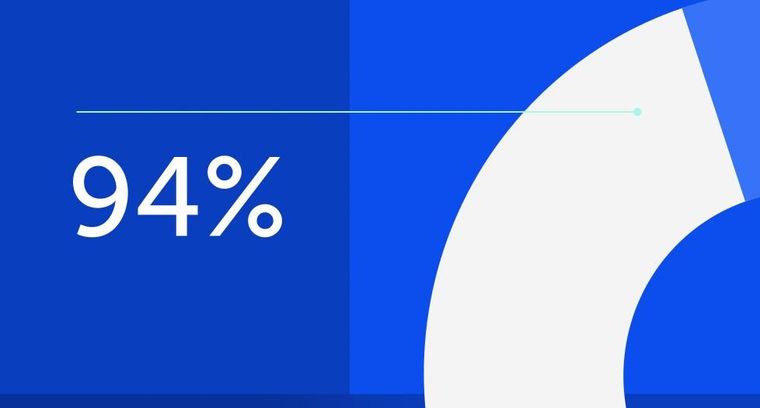
94% of researchers rate our articles as excellent or good
Learn more about the work of our research integrity team to safeguard the quality of each article we publish.
Find out more
ORIGINAL RESEARCH article
Front. Genet., 09 December 2020
Sec. Computational Genomics
Volume 11 - 2020 | https://doi.org/10.3389/fgene.2020.606940
This article is part of the Research TopicBioinformatics Analysis of Omics Data for Biomarker Identification in Clinical ResearchView all 45 articles
Background: Pancreatic cancer (PC) remains one of the most lethal cancers. In contrast to the steady increase in survival for most cancers, the 5-year survival remains low for PC patients.
Methods: We describe a new pipeline that can be used to identify prognostic molecular biomarkers by identifying miRNA-mediated subpathways associated with PC. These modules were then further extracted from a comprehensive miRNA-gene network (CMGN). An exhaustive survival analysis was performed to estimate the prognostic value of these modules.
Results: We identified 105 miRNA-mediated subpathways associated with PC. Two subpathways within the MAPK signaling and cell cycle pathways were found to be highly related to PC. Of the miRNA-mRNA modules extracted from CMGN, six modules showed good prognostic performance in both independent validated datasets.
Conclusions: Our study provides novel insight into the mechanisms of PC. We inferred that six miRNA-mRNA modules could serve as potential prognostic molecular biomarkers in PC based on the pipeline we proposed.
Pancreatic cancer (PC) is one of the most lethal digestive system tumors characterized by rapid onset, high malignancy and high mortality (Lei et al., 2017). In contrast to the steady increase in survival for most cancers, the 5-year survival of PC patients remains less than 5% (Siegel et al., 2017); however, there has been substantial progress in both diagnostic and therapeutic techniques. Prognosis for surgically treated patients is difficult, and detection of new biomarkers is urgently required in order to accurately predict PC patient outcome and better understand the associated molecular mechanisms.
MicroRNAs (miRNAs) are short, endogenous non-coding RNAs that participate in post-transcriptional gene regulation. Increasing research on PC has revealed that, miRNAs play an important role in the development of PC (Peng et al., 2015; Song et al., 2015; Imamura et al., 2017). Recent literature indicates miR-339-5p can suppress the invasion and migration of PC cells via direct regulation of ZNF689 (Yu et al., 2019). MiR-137-3p, as a direct target of circ-LDLRAD3k contributed to repressing the proliferation, migration and invasion of PC cells when circ-LDLRAD3 was downregulated (Yao et al., 2019). Although an increasing number of disease-relevant genes and miRNAs have been identified through microarray and next-generation sequencing, the precise functional mechanism that contributes to the pathology of this complex disease remains unclear.
Pathway analysis is the first choice to gain insight into biological processes and understand the underlying mechanisms of complex diseases. Several studies have shown that subpathways, rather than complete biological pathways, are abnormal in disease phenotypes (Li et al., 2009, 2013). As such, many methods have been developed to identify biological pathways or subpathways (Feng et al., 2016, 2019; Han et al., 2018, 2020; Liu et al., 2019; Ning et al., 2019; Yamaguchi et al., 2019; Li et al., 2020). For example, the Over-Representation Analysis (ORA) method (Breitling et al., 2004) identifies biological pathways by evaluating the extent to which the genes in a gene set of interest appear in given predefined pathway using a hypergeometric test. Another approach is topology enrichment analysis framework (TEAK) (Judeh et al., 2013). TEAK identifies linear and non-linear subpathways and scores them. Emerging evidence suggests that miRNAs play important roles in biological pathways, acting as regulators of pathway output or as important pathway targets (Ooi et al., 2011). Sidiropoulos et al. (2014) found Kallikrein-related peptidase 5 could induce miRNA-mediated anti-oncogenic pathways in breast cancer. Al-Ghezi et al. (2019) demonstrated that combination of Δ9-tetrahydrocannabinol and cannabidiol could induce attenuation of neuroinflammation through miRNA-mediated signaling pathway. A series of studies focused on identifying miRNA-mediated subpathways for deciphering disease mechanisms (Vrahatis et al., 2015, 2016; Ning et al., 2019). The Subpathway-Gmir (Li F. et al., 2015) method identifies miRNA-mediated metabolic subpathways relevant to various diseases by integrating genes of interest, miRNAs, and pathway topologies through building miRNA-regulated metabolic pathways. Vrahatis et al. (2015) developed an effective method for capturing miRNA-mediated signaling subpathways by integrating paired miRNA/mRNA expression data. They subsequently developed time-vaRying enriCHment integrOmics Subpathway aNalysis tOol (CHRONOS) by integrating time series mRNA/miRNA expression data with KEGG pathway maps and miRNA-target interactions (Vrahatis et al., 2016). These studies either focused on identifying miRNA-mediated pathways/subpathways associated with complex diseases, or focused on the impact of miRNA on diseases as regulators or targets of these pathways/subpathways analysis of these pathways/subpathways. However, few studies have analyzed relationships between patient survival and modules extracted from the special network constructed by miRNA-mediated subpathways associated with complex diseases such as PC.
In this study, we propose a novel pipeline that can be used to identify prognostic molecular biomarkers by identifying miRNA-mediated subpathways associated with complex diseases and further extracting modules from a comprehensive miRNA-gene network (CMGN). Briefly, 105 significant miRNA-mediated subpathways were found to be associated with PC. The CMGN was further constructed using these subpathways, and 10 miRNA-mRNA modules were extracted. Functional analyses revealed that the majority of these modules were enriched among cancer-related gene ontology (GO) terms. Finally, an evaluation of the association between survival and the level of gene and miRNA expression in the modules found that six out of the 10 miRNA-mRNA modules were able to discriminate between two groups of PC patients with different clinical outcomes. Together, we provide an effective pipeline for analyzing the relationships of patient survival and modules extracted from the special network constructed by miRNA-mediated subpathways associated with complex diseases. The findings of our study provide novel insight into the mechanisms of PC and identify six modules that may have prognostic significance for predicting the survival of PC patients.
The aim of this study was to identify significant subpathways associated with PC and evaluate the prognostic value of miRNA-gene modules in the CMGN constructed using these subpathways. Firstly, differentially expressed genes (DE genes) and miRNAs (DE miRNAs) in PC tissues were identified using microarray gene expression profile data of PC. These DE genes and DE miRNAs were subsequently mapped into undirected pathway graphs embedded by miRNA (UPEMs) to detect the miRNA-mediated subpathways associated with PC. The statistically significant subpathways were obtained and defined as PC-relevant subpathways. A comprehensive miRNA-gene network was built by merging common nodes and edges based on the PC-relevant subpathways. Finally, we detected functional modules in the network and further evaluated their value in relation to PC patient survival. An overview of the pipeline is presented in Figure 1.
The gene and miRNA expression profiles, including 14 solid-pseudopapillary neoplasms samples, six PC samples, six neuroendocrine tumor samples and five non-neoplastic pancreatic tissue samples, were initially analyzed by Park et al. (2014). We downloaded the expression profile data from the Gene Expression Omnibus (GEO) database (GSE43795 for miRNAs and GSE43796 for mRNAs) (Park et al., 2014). PC and non-neoplastic pancreatic tissue samples were used to identify DE genes and DE miRNAs associated with PC, using the significance analysis of microarrays (SAM) (Tusher et al., 2001) method. An FDR < 0.001 as used for genes and FDR < 0.05 for miRNAs. We identified 1,586 DE genes and 107 DE miRNAs. Additionally, two independent datasets were used to evaluate the prognostic power of the miRNA-mRNA modules. For independent validation dataset 1, a microarray dataset including mRNA expression profiles and clinical information with early stage PC (GSE57495) from 63 patients was downloaded from the GEO database (Edgar et al., 2002). For independent validation dataset 2, an RNA-seq dataset of mRNAs and miRNAs for primary PC tumors, was retrieved from the Cancer Genome Atlas (TCGA) database1. The same TCGA barcode structure was used for both clinical data and RNA-seq data. A total of 177 samples with clinical follow-up information were retained for further analysis.
Experimentally validated miRNA-target interaction data were collected from four databases: TarBase (version 6.0) (Vergoulis et al., 2012); miRTarBase (release 2014) (Hsu et al., 2014); mir2Disease (Jiang et al., 2009); and miRecords (release 2013) (Xiao et al., 2009). After removing redundancies, 57,846 pairs of human specific experimentally validated miRNA-target interactions (including 14,614 genes and 579 miRNAs) were obtained. Of these pairs, 12,425 (including 4,347 genes and 371 miRNAs) had been validated by low-throughput experiments.
Disease-associated coding genes were derived from OMIM (Amberger et al., 2011) and GAD (Becker et al., 2004). Disease-associated miRNAs were derived from the miRCancer (Xie et al., 2013), miR2Disease (Jiang et al., 2009), and HMDD (Li et al., 2014) databases. Genes and miRNAs of the significant miRNA-mediated subpathways associated with PC were considered as disease-associated coding genes and miRNAs according to the associations between them and PC recorded in at least one of these databases.
The UPEM was constructed based on KEGG pathways and the experimentally validated miRNA-target interaction data. In this study, 343 KEGG pathways involving 152 metabolic and 191 non-metabolic pathways were used. First, each pathway was converted into an undirected graph with the genes serving as nodes using our previously developed R package “iSubpathwayMiner” (Li et al., 2009). Second, we examined verified targets genes of each miRNA whether appeared in the converted pathway graphs. If its targets were contained in the pathway, we added the miRNA node into the pathway and linked it to their validated targets. Finally, we generated UPEMs that combined the miRNAs and miRNA-target gene interaction edges. We used the 343 pathways embedded by miRNAs to identify the PC risk subpathways.
The “lenient distance” similarity method (Li et al., 2013; Li F. et al., 2015) was used to identify the miRNA-mediated subpathways in the UPEMs according to coding genes and miRNAs as the input. Notably, the DE miRNAs and DE genes were mapped into UPEMs at the same time as the signature nodes. The shortest path between any two signature nodes in the mapped pathway was computed. Next, the two signature nodes and the molecules contained in the shortest path between them were divided into the same candidate node set, with the requirement that the length of the shortest path be less than or equal to n. The corresponding subgraph was extracted according to each candidate node, and set as a subpathway if the node number was no less than s. The parameters were set to n = 1 and s = 10. Statistically significant subpathways were further extracted by performing a hypergeometric test with a p-value < 0.001. The p-value was calculated according to the following equation:
where mg (mmir) represents the number of coding genes (miRNAs) in the entire human genome, of which tg (tmir) represents the number of miRNAs involved in a given subpathway, and ng (nmir) represents the number of DE genes (DE miRNAs), of which rg (rmir) represents the number of DE miRNAs involved in the same subpathway. The CMGN was constructed using all the genes/miRNAs extracted from the statistically significant subpathways and all inherited edges. Finally, for each miRNA in the network, a Pearson correlation between the level of miRNA expression and all genes in the network were computed, and the edges representing the strong negative correlation (r < −0.7) between the miRNA and its target genes were specially marked.
We extracted the modules from the miRNA-gene network using the Clique Percolation Method (CPM) implemented by CFinder (Adamcsek et al., 2006) software with a value of parameter k = 4 (k-clique size) for the richest modular structure. CFinder is a program that can rapidly locate and visualize overlapping, densely interconnected groups of nodes in undirected graphs. We interpreted a community constructed from adjacent cliques of the same size k in the CPM as a module. A k-clique represented a complete subgraph on k nodes, and two k-cliques were considered to be adjacent if they shared k−1 nodes. A community comprised a set of k-cliques in which all nodes could be reached via chains of adjacent k-cliques from each other.
Gene ontology analyses were performed using an R package clusterProfiler (Yu et al., 2012) for the coding genes of a given module. A p-value threshold of 0.01 was used to indicate statistical significance. The GO categories obtained from a previous study (Plaisier et al., 2012) were used as proxies for the characteristic hallmark traits of cancer.
A hypergeometric test was performed on each module to evaluate the extent to which the genes and miRNAs in the module overlapped with the nodes of statistically significant subpathways. The p-value was calculated according to the following equation:
where m is the total number of unique nodes in the subpathways located by simultaneously mapping DE miRNAs and DE genes into UPEMs as the signature nodes; t is the number of nodes in the chosen subpathway of interest; n is the number of nodes in a given module; r is the number of common nodes between the chosen subpathway and the given module. A p-value threshold of 0.001 was used to indicate statistical significance.
For the specific molecules (genes/miRNAs) in a given module, a univariate Cox regression analysis was carried out to evaluate the association between survival and the expression levels of molecules in the module. A risk score formula was used to evaluate the association between survival and molecule combinations in the given module and calculated using a linear combination of the expression levels of molecules weighted according to their respective Cox regression coefficients from the univariate Cox regression analysis as follows:
where bi is the Cox regression coefficient of molecule i from the given module, n is the number of molecules in the given module, and Exp(i) is the expression value of molecule i in the corresponding patient. Cancer patients were classified into high and low-risk groups according to the median risk score. For single gene survival prediction, the median expression value of each gene was used as a cut-off to distinguish two groups of PC patients as having either a low or high relative gene expression. A Kaplan–Meier survival analysis was performed for the two patient groups using the R survival package, and statistical significance was assessed using a two-tailed log-rank test. A p-value threshold of 0.05 was used to indicate significance.
Using the SAM method to test the PC and non-neoplastic pancreatic tissue samples, 1,586 DE genes and 107 DE miRNAs were identified at the FDR level of 0.001 and 0.05, respectively. After mapping these DE genes and DE miRNAs into UPEMs at the same time as signature nodes, 105 significant subpathways were identified at a strict cut-off value of FDR < 0.001 (see section “Materials and Methods”). These significant subpathways, referred to as PC-relevant subpathways, varied from 10 to 85 genes/miRNAs (mean: 24 genes/miRNAs), and were associated with 105 distinct complete pathways (Supplementary Table 1). The top 20 PC-relevant subpathways are listed in Table 1. The coverage rate of known cancer-associated genes and miRNAs were tested for each PC-relevant subpathway. These known cancer-associated genes and miRNAs were derived from the OMIM, GAD, miRCancer, miR2Disease, and HMDD databases. As a result, it was found that each PC-relevant subpathway was associated with an average of 36.7% known cancer-associated genes and miRNAs, some of which even reached 73.7% (Supplementary Table 2).
We further focused on the two most significant PC relevant subpathways. The first was path: 04010_1 (FDR = 0), an important sub-region within the MAPK signaling pathway (Figure 2A), which was previously reported to be a highly conserved pathway that transfers extracellular signals into cellular proliferation signals (Aguirre-Ghiso et al., 2003). The mitogen-activated protein kinase (MAPK) is one such complex interconnected signaling cascade with frequent involvement in oncogenesis, tumor progression and drug resistance. One study demonstrated the role of MAPK signaling during the initial steps of pancreatic carcinogenesis (Collins et al., 2014). Based on the topological structure, miR -320a was a DE miRNA with a higher degree in this subpathway. Moreover, the over-expression of miR-320a strongly contributes to PC pathogenesis, including the characteristics of increased proliferation, invasion, metastasis, drug-resistance and the epithelial-to-mesenchymal transition (Wang et al., 2016). In addition, several DE genes were enriched in this subpathway, among which MYC, NRAS, RAC2 were known PC related genes, and most of them play key roles in PC. The study by Adrian et al. suggested that individuals with constitutively decreased TGFBR1 expression may have a decreased risk of PC (Adrian et al., 2009). MAPK9 has also been identified as a potentially promising biomarker in exploratory studies of PC (Bracci et al., 2012). More importantly, SNPs in the inflammatory pathway genes MAPK8IP1 and SOCS3 were associated with increased overall survival in patients undergoing potentially curative resection and may be used as markers to predict PC patient survival (Reid-Lombardo et al., 2013).
Figure 2. MiRNA-mediated subpathways associated with PC. Circle, triangle nodes represented genes and miRNAs, respectively. DE genes and miRNAs are colored blue and purple, respectively, and non-DE genes and miRNAs are colored gray and yellow, respectively. The red border indicates known PC-associated genes/miRNAs. The edges represented a strong negative correlation (r < –0.7) between the miRNA and its target genes marked in green. (A) MAPK signaling subpathway (path: 04010_1, FDR = 0). (B) Cell cycle subpathway (path: 00561_1, FDR = 0).
The second significant subpathway was path: 04110_1 (FDR = 0), which is one of the most important cell cycle signaling pathways (Figure 2B) that regulates both cell division and apoptosis. DNA damage readily leads to dysregulation of the cell cycle, which is an essential step in the initiation and development of human cancer (Nan et al., 2016). The 04110_1 subpathway consisted of 64 genes/miRNAs, 15 of which were known PC-associated genes/miRNAs and 30 of which were DE genes/miRNAs. According to the topological structure of the pathway, miRNAs with a higher degree are more important in the subpathway region. It was found that miR-34a, a non-differential and disease-associated miRNA, exhibited the highest degree. Moreover, this miRNA has been reported to serve as a diagnostic biomarker for PC (Long et al., 2017). Hu et al. (2013) proposed the use of miR-34a-delivering therapeutic nanocomplexes as an effective treatment for PC. Notably, nine target genes of miR-34a were identified in this subpathway, including TP53, MYC, MCM2, MCM4, MCM6, CDC20, SFN, CCNE2, and E2F3. Four out of the nine target genes (TP53, MYC, MCM2, and MCM4) were known PC-associated genes, and eight were differentially expressed. Among these differentially expressed target genes, cell division cycle 20 (CDC20), an anaphase-promoting complex activator, has been observed to be over-expressed in a variety of human cancers and to play an oncogenic role in tumorigenesis (Wang et al., 2015). E2F3, as the target gene regulated by miR-217, has been shown to be involved in PC cell growth, invasion and apoptosis (Yang et al., 2017). In addition, miR-215 exhibited the second highest degree and is dysregulated in several human malignancies, including PC. It is speculated that miR-215 may act as a tumor suppressor in PC, and could serve as a novel therapeutic target for miRNA-based therapy (Li Q.W. et al., 2015). Interestingly, we found that a miRNA set consisting of miR-92a, miR-24, let-7a, let-7b, let-7c, miR-193b, and miR-31 was closely correlated with PC. Each of these miRNAs regulates at least one differentially expressed cell cycle-related gene, involving cyclin B1 (CCNB1), cyclin B2 (CCNB2), and cyclin-dependent kinase 1 (CDK1). Among them, let-7b, let-7c, and miR-31 are known PC-associated miRNAs, and others have also been associated with PC in previous studies (Listing et al., 2015; Xiao et al., 2017). Our results demonstrate that the PC-relevant subpathways identified by integrating DE genes, DE miRNAs and pathway topologies were closely related to PC.
Since key genes and miRNAs might participate in multiple subpathways, the CMGN was constructed by merging common nodes and edges of PC-relevant subpathways (see section “Materials and Methods”). In the network, there were 3,030 edges between 91 miRNA nodes and 640 gene nodes, of which 48 edges had a strong negative correlation between the level of miRNA and target gene expression (r < −0.7) (Figure 3A). We evaluated the degree of node distribution in the network and observed a power-law and exponential distribution, respectively (Figure 3B). Therefore, the CMGN displayed scale-free characteristics, indicating that it was not random but organized according to a core set of principles in its structure that distinguished it from randomly linked networks (Barabasi et al., 2011).
Figure 3. The view and topological features of the CMGN. (A) The view of the CMGN. The CMGN consisted of 640 genes and 91 miRNAs with 3,030 edges between them. Of these, 48 edges represented a strong negative correlation (r < –0.7) between the miRNA and its target genes marked in green. Disease-associated genes/miRNAs in the CMGN exhibited specific topological characteristics compared to the other nodes. (B) The network revealed a power law distribution with a slope of –1.343 and R2 = 0.816. The X-axis indicates the degree of node distribution. The Y-axis indicates the number of nodes according to the X-axis. (C) and (D) Disease-associated nodes had a higher degree and betweenness centrality than the other nodes. Data are shown as the mean ± SEM. Disease-associated and other nodes are indicated in red and blue along the X-axis. The average degrees of the two groups of nodes are indicated by the Y-axis.
The known PC-associated miRNAs and genes were further mapped to the network, and 93 PC-associated molecules (39 known PC-associated miRNAs and 54 known PC-associated genes) were found to be involved in the network. The topological characteristics of the network were examined, revealing that these known PC-associated miRNAs and genes showed a significantly higher degree and betweenness centrality than the other miRNAs and genes (Figures 3C,D). The specific topological patterns reflected the functional importance of the known PC-associated miRNAs and genes in the CMGN. A higher degree indicated that the nodes were likely to be hubs and had high probabilities of engaging in essentially biological functions. A higher betweenness centrality implied that they acted as bridges connecting different network components and controlling communication.
All of the nodes’ topological features of the network were ranked and the top 10 genes and miRNAs of each dimension are listed in Table 2. It was observed that all top 10 genes were differentially expressed, three of which (NRAS, MYC, and EGFR) have been well-described as known PC-associated genes. MAPK3 (also known as ERK1/2) is a member of the MAP kinase family that exhibited the highest degree, and appeared in 55 PC-relevant subpathways. MAPK3 is activated by upstream kinases, which results in its translocation to the nucleus where it phosphorylates nuclear targets. Moreover, MAPK3 plays an important role in the MAPK/ERK cascade. Indeed, MAPK3 inhibitors can inhibit the growth of PC cells through the RAS-RAF-MEK-ERK pathway (Walters et al., 2013). MAPK9 exhibited the second highest degree and was inversely correlated with the expression of seven miRNAs in our study, including four known PC-associated miRNAs (miR-93, miR-20a, miR-17, and miR-320a). MAPK9 has previously been identified as a potentially promising biomarker in exploratory studies, and was observed to be overexpressed in PC patients (300 cases, 300 controls) (Bracci et al., 2012). In addition, miR-320a had the highest degree among miRNAs and appeared in 92 PC-relevant subpathways. The study by Wang et al. (2016) found that miR-320a over-expression promoted PC cell proliferation, migration and invasion, and demonstrated that miR-320a suppressed PDCD4 mRNA expression in 5-Fluorouracil-resistant human PC cells. These findings suggest that miR-320a may serve as a potential target for PC therapy (Wang et al., 2016). When applied to clinical serum samples, miR-320a could accurately predict late chronic pancreatitis (Xin et al., 2017). Together, these results demonstrate that the CMGN based on PC-relevant subpathways can provide insight into cancer-associated transcriptional regulatory networks. Thus, the CMGN may be able to identify key factors that participate in multiple pathways.
Analysis of the CMGN can provide a global view of the regulatory relationships involved in PC-relevant subpathways. To reveal the detailed modular organization of the CMGN, the functional modules in the network were mined using the Clique Percolation Method implemented by CFinder software with a value of parameter k = 4 (see section “Materials and Methods”). A total of 30 modules were detected, among which greater attention was paid to the miRNA-mRNA modules. There were 12 modules that contained at least one miRNA and mRNA, two of which were absolutely included in the other modules. Therefore, the larger modules were retained. Ultimately, 10 non-redundant miRNA-mRNA modules were obtained, consisting of 4 ∼ 23 genes/miRNAs (Figure 4A).
Figure 4. Modular analysis of the CMGN. (A) A total of 10 miRNA-mRNA modules were derived from the CMGN, ranging between 4 and 23 nodes, with an average of 8.1 nodes per module. The modules are encircled with an ochre dotted oval. The circle and triangle nodes represent genes and miRNAs, respectively. DE genes and miRNAs are colored blue and purple, and non-DE genes and miRNAs are colored gray and yellow, respectively. Disease associated genes/miRNAs are indicated with red circles. (B) GO terms enriched for modules M5, M8, and M10.
For each miRNA-mRNA module, a GO analysis of the coding genes from a given module was carried out based on the GO terms (see section “Materials and Methods”), and each module was annotated with the enriched functions of the corresponding gene set (Supplementary Figure 1). Processes for the maintenance of cell homeostasis (e.g., cell cycle regulation and cytosolic calcium ion homeostasis) and cancer development-related processes (e.g., cell-matrix adhesion and JAK-STAT cascade and glycolysis) were highly represented. Cancer is a complex disease characterized by select hallmarks of cancer, including resistance to cell death, tissue invasion and metastasis, as well as the induction of angiogenesis (Hanahan and Weinberg, 2011). These hallmarks provide a framework for understanding the remarkable diversity of various cancers. Thus, through comparing the GO categories used as proxies for hallmark cancer traits (see section “Materials and Methods”), we found that modules M1, M5, M8, and M10 were involved in five hallmark traits, including positive regulation of signal transduction, cell adhesion, glycolysis and positive regulation of cell growth. Module M5 was enriched in cell-matrix adhesion and mesodermal cell differentiation. Module M8 was enriched in canonical glycolysis and glycolytic processes. Module M9 was enriched in cell proliferation related processes (Figure 4B).
We tested the extent to which the molecules overlap between each miRNA-mRNA module and PC-relevant subpathways using a hypergeometric test with a p-value < 0.0001 and annotation proportion ≥ 70% (see section “Materials and Methods”). As a result, nine out of 10 miRNA-mRNA modules were annotated in at least one PC-relevant subpathway (Supplementary Table 3). We found that the modules, that overlapped with other modules, were enriched in more PC-relevant subpathways, indicating that these modules may have multiple functions. For instance, module M5, which overlapped with M3, M4, and M6, was enriched in six PC-relevant subpathways: (1) path: 04510_1 that belonged to focal adhesion; (2) path: 05410_1 that belonged to hypertrophic cardiomyopathy; (3) path: 05414_1 that belonged to Dilated cardiomyopathy; (4) path: 05412_1 that belonged to arrhythmogenic right ventricular cardiomyopathy; (5) path: 04810_1 that belonged to regulation of actin cytoskeleton; and (6) path: 04520_1 that belonged to adherens junction. Interestingly, three of these subpathways enriched by module M5 were associated with cardiomyopathy. To our knowledge, advanced cancer can induce fundamental changes in metabolism and promote cardiac atrophy and heart failure. Thackeray et al. (2017) discovered systemic insulin deficiency in cachectic cancer patients and demonstrated that cancer-induced systemic insulin depletion contributes to cardiac wasting and failure. In addition, low-dose insulin supplementation was found to attenuate these processes in mice with advanced melanoma or colon carcinoma.
Module M9 contained four molecules (ERBB2, EGFR, PDGFD, and miR-21), three of which are known PC-associated genes and miRNAs. Studies have shown that increasing EGFR activity can over-activate downstream pro-oncogenic signaling pathways (e.g., RAS-RAF-MEK-ERK MAPK, and AKT-PI3K-mTOR pathways), which can then activate many biological outputs that may contribute to cancer cell proliferation (Wee and Wang, 2017). EGFR was also identified as a hub in the CMGN, indicating that EGFR is able to crosstalk with other molecules. Studies have demonstrated that a shorter EGFR intron 1 CA repeat length is associated with a worse PC clinical prognosis (Tzeng et al., 2007). ERBB2, also known as HER2, was functionally characterized by an extraordinarily strong catalytic kinase activity, and represents a key oncoprotein that can trigger cornerstone intracellular signaling events for cell growth and survival, further leading to increased signal transduction and activation of the MAPK and PI3K/Akt pathways (Wong and Hurvitz, 2014). Moreover, Cheng et al. (2017) identified the ERBB2 exon17 mutation as an independent factor associated with overall survival among metastatic PC patients. Dillhoff et al. (2008) demonstrated that miR-21 was significantly over-expressed in PC. Although we could not find any direct evidence to support an important role of PDGFD in PC, we found that PDGFD could regulate many cellular processes, including cell proliferation, apoptosis, transformation, migration, invasion, angiogenesis and metastasis (Wang et al., 2010). Recent studies (Walters et al., 2013; Ogawa et al., 2015) have also shown that PDGFD is closely related to various cancers.
To assess the prognostic performance of these miRNA-mRNA modules, a survival analysis was performed on each of the 10 miRNA-mRNA modules. After testing all the coding genes of the given module with the risk score model (see section “Materials and Methods”), eight out of 10 modules were found to be significantly related to the prognosis in independent validation dataset 1 (Figure 5A). In each of the eight modules, the patients in independent validation dataset 1 were divided into either a high-risk score or low-risk score group. Patients in the high-risk-score group had a significantly shorter overall survival than those in the low-risk score group. To test whether the whole miRNA-mRNA module showed better prognostic performance than a single coding gene, we calculated the Kaplan–Meier p-value of every single coding gene of the modules in independent validation dataset 1. Five out of eight modules (module M1, M3, M5, M7, and M9) showed better prognostic performance than any single coding gene in the module (Figure 5B). More interesting, each coding gene of module M3, M7, and M9 was almost non-significantly related to prognosis; however, the three modules were all significantly related to the prognosis in independent validation dataset 1. For example, each coding gene of module M3 showed bad prognostic performance according to non-significant Kaplan–Meier p-value. But the whole miRNA-mRNA module of M3 showed better prognostic performance. Another survival analysis was also carried out on each of the 28 non-redundant modules to test whether their expression levels were associated with PC prognosis. A total of 17 out of 28 non-redundant modules were related to the prognosis of the PC patients based on the level of gene expression. Furthermore, 13 out of 17 modules showed better prognostic performance with the whole module than any individual coding gene (Supplementary Table 4). These findings indicate that the miRNA-mRNA modules in the CMGN provide clinical guidance for cancer prognosis.
Figure 5. Survival analyses of PC patients for miRNA-mRNA modules. (A) Kaplan–Meier survival analyses of all patients with clinical follow-up information based on the 10 miRNA-mRNA modules. Survival months are shown along the X-axis. The overall survival rates are shown along the Y-axis. A p-value threshold of 0.05 was used. (B) The miRNA-mRNA module as a whole could better distinguish the two groups of patients compared to any individual coding gene within it, especially modules M3, M7, and M9. The whole module and associated coding genes are presented along the X-axis. The log value (base 10) of the overall survival rates are shown along the Y-axis.
To test the reproducibility of the 10 miRNA-mRNA modules’ prognostic performance, all coding genes of the given module in independent validation dataset 2 were tested using the same model and criteria as validation dataset 1. Two modules (modules M1 and M4) were excluded due to a matching of the genes to the mRNA expression profiles of less than 60% (Supplementary Figure 2). Seven out of eight modules (M2, M3, M5, M6, M8, M9, and M10) were related with the prognosis of the PC patients. Six out of seven modules (M3, M5, M6, M8, M9, and M10) showed good prognostic performance in both validation datasets 1 and 2, indicating good reproducibility. To test whether the combination of the miRNAs and mRNAs could predict the survival of PC patients, the relationship of the PC patients’ outcome and 10 miRNA-mRNA modules were further evaluated both coding genes and miRNAs using based on the same model and criteria. We observed a smaller Kaplan–Meier p-value based on the level of coding gene and miRNA expression levels compared to only the level of coding gene expression (Supplementary Figure 3). These findings indicate that the majority of miRNA-mRNA modules exhibit good reproducibility, and the involvement of miRNA expression levels may improve the prognostic performance of these modules for PC patients. Furthermore, the six good reproducible modules could serve as potential prognostic molecular biomarkers in PC.
Since the module consisted of connected genes and miRNAs in the network, there may be redundant genes/miRNAs in the module for predicting the survival of PC patients. To select the best prognostic signature, we compared the performances of all the gene combinations in each good reproducible module. A survival analysis was subsequently performed on every combination of genes in each good reproducible module using the same model as independent validation dataset 1. Considering the Kaplan–Meier p-value of all combinations in each module, one of these selected combinations was defined as the best signature. The most significant molecule combinations of the six good reproducible modules are listed in the Supplementary Tables 5–10. As expected, four out of six good reproducible modules (modules M3, M5, M9, and M10) exhibited the best signature, which could be represented by fewer molecules, for predicting the PC patients’ survival. For example, module M5 could be used to separate PC patients into high and low-risk groups using all genes and miRNAs to a greater extent than any single coding gene. In addition, a combination of two genes (ITGB4 and VCL) was associated with a better prognostic performance. Studies have shown that high levels of ITGB4 expression are significantly correlated with the hallmarks of epithelial-mesenchymal transition, high tumor grade, and the presence of lymph node metastasis, and also exhibit an independent prognostic effect (Masugi et al., 2015). Moreover, VCL has been identified as a potential novel oncogene in pancreatic adenocarcinoma (Loukopoulos et al., 2007). Taken together, four modules (modules M3, M5, M9, and M10) among the six good reproducible modules were found to play an important role for the prognosis of PC patients.
The dysregulation of miRNA expression has been widely observed in the development and progression of complex human diseases, such as cancer. In this study, DE genes and miRNAs were mapped into undirected pathway graphs embedded by miRNA as signature nodes. We obtained 105 significantly miRNA-mediated subpathways associated with PC as PC-related subpathways using a hypergeometric test. The PC-related subpathways provide biological insight for dissecting PC pathology. The key genes or miRNAs may participate in multiple pathways. Therefore, a comprehensive miRNA-gene network was built by merging common nodes and edges. Next, the topological characteristics of the network were analyzed and functional modules were detected. Finally, the functions of modules containing miRNAs were analyzed and their effect on the outcome of PC patients was evaluated. As a result, 105 subpathways were identified to be significantly associated with PC, in which more than a third of the nodes were found to be known cancer-associated genes and miRNAs. We focused on two PC-relevant subpathways belonging to the MAPK signaling pathway and cell cycle. The network analysis of the CMGN built by the PC-related subpathways revealed that the known PC-associated genes/miRNAs showed a significantly higher degree and betweenness centrality than other the nodes, indicating their functional importance. A total of 30 modules were detected in the CMGN using CFinder software, of which 10 miRNA-mRNA modules were further examined. A GO enrichment analysis revealed that the miRNA-mRNA modules were highly enriched in processes involved in the maintenance of cell homeostasis and cancer development. The survival analysis was performed on 10 miRNA-mRNA modules in two independent validation datasets. Of these, six of the modules showed good prognostic performance in both validation dataset 1 and 2. In particular, while each coding gene of modules M3, M7, and M9 was not significantly related with prognosis, the three modules were all significantly related to prognosis in independent validation dataset 1. These results revealed that the six good reproducible modules could serve as potential prognostic molecular biomarkers in PC.
The findings of our study provide a novel pipeline that can be used to identify prognostic molecular biomarkers from a comprehensive miRNA-gene network based on the identified miRNA-mediated subpathways associated with PC. However, the regulation between miRNA and mRNA is complex. The expression of miRNAs or mRNAs might be affected by other ncRNAs, including circRNAs and lncRNAs. For example, Zhao et al. (2017) demonstrated that lncRNA TUG1 could competitively sponge miR-382, thereby regulating EZH2. Their experiments further revealed that TUG1 overexpression promoted cellular proliferation and migration, and contributed to epithelial-mesenchymal transition (EMT) formation in PC cell lines (Zhao et al., 2017). Similar studies have demonstrated that lncRNA H19 could partially promote PC cell invasion and migration by increasing HMGA2-mediated EMT through antagonizing let-7 in PC cell lines (Ma et al., 2014). Hsa_circ_0005785 was down-regulated in 20 sets of PC tissues and was inferred to interact with miR-181a and miR-181b based on the sequence analysis. MiR-181a plays an important role in regulating PC cell growth and migration and miR-181b has been shown to be related to PC cell resistance to gemcitabine (Li et al., 2016). Identification of the upstream regulatory pathways of miRNAs contributes to research into the functional of miRNAs in various diseases. Information about the regulatory elements involved in the regulation of miRNA transcription is stored in recently published databases, including ENdb (Bai et al., 2020) and SEdb (Jiang et al., 2019). Moreover, the information associated with these regulatory elements (Qian et al., 2019) can be integrated to construct a miRNA transcription regulatory network for a specific disease. Finally, the pipeline we proposed can work flexibly in practices. It supports other methods for identifying differentially expressed subpathways in complex diseases. Similarly, it also supports other methods for mining modules in the network formed by identified subpathways. In parallel, the pipeline is suitable for analyzing integrated networks and can be applied to other complex diseases.
The datasets generated for this study can be found in online repositories. The names of the repository/repositories and accession number(s) can be found in the article/Supplementary Material.
YL analyzed data and wrote the manuscript. YL and YC collected the datasets. XH, YJ, and ML participated in the pre-processing of the datasets. XB, CF, ML, and JnZ performed the computational analysis. YC, JgZ, JH, BA, XL, JH, QP, FW, and MX performed the literature validation. CL and QW conceived the idea for the manuscript, provided guidance and critically revised the manuscript. All authors read and approved the final version to be published.
This work was supported by National Natural Science Foundation of China (Grant Nos. 61601150 and 81572341); The Fundamental Research Funds for the Provincial Universities (JFXN202004); Wu Liande Youth Science Research Fund of Harbin Medical University (Daqing) (Grant No. DQWLD201703); Natural Science Foundation of Heilongjiang Province (YQ2019C013); and Postdoctoral Researchers in Heilongjiang Scientific Research Grants. YuWeihan Outstanding Youth Training Fund of Harbin Medical University. Funding for open access charge: National Natural Science Foundation of China (61601150).
The authors declare that the research was conducted in the absence of any commercial or financial relationships that could be construed as a potential conflict of interest.
The Supplementary Material for this article can be found online at: https://www.frontiersin.org/articles/10.3389/fgene.2020.606940/full#supplementary-material
Supplementary Figure 1 | The enriched GO terms for miRNA-mRNA modules.
Supplementary Figure 2 | Survival analysis for miRNA-mRNA modules based on genes.
Supplementary Figure 3 | Survival analyses for miRNA-mRNA modules based on miRNAs and genes.
Supplementary Table 1 | PC-relevant subpathway list.
Supplementary Table 2 | Known cancer-associated genes and miRNAs inPC-relevant subpathways.
Supplementary Table 3 | Enrichment for modules on PC-relevant subpathways.
Supplementary Table 4 | Survival analysis for modules and individual genes.
Supplementary Table 5 | An exhaustive survival analysis for module M3.
Supplementary Table 6 | An exhaustive survival analysis for module M5.
Supplementary Table 7 | An exhaustive survival analysis for module M6.
Supplementary Table 8 | An exhaustive survival analysis for module M8.
Supplementary Table 9 | An exhaustive survival analysis for module M9.
Supplementary Table 10 | An exhaustive survival analysis for module M10.
Adamcsek, B., Palla, G., Farkas, I. J., Derenyi, I., and Vicsek, T. (2006). CFinder: locating cliques and overlapping modules in biological networks. Bioinformatics 22, 1021–1023. doi: 10.1093/bioinformatics/btl039
Adrian, K., Strouch, M. J., Z0eng, Q., Barron, M. R., Cheon, E. C., Honasoge, A., et al. (2009). Tgfbr1 haploinsufficiency inhibits the development of murine mutant Kras-induced pancreatic precancer. Cancer Res. 69:9169. doi: 10.1158/0008-5472.can-09-1705
Aguirre-Ghiso, J. A., Estrada, Y., Liu, D., and Ossowski, L. (2003). ERK(MAPK) activity as a determinant of tumor growth and dormancy; regulation by p38(SAPK). Cancer Res. 63, 1684–1695.
Al-Ghezi, Z. Z., Miranda, K., Nagarkatti, M., and Nagarkatti, P. S. (2019). Combination of Cannabinoids, Delta9- Tetrahydrocannabinol and Cannabidiol, ameliorates experimental multiple sclerosis by suppressing neuroinflammation through regulation of miRNA-mediated signaling pathways. Front. Immunol. 10:1921. doi: 10.3389/fimmu.2019.01921
Amberger, J., Bocchini, C., and Hamosh, A. (2011). A new face and new challenges for online mendelian inheritance in man (OMIM®). Hum. Mutat. 32, 564–567. doi: 10.1002/humu.21466
Bai, X., Shi, S., Ai, B., Jiang, Y., Liu, Y., Han, X., et al. (2020). ENdb: a manually curated database of experimentally supported enhancers for human and mouse. Nucleic Acids Res. 48, D51–D57. doi: 10.1093/nar/gkz973
Barabasi, A. L., Gulbahce, N., and Loscalzo, J. (2011). Network medicine: a network-based approach to human disease. Nat. Rev. Genet. 12, 56–68. doi: 10.1038/nrg2918
Becker, K. G., Barnes, K. C., Bright, T. J., and Wang, S. A. (2004). The genetic association database. Nat. Genet. 36, 431–432. doi: 10.1007/978-3-319-20883-1_17
Bracci, P. M., Mi, Z., Young, S., and Wiemels, J. (2012). Serum autoantibodies to pancreatic cancer antigens as biomarkers of pancreas cancer in a San Francisco Bay Area case-control study. Cancer 118, 5384–5394. doi: 10.1002/cncr.27538
Breitling, R., Amtmann, A., and Herzyk, P. (2004). Iterative Group Analysis (iGA): a simple tool to enhance sensitivity and facilitate interpretation of microarray experiments. BMC Bioinform. 5:34. doi: 10.1186/1471-2105-5-34
Cheng, H., Liu, C., Jiang, J., Luo, G., Lu, Y., Jin, K., et al. (2017). Analysis of ctDNA to predict prognosis and monitor treatment responses in metastatic pancreatic cancer patients. Intern. J. Cancer 140:2344. doi: 10.1002/ijc.30650
Collins, M. A., Yan, W., Seboltleopold, J. S., and Pasca, D. M. M. (2014). MAPK signaling is required for dedifferentiation of acinar cells and development of pancreatic intraepithelial neoplasia in mice. Gastroenterology 146, 822–834.e7.
Dillhoff, M., Liu, J., Frankel, W., Croce, C., and Bloomston, M. (2008). MicroRNA-21 is overexpressed in pancreatic cancer and a potential predictor of survival. J. Gastrointest. Surg. 12, 2171–2176. doi: 10.1007/s11605-008-0584-x
Edgar, R., Domrachev, M., and Lash, A. E. (2002). Gene expression omnibus: NCBI gene expression and hybridization array data repository. Nucleic Acids Res. 30, 207–210. doi: 10.1093/nar/30.1.207
Feng, C., Song, C., Ning, Z., Ai, B., Wang, Q., Xu, Y., et al. (2019). ce-Subpathway: identification of ceRNA-mediated subpathways via joint power of ceRNAs and pathway topologies. J. Cell Mol. Med. 23, 967–984. doi: 10.1111/jcmm.13997
Feng, C., Zhang, J., Li, X., Ai, B., Han, J., Wang, Q., et al. (2016). Subpathway-CorSP: identification of metabolic subpathways via integrating expression correlations and topological features between metabolites and genes of interest within pathways. Sci. Rep. 6:33262. doi: 10.1038/srep33262
Han, J., Han, X., Kong, Q., and Cheng, L. (2020). psSubpathway: a software package for flexible identification of phenotype-specific subpathways in cancer progression. Bioinformatics 36, 2303–2305. doi: 10.1093/bioinformatics/btz894
Han, J., Liu, S., Jiang, Y., Xu, C., Zheng, B., Jiang, M., et al. (2018). Inference of patient-specific subpathway activities reveals a functional signature associated with the prognosis of patients with breast cancer. J. Cell Mol. Med. 22, 4304–4316. doi: 10.1111/jcmm.13720
Hanahan, D., and Weinberg, R. A. (2011). Hallmarks of cancer: the next generation. Cell 144, 646–674. doi: 10.1016/j.cell.2011.02.013
Hsu, S. D., Tseng, Y. T., Shrestha, S., Lin, Y. L., Khaleel, A., Chou, C. H., et al. (2014). miRTarBase update 2014: an information resource for experimentally validated miRNA-target interactions. Nucleic Acids Res. 42, D78–D85. doi: 10.1093/nar/gkt1266
Hu, Q. L., Jiang, Q. Y., Jin, X., Shen, J., Wang, K., and Li, Y. B. (2013). Cationic microRNA-delivering nanovectors with bifunctional peptides for efficient treatment of PANC-1 xenograft model. Biomaterials 34, 2265–2276. doi: 10.1016/j.biomaterials.2012.12.016
Imamura, T., Komatsu, S., Ichikawa, D., Miyamae, M., Okajima, W., Ohashi, T., et al. (2017). Depleted tumor suppressor miR-107 in plasma relates to tumor progression and is a novel therapeutic target in pancreatic cancer. Sci. Rep. 7:e120. doi: 10.1016/j.jamcollsurg.2017.07.848
Jiang, Q., Wang, Y., Hao, Y., Juan, L., Teng, M., Zhang, X., et al. (2009). miR2Disease: a manually curated database for microRNA deregulation in human disease. Nucleic Acids Res. 37, D98–D104.
Jiang, Y., Qian, F., Bai, X., Liu, Y., Wang, Q., Ai, B., et al. (2019). SEdb: a comprehensive human super-enhancer database. Nucleic Acids Res. 47, D235–D243. doi: 10.1093/nar/gky1025
Judeh, T., Johnson, C., Kumar, A., and Zhu, D. (2013). TEAK: topology enrichment analysis framework for detecting activated biological subpathways. Nucleic Acids Res. 41, 1425–1437. doi: 10.1093/nar/gks1299
Lei, X. F., Jia, S. Z., Ye, J., Qiao, Y. L., Zhao, G. M., Li, X. H., et al. (2017). Application values of detection of serum CA199, CA242 and CA50 in the diagnosis of pancreatic cancer. J. Biolo. Regul. Homeostat. Agents 31:383.
Li, C., Han, J., Yao, Q., Zou, C., Xu, Y., Zhang, C., et al. (2013). Subpathway-GM: identification of metabolic subpathways via joint power of interesting genes and metabolites and their topologies within pathways. Nucleic Acids Res. 41:e101. doi: 10.1093/nar/gkt161
Li, C., Li, X., Miao, Y., Wang, Q., Jiang, W., Xu, C., et al. (2009). SubpathwayMiner: a software package for flexible identification of pathways. Nucleic Acids Res. 37:e131. doi: 10.1093/nar/gkp667
Li, F., Xu, Y., Zhang, Y., Sun, Z., Han, J., Zhang, C., et al. (2015). Subpathway-GMir: identifying miRNA-mediated metabolic subpathways by integrating condition-specific genes, microRNAs, and pathway topologies. Oncotarget 6, 39151–39164. doi: 10.18632/oncotarget.5341
Li, Q. W., Zhou, T., Wang, F., Jiang, M., Liu, C. B., Zhang, K. R., et al. (2015). MicroRNA-215 functions as a tumor suppressor and directly targets ZEB2 in human pancreatic cancer. Genet. Mole. Res. 14:16133. doi: 10.4238/2015.december.8.2
Li, H., Hao, X., Wang, H., Liu, Z., He, Y., Pu, M., et al. (2016). Circular RNA expression profile of pancreatic ductal adenocarcinoma revealed by microarray. Cell. Physiol. 40:1334. doi: 10.1159/000453186
Li, M., Zhao, J., Li, X., Chen, Y., Feng, C., Qian, F., et al. (2020). HiFreSP: A novel high-frequency sub-pathway mining approach to identify robust prognostic gene signatures. Brief Bioinform. 21, 1411–1424. doi: 10.1093/bib/bbz078
Li, Y., Qiu, C., Tu, J., Geng, B., Yang, J., Jiang, T., et al. (2014). HMDD v2.0: a database for experimentally supported human microRNA and disease associations. Nucleic Acids Res. 42, D1070–D1074.
Listing, H., Mardin, W. A., Wohlfromm, S., Mees, S. T., and Haier, J. (2015). MiR-23a/-24-induced gene silencing results in mesothelial cell integration of pancreatic cancer. Br. J. Cancer 112, 131–139. doi: 10.1038/bjc.2014.587
Liu, S., Zheng, B., Sheng, Y., Kong, Q., Jiang, Y., Yang, Y., et al. (2019). Identification of cancer dysfunctional subpathways by integrating DNA methylation, copy number variation, and gene-expression data. Front. Genet. 10:441. doi: 10.3389/fgene.2019.00441
Long, L. M., Zhan, J. K., Wang, H. Q., Li, S., Chen, Y. Y., and Liu, Y. S. (2017). The clinical significance of miR-34a in pancreatic ductal carcinoma and associated molecular and cellular mechanisms. Pathobiology 84, 38–48. doi: 10.1159/000447302
Loukopoulos, P., Shibata, T., Katoh, H., Kokubu, A., Sakamoto, M., Yamazaki, K., et al. (2007). Genome-wide array-based comparative genomic hybridization analysis of pancreatic adenocarcinoma: identification of genetic indicators that predict patient outcome. Cancer Sci. 98, 392–400. doi: 10.1111/j.1349-7006.2007.00395.x
Ma, C., Nong, K., Zhu, H., Wang, W., Huang, X., Yuan, Z., et al. (2014). H19 promotes pancreatic cancer metastasis by derepressing let-7’s suppression on its target HMGA2-mediated EMT. Tumour Biol. 35, 9163–9169. doi: 10.1007/s13277-014-2185-5
Masugi, Y., Yamazaki, K., Emoto, K., Effendi, K., Tsujikawa, H., Kitago, M., et al. (2015). Upregulation of integrin β4 promotes epithelial-mesenchymal transition and is a novel prognostic marker in pancreatic ductal adenocarcinoma. Lab. Invest. 95, 308–319. doi: 10.1038/labinvest.2014.166
Nan, Y. L., Hu, Y. L., Liu, Z. K., Duan, F. F., Xu, Y., Li, S., et al. (2016). Relationships between cell cycle pathway gene polymorphisms and risk of hepatocellular carcinoma. World J. Gastroenterol. 22, 5558–5567. doi: 10.3748/wjg.v22.i24.5558
Ning, Z., Feng, C., Song, C., Liu, W., Shang, D., Li, M., et al. (2019). Topologically inferring active miRNA-mediated subpathways toward precise cancer classification by directed random walk. Mol. Oncol. 13, 2211–2226. doi: 10.1002/1878-0261.12563
Ogawa, N., Inokuchi, M., Takagi, Y., Sugita, H., Kato, K., Kojima, K., et al. (2015). Clinical significance of platelet derived growth factor-C and -D in gastric cancer. Oncol. Lett. 10, 3495–3501. doi: 10.3892/ol.2015.3758
Ooi, C. H., Oh, H. K., Wang, H. Z., Tan, A. L., Wu, J., Lee, M., et al. (2011). A densely interconnected genome-wide network of microRNAs and oncogenic pathways revealed using gene expression signatures. PLoS Genet. 7:e1002415. doi: 10.1371/journal.pgen.1002415
Park, M., Kim, M., Hwang, D., Park, M., Kim, W. K., Kim, S. K., et al. (2014). Characterization of gene expression and activated signaling pathways in solid-pseudopapillary neoplasm of pancreas. Modern Pathol. Off. J. U. S. 27, 580–593. doi: 10.1038/modpathol.2013.154
Peng, Z., Guo, Z., Hu, R., He, X., Jiao, X., and Zhu, X. (2015). Interaction between microRNA-181a and TNFAIP1 regulates pancreatic cancer proliferation and migration. Tumor Biol. 36, 9693–9701. doi: 10.1007/s13277-015-3704-8
Plaisier, C. L., Pan, M., and Baliga, N. S. (2012). A miRNA-regulatory network explains how dysregulated miRNAs perturb oncogenic processes across diverse cancers. Genome Res. 22:2302. doi: 10.1101/gr.133991.111
Qian, F. C., Li, X. C., Guo, J. C., Zhao, J. M., Li, Y. Y., Tang, Z. D., et al. (2019). SEanalysis: a web tool for super-enhancer associated regulatory analysis. Nucleic Acids Res. 47, W248–W255. doi: 10.1093/nar/gkz302
Reid-Lombardo, K. M., Fridley, B. L., Bamlet, W. R., Cunningham, J. M., Sarr, M. G., and Petersen, G. M. (2013). Survival is associated with genetic variation in inflammatory pathway genes among patients with resected and unresected pancreatic cancer. Ann. Surg. 257, 1096–1102. doi: 10.1097/sla.0b013e318275b7e5
Sidiropoulos, K. G., White, N. M., Bui, A., Ding, Q., Boulos, P., Pampalakis, G., et al. (2014). Kallikrein-related peptidase 5 induces miRNA-mediated anti-oncogenic pathways in breast cancer. Oncoscience 1, 709–724. doi: 10.18632/oncoscience.91
Siegel, R. L., Miller, K. D., and Jemal, A. (2017). Cancer statistics, 2017. CA Cancer J. Clin. 67, 7–30. doi: 10.3322/caac.21387
Song, B., Zheng, K., Ma, H., Liu, A., Jing, W., Shao, C., et al. (2015). miR-429 determines poor outcome and inhibits pancreatic ductal adenocarcinoma growth by targeting TBK1. Cell. Physiol. Biochem. 35, 1846–1856. doi: 10.1159/000373995
Thackeray, J. T., Pietzsch, S., Stapel, B., Rickehoch, M., Lee, C. W., Bankstahl, J. P., et al. (2017). Insulin supplementation attenuates cancer-induced cardiomyopathy and slows tumor disease progression. JCI Insight. 2:e93098.
Tusher, V. G., Tibshirani, R., and Chu, G. (2001). Erratum: significance analysis of microarrays applied to the ionizing radiation response. Proc. Natl. Acad. Sci. U.S.A. 98, 5116–5121. doi: 10.1073/pnas.091062498
Tzeng, C. W., Frolov, A., Frolova, N., Jhala, N. C., Howard, J. H., Vickers, S. M., et al. (2007). Pancreatic cancer epidermal growth factor receptor (EGFR) intron 1 polymorphism influences postoperative patient survival and in vitro erlotinib response. Ann. Surg. Oncol. 14, 2150–2158. doi: 10.1245/s10434-007-9409-5
Vergoulis, T., Vlachos, I. S., Alexiou, P., Georgakilas, G., Maragkakis, M., Reczko, M., et al. (2012). TarBase 6.0: capturing the exponential growth of miRNA targets with experimental support. Nucleic Acids Res. 40, 222–229.
Vrahatis, A. G., Dimitrakopoulos, G. N., Tsakalidis, A. K., and Bezerianos, A. (2015). “Identifying miRNA-mediated signaling subpathways by integrating paired miRNA/mRNA expression data with pathway topology,” in Proceedings of the 2015 37th Annual International Conference of the IEEE Engineering in Medicine and Biology Society (EMBC), Milan.
Vrahatis, A. G., Dimitrakopoulou, K., Balomenos, P., Tsakalidis, A. K., and Bezerianos, A. (2016). CHRONOS: a time-varying method for microRNA-mediated subpathway enrichment analysis. Bioinformatics 32, 884–892. doi: 10.1093/bioinformatics/btv673
Walters, D. M., Lindberg, J. M., Adair, S. J., Newhook, T. E., Cowan, C. R., Stokes, J. B., et al. (2013). Inhibition of the growth of patient-derived pancreatic cancer xenografts with the MEK inhibitor trametinib is augmented by combined treatment with the epidermal growth factor receptor/HER2 inhibitor lapatinib. Neoplasia 15, U143–U207.
Wang, L., Zhang, J., Wan, L., Zhou, X., Wang, Z., and Wei, W. (2015). Targeting Cdc20 as a novel cancer therapeutic strategy. Pharmacol. Therapeut. 151, 141–151. doi: 10.1016/j.pharmthera.2015.04.002
Wang, W., Zhao, L., Wei, X., Wang, L., Liu, S., Yu, Y., et al. (2016). MicroRNA-320a promotes 5-FU resistance in human pancreatic cancer cells. Sci. Rep. 6:27641.
Wang, Z., Ahmad, A., Li, Y., Kong, D., Azmi, A. S., Banerjee, S., et al. (2010). Emerging roles of PDGF-D signaling pathway in tumor development and progression. Biochim. Biophys. Acta 1806, 122–130. doi: 10.1016/j.bbcan.2010.04.003
Wee, P., and Wang, Z. (2017). Epidermal growth factor receptor cell proliferation signaling pathways. Cancers 9:52. doi: 10.3390/cancers9050052
Wong, D. J., and Hurvitz, S. A. (2014). Recent advances in the development of anti-HER2 antibodies and antibody-drug conjugates. Ann. Transl. Med. 2:122.
Xiao, F., Zuo, Z., Cai, G., Kang, S., Gao, X., and Li, T. (2009). miRecords: an integrated resource for microRNA-target interactions. Nucleic Acids Res. 37, D105–D110. doi: 10.1093/nar/gkn851
Xiao, G., Wang, X., and Yu, Y. (2017). CXCR4/Let-7a axis regulates metastasis and chemoresistance of pancreatic cancer cells through targeting HMGA2. Cell. Physiol. Biochem. 43, 840–851. doi: 10.1159/000481610
Xie, B., Ding, Q., Han, H., and Wu, D. (2013). miRCancer: a microRNA-cancer association database constructed by text mining on literature. Bioinformatics 29, 638–644. doi: 10.1093/bioinformatics/btt014
Xin, L., Gao, J., Wang, D., Lin, J. H., Liao, Z., Ji, J. T., et al. (2017). Novel blood-based microRNA biomarker panel for early diagnosis of chronic pancreatitis. Sci. Rep. 7:40019. doi: 10.1038/srep40019
Yamaguchi, M., Okamura, S., Yamaji, T., Iwasaki, M., Tsugane, S., Shetty, V., et al. (2019). Plasma cytokine levels and the presence of colorectal cancer. PLoS One 14:e0213602. doi: 10.1371/journal.pone.0213602
Yang, J., Zhang, H. F., and Qin, C. F. (2017). MicroRNA-217 functions as a prognosis predictor and inhibits pancreatic cancer cell proliferation and invasion via targeting E2F3. Eur. Rev. Med. Pharmacol. Sci. 21, 4050–4057.
Yao, J., Zhang, C., Chen, Y., and Gao, S. (2019). Downregulation of circular RNA circ-LDLRAD3 suppresses pancreatic cancer progression through miR-137-3p/PTN axis. Life Sci. 239:116871. doi: 10.1016/j.lfs.2019.116871
Yu, G., Wang, L., Han, Y., and He, Q. (2012). clusterProfiler: an R package for comparing biological themes among gene clusters. OMICS J. Integr. Biol. 16, 284–287. doi: 10.1089/omi.2011.0118
Yu, Z., Zhao, S., Wang, L., Wang, J., and Zhou, J. (2019). miRNA-339-5p plays an important role in invasion and migration of pancreatic cancer cells. Med. Sci. Monit. 25, 7509–7517. doi: 10.12659/MSM.917038
Keywords: pathway, gene, miRNA, pancreatic cancer, survival
Citation: Liu Y, Cui Y, Bai X, Feng C, Li M, Han X, Ai B, Zhang J, Li X, Han J, Zhu J, Jiang Y, Pan Q, Wang F, Xu M, Li C and Wang Q (2020) MiRNA-Mediated Subpathway Identification and Network Module Analysis to Reveal Prognostic Markers in Human Pancreatic Cancer. Front. Genet. 11:606940. doi: 10.3389/fgene.2020.606940
Received: 16 September 2020; Accepted: 13 November 2020;
Published: 09 December 2020.
Edited by:
Lixin Cheng, Jinan University, ChinaReviewed by:
Aristidis Vrahatis, University of Thessaly, GreeceCopyright © 2020 Liu, Cui, Bai, Feng, Li, Han, Ai, Zhang, Li, Han, Zhu, Jiang, Pan, Wang, Xu, Li and Wang. This is an open-access article distributed under the terms of the Creative Commons Attribution License (CC BY). The use, distribution or reproduction in other forums is permitted, provided the original author(s) and the copyright owner(s) are credited and that the original publication in this journal is cited, in accordance with accepted academic practice. No use, distribution or reproduction is permitted which does not comply with these terms.
*Correspondence: Qiuyu Wang, d2FuZ3FpdXl1OTAwNDkwQDE2My5jb20=
†These authors have contributed equally to this work
Disclaimer: All claims expressed in this article are solely those of the authors and do not necessarily represent those of their affiliated organizations, or those of the publisher, the editors and the reviewers. Any product that may be evaluated in this article or claim that may be made by its manufacturer is not guaranteed or endorsed by the publisher.
Research integrity at Frontiers
Learn more about the work of our research integrity team to safeguard the quality of each article we publish.