- 1Department of Neurology, The First Affiliated Hospital, Sun Yat-sen University, Guangdong Provincial Key Laboratory of Diagnosis and Treatment of Major Neurological Diseases, National Key Clinical Department and Key Discipline of Neurology, Guangzhou, China
- 2Department of Neurology, The Seventh Affiliated Hospital of Sun Yat-sen University, Shenzhen, China
- 3State Key Laboratory of Quality Research in Chinese Medicine, Institute of Chinese Medical Sciences, University of Macau, Macao, China
Amyotrophic lateral sclerosis (ALS) is a neurodegenerative disorder characterized by progressive loss of motor neurons. The complex mechanisms underlying ALS are yet to be elucidated, while the lack of disease biomarkers and therapeutic options are associated with the poor prognosis of ALS patients. In this study, we performed bioinformatics analysis to clarify potential mechanisms in sporadic ALS (sALS). We compared three gene expression profiles (GSE18920, GSE56500, and GSE68605) of motor neurons obtained from sALS patients and healthy controls to discover differentially expressed genes (DEGs), and then performed integrated bioinformatics analyses to identify key molecules and pathways underlying sALS. We found that these DEGs were mainly enriched in the structure and functions of extracellular matrix (ECM), while functional enrichment in blood vessel morphogenesis was less correlated with motor neurons. The clustered subnetworks of the constructed protein-protein interaction network for DEGs and the group of selected hub genes were more significantly involved in the organization of collagen-containing ECM. The transcriptional factors database proposed RelA and NF-κB1 from NF-κB family as the key regulators of these hub genes. These results mainly demonstrated the alternations in ECM-related gene expression in motor neurons and suggested the role of NF-κB regulatory pathway in the pathogenesis of sALS.
Introduction
Amyotrophic lateral sclerosis (ALS) is a fatal disease characterized by notable degeneration in upper and lower motor neurons (Oskarsson et al., 2018). The clinical features of ALS vary in upper and lower motor neuron signs in different regions, and some patients may develop cognitive and behavioral symptoms (van Es et al., 2017; Oskarsson et al., 2018). The incidence of ALS rises in midlife and reaches the peak at the age of 70 years or above, while the prevalence has been estimated at about 4–8 in 100,000 people in most populations (Marin et al., 2018; Longinetti and Fang, 2019). Progressive weakness in muscles will lead to death eventually within 2–3 years after disease onset, mainly due to respiratory failure (Oskarsson et al., 2018). Apart from riluzole and edaravone as two widely FDA-approved drugs that can prolong the survival time for several months, ALS is still an incurable disease with limited therapeutic options (Brown and Al-Chalabi, 2017).
It is essential to explore the underlying mechanisms in ALS, but the etiology of ALS remains largely unknown. Both genetic and environmental factors play crucial roles in the pathogenesis of ALS. Since the first mutation in Cu/Zn superoxide dismutase (SOD1) gene was discovered, more than 25 ALS-related genes have been identified to date, due to the development of high-throughput DNA sequencing (Chia et al., 2018; Nguyen et al., 2018). More than 90% of ALS cases are sporadic ALS (sALS) without reported family history, while two-thirds of familial ALS (fALS) are hereditary (Renton et al., 2014). Mutations in common ALS-related genes, including SOD1, TARDBP, C9ORF72, and FUS, were found in less than 50% fALS and about 5% sALS, and genetic factors were found in only two thirds of fALS and about 10% of sALS, indicating that genetic factors cannot fully explain the pathogenesis of ALS, especially the sporadic form of ALS (Renton et al., 2014; Zou et al., 2017). Vigorous physical activity, smoking and exposure to pesticides, heavy metals, and electromagnetic fields were suspected risk factors for ALS (Zufiria et al., 2016).
The development in microarray and sequencing technology makes it possible for researchers to study diseases at DNA and RNA levels, and comprehensive analyses of multiple studies are more reliable and effective in searching for common gene targets (Kotni et al., 2016; Al-Chalabi et al., 2017). Hence, we performed integrated bioinformatics analysis among three gene expression series (GSE) from the Gene Expression Omnibus (GEO) to identify differentially expressed genes (DEGs) in motor neurons between sALS cases and healthy controls (HC). Subsequently, we conducted functional and pathway enrichment for DEGs and explored their protein-protein interactions (PPI), followed by identification of hub genes and clustered subnetworks from the PPI network, and the discovery of regulatory transcriptional factors (TFs). Our study aims to provide new insights into the pathogenesis of sALS and clues in discovering novel biomarkers and therapeutic options.
Materials and Methods
Data Collection and Processing
According to the main purpose of our study, we searched relevant gene expression profiles from the GEO database1, a public functional genomics data repository. Datasets focusing on familial ALS, in vitro studies, or studies concerning samples of motor cortex and spinal cord without the isolation of motor neurons were excluded in our analysis. At last, we collected three gene expression profiles: GSE18920, GSE56500, and GSE68605. Among them, GSE18920 and GSE56500 were based on GPL5188 platform ([HuEx-1_0-st] Affymetrix Human Exon 1.0 ST Array), while GSE68605 was based on GPL570 platform ([HG-133_Plus_2] Affymetrix Human Genome U133 Plus 2.0 Array). The original researchers obtained motor neurons through laser capture microdissection in human spinal cords, followed by extraction of total RNA and gene expression profiling with Affymatrix microarrays. Gene expression profiles of 19 neurologically HC and 19 sALS patients were included, and we excluded fALS patients in our analysis. As all gene expression profiles from GEO were public online, we did not perform any experiments on human tissues in our study. The overview of detailed information of three gene expression series selected in our study was shown in Table 1.
Identification and Analysis of DEGs
GEO2R2 was an online analysis tool to compare two or more groups of samples for DEGs by employing GEOquery and limma R packages from the Bioconductor project3. Here we utilized GEO2R to discover DEGs between sALS and HC, and Graphpad Prism 8 (GraphPad Software Inc., San Diego, CA, United States) was used for volcano plot visualization. The cut-off criteria were set at P < 0.05 and | log2Fold Change (FC)| > 1 for DEGs. An online Venn diagram was applied to discover the overlapped DEGs from all gene expression profiles4.
Functional and Pathway Enrichment Analysis of DEGs
We performed function and pathway enrichment analysis based on Metascape5, an integrated online tool for gene list annotation and biological analysis (Zhou et al., 2019). Gene ontology (GO) analysis is a common method in functional enrichment analysis, aiming to provide gene annotations in biological processes (BP), molecular functions (MF), and cellular components (CC). Kyoto Encyclopedia of Genes and Genomes (KEGG) database is crucial in understanding high-level functions and utilities of biological systems, which is especially famous for its pathway enrichment analysis. The overlapped DEGs was analyzed and visualized by Metascape with the criteria of minimum overlap > 3, P-value cut-off < 0.01, and minimum enrichment score > 1.5.
PPI Network Construction and Identification of Hub Genes
The Search Tool for the Retrieval of Interacting Genes (STRING) online database (version 11.0)6 is a common tool for predicting PPI networks with increased coverage in genome-wide experimental datasets (Szklarczyk et al., 2019). The predicted PPIs of DEGs was under the criteria at a medium confidence (minimum required interaction score > 0.4) and subsequently visualized by Cytoscape software (version 3.7.2)7, an open source software for visualization and integration of biomolecular interaction networks (Shannon et al., 2003). The Molecular Complex Detection (MCODE) plugin (version 1.6.1) was applied to discover highly connected clusters in a given network, with the analysis criteria as degree cutoff = 2, node score cutoff = 0.2, K-core = 2, and maximum depth = 100. The key nodes were selected according to the scoring of maximum correlation criterion (MCC) by using the cytoHubba plugin, which explores important nodes and subnetworks by topological algorithms. Twenty genes scoring the highest were identified as hub genes in our study.
Analysis of Hub Genes and the Regulatory Transcriptional Network
The identified clusters with more than five nodes and the selected hub genes in our study were analyzed by Metascape, respectively, for enrichment analysis. The ClueGO (version 2.5.7) and CluePedia (version 1.5.7) plugins in Cytoscape were applied for visualization of the functionally grouped networks of the hub genes. The Transcriptional Regulatory Relationships Unraveled by Sentence-based Text mining (TRRUST) (version 2)8 is a manual database of human and mouse transcriptional regulatory networks, which was utilized to discover key regulators for the hub genes in our study (Han et al., 2018). Depending on the recorded TFs-targeted genes regulatory relationships in TRRUST, we identified TFs that regulated the expression of hub genes and then visualized the regulatory network by STRING.
Results
Identification of DEGs in ALS
After the exclusion of fALS samples, three selected gene expression profiles (GSE18920, GSE56500, and GSE68605) were analyzed in our study. With the standardization of the value of gene expression and calculation by GEO2R tools, the DEGs were identified between sALS patients and HC of each series according to the filtering criteria, which were displayed in the volcano plots respectively (Figures 1A–C). A total of 3,184 DEGs were found in GSE18920, with 2,428 upregulated and 756 downregulated. In GSE56500, 4018 DEGs were found, including 2,100 upregulated and 1,918 downregulated genes. Of 2,061 DEGs found in GSE68605, 971 were upregulated and 1,090 were downregulated. In total, 206 overlapping DEGs were identified by Venn diagram and then processed for further analysis (Figure 1D).
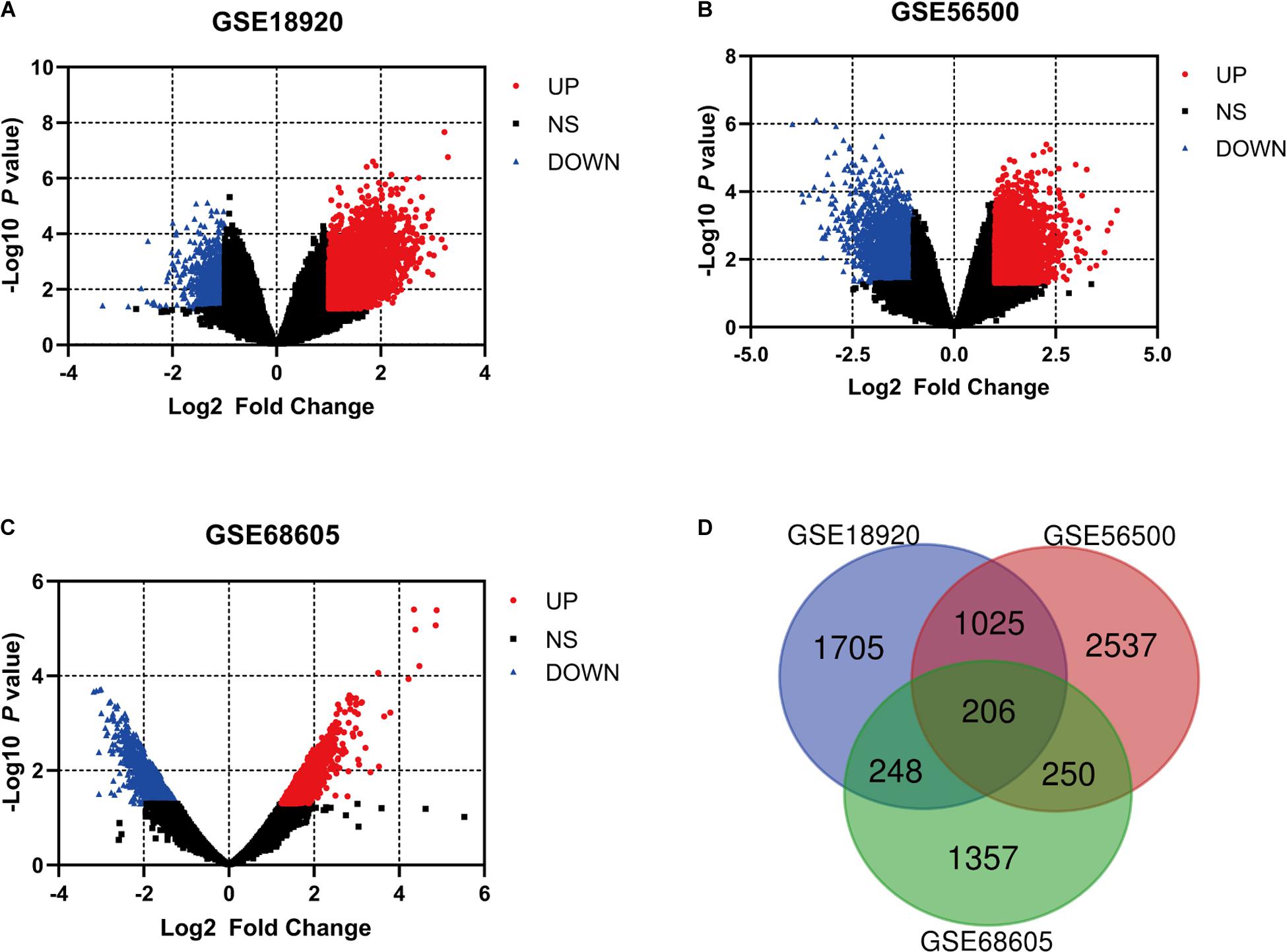
Figure 1. The analysis of differentially expressed genes (DEGs) of multiple gene expression profiles by GEO2R. (A–C) The volcano plots of the distribution of multiple gene expression series, with red dots and blue dots indicating upregulated and downregulated genes, respectively. (D) Online Venn diagram tool was applied to discover the overlapping DEGs of three datasets.
Function and Pathway Enrichment Analysis of DEGs
GO and pathway enrichment analysis of DEGs was performed by Metascape, which is useful in identifying crucial biological functions of a specific gene group. The results showed that DEGs were significantly enriched in various biological processes, including blood vessel morphogenesis, extracellular structure organization, positive regulation of hydrolase activity, and cell-substrate adhesion. DEGs were more likely to be enriched in collagen-containing extracellular matrix (ECM), platelet alpha granule, lytic vacuole, apical part of cell, and adherens junction in cellular components subgroup. In terms of molecular functions, DEGs were involved in several functions, including ECM structural constituent, enzyme activator activity, glycosaminoglycan binding, phospholipid binding, and cell adhesion molecule binding. Referring to the KEGG database, the analysis revealed enrichment mainly in pathways of complement and coagulation cascades, and ECM-receptor interaction (Figure 2). Considering the object of this study as motor neurons, the comprehensive enrichment analysis indicated crucial molecular alternation in ECM structural organization and relevant functions in sALS motor neurons.
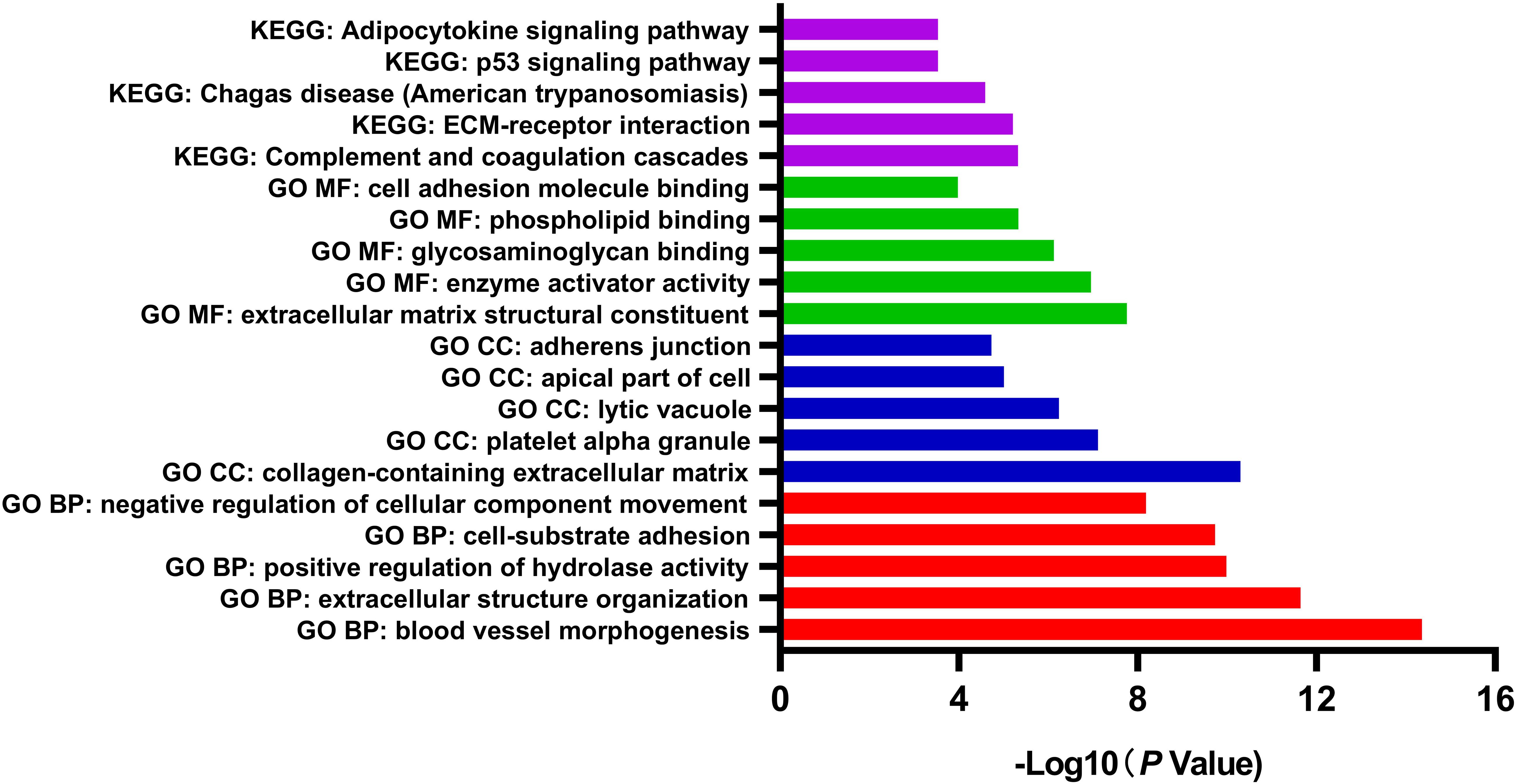
Figure 2. Gene Ontology (GO) function and pathway enrichment analysis of all differentially expressed genes by Metascape. The results were colored by different GO terms.
PPI Network Construction and Identification of Subnetworks
The protein interactions among all 206 DEGs was analyzed by STRING online database. With the confidence at medium level, the PPI network was constructed with an average node degree of 4.03 and the PPI enrichment P < 1.0 × 10–16 (Figure 3A). The obtained files were subsequently managed by Cytoscape for visualization. The analysis by MCODE plugin revealed several subnetworks in the whole DEGs network. However, only two subnetworks containing more than five nodes were identified (Figures 3B,D). The subnetwork with the highest MCODE score was significantly enriched in ECM organization, collagen-containing ECM, collagen binding, and basement membrane, while the other one was enriched in blood vessel morphogenesis, positive regulation of endocytosis, and negative regulation of epithelial cell proliferation (Figures 3C,E).
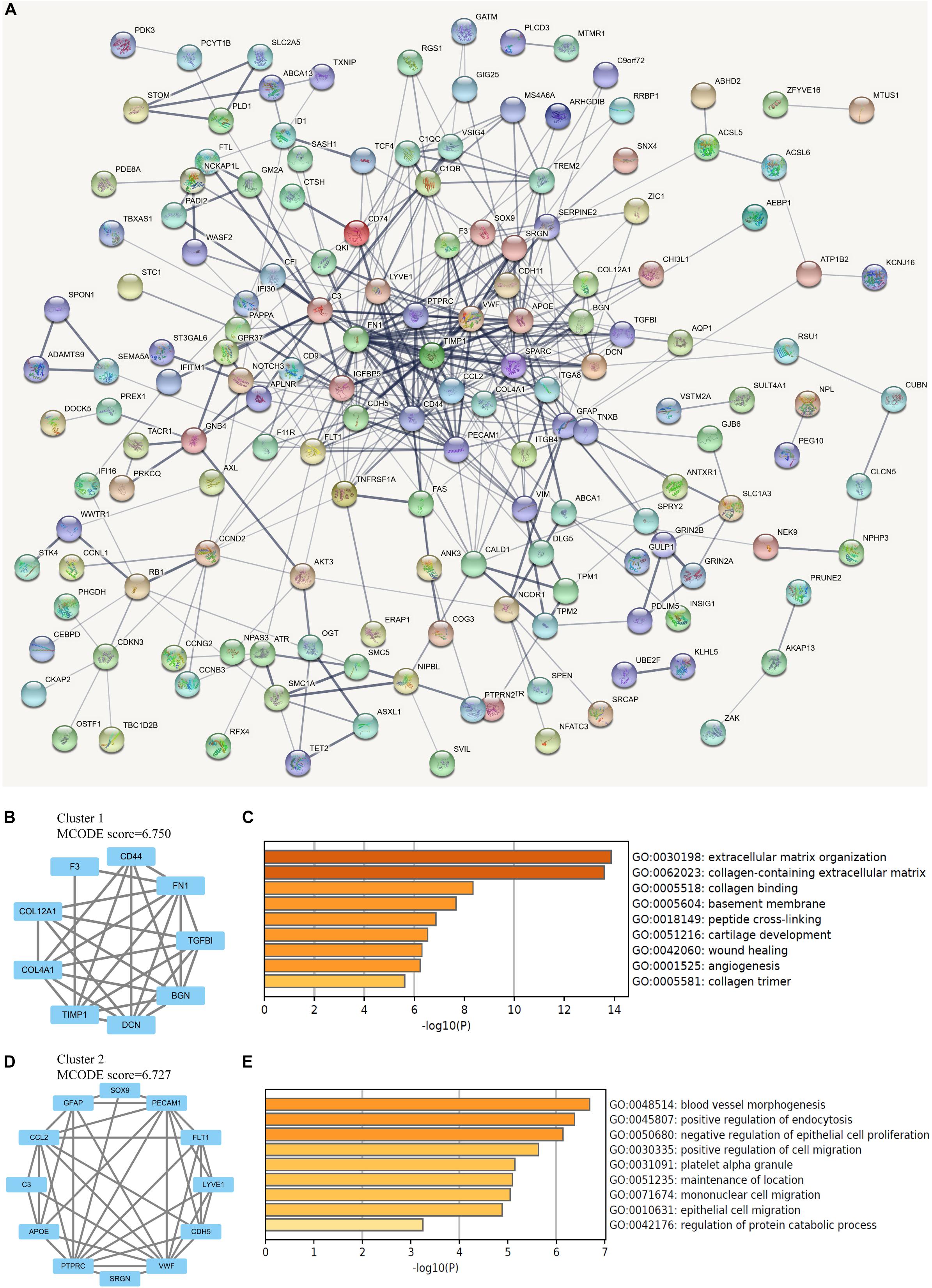
Figure 3. Network analysis of differentially expressed genes (DEGs). (A) The protein-protein interaction (PPI) network of overlapping DEGs, in which thicker line indicates stronger data support. (B) The clustered subnetwork 1 identified from the whole PPI network. (C) Enrichment analysis of the cluster 1 by Metascape. (D) The clustered subnetwork 2 identified from the whole PPI network. (E) Enrichment analysis of the cluster 2 by Metascape.
Identification of Hub Genes and Enrichment Analysis
The top 20 nodes scoring the highest in MCC by cytoHubba plugin were identified as hub genes in the network (Figure 4A). Besides, the degrees of these nodes were calculated. These hub genes were mostly upregulated in motor neurons of sALS patients in three gene expression profiles (Figure 4B). The detailed descriptions of these hub genes were shown in Table 2.
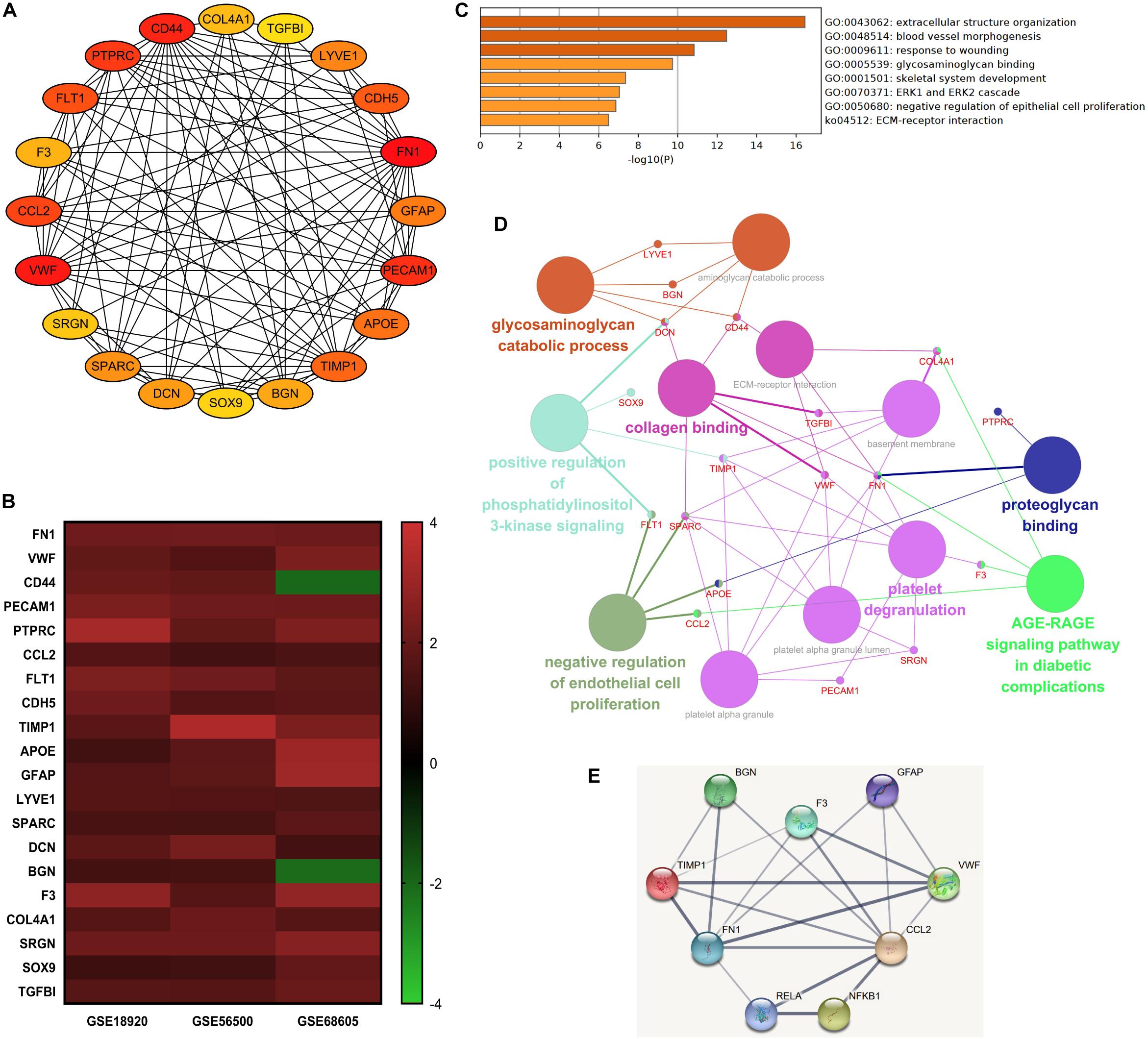
Figure 4. The integrated bioinformatics analysis on hub genes. (A) Twenty hub genes were identified by the maximum correlation criterion scoring in Cytoscape. (B) The heat map of expression levels of 20 hub genes in the original gene expression series. Red indicated higher expression level in motor neurons in sALS compared with healthy controls, while green indicated lower expression level. (C) Enrichment analysis of the identified hub genes by Metascape. (D) The visualization of the hub genes and the functional group network constructed by the ClueGO and CluePedia plugin of Cytoscape. (E) Interactions between the predicted regulatory transcriptional factors and target genes.
Gene ontology analysis of hub genes demonstrated significant enrichment in extracellular structure organization, response to wounding, glycosaminoglycan binding, and ECM-receptor interaction. But these hub genes were also associated with blood vessel morphogenesis (Figure 4C). The enrichment analysis of hub genes was similar to previous analysis of DEGs. Figure 4D visualized the association between most of these hub genes and the main enrichment GO terms by establishing a functional group network. Notably, most genes were relevant to ECM-related functions or processes, such as collagen, glycosaminoglycan, and proteoglycan binding and ECM-receptor interaction.
Regulatory Transcriptional Factors Associated With Hub Genes
Subsequently, the online TRRUST database was applied to demonstrate the regulators of the selected hub genes. The top 10 identified TFs were displayed in Table 3. We identified RelA (transcription factor p65) and NF-κB1 (transcription factor p105/p50) from NF-κB family markedly overlapped with seven genes, including BGN, CCL2, F3, FN1, GFAP, TIMP1, and VWF. The overall network of target hub genes and these two significant TFs were visualized in Figure 4E. Other TFs such as NFIC, SP1, and a pair of homologous TWIST1 and TWIST2 of the Twist family were also identified.
Discussion
Integrated Analysis Identifies ECM as the Key Regulator in sALS Motor Neurons
Bioinformatics methods directly performed on ALS motor neurons have been applied in former studies. Rabin et al. (2010) demonstrated the altered cell-matrix adhesion as well as transmembrane and secreted protein in sALS motor neurons in a single microarray analysis. The comparison of ALS patients with C9ORF72 mutation and sporadic ALS exhibited similar gene expression profiles, and a recent study highlighted the gene expression changes relevant to excitotoxicity between human oculomotor neurons and spinal motor neurons (Wong and Venkatachalam, 2019; Patel and Mathew, 2020). Although bioinformatics analysis provided insights into in-depth molecular studies, higher false positive rate and biased results were inevitable in single microarray analysis, which were due to the heterogeneity of the disease, specimen, sample size, and the platform of different microarrays to be analyzed (Wei et al., 2020). Hence, in the present study, we obtained three gene expression profiles from the public database and performed integrated bioinformatics analysis to discover genes that are differentially expressed in motor neurons from sALS patients and healthy controls. A total of 206 DEGs that are intersected in three microarray series were identified. These DEGs are significantly enriched in two parts of functions or biological processes, blood vessel morphogenesis, and extracellular structure organization. The application of STRING and Cytoscape enabled the exploration and visualization of protein-protein interactive networks, where 20 hub genes and two clustered subnetworks were identified. The two subnetworks were associated with the organization of collagen-containing ECM and blood vessel morphogenesis, respectively. These hub genes were mostly upregulated in motor neurons from sALS patients. The subsequent TF prediction proposed RelA-NF-κB1 (p65-p105/p50) as the main regulatory pathway in the hub genes network.
It was suggested by the above integrated analysis that genes relevant to both blood vessel morphogenesis and ECM were differentially expressed in sALS. But considering the study objects of our research, blood vessel morphogenesis was less likely associated with the biological processes and molecular functions of motor neurons in the spinal cord, as motor neurons were not involved in the composition of blood vessels directly. Hence, we would like to discuss more about the role of ECM and related molecules. FN1, CD44, COL4A1, and VWF were related to ECM-receptor interaction, while FN1, CD44, VWF, DCN, SPARC, and TGFBI were relevant to collagen binding process in the extracellular space. Proteoglycan binding and glycosaminoglycan catabolic process were also ECM related processes. Accounting for over 10% of the total volume of the brain between neurons and glia cells, ECM is essential in maintaining the normal functions of the central nervous system (CNS) (Benarroch, 2015). The basement membrane rich in collagen type IV, fibronectin, and laminin contributes to the integrity of brain-blood barrier (BBB), while other ECM molecules, including hyaluronic acid and multiple proteoglycans, were secreted to form ECM and perineuronal and perisynaptic nets in the extracellular space (Dityatev et al., 2010; Benarroch, 2015). Functional research has also revealed the roles of ECM in neural stem cell behavior, axonal growth, and myelination and synaptogenesis (Barros et al., 2011). Moreover, the cell adhesive network constructed by cell adhesion molecules (CAMs) provides connections between ECM molecules and intracellular components, which offers elaborate structural supports for force transmission, cytoskeletal regulation, and intercellular signaling (Kanchanawong et al., 2010; Edwards and Bix, 2019). It remained unclear whether the upregulation of these ECM related genes was the fundamental pathology of degenerated motor neurons or the secondary alternation due to other factors, but the alternation of gene expression affected the homeostasis of ECM to a certain extent.
Altered Expression of Various ECM-Related Genes in the PPI Network
Of all the upregulated hub genes enriched in ECM in the cellular component subgroup, FN1 gene scored the highest in the MCC score and node degrees, indicating strong correlations with other genes in the network. Encoded by FN1 gene, fibronectin is a kind of glycoprotein abundant in the basement membrane during embryogenesis and pathological angiogenesis in a wide range of tissues (Mongiat et al., 2016; Edwards and Bix, 2019). Fibronectin secreted by proliferating neurons and astrocytes is notably responsive in multiple neurologic disorders (Benarroch, 2015). The upregulation of fibronectin and its receptors, α5β1 and αvβ3 integrins in cerebral ischemia suggested the positive role of angiogenesis (Li et al., 2012). In multiple sclerosis (MS), the secretion of fibronectin was mediated by pro-inflammatory cytokines in a strong inflammatory process, while the aggregation of fibronectin in the white matter impaired the function of oligodendrocytes and remyelination of the lesion in MS (Stoffels et al., 2013; Werkman et al., 2020). In peripheral neuropathies, fibronectin was highly expressed in neuron regenerating sites necessary for Schwann cell differentiation and axonal regrowth, and the decreased amount of fibronectin in non-regenerating neurons was accounted for ECM degradation and leakage from disrupted basement membrane (Previtali et al., 2008). Specifically, the axon regeneration and neurite outgrowth of neurons was supported by the α5β1 integrin of fibronectin (Tonge et al., 2012). These results suggested the potential positive role of fibronectin by secreted into ECM in response to injuries. However, the role of fibronectin in the pathogenesis of ALS was still ambiguous. Fibronectin together with collagen I and III were accumulated in skeletal muscle in symptomatic hSOD1G93A mice, indicating the fibrotic process induced by the transforming growth factor-β (TGF-β) signaling pathway (Gonzalez et al., 2017). In human motor cortex, the upregulated TGF-β system also led to the enhanced expression level of FN1 and collagen IV (Peters et al., 2017). On the contrary, plasma fibronectin was found significantly lower in ALS patients and in negative correlation with duration of the disease (Ono et al., 2000). Nevertheless, the expression of fibronectin in plasma did not directly reflect the neurodegeneration in motor neurons.
Apart from FN1, the upregulated COL4A1 and COL12A1 identified in the cluster network were both genes that encode collagen related substances. COL4A1 encoding collagen IV is large molecules as the main components in the basement membrane scaffold (Yurchenco, 2011). Collagen IV deposition occurred in the perivascular space and the basement membrane in ALS patients (Garbuzova-Davis et al., 2012). Such compensatory process as response to the injury of BBB could probably hinder the drug transport into the CNS (Garbuzova-Davis et al., 2016). Collagen IV, together with laminin and vimentin, were the main components of the glial scar after injuries in the spinal cord (Saxena et al., 2012). The upregulation of collagen IV in the basal laminae correlated with the softening of the tissue, a negative factor for neuronal growth (Moeendarbary et al., 2017). On the other hand, the fibril-associated collagen XII is encoded by COL12A1 gene with two variants (Koch et al., 1995). It interacts with multiple of ECM proteins, mainly collagen I fibrils, and other components such as tenascin-X (Chiquet et al., 2014; Delbaere et al., 2020). Remarkably, the collagen XII functioned as the pro-regenerated factor in navigating the axons through non-neuronal lesion sites through the Wnt/β-catenin pathway (Wehner et al., 2017). The altered collagens expression played dual roles in the pathogenesis of ALS. In the present study, we also found the upregulation of TGFBI protein associated with collagen binding in the pathway analysis. TGFBI protein has high affinity in binding with fibronectin and functions in cell adhesion, migration, inflammation, and wound healing (Lakshminarayanan et al., 2015). Multiple studies have linked the activation of TGF-β system and other proinflammatory cytokines to the overexpression of TGFBI protein (Thapa et al., 2007). Taken together, the upregulation of FN1, COL4A1, and TGFBI genes in our study suggested that abundantly expressed fibronectin and other collagen components were associated with the degeneration of motor neurons and the fibrotic process surrounding motor neurons in the spinal cord, partly through the activation of proinflammatory TGF-β pathway.
Moreover, the upregulation of TIMP1 expression in motor neurons suggested changes in the vital dynamic balance of degradation and remodeling of ECM. This was mainly maintained by the interactions between metalloproteinases (MMPs) and tissue inhibitors of metalloproteinases (TIMPs) (Jackson et al., 2017). MMPs are crucial in the development of CNS and many physiological processes of the adult brain by degrading ECM, but the overactivation of MMPs associated with inflammatory response was discovered in multiple neurologic disorders (Singh et al., 2015; De Stefano and Herrero, 2017). As the most studied member, MMP9 was involved in the muscle degeneration process by enhancing ER stress, and its reduction rescued TDP-43 triggered death of motor neurons (Kaplan et al., 2014; Spiller et al., 2019). The upregulation of MMP9 resulted in the destruction of neurovascular unit in the presymptomatic period in ALS-G93A mice, even prior to the degeneration of motor neurons (Miyazaki et al., 2011). The proteolytic activity of MMPs was inhibited by four types of TIMPs, namely TIMP1–TIMP4 (Lukaszewicz-Zajac et al., 2014; Jackson et al., 2017). TIMP1 is secreted in the soluble form in ECM and plays the role as a strong inhibitor for MMP1, MMP3, MMP7, and MMP9 (Lukaszewicz-Zajac et al., 2014). The elevated TIMP-1 level in acute ischemic stroke patients correlated with poor prognosis, suggesting the possible compensatory mechanism to inhibit excessive MMPs activity in maintaining ECM integrity (Zhong et al., 2019). However, the specific role of TIMP-1 in ALS is still unknown, even though the upregulation of TIMP1 was found in serum, cerebrospinal fluid (CSF), and the spinal cord of ALS patients in several studies (Lim et al., 1996; Fang et al., 2009; Niebroj-Dobosz et al., 2010). It seems that the elevated level of TIMP-1 was insufficient to suppress the degradation of ECM or in a malfunction state, suggesting the role of other MMPs or TIMPs in maintaining the balance. The pathological alternation of homeostasis in ECM was hazardous to the integrity of BBB, and the mechanism is worth further exploration.
Identification of the Potential Regulatory Pathways in Motor Neurons
According to the list of hub genes, TRRUST suggested the key role of p65 and p105/p50 as the most significant prediction of the transcriptional factors, while the STRING online database demonstrated their direct interactions with seven target genes. The p65 and p105/p50 (including precursor proteins p105 and its mature form p50) are both members of NF-κB family, which is often activated in the presence of DNA damage, reactive oxygen species, and intracellular pathogens (Hayden and Ghosh, 2012). The transactivation domains (TADs) in the C-terminal enables the ability of initiating transcription in p65, while p105/p50 without TADs regulates the transcription processes by binding to TADs-containing members or other subunits (Hayden and Ghosh, 2012). The p65/p50 heterodimer is commonly regulated by IκB kinase in the NF-κB pathway (Siggers et al., 2011; Hayden and Ghosh, 2012). Recent studies revealed the complex roles of the activation of NF-κB pathway in the pathogenesis of ALS. Astrocyte-derived NF-κB activation was neuroprotective in presymptomatic stage by delaying disease onset, but it facilitated the inflammatory process by the activation of microglial, which induced motor neuron death in ALS models (Frakes et al., 2014; Ouali Alami et al., 2018). NF-κB activation in motor neurons resulted in the pathological TDP-43 aggregation in cytoplasm and nucleus as the landmark pathology in most ALS cases (Swarup et al., 2011; Picher-Martel et al., 2015). Recent studies demonstrated the fibrosis mediated by NF-κB activation in multiple diseases including cardiac hypertrophy and idiopathic pulmonary fibrosis, and the inhibitions of such pathways prevented excessive ECM deposition and remodeling (Kumar et al., 2011; Song et al., 2016). In spinal cord injury, the fibrotic scarring produced by the chronic fibrotic response limited the axons recovery in the lesion site and motor functional recovery of the mouse model, which can be reversed by eliminating the isoform of fibronectin containing the Extra Domain A (FnEDA) (Cooper et al., 2018). Similarly, FnEDA also exacerbated stroke outcome through initiating inflammation (Dhanesha et al., 2019).
On the other hand, C-C motif chemokine ligand 2 (CCL2) was also correlated with the NF-κB pathway directly in the interaction network. Also known as monocyte chemoattractant proteins 1 (MCP1), CCL2 is widely expressed in CNS and associated with the neuroinflammation process by binding to its receptor CCR2 (Conductier et al., 2010). It was previously confirmed that CCL2 (MCP1) was upregulated in serum, CSF, and peripheral blood mononuclear cell in ALS patients (Kuhle et al., 2009; Gupta et al., 2011a,b). Specifically, an early innate immune response represented by MCP1-CCR2 interaction was identified in motor cortex in mouse model, where MCP-1 were found in microglia-like cells (Jara et al., 2017). In a further research concerning motor cortex of ALS with TDP-43 pathology, the CCL2 (MCP1) expression increased in Betz cells, which was even prior to the infiltration of CCR2 positive monocytes (Jara et al., 2019). These studies were significant in proposing direct evidence for MCP1-CCR2 interaction in neuroinflammation in the motor cortex. CCL2 (MCP1) was also abundantly expressed in motor neurons in the spinal cord, and its level elevated during the disease progression in ALS mice (Kawaguchi-Niida et al., 2013). Considering the overexpression of CCL2 among the three analyzed gene expression profiles included in our study, it represented the upregulated neuroinflammation process surrounding motor neurons in sALS.
Taken together, we proposed an interaction network with several hub genes and the indicated regulatory pathways, and suggested that the dysregulation of NF-κB pathway induced by neuroinflammation contributes to the overexpression of fibronectin and a series of fibrotic processes as the major changes in the spinal cord, which could be the potential target for ALS therapy.
Limitations of the Study and Conclusion
To avoid biased results in bioinformatics analysis, we included multiple gene expression profiles of motor neurons from sALS patients and healthy controls for analysis, aiming to provide insights of the genetic patterns of the motor neurons in the advanced disease state. Nevertheless, there are still some limitations in our study. First, as the analysis on gene expression profiles in advanced state of motor neurons did not adequately explain the progression of the disease, it is still necessary to elucidate whether the upregulation of the hub genes are primary or secondary changes in the pathogenesis of sALS. Second, due to the lack of clinical data of these sALS patients, we are not able to perform survival analysis or demonstrate the association of hub genes and disease progression in sALS patients. More importantly, although we pointed out multiple upregulated molecules and related pathways in motor neurons in terminal-stage sALS, we suggest that validation from cellular, animal experiments, and even larger cohort are needed in demonstrating the overall alternation in ECM molecules during the disease progression.
In this study, the integrated bioinformatics analyses revealed the upregulation of multiple genes related to biological processes of ECM components and proposed Rela/NF-κB1 as the regulatory transcriptional factors in the pathogenesis of sALS. The bioinformatics analysis on alternation of gene expression profiles and their protein interaction networks will not only enhance our understanding of sALS but also provide insights in searching for distinct biomarkers and therapies.
Data Availability Statement
All datasets generated for this study are included in the article/supplementary material, further inquiries can be directed to the corresponding author.
Author Contributions
JL and XY contributed to the study design and management. JL and PH contributed to the data collection. PH, WC, CY, and HS contributed to the data analysis and visualization. JL contributed to writing the manuscript. HS and XY contributed to the manuscript review and editing. All authors reviewed and approved the final manuscript.
Funding
This research was funded by grants from the National Key Research and Development Program of China (2017YFC0907703), the Southern China International Cooperation Base for Early Intervention and Functional Rehabilitation of Neurological Diseases (2015B050501003), Guangdong Provincial Engineering Center for Major Neurological Disease Treatment, Guangdong Provincial Translational Medicine Innovation Platform for Diagnosis and Treatment of Major Neurological Disease, and Guangdong Provincial Clinical Research Center for Neurological Diseases.
Conflict of Interest
The authors declare that the research was conducted in the absence of any commercial or financial relationships that could be construed as a potential conflict of interest.
Footnotes
- ^ https://www.ncbi.nlm.nih.gov/geo/
- ^ https://www.ncbi.nlm.nih.gov/geo/geo2r/
- ^ http://www.bioconductor.org/
- ^ http://bioinformatics.psb.ugent.be/webtools/Venn/
- ^ https://metascape.org/
- ^ https://string-db.org/
- ^ https://cytoscape.org/index.html
- ^ https://www.grnpedia.org/trrust/
References
Al-Chalabi, A., van den Berg, L. H., and Veldink, J. (2017). Gene discovery in amyotrophic lateral sclerosis: implications for clinical management. Nat. Rev. Neurol. 13, 96–104. doi: 10.1038/nrneurol.2016.182
Barros, C. S., Franco, S. J., and Muller, U. (2011). Extracellular matrix: functions in the nervous system. Cold Spring Harb. Perspect. Biol. 3:a005108. doi: 10.1101/cshperspect.a005108
Benarroch, E. E. (2015). Extracellular matrix in the CNS: dynamic structure and clinical correlations. Neurology 85, 1417–1427. doi: 10.1212/wnl.0000000000002044
Brown, R. H., and Al-Chalabi, A. (2017). Amyotrophic lateral sclerosis. N. Engl. J. Med. 377, 162–172.
Chia, R., Chiò, A., and Traynor, B. J. (2018). Novel genes associated with amyotrophic lateral sclerosis: diagnostic and clinical implications. Lancet Neurol. 17, 94–102. doi: 10.1016/s1474-4422(17)30401-5
Chiquet, M., Birk, D. E., Bonnemann, C. G., and Koch, M. (2014). Collagen XII: protecting bone and muscle integrity by organizing collagen fibrils. Int. J. Biochem. Cell Biol. 53, 51–54. doi: 10.1016/j.biocel.2014.04.020
Conductier, G., Blondeau, N., Guyon, A., Nahon, J. L., and Rovere, C. (2010). The role of monocyte chemoattractant protein MCP1/CCL2 in neuroinflammatory diseases. J. Neuroimmunol. 224, 93–100. doi: 10.1016/j.jneuroim.2010.05.010
Cooper, J. G., Jeong, S. J., McGuire, T. L., Sharma, S., Wang, W., Bhattacharyya, S., et al. (2018). Fibronectin EDA forms the chronic fibrotic scar after contusive spinal cord injury. Neurobiol. Dis. 116, 60–68. doi: 10.1016/j.nbd.2018.04.014
De Stefano, M. E., and Herrero, M. T. (2017). The multifaceted role of metalloproteinases in physiological and pathological conditions in embryonic and adult brains. Prog. Neurobiol. 155, 36–56. doi: 10.1016/j.pneurobio.2016.08.002
Delbaere, S., Dhooge, T., Syx, D., Petit, F., Goemans, N., Destree, A., et al. (2020). Novel defects in collagen XII and VI expand the mixed myopathy/Ehlers-Danlos syndrome spectrum and lead to variant-specific alterations in the extracellular matrix. Genet. Med. 22, 112–123. doi: 10.1038/s41436-019-0599-6
Dhanesha, N., Chorawala, M. R., Jain, M., Bhalla, A., Thedens, D., Nayak, M., et al. (2019). Fn-EDA (fibronectin containing extra domain A) in the plasma, but not endothelial cells, exacerbates stroke outcome by promoting thrombo-inflammation. Stroke 50, 1201–1209. doi: 10.1161/strokeaha.118.023697
Dityatev, A., Seidenbecher, C. I., and Schachner, M. (2010). Compartmentalization from the outside: the extracellular matrix and functional microdomains in the brain. Trends Neurosci. 33, 503–512. doi: 10.1016/j.tins.2010.08.003
Edwards, D. N., and Bix, G. J. (2019). Roles of blood-brain barrier integrins and extracellular matrix in stroke. Am. J. Physiol. Cell Physiol. 316, C252–C263.
Fang, L., Huber-Abel, F., Teuchert, M., Hendrich, C., Dorst, J., Schattauer, D., et al. (2009). Linking neuron and skin: matrix metalloproteinases in amyotrophic lateral sclerosis (ALS). J. Neurol. Sci. 285, 62–66. doi: 10.1016/j.jns.2009.05.025
Frakes, A. E., Ferraiuolo, L., Haidet-Phillips, A. M., Schmelzer, L., Braun, L., Miranda, C. J., et al. (2014). Microglia induce motor neuron death via the classical NF-kappaB pathway in amyotrophic lateral sclerosis. Neuron 81, 1009–1023. doi: 10.1016/j.neuron.2014.01.013
Garbuzova-Davis, S., Hernandez-Ontiveros, D. G., Rodrigues, M. C., Haller, E., Frisina-Deyo, A., Mirtyl, S., et al. (2012). Impaired blood-brain/spinal cord barrier in ALS patients. Brain Res. 1469, 114–128. doi: 10.1016/j.brainres.2012.05.056
Garbuzova-Davis, S., Thomson, A., Kurien, C., Shytle, R. D., and Sanberg, P. R. (2016). Potential new complication in drug therapy development for amyotrophic lateral sclerosis. Expert Rev. Neurother. 16, 1397–1405. doi: 10.1080/14737175.2016.1207530
Gonzalez, D., Contreras, O., Rebolledo, D. L., Espinoza, J. P., van Zundert, B., and Brandan, E. (2017). ALS skeletal muscle shows enhanced TGF-beta signaling, fibrosis and induction of fibro/adipogenic progenitor markers. PLoS One 12:e0177649. doi: 10.1371/journal.pone.0177649
Gupta, P. K., Prabhakar, S., Abburi, C., Sharma, N. K., and Anand, A. (2011a). Vascular endothelial growth factor-A and chemokine ligand (CCL2) genes are upregulated in peripheral blood mononuclear cells in Indian amyotrophic lateral sclerosis patients. J. Neuroinflammation 8:114. doi: 10.1186/1742-2094-8-114
Gupta, P. K., Prabhakar, S., Sharma, S., and Anand, A. (2011b). Vascular endothelial growth factor-A (VEGF-A) and chemokine ligand-2 (CCL2) in amyotrophic lateral sclerosis (ALS) patients. J. Neuroinflammation 8:47. doi: 10.1186/1742-2094-8-47
Han, H., Cho, J.-W., Lee, S., Yun, A., Kim, H., Bae, D., et al. (2018). TRRUST v2: an expanded reference database of human and mouse transcriptional regulatory interactions. Nucleic Acids Res. 46, D380–D386.
Hayden, M. S., and Ghosh, S. (2012). NF-κB, the first quarter-century: remarkable progress and outstanding questions. Genes Dev. 26, 203–234. doi: 10.1101/gad.183434.111
Jackson, H. W., Defamie, V., Waterhouse, P., and Khokha, R. (2017). TIMPs: versatile extracellular regulators in cancer. Nat. Rev. Cancer 17, 38–53. doi: 10.1038/nrc.2016.115
Jara, J. H., Gautam, M., Kocak, N., Xie, E. F., Mao, Q., Bigio, E. H., et al. (2019). MCP1-CCR2 and neuroinflammation in the ALS motor cortex with TDP-43 pathology. J. Neuroinflammation 16:196.
Jara, J. H., Genc, B., Stanford, M. J., Pytel, P., Roos, R. P., Weintraub, S., et al. (2017). Evidence for an early innate immune response in the motor cortex of ALS. J. Neuroinflammation 14:129.
Kanchanawong, P., Shtengel, G., Pasapera, A. M., Ramko, E. B., Davidson, M. W., Hess, H. F., et al. (2010). Nanoscale architecture of integrin-based cell adhesions. Nature 468, 580–584. doi: 10.1038/nature09621
Kaplan, A., Spiller, K. J., Towne, C., Kanning, K. C., Choe, G. T., Geber, A., et al. (2014). Neuronal matrix metalloproteinase-9 is a determinant of selective neurodegeneration. Neuron 81, 333–348. doi: 10.1016/j.neuron.2013.12.009
Kawaguchi-Niida, M., Yamamoto, T., Kato, Y., Inose, Y., and Shibata, N. (2013). MCP-1/CCR2 signaling-mediated astrocytosis is accelerated in a transgenic mouse model of SOD1-mutated familial ALS. Acta Neuropathol. Commun. 1:21.
Koch, M., Bohrmann, B., Matthison, M., Hagios, C., Trueb, B., and Chiquet, M. (1995). Large and small splice variants of collagen XII: differential expression and ligand binding. J. Cell Biol. 130, 1005–1014. doi: 10.1083/jcb.130.4.1005
Kotni, M. K., Zhao, M., and Wei, D. Q. (2016). Gene expression profiles and protein-protein interaction networks in amyotrophic lateral sclerosis patients with C9orf72 mutation. Orphanet J. Rare Dis. 11:148.
Kuhle, J., Lindberg, R. L. P., Regeniter, A., Mehling, M., Steck, A. J., Kappos, L., et al. (2009). Increased levels of inflammatory chemokines in amyotrophic lateral sclerosis. Eur. J. Neurol. 16, 771–774. doi: 10.1111/j.1468-1331.2009.02560.x
Kumar, S., Seqqat, R., Chigurupati, S., Kumar, R., Baker, K. M., Young, D., et al. (2011). Inhibition of nuclear factor kappaB regresses cardiac hypertrophy by modulating the expression of extracellular matrix and adhesion molecules. Free Radic. Biol. Med. 50, 206–215. doi: 10.1016/j.freeradbiomed.2010.10.711
Lakshminarayanan, R., Chaurasia, S. S., Murugan, E., Venkatraman, A., Chai, S. M., Vithana, E. N., et al. (2015). Biochemical properties and aggregation propensity of transforming growth factor-induced protein (TGFBIp) and the amyloid forming mutants. Ocul. Surf. 13, 9–25. doi: 10.1016/j.jtos.2014.04.003
Li, L., Liu, F., Welser-Alves, J. V., McCullough, L. D., and Milner, R. (2012). Upregulation of fibronectin and the α5β1 and αvβ3 integrins on blood vessels within the cerebral ischemic penumbra. Exp. Neurol. 233, 283–291. doi: 10.1016/j.expneurol.2011.10.017
Lim, G. P., Backstrom, J. R., Cullen, M. J., Miller, C. A., Atkinson, R. D., and Tökés, Z. A. (1996). Matrix metalloproteinases in the neocortex and spinal cord of amyotrophic lateral sclerosis patients. J. Neurochem. 67, 251–259. doi: 10.1046/j.1471-4159.1996.67010251.x
Longinetti, E., and Fang, F. (2019). Epidemiology of amyotrophic lateral sclerosis: an update of recent literature. Curr. Opin. Neurol. 32, 771–776. doi: 10.1097/wco.0000000000000730
Lukaszewicz-Zajac, M., Mroczko, B., and Slowik, A. (2014). Matrix metalloproteinases (MMPs) and their tissue inhibitors (TIMPs) in amyotrophic lateral sclerosis (ALS). J. Neural Transm. (Vienna). 121, 1387–1397. doi: 10.1007/s00702-014-1205-3
Marin, B., Fontana, A., Arcuti, S., Copetti, M., Boumediene, F., Couratier, P., et al. (2018). Age-specific ALS incidence: a dose-response meta-analysis. Eur. J. Epidemiol. 33, 621–634. doi: 10.1007/s10654-018-0392-x
Miyazaki, K., Ohta, Y., Nagai, M., Morimoto, N., Kurata, T., Takehisa, Y., et al. (2011). Disruption of neurovascular unit prior to motor neuron degeneration in amyotrophic lateral sclerosis. J. Neurosci. Res. 89, 718–728. doi: 10.1002/jnr.22594
Moeendarbary, E., Weber, I. P., Sheridan, G. K., Koser, D. E., Soleman, S., Haenzi, B., et al. (2017). The soft mechanical signature of glial scars in the central nervous system. Nat. Commun. 8:14787.
Mongiat, M., Andreuzzi, E., Tarticchio, G., and Paulitti, A. (2016). Extracellular matrix, a hard player in angiogenesis. Int. J. Mol. Sci. 17:1822. doi: 10.3390/ijms17111822
Nguyen, H. P., Van Broeckhoven, C., and van der Zee, J. A. L. S. (2018). Genes in the Genomic Era and their Implications for FTD. Trends Genet. 34, 404–423. doi: 10.1016/j.tig.2018.03.001
Niebroj-Dobosz, I., Janik, P., Sokolowska, B., and Kwiecinski, H. (2010). Matrix metalloproteinases and their tissue inhibitors in serum and cerebrospinal fluid of patients with amyotrophic lateral sclerosis. Eur. J. Neurol. 17, 226–231. doi: 10.1111/j.1468-1331.2009.02775.x
Ono, S., Imai, T., Shimizu, N., Nakayama, M., Mihori, A., Kaneda, K., et al. (2000). Decreased plasma levels of fibronectin in amyotrophic lateral sclerosis. Acta Neurol. Scand. 101, 391–394. doi: 10.1034/j.1600-0404.2000.90289a.x
Oskarsson, B., Gendron, T. F., and Staff, N. P. (2018). Amyotrophic lateral sclerosis: an update for 2018. Mayo Clin. Proc. 93, 1617–1628.
Ouali Alami, N., Schurr, C., Olde Heuvel, F., Tang, L., Li, Q., Tasdogan, A., et al. (2018). NF-kappaB activation in astrocytes drives a stage-specific beneficial neuroimmunological response in ALS. EMBO J. 37:e98697.
Patel, A. N., and Mathew, D. (2020). A study of gene expression changes in human spinal and oculomotor neurons; identifying potential links to sporadic ALS. Genes (Basel) 11:448. doi: 10.3390/genes11040448
Peters, S., Zitzelsperger, E., Kuespert, S., Iberl, S., Heydn, R., Johannesen, S., et al. (2017). The TGF-beta system as a potential pathogenic player in disease modulation of amyotrophic lateral sclerosis. Front. Neurol. 8:669.
Picher-Martel, V., Dutta, K., Phaneuf, D., Sobue, G., and Julien, J.-P. (2015). Ubiquilin-2 drives NF-κB activity and cytosolic TDP-43 aggregation in neuronal cells. Mol. Brain 8:71.
Previtali, S. C., Malaguti, M. C., Riva, N., Scarlato, M., Dacci, P., Dina, G., et al. (2008). The extracellular matrix affects axonal regeneration in peripheral neuropathies. Neurology 71, 322–331. doi: 10.1212/01.wnl.0000319736.43628.04
Rabin, S. J., Kim, J. M. H., Baughn, M., Libby, R. T., Kim, Y. J., Fan, Y., et al. (2010). Sporadic ALS has compartment-specific aberrant exon splicing and altered cell-matrix adhesion biology. Hum. Mol. Genet. 19, 313–328. doi: 10.1093/hmg/ddp498
Renton, A. E., Chio, A., and Traynor, B. J. (2014). State of play in amyotrophic lateral sclerosis genetics. Nat. Neurosci. 17, 17–23.
Saxena, T., Gilbert, J., Stelzner, D., and Hasenwinkel, J. (2012). Mechanical characterization of the injured spinal cord after lateral spinal hemisection injury in the rat. J. Neurotrauma 29, 1747–1757. doi: 10.1089/neu.2011.1818
Shannon, P., Markiel, A., Ozier, O., Baliga, N. S., Wang, J. T., Ramage, D., et al. (2003). Cytoscape: a software environment for integrated models of biomolecular interaction networks. Genome Res. 13, 2498–2504. doi: 10.1101/gr.1239303
Siggers, T., Chang, A. B., Teixeira, A., Wong, D., Williams, K. J., Ahmed, B., et al. (2011). Principles of dimer-specific gene regulation revealed by a comprehensive characterization of NF-kappaB family DNA binding. Nat. Immunol. 13, 95–102. doi: 10.1038/ni.2151
Singh, D., Srivastava, S. K., Chaudhuri, T. K., and Upadhyay, G. (2015). Multifaceted role of matrix metalloproteinases (MMPs). Front. Mol. Biosci. 2:19.
Song, C., He, L., Zhang, J., Ma, H., Yuan, X., Hu, G., et al. (2016). Fluorofenidone attenuates pulmonary inflammation and fibrosis via inhibiting the activation of NALP3 inflammasome and IL-1beta/IL-1R1/MyD88/NF-kappaB pathway. J. Cell Mol. Med. 20, 2064–2077. doi: 10.1111/jcmm.12898
Spiller, K. J., Khan, T., Dominique, M. A., Restrepo, C. R., Cotton-Samuel, D., Levitan, M., et al. (2019). Reduction of matrix metalloproteinase 9 (MMP-9) protects motor neurons from TDP-43-triggered death in rNLS8 mice. Neurobiol. Dis. 124, 133–140. doi: 10.1016/j.nbd.2018.11.013
Stoffels, J. M., de Jonge, J. C., Stancic, M., Nomden, A., van Strien, M. E., Ma, D., et al. (2013). Fibronectin aggregation in multiple sclerosis lesions impairs remyelination. Brain 136(Pt 1), 116–131. doi: 10.1093/brain/aws313
Swarup, V., Phaneuf, D., Dupre, N., Petri, S., Strong, M., Kriz, J., et al. (2011). Deregulation of TDP-43 in amyotrophic lateral sclerosis triggers nuclear factor kappaB-mediated pathogenic pathways. J. Exp. Med. 208, 2429–2447. doi: 10.1084/jem.20111313
Szklarczyk, D., Gable, A. L., Lyon, D., Junge, A., Wyder, S., Huerta-Cepas, J., et al. (2019). STRING v11: protein-protein association networks with increased coverage, supporting functional discovery in genome-wide experimental datasets. Nucleic Acids Res. 47, D607–D613.
Thapa, N., Lee, B. H., and Kim, I. S. (2007). TGFBIp/betaig-h3 protein: a versatile matrix molecule induced by TGF-beta. Int. J. Biochem. Cell Biol. 39, 2183–2194. doi: 10.1016/j.biocel.2007.06.004
Tonge, D. A., de Burgh, H. T., Docherty, R., Humphries, M. J., Craig, S. E., and Pizzey, J. (2012). Fibronectin supports neurite outgrowth and axonal regeneration of adult brain neurons in vitro. Brain Res. 1453, 8–16. doi: 10.1016/j.brainres.2012.03.024
van Es, M. A., Hardiman, O., Chio, A., Al-Chalabi, A., Pasterkamp, R. J., Veldink, J. H., et al. (2017). Amyotrophic lateral sclerosis. Lancet 390, 2084–2098.
Wehner, D., Tsarouchas, T. M., Michael, A., Haase, C., Weidinger, G., Reimer, M. M., et al. (2017). Wnt signaling controls pro-regenerative Collagen XII in functional spinal cord regeneration in zebrafish. Nat. Commun. 8:126.
Wei, R., Qiu, H., Xu, J., Mo, J., Liu, Y., Gui, Y., et al. (2020). Expression and prognostic potential of GPX1 in human cancers based on data mining. Ann. Transl. Med. 8:124. doi: 10.21037/atm.2020.02.36
Werkman, I., Sikkema, A. H., Versluijs, J. B., Qin, J., de Boer, P., and Baron, W. (2020). TLR3 agonists induce fibronectin aggregation by activated astrocytes: a role of pro-inflammatory cytokines and fibronectin splice variants. Sci. Rep. 10:532.
Wong, C.-O., and Venkatachalam, K. (2019). Motor neurons from ALS patients with mutations in C9ORF72 and SOD1 exhibit distinct transcriptional landscapes. Hum. Mol. Genet. 28, 2799–2810. doi: 10.1093/hmg/ddz104
Yurchenco, P. D. (2011). Basement membranes: cell scaffoldings and signaling platforms. Cold Spring Harb. Perspect. Biol. 3:a004911. doi: 10.1101/cshperspect.a004911
Zhong, C., Wang, G., Xu, T., Zhu, Z., Guo, D., Zheng, X., et al. (2019). Tissue inhibitor metalloproteinase-1 and clinical outcomes after acute ischemic stroke. Neurology 93, e1675–e1685.
Zhou, Y., Zhou, B., Pache, L., Chang, M., Khodabakhshi, A. H., Tanaseichuk, O., et al. (2019). Metascape provides a biologist-oriented resource for the analysis of systems-level datasets. Nat. Commun. 10:1523.
Zou, Z. Y., Zhou, Z. R., Che, C. H., Liu, C. Y., He, R. L., and Huang, H. P. (2017). Genetic epidemiology of amyotrophic lateral sclerosis: a systematic review and meta-analysis. J. Neurol. Neurosurg. Psychiatry. 88, 540–549.
Keywords: sporadic amyotrophic lateral sclerosis, gene expression profiles, motor neurons, bioinformatics analysis, FN1 gene
Citation: Lin J, Huang P, Chen W, Ye C, Su H and Yao X (2020) Key Molecules and Pathways Underlying Sporadic Amyotrophic Lateral Sclerosis: Integrated Analysis on Gene Expression Profiles of Motor Neurons. Front. Genet. 11:578143. doi: 10.3389/fgene.2020.578143
Received: 30 June 2020; Accepted: 05 October 2020;
Published: 09 November 2020.
Edited by:
Henry Houlden, University College London, United KingdomReviewed by:
Subhabrata Sanyal, California Life Company (Calico), United StatesRossen Donev, MicroPharm Ltd., United Kingdom
Copyright © 2020 Lin, Huang, Chen, Ye, Su and Yao. This is an open-access article distributed under the terms of the Creative Commons Attribution License (CC BY). The use, distribution or reproduction in other forums is permitted, provided the original author(s) and the copyright owner(s) are credited and that the original publication in this journal is cited, in accordance with accepted academic practice. No use, distribution or reproduction is permitted which does not comply with these terms.
*Correspondence: Xiaoli Yao, yaoxiaol@mail.sysu.edu.cn