:938. doi: 10.1038/nm.3909
Hedditch, E. L., Gao, B., Russell, A. J., Lu, Y., Emmanuel, C., Beesley, J., et al. (2014). ABCA transporter gene expression and poor outcome in epithelial ovarian cancer. J. Natl. Cancer Inst. 106:dju149.
Huo, J., Wu, L., and Zang, Y. J. (2020). A prognostic model of 15 immune-related gene pairs associated with tumor mutation burden for hepatocellular carcinoma. Front. Mol. Biosci. 7:581354. doi: 10.3389/fmolb.2020.581354
Huo, J., Wu, L., and Zang, Y. J. (2021b). Development and validation of a CTNNB1-associated metabolic prognostic model for hepatocellular carcinoma. J. Cell. Mol. Med. 25, 1151–1165. doi: 10.1111/jcmm.16181
Huo, J., Wu, L., Zang, Y., Dong, H., Liu, X., He, F., et al. (2021a). Eight-gene metabolic signature related with tumor-associated macrophages predicting overall survival for hepatocellular carcinoma. BMC Cancer 21:31. doi: 10.1186/s12885-020-07734-z
Ilic, M., and Ilic, I. J. (2016). Epidemiology of pancreatic cancer. World J. Gastroenterol. 22:9694.
Ino, Y., Yamazaki-Itoh, R., Shimada, K., Iwasaki, M., Kosuge, T., Kanai, Y., et al. (2013). Immune cell infiltration as an indicator of the immune microenvironment of pancreatic cancer. Br. J. Cancer 108, 914–923. doi: 10.1038/bjc.2013.32
Langston, P. K., Nambu, A., Jung, J., Shibata, M., Aksoylar, H. I., Lei, J., et al. (2019). Glycerol phosphate shuttle enzyme GPD2 regulates macrophage inflammatory responses. Nat. Immunol. 20, 1186–1195. doi: 10.1038/s41590-019-0453-7
Leek, J. T., Johnson, W. E., Parker, H. S., Jaffe, A. E., and Storey, J. D. J. B. (2012). The sva package for removing batch effects and other unwanted variation in high-throughput experiments. Bioinformatics 28, 882–883. doi: 10.1093/bioinformatics/bts034
Li, T., Fan, J., Wang, B., Traugh, N., Chen, Q., Liu, J. S., et al. (2017). TIMER: a web server for comprehensive analysis of tumor-infiltrating immune cells. Cancer Res. 77, e108–e110.
Lomberk, G., Dusetti, N., Iovanna, J., and Urrutia, R. (2019). Emerging epigenomic landscapes of pancreatic cancer in the era of precision medicine. Nat. Commun. 10:3875. doi: 10.1038/s41467-019-11812-7
Lyssiotis, C. A., and Kimmelman, A. C. J. T. (2017). Metabolic interactions in the tumor microenvironment. Trends Cell Biol. 27, 863–875. doi: 10.1016/j.tcb.2017.06.003
Naidoo, J., Page, D., Li, B., Connell, L., Schindler, K., Lacouture, M., et al. (2015). Toxicities of the anti-PD-1 and anti-PD-L1 immune checkpoint antibodies. Ann. Oncol. 26, 2375–2391. doi: 10.1093/annonc/mdv383
Neoptolemos, J. P., Kleeff, J., Michl, P., Costello, E., Greenhalf, W., and Palmer, D. H. (2018). Therapeutic developments in pancreatic cancer: current and future perspectives. Nat. Rev. Gastroenterol. Hepatol. 15, 333–348. doi: 10.1038/s41575-018-0005-x
Pavlova, N. N., and Thompson, C. B. (2016). The emerging hallmarks of cancer metabolism. Cell Metab. 23, 27–47. doi: 10.1016/j.cmet.2015.12.006
Possemato, R., Marks, K. M., Shaul, Y. D., Pacold, M. E., Kim, D., Birsoy, K., et al. (2011). Functional genomics reveal that the serine synthesis pathway is essential in breast cancer. Nature 476, 346–350. doi: 10.1038/nature10350
Qin, C., Yang, G., Yang, J., Ren, B., Wang, H., Chen, G., et al. (2020). Metabolism of pancreatic cancer: paving the way to better anticancer strategies. Mol. Cancer 19:50. doi: 10.1186/s12943-020-01169-7
Raez, L. E., Papadopoulos, K., Ricart, A. D., Chiorean, E. G., DiPaola, R. S., Stein, M. N., et al. (2013). A phase I dose-escalation trial of 2-deoxy-D-glucose alone or combined with docetaxel in patients with advanced solid tumors. Cancer Chemother. Pharmacol. 71, 523–530. doi: 10.1007/s00280-012-2045-1
Reina-Campos, M., Moscat, J., and Diaz-Meco, M. J. (2017). Metabolism shapes the tumor microenvironment. Curr. Opin. Cell Biol. 48, 47–53. doi: 10.1016/j.ceb.2017.05.006
Tian, G., Li, G., Liu, P., Wang, Z., and Li, N. (2020). Glycolysis-Based genes associated with the clinical outcome of pancreatic ductal adenocarcinoma identified by the cancer genome Atlas Data analysis. DNA Cell Biol. 39, 417–427. doi: 10.1089/dna.2019.5089
Ward, P. S., and Thompson, C. B. (2012). Metabolic reprogramming: a cancer hallmark even warburg did not anticipate. Cancer Cell 21, 297–308. doi: 10.1016/j.ccr.2012.02.014
Wu, M., Li, X., Zhang, T., Liu, Z., and Zhao, Y. (2019). Identification of a nine-gene signature and establishment of a prognostic nomogram predicting overall survival of pancreatic cancer. Front. Oncol. 9:996. doi: 10.3389/fonc.2019.00996
Yu, H., Wang, H., Xu, H.-R., Zhang, Y.-C., Yu, X.-B., Wu, M.-C., et al. (2019). Overexpression of MTHFD1 in hepatocellular carcinoma predicts poorer survival and recurrence. Future Oncol. 15, 1771–1780. doi: 10.2217/fon-2018-0606
Yue, P., Zhu, C., Gao, Y., Li, Y., Wang, Q., Zhang, K., et al. (2020). Development of an autophagy-related signature in pancreatic adenocarcinoma. Biomed. Pharmacother. 126:110080. doi: 10.1016/j.biopha.2020.110080
Zachar, Z., Marecek, J., Maturo, C., Gupta, S., Stuart, S. D., Howell, K., et al. (2011). Non-redox-active lipoate derivates disrupt cancer cell mitochondrial metabolism and are potent anticancer agents in vivo. J. Mol. Med. 89:1137. doi: 10.1007/s00109-011-0785-8
Zhai, S., Liu, Y., Lu, X., Qian, H., Tang, X., Cheng, X., et al. (2019). INPP4B as a prognostic and diagnostic marker regulates cell growth of pancreatic cancer via activating AKT. Onco Targets Ther. 12:8287. doi: 10.2147/ott.s223221
Zhang, G., Lu, J., Yang, M., Wang, Y., Liu, H., and Xu, C. J. (2020). Elevated GALNT10 expression identifies immunosuppressive microenvironment and dismal prognosis of patients with high grade serous ovarian cancer. Cancer Immunol. Immunother. 69, 175–187. doi: 10.1007/s00262-019-02454-1
Zheng, B., Peng, J., Mollayup, A., Bakri, A., Guo, L., Zheng, J., et al. (2018). Construction of a prognostic prediction system for pancreatic ductal adenocarcinoma to investigate the key prognostic genes. Mol. Med. Rep. 17, 216–224. doi: 10.3892/mmr.2017.7850
Keywords: pancreatic cancer, metabolic, prognostic, signature, The Cancer Genome Atlas
Citation: Huo J, Wu L and Zang Y (2021) Development and Validation of a Novel Metabolic-Related Signature Predicting Overall Survival for Pancreatic Cancer. Front. Genet. 12:561254. doi: 10.3389/fgene.2021.561254
Received: 12 May 2020; Accepted: 26 April 2021;
Published: 28 May 2021.
Edited by:
Jingmei Li, Genome Institute of Singapore (A∗STAR), SingaporeCopyright © 2021 Huo, Wu and Zang. This is an open-access article distributed under the terms of the Creative Commons Attribution License (CC BY). The use, distribution or reproduction in other forums is permitted, provided the original author(s) and the copyright owner(s) are credited and that the original publication in this journal is cited, in accordance with accepted academic practice. No use, distribution or reproduction is permitted which does not comply with these terms.
*Correspondence: Liqun Wu, wulq5810@126.com