- 1Department of Genomic Science, School of Biological Sciences, Central University of Kerala, Kasaragod, India
- 2Centre for Genomics and Applied Gene Technology, Institute of Integrative Omics and Applied Biotechnology, Purba Medinipur, India
- 3Laboratório de Genética Celular e Molecular, Departamento de Biologia Geral, Instituto de Ciências Biológicas, Universidade Federal de Minas Gerais, Belo Horizonte, Brazil
- 4Department of Computer Science, Virginia Commonwealth University, Richmond, VA, United States
A multitude of factors, such as drug misuse, lack of strong regulatory measures, improper sewage disposal, and low-quality medicine and medications, have been attributed to the emergence of drug resistant microbes. The emergence and outbreaks of multidrug resistance to last-line antibiotics has become quite common. This is further fueled by the slow rate of drug development and the lack of effective resistome surveillance systems. In this review, we provide insights into the recent advances made in computational approaches for the surveillance of antibiotic resistomes, as well as experimental formulation of combinatorial drugs. We explore the multiple roles of antibiotics in nature and the current status of combinatorial and adjuvant-based antibiotic treatments with nanoparticles, phytochemical, and other non-antibiotics based on synergetic effects. Furthermore, advancements in machine learning algorithms could also be applied to combat the spread of antibiotic resistance. Development of resistance to new antibiotics is quite rapid. Hence, we review the recent literature on discoveries of novel antibiotic resistant genes though shotgun and expression-based metagenomics. To decelerate the spread of antibiotic resistant genes, surveillance of the resistome is of utmost importance. Therefore, we discuss integrative applications of whole-genome sequencing and metagenomics together with machine learning models as a means for state-of-the-art surveillance of the antibiotic resistome. We further explore the interactions and negative effects between antibiotics and microbiomes upon drug administration.
Introduction
Microorganisms are ubiquitous in nature and play crucial roles in the body’s biochemical functions. Despite their importance, opportunistic and pathogenic microbes have evolved to bypass the immune system. Gram-negative bacteria in particular are of clinical importance, as they produce metabolites, such as endotoxin and lipopolysaccharide, which are major causes of infection (Baron, 1996). Fleming’s serendipitous discovery of penicillin in 1928 began the antibiotic revolution and provided proof that natural compounds could selectively inhibit the growth of pathogens (Gaynes, 2017). Since then, antibiotics have been purified from various microbial, plant, and synthetic sources (Charest et al., 2005; de Lima Procópio et al., 2012; Khameneh et al., 2019). Today, antibiotics of various inhibitory mechanisms, such as inhibition of cell wall synthesis, protein synthesis, DNA replication, and folic acid synthesis, are widely available. The downside of such availability is the misuse of drugs and accelerated antibiotic resistance (Figure 1). Antibiotic resistance in bacterial pathogens is widespread. Approximately 2.8 million cases of new infection and about 35,000 deaths occur annually in the US alone (Tanzi, 2020). Antibiotic resistance is a global problem threatening the modern world (Table 1). According to the Global Antimicrobial Resistance Surveillance System report in 2017, the major clinically relevant antibiotic resistance species are Escherichia coli, Klebsiella pneumoniae, Acinetobacter spp., Staphylococcus aureus, Salmonella spp., Shigella spp., Streptococcus pneumoniae, and Neisseria gonorrhoeae. The spread of antibiotic resistance is also determined by geographical and climatic condition, policies, and socioeconomic status (Wellington et al., 2013). During organ transplantation, antibiotics play an indispensable role in combating nosocomial or secondary infection (Munita and Arias, 2016). The rise of antibiotic resistance had compelled researchers to discover novel antimicrobial agents and formulate polices to prevent disease through contact tracing and quarantine regimes (Roca et al., 2015). It also directly effects the economy through prolonged hospital stays, longer treatments, expensive medicines, and the involvement of manual labor in monitoring to avoid further progression. Antibiotic resistance is of special concern in Asian and African countries with weaker socioeconomic factors, including poor sanitation and general health (MacPherson et al., 2009; Collignon et al., 2018; Hendriksen et al., 2019b).
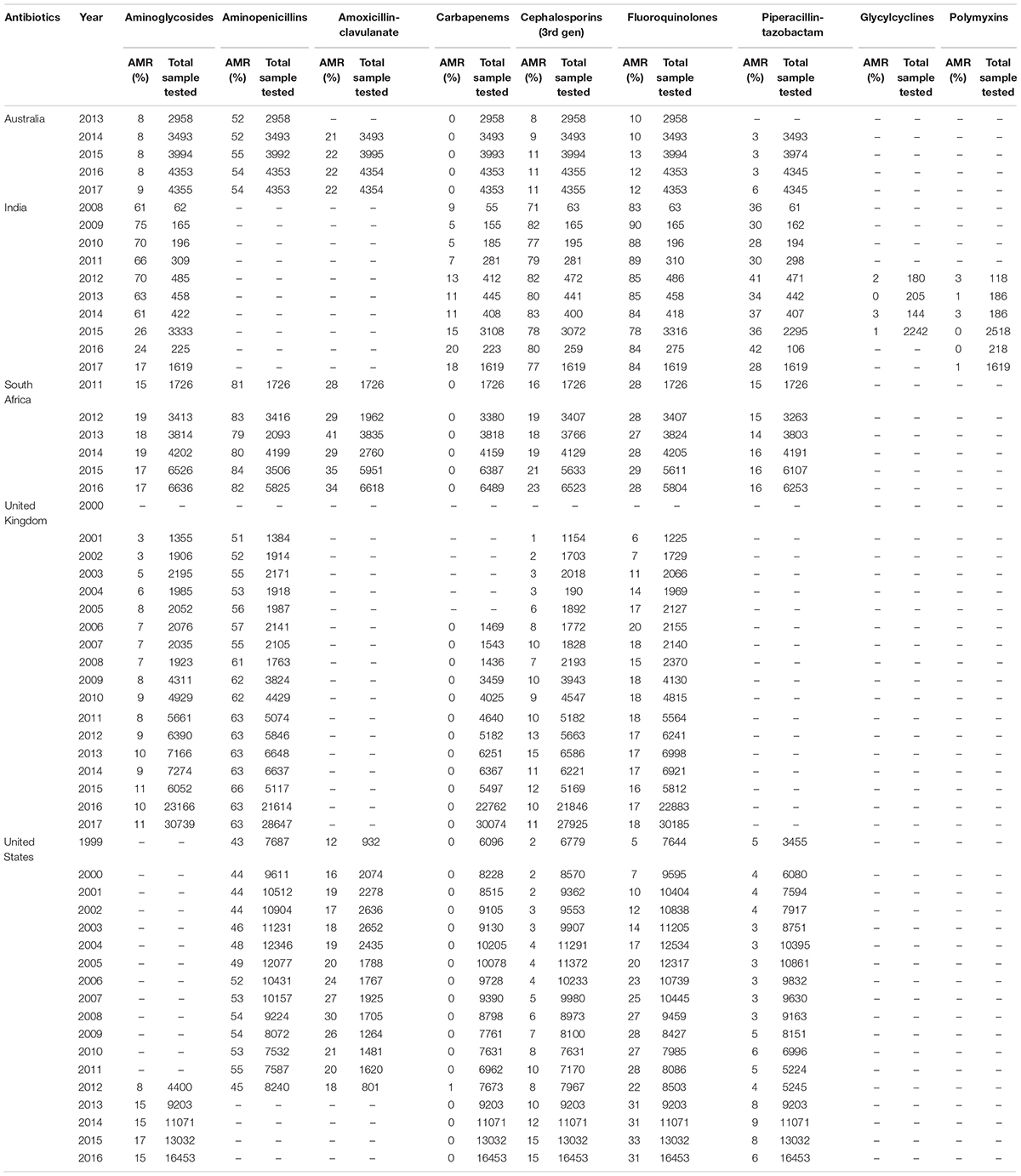
Table 1. Overview of Antibiotic resistance in E. coli toward major antibiotics in Australia, India, South Africa, the United Kingdom, and United States (Source: The Center for Disease, Dynamics Economics & Policy. ResistanceMap: Antibiotic resistance. 2020. https://resistancemap.cddep.org/AntibioticResistance.php. Date accessed: May 31, 2020).
In this review, we explore the applications of whole genome and metagenomes in the surveillance of antibiotic resistant genes in detail (Figure 2), followed by the recent updates in combinatorial drug treatments, nano-particles as antibiotic adjuvants, and drug–microbiome interactions.
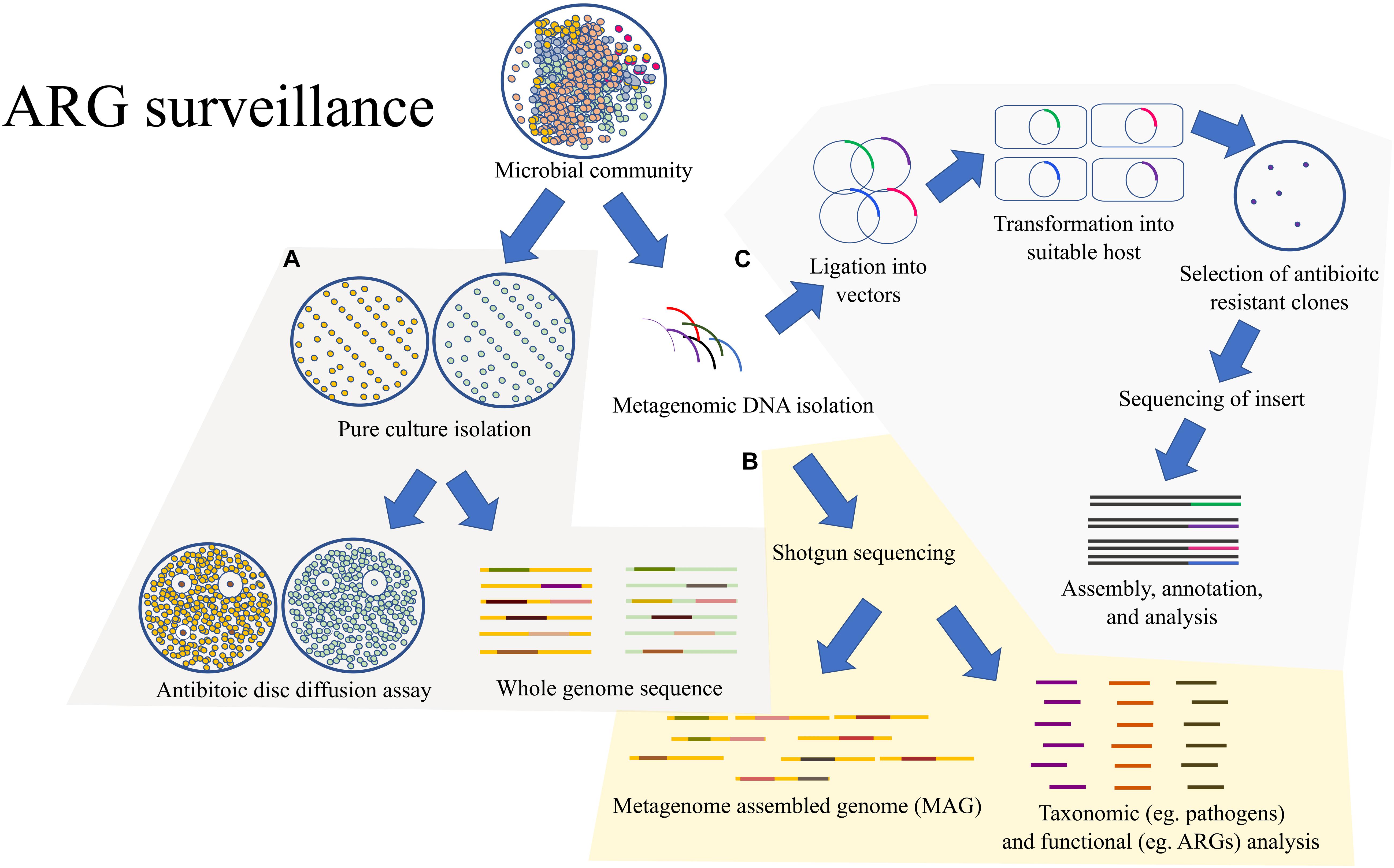
Figure 2. An outline of the methods for surveillance of ARGs: (A) A common traditional approach would rely on the pure culture isolation of the MDR bacteria followed by antibiotic susceptibility test through the twofold serial dilution method. The pure cultures could also be sent for WGS. (B) Rather than isolating pure cultures, the microbial community could be directly processed for shotgun metagenomic analysis which would provide metagenome assembled genomes (MAGs) and valuable information on the taxonomic composition and functional potential of the overall microbial community. (C) The microbial community can also be directly processed for expression-based functional metagenomics for detection of ARGs which might be missed by the shotgun metagenomic approach due to the lack of homology.
Resistome Surveillance Through Whole-Genome Sequencing
Since most pathogens are becoming resistant to a majority of conventional antibiotics, the surveillance of antibiotic resistant genes (ARGs) is an important step in monitoring the spread of antibiotic resistance and its emergence. ARG can be determined by a variety of techniques like microarray, polymerase chain reaction (PCR), and whole-genome sequencing (WGS). Despite advancements in genomic science, detection of antibiotic resistance in clinical laboratories relies on the traditional twofold serial dilution and disk diffusion methods (Hendriksen et al., 2019a). These techniques have been proven to be effective in the formulation of anti-infective therapy. However, for the surveillance of ARGs, such traditional techniques have several disadvantages, such as lack of valid methods for several microbes, differences in laboratory conditions, and also the limitation in the number of tested drugs. ARG surveillance also requires several other pieces of information, such as the comparison of genotypes from different host and environments for route tracking and the degree of dissemination (McDermott et al., 2016; Karp et al., 2017).
Whole-genome sequencing provides the finest level of resolution that comprises all genes within the genome that can be processed for microbial identification, phylogenetic relationships, detecting mutations, putative novel genes identification, retrospective analysis, and predicting phenotypic antibiotic susceptibility. The genome sequence can also provide valuable information on antibiotic resistance traits and its mobility. This is invaluable information in the investigation of outbreaks and real-time surveillance of ARG dissemination (Hendriksen et al., 2019a). Real-time surveillance of ARGs would aid in early recognition of outbreaks and also in the investigation of public health policies (Alghoribi et al., 2018). WGS has helped in several areas, such as detecting the source of outbreaks, track back studies, and forming policies in recalling contaminated food. WGS analysis also provides several other advantages over the traditional resistance testing, such as the predictions of horizontal gene transfer, co-resistance to other antibiotic including heavy meals, and its presence in chromosome or plasmids (Hendriksen et al., 2019a). WGS is currently being used mostly for epidemiological studies since it provides a higher resolution through single-nucleotide variants (SNVs) and multi-locus sequence typing (MLST) than the traditional typing tools (De Been et al., 2015; Schürch and van Schaik, 2017).
The surveillance of ARGs is an important part of the Global Antimicrobial Resistance Surveillance System (GLASS) (World Health Organization, 2015), which aims to generate standardized data that can be compared between nations, track the emergence and spread of ARGs, and serve as guidelines in forming appropriate policies and the allocation of required resources to tackle the ARG threat (Grundmann and Gelband, 2018). In the US, surveillance of ARGs from agriculture, animal, and human health has been taken up by the National Antimicrobial Resistance Monitoring System (NARMS) as a “one health” approach to monitor ARGs and track the transmission route from food that causes human illness (Karp et al., 2017). Similarly, initiatives have already been taken up by European Centre for Disease Prevention and Control (ECDC) in the EU for tracking and investigation of outbreaks (European Centre for Disease Control, 2016). Recent reports from the European Committee on Antimicrobial Susceptibility Testing (EUCAST) have concluded that the WGS-based ARG prediction could soon replace the phenotypic antimicrobial susceptibility testing (AST) for environmental ARG surveillance, which will have no negative impact on individual patients (Ellington et al., 2017). Although several measures have been put forward to address the challenges posed by antibiotic resistance, to overcome the antimicrobial resistance (AMR) crisis, or to at least decelerate the emergence and spread of ARGs, multiple scientific fields, including policy measures and public education, are still important to reduce the inessential usage of antibiotics (Schürch and van Schaik, 2017).
Recent application of WGS for real-time surveillance of multidrug resistant (MDR) pathogens in hospitals have shown that the results are precise with a low turnaround time and are also cost effective (Mellmann et al., 2016). WGS has also been shown to be sensitive in the identification and profiling of antibiotic resistance phenotypes (Bradley et al., 2015; Aanensen et al., 2016). It is also cost effective and provides results quicker than the traditional phenotypic typing, especially for slow-growing pathogens such as Mycobacterium tuberculosis. WGS can also detect pathogens in polymicrobial samples with higher sensitivity than the culture technique (Hasman et al., 2014). WGS also enables the identification of not only ARGs but also the types of alleles. Recent WGS studies have shown an 100% correlation between genotype and phenotypic resistance to tetracycline, ciprofloxacin/nalidixic acid, and erythromycin, indicating that the WGS could be a reliable tool for prediction of antibiotic resistance (Zhao et al., 2016). In another study, WGS analysis of 640 Salmonella isolates identified over 99% correlation between resistance genotypes and phenotypes with an 100% match for macrolide, quinolones, and tetracyclines, except for aminoglycosides and beta-lactams (McDermott et al., 2016). WGS has also been implemented for comparative analysis from contrasting environments. Carbapenem resistant and ESBL-producing K. pneumoniae was found to carry a higher diversity of carbapenemases genes in wastewater isolates while antiseptic-resistance gene qacE was exclusive to clinical samples (Surleac et al., 2020).
Dissemination of ARGs from food and animals to humans remains as a major source of illness. WGS of K. pneumonia isolates from clinical and retail meat, such as turkey, chicken, and pork in Arizona, United States, exhibited close genetic relatedness, with the latter isolates having a higher likelihood of MDR traits, indicating that there is hardly any resistance in the transmission route from meat to humans (Davis et al., 2015). Similarly, a retrospective analysis of ∼24 000 E. coli and Klebsiella spp. genomes from clinical and poultry meat in the United Kingdom identified mcr-1 gene on plasmids with IncHI2, IncI2, and IncX4 replicon types (Doumith et al., 2016). Furthermore, mcr-1 was also detected in E. coli ST131 isolates from chicken meat, which is a rare strain among animal E. coli, although common in human E. coli urinary tract infections (Hasman et al., 2015).
The WGS study on the outbreak of Carbapenem-producing MDR K. pneumonia in the ICU of Shanghai Huashan Hospital in east China revealed that the isolates belonged to ST11 CP-Kp. However, single nucleotide polymorphism (SNP) and phylogenetic analysis indicated that they were of different origins, mainly grouped into two different clades of origination from two index patients (Chen et al., 2019a). Similarly, WGS of E. coli and Klebsiella isolates from patients admitted to the ICU in Maryland Medical Center (UMMC) in Baltimore were identified with blaFOX–5 gene encoded in IncA/C plasmid. However, they had initially tested negative for AmpC β-lactamase, indicating that the resistance could have been acquired in the ICU (Hazen et al., 2014). The spread of ARGs in an intercontinental scale has also recently been observed through WGS analysis of over 1,832 Salmonella enterica serovar Typhi (S. Typhi) from 21 countries, particularly in Asia and Africa (Wong et al., 2015). Multiple transfers of a dominant pathogenic lineage, H58, from Asia to Africa in multiple occasions were inferred from the WGS analysis. They also harbored MDR genes integrated into the chromosome as well as in the IncHI1 plasmids (Wong et al., 2015).
Metagenomic Approaches for Resistome Surveillance
Although whole-genome sequencing is a powerful tool for ARG surveillance, its requirement for pure culture is a major setback, since the majority of the microbes are yet uncultured in the laboratory condition (Steen et al., 2019). This can be sustainably circumvented with the aid of metagenomics. Whole metagenome is the total environmental DNA sequenced through shotgun sequencing technologies bypassing the culturing step. Hence, it offers identification of, theoretically, all viable or non-viable microbes that are culturable as well as those that are not yet cultured It also helps to predict the functional potential and derive the whole-genome sequence. In addition, vast taxonomic data can be analyzed to predict species interactions and the keystone species. The generated data can also be shared globally through various metagenome repositories. In addition, the minimum requirements for infrastructure, laboratory equipment (excluding the NGS sequencer), and the comparatively less controlled environment compared to conventional microbiological laboratories offer a promising future to implement it in low income countries. Hence, metagenomics offers the potential for global surveillance of ARG at a population scale and compared with other environments, which would provide an unprecedented level of information that could serve as guidelines in environmental monitoring and policy making (Duarte et al., 2020). The advancement in shotgun metagenomics has revealed several novel ARGs. Metagenomic data samples from the Red Sea expedition sequenced with GS FLX pyrosequencer identified two novel ARGs, class A beta-lactamase and a thermostable 3’-aminoglycoside phosphotransferase, and its activity were validated in vitro (Elbehery et al., 2017).
Shotgun metagenomics offers high throughput analysis of multiple samples. It mainly depends on the homology-based alignment of the sequence with known genes in a database. Hence, in the last few years, several tools and pipelines have been developed (Table 2) (Niu et al., 2017). A typical reference-based workflow would involve quality assessment of the raw NGS data that includes adaptor removal, trimming of reads with a low phred score, and screening out reads below the minimum threshold length, and could also be followed by removal of the host genome or other unwanted contaminants and a dereplication step. Several tools are available to aid in the quality assessment, such as Cutadapt (Martin, 2011), FastQC (Andrews, 2010), Trimmomatic (Bolger et al., 2014), and fastp (Chen et al., 2018). The clean reads can be directly mapped against a reference database for annotation using bowtie2 (Langmead and Salzberg, 2013), BWA (Burrows-Wheeler transform) (Li and Durbin, 2009), or KMA (k-mer alignment) (Clausen et al., 2018). Direct mapping of the reads could lead to higher false positives. However, they are computationally less demanding and more sensitive to low abundant genes (Boolchandani et al., 2019). Although direct mapping of the clean reads have advantages, a higher accuracy in ARG detection can be achieved through an assembly based method, which also offers information on the genes flanking to the gene of interest (Lal Gupta et al., 2020). In de novo assembly, the quality controlled reads are assembled into contigs using assembler programs such as MEGAHIT (Li et al., 2015), SPAdes (Bankevich et al., 2012), Velvet (Zerbino and Birney, 2008), metaSPAdes (Nurk et al., 2017), IDBA-UD (Peng et al., 2012), and Meta Ray (Boisvert et al., 2012). The contigs are further processed for open reading frame (ORF) prediction using FragGeneScan (Rho et al., 2010) or OrfM (Woodcroft et al., 2016). The predicted ORFs are then aligned to the appropriate ARG database using DIAMOND (Buchfink et al., 2015), VSEARCH (Rognes et al., 2016), or USEARCH (Edgar, 2010). The de novo assembly based approach, despite its several advantages, could lead to data loss on rare genes. To overcome the limitations of read and assembly based methods, long sequencing technologies, such as Pacific Biosciences (PacBio) and Oxford Nanopore, were developed. The read length in NGS has increased substantially in recent years. Assembly of reads, from long reads NGS technologies to short reads, provides a longer continuous or semi-continuous genomic fragment (contigs or scaffolds) that has several advantages. It allows identification of full-length gene(s), gene clusters, polyketide synthase (PKS), and also identification of taxa at a higher resolution than that on unassembled reads (Vollmers et al., 2017). Although assembly provides such benefits, it requires fairly deep sequencing. Metagenomes are also composed of uneven microbial compositions that lead to uneven sequencing depths of the species/strains. This is particularly difficult for the rare species. Although pure cultures can be grown clonally, microbial communities in natural environments often harbor non-clonal cells, which makes it difficult to impose strict parameters in the assembly of overlapping reads (Breitwieser et al., 2018).
Metagenomics have already been implemented in the surveillance of ARGs in various environments. Metagenomic studies of built environments have shown that dust in homes harbors significantly higher ARGs compared to drinking water (Maamar et al., 2020). The authors also detected plasmid borne ARG lnuA (resistance to lincosamides) which was further confirmed in vitro. Build environments, such as biogas plants, posed a significant threat since the digested residue is used as biofertilizers in agriculture and commercial manures. Metagenomics surveillance of ARGs revealed that the thermophilic biogas reactors have a higher ARG removal efficiency than the mesophilic biogas (Luo et al., 2017). Metagenomics studies have also shown ARG enrichment in animal farming upon antibiotic administration (Munk et al., 2017). Nonetheless, ARGs in the gut microbiome of farm animals are well known reservoirs of ARGs, such as tetW, tetQ, mefA, ermB, qepA, qnrB, and, cfxA2, despite the lack of antibiotic selective pressure (Joyce et al., 2019). In addition to animal farming, aquaculture, such as mariculture, has gained considerable interest in recent years. A metagenomic study of mariculture in China identified a similar co-occurrence pattern of ARGs in Proteobacteria and Bacteroidetes. They also identified that Nitrospinae (nitrifying bacteria) were prime ARG hosts (Wang et al., 2018).
Among all reservoirs of ARGs, wastewater treatment plants (WWTPs) remain one of the major conduits of ARGs. Recent studies in Indian WWTPs from small-scale industries were found to be dominated by “ESKAPE pathogens” of MDR genotype; IncP-1 plasmid pKJK5 and pB8 were also detected (Yadav and Kapley, 2019). Similarly, sewage in Montevideo had significantly higher diversity of ARGs, such as TEM-4 and TEM-33 betalactamases, than the beach samples (Fresia et al., 2019). ARGs’ abundance were also found to increase in winter (1.79 × 1012 copies/L) rather than during summer (3.27 × 1011 copies/L) in Chinese WWTPs but no differences were observed based on geography (Su et al., 2017). ARG diversity could also be determined by the types of treatments in the plant. For instance, activated sludge harbor lower (n = 42) ARGs than digested sludge (n = 51) (Guo et al., 2017). Nonetheless, both sludge types harbor plasmids and other mobile elements such as transposons, integrons (intI1), and insertion sequences. Environmental microbes, such as Clostridium and Nitrosomonas, were also found to be the host of multiple ARGs (Guo et al., 2017). Although WWTP influent have high diversity and abundance of ARGs, it is expected since they represent waste disposal of a large population that would include human and animal excreta. The core resistome of the WWTPs were associated to the core microbial community of the WWTP, as well as the human gut microbiome, indicating the influence of humans on the ARGs in the WWTP (Ng et al., 2017; Su et al., 2017). In contrast, the effluent of WWTPs are treated for the removal of pathogens and other microbes. Hence, ARGs should reduce drastically. In line with this, ARGs in the wastewater of hospitals and municipals had reads as high as 197,816 x/Gb which reduced significantly to 2,692 x/Gb after wastewater treatment, which is lower than that of surrounding natural water bodies (7,985 x/Gb) (Ng et al., 2017). However, the core ARGs in the metagenome (wastewater and surface water) were composed of multidrug resistant efflux pumps, resistance to aminoglycoside, macrolide-lincosamide-streptogramins (MLS), quinolones, sulfonamide, and tetracycline resistance. ARGs such as blaKPC, blaCTX–M, blaSHV, and blaTEM were significantly over represented in the wastewater, which were encoded in Tn3-based transposon (Tn4401), indicating their mobility (Ng et al., 2017). Research on virome in hospital wastewater has shown an interesting finding that the phage DNA (0.26%) harbored a higher relative abundance of ARGs than the bacterial DNA (0.18%), particularly the ATP-binding cassette (ABC), Resistance-Nodulation-Cell Division (RND), β-lactamases, plasmid-mediated quinolone resistance, and beta lactam resistance genes (Subirats et al., 2016).
Antibiotic resistant gene surveillance has been aided by tools such as SRST2 (Inouye et al., 2014) and KmerResistance (Clausen et al., 2016), which are widely used for the prediction of ARGs that use alignment and the k-mer approach. However, the setback of such tools is their inability to detect mutations and SNPs that render resistance to antibiotics. Mutations in antibiotic target genes pose a significant threat. Such mutations can be identified from genomes and metagenomes with tools such as Mykrobe predictor (Bradley et al., 2015) and PointFnder (Zankari et al., 2017). Mykrobe predictor utilizes the k-mers approach to identify ARG variants in S. aureus and M. tuberculosis. The PointFinder has two databases that stores information regarding the position(s) of mutated codon(s) and the chromosomal gene database. Using BLASTn, query sequences of ≥80% identity are processed for analysis against the chromosomal mutation database. Recent developments in ARG variant detection includes tools such as Mumame (Mutation Mapping in Metagenomes) (Magesh et al., 2019) and ARIBA (Antimicrobial Resistance Identification By Assembly) (Hunt et al., 2017). ARIBA is a command line tool that accepts paired end reads and detects acquired ARGs, mutations such as deletions, and SNPs (synonymous and non-synonymous).
In addition to the alignment approach, hidden Markov models (HMMs) have been implemented in resistome studies in metagenomic data. The HMM approach involves the initial translation of nucleotide sequences into all six reading frames. The translated sequences are then input for HMM analysis using HMM models constructed with experimentally verified ARG sequences. Using this approach, 12.7 terabytes of genomic data, including metagenomes and nucleotide sequences from NCBI nucleotide database and NCBI RefSeq bacterial genomes, were screened and 362,843 sequences were identified as qnr genes (quinolone resistance genes) (Boulund et al., 2017). Among them, 611 sequences were above 200 bp and 52 were novel qnr genes. The results were reinforced by experimental validation of 20 qnr genes from the study through gene synthesis and expression in E. coli. In another metagenomic study from hospital effluent in Mumbai, India, 112 ARGs and several mobile genetic elements were identified. HMM-based analysis further identified novel metallo-beta-lactamase (MBL) genes under subclass B1 (n = 10), B2 (n = 1), and B3 (n = 10), which were further verified experimentally (n = 6) (Marathe et al., 2019). It is also important to note that the general definition of novel ARG considers a gene to be novel if it has ≤79% amino acid sequence similarly to known ARGs (Levy et al., 1999; Chopra and Roberts, 2001). Hence, several studies have considered ARGs as novel when the amino acid sequence similarity is below 80% (Berman and Riley, 2013; Berglund et al., 2019). However, amino acid sequence identities above 80% have also been considered as novel ARG (i.e., mcr-10 and aar-2) (Yong et al., 2009; Wang et al., 2020).
Homology alignment methods rely on the “best hits” against known ARGs in an existing database, which leads to a high rate of false negatives due to the high cut-off. To overcome such a limitation, machine learning approaches have provided an alternative strategy to the prediction of ARGs. Machine learning in antibiotic resistance prediction requires datasets of known ARG sequences from which the algorithm determines the common characteristics, known as “features,” from the input ARGs (Chowdhury et al., 2020). Machine learning approaches have been considerably successful in ARG prediction and were able to predict ARGs which “best hits” homology alignment failed in identifying (Arango-Argoty et al., 2018). However, machine learning is a considerably new addition in the toolbox of ARG prediction strategies, which also requires a large set of known ARGs to train the models. Hence, the robustness of machine learning approaches are dependent on the underlying model as well as the amount of ARGs in the training dataset. One of the most common machine learning models for ARG prediction from metagenomes is DeepArg (Arango-Argoty et al., 2018). It was trained against ARGs from the Antibiotic Resistance Genes Database (ARDB) (Liu and Pop, 2009), the Comprehensive Antibiotic Resistance Database (CARD) (Jia et al., 2017), and manually curated ARGs from the Universal Protein Resource (UNIPROT) database (Apweiler et al., 2004). Two models were developed: DeepARG-SS for short reads and DeepARG-LS for long sequences. The DeepARG-SS was constructed to predict ARGs from short read sequences while DeepARG-LS predict ARGs from full gene length sequences. The models are trained for over 30 ARG classes with high accuracy. The authors tested the ability of the model against 76 experimentally validated novel metallo beta lactamase genes (Berglund et al., 2017) with 85% accuracy, indicating that the model is capable of predicting novel ARGs. Furthermore, the traditional best hit approach would fail to predict the novel metallo beta lactamase genes, indicating the sensitivity and robustness of machine learning approaches in ARG surveillance (Arango-Argoty et al., 2018). DeepArg has been widely used in several studies that have revealed correlations of total ARGs with anthropogenic impacts in natural environments (Collins-Fairclough et al., 2018; Chen et al., 2019b; Zeng et al., 2019), increased ARGs in murin gut under antibiotic treatments (Korry et al., 2020), and reduction of ARGs in bioreactors upon ozone pre-treatment (Xia et al., 2020).
Short reads from Illumina sequencing remains one of the most used sequencing technologies for metagenomic studies. A novel tool, fARGene, was recently introduced that could identify ARGs from short reads without the need for assembly (Berglund et al., 2019). The tool relies on the optimized gene models that enable the detection of low similarity ARGs or those which were previously uncharacterized. The authors identified 221 β-lactamases from five metagenomic datasets, out of which 81% of 38 novel ARGs, reconstructed through fARGene, were experimentally verified to confer resistance in Escherichia coli. fARGene have been implemented in resistome studies on migratory birds that revealed several novel β-lactamase genes (Cao et al., 2020).
Despite the robustness of such tools, their reliance on sequencing alone poses a major weakness in characterizing ARGs of distant homologies in metagenomic datasets. To overcome this, a pairwise comparative modeling (PCM) system was developed based on 3D structural alignment against known antimicrobial resistance proteins. This enabled the prediction of ARGs of distant homologs to known proteins (Ruppé et al., 2019). This tool is based on the fact that active sites between similar proteins would be conserved, which might not be detected by protein sequencing alone. Using this tool, analysis of over 3 million proteins from human fecal samples predicted 6,095 ARDs (antibiotic resistance determinants) while BLASTP(Altschul, 1990) and DeepARG predicted 67 and 2,139 ARDs respectively, indicating its superiority. Interestingly, the authors also observed that the abundance of some pdARD (predicted ARDs) families declined upon combination of antibiotics treatments. However, the model suffers for predicting ARGs such as mutations in housekeeping genes, and efflux pumps (TetA, QepA, and, mcr-1).
The surveillance of AMR through the metagenomic approach required a harmonized method that should be available for coordinated collaboration between surveillance programs. Massive Open Online Course (MOOC) on ARG detection through metagenomics are available to impart knowledge to the researchers that would serve as a reference material (Duarte et al., 2020).
Discoveries in Novel Antibiotics Resistant Genes Through Expression Based Metagenomic
Functional metagenomics was initially proposed as a novel method to explore the vast uncultured microbial community (Handelsman et al., 1998). Functional metagenomics has emerged as a promising tool for biomining of novel genes, peptides, and secondary metabolites (Johnson et al., 2017). Functional metagenomic pipelines consist of environmental DNA (eDNA) isolation followed by shearing into the desired size. For a small insert library, eDNA is sheared up to 10 kb, while for a large insert library, the insert can range up to 50 kb (Nora et al., 2019). Sheared eDNA is generally blunted and ligated on to a suitable vector and transformed into a suitable host for heterologous expression. Clones with the desired phenotype are selected in a selective media. Functional metagenomics have several advantages over other techniques, such as shotgun metagenomics. It enables the screening of desired genes from the total microbial community, which is not possible with culturable techniques since the majority of the microbes are as yet uncultured. Furthermore, the high throughput nature of the functional metagenomics ensure maximum sequences are screened. The library can be stored, replicated, and reanalyzed for reproducibility. It is also applicable to every environmental sample. Functional metagenomics outperforms shotgun metagenomics since the latter relies on alignment with known genes within a database while the former is independent of any prior knowledge on the sequence information.
With the implementation of functional metagenomics, several novel ARGs have been reported from a diverse set of host and environmental samples (Forsberg et al., 2012; Pehrsson et al., 2013; Ngara and Zhang, 2018). Recently, functional metagenomics from environmental samples identified novel β-lactamase genes BlaCX1 (B1 subclass), BlaCM1 (class A), BlaH33 (B3 subclass), blaRM3 (B3 subclass), and blaAM1 (B3 subclass) (Zhang et al., 2019). Furthermore, a transposase gene, ISPme1, was also identified upstream of BlaCM1, indicating that the antibiotic resistant gene might be mobile. Novel sulfonamide resistance genes AEW9_dhps01, SEW2_dhps01, and SEW5_dhps01, and AEG2_DHPS01 were also detected in forest and grassland soils from Germany, which were relatively unexposed to human intervention (Willms et al., 2019). The study also identified major facilitator superfamily (MFS) efflux pump mediated tetracycline resistance. Multidrug efflux pumps represent a major mechanism in antibiotics resistance in several ecosystems (Imchen et al., 2018). Its broad array of binding ions and compounds for detoxification remains a major obstacle in the fight against antibiotic resistance. Furthermore, recent expression-based metagenomics have revealed 34 novel ARGs in Canadian agricultural soil, most of them representing (multi)drug efflux systems, including PPPAZI-4, that putatively encodes a novel macrolide resistance mechanism (Lau et al., 2017).
Although functional metagenomics is one of most robust approaches for novel gene discoveries without any prior information of the sequence, it has its own limitations. The expression of the insert is dependent on its functionality within the host. For the expression of the desired phenotype, full clusters of the required gene have to be captured in the insert and expressed. Furthermore, expression of foreign gene(s) can also cause perturbation of the host transcriptional profile (Warren et al., 2008), toxicity to the host (Wang et al., 2019), and incompatibility for expression (Jung et al., 2012) due to several factors (such as biased G/C contents) (Pehrsson et al., 2013). For instance, expression of novel antibiotic-resistant genes in E. coli BL21 (DE3) was reported to fail in the sub-cloning due to the toxicity caused by over production of the enzymes while E. coli EC100 successfully expressed the ARG (Zhang et al., 2019). To circumvent these drawbacks, several ranges of hosts and vectors may be used such as Bacillus subtilis (Westers, 2004), Streptomyces lividans (McMahon et al., 2012), Pseudomonas putida (Nagayama et al., 2015), Burkholderia graminis, Agrobacterium tumefaciens, Caulobacter vibrioides, and Ralstonia metallidurans (Craig and Brady, 2011). Although usage of multiple hosts would mean a substantial increase in workload, it does provide higher hits.
In order to circumvent the aforementioned limitation, a metagenomics-guided strategy was introduced recently that bypasses the library preparation step. It involves the regular sequence-based metagenomic detection of ARGs followed by PCR amplification of ARGs with moderate homology (∼40%). The PCR amplicons are cloned directly into a suitable host to validate its expression (Yang et al., 2017). Using this approach, 76 ARGs were identified, out of which 25 novel ARGs with 40-70% homology were validated in vitro.
Another approach recently introduced is the metagenomic cloning of rRNA genes in E. coli Δ7. This particular strain of E. coli has a functional compatibility with rRNA genes from distant phylogenies. Hence, introduction of metagenomic rRNA into the E. coli would render it resistant to spectinomycin if the insert has resistance-conferring mutations. Using this technique, three novel resistance genes, U1183C, C1063U, and U1189C, were identified. Interestingly, introduction of a resistant mutation in E. coli 16S rRNA gene rendered the host resistant to spectinomycin, indicating the host independent resistance of the mutation (Miyazaki and Kitahara, 2018). The authors further describe its potential applications in exploration of aminoglycoside resistant mutation, detection of novel resistance mutation from pathogens, and enrichment of samples in antibiotics to study the presence of novel resistance mutations.
Combinatorial Treatments to Combat Multidrug Resistance
Resistance to antibiotics have increased the dosage recommendation of drugs. However, such an increase in dosage could lead to adverse side effects. A combination of two or more antibiotics with multiple modes of action increases the efficiency and thus reduces the dosage requirement (Table 3). A combination of antibiotics also has synergistic effects and delays the emergence of a drug-resistant mechanism (Tamma et al., 2012). M. tuberculosis is a major causative agent of tuberculosis, which is the leading disease in immunosuppressive patients, such as those with acquired immunodeficiency syndrome (AIDS). The combination of isoniazid and rifapentine for a period of 12 weeks was found effective against M. tuberculosis (Centers for Disease Control and Prevention [Cdc]., 2011). Similarly, in a small scale study from the University of Pittsburgh Medical Center, out of 21 patients, 15 patients successfully recovered from Multidrug-resistant Pseudomonas aeruginosa infections when treated with Ceftolozane-Tazobactam combination (Haidar et al., 2017). However, resistance to Ceftolozane-Tazobactam was developed in three patients within 8 days of treatment, indicating that the resistance to combinatorial drug treatments could be rapid.
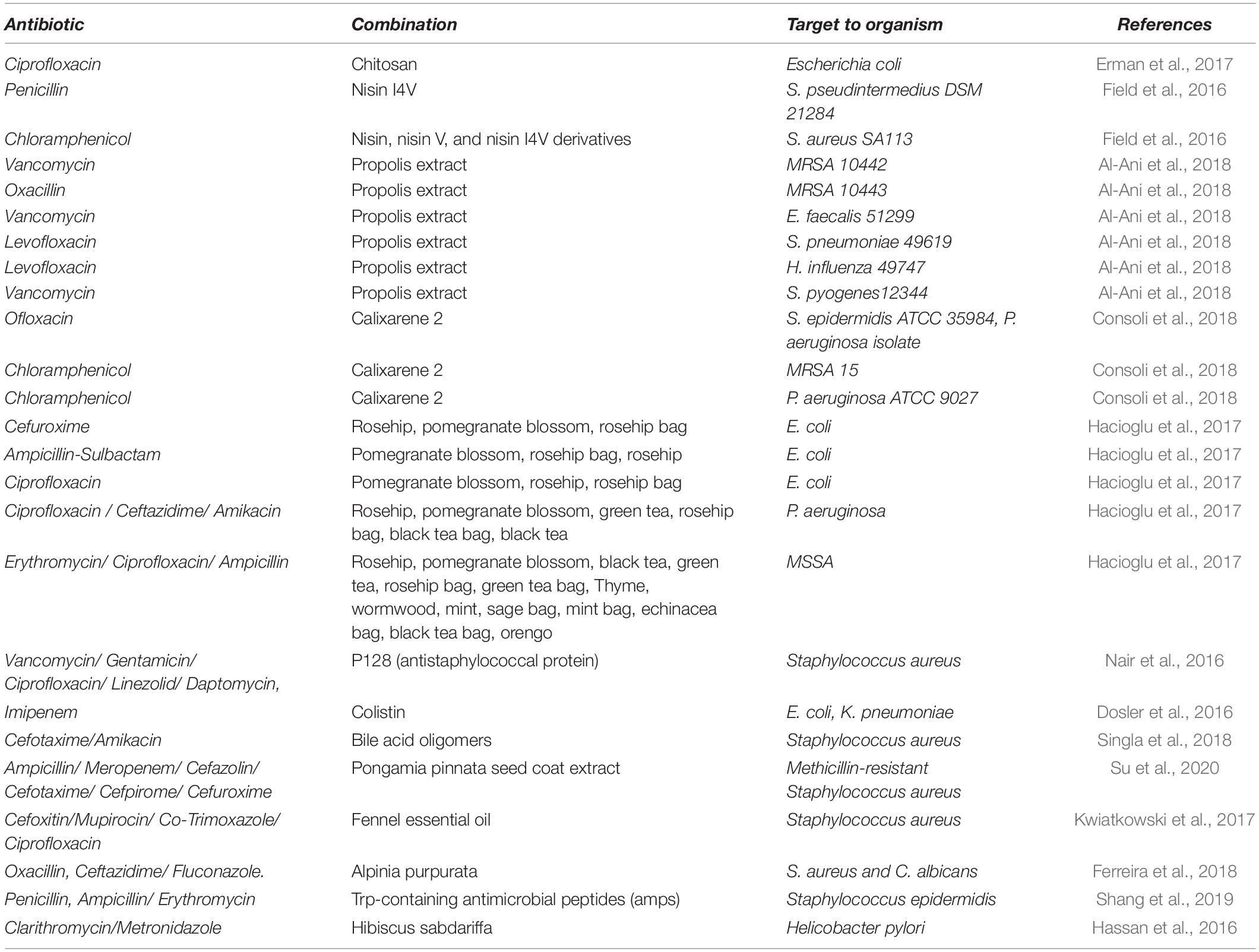
Table 3. Recent formulations of antibiotic combinations with natural compounds towards bacterial pathogen.
The widespread prevalence of antibiotic resistance has highlighted the importance of combinatorial therapy using compounds of various origins as adjuvants (Table 2). Secondary metabolites of plant origin, which are non-antimicrobial, have been found to significantly enhance the effectiveness of traditional antibiotics when administered in combination. The combinatorial administration of phytochemical morin, pyrrolidine, and quercetin with ciprofloxacin, tetracycline, and erythromycin against S. aureus increases the effectiveness of the antibiotics and reduced biofilm formation (Abreu et al., 2016). This was especially true for S. aureus SA1199B overexpressing NorA gene. Interestingly, reserpine and quercetin inhibited efflux pump and the application of quinine and morin reversed the antibiotic tolerance induced by sub-lethal concentrations of ciprofloxacin. Phytosterol stigmasterol in combination with ampicillin showed up to 98.7% inhibition of clinical gram positive and gram negative bacteria (Yenn et al., 2017). In addition to phytochemicals, biosurfactants of various origins have also exhibited effective antibiotic adjuvant properties. The amphiphilic nature of the biosurfactants form stable supramolecular complexes with the bacterial phospholipid membrane to creates pores and disturbs the membrane processes and causes cell lysis. Biosurfactants such as rhamnolipids have been found to have synergistic effects with multiple antibiotics against bacteria and fungus (Chen et al., 2017). Adherent biofilm cells formed by E. coli CFT073 were successfully removed by treatment with biosurfactant from Bacillus licheniformis V9T14 in combination with antibiotics. The combination was more than 90% effective compared to antibiotics or the use of a surfactant alone (Rivardo et al., 2011). Furthermore, dilipid ultrashort cationic lipopeptides (dUSCLs) also enhances the efficacy of several conventional antibiotics, such as chloramphenicol, rifampicin, fosfomycin, ciprofloxacin, and vancomycin, against clinical isolates of MDR P. aeruginosa, Enterobacteriaceae, and Acinetobacter baumannii (Domalaon et al., 2019). The dUSCLs were found to decrease the MIC (minimum inhibitory concentration) of chloramphenicol 32-fold as a result of membrane permeabilization and active efflux distribution.
Biofilm formation by P. aeruginosa is a major pathoadaptation that resists the effectiveness of antibiotic tobramycin therapy in cystic fibrosis (CF) patients. The Food and Drug Administration (FDA) approved triclosan, which was identified as a suitable adjuvant for tobramycin after screening several drug repurposing libraries (n = 6080 compounds) (Maiden et al., 2018). The combination reduced MIC of tobramycin 100-fold, and could even kill persister cells and were effective against Burkholderia cenocepacia and S. aureus. Moreover, triclosan was also found to enhance gentamicin and streptomycin. Non-steroidal anti-inflammatory drugs (NSAIDs) such as aspirin, ibuprofen, and diclofenac have also shown to be effective antimicrobial compounds. Combinations with cefuroxime and chloramphenicol increased the antibiotic activity against MRSA and caused a several-fold reduction in MIC for cefuroxime (up to 8192-fold) and chloramphenicol (up to 64-fold) (Chan et al., 2017). Several other compounds, such as Nebramine-cyclam and EDTA, have also shown synergistic effects with antibiotics against P. aeruginosa (Ammeter et al., 2019; Maisetta et al., 2020).
Nanoparticles as Adjuvants With Antibiotics
To combat this increased resistance to antibiotics, combination therapy of traditional antibiotics with nanoparticles have been proposed that could increase its effectiveness. Nanoparticles are metallic particles, sized between 1 and 100 nm, with a high surface structure area and high binding properties to the target. Nanoparticles alone or in combination with other antibiotics have shown high antibiotic efficiency against drug resistant bacteria with various modes of action (Figure 3). Various metallic nanoparticles are currently available, such as gold, silver, and copper. Single elemental nanoparticles (SENPs) vary in their antimicrobial activity based on the elemental nanoparticles. For instance, tungsten carbide nanoparticles have a weaker antibiotic activity than silver nanoparticles against hospital acquired S. aureus and P. aeruginosa (Bankier et al., 2019). However, SENP combinations of tungsten carbide, silver, and copper have shown significant increases in efficiency (Bankier et al., 2019). Silver nanoparticles (AgNPs) in particular are of special interest. Silver nanoparticles combined with polymixin B and rifampicin have a synergistic effect against carbapenem-resistant Acinetobacter baumannii ABA1604 in vitro as well as in vivo Acinetobacter baumannii-infected mouse models (Wan et al., 2016). The MIC of AgNPs (10–12 μg mL–1) against several gram +ve and –ve bacteria was also observed to exhibit a synergistic effect in combination with kanamycin by increasing bacterial membrane permeability (Vazquez-Muñoz et al., 2019). The authors observed that the synergistic effect of the combination could be due to the disruption of the cell wall that promotes the antibiotic activity. However, AgNPs and β-lactam antibiotics combinations had no synergistic actions, since β-lactam antibiotics and AgNPs act on the cell walls. Similarly, AgNPs combined with β-lactam (ampicillin and penicillin) did not show a synergistic effect against Salmonella typhimurium DT 104 (Deng et al., 2016). On the other hand, AgNPs combined with either enoxacin, kanamycin, neomycin, or tetracycline showed a synergetic effect against Salmonella typhimurium DT 104. The authors found that the antibiotics (except ampicillin and penicillin) could form complexes with AgNPs that promoted AgNPs binding to Salmonella and promotes Ag+ release, which increased Ag+ concentration around Salmonella and inhibited its growth. Hydrophobic AuNPs nanoparticle have also shown synergistic actions with fluoroquinolone against S. aureus, E. coli, and P. Aeruginosa that reduced the MIC of fluoroquinolone 16-fold (Gupta et al., 2017). The synergy was achieved by the downregulation of Tolc-AcrAB efflux pump proteins (bamA, bamD, and bamE), which are involved in detoxification, and also the downregulation of several other proteins involved in lipopolysaccharides synthesis (rfaQ) and other important cellular functions.
In addition to the in vitro antimicrobial properties of nanoparticles, in vivo studies on manganese nanoparticles (MnNPs) in rats (n = 60) have also shown antibacterial properties and significant reductions of wound areas in a dose-dependent manner with no non-cytotoxicity (Mahdavi et al., 2020). Copper sulfide nanoparticles (CuS NPs) were also effective against Aeromonas hydrophila, B. subtilis, and S. aureus infection in zebrafish when administered via injection as well as via a medicated bath (Ayaz Ahmed and Anbazhagan, 2017). Furthermore, inspection of the CuS NPs administered to the zebrafish’s liver and brain showed no toxicity and also the CuS NPs exhibited hemocompatibility to human red blood cells. Nanoparticles for human usage, such as Agicoat and Acticoat for dressing of wounds, are currently commercially available. However, a recent study found that such dressing could cause toxicity in the liver but not in the brain, heart, or lungs in rats (Karimi et al., 2020). Silver nanoparticles have also been implemented to combat against periprosthetic joint infection (PJI) with good biocompatibility (Wei et al., 2017). Although there are broad applications of nanoparticles, it should be noted that nanoparticles are known to attack both healthy as well as microbial cells. However, the advantages of nanoparticles could outweigh the toxicity of the nanoparticles. Normal eukaryotic cells are more resistant to the nanoparticles, owing to the immune system and less affinity compared to microbial cells (You et al., 2012).
Drug–Microbiome Interactions
The influence of antibiotic therapy on the human gut microbiome has been a major area of focus in the past decade. Understanding the impact of antibiotics on the gut microbiome is crucial in order to tackle the ARGs crises. Firstly, similar to increased ARGs in animal farms upon antibiotic administration, an increase in ARGs has also been observed in the human gut microbiome (Yassour et al., 2016). Secondly, dysbiosis of the gut microbiome has been linked to several other side-effects, such as obesity and a mutated microbial community, which could lead to further complications and need for medical care (Korpela et al., 2016; Shashkova et al., 2016). Thirdly, the gut microbiome is also known to degrade antibiotics which could lead to higher dosage requirements.
Antibiotics are an indispensable part of medical science. The misuse of antibiotics is well known to give rise to MDR pathogens. However, the direct side effects of antibiotics on our health have not been properly addressed. Antibiotics are often administered during the intrapartum period to avoid group B Streptococcus (GBS) infection, which is currently one of the major perinatal pathogens, in newborns (Chang et al., 2017; Seale et al., 2017). Although dysbiosis caused by intrapartum antibiotic prophylaxis (IAP) in the newborn gut microbiome appears to recover by 12 weeks (Stearns et al., 2017), higher incidences of ARGs have been reported (Nogacka et al., 2017). Antibiotic treatments in children have also been shown to reduce microbiome diversity with a sharp increase in resistome (Yassour et al., 2016). A study on the oral microbiome of neonates (n = 36) showed that it is composed majorly of maternal oral (65.35%) and placental (3.09%) microbiome and holds no resemblance (0%) to the maternal material gut (Gomez-Arango et al., 2017). Furthermore, the oral microbiome was segregated based on maternal exposure to antibiotics during the intrapartum period, indicating that the maternal antibiotics usage has a profound effect on the neonate Furthermore, ARGs carried in mobile elements persisted for a prolonged period. Usage of macrolide in Finnish children (2–7 years old; n = 142) was associated with long-lasting dysbiosis in microbial composition, such as reduced Actinobacteria and increased Bacteroidetes, while the change in functional aspects were represented by increased macrolide resistance and reduced bile-salt hydrolase (Korpela et al., 2016).
Antibiotic-induced microbiome depletion (AIMD) is a well-known side effect of antibiotic therapy. AIMD in the gut has been linked to anxiety and depression (Leclercq et al., 2017; Macedo et al., 2017; Pryor et al., 2020). It also causes inflammatory signs, including increased myeloid dendritic cells (mDCs) and plasmacytoid dendritic cells (pDCs) in the blood (Hagan et al., 2019). Microbiome dysbiosis induced by the consumption of macrolide is also associated with increased cases of asthma and a higher risk of obesity (Korpela et al., 2016). AIMD reduces short-chain fatty acids (SCFAs), such as butyrate and serum bile acid (BA), which shifts enterocytes to use glucose as the energy source, which ultimately leads to low serum glucose and a disturbance in glucose homeostasis (Zarrinpar et al., 2018). Studies in murine gut microbiomes have shown than amoxicillin treatment leads to blooms of Bacteroides thetaiotaomicron, which upregulate polysaccharide utilization and is further elevated by glucose (Cabral et al., 2019). Antibiotic treatments are also known to cause mutations in the bacterial community (Shashkova et al., 2016).
Recovery of the gut microbiome after antibiotic treatment is an active area of research. Interestingly, dysbiosis of the gut microbiome in humans after antibiotic interventions was found to recover within a week after autologous fecal microbiome transplantation; however, it was prolonged by probiotics which led to changes in taxonomic and functional diversity and lowered bacterial load, leaving the patients susceptible to secondary infections (Suez et al., 2018). Furthermore, in order to protect the microbiota from AIMD, a colon-targeted adsorbent, DAV132, was developed that selectively targets antibiotics and is currently under clinical trial phase 2. Co-administration of DAV132 with moxifloxacin (fluoroquinolones) has been shown to preserve gut microbiota with no adverse effects and also reduce moxifloxacin concentration to 99% in fecal samples (De Gunzburg et al., 2018). Studies of AIMD have shown that the dysbiosis could be predictable based on the initial state of the microbiome. Healthy volunteers (n = 18) under cefprozil treatment had similar changes in the microbiome with increased Lachnoclostridium bolteae in a majority of participants, despite showing variability on an individual level (Raymond et al., 2016). The authors also noted that a subset of participants, having low diversity of Bacteroides enterotype before the treatment, exhibited enriched Enterobacter cloacae after the antibiotic treatments, indicating that the initial status of the microbiome could be a major determinant of the antibiotic therapy outcome.
A majority of the drugs are administered orally, which leaves the drug exposed to various commensals in the gut. Since the human gut microbiota varies at an individual level, the drugs are exposed to unique sets of commensals as per the patient’s microbiome. The human microbiota encodes 150-fold more genes than the human genome. Such a rich reservoir of genes house drug-metabolizing genes that can have various effects on the drugs, such as sulfasalazine activation (Sousa et al., 2014), digoxin inactivation (Haiser et al., 2013), and sorivudine toxification (Okuda et al., 1998). A recent study has shown 271 drugs can be metabolized by 76 human gut bacteria and the drugs with lactones, nitro, azo, and urea groups are more prone to microbial metabolism (Zimmermann et al., 2019). Interestingly, the authors also noted that drug metabolism by the microbiome was significantly correlated to the detection of drug metabolizing gene abundance. Such advances could pave the road to personalized medicine which would provide maximum efficacy and minimal side effects.
Antibiotics at Sublethal Concentration
Although antibiotics are known to inhibit bacterial growth at MIC or lethal concentration, such concentrations are seldom present in the natural environment. Non-lethal concentrations of antibiotics are also observed in clinical situations, such as during a patient’s non-compliance (Sengupta et al., 2013). It is essential to understand the effects of antibiotics at non-lethal concentrations to shed light on the emergence of ARGs in natural as well as clinical settings. Antibiotics have a diverse role beyond the direct inhibition of competing microbes (Walsh, 2013; Yap, 2013). Global transcriptomic analysis of Acinetobacter baumannii under sub-inhibitory concentrations of ciprofloxacin and tetracycline have shown increased ISAba13-mediated mutation, including synonymous and non-synonymous SNP (Fajardo and Martínez, 2008). A multitude of literature has reported on the role of antibiotics as signaling molecules that are involved in quorum sensing, biofilm formation, virulence factors, and host–parasite interaction at sub MIC concentration (Davies, 2009; Anbazhagan et al., 2012). Several antibiotics at sublethal concentrations have been found to induce biofilm formation, indicating that antibiotics could naturally be a signaling molecule (Townsley and Shank, 2017). Recent studies on Phaeobacter inhibens treated with broad spectrum tropodithietic acid (TDA) at 100-fold below minimal inhibitory concentration was found to induce similar transcriptomic profiles to that of the quorum sensing (QS) molecule N-acyl-homoserine lactone (AHL) (Beyersmann et al., 2017). Biofilm formation requires co-ordination among the bacterial cells to aggregate and form a complex structure. Hence, cell–cell interactions through QS is a mandatory function in biofilm formation. A similar effect of TDA to that of QS molecule AHL could indicate that TDA might serve as a signaling molecule in natural settings. Another interesting example is the role of antibiotic prodiginines in Streptomyces coelicolor. The filamentous Streptomycetes reproduce by sporulation and formation of mycelium. During reproduction, older mycelium is consumed as a substrate. Prodiginines are accumulated internally during mycelium differentiation that could serve as programmed cell death and also as a chemical protection of the nutrients from competing microbes (Tenconi et al., 2018). Such studies provide clear evidence that natural antibiotics have a diverse set of roles. Antibiotics at concentrations below MIC have also been found to be beneficial to the susceptible bacteria (Linares et al., 2006). Sublethal concentrations of antibiotics have also been shown to accelerate the emergence of ARGs, and increase mutation rates and horizontal gene transfer (Andersson and Hughes, 2014; Gullberg et al., 2014; Ben et al., 2019). Furthermore, minimal concentrations of antibiotics in WWTP have also been shown to enrich ARGs (Lundström et al., 2016).
Conclusion
Although resistance to antibiotics has made the use of traditional antibiotics obsolete, combinatorial antibiotic therapy together with nanoparticles and phytochemicals could be an effective alternative solution for antibiotic resistance. Since resistance to antibiotics and their alternatives are an inevitable part of microbial evolution, surveillance of ARGs should be considered the first priority in future policy making. Whole genome analysis and metagenomics have made remarkable advancements with the aid of NGS technology and bioinformatics. The results of such techniques are quite reliable and their implementation in routine diagnostic laboratories should be encouraged. In addition to the negative impact of antibiotics’ over-usage, it should be noted that adequate application of antibiotics itself could cause major dysbiosis in the gut microbiome, which ultimately could lead to several side effects.
Author Contributions
RK, MI, DB, ST, PG, and VA conceptualized the review. RK, MI, and JM wrote the manuscript. All the authors read and approved the final manuscript.
Funding
The authors thank Science and Engineering Research Board (SERB) – Empowerment and Equity Opportunities for Excellence in Science (EMEQ)/051/2014 and Empowerment and Equity (EEQ)/2018/001085 for partial financial assistance. MI thanks University Grants Commission – National Fellowship for Higher Education Fellowship, Government of India and the research facilities supported by Central University of Kerala. JM thanks to ICMR-SRF (45/41/2019-BIO/BMS) fellowship Government of India.
Conflict of Interest
The authors declare that the research was conducted in the absence of any commercial or financial relationships that could be construed as a potential conflict of interest.
References
Aanensen, D. M., Feil, E. J., Holden, M. T. G., Dordel, J., Yeats, C. A., Fedosejev, A., et al. (2016). Whole-genome sequencing for routine pathogen surveillance in public health: a population snapshot of invasive Staphylococcus aureus in Europe. MBio 7:e00444-16. doi: 10.1128/mBio.00444-16
Abreu, A. C., Saavedra, M. J., Simões, L. C., and Simões, M. (2016). Combinatorial approaches with selected phytochemicals to increase antibiotic efficacy against Staphylococcus aureus biofilms. Biofouling 32, 1103–1114. doi: 10.1080/08927014.2016.1232402
Al-Ani, I., Zimmermann, S., Reichling, J., and Wink, M. (2018). Antimicrobial activities of european propolis collected from various geographic origins alone and in combination with antibiotics. Medicines 5:2. doi: 10.3390/medicines5010002
Alcock, B. P., Raphenya, A. R., Lau, T. T. Y., Tsang, K. K., Bouchard, M., Edalatmand, A., et al. (2020). CARD 2020: antibiotic resistome surveillance with the comprehensive antibiotic resistance database. Nucleic Acids Res. 48, D517–D525. doi: 10.1093/nar/gkz935
Alghoribi, M. F., Balkhy, H. H., Woodford, N., and Ellington, M. J. (2018). The role of whole genome sequencing in monitoring antimicrobial resistance: a biosafety and public health priority in the Arabian Peninsula. J. Infect. Public Health 11, 784–787. doi: 10.1016/j.jiph.2018.08.001
Altschul, S. (1990). Basic local alignment search tool. J. Mol. Biol. 215, 403–410. doi: 10.1006/jmbi.1990.9999
Ammeter, D., Idowu, T., Zhanel, G. G., and Schweizer, F. (2019). Development of a nebramine-cyclam conjugate as an antibacterial adjuvant to potentiate β-lactam antibiotics against multidrug-resistant P. aeruginosa. J. Antibiot. (Tokyo). 72, 816–826. doi: 10.1038/s41429-019-0221-9
Anbazhagan, D., Mansor, M., Yan, G. O. S., Yusof, M. Y. M., Hassan, H., and Sekaran, S. D. (2012). Detection of quorum sensing signal molecules and identification of an autoinducer synthase gene among biofilm forming clinical isolates of Acinetobacter spp. PLoS One 7:e36696. doi: 10.1371/journal.pone.0036696
Andersson, D. I., and Hughes, D. (2014). Microbiological effects of sublethal levels of antibiotics. Nat. Rev. Microbiol. 12, 465–478. doi: 10.1038/nrmicro3270
Andrews, S. (2010). FastQC: A Quality Control Tool for High Throughput Sequence Data. Available online at: http://www.bioinformatics.babraham.ac.uk/projects/fastqc
Apweiler, R., Bairoch, A., Wu, C. H., Barker, W. C., Boeckmann, B., Ferro, S., et al. (2004). UniProt: the universal protein knowledgebase. Nucleic Acids Res. 32, D115—D119.
Arango-Argoty, G., Garner, E., Pruden, A., Heath, L. S., Vikesland, P., and Zhang, L. (2018). DeepARG: a deep learning approach for predicting antibiotic resistance genes from metagenomic data. Microbiome 6:23.
Arango-Argoty, G. A., Guron, G. K. P., Garner, E., Riquelme, M. V., Heath, L. S., Pruden, A., et al. (2020). ARGminer: a web platform for the crowdsourcing-based curation of antibiotic resistance genes. Bioinformatics 36, 2966–2973.
Ayaz Ahmed, K. B., and Anbazhagan, V. (2017). Synthesis of copper sulfide nanoparticles and evaluation of in vitro antibacterial activity and in vivo therapeutic effect in bacteria-infected zebrafish. RSC Adv. 7, 36644–36652. doi: 10.1039/c7ra05636b
Bankevich, A., Nurk, S., Antipov, D., Gurevich, A. A., Dvorkin, M., Kulikov, A. S., et al. (2012). SPAdes: a new genome assembly algorithm and its applications to single-cell sequencing. J. Comput. Biol. 19, 455–477. doi: 10.1089/cmb.2012.0021
Bankier, C., Matharu, R. K., Cheong, Y. K., Ren, G. G., Cloutman-Green, E., and Ciric, L. (2019). Synergistic antibacterial effects of metallic nanoparticle combinations. Sci. Rep. 9:16074. doi: 10.1038/s41598-019-52473-2
Baron, S. (1996). Medical Microbiology, 4th Edn. Galveston, TX: University of Texas Medical Branch at Galveston.
Ben, Y., Fu, C., Hu, M., Liu, L., Wong, M. H., and Zheng, C. (2019). Human health risk assessment of antibiotic resistance associated with antibiotic residues in the environment: a review. Environ. Res. 169, 483–493. doi: 10.1016/j.envres.2018.11.040
Berglund, F., Marathe, N. P., Österlund, T., Bengtsson-Palme, J., Kotsakis, S., Flach, C. F., et al. (2017). Identification of 76 novel B1 metallo-β-lactamases through large-scale screening of genomic and metagenomic data. Microbiome 5:134. doi: 10.1186/s40168-017-0353-8
Berglund, F., Österlund, T., Boulund, F., Marathe, N. P., Larsson, D. G. J., and Kristiansson, E. (2019). Identification and reconstruction of novel antibiotic resistance genes from metagenomes. Microbiome 7:52. doi: 10.1186/s40168-019-0670-1
Berman, H. F., and Riley, L. W. (2013). Identification of novel antimicrobial resistance genes from microbiota on retail spinach. BMC Microbiol. 13:272. doi: 10.1186/1471-2180-13-272
Beyersmann, P. G., Tomasch, J., Son, K., Stocker, R., Göker, M., Wagner-Döbler, I., et al. (2017). Dual function of tropodithietic acid as antibiotic and signaling molecule in global gene regulation of the probiotic bacterium Phaeobacter inhibens. Sci. Rep. 7:730. doi: 10.1038/s41598-017-00784-7
Boisvert, S., Raymond, F., Godzaridis, É, Laviolette, F., and Corbeil, J. (2012). Ray meta: scalable de novo metagenome assembly and profiling. Genome Biol. 13:R122. doi: 10.1186/gb-2012-13-12-r122
Bolger, A. M., Lohse, M., and Usadel, B. (2014). Trimmomatic: a flexible trimmer for Illumina sequence data. Bioinformatics 30, 2114–2120. doi: 10.1093/bioinformatics/btu170
Boolchandani, M., D’Souza, A. W., and Dantas, G. (2019). Sequencing-based methods and resources to study antimicrobial resistance. Nat. Rev. Genet. 20, 356–370. doi: 10.1038/s41576-019-0108-4
Boulund, F., Berglund, F., Flach, C. F., Bengtsson-Palme, J., Marathe, N. P., Larsson, D. J., et al. (2017). Computational discovery and functional validation of novel fluoroquinolone resistance genes in public metagenomic data sets. BMC Genom. 18:682. doi: 10.1186/s12864-017-4064-0
Bradley, P., Gordon, N. C., Walker, T. M., Dunn, L., Heys, S., Huang, B., et al. (2015). Rapid antibiotic-resistance predictions from genome sequence data for Staphylococcus aureus and Mycobacterium tuberculosis. Nat. Commun. 6:10063. doi: 10.1038/ncomms10063
Breitwieser, F. P., Lu, J., and Salzberg, S. L. (2018). A review of methods and databases for metagenomic classification and assembly. Brief. Bioinform. 20, 1125–1139. doi: 10.1093/bib/bbx120
Buchfink, B., Xie, C., and Huson, D. H. (2015). Fast and sensitive protein alignment using DIAMOND. Nat. Methods 12, 59–60. doi: 10.1038/nmeth.3176
Cabral, D. J., Penumutchu, S., Reinhart, E. M., Zhang, C., Korry, B. J., Wurster, J. I., et al. (2019). Microbial metabolism modulates antibiotic susceptibility within the murine gut microbiome. Cell Metab. 30:800-823.e7. doi: 10.1016/j.cmet.2019.08.020
Cao, J., Hu, Y., Liu, F., Wang, Y., Bi, Y., Lv, N., et al. (2020). Metagenomic analysis reveals the microbiome and resistome in migratory birds. Microbiome 8:26. doi: 10.1186/s40168-019-0781-8
Centers for Disease Control and Prevention [Cdc]. (2011). Recommendations for use of an isoniazid-rifapentine regimen with direct observation to treat latent Mycobacterium tuberculosis infection. MMWR. Morb. Mortal. Wkly. Rep. 60, 1650–1653.
Chan, E. W. L., Yee, Z. Y., Raja, I., and Yap, J. K. Y. (2017). Synergistic effect of non-steroidal anti-inflammatory drugs (NSAIDs) on antibacterial activity of cefuroxime and chloramphenicol against methicillin-resistant Staphylococcus aureus. J. Glob. Antimicrob. Resist. 10, 70–74. doi: 10.1016/j.jgar.2017.03.012
Chang, F. W., Lee, C. I., Fan, H. C., Su, H. Y., Liu, Y. L., and Chen, C. Y. (2017). The impact of prenatal group B streptococcus screening as a national health policy in Taiwan. Taiwan J. Obstet. Gynecol. 56, 648–651. doi: 10.1016/j.tjog.2017.08.013
Charest, M. G., Siegel, D. R., and Myers, A. G. (2005). Synthesis of (-)-tetracycline. J. Am. Chem. Soc. 127, 8292–8293.
Chen, C., Zhang, Y., Yu, S. L., Zhou, Y., Yang, S. Y., Jin, J. L., et al. (2019a). Tracking carbapenem-producing Klebsiella pneumoniae outbreak in an intensive care unit by whole genome sequencing. Front. Cell. Infect. Microbiol. 9:281. doi: 10.3389/fcimb.2019.00281
Chen, H., Jing, L., Yao, Z., Meng, F., and Teng, Y. (2019b). Prevalence, source and risk of antibiotic resistance genes in the sediments of Lake Tai (China) deciphered by metagenomic assembly: a comparison with other global lakes. Environ. Int. 127, 267–275. doi: 10.1016/j.envint.2019.03.048
Chen, J., Wu, Q., Hua, Y., Chen, J., Zhang, H., and Wang, H. (2017). Potential applications of biosurfactant rhamnolipids in agriculture and biomedicine. Appl. Microbiol. Biotechnol. 101, 8309–8319. doi: 10.1007/s00253-017-8554-4
Chen, S., Zhou, Y., Chen, Y., and Gu, J. (2018). Fastp: an ultra-fast all-in-one FASTQ preprocessor. Bioinformatics 34, i884–i890. doi: 10.1093/bioinformatics/bty560
Chopra, I., and Roberts, M. (2001). Tetracycline antibiotics: mode of action, applications, molecular biology, and epidemiology of bacterial resistance. Microbiol. Mol. Biol. Rev. 65, 232–260. doi: 10.1128/mmbr.65.2.232-260.2001
Chowdhury, A. S., Call, D. R., and Broschat, S. L. (2020). Publisher correction: antimicrobial resistance prediction for gram-negative bacteria via game theory-based feature evaluation. Sci. Rep. 10:1846. doi: 10.1038/s41598-020-58759-0
Clausen, P. T. L. C., Aarestrup, F. M., and Lund, O. (2018). Rapid and precise alignment of raw reads against redundant databases with KMA. BMC Bioinformatics 19:307. doi: 10.1186/s12859-018-2336-6
Clausen, P. T. L. C., Zankari, E., Aarestrup, F. M., and Lund, O. (2016). Benchmarking of methods for identification of antimicrobial resistance genes in bacterial whole genome data. J. Antimicrob. Chemother. 71, 2484–2488. doi: 10.1093/jac/dkw184
Collignon, P., Beggs, J. J., Walsh, T. R., Gandra, S., and Laxminarayan, R. (2018). Anthropological and socioeconomic factors contributing to global antimicrobial resistance: a univariate and multivariable analysis. Lancet Planet. Heal. 2, e398–e405.
Collins-Fairclough, A. M., Co, R., Ellis, M. C., and Hug, L. A. (2018). Widespread antibiotic, biocide, and metal resistance in microbial communities inhabiting a municipal waste environment and anthropogenically impacted river. mSphere 3:e00346-18. doi: 10.1128/msphere.00346-18
Consoli, G. M. L., Granata, G., Picciotto, R., Blanco, A. R., Geraci, C., Marino, A., et al. (2018). Design, synthesis and antibacterial evaluation of a polycationic calix[4]arene derivative alone and in combination with antibiotics. Medchemcomm 9, 160–164. doi: 10.1039/c7md00527j
Craig, J. W., and Brady, S. F. (2011). “Expanding small-molecule functional metagenomics through parallel screening of broad host-range cosmid environmental Dna libraries in diverse Proteobacteria,” in in Handbook of Molecular Microbial Ecology II: Metagenomics in Different Habitats, eds F. J. de Bruijn and W. Blackwell, (Hoboken, NJ: John Wiley & Sons, Inc), 507–515. doi: 10.1002/9781118010549.ch49
Davis, G. S., Waits, K., Nordstrom, L., Weaver, B., Aziz, M., Gauld, L., et al. (2015). Intermingled Klebsiella pneumoniae populations between retail meats and human urinary tract infections. Clin. Infect. Dis. 61, 892–899. doi: 10.1093/cid/civ428
Davis, J. J., Boisvert, S., Brettin, T., Kenyon, R. W., Mao, C., Olson, R., et al. (2016). Antimicrobial resistance prediction in PATRIC and RAST. Sci. Rep. 6:27930. doi: 10.1038/srep27930
De Been, M., Pinholt, M., Top, J., Bletz, S., Mellmann, A., Van Schaik, W., et al. (2015). Core genome multilocus sequence typing scheme for high-resolution typing of enterococcus faecium. J. Clin. Microbiol. 53, 3788–3797. doi: 10.1128/JCM.01946-15
De Gunzburg, J., Ghozlane, A., Ducher, A., Le Chatelier, E., Duval, X., Ruppé, E., et al. (2018). Protection of the human gut microbiome from antibiotics. J. Infect. Dis. 217, 628–636. doi: 10.1093/infdis/jix604
de Lima Procópio, R. E., da Silva, I. R., Martins, M. K., de Azevedo, J. L., and de Araújo, J. M. (2012). Antibiotics produced by streptomyces. Brazilian J. Infect. Dis. 16, 466–471. doi: 10.1016/j.bjid.2012.08.014
de Man, T. J. B., and Limbago, B. M. (2016). SSTAR, a stand-alone easy-to-use antimicrobial resistance gene predictor. mSphere 1, e50–e15. doi: 10.1128/msphere.00050-15
Deng, H., McShan, D., Zhang, Y., Sinha, S. S., Arslan, Z., Ray, P. C., et al. (2016). Mechanistic study of the synergistic antibacterial activity of combined silver nanoparticles and common antibiotics. Environ. Sci. Technol. 50, 8840–8848. doi: 10.1021/acs.est.6b00998
Domalaon, R., Brizuela, M., Eisner, B., Findlay, B., Zhanel, G. G., and Schweizer, F. (2019). Dilipid ultrashort cationic lipopeptides as adjuvants for chloramphenicol and other conventional antibiotics against Gram-negative bacteria. Amino Acids 51, 383–393. doi: 10.1007/s00726-018-2673-9
Dosler, S., Karaaslan, E., and Alev Gerceker, A. (2016). Antibacterial and anti-biofilm activities of melittin and colistin, alone and in combination with antibiotics against Gram-negative bacteria. J. Chemother. 28, 95–103. doi: 10.1179/1973947815Y.0000000004
Doumith, M., Godbole, G., Ashton, P., Larkin, L., Dallman, T., Day, M., et al. (2016). Detection of the plasmid-mediated mcr-1 gene conferring colistin resistance in human and food isolates of Salmonella enterica and Escherichia coli in England and Wales. J. Antimicrob. Chemother. 71, 2300–2305. doi: 10.1093/jac/dkw093
Duarte, A. S. R., Stärk, K. D. C., Munk, P., Leekitcharoenphon, P., Bossers, A., Luiken, R., et al. (2020). Addressing learning needs on the use of metagenomics in antimicrobial resistance surveillance. Front. Public Heal. 8:38. doi: 10.3389/fpubh.2020.00038
Edgar, R. C. (2010). Search and clustering orders of magnitude faster than BLAST. Bioinformatics 26, 2460–2461. doi: 10.1093/bioinformatics/btq461
Elbehery, A. H. A., Leak, D. J., and Siam, R. (2017). Novel thermostable antibiotic resistance enzymes from the Atlantis II Deep Red Sea brine pool. Microb. Biotechnol. 10, 189–202. doi: 10.1111/1751-7915.12468
Ellington, M. J., Ekelund, O., Aarestrup, F. M., Canton, R., Doumith, M., Giske, C., et al. (2017). The role of whole genome sequencing in antimicrobial susceptibility testing of bacteria: report from the EUCAST Subcommittee. Clin. Microbiol. Infect. 23, 2–22. doi: 10.1016/j.cmi.2016.11.012
Erman, A., Hergouth, V. K., Blango, M. G., Kos, M. K., Mulvey, M. A., and Veranič, P. (2017). Repeated treatments with Chitosan in combination with antibiotics completely eradicate uropathogenic Escherichia coli from infected mouse urinary bladders. J. Infect. Dis. 216, 375–381. doi: 10.1093/infdis/jix023
European Centre for Disease Control (2016). Expert Opinion on Whole Genome Sequencing for Public Health Surveillance Strategy to Harness Whole Genome Sequencing to Strengthen EU Outbreak Investigations and Public Health Surveillance. Stocholm: European Centre for Disease Control
Fajardo, A., and Martínez, J. L. (2008). Antibiotics as signals that trigger specific bacterial responses. Curr. Opin. Microbiol. 11, 161–167. doi: 10.1016/j.mib.2008.02.006
Feldgarden, M., Brover, V., Haft, D. H., Prasad, A. B., Slotta, D. J., Tolstoy, I., et al. (2019). Validating the AMRFINder tool and resistance gene database by using antimicrobial resistance genotype-phenotype correlations in a collection of isolates. Antimicrob. Agents Chemother. 63:e00483-19. doi: 10.1128/AAC.00483-19
Ferreira, G. R. S., Brito, J., de, S., Procópio, T. F., Santos, N. D., de, L., et al. (2018). Antimicrobial potential of Alpinia purpurata lectin (ApuL): growth inhibitory action, synergistic effects in combination with antibiotics, and antibiofilm activity. Microb. Pathog. 124, 152–162. doi: 10.1016/j.micpath.2018.08.027
Field, D., O’Connor, R., Cotter, P. D., Ross, R. P., and Hill, C. (2016). In vitro activities of nisin and nisin derivatives alone and in combination with antibiotics against Staphylococcus biofilms. Front. Microbiol. 7:508. doi: 10.3389/fmicb.2016.00508
Flandrois, J.-P., Lina, G., and Dumitrescu, O. (2014). MUBII-TB-DB: a database of mutations associated with antibiotic resistance in Mycobacterium tuberculosis. BMC Bioinform. 15:107. doi: 10.1186/1471-2105-15-107
Forsberg, K. J., Reyes, A., Wang, B., Selleck, E. M., Sommer, M. O. A., and Dantas, G. (2012). The shared antibiotic resistome of soil bacteria and human pathogens. Science 337, 1107–1111. doi: 10.1126/science.1220761
Fresia, P., Antelo, V., Salazar, C., Giménez, M., D’Alessandro, B., Afshinnekoo, E., et al. (2019). Urban metagenomics uncover antibiotic resistance reservoirs in coastal beach and sewage waters. Microbiome 7:35. doi: 10.1186/s40168-019-0648-z
Gaynes, R. (2017). The discovery of penicillin—new insights after more than 75 years of clinical use. Emerg. Infect. Dis. 23, 849–853. doi: 10.3201/eid2305.161556
Gomez-Arango, L. F., Barrett, H. L., McIntyre, H. D., Callaway, L. K., Morrison, M., and Nitert, M. D. (2017). Antibiotic treatment at delivery shapes the initial oral microbiome in neonates. Sci. Rep. 7:43481.
Grundmann, H., and Gelband, H. (2018). Antimicrobial resistance surveillance with whole genome sequencing in Africa: It’s (about) time. Afr. J. Lab. Med. 7:761. doi: 10.4102/ajlm.v7i2.761
Gullberg, E., Albrecht, L. M., Karlsson, C., Sandegren, L., and Andersson, D. I. (2014). Selection of a multidrug resistance plasmid by sublethal levels of antibiotics and heavy metals. MBio 5:e01918-14. doi: 10.1128/mBio.01918-14
Guo, J., Li, J., Chen, H., Bond, P. L., and Yuan, Z. (2017). Metagenomic analysis reveals wastewater treatment plants as hotspots of antibiotic resistance genes and mobile genetic elements. Water Res. 123, 468–478. doi: 10.1016/j.watres.2017.07.002
Gupta, A., Saleh, N. M., Das, R., Landis, R. F., Bigdeli, A., Motamedchaboki, K., et al. (2017). Synergistic antimicrobial therapy using nanoparticles and antibiotics for the treatment of multidrug-resistant bacterial infection. Nano Futur. 1:015004. doi: 10.1088/2399-1984/aa69fb
Gupta, S. K., Padmanabhan, B. R., Diene, S. M., Lopez-Rojas, R., Kempf, M., Landraud, L., et al. (2014). ARG-ANNOT, a new bioinformatic tool to discover antibiotic resistance genes in bacterial genomes. Antimicrob. Agents Chemother. 58, 212–220.
Hacioglu, M., Dosler, S., Birteksoz Tan, A. S., and Otuk, G. (2017). Antimicrobial activities of widely consumed herbal teas, alone or in combination with antibiotics: an in vitro study. PeerJ 2017:e3467. doi: 10.7717/peerj.3467
Hagan, T., Cortese, M., Rouphael, N., Boudreau, C., Linde, C., Maddur, M. S., et al. (2019). Antibiotics-Driven gut microbiome perturbation alters immunity to vaccines in humans. Cell 178, 1313.e–1328.e. doi: 10.1016/j.cell.2019.08.010
Haidar, G., Philips, N. J., Shields, R. K., Snyder, D., Cheng, S., Potoski, B. A., et al. (2017). Ceftolozane-Tazobactam for the treatment of multidrug-resistant Pseudomonas aeruginosa infections: clinical effectiveness and evolution of resistance. Clin. Infect. Dis. 65, 110–120. doi: 10.1093/cid/cix182
Haiser, H. J., Gootenberg, D. B., Chatman, K., Sirasani, G., Balskus, E. P., and Turnbaugh, P. J. (2013). Predicting and manipulating cardiac drug inactivation by the human gut bacterium Eggerthella lenta. Science 341, 295–298. doi: 10.1126/science.1235872
Handelsman, J., Rondon, M. R., Brady, S. F., Clardy, J., and Goodman, R. M. (1998). Molecular biological access to the chemistry of unknown soil microbes: a new frontier for natural products. Chem. Biol. 5, R245–R249. doi: 10.1016/S1074-5521(98)90108-9
Hasman, H., Clausen, P. T. L. C., Kaya, H., Hansen, F., Knudsen, J. D., Wang, M., et al. (2019). LRE-Finder, a Web tool for detection of the 23S rRNA mutations and the optrA, cfr, cfr(B) and poxtA genes encoding linezolid resistance in enterococci from whole-genome sequences. J. Antimicrob. Chemother. 74, 1473–1476. doi: 10.1093/jac/dkz092
Hasman, H., Hammerum, A. M., Hansen, F., Hendriksen, R. S., Olesen, B., Agersø, Y., et al. (2015). Detection of mcr-1 encoding plasmid-mediated colistin-resistant escherichia coli isolates from human bloodstream infection and imported chicken meat, denmark 2015. Eurosurveillance 20, 1–5. doi: 10.2807/1560-7917.ES.2015.20.49.30085
Hasman, H., Saputra, D., Sicheritz-Ponten, T., Lund, O., Svendsen, C. A., Frimodt-Moller, N., et al. (2014). Rapid whole-genome sequencing for detection and characterization of microorganisms directly from clinical samples. J. Clin. Microbiol. 52, 139–146. doi: 10.1128/JCM.02452-13
Hassan, S. T. S., Berchová, K., Majerová, M., Pokorná, M., and Švajdlenka, E. (2016). In vitro synergistic effect of Hibiscus sabdariffa aqueous extract in combination with standard antibiotics against Helicobacter pylori clinical isolates. Pharm. Biol. 54, 1736–1740. doi: 10.3109/13880209.2015.1126618
Hazen, T. H., Zhao, L., Boutin, M. A., Stancil, A., Robinson, G., Harris, A. D., et al. (2014). Comparative genomics of an IncA/C multidrug resistance plasmid from Escherichia coli and Klebsiella isolates from intensive care unit patients and the utility of whole-genome sequencing in health care settings. Antimicrob. Agents Chemother. 58, 4814–4825.
Hendriksen, R. S., Bortolaia, V., Tate, H., Tyson, G. H., Aarestrup, F. M., and McDermott, P. F. (2019a). Using genomics to track global antimicrobial resistance. Front. Public Heal. 7:242. doi: 10.3389/fpubh.2019.00242
Hendriksen, R. S., Munk, P., Njage, P., Van Bunnik, B., McNally, L., Lukjancenko, O., et al. (2019b). Global monitoring of antimicrobial resistance based on metagenomics analyses of urban sewage. Nat. Commun. 10:1124.
Hunt, M., Mather, A. E., Sánchez-Busó, L., Page, A. J., Parkhill, J., Keane, J. A., et al. (2017). ARIBA: rapid antimicrobial resistance genotyping directly from sequencing reads. Microb. Genomics 3:e000131. doi: 10.1099/mgen.0.000131
Imchen, M., Kumavath, R., Barh, D., Vaz, A., Góes-Neto, A., Tiwari, S., et al. (2018). Comparative mangrove metagenome reveals global prevalence of heavy metals and antibiotic resistome across different ecosystems. Sci. Rep. 8:11187. doi: 10.1038/s41598-018-29521-4
Inouye, M., Dashnow, H., Raven, L. A., Schultz, M. B., Pope, B. J., Tomita, T., et al. (2014). SRST2: rapid genomic surveillance for public health and hospital microbiology labs. Genome Med. 6:90. doi: 10.1186/s13073-014-0090-6
Jia, B., Raphenya, A. R., Alcock, B., Waglechner, N., Guo, P., Tsang, K. K., et al. (2017). CARD 2017: expansion and model-centric curation of the comprehensive antibiotic resistance database. Nucleic Acids Res. 45, D566–D573. doi: 10.1093/nar/gkw1004
Johnson, J., Jain, K., and Madamwar, D. (2017). “functional metagenomics: exploring nature’s gold mine,” in Current Developments in Biotechnology and Bioengineering: Functional Genomics and Metabolic Engineering, eds P. Gunasekaran, N. Santosh, and P. Ashok, (Amsterdam: Elsevier Inc), 27–43. doi: 10.1016/B978-0-444-63667-6.00002-X
Joyce, A., McCarthy, C. G. P., Murphy, S., and Walsh, F. (2019). Antibiotic resistomes of healthy pig faecal metagenomes. Microb. Genom. 5:e000272. doi: 10.1099/mgen.0.000272
Jung, S. K., Parisutham, V., Jeong, S. H., and Lee, S. K. (2012). Heterologous expression of plant cell wall degrading enzymes for effective production of cellulosic biofuels. J. Biomed. Biotechnol. 2012:405842. doi: 10.1155/2012/405842
Karimi, H., Latifi, N. A., Mehrjerdi, A. Z., Jafarnejad, B., and Karimi, A. M. (2020). Histopathological changes of organs (lungs, liver, kidney, and brain) after using two types of agicoat and acticoat nanosilver dressings on deep second-degree burn in rat. J. Burn Care Res. 41, 141–150. doi: 10.1093/jbcr/irz137
Karp, B. E., Tate, H., Plumblee, J. R., Dessai, U., Whichard, J. M., Thacker, E. L., et al. (2017). National antimicrobial resistance monitoring system: two decades of advancing public health through integrated surveillance of antimicrobial resistance. Foodborne Pathog. Dis. 14, 545–557.
Khameneh, B., Iranshahy, M., Soheili, V., and Fazly Bazzaz, B. S. (2019). Review on plant antimicrobials: a mechanistic viewpoint. Antimicrob. Resist. Infect. Control 8:118. doi: 10.1186/s13756-019-0559-6
Korpela, K., Salonen, A., Virta, L. J., Kekkonen, R. A., Forslund, K., Bork, P., et al. (2016). Intestinal microbiome is related to lifetime antibiotic use in Finnish pre-school children. Nat. Commun. 7:10410. doi: 10.1038/ncomms10410
Korry, B. J., Cabral, D. J., and Belenky, P. (2020). Metatranscriptomics reveals antibiotic-induced resistance gene expression in the murine gut microbiota. Front. Microbiol. 11:322. doi: 10.3389/fmicb.2020.00322
Kwiatkowski, P., Mnichowska-Polanowska, M., Pruss, A., Masiuk, H., Dziȩcioł, M., Giedrys-Kalemba, S., et al. (2017). The effect of fennel essential oil in combination with antibiotics on Staphylococcus aureus strains isolated from carriers. Burns 43, 1544–1551. doi: 10.1016/j.burns.2017.04.014
Lakin, S. M., Dean, C., Noyes, N. R., Dettenwanger, A., Ross, A. S., Doster, E., et al. (2017). MEGARes: an antimicrobial resistance database for high throughput sequencing. Nucleic Acids Res. 45, D574–D580. doi: 10.1093/nar/gkw1009
Lal Gupta, C., Kumar Tiwari, R., and Cytryn, E. (2020). Platforms for elucidating antibiotic resistance in single genomes and complex metagenomes. Environ. Int. 138:105667. doi: 10.1016/j.envint.2020.105667
Langmead, B., and Salzberg, S. (2013). Bowtie2. Nat. Methods 9, 357–359. doi: 10.1038/nmeth.1923.Fast
Lau, C. H. F., van Engelen, K., Gordon, S., Renaud, J., and Topp, E. (2017). Novel antibiotic resistance determinants from agricultural soil exposed to antibiotics widely used in human medicine and animal farming. Appl. Environ. Microbiol. 83:e00989-17. doi: 10.1128/AEM.00989-17
Leclercq, S., Mian, F. M., Stanisz, A. M., Bindels, L. B., Cambier, E., Ben-Amram, H., et al. (2017). Low-dose penicillin in early life induces long-term changes in murine gut microbiota, brain cytokines and behavior. Nat. Commun. 8:15062. doi: 10.1038/ncomms15062
Levy, S. B., McMurry, L. M., Barbosa, T. M., Burdett, V., Courvalin, P., Hillen, W., et al. (1999). Nomenclature for new tetracycline resistance determinants. Antimicrob. Agents Chemother. 43, 1523–1524. doi: 10.1128/aac.43.6.1523
Li, D., Liu, C. M., Luo, R., Sadakane, K., and Lam, T. W. (2015). MEGAHIT: an ultra-fast single-node solution for large and complex metagenomics assembly via succinct de Bruijn graph. Bioinformatics 31, 1674–1676. doi: 10.1093/bioinformatics/btv033
Li, H., and Durbin, R. (2009). Fast and accurate short read alignment with Burrows-Wheeler transform. Bioinformatics 25, 1754–1760. doi: 10.1093/bioinformatics/btp324
Lin, J., Nishino, K., Roberts, M. C., Tolmasky, M., Aminov, R. I., and Zhang, L. (2015). Mechanisms of antibiotic resistance. Front. Microbiol. 6:481–511. doi: 10.3389/fmicb.2015.00034
Linares, J. F., Gustafsson, I., Baquero, F., and Martinez, J. L. (2006). Antibiotics as intermicrobiol signaling agents instead of weapons. Proc. Natl. Acad. Sci. U.S.A. 103, 19484–19489. doi: 10.1073/pnas.0608949103
Liu, B., and Pop, M. (2009). ARDB—antibiotic resistance genes database. Nucleic Acids Res. 37, D443—D447.
Lundström, S. V., Östman, M., Bengtsson-Palme, J., Rutgersson, C., Thoudal, M., Sircar, T., et al. (2016). Minimal selective concentrations of tetracycline in complex aquatic bacterial biofilms. Sci. Total Environ. 553, 587–595. doi: 10.1016/j.scitotenv.2016.02.103
Luo, G., Li, B., Li, L. G., Zhang, T., and Angelidaki, I. (2017). Antibiotic resistance genes and correlations with microbial community and metal resistance genes in full-scale biogas reactors as revealed by metagenomic analysis. Environ. Sci. Technol. 51, 4069–4080. doi: 10.1021/acs.est.6b05100
Maamar, S., Ben, Glawe, A. J., Brown, T. K., Hellgeth, N., Hu, J., et al. (2020). Mobilizable antibiotic resistance genes are present in dust microbial communities. PLoS Pathog 16:e1008211. doi: 10.1371/journal.ppat.1008211
Macedo, D., Filho, A. J. M. C., Soares, de Sousa, C. N., Quevedo, J., Barichello, T., et al. (2017). Antidepressants, antimicrobials or both? Gut microbiota dysbiosis in depression and possible implications of the antimicrobial effects of antidepressant drugs for antidepressant effectiveness. J. Affect. Disord. 208, 22–32. doi: 10.1016/j.jad.2016.09.012
MacPherson, D. W., Gushulak, B. D., Baine, W. B., Bala, S., Gubbins, P. O., Holtom, P., et al. (2009). Population mobility, globalization, and antimicrobial drug resistance. Emerg. Infect. Dis. 15, 1727–1732. doi: 10.3201/eid1511.090419
Magesh, S., Jonsson, V., and Bengtsson-Palme, J. (2019). Mumame: a software tool for quantifying gene-specific point-mutations in shotgun metagenomic data. Metabarcoding Metagenom. 3:e36236. doi: 10.3897/mbmg.3.36236
Mahdavi, B., Paydarfard, S., Zangeneh, M. M., Goorani, S., Seydi, N., and Zangeneh, A. (2020). Assessment of antioxidant, cytotoxicity, antibacterial, antifungal, and cutaneous wound healing activities of green synthesized manganese nanoparticles using Ziziphora clinopodioides lam leaves under in vitro and in vivo condition. Appl. Organomet. Chem. 34:e5248. doi: 10.1002/aoc.5248
Maiden, M. M., Agostinho Hunt, A. M., Zachos, M. P., Gibson, J. A., Hurwitz, M. E., Mulks, M. H., et al. (2018). Triclosan is an aminoglycoside adjuvant for eradication of Pseudomonas aeruginosa biofilms. Antimicrob. Agents Chemother. 62:e00146-18. doi: 10.1128/AAC.00146-18
Maisetta, G., Grassi, L., Esin, S., Kaya, E., Morelli, A., Puppi, D., et al. (2020). Targeting pseudomonas aeruginosa in the sputum of primary ciliary dyskinesia patients with a combinatorial strategy having antibacterial and anti-virulence potential. Int. J. Mol. Sci. 21:69. doi: 10.3390/ijms21010069
Marathe, N. P., Berglund, F., Razavi, M., Dröge, J., Samant, S., et al. (2019). Sewage effluent from an Indian hospital harbors novel carbapenemases and integron-borne antibiotic resistance genes. Microbiome 7:97. doi: 10.1186/s40168-019-0710-x
Martin, M. (2011). Cutadapt removes adapter sequences from high-throughput sequencing reads. EMBnet J. 17:10. doi: 10.14806/ej.17.1.200
McDermott, P. F., Tyson, G. H., Kabera, C., Chen, Y., Li, C., Folster, J. P., et al. (2016). Whole-genome sequencing for detecting antimicrobial resistance in nontyphoidal Salmonella. Antimicrob. Agents Chemother. 60, 5515–5520. doi: 10.1128/AAC.01030-16
McMahon, M. D., Guan, C., Handelsman, J., and Thomas, M. G. (2012). Metagenomic analysis of Streptomyces lividans reveals host-dependent functional expression. Appl. Environ. Microbiol. 78, 3622–3629.
Mellmann, A., Bletz, S., Böking, T., Kipp, F., Becker, K., Schultes, A., et al. (2016). Real-time genome sequencing of resistant bacteria provides precision infection control in an institutional setting. J. Clin. Microbiol. 54, 2874–2881. doi: 10.1128/JCM.00790-16
Miyazaki, K., and Kitahara, K. (2018). Functional metagenomic approach to identify overlooked antibiotic resistance mutations in bacterial rRNA. Sci. Rep. 8:5179. doi: 10.1038/s41598-018-23474-4
Munita, J. M., and Arias, C. A. (2016). Mechanisms of antibiotic resistance. Microbiol. Spectr. 4. doi: 10.1128/microbiolspec.VMBF-0016-2015
Munk, P., Andersen, V. D., de Knegt, L., Jensen, M. S., Knudsen, B. E., Lukjancenko, O., et al. (2017). A sampling and metagenomic sequencing-based methodology for monitoring antimicrobial resistance in swine herds. J. Antimicrob. Chemother. 72, 385–392. doi: 10.1093/jac/dkw415
Nagayama, H., Sugawara, T., Endo, R., Ono, A., Kato, H., Ohtsubo, Y., et al. (2015). Isolation of oxygenase genes for indigo-forming activity from an artificially polluted soil metagenome by functional screening using Pseudomonas putida strains as hosts. Appl. Microbiol. Biotechnol. 99, 4453–4470. doi: 10.1007/s00253-014-6322-2
Nair, S., Desai, S., Poonacha, N., Vipra, A., and Sharma, U. (2016). Antibiofilm activity and synergistic inhibition of Staphylococcus aureus biofilms by bactericidal protein P128 in combination with antibiotics. Antimicrob. Agents Chemother. 60, 7280–7289. doi: 10.1128/AAC.01118-16
Ng, C., Tay, M., Tan, B., Le, T. H., Haller, L., Chen, H., et al. (2017). Characterization of metagenomes in urban aquatic compartments reveals high prevalence of clinically relevant antibiotic resistance genes in wastewaters. Front. Microbiol. 8:2200. doi: 10.3389/fmicb.2017.02200
Ngara, T. R., and Zhang, H. (2018). Recent advances in function-based metagenomic screening. Genom. Proteom. Bioinform. 16, 405–415. doi: 10.1016/j.gpb.2018.01.002
Niu, S. Y., Yang, J., McDermaid, A., Zhao, J., Kang, Y., and Ma, Q. (2017). Bioinformatics tools for quantitative and functional metagenome and metatranscriptome data analysis in microbes. Brief. Bioinform. 19, 1415–1429. doi: 10.1093/bib/bbx051
Nogacka, A., Salazar, N., Suárez, M., Milani, C., Arboleya, S., Solís, G., et al. (2017). Impact of intrapartum antimicrobial prophylaxis upon the intestinal microbiota and the prevalence of antibiotic resistance genes in vaginally delivered full-term neonates. Microbiome 5:93. doi: 10.1186/s40168-017-0313-3
Nora, L. C., Westmann, C. A., Martins-Santana, L., Alves, L., de, F., Monteiro, L. M. O., et al. (2019). The art of vector engineering: towards the construction of next-generation genetic tools. Microb. Biotechnol. 12, 125–147. doi: 10.1111/1751-7915.13318
Nurk, S., Meleshko, D., Korobeynikov, A., and Pevzner, P. A. (2017). MetaSPAdes: a new versatile metagenomic assembler. Genome Res. 27, 824–834. doi: 10.1101/gr.213959.116
Okuda, H., Ogura, K., Kato, A., Takubo, H., and Watabe, T. (1998). A possible mechanism of eighteen patient deaths caused by interactions of sorivudine, a new antiviral drug, with oral 5-fluorouracil prodrugs. J. Pharmacol. Exp. Ther. 287, 791–799.
Panunzi, L. G. (2020). sraX: a novel comprehensive resistome analysis tool. Front. Microbiol. 11:52. doi: 10.3389/fmicb.2020.00052
Partridge, S. R., and Tsafnat, G. (2018). Automated annotation of mobile antibiotic resistance in Gram-negative bacteria: the Multiple Antibiotic Resistance Annotator (MARA) and database. J. Antimicrob. Chemother. 73, 883–890. doi: 10.1093/jac/dkx513
Pehrsson, E. C., Forsberg, K. J., Gibson, M. K., Ahmadi, S., and Dantas, G. (2013). Novel resistance functions uncovered using functional metagenomic investigations of resistance reservoirs. Front. Microbiol. 4:145. doi: 10.3389/fmicb.2013.00145
Peng, Y., Leung, H. C. M., Yiu, S. M., and Chin, F. Y. L. (2012). IDBA-UD: a de novo assembler for single-cell and metagenomic sequencing data with highly uneven depth. Bioinformatics 28, 1420–1428. doi: 10.1093/bioinformatics/bts174
Pryor, R., Martinez-Martinez, D., Quintaneiro, L., and Cabreiro, F. (2020). The role of the microbiome in drug response. Annu. Rev. Pharmacol. Toxicol. 60, 417–435. doi: 10.1146/annurev-pharmtox-010919-023612
Raymond, F., Ouameur, A. A., Déraspe, M., Iqbal, N., Gingras, H., Dridi, B., et al. (2016). The initial state of the human gut microbiome determines its reshaping by antibiotics. ISME J. 10, 707–720. doi: 10.1038/ismej.2015.148
Rho, M., Tang, H., and Ye, Y. (2010). FragGeneScan: predicting genes in short and error-prone reads. Nucleic Acids Res. 38:e191. doi: 10.1093/nar/gkq747
Rivardo, F., Martinotti, M. G., Turner, R. J., and Ceri, H. (2011). Synergistic effect of lipopeptide biosurfactant with antibiotics against Escherichia coli CFT073 biofilm. Int. J. Antimicrob. Agents 37, 324–331. doi: 10.1016/j.ijantimicag.2010.12.011
Roca, I., Akova, M., Baquero, F., Carlet, J., Cavaleri, M., Coenen, S., et al. (2015). The global threat of antimicrobial resistance: science for intervention. New Microbes New Infect. 6, 22–29. doi: 10.1016/j.nmni.2015.02.007
Rognes, T., Flouri, T., Nichols, B., Quince, C., and Mahé, F. (2016). VSEARCH: a versatile open source tool for metagenomics. PeerJ 4:e2584. doi: 10.7717/peerj.2584
Rowe, W., Baker, K. S., Verner-Jeffreys, D., Baker-Austin, C., Ryan, J. J., Maskell, D., et al. (2015). Search engine for antimicrobial resistance: a cloud compatible pipeline and web interface for rapidly detecting antimicrobial resistance genes directly from sequence data. PLoS One 10:e0133492. doi: 10.1371/journal.pone.0133492
Rowe, W., and Winn, M. (2018). Indexed variation graphs for efficient and accurate resistome profiling. Bioinformatics 34, 3601–3608. doi: 10.1101/270835
Ruppé, E., Ghozlane, A., Tap, J., Pons, N., Alvarez, A. S., Maziers, N., et al. (2019). Prediction of the intestinal resistome by a three-dimensional structure-based method. Nat. Microbiol. 4, 112–123. doi: 10.1038/s41564-018-0292-6
Scaria, J., Chandramouli, U., and Verma, S. K. (2005). Antibiotic Resistance Genes Online (ARGO): a database on vancomycin and b-lactam resistance genes. Bioinformation 1, 5–7. doi: 10.6026/97320630001005
Schürch, A. C., and van Schaik, W. (2017). Challenges and opportunities for whole-genome sequencing–based surveillance of antibiotic resistance. Ann. N. Y. Acad. Sci. 1388, 108–120. doi: 10.1111/nyas.13310
Seale, A. C., Bianchi-Jassir, F., Russell, N. J., Kohli-Lynch, M., Tann, C. J., Hall, J., et al. (2017). Estimates of the burden of group B streptococcal disease worldwide for pregnant women. Stillbirths Children. Clin. Infect. Dis. 65, S200–S219. doi: 10.1093/cid/cix664
Sengupta, S., Chattopadhyay, M. K., and Grossart, H. P. (2013). The multifaceted roles of antibiotics and antibiotic resistance in nature. Front. Microbiol. 4:47. doi: 10.3389/fmicb.2013.00047
Shang, D., Liu, Y., Jiang, F., and Han, X. (2019). Synergistic antibacterial activity of Trp-containing antibacterial peptides in combination with antibiotics against multidrug-resistant Staphylococcus epidermidis. Front. Microbiol. 10:2719. doi: 10.3389/fmicb.2019.02719
Shashkova, T., Popenko, A., Tyakht, A., Peskov, K., Kosinsky, Y., Bogolubsky, L., et al. (2016). Agent based modeling of human gut microbiome interactions and perturbations. PLoS One 11:e0148386. doi: 10.1371/journal.pone.0148386
Singla, P., Dalal, P., Kaur, M., Arya, G., Nimesh, S., Singh, R., et al. (2018). Bile acid oligomers and their combination with antibiotics to combat bacterial infections. J. Med. Chem. 61, 10265–10275. doi: 10.1021/acs.jmedchem.8b01433
Sousa, T., Yadav, V., Zann, V., Borde, A., Abrahamsson, B., and Basit, A. W. (2014). On the colonic bacterial metabolism of Azo-bonded prodrugs of 5-aminosalicylic acid. J. Pharm. Sci. 103, 3171–3175. doi: 10.1002/jps.24103
Stearns, J. C., Simioni, J., Gunn, E., McDonald, H., Holloway, A. C., Thabane, L., et al. (2017). Intrapartum antibiotics for GBS prophylaxis alter colonization patterns in the early infant gut microbiome of low risk infants. Sci. Rep. 7: 16527. doi: 10.1038/s41598-017-16606-9
Steen, A. D., Crits-Christoph, A., Carini, P., DeAngelis, K. M., Fierer, N., Lloyd, K. G., et al. (2019). High proportions of bacteria and archaea across most biomes remain uncultured. ISME J. 13, 3126–3130. doi: 10.1038/s41396-019-0484-y
Su, J. Q., An, X. L., Li, B., Chen, Q. L., Gillings, M. R., Chen, H., et al. (2017). Metagenomics of urban sewage identifies an extensively shared antibiotic resistome in China. Microbiome 5:84. doi: 10.1186/s40168-017-0298-y
Su, P. A., Li, S. L., Tang, H. J., Chen, C. C., Lu, Y. C., Cheng, K. C., et al. (2020). In vitro synergy of Pongamia pinnata extract in combination with antibiotics for inhibiting and killing methicillin-resistant Staphylococcus aureus. Antibiotics 9:103. doi: 10.3390/antibiotics9030103
Subirats, J., Sànchez-Melsió, A., Borrego, C. M., Balcázar, J. L., and Simonet, P. (2016). Metagenomic analysis reveals that bacteriophages are reservoirs of antibiotic resistance genes. Int. J. Antimicrob. Agents 48, 163–167. doi: 10.1016/j.ijantimicag.2016.04.028
Suez, J., Zmora, N., Zilberman-Schapira, G., Mor, U., Dori-Bachash, M., Bashiardes, S., et al. (2018). Post-Antibiotic gut mucosal microbiome reconstitution is impaired by probiotics and improved by autologous. FMT. Cell 174, 1406–1423.e16. doi: 10.1016/j.cell.2018.08.047
Surleac, M., Barbu, I. C., Paraschiv, S., Popa, L. I., Gheorghe, I., Marutescu, L., et al. (2020). Whole genome sequencing snapshot of multidrug resistant Klebsiella pneumoniae strains from hospitals and receiving wastewater treatment plants in Southern Romania. PLoS One 15:e0228079. doi: 10.1371/journal.pone.0228079
Tamma, P. D., Cosgrove, S. E., and Maragakis, L. L. (2012). Combination therapy for treatment of infections with gram-negative bacteria. Clin. Microbiol. Rev. 25, 450–470. doi: 10.1128/CMR.05041-11
Tanzi, M. G. (2020). With drug-resistant infections increasing. CDC urges action. Pharm. Today 26:14.
Tenconi, E., Traxler, M. F., Hoebreck, C., van Wezel, G. P., and Rigali, S. (2018). Production of prodiginines is part of a programmed cell death process in Streptomyces coelicolor. Front. Microbiol. 9:1742. doi: 10.3389/fmicb.2018.01742
Townsley, L., and Shank, E. A. (2017). Natural-Product antibiotics: cues for modulating bacterial biofilm formation. Trends Microbiol. 25, 1016–1026. doi: 10.1016/j.tim.2017.06.003
Vazquez-Muñoz, R., Meza-Villezcas, A., Fournier, P. G. J., Soria-Castro, E., Juarez-Moreno, K., Gallego-Hernández, A. L., et al. (2019). Enhancement of antibiotics antimicrobial activity due to the silver nanoparticles impact on the cell membrane. PLoS One 14:e0224904. doi: 10.1371/journal.pone.0224904
Vollmers, J., Wiegand, S., and Kaster, A. K. (2017). Comparing and evaluating metagenome assembly tools from a microbiologist’s perspective - not only size matters! PLoS One 12:e0169662. doi: 10.1371/journal.pone.0169662
Wallace, J. C., Port, J. A., Smith, M. N., and Faustman, E. M. (2017). FARME DB: a functional antibiotic resistance element database. Database 2017:baw165. doi: 10.1093/database/baw165
Walsh, F. (2013). The multiple roles of antibiotics and antibiotic resistance in nature. Front. Microbiol. 4:255. doi: 10.3389/fmicb.2013.00255
Wan, G., Ruan, L., Yin, Y., Yang, T., Ge, M., and Cheng, X. (2016). Effects of silver nanoparticles in combination with antibiotics on the resistant bacteria Acinetobacter baumannii. Int. J. Nanomed. 11, 3789–3800. doi: 10.2147/IJN.S104166
Wang, C., Feng, Y., Liu, L., Wei, L., Kang, M., and Zong, Z. (2020). Identification of novel mobile colistin resistance gene mcr-10. Emerg. Microbes Infect. 9, 508–516. doi: 10.1080/22221751.2020.1732231
Wang, H., Hart, D. J., and An, Y. (2019). Functional metagenomic technologies for the discovery of novel enzymes for biomass degradation and biofuel production. BioEnergy Res. 12, 457–470.
Wang, J. H., Lu, J., Zhang, Y. X., Wu, J., Luo, Y., and Liu, H. (2018). Metagenomic analysis of antibiotic resistance genes in coastal industrial mariculture systems. Bioresour. Technol. 253, 235–243. doi: 10.1016/j.biortech.2018.01.035
Warren, R. L., Freeman, J. D., Levesque, R. C., Smailus, D. E., Flibotte, S., and Holt, R. A. (2008). Transcription of foreign DNA in Escherichia coli. Genome Res. 18, 1798–1805. doi: 10.1101/gr.080358.108
Wei, B., Shi, Z., Xiao, J., Xu, Y., and Lv, L. (2017). In vivo and In vitro antibacterial effect of nano-structured titanium coating incorporated with silver oxide nanoparticles. J. Biomater. Tissue Eng. 7, 418–425. doi: 10.1166/jbt.2017.1581
Wei, Z., Wu, Y., Feng, K., Yang, M., Zhang, Y., Tu, Q., et al. (2019). ARGA, a pipeline for primer evaluation on antibiotic resistance genes. Environ. Int. 128, 137–145. doi: 10.1016/j.envint.2019.04.030
Wellington, E. M. H., Boxall, A. B. A., Cross, P., Feil, E. J., Gaze, W. H., Hawkey, P. M., et al. (2013). The role of the natural environment in the emergence of antibiotic resistance in Gram-negative bacteria. Lancet Infect. Dis. 13, 155–165. doi: 10.1016/S1473-3099(12)70317-1
Westers, L. (2004). Bacillus subtilis as cell factory for pharmaceutical proteins: a biotechnological approach to optimize the host organism. Biochim. Biophys. Acta - Mol. Cell Res. 1694, 299–310. doi: 10.1016/s0167-4889(04)00083-7
Willms, I. M., Kamran, A., Aßmann, N. F., Krone, D., Bolz, S. H., Fiedler, F., et al. (2019). Discovery of novel antibiotic resistance determinants in forest and grassland soil metagenomes. Front. Microbiol. 10:460. doi: 10.3389/fmicb.2019.00460
Wong, V. K., Baker, S., Pickard, D. J., Parkhill, J., Page, A. J., Feasey, N. A., et al. (2015). Phylogeographical analysis of the dominant multidrug-resistant H58 clade of Salmonella Typhi identifies inter-and intracontinental transmission events. Nat. Genet. 47, 632–639. doi: 10.1038/ng.3281
Woodcroft, B. J., Boyd, J. A., and Tyson, G. W. (2016). OrfM: a fast open reading frame predictor for metagenomic data. Bioinformatics 32, 2702–2703. doi: 10.1093/bioinformatics/btw241
World Health Organization (2015). Global Antimicrobial Resistance Surveillance System. Geneva: World Health Organization.
Xia, J., Sun, H., Ma, X., Huang, K., and Ye, L. (2020). Ozone pretreatment of wastewater containing aromatics reduces antibiotic resistance genes in bioreactors: the example of p-aminophenol. Environ. Int. 142:105864. doi: 10.1016/j.envint.2020.105864
Yadav, S., and Kapley, A. (2019). Exploration of activated sludge resistome using metagenomics. Sci. Total Environ. 692, 1155–1164. doi: 10.1016/j.scitotenv.2019.07.267
Yang, C., Yang, Y., Che, Y., Xia, Y., Li, L., Xiong, W., et al. (2017). Bioprospecting for β-lactam resistance genes using a metagenomics-guided strategy. Appl. Microbiol. Biotechnol. 101, 6253–6260. doi: 10.1007/s00253-017-8343-0
Yarygin, K. S., Kovarsky, B. A., Bibikova, T. S., Melnikov, D. S., Tyakht, A. V., and Alexeev, D. G. (2017). ResistoMap—online visualization of human gut microbiota antibiotic resistome. Bioinformatics 33, 2205–2206.
Yassour, M., Vatanen, T., Siljander, H., Hämäläinen, A. M., Härkönen, T., Ryhänen, S. J., et al. (2016). Natural history of the infant gut microbiome and impact of antibiotic treatment on bacterial strain diversity and stability. Sci. Transl. Med. 8, ra81–ra343. doi: 10.1126/scitranslmed.aad0917
Yenn, T. W., Arslan Khan, M., Amiera Syuhada, N., Chean Ring, L., Ibrahim, D., and Tan, W. N. (2017). Stigmasterol: an adjuvant for beta lactam antibiotics against beta-lactamase positive clinical isolates. Steroids 128, 68–71. doi: 10.1016/j.steroids.2017.10.016
Yin, X., Jiang, X. T., Chai, B., Li, L., Yang, Y., Cole, J. R., et al. (2018). ARGs-OAP v2.0 with an expanded SARG database and Hidden Markov Models for enhancement characterization and quantification of antibiotic resistance genes in environmental metagenomes. Bioinformatics 34, 2263–2270. doi: 10.1093/bioinformatics/bty053
Yong, D., Toleman, M. A., Giske, C. G., Cho, H. S., Sundman, K., Lee, K., et al. (2009). Characterization of a new metallo-β-lactamase gene, bla NDM-1, and a novel erythromycin esterase gene carried on a unique genetic structure in Klebsiella pneumoniae sequence type 14 from India. Antimicrob. Agents Chemother. 53, 5046–5054. doi: 10.1128/AAC.00774-09
You, C., Han, C., Wang, X., Zheng, Y., Li, Q., Hu, X., et al. (2012). The progress of silver nanoparticles in the antibacterial mechanism, clinical application and cytotoxicity. Mol. Biol. Rep. 39, 9193–9201. doi: 10.1007/s11033-012-1792-8
Zankari, E., Allesøe, R., Joensen, K. G., Cavaco, L. M., Lund, O., and Aarestrup, F. M. (2017). PointFinder: a novel web tool for WGS-based detection of antimicrobial resistance associated with chromosomal point mutations in bacterial pathogens. J. Antimicrob. Chemother. 72, 2764–2768. doi: 10.1093/jac/dkx217
Zankari, E., Hasman, H., Cosentino, S., Vestergaard, M., Rasmussen, S., Lund, O., et al. (2012). Identification of acquired antimicrobial resistance genes. J. Antimicrob. Chemother. 67, 2640–2644. doi: 10.1093/jac/dks261
Zarrinpar, A., Chaix, A., Xu, Z. Z., Chang, M. W., Marotz, C. A., Saghatelian, A., et al. (2018). Antibiotic-induced microbiome depletion alters metabolic homeostasis by affecting gut signaling and colonic metabolism. Nat. Commun. 9:2872. doi: 10.1038/s41467-018-05336-9
Zeng, J., Pan, Y., Yang, J., Hou, M., Zeng, Z., and Xiong, W. (2019). Metagenomic insights into the distribution of antibiotic resistome between the gut-associated environments and the pristine environments. Environ. Int. 126, 346–354. doi: 10.1016/j.envint.2019.02.052
Zerbino, D. R., and Birney, E. (2008). Velvet: algorithms for de novo short read assembly using de Bruijn graphs. Genome Res. 18, 821–829. doi: 10.1101/gr.074492.107
Zhang, A. N., Hou, C. J., Negi, M., Li, L. G., and Zhang, T. (2020). Online searching platform for the antibiotic resistome in bacterial tree of life and global habitats. FEMS Microbiol. Ecol. 96:fiaa107. doi: 10.1093/femsec/fiaa107
Zhang, L., Calvo-Bado, L., Murray, A. K., Amos, G. C. A., Hawkey, P. M., Wellington, E. M., et al. (2019). Novel clinically relevant antibiotic resistance genes associated with sewage sludge and industrial waste streams revealed by functional metagenomic screening. Environ. Int. 132:105120. doi: 10.1016/j.envint.2019.105120
Zhao, S., Tyson, G. H., Chen, Y., Li, C., Mukherjee, S., Young, S., et al. (2016). Whole-genome sequencing analysis accurately predicts antimicrobial resistance phenotypes in Campylobacter spp. Appl. Environ. Microbiol. 82, 459–466. doi: 10.1128/AEM.02873-15
Zhou, C. E., Smith, J., Lam, M., Zemla, A., Dyer, M. D., and Slezak, T. (2007). MvirDB - A microbial database of protein toxins, virulence factors and antibiotic resistance genes for bio-defence applications. Nucleic Acids Res. 35, D391–D394. doi: 10.1093/nar/gkl791
Zimmermann, M., Zimmermann-Kogadeeva, M., Wegmann, R., and Goodman, A. L. (2019). Mapping human microbiome drug metabolism by gut bacteria and their genes. Nature 570, 462–467. doi: 10.1038/s41586-019-1291-3
Keywords: antibiotic resistance, multidrug resistance, whole genome sequence, metagenomics, next generation sequencing, nanoparticles
Citation: Imchen M, Moopantakath J, Kumavath R, Barh D, Tiwari S, Ghosh P and Azevedo V (2020) Current Trends in Experimental and Computational Approaches to Combat Antimicrobial Resistance. Front. Genet. 11:563975. doi: 10.3389/fgene.2020.563975
Received: 20 May 2020; Accepted: 01 September 2020;
Published: 06 November 2020.
Edited by:
Mehdi Pirooznia, National Heart, Lung, and Blood Institute (NHLBI), United StatesReviewed by:
Lenwood Scott Heath, Virginia Tech, United StatesPatrick Munk, Technical University of Denmark, Denmark
Andrew C. Pawlowski, Harvard Medical School, United States
Copyright © 2020 Imchen, Moopantakath, Kumavath, Barh, Tiwari, Ghosh and Azevedo. This is an open-access article distributed under the terms of the Creative Commons Attribution License (CC BY). The use, distribution or reproduction in other forums is permitted, provided the original author(s) and the copyright owner(s) are credited and that the original publication in this journal is cited, in accordance with accepted academic practice. No use, distribution or reproduction is permitted which does not comply with these terms.
*Correspondence: Ranjith Kumavath, RNKumavath@gmail.com; RNKumavath@cukerala.ac.in