- Department of Biology, University of Regina, Regina, SK, Canada
Antimicrobial resistance is a worldwide health crisis for which new antibiotics are needed. One strategy for antibiotic discovery is identifying unique antibiotic biosynthetic gene clusters that may produce novel compounds. The aim of this study was to demonstrate how an integrated approach that combines genome mining, comparative genomics, and functional genetics can be used to successfully identify novel biosynthetic gene clusters that produce antimicrobial natural products. Secondary metabolite clusters of an antibiotic producer are first predicted using genome mining tools, generating a list of candidates. Comparative genomic approaches are then used to identify gene suites present in the antibiotic producer that are absent in closely related non-producers. Gene sets that are common to the two lists represent leading candidates, which can then be confirmed using functional genetics approaches. To validate this strategy, we identified the genes responsible for antibiotic production in Pantoea agglomerans B025670, a strain identified in a large-scale bioactivity survey. The genome of B025670 was first mined with antiSMASH, which identified 24 candidate regions. We then used the comparative genomics platform, EDGAR, to identify genes unique to B025670 that were not present in closely related strains with contrasting antibiotic production profiles. The candidate lists generated by antiSMASH and EDGAR were compared with standalone BLAST. Among the common regions was a 14 kb cluster consisting of 14 genes with predicted enzymatic, transport, and unknown functions. Site-directed mutagenesis of the gene cluster resulted in a reduction in antimicrobial activity, suggesting involvement in antibiotic production. An integrated approach that combines genome mining, comparative genomics, and functional genetics yields a powerful, yet simple strategy for identifying potentially novel antibiotics.
Introduction
Antimicrobial resistance remains one of the greatest health threats worldwide (Aslam et al., 2018). Increased resistance, combined with limited investment in antibiotic discovery and development, has left healthcare providers with few options to treat multi-drug resistant bacterial infections (Aslam et al., 2018; Morehead and Scarbrough, 2018). In 2017, in response to the growing number of drug-resistant bacteria, the World Health Organization released a list of pathogens for which there are few treatment options (World Health Organization, 2017). This included the Gram-negative species Acinetobacter baumannii, Pseudomonas aeruginosa, Salmonella sp., and other members of the Enterobacteriaceae, as well as Gram-positive species Enterococcus faecium and Staphylococcus aureus. While many promising antibiotics have entered clinical trials in recent years, the majority of these antibiotics are from existing classes of therapeutics, and may eventually become ineffective due to existing resistance determinants (Hutchings et al., 2019). Furthermore, the majority of approved antibiotics in the past decade have been ineffective against pathogens of greatest concern, such as carbapenem-resistant Gram-negative bacteria (Moss and Boucher, 2020), highlighting the importance of identifying additional unique bioactive molecules.
Natural products remain a promising source of novel therapeutics (Katz and Baltz, 2016). Many pharmaceuticals have been derived from the secondary metabolites of bacteria and fungi with a variety of clinical applications, including cardiovascular drugs, chemotherapeutics, immunomodulators, and antibiotics (Patridge et al., 2016). However, the exploration of bioactive natural product antibiotics has been limited to a relatively small pool of genera, including the bacterial genus, Streptomyces and the fungal genera, Penicillium and Cephalosporium (Patridge et al., 2016). Since the discovery of streptomycin in Streptomyces griseus in 1944, actinomycetes, namely Streptomyces, have been the primary source of antibiotics (Watve et al., 2001; de Lima Procópio et al., 2012). Nonetheless, many species outside of the Actinobacteria have also been identified as antimicrobial producers (Fischbach, 2009; Pidot et al., 2014; Hutchings et al., 2019).
One antibiotic-producing group is the bacterial genus Pantoea, which belongs to the Erwiniaceae of the Enterobacterales (Adeolu et al., 2016). Pantoea antimicrobials have been predominantly explored for their antibiosis toward agricultural pathogens, such as Erwinia amylovora the causative agent of fire blight in apple and pear crops (Johnson and Stockwell, 1998; Pusey, 2002; Stockwell et al., 2002; Walterson and Stavrinides, 2015). Among the more well-studied antibiotics effective against E. amylovora are Pantoea Natural Product 1 (identified as 4-formylaminooxyvinylglycine; PNP-1) (Walterson et al., 2014; Okrent et al., 2018; Smith et al., 2019), pantocin A (Wright et al., 2001; Jin et al., 2003a; Smits et al., 2019), pantocin B (Brady et al., 1999; Wright et al., 2001), and herbicolin I/dapdiamide E (Ishimaru et al., 1988; Sammer et al., 2009, 2012; Dawlaty et al., 2010; Kamber et al., 2012). Comparatively, fewer Pantoea natural products have been investigated for potential antagonism toward clinical pathogens. Andrimid, a well-studied antibiotic, is a hybrid non-ribosomal peptide-polyketide that exhibits broad-spectrum activity against many clinically relevant bacteria including Enterococcus, Staphylococcus, and several genera within the Enterobacteriaceae. (Needham et al., 1994; Singh et al., 1997; Sánchez et al., 2013; Matilla et al., 2016). Andrimid, which functions by inhibiting acetyl-CoA carboxylase (Freiberg et al., 2004), is not only produced by Pantoea (Jin et al., 2006), but also strains of Pseudomonas fluorescens (Needham et al., 1994; Singh et al., 1997), Vibrio sp. (Long et al., 2005; Wietz et al., 2010), and Serratia sp. (Matilla et al., 2016). More recently, the two antibiotics Pantoea Natural Product 2 (PNP-2) and Pantoea Natural Product 3 (PNP-3) have been shown to be effective against several clinical pathogens. PNP-2 exhibits antimicrobial activity against several genera within the Enterobacteriaceae and Erwiniaceae, as well as S. aureus (Robinson et al., 2020). PNP-3 inhibits growth of multi-drug resistant A. baumannii, P. aeruginosa, multiple genera of the Enterobacteriaceae, as well as S. aureus and Streptococcus mutans (Williams and Stavrinides, 2020). This suggests that further exploring the metabolic diversity of Pantoea species could yield potentially novel antimicrobial metabolites.
One strategy for bioprospecting for antimicrobials involves the identification of biosynthetic gene clusters. A biosynthetic gene cluster is a modular unit of two or more contiguous genes that are responsible for metabolite production (Medema et al., 2015). These genes encode proteins that synthesize the final product, and often include genes encoding regulatory elements, transport proteins, resistance factors, or those involved in precursor production (Cimermancic et al., 2014; Tietz and Mitchell, 2015). Identifying gene clusters that direct the biosynthesis of new antimicrobials may not only facilitate the identification of novel compounds, but also can provide the means for metabolite production through heterologous expression (Medema et al., 2015; Huo et al., 2019). Identification of these biosynthetic clusters can be achieved by surveying mutant libraries for loss of antibiotic activity (Ochman et al., 1988; Walterson et al., 2014; Robinson et al., 2020; Williams and Stavrinides, 2020), or by creating expression libraries, and surveying clones for antibiotic production (Tuan et al., 1990; Wright et al., 2001; Ziemert et al., 2016).
The genomics era has also provided genome mining tools to identify candidate antibiotic biosynthetic gene clusters (Medema and Fischbach, 2015). Algorithms that have been developed to search for secondary metabolite gene clusters include antiSMASH (Medema et al., 2011; Blin et al., 2019), BAGEL (de Jong et al., 2006; van Heel et al., 2018), NP.searcher (Li et al., 2009), and PRISM (Skinnider et al., 2015, 2017). Many of these algorithms, however, rely on patterns derived from known antibiotic gene clusters and associated protein motifs, thereby limiting de novo prediction. Modified strategies have been developed to attempt to predict novel biosynthetic clusters, such as mining for duplicated or altered housekeeping enzymes (e.g., ARTS) (Alanjary et al., 2017), surveying for the presence of specific resistance mechanisms (e.g., ARTS and CARD) (McArthur et al., 2013), or identifying regulators and/or regulator binding sites (e.g., CASSIS) (Wolf et al., 2016). These genomic signatures may point to the presence of novel biosynthetic gene clusters not identified with other genome mining methods (Ziemert et al., 2016).
Comparative genomics strategies can also be useful for identifying novel biosynthetic gene clusters, as they are pattern-independent strategies (Takeda et al., 2014; Hautbergue et al., 2018). Using comparative genomic strategies to identify all genes unique to a strain of interest, however, can potentially generate extensive lists of candidate genes. To attempt to reduce the number of candidate genes, strategies that combine genome mining with comparative genomics can be used. For example, one strategy used to identify the gene clusters for multiple antibiotics in fungal strains involved searching for secondary metabolite genes of interest (e.g., a terpene synthase to identify terpenoid clusters) in genomic scaffolds using tblastn (Chooi et al., 2010; Cacho et al., 2015). The number of scaffolds of interest was then further reduced by comparing the sequences to related strains with alternative antibiotic production profiles to identify regions unique to the strain of interest (Chooi et al., 2010; Cacho et al., 2015). Searching for secondary metabolite biosynthetic clusters using this approach is effective, but may be time consuming if the identity or class of secondary metabolite is unknown.
Here, we propose a simplification of this approach by combining online genome mining tools with comparative genomics to identify biosynthetic gene clusters encoding potentially novel antibiotics in bacterial genomes. Unlike other strategies, our approach involves a subtractive analysis whereby gene cluster candidates from genome mining prediction are cross-referenced to the list of unique genes in the strain of interest identified by comparative genomic analysis. Candidate gene clusters identified by these comparisons can then be evaluated for involvement in antibiotic production using genetic approaches. To demonstrate the utility of this approach, we surveyed a collection of 116 Pantoea strains for antibiotic production using a minimal media agar-based bioassay, and showed that 59 strains produce natural products that antagonize diverse clinically relevant pathogens. One antibiotic-producing strain, P. agglomerans B025670 (B025670), antagonized several Gram-negative pathogens, prompting us to pursue the genes responsible for metabolite biosynthesis. We compared the independent outputs of antiSMASH results for B025670 to a collection of genes unique to B025670 as determined by whole genome comparison to related strains. One promising gene cluster was common to both lists, and subsequent mutation of the cluster via homologous recombination led to a reduction in the zones of inhibition. We suggest that our proposed approach is a quick, simple and efficient method for identifying candidate antibiotic biosynthetic gene clusters without the need for extensive computational power or expertise.
Materials and Methods
Bacterial Strains and Growing Conditions
Strains used in this study are shown in Supplementary Table 1. Overnight cultures of Pantoea and Erwinia strains were grown at 30°C and all other strains were grown at 37°C with shaking at 220 RPM. All cultures were maintained on lysogeny broth (LB) (Miller; BD Biosciences Franklin Lakes, NJ, United States) agar and supplemented with gentamicin (gent; 30 μg/mL, 15 μg/mL for recombinants) when appropriate. E. faecium K0260810 was maintained on Bacto Brain Heart Infusion (BD Biosciences).
Agar Overlay Assay
Agar overlay assays (adapted from Wright et al., 2001) were used to evaluate antibiotic production of Pantoea strains. Overnight cultures of target bacteria were pelleted and resuspended in an equal volume of 10 mM MgSO4. Strains with slower growth in overlays (E. faecium K0260810, S. aureus K5-4, S. aureus K1-7) were resuspended in half- or quarter-volumes of 10 mM MgSO4 to concentrate bacteria. Overlay agar was prepared with 3.2–4 mL molten 0.9% agar, 800 μL 5× glucose-asparagine solution (1 L 5× solution: 55.75 g K2HPO4, 22.5 g KH2PO4, 0.6 g MgSO4⋅7H2O, 1.5 g L-asparagine, 0.25 g nicotinic acid, and 100 g glucose), and 300 μL of the target bacteria (Wodzinski et al., 1994). This was poured over 1× Escherichia coli Minimal Media agar [20 mL; 1 L 1× solution: 0.25 g yeast extract, 20 mL glycerol, 4 g K2HPO4, 1.72 g KH2PO4, 0.5 g NaCl, 2.0 g (NH4)2SO4, 0.2 g C6H5Na3O7⋅2H2O, 0.002 g MgSO4⋅7H2O; agar final concentration: 1.5%] and allowed to solidify (Wright et al., 2001). Pantoea strains to be tested for antibiotic production (designated ‘test strains’) were cultured overnight without antibiotics, pelleted, resuspended in an equal volume of 10 mM MgSO4, and 5 μL spotted on the agar overlays. For survey overlays of Enterobacter sp. TX1, Klebsiella sp. B011499, Kosakonia sp. 12202, and Pseudocitrobacter sp. B012497, single colonies of test strains were streaked from agar plates onto overlays with toothpicks. E. faecium K0260810 overlays were tested with both Pantoea liquid culture and streaked colonies. Plates were incubated at 30°C for 16–48 h until the layer of target bacteria was opaque. All images were captured with an Epson Perfection V330 photo scanner at 1200 DPI, and brightness and contrast were adjusted to enhance zones of inhibition. All Pantoea survey strains were tested at least once and all overlays involving P. agglomerans B025670 were replicated at least twice.
Secondary Metabolite and Comparative Genomic Analysis
Secondary metabolite profiles for 31 Pantoea species were generated using antiSMASH 5.1.2 (Blin et al., 2019). FASTA files of draft genomes (designated in Supplementary Table 1) were analyzed with the web version with both ‘relaxed’ and ‘loose’ detection strictness with the switches ‘-KnownClusterBlast,’ ‘-ClusterBlast,’ and ‘-SubClusterBlast.’ FASTA files of contigs containing PNP-1 (Pantoea ananatis BRT175), PNP-2 (P. agglomerans TX10), PNP-3 (P. agglomerans SN01080, 3581), pantocin A (P. agglomerans 3581), and cluster 675 (P. agglomerans B025670) were also analyzed with the web versions of BAGEL4 (van Heel et al., 2018), NP.Searcher (range 1–5,000; ‘-display unknown peptide or ketide units’ and ‘-none’ switches selected) (Li et al., 2009), and PRISM (all advanced settings switches on; structure limit = 50; window = 10,000 bp) (Skinnider et al., 2017). Antibiotic cluster accession numbers: BRT175 PNP-1 (ASJH00000000) (Smith et al., 2013), TX10 PNP-2 (MN329808), SN01080 PNP-3 (MN807450), 3581 PNP-3 (MN807451), B025670 675 (MT711882), and 3581 pantocin A (MT711881).
Genome comparisons of B025670 to other Pantoea strains were performed with EDGAR 2 (Blom et al., 2009, 2016). Genomes in EDGAR were annotated with Prokka 1.12 (Seemann, 2014). B025670 was compared to P. agglomerans DC432 and SP04022 (Trial 1), as well as P. agglomerans DC432, P. agglomerans SP04022, Pantoea dispersa 625, Pantoea stewartii 626, Pantoea eucalypti B011489, Pantoea brenneri B016381, P. brenneri B024858, P. eucalypti F9026, P. ananatis 15320, P. ananatis 17671, and P. ananatis 26SR6 (Trial 2). Nucleotide FASTA files of genes unique to B025670 and of antiSMASH regions were compared with standalone BLAST and parsed. Candidate cluster 675 was annotated with PATRIC with the following parameters: domain = bacteria; taxonomy name = Pantoea agglomerans (taxonomy ID = 549); genetic code = 11 (Archaea and Bacteria), and annotation recipe = default (Brettin et al., 2015). The complete nucleotide sequence of cluster 675 was searched for against the NCBI Nucleotide Collection and WGS (limit: Pantoea) databases with blastn. Amino acid sequences were analyzed with CDD batch search against CDD v3.18 at an E-value threshold of 0.01 with composition-based statistics adjustment “on.” Translated nucleotide BLASTs (blastx) were performed against the nr database, and the best hit for each gene with the lowest E-value and highest percent identity was recorded. Cluster 675 from P. dispersa M1657A was annotated with GeneMark.hmm prokaryotic v3.25 (Zhu et al., 2010). Cluster figures were generated with Easyfig 2.2.3 (Sullivan et al., 2011).
Mutagenesis
Genes 4 and 7 of cluster 675 in B025670 were disrupted via single-crossover homologous recombination. Taq DNA Polymerase (GeneDireX) was used to amplify an internal fragment of these genes from B025670 using specific primers (Supplementary Table 2). Fragments were digested for 2 h with PstI or XmaI (New England Biolabs) and cloned into the PstI or XmaI restriction site of pKNOCKGm. Plasmid and insert were ligated in a 1:3 ratio, respectively, in a 10 μL reaction with T4 DNA ligase (New England Biolabs). The ligated product (5 μL) was electroporated into 100 μL of electrocompetent E. coli CC118 cells using a micropulser (Bio-Rad) at 2.5 kV in a 1 mm cuvette, and cells were plated on LB-gent agar. The transformants were confirmed by colony PCR with Taq DNA Polymerase (GeneDireX) using primers gent+391 and gent-57 (Supplementary Table 2). Transformants were also confirmed by PCR with the fragment-specific primers (Supplementary Table 2). Plasmids were electrotransformed into electrocompetent B025670 and plated on LB-gent agar. Integration of the plasmid was confirmed by colony PCR with Taq DNA Polymerase (GeneDireX) using primers gent+391 and 675_9-790_XmaI for 675-7 and gent+391 and 675_4+1 for 675-4 (Supplementary Table 2). An integration mutant of a separate gene cluster (697-2::Gm) was generated, confirmed with primers 697_1+145_PstI and gent+391, and used as a gentamicin control strain. The resulting mutants were evaluated for loss of antibiotic production on overlays of strains highlighted in Table 2. Test strains were adjusted to the same OD600, and non-standardized relative OD600 values were obtained with the Gen5 software suite (BioTek) and Epoch Microplate Spectrophotometer in a CELLSTAR 96 well plate (Greiner Bio-One) containing 300 μL culture per well.
Results
Pantoea Strains Exhibit Antimicrobial Activity Against Several Clinical Pathogens
Pantoea strains (116 total) were surveyed for antibiotic production against both Gram-negative (A. baumannii, E. coli, Enterobacter sp., E. amylovora, Klebsiella sp., Kosakonia sp., P. aeruginosa, Pseudocitrobacter sp., and S. enterica Typhimurium) and Gram-positive species (Lactococcus lactis, S. aureus, and E. faecium) (Supplementary Table 3). Thirty-two Pantoea strains antagonized either S. aureus K1-7 or E. amylovora Ea321 (Table 1), as indicated by the formation of a zone of inhibition. Between 10 and 20 Pantoea strains were effective against L. lactis, Salmonella enterica, Enterobacter, Kosakonia, Pseudocitrobacter, E. faecium, and E. coli. However, only two Pantoea strains (P. agglomerans SN01080r and 3581r) were effective against P. aeruginosa, A. baumannii, and Klebsiella. The range of pathogens inhibited varied across strains of Pantoea. For example, 59 of the 116 strains (50.9%) antagonized at least one pathogen, with 36 of the 116 strains (31.0%) antagonizing more than one pathogen (Supplementary Table 4). The remaining 57 strains did not antagonize any of the target pathogens. In addition, 39 of the 116 strains (33.6%) antagonized at least one Gram-negative strain or one Gram-positive strain, and 19 of the 116 strains (16.4%) antagonized both Gram-positive and Gram-negative strains.
There were also differences in antagonistic capabilities across the different Pantoea species groups. Over 50% of the representatives of P. agglomerans, Pantoea anthophila, Pantoea conspicua, and P. dispersa exhibited antimicrobial activity, whereas less than 50% of P. ananatis, P. brenneri, P. eucalypti, Pantoea eucrina, and Pantoea septica strains exhibited antibiosis (Supplementary Table 4). No strains of Pantoea latae and P. stewartii had antimicrobial activity, although these species groups were represented by only two strains each. Representative strains of P. ananatis, P. conspicua, and P. eucrina antagonized only Gram-negative bacteria, while those of P. anthophila and P. septica were active against only Gram-positive bacteria. Strains of P. agglomerans, P. brenneri, P. dispersa, and P. eucalypti antagonized both groups of pathogens. Strains exhibiting inhibitory activity against a single pathogen were found across multiple species groups (Supplementary Tables 3, 5). Pantoea strains that antagonized over 50% of the target pathogens included SN01080r, which had the broadest antibacterial activity at 11 pathogens, and DC434, 3581r, SP05120, SP01202, SP05061, EH318, TX10, B025670, M1657B, B026440, and SP03412 that antagonized between 4 and 8 pathogens each (Supplementary Tables 3, 5). These Pantoea strains, with the exception of TX10, B025670, M1657B, and B026440, were isolated from the environment. Strains effective against 50% or more of both Gram-negative and Gram-positive pathogens targeted included P. agglomerans SN01080r, SP01202, DC434, SP05120, TX10, SP03412, and P. dispersa M1657B (Supplementary Table 5).
P. agglomerans B025670 Antagonizes Several Clinical Enterobacterales Strains
One strain of interest that emerged from the antibiotic survey was P. agglomerans B025670 (B025670), a clinical isolate that was able to antagonize Enterobacter, E. amylovora, E. coli, Kosakonia, Pseudocitrobacter sp., and S. enterica (Table 2 and Supplementary Table 3). Strains with similar antibiotic production profiles included P. ananatis BRT175 and P. dispersa M1657A; however, these were not shown to be effective against E. coli HB101 and Pseudocitrobacter sp. B012497 under the tested conditions. P. dispersa M1657B also had a similar antibiotic profile, although it lacked inhibitory activity against E. coli and was inhibitory against S. aureus. The inhibitory activity of B025670 was assayed against additional clinical isolates of Pseudocitrobacter sp., E. coli, Enterobacter sp., and Klebsiella sp. and was found to antagonize six total additional strains of E. coli and Enterobacter sp. (Supplementary Figure 1 and Table 2), including multi-drug resistant E. coli A6152, which is resistant to ampicillin, cephalosporins, ciprofloxacin, and gentamicin (Supplementary Table 1). The antibiosis against some strains was ambiguous, where there appeared to be reduced growth of B025670, making it difficult to assess whether a zone of inhibition was present (Supplementary Figure 1).
antiSMASH Predicts Numerous Candidate Biosynthetic Clusters
To attempt to identify the gene cluster(s) responsible for antibiotic production in B025670, antiSMASH was used to predict candidate secondary metabolite clusters (Supplementary Table 6). We also analyzed an additional 30 Pantoea strains with antiSMASH to provide a basis for comparison of gene cluster complements (Supplementary Table 6). The B025670 genome had 24 predicted regions, consistent with the other Pantoea genomes that had between 16 and 28 predicted metabolite biosynthetic clusters. Eleven of the 24 predicted B025670 gene clusters were also present in several other Pantoea genomes, including those involved in the biosynthesis of amonabactin P 750, aryl polyenes, carotenoids, desferrioxamine E, emulsan, herboxidiene, O&K-antigen, O-antigen, polysaccharide B, stewartan, and taxlllaid A (Supplementary Table 6). The other 13 predicted clusters of B025670, one of which we anticipated being involved in the biosynthesis of our antibiotic, included one homoserine lactone, three fatty acid, and nine saccharide gene clusters, each composed of 17–26 genes.
Genomes that carry the PNP-1, PNP-2, PNP-3, and pantocin A gene clusters were also included in our antiSMASH analysis to help provide a benchmark for the ability of antiSMASH to predict these novel gene clusters. The PNP-3 gene cluster in P. agglomerans SN01080, the PNP-2 cluster of P. agglomerans TX10, the PNP-1 cluster of P. ananatis BRT175, and the pantocin A cluster of P. agglomerans 3581 were not identified by antiSMASH (Supplementary Table 7). However, the algorithm predicted that the entire 8.5 kb PNP-3 cluster from P. agglomerans 3581 was part of an approximately 48 kb phosphonate/NRPS-encoding region (region 13.1; Supplementary Table 6). The PNP-1, PNP-2, PNP-3, and pantocin A gene clusters were also analyzed with genome mining tools BAGEL4, NP.searcher, and PRISM, but these tools were unable to identify the complete clusters, with the exception of pantocin A, which was correctly predicted by PRISM (Supplementary Table 7).
Identification of Candidate Antibiotic Clusters Using Comparative Genomics
To narrow down the candidate list provided by antiSMASH, we used a comparative genomics approach to generate a list of genes unique to B025670 that could be responsible for antibiotic production. EDGAR identified 436 genes that were present in B025670, but absent in two closely related P. agglomerans strains lacking antimicrobial activity against Enterobacter sp. TX1 and Kosakonia sp. 12202. The list of unique genes was compared to the B025670 antiSMASH predictions using BLAST, and 40 genes across 5 antiSMASH regions (4.1, 18.1, 31.1, 32.2) were found to be common between the two datasets (Supplementary Table 8). Within antiSMASH regions 4.1, 18.1, 21.2, and 32.2, the majority of the common genes were contiguous and syntenic. The B025670 genome was then compared to a larger set of 11 genomes, which included more distantly related Pantoea strains. This comparison yielded 221 genes unique to B025670. Comparison of the gene set to the antiSMASH regions yielded 23 genes in common across four antiSMASH regions (4.1, 18.1, 21.2, 32.2) (Supplementary Table 9). Groups of sequential genes were identified in regions 4.1 (13 genes), and 32.2 (4 genes). The group of 13 genes, which was identified by both sets of comparisons, was determined to be the most likely cluster and was designated cluster 675 (Figure 1).

Figure 1. Candidate antibiotic cluster (cluster 675) of P. agglomerans B025670 and a homologous cluster found in P. dispersa M1657A (78.06% nucleotide identity, 100% query cover, E = 0). The lines above each region indicate the cluster prediction of antiSMASH (region 4.1), as compared to the region predicted by comparative genomic approaches using EDGAR. Asterisks denote genes that were targeted for disruption by homologous recombination. Flanking genes are shown as open (unshaded) arrows.
To delineate the boundaries of the complete cluster, a standalone BLAST of the genes and the surrounding genomic region was performed against our collection of Pantoea genomes. An identical cluster was identified in the clinical isolate P. dispersa M1675A (78% nucleotide identity, 100% query cover, E = 0); however, a comparison of the cluster and its flanking region indicated that the conserved portion was 14 genes and approximately 14 kb in length, the entirety of which was found in the EDGAR datasets (Figure 1). We then examined the distribution of the nucleotide sequence across bacteria in the public databases. The cluster was found in its entirety in four additional Pantoea draft genomes: Pantoea sp. EKM22T (JAALFX010000004.1, 99.54% identity, 100% query cover, E = 0), Pantoea sp. EKM21T (JAALFV010000007.1, 99.54% identity, 100% query cover, E = 0), P. agglomerans DAPP-PG734 (JNVA01000020.1, 99.52% identity, 99% query cover, E = 0), and Pantoea deleyi LMG 24200 (MIPO01000022.1; 99.18% identity, 100% query cover, E = 0). Cluster 675 was also analyzed with BAGEL4, NP.searcher, and PRISM, but these algorithms did not identify the region as a metabolite biosynthetic gene cluster (Supplementary Table 7).
Characterization of Cluster 675
As cluster 675 was the leading biosynthetic gene cluster responsible for antibiotic production in B025670, its genetic composition was characterized further. The individual cluster proteins were analyzed with the CDD and BLAST and were found to include predicted enzymes involved in modification and transport (Table 3). Genes 1, 4, 5, 6, 8, 9, 10, and 11 encode predicted reductases, synthases, ligases/synthetases, and transferases. Gene 7 encodes a protein similar to an RND multidrug efflux pump subunit, sharing 41.73% amino acid identity (96% query cover, E = 0) with the multispecies AcrB permease subunit (WP_001132469.1). There were no predicted domains for gene 14, although the PATRIC annotation identified gene 14 as a putative transmembrane protein. Genes 3, 12, and 13 encode hypothetical proteins lacking conserved domains.
To assess whether cluster 675 of B025670 was related to antibiotic production, individual genes were disrupted by single-integration homologous recombination and mutants assayed for loss of antibiotic production. Disruption of either gene 4 (beta-ketoacyl synthase) or gene 7 (RND multidrug efflux pump subunit) resulted in a loss of zone of inhibition on Enterobacter sp. ATCC 700323, D6370, D6437, TX1, and TX2, E. coli A6152, Pseudocitrobacter sp. B012497, and Kosakonia sp. 12202 overlays when compared to the wild-type strain and a gentamicin control (Figures 1, 2). Zones of inhibition were still observed for mutants tested on overlays of E. coli C31C4 and HB101 (Figure 2), possibly indicating the presence of additional antimicrobial metabolites.
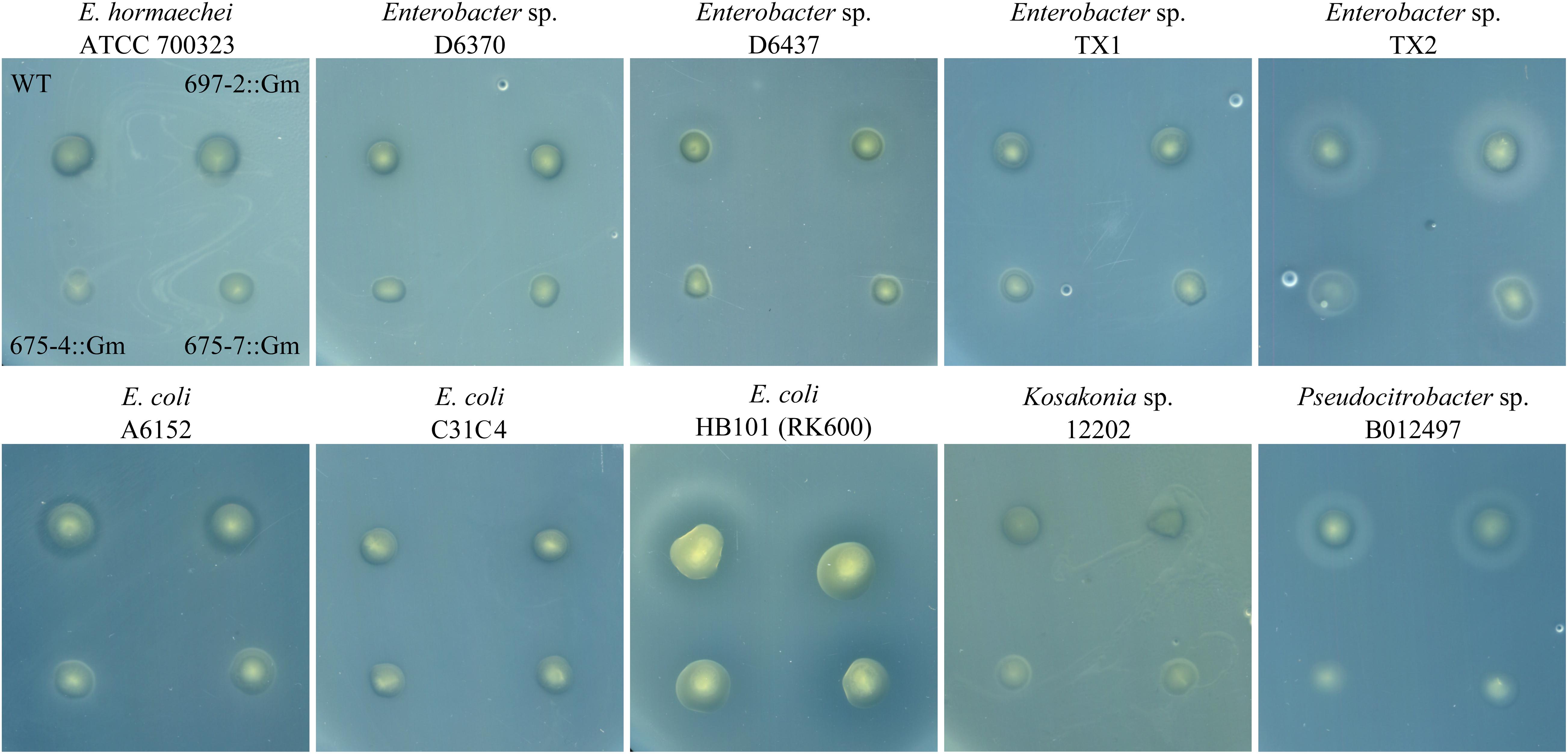
Figure 2. Antibiotic production assay showing an overlay of multiple target bacteria spotted with wild-type P. agglomerans B025670 (WT), next to a gentamicin control mutant of B025670 (697-2::Gm). The bottom set of spots on each plate show B025670 mutants with disruptions in two different genes of cluster 675 (675-4::Gm, 675-7::Gm).
Discussion
In this study, we sought to identify candidate antibiotic gene clusters in bioactive Pantoea strains by combining genome mining, comparative genomics, and functional genetics approaches. We first identified bioactive Pantoea by surveying 116 strains for antimicrobial activity against a panel of pathogens on a minimal medium (Supplementary Table 3). Our use of a minimal medium was previously shown to be important for conditional expression of secondary metabolite gene clusters in Pantoea, including PNP-1 (Walterson et al., 2014), PNP-2 (Robinson et al., 2020), and PNP-3 (Williams and Stavrinides, 2020). This is also the case for pantocin A and B in P. agglomerans EH318 (Wright et al., 2001). Gene clusters that are conditionally expressed are often referred to as silent biosynthetic gene clusters, but can be activated through a variety of means, including manipulation of media composition and other growth conditions (Reen et al., 2015; Zhang et al., 2019). For PNP-1 and PNP-3, altering the composition of the top and base medium in agar overlays led to changes in the size of the zones of inhibition, and in the case of PNP-3, use of a complex medium in both layers caused complete loss of zone of inhibition (Walterson et al., 2014; Williams and Stavrinides, 2020). For pantocin A, introduction of exogenous histidine neutralizes the effects of the antibiotic on the target pathogen, and also decreases paaA transcription, demonstrating a link between nutrition and antibiotic expression (Wright et al., 2001; Klein et al., 2017). Therefore, the choice of medium can have a considerable impact on the observation of antimicrobial activity.
Our antibiotic production survey on a minimal medium identified 59 Pantoea strains exhibiting antimicrobial activity against clinically relevant genera, including A. baumannii, Enterobacter, E. faecium, E. coli, Klebsiella, Kosakonia, Pseudocitrobacter, P. aeruginosa, S. enterica, and S. aureus (Supplementary Tables 3, 4). Among the bioactive strains, 36 Pantoea were active against multiple pathogens, 19 exhibited activity against both Gram-negative and Gram-positive bacteria, and 23 had activity against a single target, although some of these strains were tested against a limited number of target pathogens (Supplementary Tables 3, 4). For Pantoea strains exhibiting antimicrobial activity against multiple pathogens, the antagonism of multiple species could be due to a single broad-spectrum antibiotic or multiple antibiotics each having narrower activity. In the case of P. agglomerans TX10 and 3581, multiple antibiotics, including pantocin A and their respective PNP antibiotics, were responsible for the observed spectrum of activity under the tested conditions (Robinson et al., 2020; Williams and Stavrinides, 2020). Both broad- and narrow-spectrum antibiotics have applications as therapeutics; however, as screening methods to identify causal agents of disease improve, the ability to target specific bacteria is becoming increasingly important (Melander et al., 2018).
Among the Pantoea strains with a more narrow-spectrum activity was P. agglomerans B025670 (B025670), which is effective against the Gram-negative human pathogenic species Enterobacter sp., E. coli, Kosakonia sp., Pseudocitrobacter sp., and S. enterica Typhimurium (Supplementary Table 3; Table 2). Of these bacteria, drug-resistant Enterobacter sp., E. coli, and Salmonella sp. are listed as critical and high priority pathogens for which new therapeutics are needed (World Health Organization, 2017). Therefore, B025670 was a promising strain for further exploration. The approach for identifying the B025670 antibiotic biosynthetic gene cluster involved comparing the list of antiSMASH-predicted secondary metabolite clusters of B025670 to a list of genes that were unique to B025670, but absent in strains with contrasting antibiotic profiles. Cluster 675 emerged as one of the leading candidates (Figure 1).
An analysis of the genetic composition and organization of cluster 675 revealed that it shared some characteristics with other antibiotic gene clusters including the presence of modifying enzymes such as synthases, reductases, ligases/synthetases, and transferases, as well as a transporter (Table 3). To confirm the involvement of the candidate cluster in antibiotic production, we used homologous recombination to disrupt two independent genes within the cluster, which led to a reduction in antibiotic production against several of our target bacteria (Figure 2 and Table 2). This suggests that this cluster is likely involved in the biosynthesis of an antibiotic product, or at least a necessary precursor. Whether or not the gene cluster is operonic, it is apparent that the beta-ketoacyl synthase (gene 4) and multidrug efflux pump subunit AcrB (gene 7) are involved in antibiotic production (Figure 2). Normally, beta-ketoacyl synthases are involved in elongation during fatty acid biosynthesis (Rock and Jackowski, 2002); therefore, is it possible that the synthesized antibiotic may have a fatty acid component. As for gene 7, the E. coli AcrB subunit is part of an RND transporter that has a wide substrate specificity (Yu et al., 2003). These tripartite efflux pumps have been implicated in multi-drug resistance in Gram-negative bacteria (Nikaido and Takatsuka, 2009), suggesting the transporter may be involved in export of the final antimicrobial product from the cell. Interestingly, the loss of the zone of inhibition for cluster 675 mutants was not observed for all the tested pathogens, suggesting the production of additional mechanisms of antibiosis by B025670 (Figure 2 and Table 2). If cluster 675 is responsible for synthesis of a precursor rather than the antibiotic itself, additional genes or gene clusters identified with the comparative genomic analysis may be involved in synthesis of the final product. Among the genes identified by the second EDGAR analysis (pre-antiSMASH comparison) were 18 clusters consisting of at least three contiguous genes (data not shown), which could be evaluated further for any possible involvement in antibiotic production. There are also additional genetic regions that are common to both the EDGAR and antiSMASH gene lists that could be explored (Supplementary Tables 8, 9).
While cross-referencing genome mining and comparative genomics data can very quickly identify candidate clusters, this strategy may not always identify the genes of interest. There may be cases where there is no overlap between datasets or identified candidates are not involved in antibiotic production. Under these circumstances, clusters and genes from individual datasets could be eliminated based on the distribution and size of typical antibiotic biosynthetic gene clusters. For example, our previous studies have shown that some Pantoea natural products are not widely distributed (Walterson et al., 2014; Robinson et al., 2020; Williams and Stavrinides, 2020). This is also true for the biosynthetic gene cluster of pantocin A, which was found to be present in 5 of 45 (11%) Pantoea strains of a variety of species (Kamber et al., 2012). In the case of B025670, several candidate clusters identified by antiSMASH could be excluded due to a wide distribution and known function, such as the cluster encoding the siderophore, desferrioxamine E, which is broadly distributed across Pantoea species (Supplementary Table 6) (Soutar and Stavrinides, 2018). Unlike the antibiotic gene clusters of many Streptomyces and other bacterial species that can exceed 100 kb (Nah et al., 2017), the conditionally-observed Pantoea antibiotic clusters tend to be smaller. For example, the PNP-1 cluster of P. ananatis BRT175 has seven genes (8.2 kb) (Walterson et al., 2014), the PNP-2 cluster of P. agglomerans TX10 has six genes (5.7 kb) (Robinson et al., 2020), the PNP-3 cluster of P. agglomerans 3581 and SN01080 has eight genes (8.5 kb) (Williams and Stavrinides, 2020), the pantocin A cluster of P. agglomerans EH318 has three genes plus a precursor peptide (Jin et al., 2003b), and the herbicolin I cluster of P. vagans C9-1 is composed of ten genes (Kamber et al., 2012). While many Pantoea antibiotics are the product of small biosynthetic gene clusters, some can be larger, such the cluster responsible for andrimid synthesis in P. agglomerans EH335 that is composed of 21 genes (Jin et al., 2006). Smaller cluster sizes can be easier to overexpress, facilitating the efficient isolation and identification of the bioactive molecule. For example, heterologous expression of several Pantoea antibiotics has been successful, like pantocin A, which was discovered by cosmid expression (Wright et al., 2001; Jin et al., 2003b). More recently, the PNP-2 cluster was cloned and expressed in E. coli (Robinson et al., 2020), while heterologous expression of the four predicted biosynthetic genes of the PNP-3 cluster in E. amylovora led to antibiotic production (Williams and Stavrinides, 2020). Therefore, depending on the organism of interest, antibiotic cluster size as well as distribution can be used as criteria for filtering candidate gene cluster lists.
A combinatorial approach involving genome mining, comparative genomics, and functional genetics, is a powerful method for identifying potentially novel antibiotics. On their own, genome mining approaches have considerable utility for predicting typical or known antibiotic clusters, for assigning potential function, and even predicting the final product (Kim et al., 2017). However, these computational tools have limitations, as exemplified by their inability to predict the clusters of the PNP antibiotics, and pantocin A (Supplementary Table 7). Unlike genome mining tools, the use of comparative genomics allows for the identification of all genomic content that differs between groups of genomes, irrespective of location in the genome. This removes the bias of rule-based cluster predictions, facilitating the identification of unique and unusual antibiotic clusters as well as individual genes related to antibiotic production, thereby expanding the data available for mining tool-based cluster searching (Foulston, 2019). A comparative genomics approach, however, requires that genomes of closely related strains are available to provide a reference for comparison. While these comparisons require a minimum of two genomes, the use of numerous genomes produces a more refined list of candidates. For B025670, the increase from 2 to 11 strains for comparison led to a reduction in gene number by approximately 50%. Further, comparative genomics does not indicate whether the gene products in a cluster form a functional unit, unlike genome mining tools like antiSMASH, which can predict functional units involved in the synthesis of a specific type of secondary metabolite (Medema et al., 2011). By combining comparative genomics with the output of genome mining tools, the independent limitations of these techniques can be overcome, selecting more promising candidates that can be confirmed with functional genetics approaches such as mutagenesis or heterologous expression. Additionally, by generating independent candidate lists for comparison, our strategy prevents the exclusion of potential candidate clusters that may be missed in a step-wise procedure, such as taking the output of genome mining tools and using those sequences alone in a comparative genomics analysis. Further, this protocol is not dependent upon previous knowledge about the molecule of interest and can, therefore, be used to identify biosynthetic gene clusters for unknown compounds. Overall, expanding our repertoire of antibiotics by identifying novel biosynthetic gene clusters from underexplored sources will be critical for ensuring the availability of therapeutics for treating multi-drug resistant bacteria.
Data Availability Statement
The datasets generated for this study can be found in online repositories. The names of the repository/repositories and accession number(s) can be found in the article/Supplementary Material.
Author Contributions
AW, NS, and AC: experiments and writing of manuscript. JS: supervision, guiding project, and writing of manuscript. All authors contributed to the article and approved the submitted version.
Conflict of Interest
The authors declare that the research was conducted in the absence of any commercial or financial relationships that could be construed as a potential conflict of interest.
Acknowledgments
The authors gratefully acknowledge financial support from the Natural Sciences and Engineering Research Council of Canada (2015-06417) and the Canada Foundation for Innovation (28591). AW was supported by a Canadian Institutes of Health Research doctoral award (GSD-157908), Saskatchewan Innovation and Opportunity scholarships, and the University of Regina. We are also grateful to Dr. Derek D. N. Smith for providing helpful feedback on the manuscript. Portions of the data from this manuscript also appear in the thesis of NS (Sorout, 2019).
Supplementary Material
The Supplementary Material for this article can be found online at: https://www.frontiersin.org/articles/10.3389/fgene.2020.600116/full#supplementary-material
References
Adeolu, M., Alnajar, S., Naushad, S., and Gupta, R. S. (2016). Genome-based phylogeny and taxonomy of the ‘Enterobacteriales’: proposal for Enterobacterales ord. nov. divided into the families Enterobacteriaceae, Erwiniaceae fam. nov., Pectobacteriaceae fam. nov., Yersiniaceae fam. nov., Hafniaceae fam. nov., Morganellaceae fam. nov., and Budviciaceae fam. nov. Int. J. Syst. Evol. Microbiol. 66, 5575–5599. doi: 10.1099/ijsem.0.001485
Alanjary, M., Kronmiller, B., Adamek, M., Blin, K., Weber, T., Huson, D., et al. (2017). The Antibiotic Resistant Target Seeker (ARTS), an exploration engine for antibiotic cluster prioritization and novel drug target discovery. Nucleic Acids Res. 45, W42–W48. doi: 10.1093/nar/gkx360
Aslam, B., Wang, W., Arshad, M. I., Khurshid, M., Muzammil, S., Rasool, M. H., et al. (2018). Antibiotic resistance: a rundown of a global crisis. Infect. Drug Resist. 11, 1645–1658. doi: 10.2147/IDR.S173867
Blin, K., Shaw, S., Steinke, K., Villebro, R., Ziemert, N., Lee, S. Y., et al. (2019). antiSMASH 5.0: updates to the secondary metabolite genome mining pipeline. Nucleic Acids Res. 47, W81–W87. doi: 10.1093/nar/gkz310
Blom, J., Albaum, S. P., Doppmeier, D., Pühler, A., Vorhölter, F.-J., Zakrzewski, M., et al. (2009). EDGAR: a software framework for the comparative analysis of prokaryotic genomes. BMC Bioinform. 10:154. doi: 10.1186/1471-2105-10-154
Blom, J., Kreis, J., Spänig, S., Juhre, T., Bertelli, C., Ernst, C., et al. (2016). EDGAR 2.0: an enhanced software platform for comparative gene content analyses. Nucleic Acids Res. 44, W22–W28. doi: 10.1093/nar/gkw255
Brady, S. F., Wright, S. A., Lee, J. C., Sutton, A. E., Zumoff, C. H., Wodzinski, R. S., et al. (1999). Pantocin B, an antibiotic from Erwinia herbicola discovered by heterologous expression of cloned genes. J. Am. Chem. Soc. 121, 11912–11913. doi: 10.1021/ja992790m
Brettin, T., Davis, J. J., Disz, T., Edwards, R. A., Gerdes, S., Olsen, G. J., et al. (2015). RASTtk: a modular and extensible implementation of the RAST algorithm for building custom annotation pipelines and annotating batches of genomes. Sci. Rep. 5:8365. doi: 10.1038/srep08365
Cacho, R. A., Tang, Y., and Chooi, Y. H. (2015). Next-generation sequencing approach for connecting secondary metabolites to biosynthetic gene clusters in fungi. Front. Microbiol. 5:774. doi: 10.3389/fmicb.2014.00774
Chooi, Y. H., Cacho, R., and Tang, Y. (2010). Identification of the viridicatumtoxin and griseofulvin gene clusters from Penicillium aethiopicum. Chem. Biol. 17, 483–494. doi: 10.1016/j.chembiol.2010.03.015
Cimermancic, P., Medema, M. H., Claesen, J., Kurita, K., Wieland Brown, L. C., Mavrommatis, K., et al. (2014). Insights into secondary metabolism from a global analysis of prokaryotic biosynthetic gene clusters. Cell 158, 412–421. doi: 10.1016/j.cell.2014.06.034
Dawlaty, J., Zhang, X., Fischbach, M. A., and Clardy, J. (2010). Dapdiamides, tripeptide antibiotics formed by unconventional amide ligases. J. Nat. Prod. 73, 441–446. doi: 10.1021/np900685z
de Jong, A., van Hijum, S. A. F. T., Bijlsma, J. J. E., Kok, J., and Kuipers, O. P. (2006). BAGEL: a web-based bacteriocin genome mining tool. Nucleic Acids Res. 34, W273–W279. doi: 10.1093/nar/gkl237
de Lima Procópio, R. E., da Silva, I. R., Martins, M. K., de Azevedo, J. L., and de Araújo, J. M. (2012). Antibiotics produced by Streptomyces. Braz. J. Infect. Dis. 16, 466–471. doi: 10.1016/j.bjid.2012.08.014
Fischbach, M. A. (2009). Antibiotics from microbes: converging to kill. Curr. Opin. Microbiol. 12, 520–527. doi: 10.1016/j.mib.2009.07.002
Foulston, L. (2019). Genome mining and prospects for antibiotic discovery. Curr. Opin. Microbiol. 51, 1–8. doi: 10.1016/j.mib.2019.01.001
Freiberg, C., Brunner, N. A., Schiffer, G., Lampe, T., Pohlmann, J., Brands, M., et al. (2004). Identification and characterization of the first class of potent bacterial acetyl-CoA carboxylase inhibitors with antibacterial activity. J. Biol. Chem. 279, 26066–26073. doi: 10.1074/jbc.M402989200
Hautbergue, T., Jamin, E. L., Debrauwer, L., Puel, O., and Oswald, I. P. (2018). From genomics to metabolomics, moving toward an integrated strategy for the discovery of fungal secondary metabolites. Nat. Prod. Rep. 35, 147–173. doi: 10.1039/c7np00032d
Huo, L., Hug, J. J., Fu, C., Bian, X., Zhang, Y., and Müller, R. (2019). Heterologous expression of bacterial natural product biosynthetic pathways. Nat. Prod. Rep. 36, 1412–1436. doi: 10.1039/c8np00091c
Hutchings, M. I., Truman, A. W., and Wilkinson, B. (2019). Antibiotics: past, present and future. Curr. Opin. Microbiol. 51, 72–80. doi: 10.1016/j.mib.2019.10.008
Ishimaru, C. A., Klos, E. J., and Brubaker, R. R. (1988). Multiple antibiotic production by Erwinia herbicola. Phytopathology 78, 746–750. doi: 10.1094/Phyto-78-746
Jin, M., Fischbach, M. A., and Clardy, J. (2006). A biosynthetic gene cluster for the acetyl-CoA carboxylase inhibitor andrimid. J. Am. Chem. Soc. 128, 10660–10661. doi: 10.1021/ja063194c
Jin, M., Liu, L., Wright, S. A. I., Beer, S. V., and Clardy, J. (2003a). Structural and functional analysis of pantocin A: an antibiotic from Pantoea agglomerans discovered by heterologous expression of cloned genes. Angew. Chem. Int. Edn. 42, 2898–2901. doi: 10.1002/anie.200351053
Jin, M., Wright, S. A. I., Beer, S. V., and Clardy, J. (2003b). The biosynthetic gene cluster of pantocin A provides insights into biosynthesis and a tool for screening. Angew. Chem. Int. Edn. 42, 2902–2905. doi: 10.1002/anie.200351054
Johnson, K. B., and Stockwell, V. O. (1998). Management of fire blight: a case study in microbial ecology. Annu. Rev. Phytopathol. 36, 227–248. doi: 10.1146/annurev.phyto.36.1.227
Kamber, T., Lansdell, T. A., Stockwell, V. O., Ishimaru, C. A., Smits, T. H. M., and Duffy, B. (2012). Characterization of the biosynthetic operon for the antibacterial peptide herbicolin in Pantoea vagans biocontrol strain C9-1 and incidence in Pantoea species. Appl. Environ. Microbiol. 78, 4412–4419. doi: 10.1128/AEM.07351-11
Katz, L., and Baltz, R. H. (2016). Natural product discovery: past, present, and future. J. Ind. Microbiol. Biotechnol. 43, 155–176. doi: 10.1007/s10295-015-1723-5
Kim, H. U., Blin, K., Lee, S. Y., and Weber, T. (2017). Recent development of computational resources for new antibiotics discovery. Curr. Opin. Microbiol. 39, 113–120. doi: 10.1016/j.mib.2017.10.027
Klein, J. M., Wong, P., Loper, J. E., and Stockwell, V. O. (2017). Nutritional environment influences transcription of a pantocin A biosynthesis gene in Pantoea vagans strain C9-1. J. Plant Pathol. 99, 99–103. doi: 10.4454/jpp.v99i0.3911
Li, M. H. T., Ung, P. M. U., Zajkowski, J., Garneau-Tsodikova, S., and Sherman, D. H. (2009). Automated genome mining for natural products. BMC Bioinform. 10:185. doi: 10.1186/1471-2105-10-185
Long, R. A., Rowley, D. C., Zamora, E., Liu, J., Bartlett, D. H., and Azam, F. (2005). Antagonistic interactions among marine bacteria impede the proliferation of Vibrio cholerae. Appl. Environ. Microbiol. 71, 8531–8536. doi: 10.1128/AEM.71.12.8531-8536.2005
Matilla, M. A., Nogellova, V., Morel, B., Krell, T., and Salmond, G. P. C. (2016). Biosynthesis of the acetyl-coA carboxylase-inhibiting antibiotic, andrimid in Serratia is regulated by Hfq and the LysR-type transcriptional regulator, AdmX. Environ. Microbiol. 18, 3635–3650. doi: 10.1111/1462-2920.13241
McArthur, A. G., Waglechner, N., Nizam, F., Yan, A., Azad, M. A., Baylay, A. J., et al. (2013). The comprehensive antibiotic resistance database. Antimicrob. Agents Chemother. 57, 3348–3357. doi: 10.1128/AAC.00419-13
Medema, M. H., Blin, K., Cimermancic, P., De Jager, V., Zakrzewski, P., Fischbach, M. A., et al. (2011). antiSMASH: rapid identification, annotation and analysis of secondary metabolite biosynthesis gene clusters in bacterial and fungal genome sequences. Nucleic Acids Res. 39, W339–W346. doi: 10.1093/nar/gkr466
Medema, M. H., and Fischbach, M. A. (2015). Computational approaches to natural product discovery. Nat. Chem. Biol. 11, 639–648. doi: 10.1038/nchembio.1884
Medema, M. H., Kottmann, R., Yilmaz, P., Cummings, M., Biggins, J. B., Blin, K., et al. (2015). Minimum information about a biosynthetic gene cluster. Nat. Chem. Biol. 11, 625–631. doi: 10.1038/nchembio.1890
Melander, R. J., Zurawski, D. V., and Melander, C. (2018). Narrow-spectrum antibacterial agents. Med. Chem. Commun. 9, 12–21. doi: 10.1039/c7md00528h
Morehead, M. S., and Scarbrough, C. (2018). Emergence of global antibiotic resistance. Prim. Care 45, 467–484. doi: 10.1016/j.pop.2018.05.006
Moss, S., and Boucher, H. W. (2020). What’s hot in clinical infectious diseases? 2019 IDWeek summary. Open Forum Infect. Dis. 7:ofaa104. doi: 10.1093/ofid/ofaa104
Nah, H.-J., Pyeon, H.-R., Kang, S.-H., Choi, S.-S., and Kim, E.-S. (2017). Cloning and heterologous expression of a large-sized natural product biosynthetic gene cluster in Streptomyces species. Front. Microbiol. 8:394. doi: 10.3389/fmicb.2017.00394
Needham, J., Kelly, M. T., Ishige, M., and Andersen, R. J. (1994). Andrimid and moiramides A-C, metabolites produced in culture by a marine isolate of the bacterium Pseudomonas fluorescens: structure elucidation and biosynthesis. J. Org. Chem. 59, 2058–2063. doi: 10.1021/jo00087a020
Nikaido, H., and Takatsuka, Y. (2009). Mechanisms of RND multidrug efflux pumps. Biochim. Biophys. Acta 1794, 769–781. doi: 10.1016/j.bbapap.2008.10.004
Ochman, H., Gerber, A. S., and Hartl, D. L. (1988). Genetic applications of an inverse polymerase chain reaction. Genetics 120, 621–623.
Okrent, R. A., Trippe, K. M., Manning, V. A., and Walsh, C. M. (2018). Detection of 4-formylaminooxyvinylglycine in culture filtrates of Pseudomonas fluorescens WH6 and Pantoea ananatis BRT175 by laser ablation electrospray ionization-mass spectrometry. PLoS One 13:e0200481. doi: 10.1371/journal.pone.0200481
Patridge, E., Gareiss, P., Kinch, M. S., and Hoyer, D. (2016). An analysis of FDA-approved drugs: natural products and their derivatives. Drug Discov. Today 21, 204–207. doi: 10.1016/j.drudis.2015.01.009
Pidot, S. J., Coyne, S., Kloss, F., and Hertweck, C. (2014). Antibiotics from neglected bacterial sources. Int. J. Med. Microbiol. 304, 14–22. doi: 10.1016/j.ijmm.2013.08.011
Pusey, P. L. (2002). Biological control agents for fire blight of apple compared under conditions limiting natural dispersal. Plant Dis. 86, 639–644. doi: 10.1094/PDIS.2002.86.6.639
Reen, F. J., Romano, S., Dobson, A. D. W., and O’Gara, F. (2015). The sound of silence: activating silent biosynthetic gene clusters in marine microorganisms. Mar. Drugs 13, 4754–4783. doi: 10.3390/md13084754
Robinson, L. J., Verrett, J. N., Sorout, N., and Stavrinides, J. (2020). A broad-spectrum antibacterial natural product from the cystic fibrosis isolate, Pantoea agglomerans Tx10. Microbiol. Res. 237:126479. doi: 10.1016/j.micres.2020.126479
Rock, C. O., and Jackowski, S. (2002). Forty years of bacterial fatty acid synthesis. Biochem. Biophys. Res. Commun. 292, 1155–1166. doi: 10.1006/bbrc.2001.2022
Sammer, U. F., Reiher, K., Spiteller, D., Wensing, A., and Völksch, B. (2012). Assessment of the relevance of the antibiotic 2-amino-3-(oxirane-2,3-dicarboxamido)-propanoyl-valine from Pantoea agglomerans biological control strains against bacterial plant pathogens. Microbiol. Open 1, 438–449. doi: 10.1002/mbo3.43
Sammer, U. F., Völksch, B., Möllmann, U., Schmidtke, M., Spiteller, P., Spiteller, M., et al. (2009). 2-amino-3-(oxirane-2,3-dicarboxamido)-propanoyl-valine, an effective peptide antibiotic from the epiphyte Pantoea agglomerans 48b/90. Appl. Environ. Microbiol. 75, 7710–7717. doi: 10.1128/AEM.01244-09
Sánchez, L. A., Sierra, M. G., Siñeriz, F., and Delgado, O. (2013). Andrimid production at low temperature by a psychrotolerant Serratia proteamaculans strain. World J. Microbiol. Biotechnol. 29, 1773–1781. doi: 10.1007/s11274-013-1338-9
Seemann, T. (2014). Prokka: rapid prokaryotic genome annotation. Bioinformatics 30, 2068–2069. doi: 10.1093/bioinformatics/btu153
Singh, M. P., Mroczenski-Wildey, M. J., Steinberg, D. A., Andersen, R. J., Maiese, W. M., and Greenstein, M. (1997). Biological activity and mechanistic studies of andrimid. J. Antibiot. 50, 270–273. doi: 10.7164/antibiotics.50.270
Skinnider, M. A., Dejong, C. A., Rees, P. N., Johnston, C. W., Li, H., Webster, A. L. H., et al. (2015). Genomes to natural products PRediction informatics for secondary metabolomes (PRISM). Nucleic Acids Res. 43, 9645–9662. doi: 10.1093/nar/gkv1012
Skinnider, M. A., Merwin, N. J., Johnston, C. W., and Magarvey, N. A. (2017). PRISM 3: expanded prediction of natural product chemical structures from microbial genomes. Nucleic Acids Res. 45, W49–W54. doi: 10.1093/nar/gkx320
Smith, D. D. N., Kirzinger, M. W. B., and Stavrinides, J. (2013). Draft genome sequence of the antibiotic-producing epiphytic isolate Pantoea ananatis BRT175. Genome Announc. 1, e902–e913. doi: 10.1128/genomeA.00902-13
Smith, D. D. N., Williams, A. N., Verrett, J. N., Bergbusch, N. T., Manning, V., Trippe, K., et al. (2019). Resistance to two vinylglycine antibiotic analogs is conferred by inactivation of two separate amino acid transporters in Erwinia amylovora. J. Bacteriol. 201:e0658-18. doi: 10.1128/JB.00658-18
Smits, T. H. M., Duffy, B., Blom, J., Ishimaru, C. A., and Stockwell, V. O. (2019). Pantocin A, a peptide-derived antibiotic involved in biological control by plant-associated Pantoea species. Arch. Microbiol. 201, 713–722. doi: 10.1007/s00203-019-01647-7
Sorout, N. (2019). Identification of an Antibiotic Biosynthetic Gene Cluster in Pantoea agglomerans B025670. Master’s thesis, University of Regina, Regina, SK.
Soutar, C. D., and Stavrinides, J. (2018). The evolution of three siderophore biosynthetic clusters in environmental and host-associating strains of Pantoea. Mol. Genet. Genom. 293, 1453–1467. doi: 10.1007/s00438-018-1477-7
Stockwell, V. O., Johnson, K. B., Sugar, D., and Loper, J. E. (2002). Antibiosis contributes to biological control of fire blight by Pantoea agglomerans strain Eh252 in orchards. Phytopathology 92, 1202–1209. doi: 10.1094/PHYTO.2002.92.11.1202
Sullivan, M. J., Petty, N. K., and Beatson, S. A. (2011). Easyfig: a genome comparison visualizer. Bioinformatics 27, 1009–1010. doi: 10.1093/bioinformatics/btr039
Takeda, I., Umemura, M., Koike, H., Asai, K., and Machida, M. (2014). Motif-independent prediction of a secondary metabolism gene cluster using comparative genomics: application to sequenced genomes of Aspergillus and ten other filamentous fungal species. DNA Res. 21, 447–457. doi: 10.1093/dnares/dsu010
Tietz, J. I., and Mitchell, D. A. (2015). Using genomics for natural product structure elucidation. Curr. Top. Med. Chem. 16, 1645–1694. doi: 10.2174/1568026616666151012111439
Tuan, J. S., Weber, J. M., Staver, M. J., Leung, J. O., Donadio, S., and Katz, L. (1990). Cloning of genes involved in erythromycin biosynthesis from Saccharopolyspora erythraea using a novel actinomycete-Escherichia coli cosmid. Gene 90, 21–29. doi: 10.1016/0378-1119(90)90435-T
van Heel, A. J., de Jong, A., Song, C., Viel, J. H., Kok, J., and Kuipers, O. P. (2018). BAGEL4: a user-friendly web server to thoroughly mine RiPPs and bacteriocins. Nucleic Acids Res. 46, W278–W281. doi: 10.1093/nar/gky383
Walterson, A. M., Smith, D. D. N., and Stavrinides, J. (2014). Identification of a Pantoea biosynthetic cluster that directs the synthesis of an antimicrobial natural product. PLoS One 9:e96208. doi: 10.1371/journal.pone.0096208
Walterson, A. M., and Stavrinides, J. (2015). Pantoea: insights into a highly versatile and diverse genus within the Enterobacteriaceae. FEMS Microbiol. Rev. 39, 968–984. doi: 10.1093/femsre/fuv027
Watve, M. G., Tickoo, R., Jog, M. M., and Bhole, B. D. (2001). How many antibiotics are produced by the genus Streptomyces? Arch. Microbiol. 176, 386–390. doi: 10.1007/s002030100345
Wietz, M., Mansson, M., Gotfredsen, C. H., Larsen, T. O., and Gram, L. (2010). Antibacterial compounds from marine Vibrionaceae isolated on a global expedition. Mar. Drugs 8, 2946–2960. doi: 10.3390/md8122946
Williams, A. N., and Stavrinides, J. (2020). Pantoea natural product 3 is encoded by an eight-gene biosynthetic gene cluster and exhibits antimicrobial activity against multi-drug resistant Acinetobacter baumannii and Pseudomonas aeruginosa. Microbiol. Res. 234:126412. doi: 10.1016/j.micres.2020.126412
Wodzinski, R. S., Umholtz, T. E., Rundle, J. R., and Beer, S. V. (1994). Mechanisms of inhibition of Erwinia amylovora by Erw. herbicola in vitro and in vivo. J. Appl. Bacteriol. 76, 22–29. doi: 10.1111/j.1365-2672.1994.tb04410.x
Wolf, T., Shelest, V., Nath, N., and Shelest, E. (2016). CASSIS and SMIPS: promoter-based prediction of secondary metabolite gene clusters in eukaryotic genomes. Bioinformatics 32, 1138–1143. doi: 10.1093/bioinformatics/btv713
World Health Organization (2017). Global Priority List of Antibiotic-Resistant Bacteria to Guide Research, Discovery, and Development of New Antibiotics. Essential Medicines and Health Products, 1-7. Available online at: https://www.who.int/medicines/publications/global-priority-list-antibiotic-resistant-bacteria/en/ (accessed September 9, 2019).
Wright, S. A. I., Zumoff, C. H., Schneider, L., and Beer, S. V. (2001). Pantoea agglomerans strain EH318 produces two antibiotics that inhibit Erwinia amylovora in vitro. Appl. Environ. Microbiol. 67, 284–292. doi: 10.1128/AEM.67.1.284-292.2001
Yu, E. W., Aires, J. R., and Nikaido, H. (2003). AcrB multidrug efflux pump of Escherichia coli: composite substrate-binding cavity of exceptional flexibility generates its extremely wide substrate specificity. J. Bacteriol. 185, 5657–5664. doi: 10.1128/JB.185.19.5657-5664.2003
Zhang, X., Hindra, and Elliot, M. A. (2019). Unlocking the trove of metabolic treasures: activating silent biosynthetic gene clusters in bacteria and fungi. Curr. Opin. Microbiol. 51, 9–15. doi: 10.1016/j.mib.2019.03.003
Zhu, W., Lomsadze, A., and Borodovsky, M. (2010). Ab initio gene identification in metagenomic sequences. Nucleic Acids Res. 38:e132. doi: 10.1093/nar/gkq275
Keywords: Pantoea, secondary metabolites, biosynthetic gene cluster, agar overlay assay, genome mining, antiSMASH, comparative genomics, EDGAR 2
Citation: Williams AN, Sorout N, Cameron AJ and Stavrinides J (2020) The Integration of Genome Mining, Comparative Genomics, and Functional Genetics for Biosynthetic Gene Cluster Identification. Front. Genet. 11:600116. doi: 10.3389/fgene.2020.600116
Received: 28 August 2020; Accepted: 11 November 2020;
Published: 03 December 2020.
Edited by:
Fabia Ursula Battistuzzi, Oakland University, United StatesReviewed by:
Syed Aun Muhammad, Bahauddin Zakariya University, PakistanElena Perrin, University of Florence, Italy
Copyright © 2020 Williams, Sorout, Cameron and Stavrinides. This is an open-access article distributed under the terms of the Creative Commons Attribution License (CC BY). The use, distribution or reproduction in other forums is permitted, provided the original author(s) and the copyright owner(s) are credited and that the original publication in this journal is cited, in accordance with accepted academic practice. No use, distribution or reproduction is permitted which does not comply with these terms.
*Correspondence: John Stavrinides, john.stavrinides@uregina.ca; john.stavrinides@gmail.com