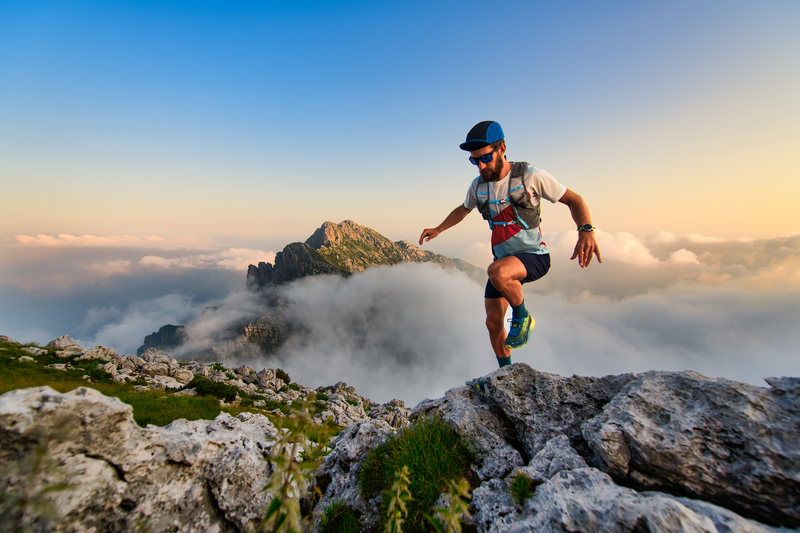
95% of researchers rate our articles as excellent or good
Learn more about the work of our research integrity team to safeguard the quality of each article we publish.
Find out more
EDITORIAL article
Front. Genet. , 25 August 2020
Sec. Statistical Genetics and Methodology
Volume 11 - 2020 | https://doi.org/10.3389/fgene.2020.00927
This article is part of the Research Topic Statistical and Computational Methods for Microbiome Multi-Omics Data View all 11 articles
Editorial on the Research Topic
Statistical and Computational Methods for Microbiome Multi-Omics Data
There has never been a more exciting time to do microbiome research thanks to the recent completion of several population-scale, longitudinal multi-omics studies including the NIH integrative human microbiome project (iHMP; iHMP Consortium, 2019) that have facilitated a multitude of new avenues of research for future investigations. These breakthroughs utilizing multiple ‘omics technologies have paved the way toward investigating biological systems at an unprecedented level of detail, allowing a simultaneous assessment of community function, dynamics, and biochemical signatures across diverse disease states and environments. The field of microbiome multi-omics, however, has not yet reached the maturity attained in other established molecular epidemiology fields such as cancer biomarker discovery and genome-wide association studies (Mallick et al., 2017). As a result, it remains wide open to an in-depth exploration of new analytical methods in order to make the leap from bench to bedside.
This Research Topic is a timely endeavor toward this goal to expand our knowledge on systems biology approaches in understanding microbial communities. Due to the complexity of the associated data, the downstream analysis of microbiome multi-omics remains challenging. While most of the initial studies focused on analyzing single omics (e.g., taxonomic or functional profiles), there has been a shift in the field toward the concurrent investigation of the microbiome and host phenotypes (e.g., metabolomics and host transcriptomics). To this end, many of the articles in this Research Topic focus on new ways to analyze and integrate multi-table data using cutting-edge statistical and computational methods.
Sankaran and Holmes revisit an overwhelmingly large literature and algorithms already available on multi-table data analysis by reviewing both the algorithmic foundations and practical applications of a wide range of analysis approaches and re-evaluate these paradigms with respect to heterogeneity, dimensionality, and sparsity in a fully reproducible setup. In a similar vein, Bodein et al. propose a computational framework to integrate longitudinal microbiome data with other omics and clinical data generated on the same biological specimens based on smoothing splines and multivariate dimension reduction methods. Both these constitute a critical contribution to the field, given the growing commonality of multi-table datasets and the complexity of related study designs, including dietary, pharmaceutical, clinical, and environmental covariates, often with samples from multiple time points or tissues.
Many important questions on microbiome multi-omics data integration remain unaddressed, especially those relating to extracting disease-relevant mechanistic networks that can provide insight into the complex web of host-microbiome interactions. Jiang et al. extensively review statistical aspects of relevant microbiome multi-omics network analysis methods by demystifying each class of methods with respect to their practical applicability and biological interpretability. Zhou and Gallins present a tutorial overview of commonly-used machine learning methods for microbiome host trait prediction, accompanied by validated R/Python implementations. The open-access source codes from these publications not only provide an important resource for algorithm developers but also ensure widespread usage and impact of these methods, facilitating future methodological research advances.
Moving beyond routine univariate analysis methods that ignore the correlations between features, Banerjee et al. take a multivariate approach to differential abundance analysis by jointly modeling all features in a set while maintaining the correct type I error and high power, which is not trivial for many existing per-feature methods (McMurdie and Holmes, 2014; Mandal et al., 2015; Jonsson et al., 2016, 2017; Thorsen et al., 2016; Mallick et al., 2017; Weiss et al., 2017; Hawinkel et al., 2019). Koh et al. introduce a distance-based kernel association test for family-based or longitudinal microbiome studies to associate microbial community composition with any type of host traits based on the generalized linear mixed model, vastly expanding the capability to incorporate non-Gaussian host traits as well as multiple kernels.
Quantitative methods of microbiome multi-omics are by no means limited to downstream analysis of targeted amplicon-based and metagenomic profiling. This Research Topic also contains papers addressing important questions in upstream data processing and quantitative microbiome profiling. For instance, Song et al. focus on the comparison of metagenomic samples using alignment-free methods with reads binning and conclude that alignment-free and alignment-based methods for metagenome comparison complement each other and should be used interactively to understand the dynamics of microbial communities. Yoon et al. estimate feature-feature correlations and partial correlations from robust measurements of microbial cell count, in particular, flow cytometry, and validate the results in a recent quantitative gut microbiome dataset ensuring both statistical rigor and biological relevance.
Several articles in the Research Topic go beyond integrating multiple omics datasets to establishing causation and molecular mechanism, with an emphasis on methods that aim to detect microbiome-mediated signals through causal mediation analysis. While existing methods in this space make strong parametric assumptions, which can be quite detrimental when the assumptions are violated, Carter et al. turn to nonparametric entropy models to detect significant mediation effects in the presence of high-dimensional exposures and mediators. Tang et al. utilize state-of-the-art microbiome compositional mediation analysis procedures to investigate the diet-microbiome-metabolome interaction in cross-sectional multi-omics samples from healthy subjects. Both these analyses estimate the total mediation effects of microbiome composition, as well as feature-specific mediation effects, providing additional mechanistic insights above and beyond a direct causal relationship.
Taken together, the papers in this Research Topic represent both an incredible amount of progress and an enormous potential for further advances in the near future. As a result, we have launched a second edition of the Research Topic where we will continue to add additional methods, research, and review articles over the next year or so.
All authors listed have made a substantial, direct and intellectual contribution to the work, and approved it for publication.
HM is employed by Merck Sharp & Dohme Corp., a subsidiary of Merck & Co., Inc., Kenilworth, NJ, USA.
The remaining authors declare that the research was conducted in the absence of any commercial or financial relationships that could be construed as a potential conflict of interest.
We thank the Frontiers editorial staff for providing outstanding assistance in putting together this Research Topic collection.
Hawinkel, S., Mattiello, F., Bijnens, L., and Thas, O. (2019). A broken promise: microbiome differential abundance methods do not control the false discovery rate. Brief Bioinform. 20, 210–221. doi: 10.1093/bib/bbx104
iHMP Consortium (2019). The integrative human microbiome project. Nature 569, 641–648. doi: 10.1038/s41586-019-1238-8
Jonsson, V., Österlund, T., Nerman, O., and Kristiansson, E. (2016). Statistical evaluation of methods for identification of differentially abundant genes in comparative metagenomics. BMC Genomics 17:78. doi: 10.1186/s12864-016-2386-y
Jonsson, V., Österlund, T., Nerman, O., and Kristiansson, E. (2017). Variability in metagenomic count data and its influence on the identification of differentially abundant genes. J. Comput. Biol. 24, 311–326. doi: 10.1089/cmb.2016.0180
Mallick, H., Ma, S., Franzosa, E. A., Vatanen, T., Morgan, X. C., and Huttenhower, C. (2017). Experimental design and quantitative analysis of microbial community multiomics. Genome Biol. 18:228. doi: 10.1186/s13059-017-1359-z
Mandal, S., Van Treuren, W., White, R. A., Eggesbø, M., Knight, R., and Peddada, S. D. (2015). Analysis of composition of microbiomes: a novel method for studying microbial composition. Microb. Ecol. Health Dis. 26:27663. doi: 10.3402/mehd.v26.27663
McMurdie, P. J., and Holmes, S. (2014). Waste not, want not: why rarefying microbiome data is inadmissible. PLoS Comput. Biol. 10:e1003531. doi: 10.1371/journal.pcbi.1003531
Thorsen, J., Brejnrod, A., Mortensen, M., Rasmussen, M. A., Stokholm, J., Al-Soud, W. A., et al. (2016). Large-scale benchmarking reveals false discoveries and count transformation sensitivity in 16S rRNA gene amplicon data analysis methods used in microbiome studies. Microbiome 4:62. doi: 10.1186/s40168-016-0208-8
Keywords: microbiome, metagenomics, metabolomics, multi-omics, biostatistics, computational biology, statistical genomics, data science
Citation: Mallick H, Bucci V and An L (2020) Editorial: Statistical and Computational Methods for Microbiome Multi-Omics Data. Front. Genet. 11:927. doi: 10.3389/fgene.2020.00927
Received: 05 July 2020; Accepted: 24 July 2020;
Published: 25 August 2020.
Edited and reviewed by: Simon Charles Heath, Center for Genomic Regulation (CRG), Spain
Copyright © 2020 Mallick, Bucci and An. This is an open-access article distributed under the terms of the Creative Commons Attribution License (CC BY). The use, distribution or reproduction in other forums is permitted, provided the original author(s) and the copyright owner(s) are credited and that the original publication in this journal is cited, in accordance with accepted academic practice. No use, distribution or reproduction is permitted which does not comply with these terms.
*Correspondence: Himel Mallick, aGltZWwubWFsbGlja0BtZXJjay5jb20=
Disclaimer: All claims expressed in this article are solely those of the authors and do not necessarily represent those of their affiliated organizations, or those of the publisher, the editors and the reviewers. Any product that may be evaluated in this article or claim that may be made by its manufacturer is not guaranteed or endorsed by the publisher.
Research integrity at Frontiers
Learn more about the work of our research integrity team to safeguard the quality of each article we publish.