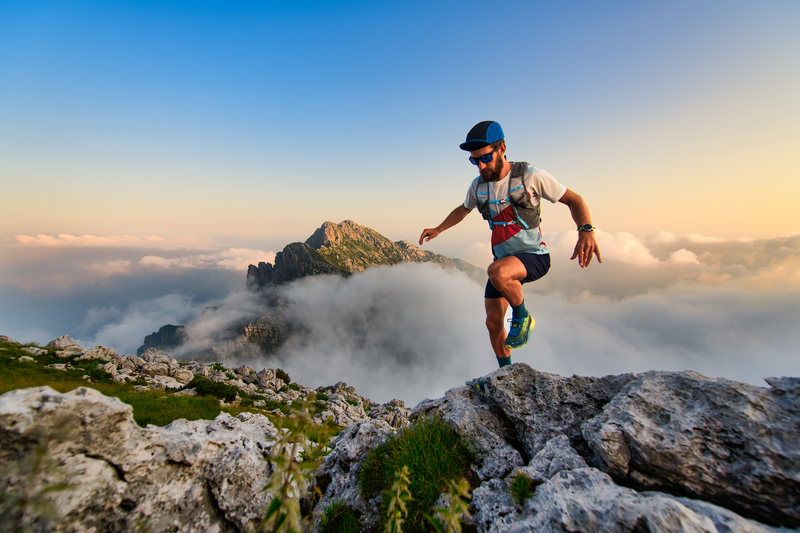
94% of researchers rate our articles as excellent or good
Learn more about the work of our research integrity team to safeguard the quality of each article we publish.
Find out more
ORIGINAL RESEARCH article
Front. Genet. , 02 December 2020
Sec. Computational Genomics
Volume 11 - 2020 | https://doi.org/10.3389/fgene.2020.596078
This article is part of the Research Topic Computational Approaches for Biomarker Detection and Precision Therapeutics in Cancers View all 21 articles
As one of the most malicious cancers, pancreatic cancer is difficult to treat due to the lack of effective early diagnosis. Therefore, it is urgent to find reliable diagnostic and predictive markers for the early detection of pancreatic cancer. In recent years, the detection of circulating cell-free DNA (cfDNA) methylation in plasma has attracted global attention for non-invasive and early cancer diagnosis. Here, we carried out a genome-wide cfDNA methylation profiling study of pancreatic ductal adenocarcinoma (PDAC) patients by methylated DNA immunoprecipitation coupled with high-throughput sequencing (MeDIP-seq). Compared with healthy individuals, 775 differentially methylated regions (DMRs) located in promoter regions were identified in PDAC patients with 761 hypermethylated and 14 hypomethylated regions; meanwhile, 761 DMRs in CpG islands (CGIs) were identified in PDAC patients with 734 hypermethylated and 27 hypomethylated regions (p-value < 0.0001). Then, 143 hypermethylated DMRs were further selected which were located in promoter regions and completely overlapped with CGIs. After performing the least absolute shrinkage and selection operator (LASSO) method, a total of eight markers were found to fairly distinguish PDAC patients from healthy individuals, including TRIM73, FAM150A, EPB41L3, SIX3, MIR663, MAPT, LOC100128977, and LOC100130148. In conclusion, this work identified a set of eight differentially methylated markers that may be potentially applied in non-invasive diagnosis of pancreatic cancer.
Pancreatic ductal adenocarcinoma (PDAC) is one of the most highly aggressive diseases in the world. Due to the hard challenge of detecting the disease at an early stage, poor prognosis often occurs. The morbidity of PDAC is approximately close to that of mortality. Nearly 80% of PDAC patients have no early symptoms before the advanced stage (Kaur et al., 2012) with a 5-year survival rate as low as 9% (Siegel et al., 2019). Accordingly, PDAC is the fourth leading cause of cancer-related death worldwide and is predicted to rise to second place by 2030 (Rahib et al., 2014). Currently, ultrasonography, computed tomography, positron emission tomography, magnetic resonance imaging, and endoscopic ultrasonography are the most commonly used diagnostic methods for PDAC (Kamisawa et al., 2016; Chu et al., 2017). However, operator experience, patient obesity and intestinal gas, and other factors affect the accuracy of diagnosis (Kamisawa et al., 2016). In addition, due to the location of the pancreas, it is not easy to make an early diagnosis compared to other digestive tract tumors (Lowenfels and Maisonneuve, 2004). Therefore, it would be very valuable to identify both sensitive and specific non-invasive biomarkers for the early diagnosis of PDAC.
Epigenetic regulation, especially DNA methylation, plays an important role in the regulation of gene expression and the development of cancers. Genome-wide hypomethylation is common in cancer cells, leading to genomic instability. Some tumor suppressor genes with promoter hypermethylation are observed to cause gene silencing (Hanahan and Weinberg, 2000; Esteller, 2007). Hypermethylation of CpG islands (CGIs) in the promoters of tumor suppressor genes is a major and early event during tumorigenesis (Hanahan and Weinberg, 2000; Park et al., 2011; Udensi and Tchounwou, 2016; Liu et al., 2019). Aberrant methylation of promoter CGI regions in some genes has been proven to be associated with tumorigenesis and tumor growth (Cai et al., 2011; Pistore et al., 2017). Therefore, it is vital to detect the hypermethylation of promoter CpG islands for early diagnosis. This may contribute to the early detection of cancer and improve the therapeutic effect.
In recent years, circulating cell-free DNA (cfDNA), known as liquid biopsy, has attracted much more attention from the medical community due to its clinical advantages. As small double-stranded DNA fragments, cfDNA is released by necrotic or apoptotic cells and is circulated in the peripheral blood (Jahr et al., 2001; Stroun et al., 2001). During tumorigenesis, the increase of cell necrosis and apoptosis leads to the accumulation of cfDNA, which can be detected at a relatively early stage. Furthermore, cfDNA not only contains the same mutations as tumor cells, but also has the same methylation pattern, making it possible and convenient for early cancer diagnosis, even for those hidden organs such as the pancreas and bile ducts (Schwarzenbach et al., 2011).
Methylated DNA immunoprecipitation coupled with high-throughput sequencing (MeDIP-seq) is a sensitive technology for the detection of DNA methylation, which can even detect an initial DNA amount as low as 1 ng (Taiwo et al., 2012; Zhao et al., 2014). Genome-wide detection of cfDNA methylation profiling using the MeDIP-seq method has been developed recently for screening potential biomarkers of cancers in early stages. Based on cfDNA methylation patterns by MeDIP-seq analysis, (Shen et al., 2018) identified different potential biomarkers in pancreatic ductal adenocarcinoma, colorectal cancer, breast cancer, lung cancer, renal cancer, bladder cancer, and acute myeloid leukemia for early-stage detection. Xu et al. (2019) also identified a set of potential biomarkers that could be served in lung cancer clinical diagnosis by screening cfDNA methylation profiling using MeDIP-seq.
Therefore, in this study, we aimed to investigate the potential cfDNA methylation biomarkers in the diagnosis of PDAC. By MeDIP-seq analysis, we compared the differentially methylated regions (DMRs) of PDAC cfDNA with that of normal control, and identified 143 hypermethylated DMRs which were located in promoter regions and completely overlapped with CGIs in PDAC patients. After cross-validation with publicly available DNA methylation data, including 339 pancreatic adenocarcinoma (PAAD) patients and 357 normal controls, we successfully identified eight probes from six differentially methylated genes, containing TRIM73, FAM150A, EPB41L3, SIX3, MIR663, MAPT, LOC100128977, and LOC100130148, which could be used as potential biomarkers for early detection for PDAC patients.
A total of six samples including four PDAC patients and two healthy controls were used for this study. Four serum samples from PDAC patients were supplied by ChangHai Hospital. All of them signed informed consent forms. Specimens were collected and analyzed with the approval of the ethics committees of ChangHai Hospital and School of Medicine, Northwest University, respectively.
First, 5 ml peripheral blood was collected using EDTA anticoagulant tubes before surgery and drug treatment. The plasma was purified by centrifuge for 15 min at 1500 × g within 6 h of collection. cfDNA was extracted from 800 μl aliquots of plasma using a QIAamp Circulating Nucleic Acid Kit (Qiagen, 55114) according to manufacturer’s protocol and quantified with Bioanalyzer 2100 (Agilent Technologies).
The cfDNA MeDIP-seq library was prepared as we described previously (Xu et al., 2019). In short, approximately 20 ng cfDNA was ligated with Illumina barcode adapters using a KAPA Hyper Prep Kit (KAPA, KK8502). The constructed cfDNA libraries were denatured at 95°C for 10 min. The methylated cfDNA was separated from the cfDNA libraries by immunoprecipitation using the 5-Methylcytosine (5mC) Monoclonal Antibody (Epigentek, A-1014). MeDIP DNA was further amplified using a Q5 High-Fidelity DNA Polymerase (NEB, M0491). After quality assessment using Bioanalyzer 2100 (Agilent Technologies), amplified libraries were subjected to deep sequencing by the Illumina HiSeq 2000 platform.
MeDIP-seq raw data were processed using the Trimmomatic software (version 0.38) to filter out low-quality reads and Illumina adapters. The clean reads were mapped to the human reference genome GRCh37/hg19 (UCSC) using the Bowtie software (version 2.3.3.1) (Langmead et al., 2009). The differentially methylated regions (DMRs) between pancreatic cancer patients and healthy controls were calculated with the R package MEDIPS (version 1.36.0) (Lienhard et al., 2014), the coupling factor for CpG density was generated based on the normalization of the patient MeDIP-seq data. The function of region of interest (ROI) analysis in the MEDIPS package was specifically used to investigate the DNA methylation levels in UCSC CpG islands, CpG shore (∼2 Kb from islands), and CpG shelf regions (∼4 Kb from islands)1. Mapping results were visualized using Integrative Genomics Viewer (IGV) (Thorvaldsdottir et al., 2013). Pathway analysis was carried with the Ingenuity Pathway Analysis (IPA) software (Qiagen).
Illumina Infinium HumanMethylation 450K BeadChip Array (HM450K) data from The Cancer Genome Atlas (TCGA) project and Gene Expression Omnibus (GEO) were used to validate our MeDIP-seq results. A total of 696 HM450K sample sets including 339 PAAD patients and 357 normal controls were assembled from the TCGA2 and GEO (GSE49149 and GSE40279) databases. The information about the patient age and gender of 696 HM450K sample sets are supplied in Supplementary Table 1. The bioinformatics pipeline and R codes are available as supplementary code in zenodo3. The variable selection was performed using the LASSO method (Xu et al., 2017). We subsampled 75% of the dataset for model building. After 500 iterations, we selected the probes that appeared more than 450 times as covariates, and obtained a total of eight probes. We fitted a logistic regression model with these candidate markers and measured the classification performance of the binary classifier using an area under the ROC curve (AUC).
The Paired Student’s t-test was performed using the processed beta (β) values (proportion of the methylated signal over the total signal) to compare the DNA methylation levels in the probe regions between 339 PAAD sample and 357 normal samples, the p-value for each maker was corrected by multiple testing with the Benjamini-Hochberg procedure (Benjamini and Yekutieli, 2001).
Multivariate Cox regression analysis was performed to construct the prognostic model based on the AIC value. Kaplan-Meier curves were generated and used to perform survival analysis using GEPIA4.
Four plasma samples of PDAC patients and two of healthy controls were collected, the clinical information of patients is shown in Table 1. The four PDAC samples were in the IB or IIB stage which had entered into the early or middle stage of pancreatic cancer (Table 1) (van Roessel et al., 2018). After being subjected to quality testing, the size of the cfDNA fragments was mainly distributed in the range of 150–200 bp with a main peak of 172 bp, which met the previous criteria where cfDNA showed a specific size of ∼167 bp (Lo et al., 2010; Thierry et al., 2010). After immunoprecipitation and amplification, the size distribution profiles of all cfDNA libraries showed a range from 172 to 292 bp with a main peak of ∼292 bp including ∼120 bp sequencing adapters (Supplementary Figure 1). The cfDNA MeDIP libraries were sequenced with Illumina HiSeq 2000 (a flow chart of the steps in the analysis is presented in Figure 1). A total of 41 million raw sequenced reads were obtained from PDAC patients, 72.7% of which was mapped to the reference genome (Human hg19), and 32 million reads from healthy controls of which 54.8% was mapped. After quality filtering, there were approximately 24 million unique reads of patients and 17 million unique reads of healthy controls (Table 2).
In order to analyze the whole-genome methylation patterns between PDAC patients and healthy controls, we performed the principal component analysis (PCA) to investigate the genome-wide methylation profiles in the two groups. The methylation patterns in PDAC patients exhibited a significant difference from the healthy control groups (Figure 2A). The unsupervised clustering analysis result further showed that there was a dramatic change in methylation patterns between PDAC patients and healthy controls (Figure 2B). This indicates that there are epigenetic differences between PDAC patients and healthy people.
Figure 2. The methylation patterns of pancreatic cancer patients and healthy controls after MeDIP-seq datasets analysis. (A) Principal component analysis (PCA) of the methylation profiles between patients and controls. (B) The unsupervised cluster analysis of the genome-wide methylation profiles in patients and controls.
A total of 5,205 differentially methylated regions (DMRs) were identified through MeDIP-seq analysis in PDAC patients (p < 0.05), which included 5,117 hypermethylated regions (98.3%) and 88 hypomethylated regions (1.7%) as shown in Supplementary Table 2. The clustering analysis also exhibited a significant alteration between PDAC patients and controls (Figure 3A). Previous studies have revealed that aberrant methylation patterns in the promoter region of tumor suppressor genes may cause transcriptional silencing which could be a driving force for cancer development (Herman and Baylin, 2003). We focused on promoter regions and recognized 775 different DMRs (p < 0.0001), including 761 hypermethylated regions (98.2%) from 532 genes and 14 hypomethylated regions (1.8%) from 14 genes (Figure 3B and Supplementary Table 3). These data suggest that most of the promoter regions are hypermethylated in pancreatic cancer samples, which is consistent with previous findings that specific hypermethylation occurring at specific promoter sites likely leads to cancer (Park et al., 2011; Liu et al., 2019; Zhang et al., 2020).
Figure 3. Differentially methylated regions (DMRs) in pancreatic cancer patients and healthy controls. (A) Heat map of total 5,205 DMRs located in the whole genome of PDAC patients compared to healthy controls, including 5,117 hypermethylated and 88 hypomethylated regions. (B) Heat map of total 775 DMRs located in the promoter regions of patients compared to healthy controls, including 761 hypermethylated and 14 hypomethylated regions.
According to the division of the CG content, some areas in the genome can be determined as CpG islands (CG content > 50%) (Gardiner-Garden and Frommer, 1987), CpG shores (up to 2 kb from CpG islands) (Irizarry et al., 2009), and CpG shelfs (≥2 kb from CpG islands) (Nones et al., 2014). It is reported that 72% of promoters are unmethylated GC-rich (Saxonov et al., 2006). Here we found that the general methylation levels of CpG regions in pancreatic cancer patients were higher than those in normal controls, which showed the median methylation levels in CGI, CpG shore, and CpG shelf to be 0.39, 0.57, and 0.5475, respectively, compared with 0.265, 0.45, and 0.41, respectively in controls (Figure 4A). Hypermethylation of CGI sites in promoter regions is considered as a risk marker for cancer development and progression (Costello et al., 2000; Esteller et al., 2001; Widschwendter and Jones, 2002), therefore, only DMR in CGIs were in focus and used for further analysis.
Figure 4. Differentially methylated regions (DMRs) of the CpG regions in pancreatic cancer patients and healthy controls. (A) Violin plots of DMRs located in CpG islands, CpG shores, and CpG shelfs of PDAC patients compared to controls. (B) Whole genomic and chromosomal location of DMRs in CGIs. (C) The different features of CGI distribution according to hypermethylated and hypomethylated regions.
A total of 761 DMRs was identified in CGIs of the whole genome in PDAC patients (p value < 0.0001). Among them, there were 734 (96.5%) hypermethylation regions from 507 genes and 27 (3.5%) hypomethylation regions from 26 genes (Supplementary Table 4). The visual DMR signals of hypermethylation and hypomethylation in CGIs mapped to the whole genome are shown in Figure 4B. The distribution features of hypermethylated and hypomethylated regions in CGIs were further classified as shown in Figure 4C. A predominant hypermethylation of DMRs in CGIs was observed, except in the 3′UTR region (Figure 4C).
It is reported that the hypermethylation of promoter CGIs is supposed to be an indicator of the risk of progression or development of cancers which is associated with the silencing of tumor suppressor genes (Feinberg, 2005; Park et al., 2011). We further screened those DMRs which were located in CGIs promoters. A total of 143 hypermethylated DMRs located in promoter regions that completely overlapped with CGIs were identified as candidate DMRs (Figure 5A). The 143 candidate DMRs were derived from 70 genes. To further understand the biological associations of the 70 genes, ingenuity pathway analysis (IPA) was performed and showed that cancer was included in the top diseases (Figure 5B).
Figure 5. Selection and definition of differentially methylated genes in both the CGIs and promoter regions. (A) Hypermethylated DMRs in the overlap of promoter regions and CGIs. (B) Top disease and bio functions by IPA analysis for genes derived from hypermethylated DMRs located in both the promoter regions and CGIs.
The 143 candidate DMRs were further annotated to 131 probes on an Illumina HM450K BeadChip Array (Supplementary Table 5) and were analyzed by the Least Absolute Shrinkage and Selection Operator (LASSO) method to select the most discriminating markers. The 75% HM450K datasets were randomly selected each time for loop modeling. Eventually, eight probes were identified as a final selection of markers which were required to appear over 450 times out of a total of 500 repetitions in the model (Table 3). To evaluate the diagnostic value of the eight markers, we built a risk prediction model in training and validation dataset using the logistic regression method. The HM450K datasets were then divided into a training cohort of 488 individuals (238 PAAD patients and 250 normal controls) and a validation cohort of 208 individuals (101 PAAD patients and 107 normal controls). The final prediction model achieved a sensitivity of 97.1% and a specificity of 98.0% on the training cohort, the sensitivity and specificity of the validating cohort was 93.2 and 95.2%, respectively (Figure 6A). This model could distinguish PAAD patients from the normal controls both in the training dataset (the area under the ROC curve, AUC = 0.975) and the validation dataset (AUC = 0.943). The prediction performance of the model in two datasets is shown in Figure 6B. To further characterize the methylation status of the eight markers in PAAD patients and normal controls, unsupervised hierarchical clustering was performed in 696 cases of the HM450K datasets (Figure 6C). The result demonstrated that these eight markers were able to distinguish PAAD patients from normal controls with high sensitivity and specificity.
Table 3. Characteristics of the eight methylation markers and their coefficients in PAAD diagnosis prediction.
Figure 6. Identification of novel pancreatic cancer diagnostic markers from cfDNA methylation analysis. (A) Confusion tables of binary results of the diagnostic prediction model in the training and validation datasets. (B) ROC of the diagnostic prediction model with methylation markers in the training and validation datasets. (C) Unsupervised hierarchical clustering of the eight methylation markers selected for use in the diagnostic prediction model.
To further address whether the eight markers we identified can distinguish pancreatic cancer patients from the healthy individuals, we next assessed the methylation levels of the eight markers in 696 cases including 339 PAAD patients and 357 normal controls. For all eight markers, there was a significantly difference in the overall methylation levels between the PAAD patients and normal controls (BH-adjusted p < 0.0001) (Figure 7). It suggested that the eight markers: MAPT, SIX3, MIR663, EPB41L3, FAM150A, TRIM73, LOC100128977, and LOC100130148 may serve as potential biomarkers for the early diagnosis of pancreatic cancer.
Figure 7. The comparison of the methylation level of the eight selected markers between pancreatic cancer patients and healthy controls.
Here, we performed a genome-wide epigenetic profiling assessment in pancreatic cancer patients for screening potential biomarkers using MeDIP-seq technology in cfDNA. Our analysis exhibited global changes in cfDNA methylation patterns in pancreatic cancer patients. In our study, we found 761 hypermethylated DMRs in promoter regions and 734 hypermethylated DMRs in CGIs derived from pancreatic cancer patients, furthermore, a total of 143 candidate DMRs were identified, located in both the promoter regions and CGIs. For subsequent analysis, tissue-derived data from TCGA and GEO was used due to the lack of cfDNA metalation data in public datasets. Finally, the diagnostic prediction model of the eight probes was established, including MAPT, SIX3, MIR663, EPB41L3, FAM150A, TRIM73, LOC100128977, and LOC100130148. Among these, MAPT, LOC100128977, and LOC100130148 are the three differentially methylated CpG sites that hit only one gene locus. The diagnostic prediction model could effectively distinguish between PAAD patients and normal controls according to both the training cohort (AUC = 0.975) and validation cohort (AUC = 0.943). These results represented promising novel methylation markers for the early diagnosis of pancreatic cancer.
To determine the prognostic value of the eight markers in pancreatic cancer patients, Kaplan–Meier survival analysis was performed (Supplementary Figure 2). Pancreatic cancer patients with a high expression of MAPT, EPB41L3, LOC100128977, and LOC100130148 had an evidently higher overall survival as compared with those with a low expression of MAPT (p = 0.0034), EPB41L3 (p = 0.0088), LOC100128977 (p = 0.0077), and LOC100130148 (p = 0.0017). However, the multivariate Cox regression analysis indicated that TRIM73, FAM150A, EPB41L3, SIX3, MAPT, LOC100128977, and LOC100130148 might not be independent factors for the prognosis of pancreatic cancer patients (Supplementary Table 6). This may indicate that gene expression is not only regulated by methylation, but also under a complex regulatory system. Therefore, these eight markers may be effective biomarkers for the diagnosis of pancreatic cancer, but they can not be used as prognostic indicators.
In recent years, there have been a few studies into the genome-wide detection of cfDNA methylation profiling using the MeDIP-seq method to screen potential tumor biomarkers. Shen et al. (2018) collected seven kinds of cancer samples for MeDIP-seq data analysis and took transcription factors into consideration while processing the biomarker analysis. Xu et al. (2019) identified hypermethylated DMRs in the promoter region for finding early diagnosis markers of lung cancer. In this study, we aimed to identify biomarkers in cfDNA which were located both in promoter regions and CGIs. CGIs are closely related to tumor epigenome, especially in promoter regions. Lay et al. (2015) demonstrated that compared to non-CGI promoters, methylation in CGI promoters had a greater impact on nucleosome phasing and histone modifications which have an influence on directing the functional organization of cancer epigenome. Tumorigenesis often coincides with CGI hypermethylation, leading to the inactivation of tumor suppressor genes (Namba et al., 2019). In a study of the genome-wide search for identifying potentially methylated changes during the progression of colorectal neoplasia, (Gu et al., 2019) found that hypermethylation occurred mainly in the overlap regions of CGIs and promoters, while hypomethylation tended to be far away from functional regions. Studies in hepatocellular carcinoma and ovarian cancer also revealed that the methylation status of some genes in the promoter and CGI regions can be used as prognosis markers for cancer patients (Dai et al., 2013; Lee et al., 2016).
Allele-specific methylation (ASM) has been well documented in imprinted loci. The parental allele 5mC asymmetry would create allele-specific imprinted differentially methylated regions (iDMRs). Moreover, it has been recently reported that some ASM loci undergo cancer-associated epigenetic changes in hematopoietic cancer. de Sa Machado Araujo et al. (2018) reported that the maternally inherited 5mCpG imprints for one gametic (PARD6GAS1) and one somatic (GCSAML) iDMRs are dysregulated in hematopoietic cancers. Among the eight methylated probes that could potentially serve as diagnosis markers in this study, we found four markers that were allele-specific methylated, including EPB41L3, SIX3, MIR663, and MAPT, suggesting that ASM also occurs in solid malignancies. Unlike whole-genome bisulfite sequencing (WGBS), which could detect the methylation state of nearly each CpG site, MeDIP technology uses an anti-methylcytosine antibody at a resolution of 100–300 bp. Therefore, MeDIP could not distinguish DNA methylation at a single base resolution (Yong et al., 2016). So ASM could not be included in the current study. Pancreatic cancer is a highly lethal disease, the lack of early detection and optional treatment is the main reason. Therefore, as a non-invasive micro diagnostic technology, cfDNA combined with MeDIP-seq is expected to be an effective method for early clinical diagnosis. In our analysis, MAPT, SIX3, MIR663, EPB41L3, FAM150A, TRIM73, LOC100128977, and LOC100130148 exhibited statistically significant differences between pancreatic cancer patients and the healthy controls (Figure 7). MAPT is a potential predictive biomarker of the efficacy of SG410, a benzoylphenylurea sulfur analog for pancreatic cancer treatment (Jimeno et al., 2007). Tumor suppressor SIX3 is reported to inhibit cell proliferation, migration, and invasion in glioblastoma and breast cancer (Zhang et al., 2017; Zheng et al., 2018; Yu et al., 2020). MIR663 could act as a tumor suppressor in gastric cancer (Pan et al., 2010) and glioblastoma (Shi et al., 2014). FAM150A is a potential prognostic marker of clear cell renal cell carcinomas (Tian et al., 2014). Taken together, these markers, which we identified in the plasma of pancreatic cancer, may have potential clinical values.
In summary, by analyzing genome-wide cfDNA methylation profiling using the MeDIP-seq method, we established a set of eight potential biomarkers which might be applied in non-invasive diagnosis of early-stage pancreatic cancer.
Publicly available datasets in the TCGA (https://portal.gdc.cancer.gov/projects/TCGA-PAAD) and GEO (GSE49149 and GSE40279) databases were used in this study. The pancreatic cancer patients’ raw data of MeDIP-seq in this study are available in the EMBL database (http://www.ebi.ac.uk/arrayexpress/) under accession number E-MTAB-9678, and the healthy controls C1 (ERS2672506, ERS2672505) and C2 (ERS2672508, ERS2672507) are available in the EMBL database under accession number E-MTAB-7163
The studies involving human participants were reviewed and approved by Ethics committees of ChangHai Hospital and School of Medicine, Northwest University. The patients/participants provided their written informed consent to participate in this study.
JT and GJ conceived the study and were in charge of the overall direction and planning. JT and SyL wrote the manuscript with input from all authors. SyL and LW collected the samples. QZ and YK performed the computational framework. SyL, LW, and QZ analyzed the data. ZW and SxL provided the technical support. JT and GJ provided the funding support. All authors reviewed the manuscript and approved the final version for publication.
This work was supported by the Shaanxi Key Industry Innovation Chain (Group) Foundation in the Social Development Field, China (2019ZDLSF02-05) and the National Key Research and Development Project (2019YFC1315904).
The authors declare that there is no conflict of interest regarding the publication of this paper.
We sincerely thank all participants in the study.
The Supplementary Material for this article can be found online at: https://www.frontiersin.org/articles/10.3389/fgene.2020.596078/full#supplementary-material
Supplementary Figure 1 | Representative bioanalyzer profiles of cfDNA and MeDIP-seq libraries.
Supplementary Figure 2 | Kaplan–Meier analysis of PAAD patients revealed the prognosis ability of seven markers.
Supplementary Table 1 | Clinical information of 696 samples from Illumina HM450K.
Supplementary Table 2 | Genome DMRs identified in cfDNA of pancreatic cancer patient plasma.
Supplementary Table 3 | Promoter DMRs identified in cfDNA of pancreatic cancer patient plasma.
Supplementary Table 4 | CGI DMRs identified in cfDNA of pancreatic cancer patient plasma.
Supplementary Table 5 | 131 Illumina HM450K BeadChip Array probes corresponding to 143 candidate DMRs.
Supplementary Table 6 | Multivariate Cox regression analysis shows the prognosis value of the seven markers.
Benjamini, Y., and Yekutieli, D. (2001). The control of the false discovery rate in multiple testing under dependency. Ann. Stat. 29, 1165–1188.
Cai, H. H., Sun, Y. M., Miao, Y., Gao, W. T., Peng, Q., Yao, J., et al. (2011). Aberrant methylation frequency of TNFRSF10C promoter in pancreatic cancer cell lines. Hepatobiliary Pancreat. Dis. Int. 10, 95–100. doi: 10.1016/s1499-3872(11)60014-3
Chu, L. C., Goggins, M. G., and Fishman, E. K. (2017). Diagnosis and detection of pancreatic cancer. Cancer J. 23, 333–342.
Costello, J. F., Fruhwald, M. C., Smiraglia, D. J., Rush, L. J., Robertson, G. P., Gao, X., et al. (2000). Aberrant CpG-island methylation has non-random and tumour-type-specific patterns. Nat. Genet. 24, 132–138. doi: 10.1038/72785
Dai, W., Zeller, C., Masrour, N., Siddiqui, N., Paul, J., and Brown, R. (2013). Promoter CpG island methylation of genes in key cancer pathways associates with clinical outcome in high-grade serous ovarian cancer. Clin. Cancer Res. 19, 5788–5797. doi: 10.1158/1078-0432.CCR-13-1217
de Sa Machado Araujo, G., Da Silva Francisco Junior, R., Dos Santos Ferreira, C., Mozer Rodrigues, P. T., Terra Machado, D., Louvain De Souza, T., et al. (2018). Maternal 5(m)CpG imprints at the PARD6G-AS1 and GCSAML differentially methylated regions are decoupled from parent-of-origin expression effects in multiple human tissues. Front. Genet. 9:36. doi: 10.3389/fgene.2018.00036
Esteller, M. (2007). Cancer epigenomics: DNA methylomes and histone-modification maps. Nat. Rev. Genet. 8, 286–298. doi: 10.1038/nrg2005
Esteller, M., Corn, P. G., Baylin, S. B., and Herman, J. G. (2001). A gene hypermethylation profile of human cancer. Cancer Res. 61, 3225–3229.
Feinberg, A. P. (2005). Cancer epigenetics is no mickey mouse. Cancer Cell 8, 267–268. doi: 10.1016/j.ccr.2005.09.014
Gardiner-Garden, M., and Frommer, M. (1987). CpG islands in vertebrate genomes. J. Mol. Biol. 196, 261–282. doi: 10.1016/0022-2836(87)90689-9
Gu, S., Lin, S., Ye, D., Qian, S., Jiang, D., Zhang, X., et al. (2019). Genome-wide methylation profiling identified novel differentially hypermethylated biomarker MPPED2 in colorectal cancer. Clin. Epigenet. 11:41. doi: 10.1186/s13148-019-0628-y
Hanahan, D., and Weinberg, R. A. (2000). The hallmarks of cancer. Cell 100, 57–70. doi: 10.1016/s0092-8674(00)81683-9
Herman, J. G., and Baylin, S. B. (2003). Gene silencing in cancer in association with promoter hypermethylation. N. Engl. J. Med. 349, 2042–2054. doi: 10.1056/NEJMra023075
Irizarry, R. A., Ladd-Acosta, C., Wen, B., Wu, Z. J., Montano, C., Onyango, P., et al. (2009). The human colon cancer methylome shows similar hypo- and hypermethylation at conserved tissue-specific CpG island shores. Nat. Genet. 41, 178–186. doi: 10.1038/ng.298
Jahr, S., Hentze, H., Englisch, S., Hardt, D., Fackelmayer, F. O., Hesch, R. D., et al. (2001). DNA fragments in the blood plasma of cancer patients: quantitations and evidence for their origin from apoptotic and necrotic cells. Cancer Res. 61, 1659–1665.
Jimeno, A., Hallur, G., Chan, A., Zhang, X., Cusatis, G., Chan, F., et al. (2007). Development of two novel benzoylphenylurea sulfur analogues and evidence that the microtubule-associated protein tau is predictive of their activity in pancreatic cancer. Mol. Cancer Ther. 6, 1509–1516. doi: 10.1158/1535-7163.MCT-06-0592
Kamisawa, T., Wood, L. D., Itoi, T., and Takaori, K. (2016). Pancreatic cancer. Lancet 388, 73–85. doi: 10.1016/s0140-6736(16)00141-0
Kaur, S., Baine, M. J., Jain, M., Sasson, A. R., and Batra, S. K. (2012). Early diagnosis of pancreatic cancer: challenges and new developments. Biomark. Med. 6, 597–612. doi: 10.2217/bmm.12.69
Langmead, B., Trapnell, C., Pop, M., and Salzberg, S. L. (2009). Ultrafast and memory-efficient alignment of short DNA sequences to the human genome. Genome Biol. 10:R25. doi: 10.1186/gb-2009-10-3-r25
Lay, F. D., Liu, Y., Kelly, T. K., Witt, H., Farnham, P. J., Jones, P. A., et al. (2015). The role of DNA methylation in directing the functional organization of the cancer epigenome. Genome Res. 25, 467–477. doi: 10.1101/gr.183368.114
Lee, H. G., Kim, H., Son, T., Jeong, Y., Kim, S. U., Dong, S. M., et al. (2016). Regulation of HK2 expression through alterations in CpG methylation of the HK2 promoter during progression of hepatocellular carcinoma. Oncotarget 7, 41798–41810. doi: 10.18632/oncotarget.9723
Lienhard, M., Grimm, C., Morkel, M., Herwig, R., and Chavez, L. (2014). MEDIPS: genome-wide differential coverage analysis of sequencing data derived from DNA enrichment experiments. Bioinformatics 30, 284–286. doi: 10.1093/bioinformatics/btt650
Liu, Y. Z., Qiao, Y. C., Zhang, H. Y., Li, W. T., and Zheng, J. (2019). Wnt7a, frequently silenced by CpG methylation, inhibits tumor growth and metastasis via suppressing epithelial-mesenchymal transition in gastric cancer. J. Cell. Biochem. 120, 18142–18151. doi: 10.1002/jcb.29118
Lo, Y. M., Chan, K. C., Sun, H., Chen, E. Z., Jiang, P., Lun, F. M., et al. (2010). Maternal plasma DNA sequencing reveals the genome-wide genetic and mutational profile of the fetus. Sci. Transl. Med. 2:61ra91. doi: 10.1126/scitranslmed.3001720
Lowenfels, A. B., and Maisonneuve, P. (2004). Epidemiology and prevention of pancreatic cancer. Jpn. J. Clin. Oncol. 34, 238–244. doi: 10.1093/jjco/hyh045
Namba, S., Sato, K., Kojima, S., Ueno, T., Yamamoto, Y., Tanaka, Y., et al. (2019). Differential regulation of CpG island methylation within divergent and unidirectional promoters in colorectal cancer. Cancer Sci. 110, 1096–1104. doi: 10.1111/cas.13937
Nones, K., Waddell, N., Song, S., Patch, A. M., Miller, D., Johns, A., et al. (2014). Genome-wide DNA methylation patterns in pancreatic ductal adenocarcinoma reveal epigenetic deregulation of SLIT-ROBO, ITGA2 and MET signaling. Int. J. Cancer 135, 1110–1118. doi: 10.1002/ijc.28765
Pan, J., Hu, H., Zhou, Z., Sun, L., Peng, L., Yu, L., et al. (2010). Tumor-suppressive mir-663 gene induces mitotic catastrophe growth arrest in human gastric cancer cells. Oncol. Rep. 24, 105–112. doi: 10.3892/or_00000834
Park, S. Y., Kwon, H. J., Lee, H. E., Ryu, H. S., Kim, S. W., Kim, J. H., et al. (2011). Promoter CpG island hypermethylation during breast cancer progression. Virchows Archiv. 458, 73–84. doi: 10.1007/s00428-010-1013-6
Pistore, C., Giannoni, E., Colangelo, T., Rizzo, F., Magnani, E., Muccillo, L., et al. (2017). DNA methylation variations are required for epithelial-to-mesenchymal transition induced by cancer-associated fibroblasts in prostate cancer cells. Oncogene 36, 5551–5566. doi: 10.1038/onc.2017.159
Rahib, L., Smith, B. D., Aizenberg, R., Rosenzweig, A. B., Fleshman, J. M., and Matrisian, L. M. (2014). Projecting cancer incidence and deaths to 2030: the unexpected burden of thyroid, liver, and pancreas cancers in the United States. Cancer Res. 74, 2913–2921. doi: 10.1158/0008-5472.CAN-14-0155
Saxonov, S., Berg, P., and Brutlag, D. L. (2006). A genome-wide analysis of CpG dinucleotides in the human genome distinguishes two distinct classes of promoters. Proc. Natl. Acad. Sci. U.S.A. 103, 1412–1417. doi: 10.1073/pnas.0510310103
Schwarzenbach, H., Hoon, D. S., and Pantel, K. (2011). Cell-free nucleic acids as biomarkers in cancer patients. Nat. Rev. Cancer 11, 426–437. doi: 10.1038/nrc3066
Shen, S. Y., Singhania, R., Fehringer, G., Chakravarthy, A., Roehrl, M. H. A., Chadwick, D., et al. (2018). Sensitive tumour detection and classification using plasma cell-free DNA methylomes. Nature 563, 579–583. doi: 10.1038/s41586-018-0703-0
Shi, Y., Chen, C., Zhang, X., Liu, Q., Xu, J. L., Zhang, H. R., et al. (2014). Primate-specific miR-663 functions as a tumor suppressor by targeting PIK3CD and predicts the prognosis of human glioblastoma. Clin. Cancer Res. 20, 1803–1813. doi: 10.1158/1078-0432.CCR-13-2284
Siegel, R. L., Miller, K. D., and Jemal, A. (2019). Cancer statistics, 2019. CA Cancer J. Clin. 69, 7–34. doi: 10.3322/caac.21551
Stroun, M., Lyautey, J., Lederrey, C., Olson-Sand, A., and Anker, P. (2001). About the possible origin and mechanism of circulating DNA apoptosis and active DNA release. Clin. Chim. Acta 313, 139–142. doi: 10.1016/s0009-8981(01)00665-9
Taiwo, O., Wilson, G. A., Morris, T., Seisenberger, S., Reik, W., Pearce, D., et al. (2012). Methylome analysis using MeDIP-seq with low DNA concentrations. Nat. Protoc. 7, 617–636. doi: 10.1038/nprot.2012.012
Thierry, A. R., Mouliere, F., Gongora, C., Ollier, J., Robert, B., Ychou, M., et al. (2010). Origin and quantification of circulating DNA in mice with human colorectal cancer xenografts. Nucleic Acids Res. 38, 6159–6175. doi: 10.1093/nar/gkq421
Thorvaldsdottir, H., Robinson, J. T., and Mesirov, J. P. (2013). Integrative genomics viewer (IGV): high-performance genomics data visualization and exploration. Brief. Bioinform. 14, 178–192. doi: 10.1093/bib/bbs017
Tian, Y., Arai, E., Gotoh, M., Komiyama, M., Fujimoto, H., and Kanai, Y. (2014). Prognostication of patients with clear cell renal cell carcinomas based on quantification of DNA methylation levels of CpG island methylator phenotype marker genes. BMC Cancer 14:772. doi: 10.1186/1471-2407-14-772
Udensi, U. K., and Tchounwou, P. B. (2016). Oxidative stress in prostate hyperplasia and carcinogenesis. J. Exp. Clin. Cancer Res. 35:139. doi: 10.1186/s13046-016-0418-8
van Roessel, S., Kasumova, G. G., Verheij, J., Najarian, R. M., Maggino, L., de Pastena, M., et al. (2018). International validation of the eighth edition of the American Joint Committee on Cancer (AJCC) TNM staging system in patients with resected pancreatic cancer. JAMA Surg. 153:e183617. doi: 10.1001/jamasurg.2018.3617
Widschwendter, M., and Jones, P. A. (2002). DNA methylation and breast carcinogenesis. Oncogene 21, 5462–5482. doi: 10.1038/sj.onc.1205606
Xu, R. H., Wei, W., Krawczyk, M., Wang, W., Luo, H., Flagg, K., et al. (2017). Circulating tumour DNA methylation markers for diagnosis and prognosis of hepatocellular carcinoma. Nat. Mater. 16, 1155–1161. doi: 10.1038/nmat4997
Xu, W., Lu, J., Zhao, Q., Wu, J., Sun, J., Han, B., et al. (2019). Genome-wide plasma cell-free DNA methylation profiling identifies potential biomarkers for lung cancer. Dis. Markers 2019:4108474. doi: 10.1155/2019/4108474
Yong, W. S., Hsu, F. M., and Chen, P. Y. (2016). Profiling genome-wide DNA methylation. Epigenet. Chromatin 9:26. doi: 10.1186/s13072-016-0075-3
Yu, Z., Feng, J., Wang, W., Deng, Z., Zhang, Y., Xiao, L., et al. (2020). The EGFR-ZNF263 signaling axis silences SIX3 in glioblastoma epigenetically. Oncogene 39, 3163–3178. doi: 10.1038/s41388-020-1206-7
Zhang, B., Shen, C., Ge, F., Ma, T., and Zhang, Z. (2017). Epigenetically controlled Six3 expression regulates glioblastoma cell proliferation and invasion alongside modulating the activation levels of WNT pathway members. J. Neurooncol. 133, 509–518. doi: 10.1007/s11060-017-2476-y
Zhang, L., Meng, X., Pan, C., Qu, F., Gan, W., Xiang, Z., et al. (2020). piR-31470 epigenetically suppresses the expression of glutathione S-transferase pi 1 in prostate cancer via DNA methylation. Cell. Signal. 67:109501. doi: 10.1016/j.cellsig.2019.109501
Zhao, M. T., Whyte, J. J., Hopkins, G. M., Kirk, M. D., and Prather, R. S. (2014). Methylated DNA immunoprecipitation and high-throughput sequencing (MeDIP-seq) using low amounts of genomic DNA. Cell Reprogram 16, 175–184. doi: 10.1089/cell.2014.0002
Keywords: pancreatic ductal adenocarcinoma, cfDNA, MeDIP-seq, methylation, biomarkers
Citation: Li S, Wang L, Zhao Q, Wang Z, Lu S, Kang Y, Jin G and Tian J (2020) Genome-Wide Analysis of Cell-Free DNA Methylation Profiling for the Early Diagnosis of Pancreatic Cancer. Front. Genet. 11:596078. doi: 10.3389/fgene.2020.596078
Received: 18 August 2020; Accepted: 05 November 2020;
Published: 02 December 2020.
Edited by:
Suman Ghosal, National Institutes of Health (NIH), United StatesReviewed by:
Cuncong Zhong, University of Kansas, United StatesCopyright © 2020 Li, Wang, Zhao, Wang, Lu, Kang, Jin and Tian. This is an open-access article distributed under the terms of the Creative Commons Attribution License (CC BY). The use, distribution or reproduction in other forums is permitted, provided the original author(s) and the copyright owner(s) are credited and that the original publication in this journal is cited, in accordance with accepted academic practice. No use, distribution or reproduction is permitted which does not comply with these terms.
*Correspondence: Jing Tian, dGlhbmppbmdAbnd1LmVkdS5jbg==; Gang Jin, SmluZ2FuZ0BzbW11LmVkbi5jbg==
†These authors have contributed equally to this work
Disclaimer: All claims expressed in this article are solely those of the authors and do not necessarily represent those of their affiliated organizations, or those of the publisher, the editors and the reviewers. Any product that may be evaluated in this article or claim that may be made by its manufacturer is not guaranteed or endorsed by the publisher.
Research integrity at Frontiers
Learn more about the work of our research integrity team to safeguard the quality of each article we publish.