- 1Yangzhou Maternal and Child Health Hospital, Yangzhou, China
- 2Harbin Children's Hospital, Harbin, China
- 3Xuzhou Children's Hospital, Xuzhou Medical University, Xuzhou, China
- 4School of Civil Engineering, Northeast Forestry University, Harbin, China
- 5Translational Medicine Research and Cooperation Center of Northern China, Heilongjiang Academy of Medical Sciences, Harbin, China
- 6Department of Pharmacology (State-Province Key Laboratories of Biomedicine- Pharmaceutics of China, Key Laboratory of Cardiovascular Research, Ministry of Education), College of Pharmacy, Harbin Medical University, Harbin, China
Autism is a common disease that seriously affects the quality of life. The role of circular RNAs (circRNAs) in autism remains largely unexplored. We aimed to detect the circRNA expression profile and construct a circRNA-based competing endogenous RNA (ceRNA) network in autism. Valproate acid was used to establish an in vivo model of autism in mice. A total of 1,059 differentially expressed circRNAs (477 upregulated and 582 downregulated) in autism group was identified by RNA sequencing. The expression of novel_circ_015779 and novel_circ_035247 were detected by real-time PCR. A ceRNA network based on altered circRNAs was established, with 9,715 nodes and 150,408 edges. Module analysis was conducted followed by GO and KEGG pathway enrichment analysis. The top three modules were all correlated with autism-related pathways involving “TGF-beta signaling pathway,” “Notch signaling pathway,” “MAPK signaling pathway,” “long term depression,” “thyroid hormone signaling pathway,” etc. The present study reveals a novel circRNA involved mechanisms in the pathogenesis of autism.
Introduction
Autism spectrum disorder (ASD) is a multifactorial neurodevelopmental disorder diagnosed mainly during early life onset, which is often combined with attention deficit/hyperactivity disorder, mental disorder, and intellectual disability (Vahabzadeh et al., 2018; Valiente-Palleja et al., 2018; Miryounesi et al., 2019). According to the Diagnostic and Statistical Manual of Mental Disorders, 5th ed (DSM-5), it is characterized by impaired social interactions and elevated stereotyped activities, and the global prevalence is about 1% (Lai et al., 2014). Autism prevalence is increasing globally. According to the latest data of Autism and Developmental Disabilities Monitoring (ADDM) network in 2018, ASD prevalence was 1 in 54 (https://www.cdc.gov/ncbddd/autism/data.html). Early screening and diagnosis are very important to improve the outcome of autism patients. Unfortunately, primary health care professionals are usually unaware of the early manifestations of autism, and the gold standard diagnostic tools, autism Diagnostic Interview Revised (ADI-R) and Autism Diagnostic Observation Schedule (ADOS), are time-consuming and expensive. The pathogenesis of autism is associated with genetic and epigenetic alterations (Olde Loohuis et al., 2015; Turner et al., 2017). However, there is no specific autism therapeutic drug because the pathogenesis mechanism of autism is still not fully clarified.
Circular RNAs (circRNAs) are a kind of non-coding RNAs with circular structure, which are rapidly becoming considered as critical regulators of gene expression networks (Salzman et al., 2013). Furthermore, studies have proved that circRNAs are widely expressed in tissue-and-developmental-stage-specific patterns, and a fraction of them displays conservation across species (Rybak-Wolf et al., 2015). Importantly, a general phenomenon was discovered that circRNAs bind to miRNAs, acting as miRNA sponges, and thereby affect the expression of target genes (Hansen et al., 2013). Several studies have shown that circRNAs are more abundantly expressed in the brain than other tissues in mammals (You et al., 2015; Li et al., 2017). CircRNAs also show dynamic expression during neurogenesis, synaptogenesis, and neuronal differentiation (Izuogu et al., 2018). These findings indicated that circRNAs are likely to play functional roles in neuron development and diseases.
Even though studies revealed that circRNAs are highly abundant in the brain, the expression profile, function, and mechanism of most circRNAs in autism are still largely unelucidated. Chen et al. (2020) found a series of autism-associated circRNAs in autism cortex samples. Our study aimed to determine the circRNA profile in brain tissues from valproic acid-induced mouse autism model and analyze their function and potential mechanism.
Materials and Methods
Establishment of A Mouse Model of Autism
C57BL/6 mice were bought from Liaoning Changsheng Biotechnology Co., Ltd. (Liaoning, China). Exposure to some neurotoxic drugs may induce fetal nervous system development disorders. Valproate acid (VPA) is a widely used drug to induce autism (Baronio et al., 2018; Eissa et al., 2018). The animal model of autism was established as previously described (Zheng et al., 2019). Briefly, female adult mice were mated to males overnight. Pregnant mice were injected with valproic acid (Sigma, Ronkonkoma, NY, USA) 500 mg/kg at embryonic day 12.5 (E12.5). Mice in control group received a considerable amount of normal saline. All pregnant mice were allowed to give birth naturally, and the 1st day of birth was recorded as postnatal day 1 (P1). Pups were weaned at the 23rd day (p23) after birth. The animal protocol was approved by the Harbin Children's Hospital Animal Care and Use Committee (JJ2017ZR0484).
Repetitive Self-Grooming Behavior Measurement
On the 32nd day after birth, mice were placed in open field box for 10 min to adapt to the environment, then start timing for 10 min to observe mice behavior (Onaolapo et al., 2017). The number of body cleaning with paws and face-washing actions was calculated.
Social Interaction Test
Social interaction test was used to assess active interaction time in a test mouse with a novel mouse. (1) Adaptation stage: Gently put the tested mice into the device (material: acrylic glass, 40 × 40 × 30 cm, covered with 2–3 cm thick bedding), and let them move freely inside 10 min. (2) Test stage: Take out the test mice that have just adapted, and put them in the test mice and interactive mice (the same strains of mice that have the same sex and age as the test mice that have not been raised in the same cage). The number of social behaviors (physical contact and following peers) and non-social behaviors (investigation) of the mice were recorded within 10 min.
Real-Time PCR Analysis
TRIzol reagent (Invitrogen, CA, USA) was used to extract total RNA. A reverse transcriptase kit (Roche, Mannheim, Germany) was used to synthesize first-strand cDNA. Reverse transcription was performed using HiScript® II Q RT SuperMixfor qPCR (Vazyme Biotech Co., Ltd., Nanjing, China). Real-time PCR analysis was performed on an ABI step one plus system (Applied Biosystems, CA, USA). Primer sequences were as follows: novel_circ_000430, 5′-ACCCGTCTTCAGTCTCCGT-3′ (forward), 5′-AATATCACCCACACCCTCAGC-3′ (reverse); novel_circ_015779, 5′-CTCTGCCTGGTGCTGGTATTG-3′ (forward), 5′-ATGTAACTCTCTCCCTCCCCTG-3′ (reverse); novel_circ_063340, 5′-GCTTACCGTGGAGATGTTTGAC-3′ (forward), 5′-CGCCTTCTCCAACACCTCA-3′ (reverse); β-actin, 5′-ACCCATTCTCTGTCTCGCAC-3′ (forward), 5′-ATCGTCACCCCCAAAACCTG-3′ (reverse). β-actin was used as an internal control. Target gene expression was analyzed using the 2−ΔΔCT method.
RNA Sequencing
RNA sequencing was conducted by Gene Denovo Biotechnology Co. (Guangzhou, China). The circRNA were identified using find_circ (Memczak et al., 2013). The edge R package (http://www.rproject.org/) was used to identify differentially expressed circRNAs. CircRNAs with P < 0.05 and |log2FoldChange| > 1 in a comparison between control and autism groups was identified as differentially expressed.
CircRNA-miRNA-mRNA Network Construction
The miRNA binding sites of annotated circRNAs in circBase was predicted by StarBase (v2.0) (Li et al., 2014). The miRNA binding sites of novel circRNAs were predicted by Mireap, Miranda (v3.3a) (Betel et al., 2010), and TargetScan (v7.0) (Agarwal et al., 2015). Subsequently, miRTarBase (v6.1) (Hsu et al., 2011) was used to predict mRNAs that interact with circRNAs through miRNAs. The established competing endogenous RNA (ceRNA) network was visualized by Cytoscape (Version 3.7.2) (Kohl et al., 2011).
Gene Ontology and Pathway Enrichment Analysis
Significantly enriched Gene Ontology (GO) terms in source genes comparing to the genome background were defined by hypergeometric test (Ashburner et al., 2000). Kyoto Encyclopedia of Genes and Genomes (KEGG) database was used to perform enrichment analysis (Kanehisa et al., 2008).
Statistical Analysis
Data are expressed as mean ± SEM and compared using Student's t-test. A two-tailed P < 0.05 was required for significance.
Results
A Mouse Model of Autism Was Successfully Established
To analyze different expression of circRNA in the brain of autistic mice, we established a mouse model of autism and verified the model through behavioral testing. The animal model of autism was induced as previously described (Zheng et al., 2019). On the 28th day after birth, their behavioral ontogeny was evaluated using the social interaction test, repetitive self-grooming behavior. Results showed that the offspring mice in the model group exhibited autism-like behavioral abnormalities (Figure 1).
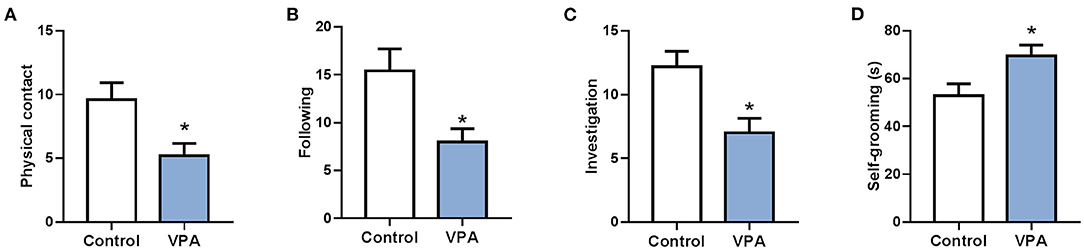
Figure 1. A mouse model of autism was successfully established. (A) The social interaction test (physical contact). (B) The social interaction test (following). (C) The social interaction test (investigation). (D) Repetitive self-grooming behavior. *P < 0.05 vs. Control; n = 4–5.
Expression Profile of circRNAs in A Mouse Model of Autism
The expression profile of circRNAs in brain tissues from autism mouse model and corresponding controls was evaluated using RNA sequencing. The hierarchical cluster analysis of circRNA is shown in Figure 2A. The volcano plots of circRNAs are shown in Figure 2B. A total of 1,059 altered circRNAs were identified in the autism group, with 477 upregulated and 582 downregulated (GSE163904, Figure 2C, Table 1, and Supplementary Material).
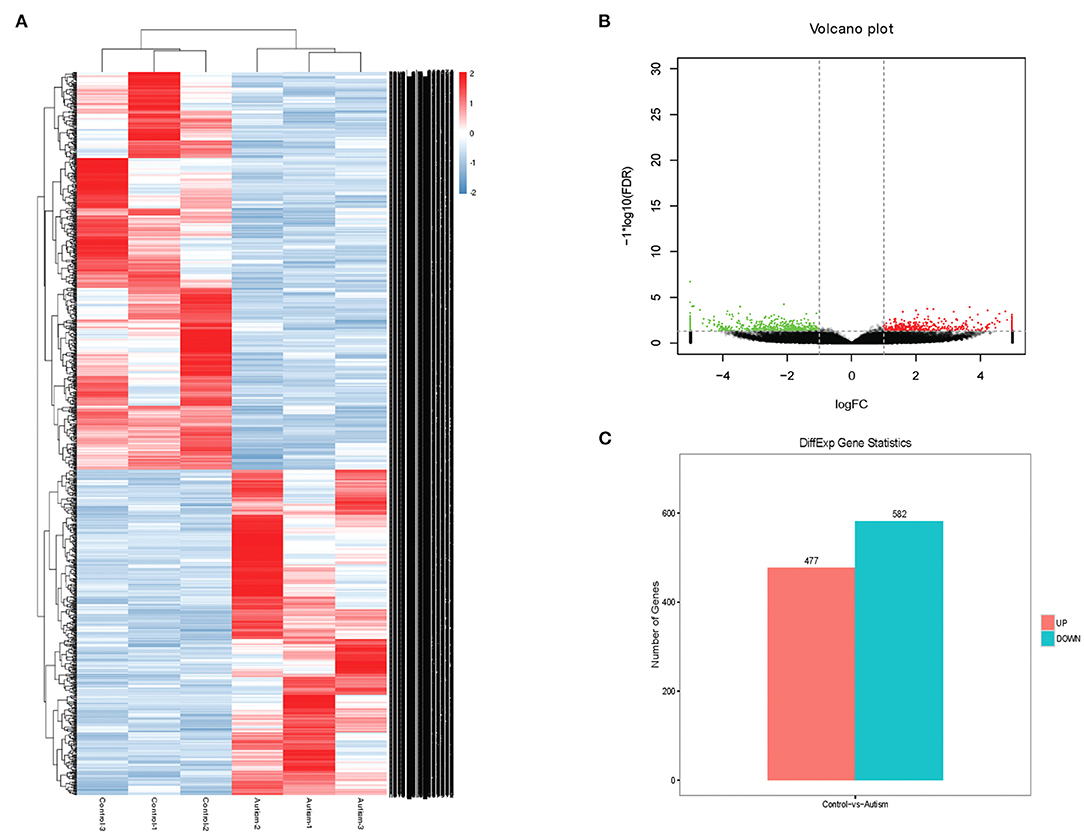
Figure 2. Differentially expressed circRNAs in brain from autism mice. (A) Heat map. (B) Volcano plots. (C) The number of differentially expressed circRNAs in a mouse model of autism.
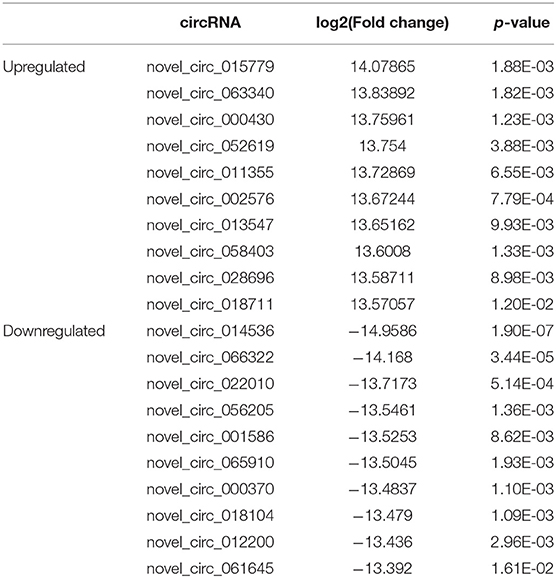
Table 1. Biological information regarding the top 10 upregulated and downregulated circRNAs in autism mouse model.
CircRNA Expression Verified by Real-Time PCR
The expressions of novel_circ_015779 and novel_circ_035247 were detected by real-time PCR assay. The expression levels of novel_circ_015779 and novel_circ_035247 were upregulated in the autism group (Figure 3). These results were in accordance with that in RNA sequencing.
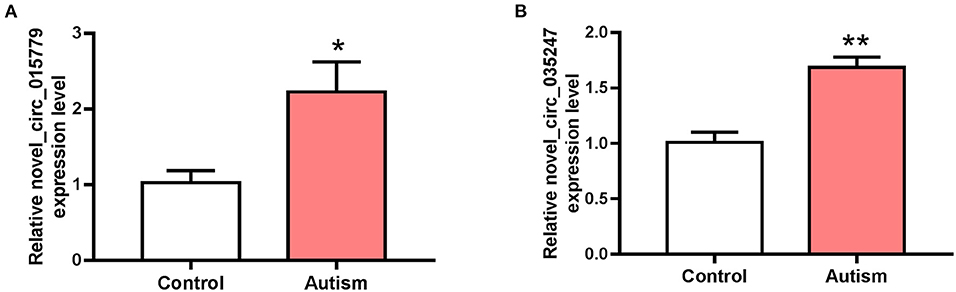
Figure 3. The expression of novel_circ_015779 and novel_circ_035247 in control and autism groups. (A) The expression of novel_circ_015779. (B) The expression of novel_circ_035247. *P < 0.05, **P < 0.01 vs. Control, n = 3.
The ceRNA Network Construction
The interactions between circRNAs/mRNA and miRNAs were predicted using StarBase (v2.0), Miranda (v3.3a), TargetScan (v7.0), and miRTarBase (v6.1). Then, a ceRNA network was constructed using differentially expressed circRNAs and bioinformatic predication results. The established circRNAs-miRNA-mRNA ceRNA network contains 9,715 nodes (including 1,059 circRNAs, 6,730 mRNA, and 1,926 miRNA) and 150,408 edges. A top 5 miRNA-based circRNA-miRNA-mRNA network is shown in Figure 4. The top 10 hub nodes are shown in Table 2. GO analysis showed that the ceRNA network was mainly associated with several biological processes, including “cellular process,” “biological regulation,” “regulation of biological process,” and “metabiological process.” Meanwhile, KEGG pathway analysis showed the ceRNA network was mainly associated with “axon guidance,” “MAPK signaling,” “Hippo signaling pathway,” and “ErbB signaling pathway” (Figure 5).
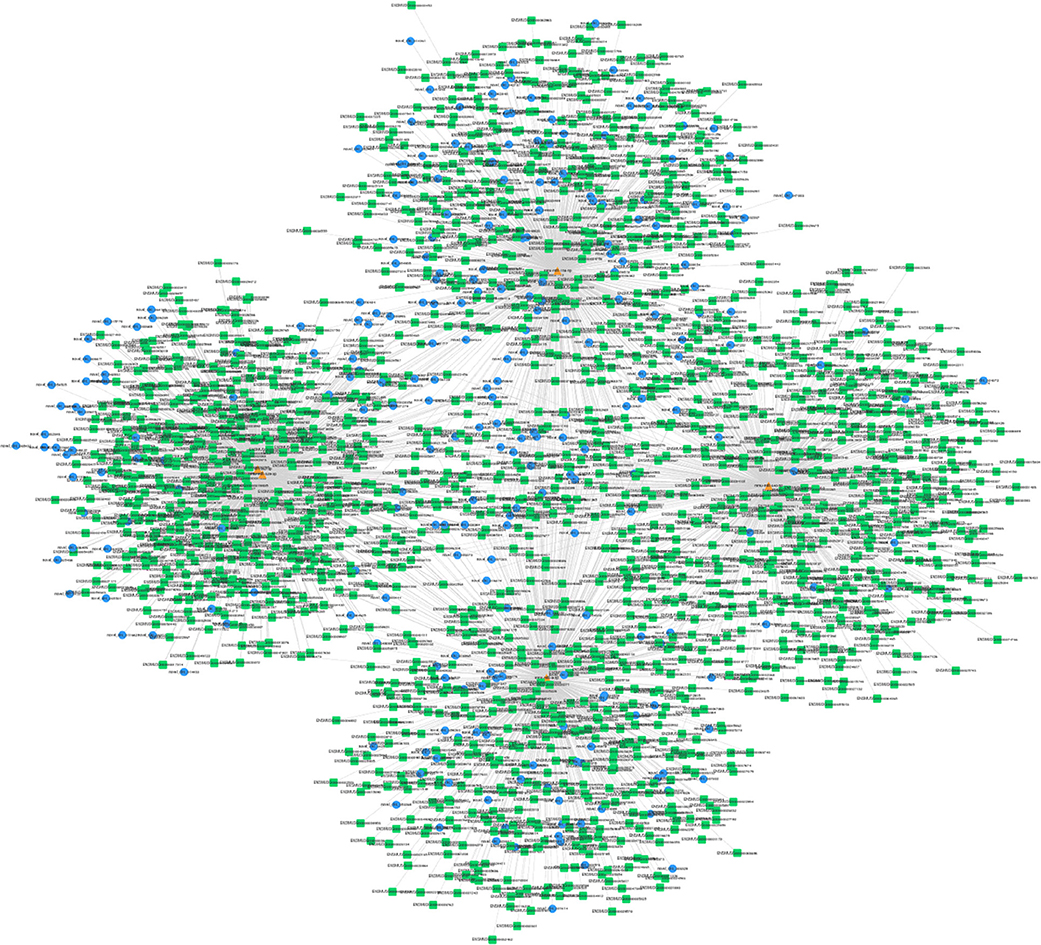
Figure 4. Top 5 miRNA-related circRNAs-miRNA-mRNA ceRNA network. Circular blue, triangular orange, and square green nodes represent circRNAs, miRNAs, and mRNAs, respectively.
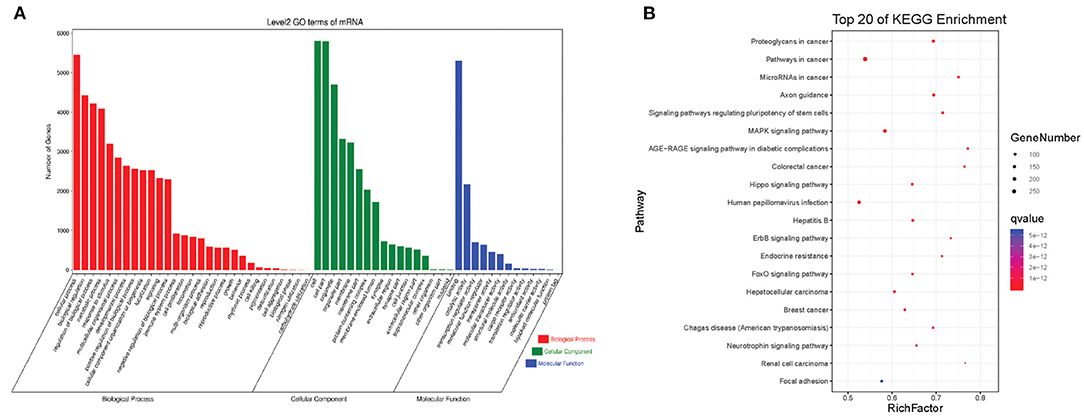
Figure 5. The GO and KEGG pathway enrichment of autism-related circRNAs-miRNA-mRNA ceRNA network. (A) GO enrichment. (B) KEGG pathway enrichment. The color bar represents the q-value.
Module Analysis of the ceRNA Network
Modules were screened using plug-in MCODE. GO and KEGG pathway enrichments were performed in the top three modules, respectively. Module 1 consists of 393 nodes (68 circRNAs, 239 miRNAs, and 86 mRNAs) and 1,508 edges, which are mainly associated with “HIF-1 signaling pathway,” “arginine and proline metabolism,” “TGF-beta signaling pathway,” “IL-17 signaling pathway,” etc. (Figure 6). Module 2 consists of 242 nodes (67circRNAs, 73 miRNAs, and 102 mRNAs) and 891 edges, which are mainly related to “renin secretion,” “cGMP-PKG signaling pathway,” “Notch signaling pathway,” “long term depression,” etc. (Figure 7). Module 3 consists of 289 nodes (75 circRNAs, 95 miRNAs, and 119 mRNAs) and 1,011 edges, which are mainly associated with “IL-17 signaling pathway,” “MAPK signaling pathway,” “C-type lectin receptor signaling pathway,” “thyroid hormone signaling pathway,” etc. (Figure 8).
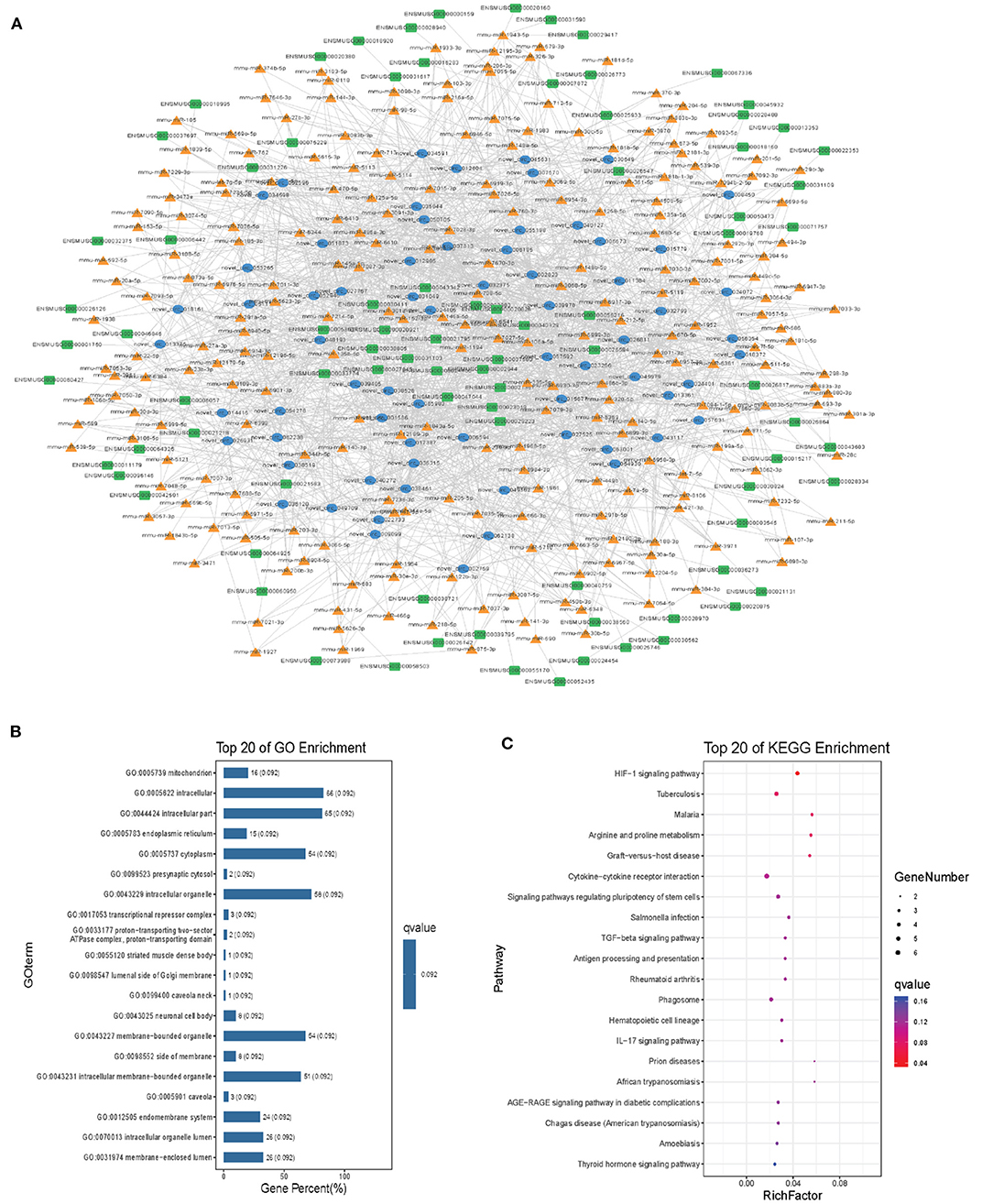
Figure 6. Module 1 from the autism-related ceRNA network. (A) Module 1 network. (B) GO enrichment. (C) KEGG pathway enrichment. Circular blue, triangular orange, and square green nodes represent circRNAs, miRNAs, and mRNAs, respectively.
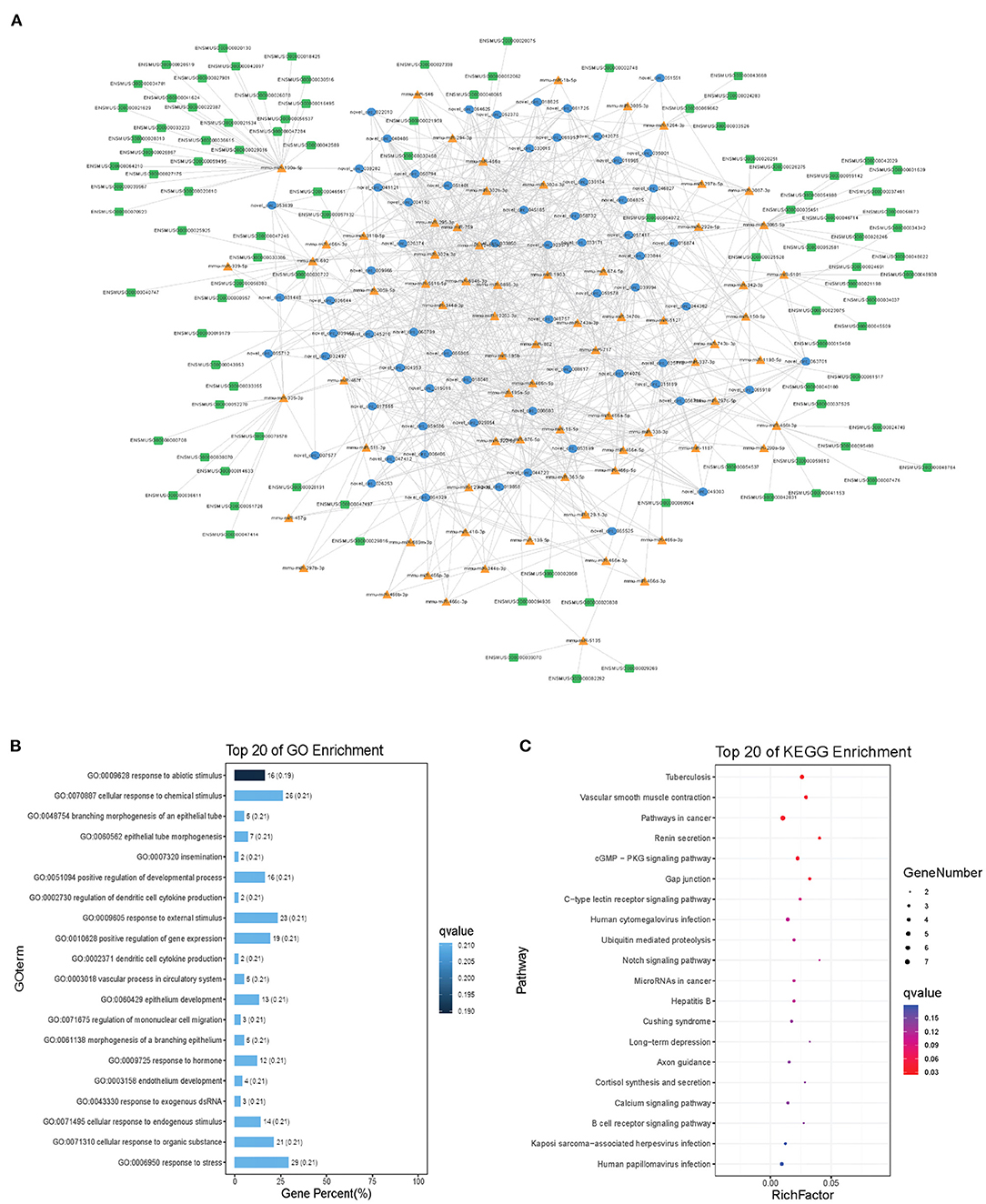
Figure 7. Module 2 from the autism-related ceRNA network. (A) Module 2 network. (B) GO enrichment. (C) KEGG pathway enrichment. Circular blue, triangular orange, and square green nodes represent circRNAs, miRNAs, and mRNAs, respectively.
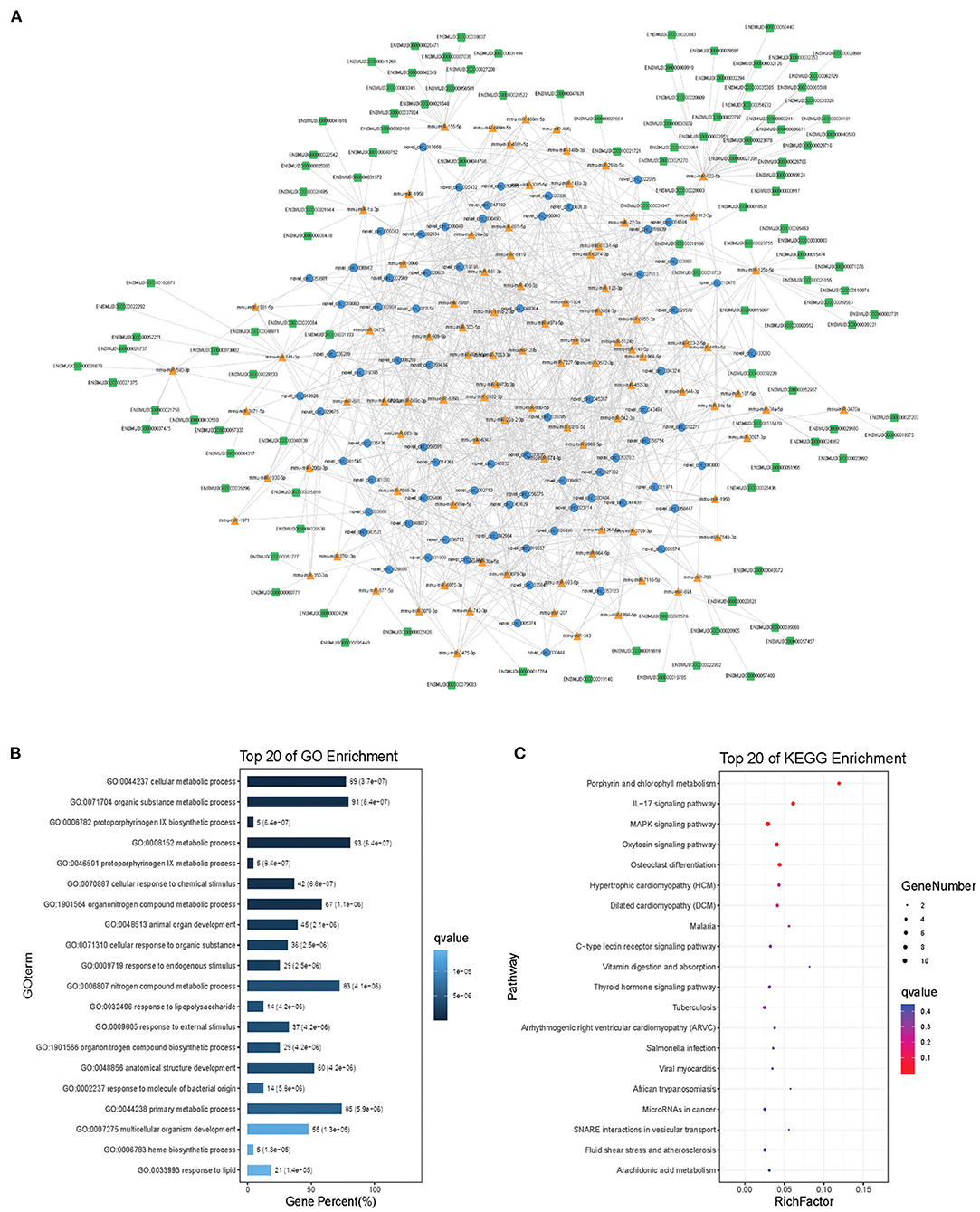
Figure 8. Module 3 from the autism-related ceRNA network. (A) Module 3 network. (B) GO enrichment. (C) KEGG pathway enrichment. Circular blue, triangular orange, and square green nodes represent circRNAs, miRNAs, and mRNAs, respectively.
Discussion
The incidence of autism is still increasing, which seriously affects the quality of human life. Despite the research progress that has been made in recent years, the pathogenesis mechanisms of autism are still not fully clarified. The development of bioinformatics facilitated the investigation of disease mechanisms and therapeutic strategies (Peng et al., 2017, 2018; Zhou et al., 2019). Our study comprehensively elucidated circRNA expression profile in a mouse model of autism and constructed a circRNA-associated ceRNA network.
CircRNAs are a special kind of non-coding RNAs with circular structure. CircRNAs could exert biological function through a ceRNA mechanism (circRNA-miRNA-mRNA), circRNA–protein interaction, or regulate translation (Du et al., 2017; Sun et al., 2019; Yi et al., 2019). This kind of non-coding RNAs is involved in various diseases, including cancers, cardiovascular diseases, and neuronal diseases (Chen et al., 2017; Sekar et al., 2018; Yang et al., 2019). CircRNAs are shown to be associated with schizophrenia and cognitive dysfunction (Zhang et al., 2017; Mahmoudi et al., 2019). However, the expression and role of circRNAs in autism is still poorly understood. Therefore, we detected the circRNA expression profile using RNA sequencing in a mouse model of autism.
RNA sequencing identified a total of 1,059 differentially expressed circRNAs in the autism group. The expression level of novel_circ_015779 and novel_circ_035247 was detected by real-time PCR assay. The RNA sequencing and real-time PCR assay achieved similar results.
CircRNAs could act as a ceRNA and thus exert biological functions. Using bioinformatic methods, we predicted the interaction between circRNAs and miRNA and constructed an autism-related ceRNA network. The ceRNA network contains 150,408 edges and 9,715 nodes, including 1,059 circRNAs, 1,926 miRNA, and 6,730 mRNA.
We then performed GO and KEGG analysis. The autism-related ceRNA network was signaling pathways including “axon guidance,” “MAPK signaling,” “Hippo signaling pathway,” and “ErbB signaling pathway.” Among these signaling pathways, the role of “axon guidance” and “MAPK signaling” in autism has been proved (Suda et al., 2011; Rosina et al., 2019). It has been reported that aberrant axon-guidance protein expression was observed in the brains of people with autism, including the decreased expression of PLXNA4 and ROBO2 (Suda et al., 2011). And the disruption of the MAPK pathways correlates with severity in idiopathic autism (Rosina et al., 2019).
The hub nodes, take miR-142-3p, miR-142a-5p, and miR-142b for example, may play an important role in autism. Mor et al. (2015) found that miR-142a-5p and miR-142a-3p were upregulated in brain tissues of autism patients compared with that in controls. It has been reported that the upregulation of these two miRNAs could induce apoptosis (Lu et al., 2015; Kim et al., 2018). Apoptosis was also related to autism (Dong et al., 2018). Therefore, miR-142 cluster and its related circRNAs may be involved in the pathogenesis of autism, which needs further investigation. miR-9-5p was also a hub nod, which targets Dlg4 (encode PSD-95). The deletion or variation of Dlg4 was associated with autism (Feyder et al., 2010). In the ceRNA network, novel_circ_000370, novel_circ_050499, novel_circ_021126, etc. may regulate Dlg4 through miR-9-5p.
Subsequently, network modules analysis was conducted. The top three modules were all correlated with autism-related pathways including “TGF-beta signaling pathway,” “Notch signaling pathway,” “MAPK signaling pathway,” “long-term depression,” etc. Take “TGF-beta signaling pathway” as an example; TGF-β1 was upregulated in the brain of autistic patients (Vargas et al., 2005). Early and adult hippocampal TGF-β1 overexpression led to a series of autism-related behaviors (Depino et al., 2011). The circRNAs involved in these signaling pathways may play a regulatory role in autism.
Circulating miRNAs were aberrantly expressed in autism individuals compared with that in controls (Mundalil Vasu et al., 2014; Hicks et al., 2016; Jyonouchi et al., 2019). In the serum of individuals with autism, 13 miRNAs were differentially expressed, some of which showed diagnostic potential for patients with autism (Mundalil Vasu et al., 2014). Subsequent research achieved similar results, that serum miRNAs may be promising biomarkers for autism (Kichukova et al., 2017; Jyonouchi et al., 2019). Besides, 14 miRNAs were identified differentially expressed in salivary samples from autism patients, and most of them significantly correlate with Vineland neurodevelopmental scores, including miR-27a, miR-23a, and miR-628-5p (Hicks et al., 2016). However, the function of these miRNAs in autism remains largely uninvestigated. In our ceRNA network, we identified a series of potential signaling pathways involved in the pathogenesis of autism, which may be helpful to clarify the role of miRNAs in autism. Besides, circRNAs also exist in the circulation system, which may also serve as biomarkers for autism. The expression of circRNAs in serum from autism patients and animal models still needs further investigation.
In conclusion, our study provides the expression profile of circRNAs in a mouse model of autism, which promotes our understanding in the pathogenesis mechanism of autism.
Data Availability Statement
The RNA sequencing results for this study could be found in the GEO repository with the accession number of GSE163904.
Ethics Statement
The animal study was reviewed and approved by Harbin children's hospital Animal Care and Use Committee [JJ2017ZR0484].
Author Contributions
JW, YJ, and WZ designed the study. HW and BL performed the animal experiment. ZY, CC, and YX analyzed the data. JW and YJ wrote the manuscript. All authors reviewed the manuscript.
Funding
This project was supported by the National Natural Science Foundation of China (81803524 and 51708092); the China Postdoctoral Science Foundation (2018M641878); the Heilongjiang Postdoctoral Foundation (LBH-Z18168); the Key Laboratory of Myocardial ischemia, Harbin Medical University, Ministry of Education, Heilongjiang Province, China (KF201611); Natural Science Foundation of Heilongjiang Province of China (H2017004); research project of maternal and child health in Jiangsu Province (F201920); and research project of Jiangsu Province Health Commission (H2019021).
Conflict of Interest
The authors declare that the research was conducted in the absence of any commercial or financial relationships that could be construed as a potential conflict of interest.
Acknowledgments
The authors want to thank all the participants in the research.
Supplementary Material
The Supplementary Material for this article can be found online at: https://www.frontiersin.org/articles/10.3389/fgene.2020.623584/full#supplementary-material
References
Agarwal, V., Bell, G. W., Nam, J. W., and Bartel, D. P. (2015). Predicting effective microRNA target sites in mammalian mRNAs. Elife 4:e05005. doi: 10.7554/eLife.05005.028
Ashburner, M., Ball, C. A., Blake, J. A., Botstein, D., Butler, H., Cherry, J. M., et al. (2000). Gene ontology: tool for the unification of biology. The gene ontology consortium. Nat. Genet. 25, 25–29. doi: 10.1038/75556
Baronio, D., Bauer-Negrini, G., Castro, K., Della-Flora Nunes, G., Riesgo, R., Mendes-da-Cruz, D. A., et al. (2018). Reduced CD4 T lymphocytes in lymph nodes of the mouse model of autism induced by valproic acid. Neuroimmunomodulation 25, 280–284. doi: 10.1159/000491395
Betel, D., Koppal, A., Agius, P., Sander, C., and Leslie, C. (2010). Comprehensive modeling of microRNA targets predicts functional non-conserved and non-canonical sites. Genome Biol. 11:R90. doi: 10.1186/gb-2010-11-8-r90
Chen, J., Li, Y., Zheng, Q., Bao, C., He, J., Chen, B., et al. (2017). Circular RNA profile identifies circPVT1 as a proliferative factor and prognostic marker in gastric cancer. Cancer Lett. 388, 208–219. doi: 10.1016/j.canlet.2016.12.006
Chen, Y. J., Chen, C. Y., Mai, T. L., Chuang, C. F., Chen, Y. C., Gupta, S. K., et al. (2020). Genome-wide, integrative analysis of circular RNA dysregulation and the corresponding circular RNA-microRNA-mRNA regulatory axes in autism. Genome Res. 30, 375–391. doi: 10.1101/gr.255463.119
Depino, A. M., Lucchina, L., and Pitossi, F. (2011). Early and adult hippocampal TGF-beta1 overexpression have opposite effects on behavior. Brain Behav. Immun. 25, 1582–1591. doi: 10.1016/j.bbi.2011.05.007
Dong, D., Zielke, H. R., Yeh, D., and Yang, P. (2018). Cellular stress and apoptosis contribute to the pathogenesis of autism spectrum disorder. Autism Res. 11, 1076–1090. doi: 10.1002/aur.1966
Du, W. W., Zhang, C., Yang, W., Yong, T., Awan, F. M., and Yang, B. B. (2017). Identifying and characterizing circRNA-protein interaction. Theranostics 7, 4183–4191. doi: 10.7150/thno.21299
Eissa, N., Jayaprakash, P., Azimullah, S., Ojha, S. K., Al-Houqani, M., Jalal, F. Y., et al. (2018). The histamine H3R antagonist DL77 attenuates autistic behaviors in a prenatal valproic acid-induced mouse model of autism. Sci. Rep. 8:13077. doi: 10.1038/s41598-018-31385-7
Feyder, M., Karlsson, R. M., Mathur, P., Lyman, M., Bock, R., Momenan, R., et al. (2010). Association of mouse Dlg4 (PSD-95) gene deletion and human DLG4 gene variation with phenotypes relevant to autism spectrum disorders and Williams' syndrome. Am. J. Psychiatry 167, 1508–1517. doi: 10.1176/appi.ajp.2010.10040484
Hansen, T. B., Jensen, T. I., Clausen, B. H., Bramsen, J. B., Finsen, B., Damgaard, C. K., et al. (2013). Natural RNA circles function as efficient microRNA sponges. Nature 495, 384–388. doi: 10.1038/nature11993
Hicks, S. D., Ignacio, C., Gentile, K., and Middleton, F. A. (2016). Salivary miRNA profiles identify children with autism spectrum disorder, correlate with adaptive behavior, and implicate ASD candidate genes involved in neurodevelopment. BMC Pediatr. 16:52. doi: 10.1186/s12887-016-0586-x
Hsu, S. D., Lin, F. M., Wu, W. Y., Liang, C., Huang, W. C., Chan, W. L., et al. (2011). miRTarBase: a database curates experimentally validated microRNA-target interactions. Nucleic Acids Res. 39, D163–D169. doi: 10.1093/nar/gkq1107
Izuogu, O. G., Alhasan, A. A., Mellough, C., Collin, J., Gallon, R., Hyslop, J., et al. (2018). Analysis of human ES cell differentiation establishes that the dominant isoforms of the lncRNAs RMST and FIRRE are circular. BMC Genomics 19:276. doi: 10.1186/s12864-018-4660-7
Jyonouchi, H., Geng, L., Toruner, G. A., Rose, S., Bennuri, S. C., and Frye, R. E. (2019). Serum microRNAs in ASD: association with monocyte cytokine profiles and mitochondrial respiration. Front. Psychiatry 10:614. doi: 10.3389/fpsyt.2019.00614
Kanehisa, M., Araki, M., Goto, S., Hattori, M., Hirakawa, M., Itoh, M., et al. (2008). KEGG for linking genomes to life and the environment. Nucleic Acids Res. 36, D480–D484. doi: 10.1093/nar/gkm882
Kichukova, T. M., Popov, N. T., Ivanov, I. S., and Vachev, T. I. (2017). Profiling of circulating serum MicroRNAs in children with autism spectrum disorder using stem-loop qRT-PCR assay. Folia Med. 59, 43–52. doi: 10.1515/folmed-2017-0009
Kim, J. O., Park, J. H., Kim, T., Hong, S. E., Lee, J. Y., Nho, K. J., et al. (2018). A novel system-level approach using RNA-sequencing data identifies miR-30-5p and miR-142a-5p as key regulators of apoptosis in myocardial infarction. Sci. Rep. 8:14638. doi: 10.1038/s41598-018-33020-x
Kohl, M., Wiese, S., and Warscheid, B. (2011). Cytoscape: software for visualization and analysis of biological networks. Methods Mol. Biol. 696, 291–303. doi: 10.1007/978-1-60761-987-1_18
Lai, M. C., Lombardo, M. V., and Baron-Cohen, S. (2014). Autism. Lancet 383, 896–910. doi: 10.1016/S0140-6736(13)61539-1
Li, J. H., Liu, S., Zhou, H., Qu, L. H., and Yang, J. H. (2014). starBase v2.0: decoding miRNA-ceRNA, miRNA-ncRNA and protein-RNA interaction networks from large-scale CLIP-Seq data. Nucleic Acids Res. 42, D92–D97. doi: 10.1093/nar/gkt1248
Li, L., Zheng, Y. C., Kayani, M. U. R., Xu, W., Wang, G. Q., Sun, P., et al. (2017). Comprehensive analysis of circRNA expression profiles in humans by RAISE. Int. J. Oncol. 51, 1625–1638. doi: 10.3892/ijo.2017.4162
Lu, X., Wei, Y., and Liu, F. (2015). Direct regulation of p53 by miR-142a-3p mediates the survival of hematopoietic stem and progenitor cells in zebrafish. Cell Discov. 1:15027. doi: 10.1038/celldisc.2015.27
Mahmoudi, E., Fitzsimmons, C., Geaghan, M. P., Shannon Weickert, C., Atkins, J. R., Wang, X., et al. (2019). Circular RNA biogenesis is decreased in postmortem cortical gray matter in schizophrenia and may alter the bioavailability of associated miRNA. Neuropsychopharmacology 44, 1043–1054. doi: 10.1038/s41386-019-0348-1
Memczak, S., Jens, M., Elefsinioti, A., Torti, F., Krueger, J., Rybak, A., et al. (2013). Circular RNAs are a large class of animal RNAs with regulatory potency. Nature 495, 333–338. doi: 10.1038/nature11928
Miryounesi, M., Bahari, S., Salehpour, S., Alipour, N., and Ghafouri-Fard, S. (2019). ELMO domain containing 1 (ELMOD1) gene mutation is associated with mental retardation and autism spectrum disorder. J. Mol. Neurosci. 69, 312–315. doi: 10.1007/s12031-019-01359-z
Mor, M., Nardone, S., Sams, D. S., and Elliott, E. (2015). Hypomethylation of miR-142 promoter and upregulation of microRNAs that target the oxytocin receptor gene in the autism prefrontal cortex. Mol. Autism 6:46. doi: 10.1186/s13229-015-0040-1
Mundalil Vasu, M., Anitha, A., Thanseem, I., Suzuki, K., Yamada, K., Takahashi, T., et al. (2014). Serum microRNA profiles in children with autism. Mol. Autism 5:40. doi: 10.1186/2040-2392-5-40
Olde Loohuis, N. F., Kole, K., Glennon, J. C., Karel, P. G., Van der Borg, Van Gemert, Y., et al. (2015). Elevated microRNA-181c and microRNA-30d levels in the enlarged amygdala of the valproic acid rat model of autism. Neurobiol. Dis. 80, 42–53. doi: 10.1016/j.nbd.2015.05.006
Onaolapo, O. J., Paul, T. B., and Onaolapo, A. Y. (2017). Comparative effects of sertraline, haloperidol or olanzapine treatments on ketamine-induced changes in mouse behaviours. Metab. Brain Dis. 32, 1475–1489. doi: 10.1007/s11011-017-0031-3
Peng, L., Zhu, W., Liao, B., Duan, Y., Chen, M., Chen, Y., et al. (2017). Screening drug-target interactions with positive-unlabeled learning. Sci. Rep. 7:8087. doi: 10.1038/s41598-017-08079-7
Peng, L. H., Yin, J., Zhou, L., Liu, M. X., and Zhao, Y. (2018). Human microbe-disease association prediction based on adaptive boosting. Front. Microbiol. 9:2440. doi: 10.3389/fmicb.2018.02440
Rosina, E., Battan, B., Siracusano, M., Di Criscio, L., Hollis, F., Pacini, L., et al. (2019). Disruption of mTOR and MAPK pathways correlates with severity in idiopathic autism. Transl. Psychiatry 9:50. doi: 10.1038/s41398-018-0335-z
Rybak-Wolf, A., Stottmeister, C., Glazar, P., Jens, M., Pino, N., Giusti, S., et al. (2015). Circular RNAs in the mammalian brain are highly abundant, conserved, dynamically expressed. Mol. Cell 58, 870–885. doi: 10.1016/j.molcel.2015.03.027
Salzman, J., Chen, R. E., Olsen, M. N., Wang, P. L., and Brown, P. O. (2013). Cell-type specific features of circular RNA expression. PLoS Genet. 9:e1003777. doi: 10.1371/journal.pgen.1003777
Sekar, S., Cuyugan, L., Adkins, J., Geiger, P., and Liang, W. S. (2018). Circular RNA expression and regulatory network prediction in posterior cingulate astrocytes in elderly subjects. BMC Genomics 19:340. doi: 10.1186/s12864-018-4670-5
Suda, S., Iwata, K., Shimmura, C., Kameno, Y., Anitha, A., Thanseem, I., et al. (2011). Decreased expression of axon-guidance receptors in the anterior cingulate cortex in autism. Mol. Autism 2:14. doi: 10.1186/2040-2392-2-14
Sun, Y. M., Wang, W. T., Zeng, Z. C., Chen, T. Q., Han, C., Pan, Q., et al. (2019). circMYBL2, a circRNA from MYBL2, regulates FLT3 translation by recruiting PTBP1 to promote FLT3-ITD AML progression. Blood 134, 1533–1546. doi: 10.1182/blood.2019000802
Turner, T. N., Coe, B. P., Dickel, D. E., Hoekzema, K., Nelson, B. J., Zody, M. C., et al. (2017). Genomic patterns of de novo mutation in simplex autism. Cell 171, 710–722 e12. doi: 10.1016/j.cell.2017.08.047
Vahabzadeh, A., Keshav, N. U., Salisbury, J. P., and Sahin, N. T. (2018). Improvement of attention-deficit/hyperactivity disorder symptoms in school-aged children, adolescents, and young adults with autism via a digital smartglasses-based socioemotional coaching aid: short-term, uncontrolled pilot study. JMIR Ment. Health 5:e25. doi: 10.2196/mental.9631
Valiente-Palleja, A., Torrell, H., Muntane, G., Cortes, M. J., Martinez-Leal, R., Abasolo, N., et al. (2018). Genetic and clinical evidence of mitochondrial dysfunction in autism spectrum disorder and intellectual disability. Hum. Mol. Genet 27, 891–900. doi: 10.1093/hmg/ddy009
Vargas, D. L., Nascimbene, C., Krishnan, C., Zimmerman, A. W., and Pardo, C. A. (2005). Neuroglial activation and neuroinflammation in the brain of patients with autism. Ann. Neurol. 57, 67–81. doi: 10.1002/ana.20315
Yang, F., Li, A., Qin, Y., Che, H., Wang, Y., Lv, J., et al. (2019). A novel circular RNA mediates pyroptosis of diabetic cardiomyopathy by functioning as a competing endogenous RNA. Mol. Ther. Nucleic Acids 17, 636–643. doi: 10.1016/j.omtn.2019.06.026
Yi, Y., Liu, Y., Wu, W., Wu, K., and Zhang, W. (2019). Reconstruction and analysis of circRNAmiRNAmRNA network in the pathology of cervical cancer. Oncol. Rep. 41, 2209–2225. doi: 10.3892/or.2019.7028
You, X., Vlatkovic, I., Babic, A., Will, T., Epstein, I., Tushev, G., et al. (2015). Neural circular RNAs are derived from synaptic genes and regulated by development and plasticity. Nat. Neurosci. 18, 603–610. doi: 10.1038/nn.3975
Zhang, S., Zhu, D., Li, H., Li, H., Feng, C., and Zhang, W. (2017). Characterization of circRNA-associated-ceRNA networks in a senescence-accelerated mouse prone 8 brain. Mol. Ther. 25, 2053–2061. doi: 10.1016/j.ymthe.2017.06.009
Zheng, W., Hu, Y., Chen, D., Li, Y., and Wang, S. (2019). Improvement of a mouse model of valproic acid-induced autism. Nan Fang Yi Ke Da Xue Xue Bao 39, 718–723. doi: 10.12122/j.issn.1673-4254.2019.06.14
Keywords: autism, circular RNA (circRNA), RNA sequencing (RNA-Seq), ceRNA network, in silico analysis
Citation: Wang J, Yang Z, Chen C, Xu Y, Wang H, Liu B, Zhang W and Jiang Y (2021) Comprehensive circRNA Expression Profile and Construction of circRNAs-Related ceRNA Network in a Mouse Model of Autism. Front. Genet. 11:623584. doi: 10.3389/fgene.2020.623584
Received: 30 October 2020; Accepted: 23 December 2020;
Published: 16 February 2021.
Edited by:
Lihong Peng, Hunan University of Technology, ChinaReviewed by:
Feng Yang, Shenzhen Second People's Hospital, ChinaYanjuan Wang, Lianyungang Maternal and Children's Hospital, China
Copyright © 2021 Wang, Yang, Chen, Xu, Wang, Liu, Zhang and Jiang. This is an open-access article distributed under the terms of the Creative Commons Attribution License (CC BY). The use, distribution or reproduction in other forums is permitted, provided the original author(s) and the copyright owner(s) are credited and that the original publication in this journal is cited, in accordance with accepted academic practice. No use, distribution or reproduction is permitted which does not comply with these terms.
*Correspondence: Yanan Jiang, jiangyanan@hrbmu.edu.cn; Wei Zhang, zhangwei830530@163.com