- 1Division of Gastroenterology and Hepatology, Key Laboratory of Gastroenterology and Hepatology, Ministry of Health, Inflammatory Bowel Disease Research Center, Renji Hospital, School of Medicine, Shanghai Jiao Tong University, Shanghai Institute of Digestive Disease, Shanghai, China
- 2Department of Radiology, Renji Hospital, School of Medicine, Shanghai Jiao Tong University, Shanghai, China
Ulcerative colitis (UC) and rheumatoid arthritis (RA) are immune-mediated inflammatory diseases (IMIDs) with similar symptoms and common genomics. However, the relationship between UC and RA has not been investigated thoroughly. Therefore, this study aimed to establish the differentially expressed genes (DEGs) and potential therapeutic targets in UC and RA. Three microarray datasets (GSE38713, GSE1919, and GSE12251) were selected from the Gene Expression Omnibus (GEO) database for analysis. We used R software to identify the DEGs and performed enrichment analyses. Search Tool for the Retrieval of Interacting Genes/Proteins (STRING) and Cytoscape software were used to construct the protein-protein interaction (PPI) network and identify the hub genes. A regulatory network based on the constructed PPI was generated using StarBase and PROMO databases. We identified a total of 1542 and 261 DEGs in UC and RA. There were 169 common DEGs identified in both UC and RA, including 63 upregulated genes (DEGs1) and nine downregulated genes (DEGs2). The Gene Ontology (GO) and Kyoto Encyclopedia of Genes and Genomes (KEGG) pathway analyses of DEGs1 and DEGs2 in the PPI network revealed that the genes enriched were involved in immunity. A total of 45 hub genes were selected based on high scores of correlation; three hub genes (SRGN, PLEK, and FCGR3B) were found to be upregulated in UC and RA, and downregulated in UC patients with response to infliximab treatment. The identification of novel DEGs and hub genes in the current study contributes to a novel perception for latent functional mechanisms and presents potential prognostic indicators and therapeutic targets in UC and RA.
Introduction
Ulcerative colitis (UC) is a chronic inflammatory disease that mainly involves the colon. The incidence and prevalence of UC have increased worldwide, thus placing a significant burden on human society (Ng et al., 2018). The intestinal symptoms that accompany UC include bloody diarrhea, and a third of patients with UC present with extraintestinal manifestations. Among these manifestations, arthritis has been the most commonly identified (Ungaro et al., 2017). Rheumatoid arthritis (RA) is an autoimmune disease that is characterized by inflammation, stiffness of joints accompanied by pain, loss of mobility, and joint deformity, and its incidence has increased substantially in the past 30 years (Safiri et al., 2019).
Studies report that patients with UC have an increased risk of RA (Wilson et al., 2016; Bae et al., 2017; Halling et al., 2017). UC and RA are immune-mediated inflammatory diseases (IMIDs); hence, they likely share similar pathogenesis, genes, and antigens. Previous studies have revealed several common genes associated with both UC and RA, including the human leukocyte antigen (HLA-B27), interleukin 15, peptidyl arginine deiminase type 4 (PADI4), and prostaglandin receptor EP4 (PTGER4) (Klausen et al., 1992; Mosquera-Martinez, 2001; Chen et al., 2008; Perdigones et al., 2010). UC and RA also share some common drugs for their treatment. TNF-α antagonists such as infliximab, have been approved as first- or second-line treatment of patients with UC and RA (Rubin et al., 2019; Smolen et al., 2020).
Despite extensive research on UC and RA, there still is a gap in understanding differentially expressed genes (DEGs) and possible targets for the treatment of UC and RA. Our study aimed to determine DEGs and possible targets for the treatment of UC and RA through bioinformatical analysis. In this study, we analyzed three gene expression datasets (GSE38713, GSE1919, and GSE12251) downloaded from the Restructured Gene Expression Omnibus (ReGEO) database. Comprehensive bioinformatics and enrichment analyses were used to determine independent DEGs and differentially coexpressed genes (DCGs). We constructed a protein-protein interaction (PPI) network to identify hub genes using the Search Tool for the Retrieval of Interacting Genes/Proteins (STRING) database and Cytoscape ver. 3.7.2 software. Moreover, we identified four potential therapeutic target genes related to UC and RA and constructed their regulatory network, using starBase and PROMO databases. These target genes include those of microRNAs (miRNAs), long non-coding RNAs (lncRNAs), and transcription factors (TFs). The potential therapeutic targets between UC and RA identified here are expected to provide novel insights into the biological mechanisms linked with these two diseases.
Materials and Methods
Data Source
GEO1 is a public repository containing high throughout sequencing and microarray data sets. We selected three gene expression microarray datasets (GSE38713, GSE1919, and GSE12251) from the GEO database. The GSE38713 and GSE12251 datasets were available on the GPL570 platform (HG-U133_Plus_2; Affymetrix Human Genome U133 Plus 2.0 Array), while GSE1919 was accessible on the GPL91 platform (HG_U95A; Affymetrix Human Genome U95A Array).
Identification of DEGs
The R software (version 3.6.3)2 and limma package3 in Bioconductor4 were used to detect the DEGs affected by UC, RA, infliximab treatment response samples, and corresponding control groups (Ritchie et al., 2015). DEGs were identified using the selection criteria of adjusted P-value < 0.05 and | logFC| >1.0. The intersecting parts of DEGs were calculated using a Venn diagram webtool5.
Gene Ontology and Pathway Enrichment Analysis of DEGs
Gene Ontology (GO) is a universal tool for defining the biological process (BP), cellular component (CC), and molecular function (MF) of numerous genes. Kyoto Encyclopedia of Genes and Genomes (KEGG) pathway is a database that contains multiple biological pathways for several organisms. GO and pathway analysis provide a deep insight into the relations of functions or pathways, and the primary roles of these genes. The enrichment analyses of DEGs were performed using the Cluster Profile package6 in Bioconductor, and a P-value less than 0.05 was considered as statistically significant (Yu et al., 2012).
Protein-Protein Interaction Network Construction and Module Analysis
Protein-protein interaction (PPI) network reveals the specific and unspecific interactions of proteins, and identifies the core protein genes. STRING (version 11.0)7, is a freely accessible database, that collects, scores, and integrates data, is used to predict functional relationships between proteins (Szklarczyk et al., 2019). A PPI network of the DEGs with combined score >0.4 in STRING was considered as a functional link, and was constructed using the Cytoscape software (version 3.7.2)8 (Smoot et al., 2011; Wang et al., 2016a, 2018; Li et al., 2017; Zhao et al., 2019). Subsequently, we used the MCODE plugin to identify densely connected modules from the PPI network with the criteria of K-core = 2, degree cutoff = 2, max depth = 100, and node score cutoff = 0.2 (Bandettini et al., 2012).
Selection and Analysis of Hub Genes
The degree of protein nodes was calculated by using the Cytoscape plugin, CytoHubba, to find hub genes (Chin et al., 2014). In this study, hub genes were selected with degrees ≥10. Subsequently, we used the corrplot package in R software to calculate the correlation between hub genes based on Pearson correlation analysis.
Construction of Regulatory Network
The network of genes and their corresponding miRNAs and lncRNAs was constructed using StarBase9, a publicly available database that mainly focuses on miRNA-target interactions (Li et al., 2014). The transcription factors (TFs) of genes were downloaded from PROMO10, a public database for predicting the TFs of various genes through DNA sequences (Farre et al., 2003). The above-mentioned tools were combined to construct a multi-factor regulation network.
Results
Identification of DEGs
Three gene expression datasets (GSE38713, GSE1919, and GSE12251) were selected in this study (Figure 1). The GSE38713 dataset was derived from 15 UC tissue samples and 10 control samples. GSE1919 dataset was derived from 5 RA samples and 5 control samples. GSE12251 dataset was derived from 22 patients with UC, of which 12 responded to and 10 did not respond to infliximab treatment. We used limma package to identify the DEGs in the three datasets with P < 0.05 and | logFC| >1. There were 1542 DEGs in the GSE38713 dataset, including 978 upregulated genes and 564 downregulated genes (Supplementary Table 1). There were 260 DEGs in the GSE1919 dataset, including 134 upregulated and 126 downregulated genes (Supplementary Table 2). In the GSE12251 dataset, 68 downregulated genes were identified (Supplementary Table 3). A Venn diagram was generated to show the overlap between GSE38713 and GSE1919 datasets; these include 63 upregulated genes (DEGs1) (Figure 2A) and 9 downregulated genes (DEGs2) (Figure 2B). Furthermore, 1470 DEGs (DEGs3) and 188 DEGs (DEGs4) were identified independently from the DEGs in UC and RA, respectively.
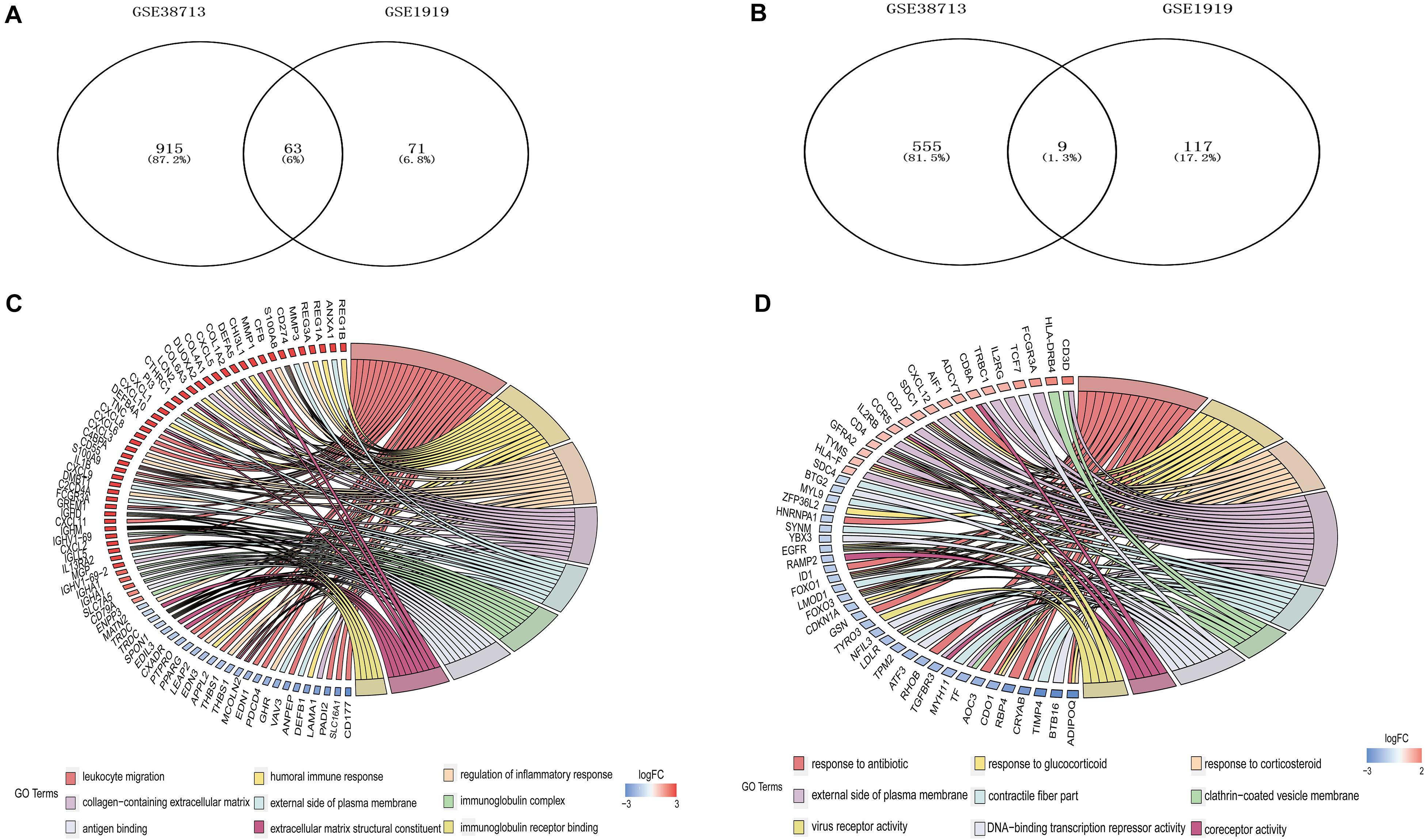
Figure 2. Differentially expressed genes (DEGs) among UC and RA. 63 upregulated DEGs (A) and 9 downregulated DEGs (B) expressed both in UC and RA. GO analyses of DEGs independently in UC (C) and RA (D) with adujust P-value.
GO Enrichment Analyses of Independent DEGs in UC and RA
GO analysis of GSE38713 indicated that the DEGs3 in UC were mainly involved in leukocyte migration, humoral immune response, and regulation of the inflammatory response under BP. The analysis also indicated that these DEGs were mainly involved in collagen-containing extracellular matrix, external side of plasma membrane, and immunoglobulin complex under CC. Likewise, the terms antigen binding, extracellular matrix structural constituent and immunoglobulin receptor binding were enriched under MF (Figure 2C). The GO analysis in the GSE1919 dataset for DEGs4 returned that the terms response to antibiotic, glucocorticoid, and corticosteroid under BP were mainly enriched. The terms enriched under CC were external side of plasma membrane, contractile fiber part, and clathrin-coated vesicle membrane. Moreover, the terms enriched under MF were DNA-binding transcription repressor activity, coreceptor activity, and virus receptor activity (Table 1 and Figure 2D).
Protein-Protein Interaction Network Construction and Module Analysis
Protein-protein interaction network analysis is a remarkable method in understanding the biological responses in health and disease. In this study, protein interactions between the DEGs1 and DEGs2 were analyzed using the STRING database. A total of 69 nodes and 251 edges were included with combined scores >0.4, and visualized using Cytoscape software (Figure 3). The MCODE plugin identified five densely connected modules in which 39 DEGs were among DEGs1 and DEGs2 (Figure 4A). KEGG and GO enrichment analyses of these 39 genes were carried out using the ClusterProfiler package. GO analysis revealed that these genes are involved in immunity (Figure 4B), and KEGG pathway analysis revealed them to be mainly involved in viral myocarditis, leishmaniasis, and allograft rejection (Figure 4C).
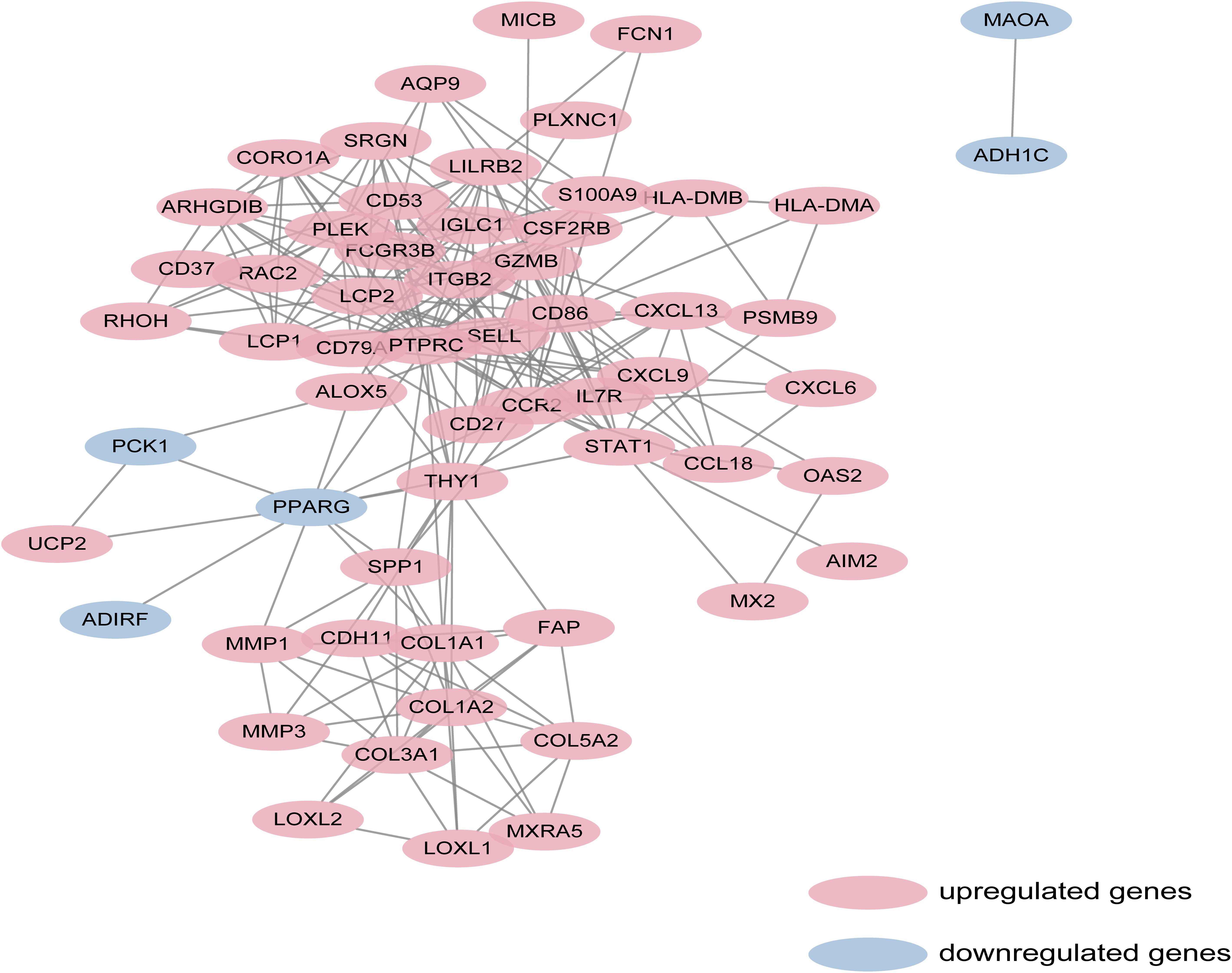
Figure 3. Based on database STRING and Cytoscape software, PPI networks of the DEGs1 and DEGs2 were constructed. The red point represents upregulated genes, and blue point represents downregulated genes.
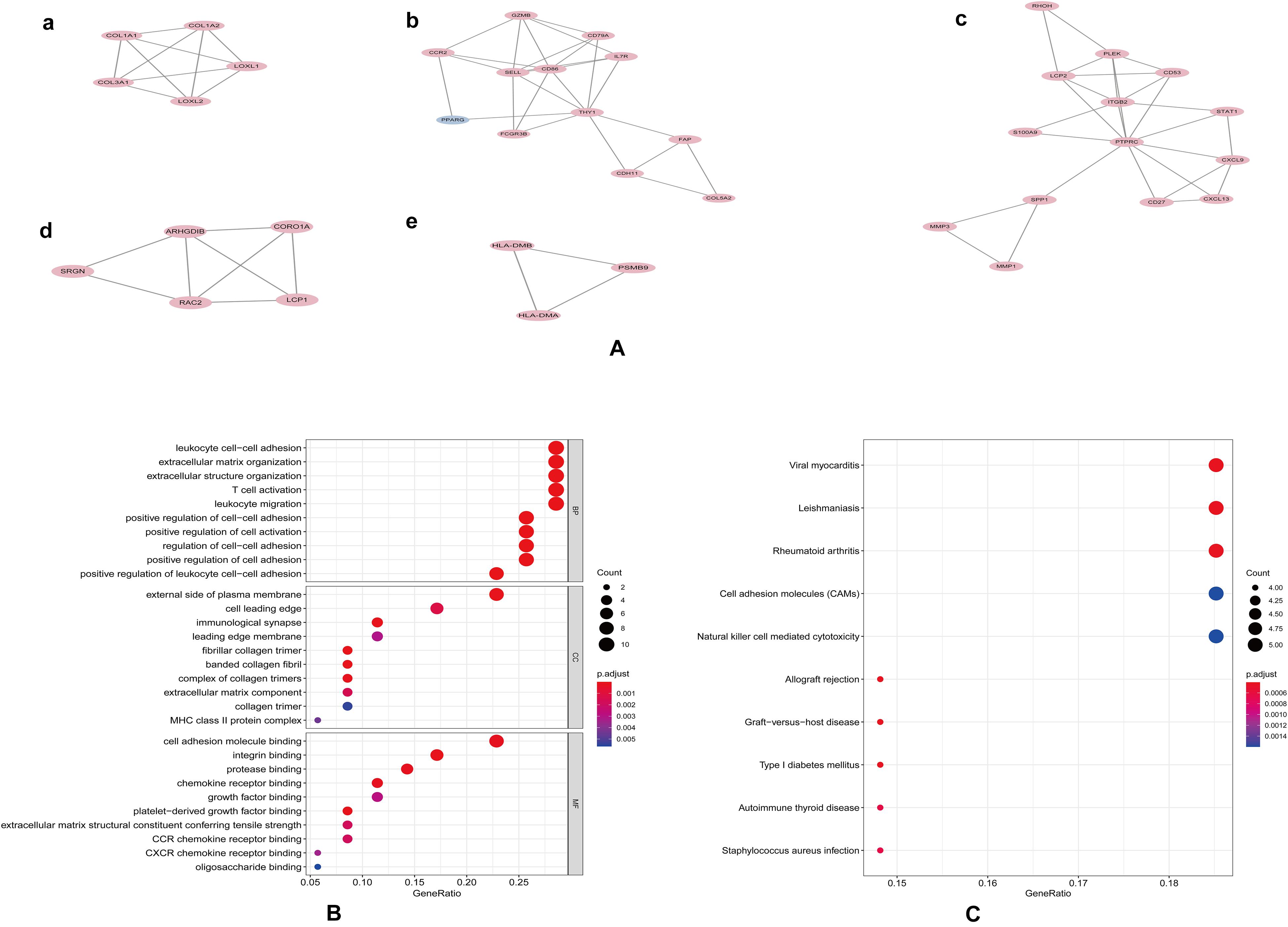
Figure 4. Modular analyses of PPI found five key modules (A). GO (B) and KEGG (C) enrichment analyses of 39 genes in these modules with P-value.
Hub Gene Selection and Analysis
With a criteria of degrees ≥10 using CytoHubba plugin, we identified a total of 30 hub genes. The scores of hub genes are presented in Figure 5A. The correlation between these 30 hub genes was investigated using the corrplot package, and Pearson scores >0.95 indicated a strong correlation between the hub genes. The correlation between 29 pairs of hub genes was considered significant (Figure 5B and Supplementary Table 4).
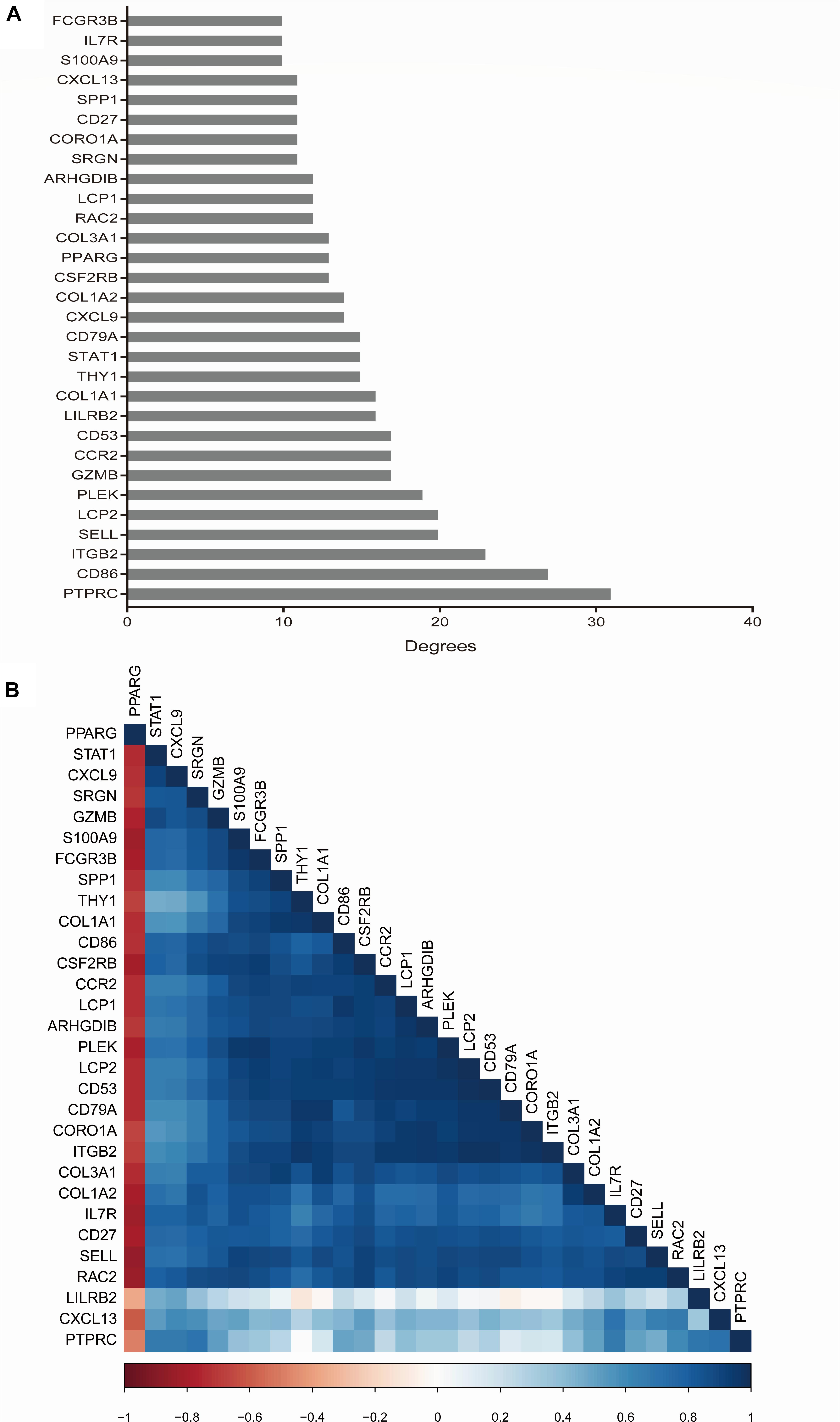
Figure 5. Hub gene selection and analysis performed by cytohubba. The score of hub genes was based on the EPC Algorithm (A). Pearson correlation analysis was used to calculate the correlation of hub genes (B).
Hub Genes in UC Response to Infliximab Treatment
We used limma packages to identify the DEGs in the GSE12251 dataset, and found 68 downregulated genes. The overlap between the hub genes of GSE38713, GSE1919, and GSE12251 include four protein-coding (pc) genes, such as SRGN (serglycin), PLEK (pleckstrin), and FCGR3B (Fc fragment of IgG receptor IIIb). All four genes were upregulated in UC and RA samples compared to those in the control samples in GSE38713 and GSE1919 datasets. On the other hand, these genes were downregulated in UC patients with response to infliximab treatment in the GSE12251 dataset. It could be concluded that the four genes play an important role during infliximab treatment of UC and RA.
Multi-factor Regulation Network Construction
We used StarBase and PROMO databases to predict the miRNAs, lncRNAs, and TFs of SRGN, PLEK, and FCGR3B, and found a total of 16 miRNAs, 40 lncRNAs, and 41 TFs. The data of these four genes and their miRNAs, lncRNAs, and TFs were integrated into a regulatory network, and visualized using Cytoscape software (Figure 6).
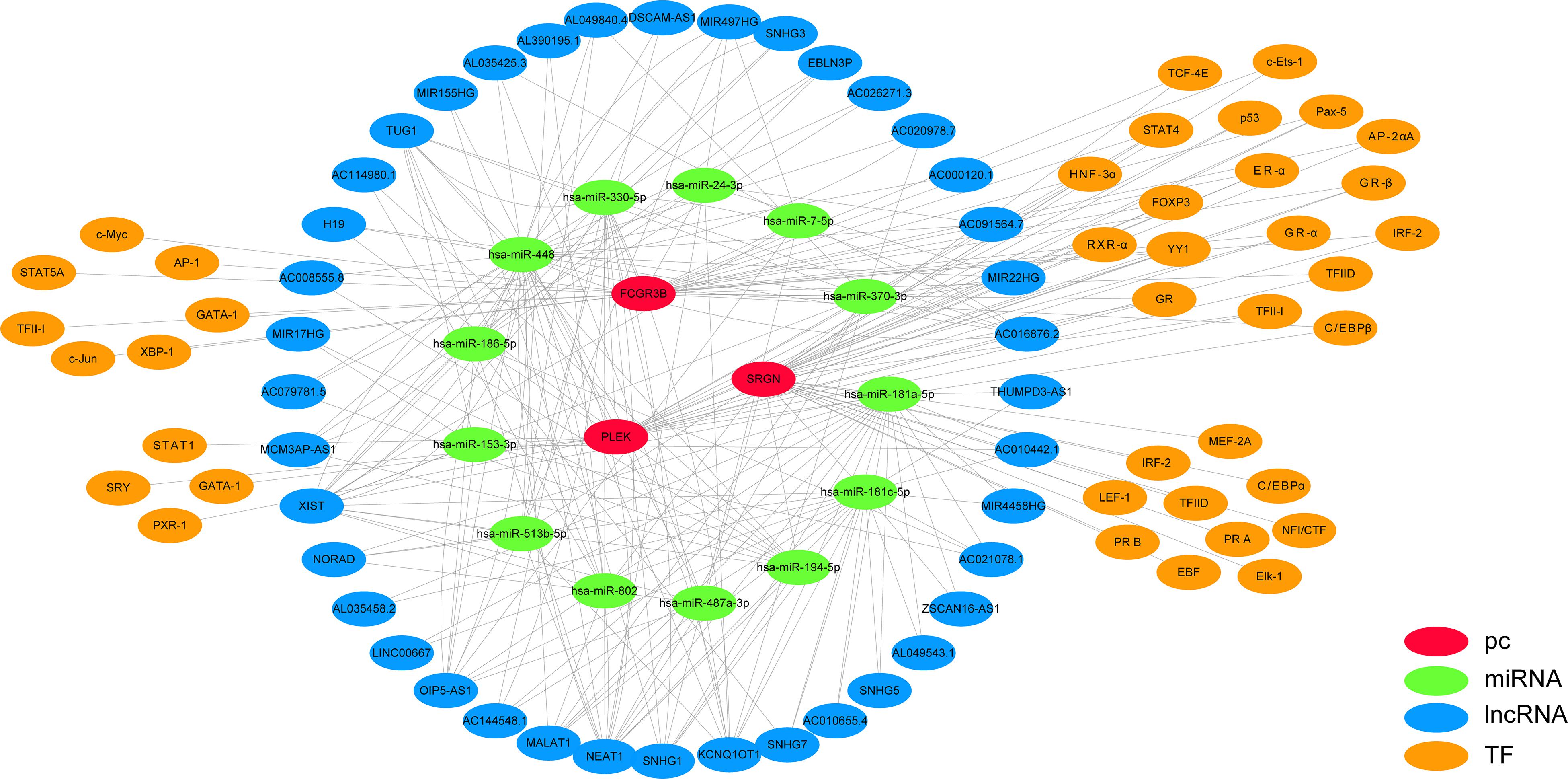
Figure 6. Multi-factor regulation network of SRGN, PLEK, and FCGR3B was constructed by starBase and PROMO database.
Discussion
There has been a considerable increase in the incidence and prevalence of UC and RA worldwide (Molodecky et al., 2012; Safiri et al., 2019). These diseases can lead to functional disabilities, severe decline in quality of life, and an increased risk of cancer (Jess et al., 2012; Simon et al., 2015; Myasoedova et al., 2019). Furthermore, UC has been reported to be concomitant with RA (Wilson et al., 2016). As IMIDs, UC and RA might have overlapping pathogenic pathways. Inflammatory and immune regulatory pathways, such as Fcγ receptor signaling, are linked to the pathogenesis of IMIDs (Castro-Dopico and Clatworthy, 2019; Virtanen et al., 2019). Additionally, gut microbiota has been reported to play a role in IMIDs (Liu et al., 2013; Forbes et al., 2018; Imhann et al., 2018). Treatment with TNF-α antagonists has been firmly established as an effective therapeutic approach for RA (Kievit et al., 2008); however, non-responsiveness to infliximab (a TNF-α antagonist) is common in patients with UC (Kievit et al., 2008; Wong and Cross, 2017). The main purpose of our study was to identify the common DEGs in UC and RA, thereby revealing potential targets for predicting the therapeutic effect of TNF-α antagonist and treating UC and RA.
In this study, we identified 72 overlapping DEGs in both UC and RA, of which 63 were upregulated (DEGs1) and 9 were downregulated genes (DEGs2). Independent DEGs included 915 upregulated and 555 downregulated genes in UC (DEGs3), and 71 upregulated and 117 downregulated genes in RA (DEGs4). GO analysis revealed that DEGs3 were significantly enriched in inflammatory and immune pathways, which played a central role in the development of UC and RA. While DEGs4 were mainly enriched in drug responses. Enrichment analyses of the genes in the key modules of the constructed PPI network revealed that they were mainly enriched in some immune-related pathways and cellular organization processing, such as leukocyte cell-cell adhesion, extracellular matrix organization, T cell activation, and leukocyte migration. The adhesion and migration of leukocyte, as well as the activation of T cells have been linked to the pathogenesis of UC and RA (Thomas and Baumgart, 2012; Reynisdottir et al., 2016; McNaughton et al., 2018; Rabe et al., 2019). Finally, a total of 45 hub genes were identified, among which four hub genes (SRGN, PLEK, and FCGR3B) were predicted to be upregulated in UC and RA samples (GSE38713 and GSE1919) and downregulated in UC patients with response to infliximab treatment (GSE12251). These findings suggest that these four genes are predictive markers and therapeutic targets for UC and RA.
SRGN encodes the proteoglycan protein, and is mainly expressed in hematopoietic cells. Many studies have confirmed that SRGN promotes tumor invasion and metastasis in colorectal cancer, non-small cell lung cancers, multiple myeloma, nasopharyngeal carcinoma, and breast cancer (Li et al., 2011; Korpetinou et al., 2013; Purushothaman and Toole, 2014; Guo et al., 2017; Xu et al., 2018). SRGN is also involved in inflammatory processes through the regulation of numerous inflammatory mediators such as TNF-α, and activating the NF-κB signaling pathway (Zernichow et al., 2006; Korpetinou et al., 2014; Scuruchi et al., 2019). These processes caused by the combination of SRGN and CD44 receptor, could promote inflammation (Misra et al., 2015). PLEK, a substrate of protein kinase C, is involved in various adaptive immune responses (Cremonesi et al., 2012). Although the underlying mechanisms of PLEK are still unclear, many studies have linked it to certain diseases. PLEK might be a susceptibility locus for venous thromboembolism, and its expression is increased in UC, periodontitis, and celiac disease (Song et al., 2015; Pascual et al., 2016; Lindstrom et al., 2019; Medrano et al., 2019). In diabetes, PLEK has been reported to promote the secretion of proinflammatory cytokines such as TNF-α and IL-1β in mononuclear phagocytes; these cytokines have already been linked to increased risk of UC and RA (Ding et al., 2007; Hermanns et al., 2016). We speculate that SRGN and PLEK are involved in the pathogenesis of UC and RA through the increase in inflammatory factors. Many researches have proven that the copy number variation (CNV) of FCGR3B is linked to autoimmune and inflammatory diseases. Low copy number and the deletion of FCGR3B increase the risk of RA (Tsang-A-Sjoe et al., 2016; Wang et al., 2016b; Rahbari et al., 2017; Zheng et al., 2017), and FCGR3B gene copy number has also been suggested to increase susceptibility to UC, which indicates that FCGR3B might be the key gene involved in their pathogenesis (Asano et al., 2013). Although the specific mechanisms of action of FcγRIIIb in IMIDs remain unclear, studies revealed that FcγRIIIb is a stimulatory Fc gamma receptor which promotes neutrophil recruitment and the capture and clearance of immune complexes (ICs); the deletion of FCGR3B lead to immune-complex-mediated diseases (Dijstelbloem et al., 2001; Willcocks et al., 2008; Chen et al., 2012). We speculate that FCGR3B is involved in the inflammatory processes in IgG-IC-FcγR signaling (Mathsson et al., 2006; Uo et al., 2013; Bersellini Farinotti et al., 2019).
The limitations of our study are as follows. Our study is a retrospective analysis and has small sample size. Hence, our findings need to be validated using a larger cohort and prospective studies. We did not assess the potential therapeutic roles of SRGN, PLEK, and FCGR3B in UC and RA; therefore, further clinical research is needed to investigate whether they could be used as predictive factors for infliximab efficacy in patients with UC and RA. Finally, we did not explore the specific mechanisms of these four genes in UC and RA, which warrants further studies.
Conclusion
In conclusion, our study identified 169 novel DEGs and 45 hub genes common in both UC and RA. GO and KEGG analyses of independent DEGs and the hub genes in UC and RA might reveal a novel prospective relationship between UC and RA. In addition, we found four hub genes (SRGN, PLEK, and FCGR3B) that were significantly associated with infliximab treatment in UC. These genes need to be explored further for their clinical relevance as potential prognostic indicators and therapeutic targets in UC and RA.
Data Availability Statement
The datasets presented in this study can be found in online repositories. The names of the repository/repositories and accession number(s) can be found in the article/Supplementary Material.
Author Contributions
YC and HL collected the papers and analyzed data, analyzed the conclusions, and drafted the manuscript. LL reviewed the data and conclusions. JS presented the idea of this manuscript, supported the funding, analyzed the conclusions, drafted and revised the manuscript. All authors contributed to the article and approved the submitted version.
Funding
Supported by grants from the National Natural Science Foundation of China (No. 81770545 and 81701746) and MDT Project of Clinical Research Innovation Foundation, Renji Hospital, School of Medicine, and Shanghai Jiao Tong University (PYI-17-003).
Conflict of Interest
The authors declare that the research was conducted in the absence of any commercial or financial relationships that could be construed as a potential conflict of interest.
Supplementary Material
The Supplementary Material for this article can be found online at: https://www.frontiersin.org/articles/10.3389/fgene.2020.572194/full#supplementary-material
Supplementary Table 1 | Differentially expressed genes in GSE38713.
Supplementary Table 2 | Differentially expressed genes in GSE1919.
Supplementary Table 3 | Differentially expressed genes in GSE12251.
Supplementary Table 4 | Correlations between hub genes.
Abbreviations
BP, biological process; CC, cellular component; CNV, copy number variation; DEGs, differentially expressed genes; FCGR3B, Fc fragment of IgG receptor IIIb; ICs, immune complexes; lncRNAs, long non-coding RNAs; IMIDs, immune-mediated inflammatory diseases; MF, molecular function; miRNAs, microRNAs; NFkB, nuclear transcription factor kappaB; OS, overall survival; PPI, protein-protein interaction; PLEK, pleckstrin; RA, rheumatoid arthritis; SRGN, serglycin; TF, transcription factors; UC, ulcerative colitis.
Footnotes
- ^ http://www.ncbi.nlm.nih.gov/geo/
- ^ https://www.r-project.org/
- ^ http://www.bioconductor.org/packages/release/bioc/html/limma.html
- ^ http://www.bioconductor.org/
- ^ http://bioinfogp.cnb.csic.es/tools/venny/index.html
- ^ http://www.bioconductor.org/packages/release/bioc/html/clusterProfiler.html
- ^ http://string-db.org
- ^ http://www.cytoscape.org/
- ^ http://starbase.sysu.edu.cn/index.php
- ^ http://alggen.lsi.upc.es/cgi-bin/promo_v3/promo/promoinit.cgi?dirDB=TF_8.3
References
Asano, K., Matsumoto, T., Umeno, J., Hirano, A., Esaki, M., and Hosono, N. (2013). Impact of allele copy number of polymorphisms in FCGR3A and FCGR3B genes on susceptibility to ulcerative colitis. Inflamm. Bowel Dis. 19, 2061–2068. doi: 10.1097/MIB.0b013e318298118e
Bae, J. M., Choo, J. Y., Kim, K. J., and Park, K. S. (2017). Association of inflammatory bowel disease with ankylosing spondylitis and rheumatoid arthritis: a nationwide population-based study. Mod. Rheumatol. 27, 435–440. doi: 10.1080/14397595.2016.1211229
Bandettini, W. P., Kellman, P., Mancini, C., Booker, O. J., Vasu, S., Leung, S. W., et al. (2012). MultiContrast Delayed Enhancement (MCODE) improves detection of subendocardial myocardial infarction by late gadolinium enhancement cardiovascular magnetic resonance: a clinical validation study. J. Cardiovasc. Magn. Reson. 14:83. doi: 10.1186/1532-429x-14-83
Bersellini Farinotti, A., Wigerblad, G., Nascimento, D., Bas, D. B., Morado Urbina, C., Nandakumar, K. S., et al. (2019). Cartilage-binding antibodies induce pain through immune complex-mediated activation of neurons. J. Exp. Med. 216, 1904–1924. doi: 10.1084/jem.20181657
Castro-Dopico, T., and Clatworthy, M. R. (2019). IgG and Fcgamma receptors in intestinal immunity and inflammation. Front. Immunol. 10:805. doi: 10.3389/fimmu.2019.00805
Chen, C. C., Isomoto, H., Narumi, Y., Sato, K., Oishi, Y., and Kobayashi, T. (2008). Haplotypes of PADI4 susceptible to rheumatoid arthritis are also associated with ulcerative colitis in the Japanese population. Clin. Immunol. 126, 165–171. doi: 10.1016/j.clim.2007.09.001
Chen, K., Nishi, H., Travers, R., Tsuboi, N., Martinod, K., and Wagner, D. D. (2012). Endocytosis of soluble immune complexes leads to their clearance by FcgammaRIIIB but induces neutrophil extracellular traps via FcgammaRIIA in vivo. Blood 120, 4421–4431. doi: 10.1182/blood-2011-12-401133
Chin, C. H., Chen, S. H., Wu, H. H., Ho, C. W., Ko, M. T., and Lin, C. Y. (2014). cytoHubba: identifying hub objects and sub-networks from complex interactome. BMC Syst. Biol. 8(Suppl. 4):S11. doi: 10.1186/1752-0509-8-s4-s11
Cremonesi, P., Capoferri, R., Pisoni, G., Del Corvo, M., Strozzi, F., and Rupp, R. (2012). Response of the goat mammary gland to infection with Staphylococcus aureus revealed by gene expression profiling in milk somatic and white blood cells. BMC Genomics 13:540. doi: 10.1186/1471-2164-13-540
Dijstelbloem, H. M., van de Winkel, J. G., and Kallenberg, C. G. (2001). Inflammation in autoimmunity: receptors for IgG revisited. Trends Immunol. 22, 510–516. doi: 10.1016/s1471-4906(01)02014-2
Ding, Y., Kantarci, A., Badwey, J. A., Hasturk, H., Malabanan, A., and Van Dyke, T. E. (2007). Phosphorylation of pleckstrin increases proinflammatory cytokine secretion by mononuclear phagocytes in diabetes mellitus. J. Immunol. 179, 647–654. doi: 10.4049/jimmunol.179.1.647
Farre, D., Roset, R., Huerta, M., Adsuara, J. E., Rosello, L., Alba, M. M., et al. (2003). Identification of patterns in biological sequences at the ALGGEN server: PROMO and MALGENucleic. Nucleic Acids Res. 31, 3651–3653. doi: 10.1093/nar/gkg605
Forbes, J. D., Chen, C. Y., Knox, N. C., Marrie, R. A., El-Gabalawy, H., and de Kievit, T. (2018). A comparative study of the gut microbiota in immune-mediated inflammatory diseases-does a common dysbiosis exist? Microbiome 6:221. doi: 10.1186/s40168-018-0603-4
Guo, J. Y., Hsu, H. S., Tyan, S. W., Li, F. Y., Shew, J. Y., Lee, W. H., et al. (2017). Serglycin in tumor microenvironment promotes non-small cell lung cancer aggressiveness in a CD44-dependent manner. Oncogene 36, 2457–2471. doi: 10.1038/onc.2016.404
Virtanen, T. A., Haikarainen, T., Raivola, J., and Silvennoinen, O. (2019). Selective JAKinibs: prospects in inflammatory and autoimmune diseases. BioDrugs 33, 15–32. doi: 10.1007/s40259-019-00333-w
Halling, M. L., Kjeldsen, J., Knudsen, T., Nielsen, J., and Hansen, L. K. (2017). Patients with inflammatory bowel disease have increased risk of autoimmune and inflammatory diseases. World J. Gastroenterol. 23, 6137–6146. doi: 10.3748/wjg.v23.i33.6137
Hermanns, H. M., Wohlfahrt, J., Mais, C., Hergovits, S., Jahn, D., and Geier, A. (2016). Endocytosis of pro-inflammatory cytokine receptors and its relevance for signal transduction. Biol. Chem. 397, 695–708. doi: 10.1515/hsz-2015-0277
Imhann, F., Vich Vila, A., Bonder, M. J., Fu, J., Gevers, D., and Visschedijk, M. C. (2018). Interplay of host genetics and gut microbiota underlying the onset and clinical presentation of inflammatory bowel disease. Gut 67, 108–119. doi: 10.1136/gutjnl-2016-312135
Jess, T., Rungoe, C., and Peyrin-Biroulet, L. (2012). Risk of colorectal cancer in patients with ulcerative colitis: a meta-analysis of population-based cohort studies. Clin. Gastroenterol. Hepatol. 10, 639–645. doi: 10.1016/j.cgh.2012.01.010
Kievit, W., Adang, E. M., Fransen, J., Kuper, H. H., van de Laar, M. A., and Jansen, T. L. (2008). The effectiveness and medication costs of three anti-tumour necrosis factor alpha agents in the treatment of rheumatoid arthritis from prospective clinical practice data. Ann. Rheum. Dis. 67, 1229–1234. doi: 10.1136/ard.2007.083675
Klausen, T., Amris, K., and Helin, P. (1992). Ulcerative colitis complicating seronegative HLA-A2-B27 rheumatoid arthritis with sacroiliitis. Ann. Rheum. Dis. 51, 1009–1011. doi: 10.1136/ard.51.8.1009
Korpetinou, A., Skandalis, S. S., Labropoulou, V. T., Smirlaki, G., Noulas, A., Karamanos, N. K., et al. (2014). Serglycin: at the crossroad of inflammation and malignancy. Front. Oncol. 3:327. doi: 10.3389/fonc.2013.00327
Korpetinou, A., Skandalis, S. S., Moustakas, A., Happonen, K. E., Tveit, H., and Prydz, K. (2013). Serglycin is implicated in the promotion of aggressive phenotype of breast cancer cells. PLoS One 8:e78157. doi: 10.1371/journal.pone.0078157
Li, J. H., Liu, S., Zhou, H., Qu, L. H., and Yang, J. H. (2014). starBase v2.0: decoding miRNA-ceRNA, miRNA-ncRNA and protein-RNA interaction networks from large-scale CLIP-Seq data. Nucleic Acids Res. 42, D92–D97. doi: 10.1093/nar/gkt1248
Li, L., Lei, Q., Zhang, S., Kong, L., and Qin, B. (2017). Screening and identification of key biomarkers in hepatocellular carcinoma: evidence from bioinformatic analysis. Oncol. Rep. 38, 2607–2618. doi: 10.3892/or.2017.5946
Li, X. J., Ong, C. K., Cao, Y., Xiang, Y. Q., Shao, J. Y., Ooi, A., et al. (2011). Serglycin is a theranostic target in nasopharyngeal carcinoma that promotes metastasis. Cancer Res. 71, 3162–3172. doi: 10.1158/0008-5472.Can-10-3557
Lindstrom, S., Wang, L., Smith, E. N., Gordon, W., van Hylckama, A., and Vlieg de, M. (2019). Genomic and transcriptomic association studies identify 16 novel susceptibility loci for venous thromboembolism. Blood 134, 1645–1657. doi: 10.1182/blood.2019000435
Liu, X., Zou, Q., Zeng, B., Fang, Y., and Wei, H. (2013). Analysis of fecal Lactobacillus community structure in patients with early rheumatoid arthritis. Curr. Microbiol. 67, 170–176. doi: 10.1007/s00284-013-0338-1
Mathsson, L., Lampa, J., Mullazehi, M., and Ronnelid, J. (2006). Immune complexes from rheumatoid arthritis synovial fluid induce FcgammaRIIa dependent and rheumatoid factor correlated production of tumour necrosis factor-alpha by peripheral blood mononuclear cells. Arthritis Res. Ther. 8:R64. doi: 10.1186/ar1926
McNaughton, E. F., Eustace, A. D., King, S., Sessions, R. B., Kay, A., and Farris, M. (2018). Novel anti-inflammatory peptides based on chemokine-glycosaminoglycan interactions reduce leukocyte migration and disease severity in a model of rheumatoid arthritis. J. Immunol. 200, 3201–3217. doi: 10.4049/jimmunol.1701187
Medrano, L. M., Pascual, V., Bodas, A., Lopez-Palacios, N., Salazar, I., and Espino-Paisan, L. (2019). Expression patterns common and unique to ulcerative colitis and celiac disease. Ann. Hum. Genet. 83, 86–94. doi: 10.1111/ahg.12293
Misra, S., Hascall, V. C., Markwald, R. R., and Ghatak, S. (2015). Interactions between Hyaluronan and Its Receptors (CD44, RHAMM) regulate the activities of inflammation and cancer. Front. Immunol. 6:201. doi: 10.3389/fimmu.2015.00201
Molodecky, N. A., Soon, I. S., Rabi, D. M., Ghali, W. A., Ferris, M., and Chernoff, G. (2012). Increasing incidence and prevalence of the inflammatory bowel diseases with time, based on systematic review. Gastroenterology 142, 46.e42–54.e42. doi: 10.1053/j.gastro.2011.10.001
Mosquera-Martinez, J. A. (2001). Rheumatoid arthritis associated with ulcerative colitis. Ann. Rheum. Dis. 60:1155. doi: 10.1136/ard.60.12.1155
Myasoedova, E., Davis, J. M. III, Achenbach, S. J., Matteson, E. L., and Crowson, C. S. (2019). Trends in prevalence of functional disability in rheumatoid arthritis compared with the general population. Mayo Clin. Proc. 94, 1035–1039. doi: 10.1016/j.mayocp.2019.01.002
Ng, S. C., Shi, H. Y., Hamidi, N., Underwood, F. E., Tang, W., and Benchimol, E. I. (2018). Worldwide incidence and prevalence of inflammatory bowel disease in the 21st century: a systematic review of population-based studies. Lancet 390, 2769–2778. doi: 10.1016/s0140-6736(17)32448-0
Pascual, V., Medrano, L. M., Lopez-Palacios, N., Bodas, A., Dema, B., and Fernandez-Arquero, M. (2016). Different gene expression signatures in children and adults with celiac disease. PLoS One 11:e0146276. doi: 10.1371/journal.pone.0146276
Perdigones, N., Martin, E., Robledo, G., Lamas, J. R., Taxonera, C., and Diaz-Rubio, M. (2010). Study of chromosomal region 5p13.1 in Crohn’s disease, ulcerative colitis, and rheumatoid arthritis. Hum. Immunol. 71, 826–828. doi: 10.1016/j.humimm.2010.05.010
Purushothaman, A., and Toole, B. P. (2014). Serglycin proteoglycan is required for multiple myeloma cell adhesion, in vivo growth, and vascularization. J. Biol. Chem. 289, 5499–5509. doi: 10.1074/jbc.M113.532143
Rabe, H., Malmquist, M., Barkman, C., Östman, S., Gjertsson, I., Saalman, R., et al. (2019). Distinct patterns of naive, activated and memory T and B cells in blood of patients with ulcerative colitis or Crohn’s disease. Clin. Exp. Immunol. 197, 111–129. doi: 10.1111/cei.13294
Rahbari, R., Zuccherato, L. W., Tischler, G., Chihota, B., Ozturk, H., and Saleem, S. (2017). Understanding the genomic structure of copy-number variation of the low-affinity fcgamma receptor region allows confirmation of the association of FCGR3B deletion with rheumatoid arthritis. Hum. Mutat. 38, 390–399. doi: 10.1002/humu.23159
Reynisdottir, G., Olsen, H., Joshua, V., Engström, M., Forsslund, H., and Karimi, R. (2016). Signs of immune activation and local inflammation are present in the bronchial tissue of patients with untreated early rheumatoid arthritis. Ann. Rheum. Dis. 75, 1722–1727. doi: 10.1136/annrheumdis-2015-208216
Ritchie, M. E., Phipson, B., Wu, D., Hu, Y., Law, C. W., Shi, W., et al. (2015). limma powers differential expression analyses for RNA-sequencing and microarray studies. Nucleic Acids Res. 43:e47. doi: 10.1093/nar/gkv007
Rubin, D. T., Ananthakrishnan, A. N., Siegel, C. A., Sauer, B. G., et al. (2019). ACG clinical guideline: ulcerative colitis in adults. Am. J. Gastroenterol. 114, 384–413. doi: 10.14309/ajg.0000000000000152
Safiri, S., Kolahi, A. A., Hoy, D., Smith, E., Bettampadi, D., and Mansournia, M. A. (2019). Global, regional and national burden of rheumatoid arthritis 1990-2017: a systematic analysis of the Global Burden of Disease study 2017. Ann. Rheum. Dis. 78, 1463–1471. doi: 10.1136/annrheumdis-2019-215920
Scuruchi, M., D’Ascola, A., Avenoso, A., Mandraffino, G. G., Campo, S. S., and Campo, G. M. (2019). Serglycin as part of IL-1beta induced inflammation in human chondrocytes. Arch. Biochem. Biophys. 669, 80–86. doi: 10.1016/j.abb.2019.05.021
Simon, T. A., Thompson, A., Gandhi, K. K., Hochberg, M. C., and Suissa, S. (2015). Incidence of malignancy in adult patients with rheumatoid arthritis: a meta-analysis. Arthritis Res. Ther. 17:212. doi: 10.1186/s13075-015-0728-9
Smolen, J. S., Landewe, R. B. M., Bijlsma, J. W. J., Burmester, G. R., Dougados, M., and Kerschbaumer, A. (2020). EULAR recommendations for the management of rheumatoid arthritis with synthetic and biological disease-modifying antirheumatic drugs: 2019 update. Ann. Rheum. Dis. 79, 685–699. doi: 10.1136/annrheumdis-2019-216655
Smoot, M. E., Ono, K., Ruscheinski, J., Wang, P. L., and Ideker, T. (2011). Cytoscape 2.8: new features for data integration and network visualization. Bioinformatics 27, 431–432. doi: 10.1093/bioinformatics/btq675
Song, L., Yao, J., He, Z., and Xu, B. (2015). Genes related to inflammation and bone loss process in periodontitis suggested by bioinformatics methods. BMC Oral Health 15:105. doi: 10.1186/s12903-015-0086-7
Szklarczyk, D., Gable, A. L., Lyon, D., Junge, A., Wyder, S., and Huerta-Cepas, J. (2019). STRING v11: protein-protein association networks with increased coverage, supporting functional discovery in genome-wide experimental datasets. Nucleic Acids Res. 47, D607–D613. doi: 10.1093/nar/gky1131
Thomas, S., and Baumgart, D. C. (2012). Targeting leukocyte migration and adhesion in Crohn’s disease and ulcerative colitis. Inflammopharmacology 20, 1–18. doi: 10.1007/s10787-011-0104-6
Tsang-A-Sjoe, M. W., Nagelkerke, S. Q., Bultink, I. E., Geissler, J., Tanck, M. W., et al. (2016). Fc-gamma receptor polymorphisms differentially influence susceptibility to systemic lupus erythematosus and lupus nephritis. Rheumatology 55, 939–948. doi: 10.1093/rheumatology/kev433
Ungaro, R., Mehandru, S., Allen, P. B., Peyrin-Biroulet, L., and Colombel, J. F. (2017). Ulcerative colitis. Lancet 389, 1756–1770. doi: 10.1016/s0140-6736(16)32126-2
Uo, M., Hisamatsu, T., Miyoshi, J., Kaito, D., Yoneno, K., and Kitazume, M. T. (2013). Mucosal CXCR4+ IgG plasma cells contribute to the pathogenesis of human ulcerative colitis through FcgammaR-mediated CD14 macrophage activation. Gut 62, 1734–1744. doi: 10.1136/gutjnl-2012-303063
Wang, L., Li, Z., Song, X., Liu, L., Su, G., and Cui, Y. (2016a). Bioinformatic analysis of genes and MicroRNAs associated with atrioventricular septal defect in down syndrome patients. Int. Heart J. 57, 490–495. doi: 10.1536/ihj.15-319
Wang, L., Yang, X., Cai, G., Xin, L., Xia, Q., and Zhang, X. (2016b). Association study of copy number variants in FCGR3A and FCGR3B gene with risk of ankylosing spondylitis in a Chinese population. Rheumatol. Int. 36, 437–442. doi: 10.1007/s00296-015-3384-0
Wang, R., Li, J., Zhao, Y., Li, Y., and Yin, L. (2018). Investigating the therapeutic potential and mechanism of curcumin in breast cancer based on RNA sequencing and bioinformatics analysis. Breast Cancer 25, 206–212. doi: 10.1007/s12282-017-0816-6
Willcocks, L. C., Lyons, P. A., Clatworthy, M. R., Robinson, J. I., Yang, W., and Newland, S. A. (2008). Copy number of FCGR3B, which is associated with systemic lupus erythematosus, correlates with protein expression and immune complex uptake. J. Exp. Med. 205, 1573–1582. doi: 10.1084/jem.20072413
Wilson, J. C., Furlano, R. I., Jick, S. S., and Meier, C. R. (2016). Inflammatory bowel disease and the risk of autoimmune diseases. J. Crohns Colitis 10, 186–193. doi: 10.1093/ecco-jcc/jjv193
Wong, U., and Cross, R. K. (2017). Primary and secondary nonresponse to infliximab: mechanisms and countermeasures. Expert Opin. Drug Metab. Toxicol. 13, 1039–1046. doi: 10.1080/17425255.2017.1377180
Xu, Y., Xu, J., Yang, Y., Zhu, L., Li, X., and Zhao, W. (2018). SRGN promotes colorectal cancer metastasis as a critical downstream target of HIF-1alpha. Cell Physiol. Biochem 48, 2429–2440. doi: 10.1159/000492657
Yu, G., Wang, L. G., Han, Y., and He, Q. Y. (2012). clusterProfiler: an R package for comparing biological themes among gene clusters. OMICS 16, 284–287. doi: 10.1089/omi.2011.0118
Zernichow, L., Abrink, M., Hallgren, J., Grujic, M., Pejler, G., and Kolset, S. O. (2006). Serglycin is the major secreted proteoglycan in macrophages and has a role in the regulation of macrophage tumor necrosis factor-alpha secretion in response to lipopolysaccharide. J. Biol. Chem. 281, 26792–26801. doi: 10.1074/jbc.M512889200
Zhao, S., Li, B., Li, C., Gao, H., Miao, Y., He, Y., et al. (2019). The apoptosis regulator 14-3-3η and its potential as a therapeutic target in pituitary oncocytoma. Front. Endocrinol. 10:797. doi: 10.3389/fendo.2019.00797
Keywords: bioinformatical analysis, hub genes, ulcerative colitis, rheumatoid arthritis, differentially expressed genes
Citation: Chen Y, Li H, Lai L, Feng Q and Shen J (2020) Identification of Common Differentially Expressed Genes and Potential Therapeutic Targets in Ulcerative Colitis and Rheumatoid Arthritis. Front. Genet. 11:572194. doi: 10.3389/fgene.2020.572194
Received: 15 June 2020; Accepted: 13 October 2020;
Published: 11 November 2020.
Edited by:
Lin Zhang, Shandong Maternal and Child Health Hospital, ChinaReviewed by:
Md. Rakibul Islam, Daffodil International University, BangladeshLei Bao, Zhengzhou University, China
Copyright © 2020 Chen, Li, Lai, Feng and Shen. This is an open-access article distributed under the terms of the Creative Commons Attribution License (CC BY). The use, distribution or reproduction in other forums is permitted, provided the original author(s) and the copyright owner(s) are credited and that the original publication in this journal is cited, in accordance with accepted academic practice. No use, distribution or reproduction is permitted which does not comply with these terms.
*Correspondence: Jun Shen, shenjun_renji@163.com