- 1Theodosius Dobzhansky Center for Genome Bioinformatics, Saint Petersburg State University, Saint Petersburg, Russia
- 2Tomsk National Research Medical Center, Mental Health Research Institute, Russian Academy of Sciences, Tomsk, Russia
- 3Institute of Translational Biomedicine, Saint Petersburg State University, Saint Petersburg, Russia
- 4National Research Tomsk State University, Tomsk, Russia
- 5Siberian State Medical University, Tomsk, Russia
- 6National Research Tomsk Polytechnic University, Tomsk, Russia
GSK3B, BDNF, NGF, NRG1, HTR2C, and PIP4K2A play important roles in molecular mechanisms of psychiatric disorders. GSK3B occupies a central position in these molecular mechanisms and is also modulated by psychotropic drugs. BDNF regulates a number of key aspects in neurodevelopment and synaptic plasticity. NGF exerts a trophic action and is implicated in cerebral alterations associated with psychiatric disorders. NRG1 is active in neural development, synaptic plasticity, and neurotransmission. HTR2C is another important psychopharmacological target. PIP4K2A catalyzes the phosphorylation of PI5P to form PIP2, the latter being implicated in various aspects of neuronal signal transduction. In the present study, the six genes were sequenced in a cohort of 19 patients with bipolar affective disorder, 41 patients with recurrent depressive disorder, and 55 patients with depressive episode. The study revealed a number of genetic variants associated with antidepressant treatment response, time to recurrence of episodes, and depression severity. Namely, alleles of rs35641374 and rs10508649 (NRG1 and PIP4K2A) may be prognostic biomarkers of time to recurrence of depressive and manic/mixed episodes among patients with bipolar affective disorder. Alleles of NC_000008.11:g.32614509_32614510del, rs61731109, and rs10508649 (also NRG1 and PIP4K2A) seem to be predictive biomarkers of response to pharmacological antidepressant treatment on the 28th day assessed by the HDRS-17 or CGI-I scale. In particular, the allele G of rs10508649 (PIP4K2A) may increase resistance to antidepressant treatment and be at the same time protective against recurrent manic/mixed episodes. These results support previous data indicating a biological link between resistance to antidepressant treatment and mania. Bioinformatic functional annotation of associated variants revealed possible impact for transcriptional regulation of PIP4K2A. In addition, the allele A of rs2248440 (HTR2C) may be a prognostic biomarker of depression severity. This allele decreases expression of the neighboring immune system gene IL13RA2 in the putamen according to the GTEx portal. The variant rs2248440 is near rs6318 (previously associated with depression and effects of psychotropic drugs) that is an eQTL for the same gene and tissue. Finally, the study points to several protein interactions relevant in the pathogenesis of mood disorders. Functional studies using cellular or animal models are warranted to support these results.
Introduction
GSK3B (Beaulieu, 2012), BDNF (Lu et al., 2014), NGF (Cirulli and Alleva, 2009), NRG1 (Mei and Nave, 2014), HTR2C (Chagraoui et al., 2016; Palacios et al., 2017), and PIP4K2A (Wilcox and Hinchliffe, 2008) are genes that showed some importance in the study of the molecular etiology of psychiatric disorders (Table 1). Specifically, GSK3B that codes for glycogen synthase kinase 3β plays a central role in the pathogenesis of psychiatric disorders (Beaulieu, 2012; Emamian, 2012). This gene is directly or indirectly inhibited by antidepressants, lithium, and antipsychotics (Beaulieu et al., 2009; Freyberg et al., 2010). It is furthermore associated with response to antidepressant medication in patients with depressive disorders (Tsai et al., 2008; Levchenko et al., 2018) and lithium treatment in patients with bipolar disorder (Benedetti et al., 2005; Lin et al., 2013; Iwahashi et al., 2014; Benedetti et al., 2015).
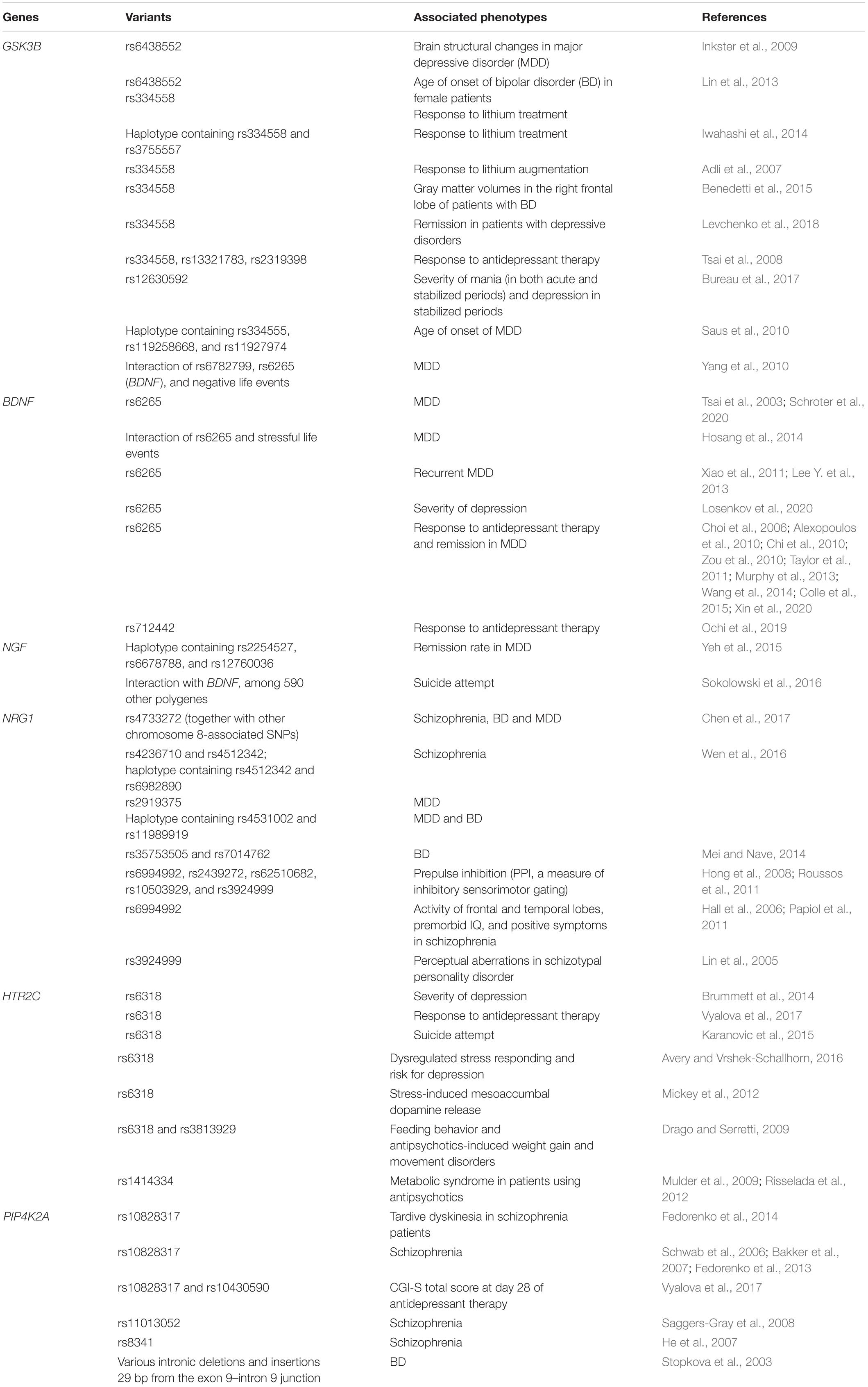
Table 1. Previous reports of association between the six sequenced genes and psychiatric phenotypes, including response to mediation.
BDNF codes for Brain-Derived Neurotrophic Factor, active in neurodevelopment and synaptic plasticity (Begni et al., 2017; Numakawa et al., 2018); the gene has been extensively studied in the context of psychiatric disorders (Numakawa et al., 2018). In patients with depression, BDNF levels are decreased and methylation levels in the gene’s promoter are increased, indicating attenuated gene expression (Kishi et al., 2017; Schroter et al., 2019). Variants in this gene are associated with severity of depression in drug-naive depressed patients (Losenkov et al., 2020) and with drug response in patients who were taking antidepressant medication for the first time (Ochi et al., 2019).
NGF codes for nerve growth factor that exerts a trophic action on the cholinergic neurons of the basal forebrain nuclei and is implicated in cerebral alterations associated with psychiatric disorders (Bersani et al., 2000). Levels of this neurotrophin are decreased in bipolar disorder (Barbosa et al., 2011) and schizophrenia (Qin et al., 2017).
NRG1, coding for neuregulin, is implicated in neural development, including myelination, synaptic plasticity, and neurotransmission (Mei and Nave, 2014). It is associated with schizophrenia, bipolar disorder, and depression (Mei and Nave, 2014; Dang et al., 2016; Wen et al., 2016; Chen et al., 2017).
HTR2C codes for the serotonin 2C receptor that is an extremely important target of drugs used to treat a number of psychiatric disorders (Di Giovanni and De Deurwaerdere, 2016;
Palacios et al., 2017), including depression (Chagraoui et al., 2016). In addition, this gene is associated with severity of depression and response to pharmacological antidepressant treatment (Brummett et al., 2014; Vyalova et al., 2017).
PIP4K2A codes for phosphatidylinositol-5-phosphate 4-kinase type 2α, a kinase that catalyzes the phosphorylation of phosphatidylinositol-5-phosphate (PI5P) to form phosphatidylinositol-5,4-bisphosphate (PIP2) (Wilcox and Hinchliffe, 2008). The lipid PIP2 is implicated in various aspects of neuronal signal transduction (Di Paolo et al., 2004; Fedorenko et al., 2008; Fedorenko et al., 2009; Seebohm et al., 2014). Genetic studies of PIP4K2A showed an association with bipolar disorder and schizophrenia (Stopkova et al., 2003; Schwab et al., 2006), as well as with response to pharmacological antidepressant treatment (Vyalova et al., 2017). In addition, the mechanisms underlying lithium’s therapeutic efficacy in the chronic treatment of bipolar disorder include differential expression of PIP4K2A (Seelan et al., 2008).
GSK3B, BDNF, NGF, NRG1, HTR2C, and PIP4K2A are functionally connected. GSK3B plays a central role in the AKT/GSK3 molecular pathway (Beaulieu, 2012). The binding of either BDNF, NGF, or NRG1 to their respective receptor tyrosine kinases (TrkB, encoded by NTRK2, TrkA, encoded by NTRK1, and ERBB4, encoded by the gene with the same name) leads to inhibition of GSK3B via activation of phosphoinositide 3-kinase (PI3K), 3-phosphoinositide-dependent protein kinase-1 (PDPK1), and AKT1 (Beaulieu, 2012; Kim et al., 2014; Mei and Nave, 2014; Numakawa et al., 2018). PI3K is a heterodimer made of a catalytic p110 subunit and an adapter regulatory p85 subunit; in the brain, the former is encoded by PIK3CA and PIK3CB, while the latter is encoded by PIK3R1 and PIK3R2 (Dwivedi et al., 2008). Binding of serotonin to HTR2C leads to the opposite effect: GSK3B activation (Beaulieu, 2012). A link between PIP4K2A and GSK3B involves activation of M-channels by PIP2 and GSK3B (Figure 1; Delmas and Brown, 2005; Carter, 2007; Fedorenko et al., 2008; Wildburger and Laezza, 2012; Jiang et al., 2015). These channels, formed in the brain by tetramers of subunits potassium voltage-gated channel subfamily Q members 2, 3, and 5 (KCNQ2, KCNQ3, and KCNQ5), play a critical role in the regulation of neuronal excitability (Greene and Hoshi, 2017) and are potential treatment targets for manic symptoms (Grunnet et al., 2014).
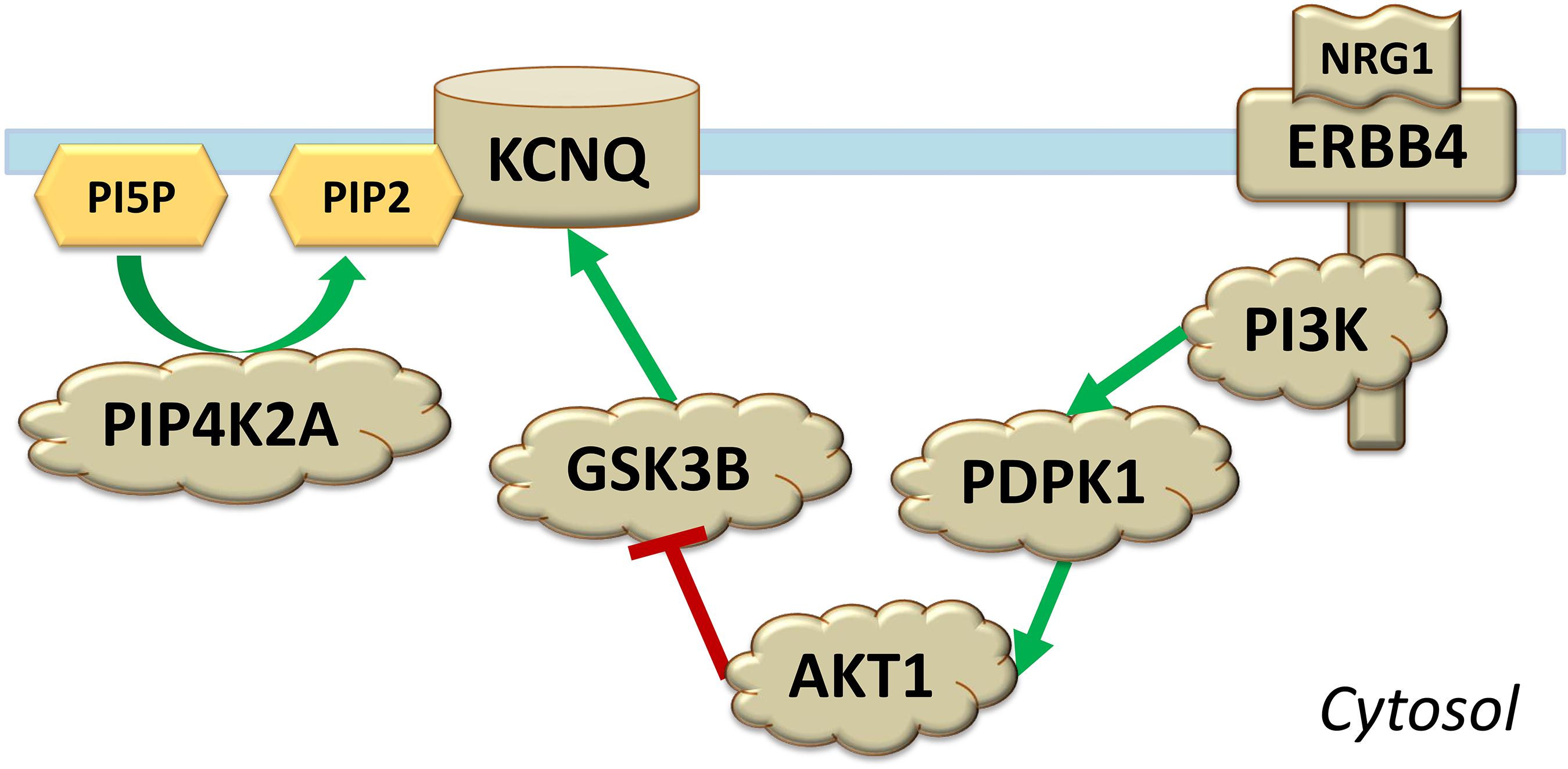
Figure 1. Shown are molecular interactions involving protein products of PIP4K2A and NRG1 associated with time to recurrence of an episode in bipolar disorder and antidepressant treatment response. Phospholipids phosphatidylinositol-5-phosphate (PI5P) and phosphatidylinositol-5,4-bisphosphate (PIP2) are located on the intracellular leaflet of the plasma membrane, while the channel KCNQ and the receptor ERBB4 are transmembrane. The binding of NRG1 to the receptor tyrosine kinase ERBB4 results in subsequent activation of phosphoinositide 3-kinase (PI3K), 3-phosphoinositide-dependent protein kinase-1 (PDPK1), and AKT1; the latter kinase inhibits GSK3B.
In the present study, we sequenced exons and flanking intronic regions of GSK3B, BDNF, NGF, NRG1, HTR2C, and PIP4K2A, using the ion semiconductor next-generation sequencing technology. A statistical evaluation revealed that several alleles of NRG1, HTR2C, and PIP4K2A are associated with a number of clinical subphenotypes of depression and bipolar disorder, namely, time to recurrence of a depressive episode and of manic or mixed episode, response to pharmacological antidepressant treatment on the 28th day assessed by the Hamilton Depression Rating Scale—17 items (HDRS-17) or the Clinical Global Impression – Improvement (CGI-I) scale, and severity of depression. A bioinformatic assessment of the functional impact of these alleles suggests possible molecular mechanisms in the etiology of these clinical subphenotypes.
Materials and Methods
Study Subjects
The genetic study was carried out in accordance with The Code of Ethics of the World Medical Association (Declaration of Helsinki 1975, revised in Fortaleza, Brazil, 2013) for experiments involving humans. After approval of the study protocol by the Local Bioethics Committee of the Mental Health Research Institute in Tomsk, Russia, 115 patients were recruited from an in-patient facility of the same institute. As a negative control, 34 individuals without psychiatric disorders were also recruited into the study. Only subjects with European ancestry were considered. All participants gave written informed consent after a proper explanation of the prospective study.
In particular, we included the following numbers of patients with mood disorders, diagnosed using the criteria of the International Statistical Classification of Diseases and Related Health Problems 10th Revision (ICD-10): 19 patients with bipolar affective disorder (ICD-10: F31), 55 patients with depressive episode (ICD-10: F32), and 41 patients with recurrent depressive disorder (ICD-10: F33). A summary of clinical features for this cohort is shown in Table 2. Bipolar I and II disorders among patients with bipolar affective disorder were established using the Diagnostic and Statistical Manual of Mental Disorders, 4th edition, criteria. Severity of illness was assessed using the Clinical Global Impression—Severity scale (CGI-S) (Guy, 1976). Manic and mixed episodes were considered in the association study under a single category, because some patients only had depressive and mixed episodes. The 115 patients taken together constituted the cross-disorder group.
The duration of antidepressant treatment was not less than 28 days. During their follow-up in the clinic, patients were given several different groups of antidepressants, including tricyclic antidepressants, selective serotonin reuptake inhibitors, serotonin–norepinephrine reuptake inhibitors, noradrenergic and specific serotonergic antidepressants, and agomelatine (an agonist at melatonin receptors and an antagonist at serotonin 2C receptors). All antidepressants were used in recommended average therapeutic doses. For definition of response and remission, the HDRS-17 (Hamilton, 1960) and CGI scale (Guy, 1976) was used. Evaluation was made on the 28th day of treatment. Responders were identified if the HDRS-17 scores were reduced by 50% or if the CGI-I scores were ≤ 2. Remitters were identified if the HDRS-17 scores ≤ 7 or if the CGI-S scores were ≤ 2.
Targeted DNA Sequencing
Evacuated blood collection tubes “Vacutainer” (Becton Dickinson, Franklin Lakes, NJ, United States) with EDTA as the anticoagulant were used. Extraction of DNA from whole venous blood was performed using the phenol–chloroform method. Concentration and purity of DNA were measured using the NanoDrop 8000 UV-Vis spectrophotometer (Thermo Fisher Scientific, Waltham, MA, United States).
Targeted next-generation sequencing was performed using the Ion Torrent semiconductor technology (Thermo Fisher Scientific, Waltham, MA, United States) at the Center “Medical Genomics” of the Tomsk National Research Medical Center. A custom DNA panel of 86 amplicons, covering 57 targets, was designed using the Ion AmpliSeq Designer1. The targets comprised exons and flanking intronic regions of at least 50 base pairs (bp) of GSK3B, BDNF, NRG1, NGF, HTR2C, and PIP4K2A in the human genome assembly GRCh37/hg19. The Ion AmpliSeq DNA Library kit 2.0 and the Ion Xpress Barcode Adapters 1–16 and 17–32 kits were used to prepare amplicon libraries based on the custom Ion AmpliSeq panels. Library normalization was performed using the Ion Library Equalizer kit. The Ion PGM Template OT2 200 Kit was used to prepare the template on the Ion OneTouch 2 System. Sequencing was done with the mean coverage of 473 × on the Ion Torrent Personal Genome Machine (PGM) System, using the Ion PGM Sequencing 200 kit v2 and Ion 316 Chips v2.
Sequencing Analysis Workflow
The unmapped BAM files were converted to the FASTQ format using Picard tools2. Quality control (QC) of raw sequencing data and trimming of low-quality bases and adapters was done using FastQC3 and Trimmomatic (Bolger et al., 2014), respectively. The following Trimmomatic options were used: SLIDINGWINDOW: 4:20; LEADING: 15; TRAILING: 15; MINLEN: 36. QC of trimmed reads was also done using FastQC. Reads were aligned to the human genome assembly GRCh38.p12/hg38 using the default options of BWA-MEM4. SAMtools (Li et al., 2009) flagstat indicated that 99.84% of reads were aligned. SAMtools view with options -F 4 and –Sb, SAMtools sort, and SAMtools index were run to obtain indexed BAM files.
Discovery of single-nucleotide variants (SNV) and short indels was done using GATK (version 4.1.2.0) (McKenna et al., 2010)5. Genomic coordinates were lifted from the hg19 to hg38 assembly using the Lift Genome Annotations tool6. GATK HaplotypeCaller option stand-call-conf 20 was used. Variants were annotated with rsIDs listed in the Database of Single Nucleotide Polymorphisms build 153 (dbSNP)7, the Exome Aggregation Consortium (ExAC)8, and the Genome Aggregation Database (gnomAD)9.
In order to assure the extraction of reliable sequencing results, we used hard filtering parameters (DePristo et al., 2011; Van der Auwera et al., 2013)10. First, the following GATK VariantsFiltration cutoff was applied: QUAL < 100.0. Variants, which were mostly indels, called with this filter in ten or more samples, but not listed in the three databases, were considered ion semiconductor technology artifacts (Laehnemann et al., 2016) and removed. Next, the following GATK VariantsFiltration cutoffs were applied to the remaining variants: QD < 2.0, FS > 200.0, MQ < 40.0, MQRankSum < -12.5, ReadPosRankSum < -8. Males were considered as homozygotes for variants on chromosome X. PLINK 2.011 was used to make the output files .bed, .bim, and .fam for the upcoming association study.
Functional Annotation of Variants
Functional annotation of all discovered variants was done with ANNOVAR (Wang et al., 2010) that estimates the degree of deleteriousness of coding variants on protein function using 21 different prediction algorithms/conservation scores, SnpEff (Cingolani et al., 2012) (version 4.3) that was used to predict deleterious effects of both coding and non-coding variants (including effects of intronic variants on consensus sequences for splicing), HumanSplicingFinder (Desmet et al., 2009) that was used to predict impact of exonic variants on splicing, the GeneCards database (Stelzer et al., 2016) that lists GeneHancer (Fishilevich et al., 2017) regulatory elements for genes (for the purposes of this study, we considered only Elite GeneHancer elements), and the Genotype-Tissue Expression (GTEx) portal12 that lists expression quantitative trait loci (eQTLs) in various tissues. The GTEx Project was supported by the Common Fund of the Office of the Director of the National Institutes of Health, and by NCI, NHGRI, NHLBI, NIDA, NIMH, and NINDS. The data used for the analyses described in this manuscript were obtained from the GTEx Portal on 10/16/2019.
Association Study
Tests under a number of statistical models were run to evaluate the association between SNVs or indels, discovered during sequencing, and clinical subphenotypes. For analysis of quantitative data (time to recurrence of an episode, age of onset) linear regression was used, whereas for binary data (response to treatment, remission following treatment) the statistical model was binomial logistic regression. These two types of tests were carried out using a series of applications that run in the R environment: snpStats (Sole et al., 2006), SNPRelate13, dplyr14, GenABEL15, ggplot216, manhattanly17, GWASTools18, and GENESIS19. Multiple comparisons were dealt with using the Bonferroni correction and by controlling the false discovery rate (FDR). The type I error rate in this case was set to 5% (i.e., Bonferroni- and/or FDR-corrected p-values were considered significant when ≤ 0.05).
To evaluate the association between genetic variants and multinomial data (three disorders vs. controls, bipolar I and II disorders vs. controls, syndromes among patients with depression), multinomial logistic regression was deployed, while for ordinal data (severity of disease) the model was ordinal logistic regression. These last two types of statistical tests were carried out using Trinculo, a program run in the C++ environment that evaluates likelihood ratios within Bayesian and frequentist frameworks (Jostins and McVean, 2016). The type I error rate was set to 1% (i.e., likelihood ratio p-values were considered significant when ≤ 0.01).
Results
Sequencing Analysis
Sequencing analysis that included the indicated parameters of hard filtering resulted in 149 variant call format (VCF) files with 49 different variants, of which eight are novel, i.e., not listed in any of the three databases – dbSNP, ExAC, or gnomAD. Among the novel variants, four are 2–81 bp deletions, one is a 1 bp duplication, and three are 1 bp substitutions (Table 3). The novel variants (all in a heterozygous state, except for the 81 bp deletion present on the X chromosome in a male) were each present in one to three patients with depression, indicating minor allele frequency from 0.7 to 2% in the population under study.
Predicted Biological Impact of Discovered Variants
The results of functional annotation with SnpEff did not indicate high impact of either variant. Likewise, neither variant according to ANNOVAR is considered damaging/deleterious by all 21 prediction algorithms/conservation scores. HumanSplicingFinder indicated a number of new or disrupted exonic splicing enhancers (ESE) and exonic splicing silencers (ESS) (we show results only for novel and associated variants in section “Further Analysis of Biological Function of Associated and Novel Variants”). According to the list of Elite GeneHancer regulatory elements, both rs66866077 and rs79679324 (polymorphic in the cohort under study, but not associated with either clinical subphenotype) are found within the promoter GH11J027696 for BDNF. GeneHancer results for the variant rs10508649, associated with clinical subphenotypes, are described in section “Variants in PIP4K2A.” The GTEx database indicated that rs1053454 and rs2230469 (polymorphic in the cohort under study, but not associated with either clinical subphenotype) are expression quantitative trait loci (eQTLs) for PIP4K2A in various parts of the brain cortex and a number of subcortical nuclei. GTEx results for rs2248440 and rs6318 are described in section “Variant in HTR2C.”
Several Alleles Are Associated With Clinical Subphenotypes
Statistical tests indicated eight associations between alleles and clinical subphenotypes (Table 4). Two variants in NRG1, NC_000008.11:g.32614509_32614510del, and rs35641374, are associated with a number of subphenotypes related to depressive symptoms. Namely, the allele “del” of NC_000008.11:g.32614509_32614510del is associated with absence of response to antidepressant medication on the 28th day assessed by the HDRS-17 among patients with depression. Longer intervals between depressive episodes in the bipolar and cross-disorder groups are associated with the allele C of rs35641374. The same is for longer intervals between episodes of any type (i.e., depressive, manic or mixed) in the bipolar disorder group.
The allele A (in reverse complement) of rs61731109, found in PIP4K2A, is associated with absence of response to antidepressant medication on the 28th day assessed by HDRS-17 among patients with depression. This association passed FDR, but not Bonferroni correction for multiple comparisons. The allele G (in reverse complement) of rs10508649, located in the same gene, is associated with absence of response to antidepressant medication on the 28th day assessed by the CGI-I scale among patients with depression and with longer intervals between manic or mixed episodes among patients with bipolar disorder.
There was no association with response to antidepressant medication for different groups of antidepressants.
Finally, the allele A of rs2248440 (it is a reference allele that is in fact minor, ranging in frequency from 0 to 25% in all eight mentioned populations in dbSNP build 153), located in HTR2C, is associated with higher severity of depression among patients with depression.
Further Analysis of Biological Function of Associated and Novel Variants
Functional Annotation of Associated Variants
Variants in NRG1
The variant NC_000008.11:g.32614509_32614510del, associated with response to antidepressant therapy assessed by the HDRS-17 is intronic, with no obvious biological function that could be predicted with bioinformatic tools used (Table 4). Although rs35641374, associated with time to recurrence of depression among bipolar and cross-disorder patients and with time to recurrence of episodes of any type among bipolar disorder patients, is a missense variant, it does not seem to affect protein function (both amino acids in the Val > Leu substitution have uncharged hydrophobic side chain).
Variants in PIP4K2A
The allele A (in reverse complement) of the synonymous variant rs61731109, associated with response to antidepressant therapy assessed by the HDRS-17, may affect splicing of introns flanking the constitutive exon 8 (ENST00000376573.9 and ENST00000545335.5) or 6 (ENST00000323883.11) of the PIP4K2A’s transcripts, by creating an exonic splicing silencer, as predicted by HumanSplicingFinder. The allele G (in reverse compliment) of another synonymous variant rs10508649, associated with response to antidepressant therapy assessed by the CGI-I scale, and with time to recurrence of a manic or mixed episode, may also affect splicing of introns flanking the constitutive exon 5 (ENST00000376573.9 and ENST00000545335.5) or 2 (ENST00000323883.11) of the PIP4K2A’s transcripts, by erasing an exonic splicing enhancer motif, as predicted by HumanSplicingFinder. Furthermore, this variant is found within the GeneHancer’s elite enhancer GH10J022572 that regulates the expression of PIP4K2A.
Variant in HTR2C
The allele A of the intronic variant rs2248440, associated with higher severity of depression, decreases the expression of the neighboring gene IL13RA2 in the putamen, according to GTEx. The variant rs6318 also regulates the expression level of IL13RA2 in the putamen; it is only polymorphic in the cohort under study but was previously associated with a number of phenotypes relevant to the pathogenesis of depression and to effects of psychotropic drugs (Drago and Serretti, 2009; Mickey et al., 2012; Brummett et al., 2014; Karanovic et al., 2015; Avery and Vrshek-Schallhorn, 2016; Chagraoui et al., 2016; Vyalova et al., 2017). The two variants are located 4325 bp apart and are in linkage disequilibrium (LD), according to LDlink20. IL13RA2 is expressed in the brain, codes for the interleukin 13 receptor α2 subunit, and is found almost 94 kilobases (kb) downstream from HTR2C.
Functional Annotation of Novel Variants
The intronic variants NC_000003.12:g.119947358A > G, NC_000008.11:g.32614509_32614510del (associated with absence of response to antidepressant medication), NC_ 000008.11:g.32756363dup, NC_000008.11:g.32763214T > G, NC_000010.11:g.22539905_22539911del, and NC_000010.11:g. 22539924_22539937del, found in genes GSK3B, NRG1, and PIP4K2A, bear no obvious biological function as reported by the bioinformatic tools used.
The missense variant NC_000011.10:g.27658302T > C in BDNF creates the substitution Asp > Gly, i.e., a change from an acidic to uncharged hydrophobic amino acid. This change is deemed deleterious/damaging for the protein function by PROVEAN, PolyPhen-2, LRT, MutationTaster, and FATHMM-MKL algorithms run in ANNOVAR. The implicated allele G (in reverse compliment) may also affect splicing of the last intron of all BDNF transcripts, by erasing an exonic splicing enhancer motif, as predicted by HumanSplicingFinder. This variant is present in a heterozygous state in one patient with depressive episode of moderate severity and is not associated with clinical subphenotypes of mood disorders.
Finally, the 81 bp deletion in the last exon of all HTR2C transcripts, NC_000023.11:g.114906768_114906848del, creates an in-frame deletion of 27 amino acids (ENST00000276198.5 and ENST00000371951.5) or frameshift deletion of 27 amino acids, resulting in 1 aa inserted (ENST00000371950.3). The variant is deleterious for ENST00000276198.5 and ENST00000371951.5 according to PROVEAN (Choi and Chan, 2015). Moreover, it may affect splicing of the last intron of all HTR2C transcripts, by erasing multiple exonic splicing enhancer motifs, as deemed by HumanSplicingFinder. This deletion was present on the X chromosome in one male patient with recurrent depressive disorder of moderate severity. No association with clinical subphenotypes was established for that variant.
Discussion
Possible Genetic Biomarkers of Clinical Subphenotypes of Depression and Bipolar Disorder
Prognostic Biomarkers of Time to Recurrence of Mania and Depression in Bipolar Disorder
Time to recurrence of a depressive episode and time to recurrence of a manic or mixed episode among patients with bipolar disorder may be indicated by alleles of two different variants: rs35641374 in NRG1 and rs10508649 in PIP4K2A, respectively (Table 4). The association between rs35641374 and time to recurrence of an episode of any type in the bipolar disorder group seems to be driven by the association between this genetic marker and time to recurrence of depression (the p-value increases from 4.37 × 10–7 for the latter association to 3.43 × 10–4). Likewise, the association signal at the same marker in the cross-disorder group may in fact be driven by the same association in the bipolar disorder group (the p-value increases from 4.37 × 10–7 for the latter association to 3.14 × 10–6). In other words, rs35641374 may be only associated with time to recurrence of a depressive episode among patients with bipolar disorder.
These results indicate that the allele C of rs35641374 located in NRG1 and the allele G (reverse compliment) of rs10508649 located in PIP4K2A may be protective against recurrent depression and recurrent manic or mixed episodes, respectively. Although rs35641374 seems to be benign to the biological function of mRNA and protein, rs10508649 is found within the GeneHancer’s elite enhancer GH10J022572 that regulates the expression of PIP4K2A. Furthermore, the allele G of rs10508649 may affect mRNA splicing, which indicates that this variant may be functional for the PIP4K2A gene expression on two levels.
This conclusion suggests that PIP4K2A plays a role in the pathogenesis of manic or mixed symptoms. Previous data indicate that this gene is associated with bipolar disorder and schizophrenia (Stopkova et al., 2003; Schwab et al., 2006), both disorders sharing a number of clinical and molecular features (International Schizophrenia Consortium et al., 2009; Bipolar Disorder and Schizophrenia Working Group of the Psychiatric Genomics Consortium, 2018).
Implication of NRG1 in the pathogenesis of depressive symptoms among patients with bipolar disorder is also supported by previous studies, linking this gene to bipolar disorder and depression (Mei and Nave, 2014; Dang et al., 2016; Wen et al., 2016; Chen et al., 2017).
Predictive Biomarkers of Response to Antidepressant Medication
Absence of response on the 28th day assessed by the HDRS-17 or CGI-I scale among patients with depression might be predicted by alleles of the variant NC_000008.11:g.32614509_32614510del in NRG1 and of the two variants in PIP4K2A: rs61731109 and rs10508649. The indicated novel intronic variant should perhaps be listed under the dbSNP entry rs750640301 that differs from it only by the number of deleted nucleotides (one T instead of two) at the same genomic location. Although NC_000008.11:g.32614509_32614510del bears no obvious biological function, both rs61731109 and rs10508649 seem to affect splicing and, in case of rs10508649, transcriptional regulation of PIP4K2A.
Cross-Disorder Aspects of Mania and Antidepressant Therapeutic Response
It is worthy of note that the alleles of NRG1 and PIP4K2A may be simultaneous predictors of time to recurrence of manic and depressive episodes among patients with bipolar disorder and of absence of drug treatment response among patients with depression (Table 4). In particular, the allele G (reverse compliment) of rs10508649 in PIP4K2A may increase resistance to antidepressant treatment and be at the same time protective against recurrent manic or mixed episodes. These data suggest interesting avenues for the study of the pathogenesis of mania and its possible connections with the pathogenesis of treatment-resistant depression (McIntyre et al., 2014). It is known that antidepressant drugs are not suitable in treatment of bipolar disorder, because they do not provide the desired response; moreover, evidence from clinical practice suggests that antidepressants may precipitate manic symptoms (Hirschfeld, 2014). It has even been suggested that switching to mania is a consequence of increased antidepressant efficacy (Lee H. J. et al., 2013), which would support the results implicating rs10508649. Both treatment-resistant depression and mania were also suggested to have one common underlying biological mechanism: circadian rhythms (Lee H. J. et al., 2013). Although NRG1 and PIP4K2A are not regarded as biological clock genes, they both take part in signaling networks where GSK3B (Carter, 2007; Beaulieu, 2012), a gene implicated in circadian rhythms (Harada et al., 2005), plays a prominent role. An additional clue in this puzzle is that lithium, which acts upon this pathway and inhibits GSK3B (Freland and Beaulieu, 2012), modulates circadian rhythms in patients with bipolar disorder (Lee H. J. et al., 2013). Further research is necessary to continue uncovering the complex interplay between molecular networks involving GSK3B, circadian rhythms, manic symptoms, and antidepressant resistance.
Prognostic Biomarker of Depression Severity
Depression severity in patients with a diagnosis of depression may be determined by alleles of the variant rs2248440 found in the intron following the first coding exon of HTR2C. This variant is in LD with the missense HTR2C variant rs6318, previously associated with mood disorders and response to antidepressant medication (Chagraoui et al., 2016; Vyalova et al., 2017). An interesting finding is that both variants are eQTLs in the putamen for the gene of a subunit of the interleukin 13 (IL-13) receptor (IL13RA2). This might indicate either that the serotonin 2C receptor is not a genetic factor influencing on severity of depressive symptoms or that both receptors act in concert in influencing on this clinical subphenotype. Knowing that the involvement of the serotonin 2C receptor in the pathogenesis of depression is supported by a considerable amount of scientific data (Chagraoui et al., 2016; Palacios et al., 2017) and that the pathogenesis of depression is associated with the immune system abnormalities (Felger and Lotrich, 2013), the latter scenario seems to be more plausible. Although IL13RA2 is known to be implicated mainly in cancer (Sengupta et al., 2014), its ligand IL-13 regulates inflammation (Mao et al., 2019), so this gene could also play a part in increased inflammation seen in patients with depression. More research is needed to clarify these data.
Estimation of Sample Size
Estimation of sample size is possible, but not trivial, because it necessitates prior knowledge of relative risks and frequencies of genotypes associated with the disease. These parameters are unavailable in the case of genetic variants that were not studied previously. It is also worthy of note that application of algorithms that can calculate sample size for genetic association studies is limited in the case of complex study designs. For example, Genetic Power Calculator21 can be used only for discrete traits. Application of this calculator, assuming that disease prevalence = 0.05, disease allele frequency = 0.05, and genotype relative risk = 2, indicates a sample size of 676 needed to achieve power of 80% for an allelic test at α = 0.05. However, our study design also includes quantitative traits. The instrument Power for Genetic Association Analyses22 (Menashe et al., 2008) does not include allelic tests. Nevertheless, assuming the dominant mode of inheritance, this statistical instrument indicates approximately the same sample size under the same parameters. General sample size calculators (such as23) indicate a sample size of 385 at α = 0.05, assuming that the actual population is very large (more than 1 million).
Power refers to the number of patients required to avoid a type II error (false-negative results) in a comparative study. Sample size estimation indicates that power of the present study is low. Nevertheless, the study showed a number of statistically significant results, probably due to detailed phenotyping that enabled using a smaller subset of patients (Yehia and Eng, 2019).
Protein Networks
Interactions Suggested by This Study
NRG1 and PIP4K2A could act in concert via their connections with GSK3B (Figure 1; Carter, 2007; Beaulieu, 2012; Mei and Nave, 2014; Jiang et al., 2015; Greene and Hoshi, 2017). ERBB4 and its ligand NRG1 play an important role in neurodevelopment, neurotransmission, and synaptic plasticity, and this receptor is present on GABAergic, glutamatergic and dopaminergic neurons (Mei and Nave, 2014). One of its functions is to promote the inhibitory GABA release. Thus, together with KCNQ/M-channels activated by PIP2 (Greene and Hoshi, 2017), ERBB4 takes part in regulation of neuronal excitability, an aspect that could be important in the pathogenesis of bipolar disorder (Berridge, 2014).
Serotonin acts upon the brain and peripheral immune system components, while both types of these components regulate the serotonin neurotransmission (Robson et al., 2017; Wu et al., 2019). This connection could be the key to the observed immune system abnormalities strongly associated with depression (Felger and Lotrich, 2013) and suggests a possibility of an interaction between HTR2C and IL13RA2. The immune system could therefore be a biological factor that modulates the severity of depression (Felger and Lotrich, 2013).
On the other hand, knowing that serotonin leads to inhibition of KCNQ/M-channels (Colino and Halliwell, 1987; Stephens et al., 2018) and inflammation leads to production of reactive oxygen species resulting in increased neuronal excitability (Berridge, 2014), NRG1, PIP4K2A, HTR2C, and IL13RA2 may have further interconnected roles in the pathophysiology of depressive and manic episodes and the response to antidepressant medication.
Interactions Predicted by the String Database Relevant to This Study
Generalized protein networks may be visualized with String V.11 (Szklarczyk et al., 2019). In fact, the protein products of the genes described in this study – GSK3B, BDNF, NRG1, NGF, HTR2C, PIP4K2A, IL13RA2, IL13, ERBB4, PIK3CA, PIK3CB, PDPK1, AKT1, the three M-channel subunits KCNQ2, KCNQ3, and KCNQ5, and neurotrophic receptors NTRK1 and NTRK2 – are deemed to be functionally connected (Supplementary Figure S1A). Adding top 20 predicted functional partners to this network within the first shell of interactions (a direct connection with the input proteins) reveals a larger network (Supplementary Figure S1B).
It is important to note that predicted interactions should be interpreted with caution: bioinformatic instruments that predict interaction networks (such as String) cannot extract all connections relevant in psychiatric disorders, not they can draw protein networks relevant only in psychiatric disorders. For example, despite evidence in literature indicating regulation of KCNQ2 by GSK3B (Carter, 2007; Wildburger and Laezza, 2012; Jiang et al., 2015; Figure 1), no such interaction was predicted by String (Supplementary Figures S1A,B).
Despite this, predicted interactions may be used to pinpoint interesting functional candidates that may become subjects of future studies. For instance, String indicates a functional connection between HTR2C and IL13RA2 through the interleukin 4 receptor subunit α (IL-4R). A heterodimer formed by this subunit and the interleukin 13 receptor subunit α1 binds both IL-13 and IL-4 (Karo-Atar et al., 2018). IL-4 is known to be implicated in psychiatric disorders, in particular, in the pathogenesis of depression (Wachholz et al., 2017). One of IL-4’s roles is regulation of the serotonin transporter (Mossner et al., 2001). PPP2CA codes for the protein phosphatase 2 catalytic subunit α of protein phosphatase 2A (PP2A), an important component of AKT/GSK3 signaling implicated in a number of psychiatric disorders, including depression and bipolar disorder (Beaulieu, 2012), as well as response to psychotropic drugs, including antidepressants and lithium (Beaulieu et al., 2009). Protein products of MTOR and TSC2 are implicated via their connections with AKT1 in regulation of synaptic plasticity and memory (Emamian, 2012); these are impaired in depression, possibly as a result of a reduction of hippocampal volumes (MacQueen and Frodl, 2011). As mentioned earlier, PIK3R1 and PIK3R2 code for regulatory subunits p85α and p85β, while PIK3CA codes for the catalytic subunit p110α of PI3K; expression levels of these subunits were significantly altered in suicide completers (Dwivedi et al., 2008). Suicide is a psychiatric phenotype associated with depression (Mullins et al., 2019), so pathogenic mechanisms may be shared between the two phenotypes. Finally, β-catenin encoded by CTNNB1 is implicated in a number of molecular pathways relevant in psychiatric disorders (Freyberg et al., 2010; Wisniewska, 2013). CTNNB1 also contains damaging genetic variants in several neurodevelopmental disorders, including schizophrenia (Levchenko et al., 2015), while some antipsychotic drugs result in increased levels of β-catenin in the brain (Freyberg et al., 2010). Bipolar disorder and schizophrenia share pathogenic mechanisms (Gordovez and McMahon, 2020), so β-catenin might be relevant in the pathogenesis of bipolar disorder as well (Guo et al., 2019).
Study Limitations
The main limitation of this genetic study is a modest number of patients, which is reflected in reduced statistical power. This might have been the reason several interesting (from the point of view of predicted biological function) genetic candidates failed to show an association with clinical subphenotypes. Among these variants are rs66866077 and rs79679324 (that may modify the BDNF promoter activity), as well as rs1053454 and rs2230469 (that determine the PIP4K2A level of expression in various parts of the brain). The same may be noted about two novel, apparently deleterious variants NC_000011.10:g.27658302T > C (BDNF) and NC_000023.11:g.114906768_114906848del (HTR2C). Larger cohorts are necessary in order to investigate significance of these putative functional variants.
Another limitation is that any statistics-based study only points out avenues for further research, so in order to prove biological relevance of candidate genetic biomarkers, described in this paper, functional studies deploying cellular or animal models are warranted.
Data Availability Statement
The datasets presented in this article are available at dbSNP: https://www.ncbi.nlm.nih.gov/projects/SNP/snp_ss.cgi?subsnp_ id=ss2137544101, https://www.ncbi.nlm.nih.gov/projects/SNP/snp_ss.cgi?subsnp_id=ss3986007706, https://www.ncbi.nlm.nih.gov/projects/SNP/snp_ss.cgi?subsnp_id=ss3986007707, https://www.ncbi.nlm.nih.gov/projects/SNP/snp_ss.cgi?subsnp_id=ss3986007708, https://www.ncbi.nlm.nih.gov/projects/SNP/snp_ss. cgi?subsnp_id=ss3986007709, https://www.ncbi.nlm.nih.gov/projects/SNP/snp_ss.cgi?subsnp_id=ss3986007710, https://www. ncbi.nlm.nih.gov/projects/SNP/snp_ss.cgi?subsnp_id=ss398600 7711, and https://www.ncbi.nlm.nih.gov/dbvar/studies/nstd180.
Ethics Statement
The studies involving human participants were reviewed and approved by the Local Bioethics Committee of the Mental Health Research Institute in Tomsk, Russia. The patients/participants provided their written informed consent to participate in this study.
Author Contributions
NV, NB, and SI contributed to the conception and design of the study. GS was the psychiatrist who recruited and clinically assessed the patients. SI recruited the controls. GS and NV built the clinical database. NV and IP performed the sequencing. TN performed the sequencing analysis, association study, and a part of functional annotation. AL supervised the work of TN, performed the remaining part of functional annotation, integrated the study results, and wrote the manuscript. All authors contributed to the manuscript revision and read and approved the submitted version.
Funding
This work was supported by the Russian Foundation for Basic Research (grant number 17-29-02205). AL was supported by funding for the project number 51148284 from Saint Petersburg State University, Saint Petersburg, Russia. TN was supported by funding for the project number 51143531 from Saint Petersburg State University, Saint Petersburg, Russia.
Conflict of Interest
The authors declare that the research was conducted in the absence of any commercial or financial relationships that could be construed as a potential conflict of interest.
The reviewer, AK, declared a shared affiliation, with no collaboration, with one of the authors, AL, to the handling editor at the time of review.
Acknowledgments
The work was in part made possible thanks to the Competitiveness Enhancement Program of National Research Tomsk Polytechnic University, Tomsk, Russia.
Supplementary Material
The Supplementary Material for this article can be found online at: https://www.frontiersin.org/articles/10.3389/fgene.2020.00936/full#supplementary-material
FIGURE S1 | Shown are molecular interactions as predicted by String. (A) A network limited only to input proteins. In evidence mode in String, an edge is drawn with differently colored lines that represent the existence of various types of evidence used in predicting the associations. Blue line – cooccurrence evidence, purple line – experimental evidence, yellow line – textmining evidence (i.e., the two proteins are co-mentioned in PubMed abstracts), light blue line – database evidence, and black line – coexpression evidence. (B) The same network expanded with additional top 20 predicted functional partners within the first shell of interactions (a direct connection with the input proteins). In confidence mode in String the thickness of the line indicate the degree of confidence prediction of the interaction.
Footnotes
- ^ https://www.ampliseq.com
- ^ https://broadinstitute.github.io/picard/
- ^ http://www.bioinformatics.babraham.ac.uk/projects/fastqc/
- ^ http://bio-bwa.sourceforge.net/
- ^ https://software.broadinstitute.org/gatk/documentation/tooldocs/4.1.2.0/
- ^ http://genome.ucsc.edu/cgi-bin/hgLiftOver
- ^ https://www.ncbi.nlm.nih.gov/snp/
- ^ http://exac.broadinstitute.org/
- ^ https://gnomad.broadinstitute.org/
- ^ https://software.broadinstitute.org/gatk/
- ^ https://www.cog-genomics.org/plink/2.0/
- ^ https://gtexportal.org
- ^ https://www.rdocumentation.org/packages/SNPRelate
- ^ https://www.rdocumentation.org/packages/dplyr
- ^ https://www.rdocumentation.org/packages/GenABEL
- ^ https://www.rdocumentation.org/packages/ggplot2
- ^ https://www.rdocumentation.org/packages/manhattanly
- ^ https://www.rdocumentation.org/packages/GWASTools
- ^ https://www.rdocumentation.org/packages/GENESIS
- ^ https://ldlink.nci.nih.gov/
- ^ http://zzz.bwh.harvard.edu/gpc/
- ^ https://dceg.cancer.gov/tools/design/pga
- ^ https://www.surveymonkey.com/mp/sample-size-calculator/
References
Adli, M., Hollinde, D. L., Stamm, T., Wiethoff, K., Tsahuridu, M., Kirchheiner, J., et al. (2007). Response to lithium augmentation in depression is associated with the glycogen synthase kinase 3-beta -50T/C single nucleotide polymorphism. Biol. Psychiatry 62, 1295–1302. doi: 10.1016/j.biopsych.2007.03.023
Alexopoulos, G. S., Glatt, C. E., Hoptman, M. J., Kanellopoulos, D., Murphy, C. F., Kelly, R. E., et al. (2010). BDNF val66met polymorphism, white matter abnormalities and remission of geriatric depression. J. Affect. Disord. 125, 262–268. doi: 10.1016/j.jad.2010.02.115
Avery, B. M., and Vrshek-Schallhorn, S. (2016). Nonsynonymous HTR2C polymorphism predicts cortisol response to psychosocial stress I: effects in males and females. Psychoneuroendocrinology 70, 134–141. doi: 10.1016/j.psyneuen.2015.12.023
Bakker, S. C., Hoogendoorn, M. L., Hendriks, J., Verzijlbergen, K., Caron, S., Verduijn, W., et al. (2007). The PIP5K2A and RGS4 genes are differentially associated with deficit and non-deficit schizophrenia. Genes Brain Behav. 6, 113–119. doi: 10.1111/j.1601-183x.2006.00234.x
Barbosa, I. G., Huguet, R. B., Neves, F. S., Reis, H. J., Bauer, M. E., Janka, Z., et al. (2011). Impaired nerve growth factor homeostasis in patients with bipolar disorder. World J Biol Psychiatry 12, 228–232. doi: 10.3109/15622975.2010.518629
Beaulieu, J. M. (2012). A role for Akt and glycogen synthase kinase-3 as integrators of dopamine and serotonin neurotransmission in mental health. J. Psychiatry Neurosci. 37, 7–16. doi: 10.1503/jpn.110011
Beaulieu, J. M., Gainetdinov, R. R., and Caron, M. G. (2009). Akt/GSK3 signaling in the action of psychotropic drugs. Annu. Rev. Pharmacol. Toxicol. 49, 327–347. doi: 10.1146/annurev.pharmtox.011008.145634
Begni, V., Riva, M. A., and Cattaneo, A. (2017). Cellular and molecular mechanisms of the brain-derived neurotrophic factor in physiological and pathological conditions. Clin. Sci. 131, 123–138. doi: 10.1042/cs20160009
Benedetti, F., Poletti, S., Radaelli, D., Locatelli, C., Pirovano, A., Lorenzi, C., et al. (2015). Lithium and GSK-3beta promoter gene variants influence cortical gray matter volumes in bipolar disorder. Psychopharmacology 232, 1325–1336. doi: 10.1007/s00213-014-3770-4
Benedetti, F., Serretti, A., Pontiggia, A., Bernasconi, A., Lorenzi, C., Colombo, C., et al. (2005). Long-term response to lithium salts in bipolar illness is influenced by the glycogen synthase kinase 3-beta -50 T/C SNP. Neurosci. Lett. 376, 51–55. doi: 10.1016/j.neulet.2004.11.022
Berridge, M. J. (2014). Calcium signalling and psychiatric disease: bipolar disorder and schizophrenia. Cell Tissue Res. 357, 477–492. doi: 10.1007/s00441-014-1806-z
Bersani, G., Iannitelli, A., Fiore, M., Angelucci, F., and Aloe, L. (2000). Data and hypotheses on the role of nerve growth factor and other neurotrophins in psychiatric disorders. Med. Hypoth. 55, 199–207. doi: 10.1054/mehy.1999.1044
Bipolar Disorder and Schizophrenia Working Group of the Psychiatric Genomics Consortium (2018). Genomic dissection of bipolar disorder and schizophrenia, including 28 subphenotypes. Cell 173, 1705–1715.
Bolger, A. M., Lohse, M., and Usadel, B. (2014). Trimmomatic: a flexible trimmer for Illumina sequence data. Bioinformatics 30, 2114–2120. doi: 10.1093/bioinformatics/btu170
Brummett, B. H., Babyak, M. A., Williams, R. B., Harris, K. M., Jiang, R., Kraus, W. E., et al. (2014). A putatively functional polymorphism in the HTR2C gene is associated with depressive symptoms in white females reporting significant life stress. PLoS One 9:e114451. doi: 10.1371/journal.pone.0114451
Bureau, A., Beaulieu, J. M., Paccalet, T., Chagnon, Y. C., and Maziade, M. (2017). The interaction of GSK3B and FXR1 genotypes may influence the mania and depression dimensions in mood disorders. J. Affect. Disord. 213, 172–177. doi: 10.1016/j.jad.2017.02.023
Carter, C. J. (2007). Multiple genes and factors associated with bipolar disorder converge on growth factor and stress activated kinase pathways controlling translation initiation: implications for oligodendrocyte viability. Neurochem. Int. 50, 461–490. doi: 10.1016/j.neuint.2006.11.009
Chagraoui, A., Thibaut, F., Skiba, M., Thuillez, C., and Bourin, M. (2016). 5-HT2C receptors in psychiatric disorders: a review. Prog. Neuropsychopharmacol. Biol. Psychiatry 66, 120–135. doi: 10.1016/j.pnpbp.2015.12.006
Chen, X., Long, F., Cai, B., Chen, X., Qin, L., and Chen, G. (2017). A novel relationship for schizophrenia, bipolar, and major depressive disorder. part 8: a hint from chromosome 8 high density association screen. Mol. Neurobiol. 54, 5868–5882. doi: 10.1007/s12035-016-0102-1
Chi, M. H., Chang, H. H., Lee, S. Y., Lee, I. H., Gean, P. W., Yang, Y. K., et al. (2010). Brain derived neurotrophic factor gene polymorphism (Val66Met) and short-term antidepressant response in major depressive disorder. J. Affect. Disord. 126, 430–435. doi: 10.1016/j.jad.2010.07.006
Choi, M. J., Kang, R. H., Lim, S. W., Oh, K. S., and Lee, M. S. (2006). Brain-derived neurotrophic factor gene polymorphism (Val66Met) and citalopram response in major depressive disorder. Brain Res. 1118, 176–182. doi: 10.1016/j.brainres.2006.08.012
Choi, Y., and Chan, A. P. (2015). PROVEAN web server: a tool to predict the functional effect of amino acid substitutions and indels. Bioinformatics 31, 2745–2747. doi: 10.1093/bioinformatics/btv195
Cingolani, P., Platts, A., Wang, L., Le, L., Coon, M., Nguyen, T., et al. (2012). A program for annotating and predicting the effects of single nucleotide polymorphisms, SnpEff: SNPs in the genome of Drosophila melanogaster strain w1118; iso-2; iso-3. Fly 6, 80–92. doi: 10.4161/fly.19695
Cirulli, F., and Alleva, E. (2009). The NGF saga: from animal models of psychosocial stress to stress-related psychopathology. Front. Neuroendocrinol. 30, 379–395. doi: 10.1016/j.yfrne.2009.05.002
Colino, A., and Halliwell, J. V. (1987). Differential modulation of three separate K-conductances in hippocampal CA1 neurons by serotonin. Nature 328, 73–77. doi: 10.1038/328073a0
Colle, R., Gressier, F., Verstuyft, C., Deflesselle, E., Lepine, J. P., Ferreri, F., et al. (2015). Brain-derived neurotrophic factor Val66Met polymorphism and 6-month antidepressant remission in depressed caucasian patients. J. Affect. Disord. 175, 233–240. doi: 10.1016/j.jad.2015.01.013
Dang, R., Cai, H., Zhang, L., Liang, D., Lv, C., Guo, Y., et al. (2016). Dysregulation of Neuregulin-1/ErbB signaling in the prefrontal cortex and hippocampus of rats exposed to chronic unpredictable mild stress. Physiol. Behav. 154, 145–150. doi: 10.1016/j.physbeh.2015.11.023
Delmas, P., and Brown, D. A. (2005). Pathways modulating neural KCNQ/M (Kv7) potassium channels. Nat. Rev. Neurosci. 6, 850–862. doi: 10.1038/nrn1785
DePristo, M. A., Banks, E., Poplin, R., Garimella, K. V., Maguire, J. R., Hartl, C., et al. (2011). A framework for variation discovery and genotyping using next-generation DNA sequencing data. Nat. Genet. 43, 491–498.
Desmet, F. O., Hamroun, D., Lalande, M., Collod-Beroud, G., Claustres, M., and Beroud, C. (2009). Human splicing finder: an online bioinformatics tool to predict splicing signals. Nucleic Acids Res. 37:e67. doi: 10.1093/nar/gkp215
Di Giovanni, G., and De Deurwaerdere, P. (2016). New therapeutic opportunities for 5-HT2C receptor ligands in neuropsychiatric disorders. Pharmacol. Ther. 157, 125–162. doi: 10.1016/j.pharmthera.2015.11.009
Di Paolo, G., Moskowitz, H. S., Gipson, K., Wenk, M. R., Voronov, S., Obayashi, M., et al. (2004). Impaired PtdIns(4,5)P2 synthesis in nerve terminals produces defects in synaptic vesicle trafficking. Nature 431, 415–422. doi: 10.1038/nature02896
Drago, A., and Serretti, A. (2009). Focus on HTR2C: a possible suggestion for genetic studies of complex disorders. Am. J. Med. Genet. B Neuropsychiatr. Genet. 150B, 601–637. doi: 10.1002/ajmg.b.30864
Dwivedi, Y., Rizavi, H. S., Teppen, T., Zhang, H., Mondal, A., Roberts, R. C., et al. (2008). Lower phosphoinositide 3-kinase (PI 3-kinase) activity and differential expression levels of selective catalytic and regulatory PI 3-kinase subunit isoforms in prefrontal cortex and hippocampus of suicide subjects. Neuropsychopharmacology 33, 2324–2340. doi: 10.1038/sj.npp.1301641
Emamian, E. S. (2012). AKT/GSK3 signaling pathway and schizophrenia. Front. Mol. Neurosci. 5:33. doi: 10.3389/fnmol.2012.00033
Fedorenko, O., Strutz-Seebohm, N., Henrion, U., Ureche, O. N., Lang, F., Seebohm, G., et al. (2008). A schizophrenia-linked mutation in PIP5K2A fails to activate neuronal M channels. Psychopharmacology 199, 47–54. doi: 10.1007/s00213-008-1095-x
Fedorenko, O., Tang, C., Sopjani, M., Foller, M., Gehring, E. M., Strutz-Seebohm, N., et al. (2009). PIP5K2A-dependent regulation of excitatory amino acid transporter EAAT3. Psychopharmacology 206, 429–435. doi: 10.1007/s00213-009-1621-5
Fedorenko, O. Y., Loonen, A. J., Lang, F., Toshchakova, V. A., Boyarko, E. G., Semke, A. V., et al. (2014). Association study indicates a protective role of phosphatidylinositol-4-phosphate-5-kinase against tardive dyskinesia. Int. J. Neuropsychopharmacol. 18:yu098.
Fedorenko, O. Y., Rudikov, E. V., Gavrilova, V. A., Boiarko, E. G., Semke, A. V., and Ivanova, S. A. (2013). Association of (N251S)-PIP5K2A with schizophrenic disorders: a study of the Russian population of Siberia. Zh Nevrol Psikhiatr Im S S Korsakova 113, 58–61.
Felger, J. C., and Lotrich, F. E. (2013). Inflammatory cytokines in depression: neurobiological mechanisms and therapeutic implications. Neuroscience 246, 199–229. doi: 10.1016/j.neuroscience.2013.04.060
Fishilevich, S., Nudel, R., Rappaport, N., Hadar, R., Plaschkes, I., Iny Stein, T., et al. (2017). GeneHancer: genome-wide integration of enhancers and target genes in GeneCards. Database 2017:bax028.
Freland, L., and Beaulieu, J. M. (2012). Inhibition of GSK3 by lithium, from single molecules to signaling networks. Front. Mol. Neurosci. 5:14. doi: 10.3389/fnmol.2012.00014
Freyberg, Z., Ferrando, S. J., and Javitch, J. A. (2010). Roles of the Akt/GSK-3 and Wnt signaling pathways in schizophrenia and antipsychotic drug action. Am. J. Psychiatry 167, 388–396. doi: 10.1176/appi.ajp.2009.08121873
Gordovez, F. J. A., and McMahon, F. J. (2020). The genetics of bipolar disorder. Mol. Psychiatry 25, 544–559.
Greene, D. L., and Hoshi, N. (2017). Modulation of Kv7 channels and excitability in the brain. Cell Mol. Life. Sci. 74, 495–508. doi: 10.1007/s00018-016-2359-y
Grunnet, M., Strobaek, D., Hougaard, C., and Christophersen, P. (2014). Kv7 channels as targets for anti-epileptic and psychiatric drug-development. Eur. J. Pharmacol. 726, 133–137. doi: 10.1016/j.ejphar.2014.01.017
Guo, X., Yang, J., Huang, J., Chen, Z., Wu, X., Zhu, L., et al. (2019). Influence of CTNNB1 rs2953 polymorphism on schizophrenia susceptibility in Chinese Han population through modifying miR-485 binding to CTNNB1. Genes Brain Behav. 18:e12524. doi: 10.1111/gbb.12524
Hall, J., Whalley, H. C., Job, D. E., Baig, B. J., Mcintosh, A. M., Evans, K. L., et al. (2006). A neuregulin 1 variant associated with abnormal cortical function and psychotic symptoms. Nat. Neurosci. 9, 1477–1478. doi: 10.1038/nn1795
Harada, Y., Sakai, M., Kurabayashi, N., Hirota, T., and Fukada, Y. (2005). Ser-557-phosphorylated mCRY2 is degraded upon synergistic phosphorylation by glycogen synthase kinase-3 beta. J. Biol. Chem. 280, 31714–31721. doi: 10.1074/jbc.m506225200
He, Z., Li, Z., Shi, Y., Tang, W., Huang, K., Ma, G., et al. (2007). The PIP5K2A gene and schizophrenia in the Chinese population–a case-control study. Schizophr. Res. 94, 359–365. doi: 10.1016/j.schres.2007.04.013
Hirschfeld, R. M. (2014). Differential diagnosis of bipolar disorder and major depressive disorder. J. Affect. Disord. 169(Suppl. 1), S12–S16.
Hong, L. E., Wonodi, I., Stine, O. C., Mitchell, B. D., and Thaker, G. K. (2008). Evidence of missense mutations on the neuregulin 1 gene affecting function of prepulse inhibition. Biol. Psychiatry 63, 17–23. doi: 10.1016/j.biopsych.2007.05.011
Hosang, G. M., Shiles, C., Tansey, K. E., Mcguffin, P., and Uher, R. (2014). Interaction between stress and the BDNF Val66Met polymorphism in depression: a systematic review and meta-analysis. BMC Med. 12:7. doi: 10.1186/1741-7015-12-7
Inkster, B., Nichols, T. E., Saemann, P. G., Auer, D. P., Holsboer, F., Muglia, P., et al. (2009). Association of GSK3beta polymorphisms with brain structural changes in major depressive disorder. Arch. Gen. Psychiatry 66, 721–728.
International Schizophrenia Consortium, Purcell, S. M., Wray, N. R., Stone, J. L., Visscher, P. M., O’donovan, M. C., et al. (2009). Common polygenic variation contributes to risk of schizophrenia and bipolar disorder. Nature 460, 748–752. doi: 10.1038/nature08185
Iwahashi, K., Nishizawa, D., Narita, S., Numajiri, M., Murayama, O., Yoshihara, E., et al. (2014). Haplotype analysis of GSK-3beta gene polymorphisms in bipolar disorder lithium responders and nonresponders. Clin. Neuropharmacol. 37, 108–110. doi: 10.1097/wnf.0000000000000039
Jiang, L., Kosenko, A., Yu, C., Huang, L., Li, X., and Hoshi, N. (2015). Activation of m1 muscarinic acetylcholine receptor induces surface transport of KCNQ channels through a CRMP-2-mediated pathway. J. Cell Sci. 128, 4235–4245. doi: 10.1242/jcs.175547
Jostins, L., and McVean, G. (2016). Trinculo: bayesian and frequentist multinomial logistic regression for genome-wide association studies of multi-category phenotypes. Bioinformatics 32, 1898–1900. doi: 10.1093/bioinformatics/btw075
Karanovic, J., Svikovic, S., Pantovic, M., Durica, S., Brajuskovic, G., Damjanovic, A., et al. (2015). Joint effect of ADARB1 gene, HTR2C gene and stressful life events on suicide attempt risk in patients with major psychiatric disorders. World J. Biol. Psychiatry 16, 261–271. doi: 10.3109/15622975.2014.1000374
Karo-Atar, D., Bitton, A., Benhar, I., and Munitz, A. (2018). Therapeutic Targeting of the Interleukin-4/Interleukin-13 signaling pathway: in allergy and beyond. Biodrugs 32, 201–220. doi: 10.1007/s40259-018-0280-7
Kim, M. S., Shutov, L. P., Gnanasekaran, A., Lin, Z., Rysted, J. E., Ulrich, J. D., et al. (2014). Nerve growth factor (NGF) regulates activity of nuclear factor of activated T-cells (NFAT) in neurons via the phosphatidylinositol 3-kinase (PI3K)-Akt-glycogen synthase kinase 3beta (GSK3beta) pathway. J. Biol. Chem. 289, 31349–31360. doi: 10.1074/jbc.m114.587188
Kishi, T., Yoshimura, R., Ikuta, T., and Iwata, N. (2017). Brain-derived neurotrophic factor and major depressive disorder: evidence from meta-analyses. Front. Psychiatry 8:308. doi: 10.3389/fnmol.2012.00308
Laehnemann, D., Borkhardt, A., and Mchardy, A. C. (2016). Denoising DNA deep sequencing data-high-throughput sequencing errors and their correction. Brief. Bioinform. 17, 154–179. doi: 10.1093/bib/bbv029
Lee, H. J., Son, G. H., and Geum, D. (2013). Circadian rhythm hypotheses of mixed features, antidepressant treatment resistance, and manic switching in bipolar disorder. Psychiatry Investig. 10, 225–232.
Lee, Y., Lim, S. W., Kim, S. Y., Chung, J. W., Kim, J., Myung, W., et al. (2013). Association between the BDNF Val66Met polymorphism and chronicity of depression. Psychiatry Investig. 10, 56–61.
Levchenko, A., Davtian, S., Freylichman, O., Zagrivnaya, M., Kostareva, A., and Malashichev, Y. (2015). Beta-catenin in schizophrenia: possibly deleterious novel mutation. Psychiatry Res. 228, 843–848. doi: 10.1016/j.psychres.2015.05.014
Levchenko, A., Losenkov, I. S., Vyalova, N. M., Simutkin, G. G., Bokhan, N. A., Wilffert, B., et al. (2018). The functional variant rs334558 of GSK3B is associated with remission in patients with depressive disorders. Pharmgenom. Pers. Med. 11, 121–126. doi: 10.2147/pgpm.s171423
Li, H., Handsaker, B., Wysoker, A., Fennell, T., Ruan, J., Homer, N., et al. (2009). The sequence alignment/Map format and SAMtools. Bioinformatics 25, 2078–2079. doi: 10.1093/bioinformatics/btp352
Lin, H. F., Liu, Y. L., Liu, C. M., Hung, S. I., Hwu, H. G., and Chen, W. J. (2005). Neuregulin 1 gene and variations in perceptual aberration of schizotypal personality in adolescents. Psychol. Med. 35, 1589–1598. doi: 10.1017/s0033291705005957
Lin, Y. F., Huang, M. C., and Liu, H. C. (2013). Glycogen synthase kinase 3beta gene polymorphisms may be associated with bipolar I disorder and the therapeutic response to lithium. J. Affect. Disord. 147, 401–406.
Losenkov, I. S., Mulder, N. J. V., Levchuk, L. A., Vyalova, N. M., Loonen, A. J. M., Bosker, F. J., et al. (2020). Association between BDNF gene variant Rs6265 and the severity of depression in antidepressant treatment-free depressed patients. Front. Psychiatry 11:38. doi: 10.3389/fnmol.2012.00038
Lu, B., Nagappan, G., and Lu, Y. (2014). BDNF and synaptic plasticity, cognitive function, and dysfunction. Handb. Exp. Pharmacol. 220, 223–250.
MacQueen, G., and Frodl, T. (2011). The hippocampus in major depression: evidence for the convergence of the bench and bedside in psychiatric research? Mol. Psychiatry 16, 252–264.
Mao, Y. M., Zhao, C. N., Leng, J., Leng, R. X., Ye, D. Q., Zheng, S. G., et al. (2019). Interleukin-13: a promising therapeutic target for autoimmune disease. Cytokine Growth. Factor. Rev. 45, 9–23.
McIntyre, R. S., Filteau, M. J., Martin, L., Patry, S., Carvalho, A., Cha, D. S., et al. (2014). Treatment-resistant depression: definitions, review of the evidence, and algorithmic approach. J. Affect. Disord. 156, 1–7.
McKenna, A., Hanna, M., Banks, E., Sivachenko, A., Cibulskis, K., Kernytsky, A., et al. (2010). The genome analysis toolkit: a MapReduce framework for analyzing next-generation DNA sequencing data. Genome Res. 20, 1297–1303.
Mei, L., and Nave, K. A. (2014). Neuregulin-ERBB signaling in the nervous system and neuropsychiatric diseases. Neuron 83, 27–49.
Menashe, I., Rosenberg, P. S., and Chen, B. E. (2008). PGA: power calculator for case-control genetic association analyses. BMC Genet. 9:36. doi: 10.1186/1741-7015-12-36
Mickey, B. J., Sanford, B. J., Love, T. M., Shen, P. H., Hodgkinson, C. A., Stohler, C. S., et al. (2012). Striatal dopamine release and genetic variation of the serotonin 2C receptor in humans. J. Neurosci. 32, 9344–9350.
Mossner, R., Daniel, S., Schmitt, A., Albert, D., and Lesch, K. P. (2001). Modulation of serotonin transporter function by interleukin-4. Life Sci. 68, 873–880.
Mulder, H., Cohen, D., Scheffer, H., Gispen-De Wied, C., Arends, J., Wilmink, F. W., et al. (2009). HTR2C gene polymorphisms and the metabolic syndrome in patients with schizophrenia: a replication study. J. Clin. Psychopharmacol. 29, 16–20.
Mullins, N., Bigdeli, T. B., Borglum, A. D., Coleman, J. R. I., Demontis, D., Mehta, D., et al. (2019). GWAS of suicide attempt in psychiatric disorders and association with major depression polygenic risk scores. Am. J. Psychiatry 176, 651–660.
Murphy, G. M. Jr., Sarginson, J. E., Ryan, H. S., O’hara, R., and Schatzberg, A. F. (2013). BDNF and CREB1 genetic variants interact to affect antidepressant treatment outcomes in geriatric depression. Pharmacogenet. Genom. 23, 301–313.
Numakawa, T., Odaka, H., and Adachi, N. (2018). Actions of brain-derived neurotrophin factor in the neurogenesis and neuronal function, and its involvement in the pathophysiology of brain diseases. Int. J. Mol. Sci. 19:3650.
Ochi, T., Vyalova, N. M., Losenkov, I. S., Levchuk, L. A., Osmanova, D. Z., Mikhalitskaya, E. V., et al. (2019). Investigating the potential role of BDNF and PRL genotypes on antidepressant response in depression patients: a prospective inception cohort study in treatment-free patients. J. Affect. Disord. 259, 432–439.
Palacios, J. M., Pazos, A., and Hoyer, D. (2017). A short history of the 5-HT2C receptor: from the choroid plexus to depression, obesity and addiction treatment. Psychopharmacology 234, 1395–1418.
Papiol, S., Begemann, M., Rosenberger, A., Friedrichs, H., Ribbe, K., Grube, S., et al. (2011). A phenotype-based genetic association study reveals the contribution of neuregulin1 gene variants to age of onset and positive symptom severity in schizophrenia. Am. J. Med. Genet. B Neuropsychiatr. Genet. 156B, 340–345.
Qin, X. Y., Wu, H. T., Cao, C., Loh, Y. P., and Cheng, Y. (2017). A meta-analysis of peripheral blood nerve growth factor levels in patients with schizophrenia. Mol. Psychiatry 22, 1306–1312.
Risselada, A. J., Vehof, J., Bruggeman, R., Wilffert, B., Cohen, D., Al Hadithy, A. F., et al. (2012). Association between HTR2C gene polymorphisms and the metabolic syndrome in patients using antipsychotics: a replication study. Pharmacogenom. J. 12, 62–67.
Robson, M. J., Quinlan, M. A., and Blakely, R. D. (2017). Immune system activation and depression: roles of serotonin in the central nervous system and periphery. ACS Chem. Neurosci. 8, 932–942.
Roussos, P., Giakoumaki, S. G., Adamaki, E., and Bitsios, P. (2011). The influence of schizophrenia-related neuregulin-1 polymorphisms on sensorimotor gating in healthy males. Biol. Psychiatry 69, 479–486.
Saggers-Gray, L., Heriani, H., Handoko, H. Y., Irmansyah, I., Kusumawardhani, A. A., Widyawati, I., et al. (2008). Association of PIP5K2A with schizophrenia: a study in an indonesian family sample. Am. J. Med. Genet. B Neuropsychiatr. Genet. 147B, 1310–1313.
Saus, E., Soria, V., Escaramis, G., Crespo, J. M., Valero, J., Gutierrez-Zotes, A., et al. (2010). A haplotype of glycogen synthase kinase 3beta is associated with early onset of unipolar major depression. Genes Brain Behav. 9, 799–807.
Schroter, K., Brum, M., Brunkhorst-Kanaan, N., Tole, F., Ziegler, C., Domschke, K., et al. (2019). Longitudinal multi-level biomarker analysis of BDNF in major depression and bipolar disorder. Eur. Arch. Psychiatry Clin. Neurosci. 270, 169–181.
Schroter, K., Brum, M., Brunkhorst-Kanaan, N., Tole, F., Ziegler, C., Domschke, K., et al. (2020). Longitudinal multi-level biomarker analysis of BDNF in major depression and bipolar disorder. Eur. Arch. Psychiatry Clin. Neurosci. 270, 169–181.
Schwab, S. G., Knapp, M., Sklar, P., Eckstein, G. N., Sewekow, C., Borrmann-Hassenbach, M., et al. (2006). Evidence for association of DNA sequence variants in the phosphatidylinositol-4-phosphate 5-kinase IIalpha gene (PIP5K2A) with schizophrenia. Mol. Psychiatry 11, 837–846.
Seebohm, G., Wrobel, E., Pusch, M., Dicks, M., Terhag, J., Matschke, V., et al. (2014). Structural basis of PI(4,5)P2-dependent regulation of GluA1 by phosphatidylinositol-5-phosphate 4-kinase, type II, alpha (PIP5K2A). Pflugers. Arch. 466, 1885–1897.
Seelan, R. S., Khalyfa, A., Lakshmanan, J., Casanova, M. F., and Parthasarathy, R. N. (2008). Deciphering the lithium transcriptome: microarray profiling of lithium-modulated gene expression in human neuronal cells. Neuroscience 151, 1184–1197.
Sengupta, S., Thaci, B., Crawford, A. C., and Sampath, P. (2014). Interleukin-13 receptor alpha 2-targeted glioblastoma immunotherapy. Biomed. Res. Int. 2014:952128.
Sokolowski, M., Wasserman, J., and Wasserman, D. (2016). Polygenic associations of neurodevelopmental genes in suicide attempt. Mol. Psychiatry 21, 1381–1390.
Sole, X., Guino, E., Valls, J., Iniesta, R., and Moreno, V. (2006). SNPStats: a web tool for the analysis of association studies. Bioinformatics 22, 1928–1929.
Stelzer, G., Rosen, N., Plaschkes, I., Zimmerman, S., Twik, M., Fishilevich, S., et al. (2016). The GeneCards suite: from gene data mining to disease genome sequence analyses. Curr. Protoc. Bioinform. 54, 1 30 31–31 30 33.
Stephens, E. K., Baker, A. L., and Gulledge, A. T. (2018). Mechanisms underlying serotonergic excitation of callosal projection neurons in the mouse medial prefrontal cortex. Front. Neural Circ. 12:2. doi: 10.3389/fnmol.2012.00002
Stopkova, P., Saito, T., Fann, C. S., Papolos, D. F., Vevera, J., Paclt, I., et al. (2003). Polymorphism screening of PIP5K2A: a candidate gene for chromosome 10p-linked psychiatric disorders. Am. J. Med. Genet. B Neuropsychiatr. Genet. 123B, 50–58.
Szklarczyk, D., Gable, A. L., Lyon, D., Junge, A., Wyder, S., Huerta-Cepas, J., et al. (2019). STRING v11: protein-protein association networks with increased coverage, supporting functional discovery in genome-wide experimental datasets. Nucleic Acids Res. 47, D607–D613.
Taylor, W. D., Mcquoid, D. R., Ashley-Koch, A., Macfall, J. R., Bridgers, J., Krishnan, R. R., et al. (2011). BDNF Val66Met genotype and 6-month remission rates in late-life depression. Pharmacogenom. J. 11, 146–154.
Tsai, S. J., Cheng, C. Y., Yu, Y. W., Chen, T. J., and Hong, C. J. (2003). Association study of a brain-derived neurotrophic-factor genetic polymorphism and major depressive disorders, symptomatology, and antidepressant response. Am. J. Med. Genet. B Neuropsychiatr. Genet. 123B, 19–22.
Tsai, S. J., Liou, Y. J., Hong, C. J., Yu, Y. W., and Chen, T. J. (2008). Glycogen synthase kinase-3beta gene is associated with antidepressant treatment response in Chinese major depressive disorder. Pharmacogenom. J. 8, 384–390.
Van der Auwera, G. A., Carneiro, M. O., Hartl, C., Poplin, R., Del Angel, G., Levy-Moonshine, A., et al. (2013). From FastQ data to high confidence variant calls: the genome analysis toolkit best practices pipeline. Curr. Protoc. Bioinform. 43, 11.10.1–11.10.
Vyalova, N. M., Pozhidaev, I. V., Osmanova, D. Z., Simutkin, G. G., Ivanova, S. A., and Bokhan, N. A. (2017). Association of polymorphic variants of PIP5K2A and HTR2C genes with response to antidepressant therapy of patients with a current depressive episode. Zh Nevrol Psikhiatr Im S S Korsakova 117, 58–61.
Wachholz, S., Knorr, A., Mengert, L., Plumper, J., Sommer, R., Juckel, G., et al. (2017). Interleukin-4 is a participant in the regulation of depressive-like behavior. Behav. Brain Res. 326, 165–172.
Wang, K., Li, M., and Hakonarson, H. (2010). ANNOVAR: functional annotation of genetic variants from high-throughput sequencing data. Nucleic Acids Res. 38, e164.
Wang, X. C., Xu, D. J., Chen, G. H., Xia, Q., and Liu, L. N. (2014). Association of 2 neurotrophic factor polymorphisms with efficacy of paroxetine in patients with major depressive disorder in a Chinese population. Ther. Drug Monit. 36, 612–617.
Wen, Z., Chen, J., Khan, R. A., Song, Z., Wang, M., Li, Z., et al. (2016). Genetic association between NRG1 and schizophrenia, major depressive disorder, bipolar disorder in Han Chinese population. Am. J. Med. Genet. B Neuropsychiatr. Genet. 171B, 468–478.
Wilcox, A., and Hinchliffe, K. A. (2008). Regulation of extranuclear PtdIns5P production by phosphatidylinositol phosphate 4-kinase 2alpha. FEBS Lett. 582, 1391–1394.
Wildburger, N. C., and Laezza, F. (2012). Control of neuronal ion channel function by glycogen synthase kinase-3: new prospective for an old kinase. Front. Mol. Neurosci. 5:80. doi: 10.3389/fnmol.2012.00080
Wisniewska, M. B. (2013). Physiological role of beta-catenin/TCF signaling in neurons of the adult brain. Neurochem. Res. 38, 1144–1155.
Wu, H., Denna, T. H., Storkersen, J. N., and Gerriets, V. A. (2019). Beyond a neurotransmitter: the role of serotonin in inflammation and immunity. Pharmacol. Res. 140, 100–114.
Xiao, Z., Liu, W., Gao, K., Wan, Q., Yang, C., Wang, H., et al. (2011). Interaction between CRHR1 and BDNF genes increases the risk of recurrent major depressive disorder in Chinese population. PLoS One 6:e28733. doi: 10.1371/journal.pone.028733
Xin, J., Yuan, M., Peng, Y., and Wang, J. (2020). Analysis of the deleterious single-nucleotide polymorphisms associated with antidepressant efficacy in major depressive disorder. Front. Psychiatry 11:151. doi: 10.3389/fnmol.2012.0000151
Yang, C., Xu, Y., Sun, N., Ren, Y., Liu, Z., Cao, X., et al. (2010). The combined effects of the BDNF and GSK3B genes modulate the relationship between negative life events and major depressive disorder. Brain Res. 1355, 1–6.
Yeh, Y. W., Kuo, S. C., Chen, C. Y., Liang, C. S., Ho, P. S., Yen, C. H., et al. (2015). Harm avoidance involved in mediating the association between nerve growth factor (NGF) gene polymorphisms and antidepressant efficacy in patients with major depressive disorder. J. Affect. Disord. 183, 187–194.
Yehia, L., and Eng, C. (2019). Largescale population genomics versus deep phenotyping: brute force or elegant pragmatism towards precision medicine. NPJ Genom. Med. 4:6.
Keywords: neuregulin 1, serotonin 2C receptor, phosphatidylinositol-5-phosphate 4-kinase type 2 alpha, depressive episode, bipolar affective disorder, treatment response, severity, time to recurrence
Citation: Levchenko A, Vyalova NM, Nurgaliev T, Pozhidaev IV, Simutkin GG, Bokhan NA and Ivanova SA (2020) NRG1, PIP4K2A, and HTR2C as Potential Candidate Biomarker Genes for Several Clinical Subphenotypes of Depression and Bipolar Disorder. Front. Genet. 11:936. doi: 10.3389/fgene.2020.00936
Received: 12 November 2019; Accepted: 27 July 2020;
Published: 25 August 2020.
Edited by:
Xiancang Ma, First Affiliated Hospital of Xi’an Jiaotong University, ChinaReviewed by:
Chen Zhang, Shanghai Jiao Tong University, ChinaAllan V. Kalueff, Saint Petersburg State University, Russia
Copyright © 2020 Levchenko, Vyalova, Nurgaliev, Pozhidaev, Simutkin, Bokhan and Ivanova. This is an open-access article distributed under the terms of the Creative Commons Attribution License (CC BY). The use, distribution or reproduction in other forums is permitted, provided the original author(s) and the copyright owner(s) are credited and that the original publication in this journal is cited, in accordance with accepted academic practice. No use, distribution or reproduction is permitted which does not comply with these terms.
*Correspondence: Anastasia Levchenko, a.levchenko@spbu.ru; anastasia.levchenko@mail.mcgill.ca