- 1Key Laboratory of Animal Genetics, Breeding and Reproduction of Shaanxi Province, College of Animal Science and Technology, Northwest A&F University, Xianyang, China
- 2Key Laboratory of Qinghai-Tibetan Plateau Animal Genetic Resource Reservation and Utilization, Sichuan Province and Ministry of Education, Southwest Minzu University, Chengdu, China
- 3College of Animal Science, Zhejiang University, Hangzhou, China
- 4Mammalian NutriPhysioGenomics, Department of Animal Sciences and Division of Nutritional Sciences, University of Illinois, Urbana, IL, United States
Milk fatty acids secreted by the mammary gland are one of the most important determinants of the nutritional value of goat milk. Unlike cow milk, limited data are available on the transcriptome-wide changes across stages of lactation in dairy goats. In this study, goat mammary gland tissue collected at peak lactation, cessation of milking, and involution were analyzed with digital gene expression (DGE) sequencing to generate longitudinal transcript profiles. A total of 51,299 unigenes were identified and further annotated to 12,763 genes, of which 9,131 were differentially expressed across various stages of lactation. Most abundant genes and differentially expressed genes (DEGs) were functionally classified through clusters of euKaryotic Orthologous Groups (KOG), Gene Ontology (GO), and Kyoto Encyclopedia of Genes and Genomes (KEGG) databases. A total of 16 possible expression patterns were uncovered, and 13 genes were deemed novel candidates for regulation of lactation in the goat: POLG, SPTA1, KLC, GIT2, COPS3, PDP, CD31, USP16/29/37, TLL1, NCAPH, ABI2, DNAJC4, and MAPK8IP3. In addition, PLA2, CPT1, PLD, GGA, SRPRB, and AP4S1 are proposed as novel and promising candidates regulating mammary fatty acid metabolism. “Butirosin and neomycin biosynthesis” and “Glyoxylate and dicarboxylate metabolism” were the most impacted pathways, and revealed novel metabolic alterations in lipid metabolism as lactation progressed. Overall, the present study provides new insights into the synthesis and metabolism of fatty acids and lipid species in the mammary gland along with more detailed information on molecular regulation of lactogenesis. The major findings will benefit efforts to further improve milk quality in dairy goats.
Introduction
Goat milk contains many macro- and micro-nutrients that are essential for the growth and development of a newborn, and has been recognized as beneficial for human health (German et al., 2002; Casado et al., 2009). The nutritional value of goat milk is mainly attributable to its fat and protein fractions, which are critically important to provide both energy and essential nutrients for the humans (Shi et al., 2015). One of the major goals of goat farming is to improve milk quality through the alteration of milk composition, according to specific needs of target groups such as infants or immune-compromised individuals (Wickramasinghe et al., 2012). To achieve this goal, a thorough understanding of milk components and their regulatory factors is required.
The mammary gland has been widely-used as a research model to investigate the mechanisms of milk formation and secretion (Shennan and Peaker, 2000). However, it was not until the mid-2000s that the first study utilizing large-scale transcriptome profiling was published (Rudolph et al., 2003). That original study, although broad in its focus, and a subsequent one underscored that biosynthesis and secretion of lipid in mammary gland tissue is regulated by complex gene networks (Rudolph et al., 2007; Andres and Djonov, 2010). Subsequent work in dairy cows focusing on a curated list of 45 genes associated with lipid synthesis (triacylglycerol and phospholipids) and secretion reported the first longitudinal profiles from late pre-partum to the end of subsequent lactation (Bionaz and Loor, 2008). These observations allowed for the development of a network of genes participating in the coordination of milk fat synthesis and secretion.
Despite the substantial body of work in bovine, research on the mammary gland of small ruminant species, particularly in goats, is limited. For instance, most published studies on goats have focused on functional verification of candidate genes associated with lipid metabolism using data from human, mouse and cattle, including SCD1 (Yao et al., 2017), SREBP1 (Xu et al., 2016), FASN (Zhu et al., 2014), INSIG (Li et al., 2019), and ELOVL7 (Shi et al., 2019). Although those efforts have enhanced our knowledgebase, economically-important traits in livestock such as milk lipid synthesis and composition are genetically affected by some causal genes together with many genes with minor effects, which together result in complex regulatory networks (Goddard and Hayes, 2009; Georges et al., 2019). Extensive and detailed molecular research in dairy cows has underscored that the ruminant mammary gland is a very dynamic organ with remarkable plasticity (Akers, 2017), especially during the transition from pregnancy to lactation. However, there is a paucity of data regarding changes occurring in the goat mammary gland during the physiological transition from established lactation to dry-off to involution. Therefore, for a better understanding of regulatory mechanisms underlying milk fatty acid metabolism and lactation in dairy goats, a systematic screen of gene expression profiles is needed.
Development of high-throughput sequencing has made it feasible to generate a whole genome sequence in the domestic goat (Mardis, 2008; Metzker, 2010). In addition, mining the differentially expressed genes (DEGs) in mammary tissue across discrete stages of lactation could vastly expand our knowledge of the molecular regulation of mammary gland function in small ruminants. Therefore, in the present study we used Illumina digital gene expression (DGE) profiling sequencing to characterize transcript profiles in mammary gland tissue across 3 stages of the lactation cycle in Xinong Saanen dairy goats. Beyond expanding our mechanistic knowledge, identifying novel promising genes responsible for the genetic variation could contribute greatly to our understanding of lactation and milk fatty acid metabolism. It could help in the future to develop a molecular breeding program with the aim of improving fatty acid composition in dairy goat milk.
Materials and Methods
Ethics Statement
The present experiment was conducted in accordance with approved guidelines by the Animal Care and Use Committee of the Northwest A&F University. The committee approved all procedures and experiments with live animals.
Goat Mammary Gland Collection and RNA Extraction
Mammary gland tissue was collected based on methods described previously (Shi et al., 2015). Briefly, a total of nine healthy Xinong Saanen dairy goats (approximately 3 to 4 years old from 2 to 3 parities) were selected from the experimental farm at Northwest A&F University (Shaanxi, China). All goats were managed similarly and were fed a mixed diet comprised of corn, soybean meal, bran, rapeseed meal and mineral-vitamin premix. Approximately 2 g mammary gland tissue was collected from the mid-region of the right mammary gland of each goat at peak lactation (3 goats, 100 days postpartum; L group), dry-off (cessation of milking, 3 goats, 310 days postpartum; D group) and non-lactating/non-pregnant period (involution, 3 goats; NP group). All tissue samples were obtained under sterile conditions on the same day, harvested within 20 min for each and immediately frozen in liquid nitrogen until RNA extraction.
Total RNA extraction, mRNA purification, and cDNA library construction were conducted by LC Sciences (Houston, TX, United States). In brief, total RNA was obtained from mammary gland tissue using a total RNA purification kit (LC Sciences, Houston, TX, United States), treated with RNAase-free DNAase, and re-purified with the RNA easy kit (Qiagen, Valencia, CA, United States) following the manufacturer’s instructions. Total RNA quantity and quality were analyzed with the RNA 6000 Nano LabChip Kit in the Bioanalyzer 2100 system (Agilent Technologies, CA, United States).
cDNA Library Construction and Illumina Sequencing
The mRNA was purified from total RNA using poly-T-oligo-attached magnetic beads (Invitrogen, United States). Following purification, the mRNA was fragmented into small pieces using divalent cations at a high temperature. After phosphatase and polynucleotide kinase (PNK) treatment, the cleaved mRNA fragments were reverse-transcribed. The 200–300 bp fragments were purified using 6% polyacrylamide Tris-borate-EDTA to create the final cDNA library. One end sequencing of 1 × 36 bp was then performed on an Illumina HiSeq 2000 platform following the vendor’s recommended protocols. The application of DGE sequencing to uncover DEGs has advantages such as low cost, rapid analysis, and high accuracy (t Hoen et al., 2008).
Data Filtering and Expression Level Determination
The raw reads were first determined by fastQC1 and filtered by removing potential contamination, reads with unknown base more than 1 N (base not available), and low quality sequences (parameter -q 36 -p 90) using the Fastx_toolkit (0.0.13) (Lohse et al., 2012). All short reads were assembled as transcripts using Trinity software (Haas et al., 2013). As a subset of the transcript, unigene was the longest transcript of each cluster that matched the same reference gene. HTSeq v0.6.1 was used to count the reads numbers mapped to each unigene. By aligning sequences with a goat database constructed previously (BioProject ID: PRJNA243005), the gene expression level was recorded according to the sum frequencies of compared reads. Reads per kilo base of exon model per million mapped reads (RPKM) were used for expression normalization, and the gene expression RPKM values were categorized into three groups: high (≥500 RPKM), medium-to-high (10 to 500 RPKM) and low (≤10 RPKM) (Wickramasinghe et al., 2012; Canovas et al., 2014). Assembled unigenes were annotated to the reference dataset using BLAST. Databases including a manually Annotated and Reviewed Protein Sequence Database (SWISS-PROT), NCBI non-redundant protein sequence database (NR), Pfam database, KOG, GO, and KEGG were performed with an E-value cutoff of 1E-10 according to previously published studies (Ogata et al., 1999; Shi et al., 2015).
Analysis of DEG Data
The normalized ratio of the gene expression signals was log2 transformed. The RVM (Random variance model) N2-test and Chi-square test were applied to filter DEGs. After analyses for statistical significance and FDR (false discovery rate), DEGs were selected according to P < 0.05 and FDR < 0.05 (Wright and Simon, 2003; Yang et al., 2005; Clarke et al., 2008). Bowtie 0.12.8 was used for abundance analysis of gene expression. Short Time-series Expression Miner (STEM) was used for expression pattern analysis (Ernst and Bar-Joseph, 2006). Using the log normalized data method, the “maximum number of model profiles” was set at 20, and the “maximum unit change in model profiles between time points” was set at 2 (Ernst and Bar-Joseph, 2006). Gene co-expression analysis (Pujana et al., 2007; Xu et al., 2013) was performed to track interactions among DEGs. Pearson correlation analysis was performed for each pair of genes, and significantly correlated pairs were used to construct the network with a threshold of 0.92 using a Perl script (Prieto et al., 2008). The number of correlated genes was used for node gene selection. Based on all DEGs identified when adding up the three pairs of comparisons (L vs. D, L vs. NP, D vs. NP), potential candidate genes regulating lactation and milk fatty acid metabolism were identified.
Dynamic Impact Approach (DIA) Analysis
Data were filtered with the threshold at fold-change >2 and P < 0.001, and were mined through an integrative systems biology approach applying the DIA method (Shi et al., 2017). The bovine KEGG pathways and GO biological process category database were used for functional analysis with the DIA. Detailed methodology for data analysis using DIA was described previously (Bionaz et al., 2012b). The estimate of the perturbation in a biological pathway is represented by the “Impact” while the overall direction of the perturbation is represented by the “Flux” (or direction of the impact) (Shi et al., 2017). The impact was obtained by combining the proportion of DEGs with the log2 mean fold-change and mean –log P-value of genes associated with the biological term. The direction of the impact was calculated as the difference of the impact of up-regulated DEGs and downregulated DEGs associated with the biological term (Bionaz et al., 2012b). The analytical flow chart used in the present study is described in Supplementary Figure S1.
Results
Illumina Sequencing and Gene Annotation
A total of 63,256,236 clean reads were obtained with an average of 7,028,470 (range from 3,423,245 to 10,365,063 reads) for each sample. After sequence assembly according to the goat transcriptome sequence published in our previous study (Shi et al., 2015), out of 98,864 transcripts (Supplementary File S2A) the Illumina sequencing uncovered a total of 51,299 unigenes. Among these unigenes, 48,428, 50,442, and 50,684 unigenes were associated with the lactation groups “L,” “D,” and “NP” respectively. The number of unigenes uniquely expressed in the “L,” “D,” and “NP” groups were 94, 225, and 126, respectively (Figure 1A). Importantly, a total of 12,763 genes were identified through annotation, with 12,239, 12,713, and 12,710 genes in the “L,” “D,” and “NP” group, respectively. Among those identified genes, 3, 24, and 6 genes, respectively, were uniquely expressed in each group (Figure 1B).
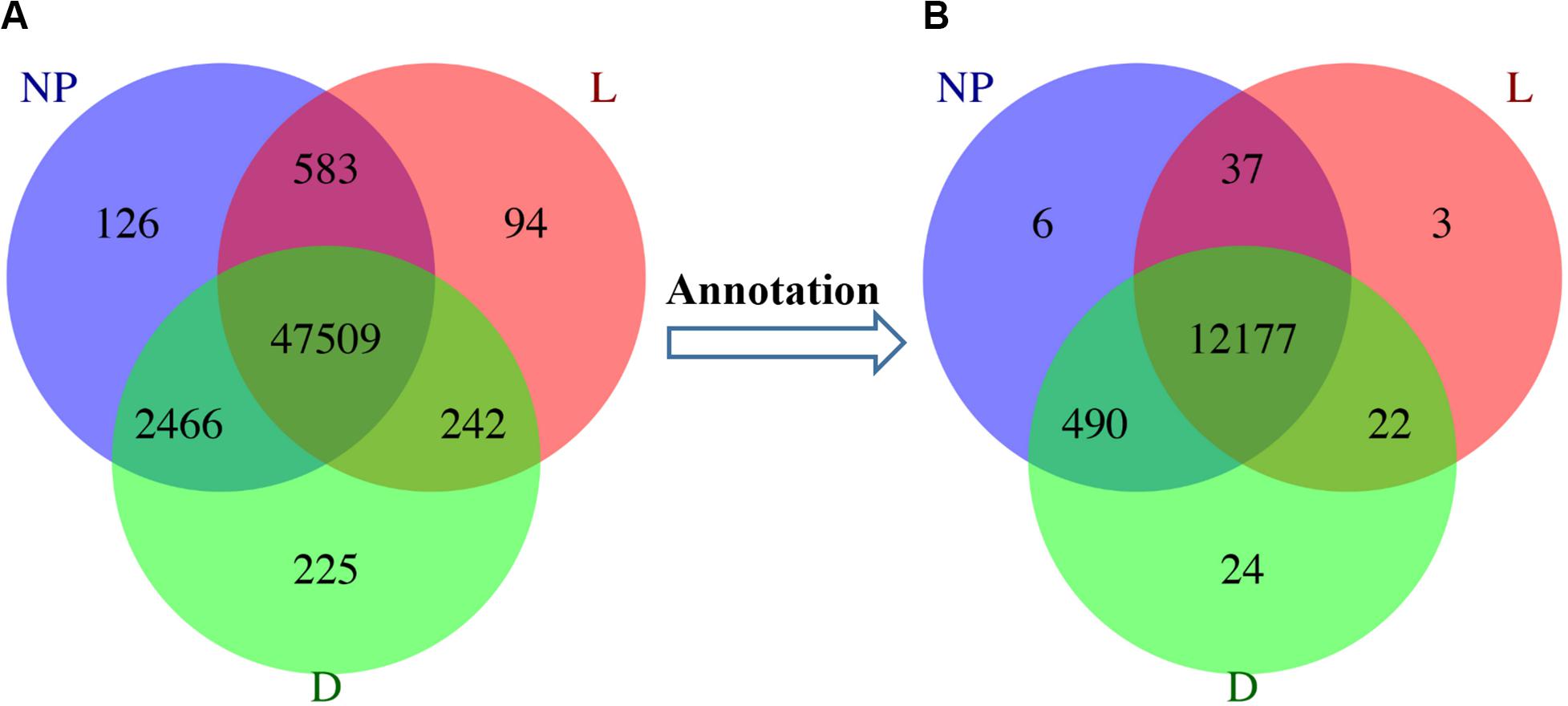
Figure 1. Unigene distribution in goat mammary gland during different stages of lactation. (A) Unigenes distributed in peak lactation (L), dry-off (D) and non-lactating/non-pregnant (NP) periods. (B) The distribution of functional genes during different stages of lactation.
Lipid Biosynthesis and Metabolism-Associated Genes
Gene expression level was evaluated using the RPKM method. Goats in the “L” group expressed a total of 12,239 genes including 65 with the RPKM greater than 500, 1,102 genes with RPKM between 10 and 500, but most genes (11,072) had expression below 10 RPKM. According to the same RPKM classification, out of a total of 12,713 and 10,710 genes in the “D” and “NP” group there were 104 and 77 genes with RPKM greater than 500, 3,470 and 2,262 genes with RPKM between 10 and 500, while 9,139 and 10,371 genes had expression below 10 RPKM.
The most abundant genes were further filtered by focusing on fatty acid biosynthesis and metabolism as well as lipid transport and metabolism pathways or terms using the KOG, GO, and KEGG categories. Of these, the 15 most abundant genes related to lipid transport and metabolism are reported in Supplementary Table S1. FASN, FABP4, ACBP, EBP, RNPEPL1, DEGS1, and SAPOSIN were detected in all three stages of lactation. The most abundant genes involved in intracellular trafficking, secretion, and vesicular transport were SSR4, lepB, SEC61G, AP2M1, ARF1, CHMP2A, CLTA, RAB11B, RAB1A, SDHD, and SEC61 (Supplementary Table S2).
Within the GO fatty acid metabolism category, EHHADH was the most abundant gene in lactation stages “L,” “D,” and “NP,” with RPKM of 24.134, 34.567, and 34.016, respectively. Well-known genes involved in fatty acid metabolism such as ACAA2, ACSL and ACAA had a medium-to-high expression level across all lactation stages (Supplementary Table S3). Within fatty acid biosynthetic process in the GO category, FASN was the most abundant gene across lactation stages with 376.222, 120.146, and 537.391 RPKM in “L,” “D,” and “NP,” respectively. In addition, the most abundant genes observed in all three lactation stages were SCD, ELOVL1, DEGS1, NADH, NDUFAB1, ABDH2, MSMO1, PRKAG1, SC5D, STK11, and TECR (Supplementary Table S4).
For genes involved in fatty acid metabolism signaling pathways revealed by KEGG, ACAA2, ACSL, ACADL, ACADM, ACADS, ACADSB, ACOX1, ACAT1, ADH1, ALDH1, CPT1A, CPT1B, and GCDH were the most abundant genes detected across lactation stages (Supplementary Table S5). Within fatty acid biosynthesis signaling pathway identified through KEGG, FASN, HLCS, MCAT, and OXSM were the top four most abundant genes across lactation stages (Supplementary Table S6).
Identification of Differential Gene Expression Patterns
A total of 29,880 differentially expressed unigenes were detected among different groups, annotated as 9,131 DEGs (Supplementary File S2B). In the “D” and “NP” groups, a total of 46 and 18 unique unigenes were annotated to 6 and 2 DEGs, respectively. Although five uniquely expressed unigenes were detected in the “L” group, they were not annotated to any functional gene (Figure 2). A total of eight unique DEGs across lactation stages are reported in Table 1.
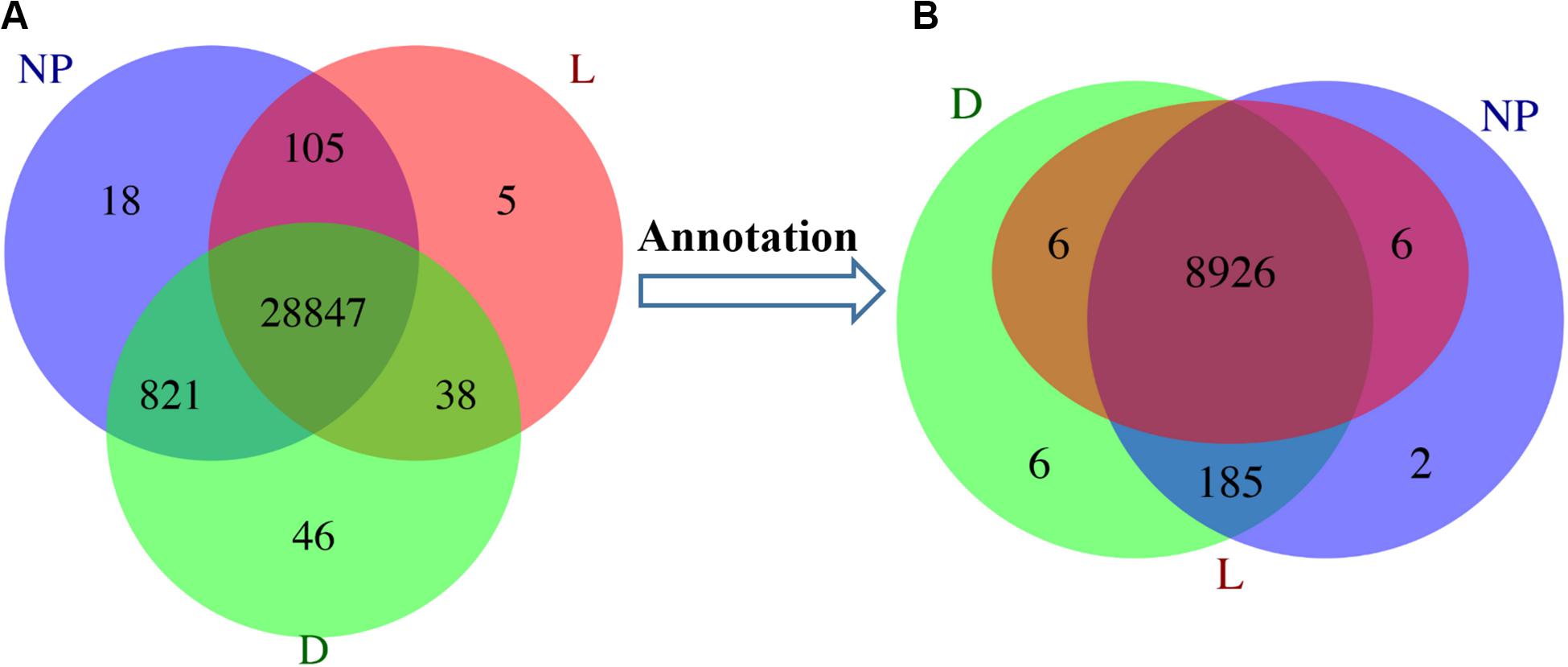
Figure 2. Distribution of DEGs during different stages of lactation. L, peak lactation period; D, dry-off period; NP, non-lactating/non-pregnant period. (A) Differentially expressed unigenes distributed in peak lactation (L), dry-off (D) and non-lactating/non-pregnant (NP) periods. (B) Annotation of DEGs during different stages of lactation.
In total, 50 GO terms including biological process, cellular component and molecular function were significantly enriched with 8,311 DEGs (Figure 3). Of these, transcription, DNA-dependent and regulation of transcription had the most-abundant DEGs across 25 enriched biological processes. With 15 terms in the cellular component category, most DEGs were related to the nucleus, integral to membrane, and cytoplasm. A total of 10 terms involved in molecular function including zinc ion binding, ATP binding, and protein binding had the most DEGs (Figure 3). In addition, a total of 246 KEGG pathways were enriched 5,048 DEGs (Supplementary File S2C) with Insulin signaling, Jak-STAT signaling, Glycerophospholipid metabolism, mTOR signaling and PPAR signaling among the most-relevant to lactation and milk component synthesis regulation.
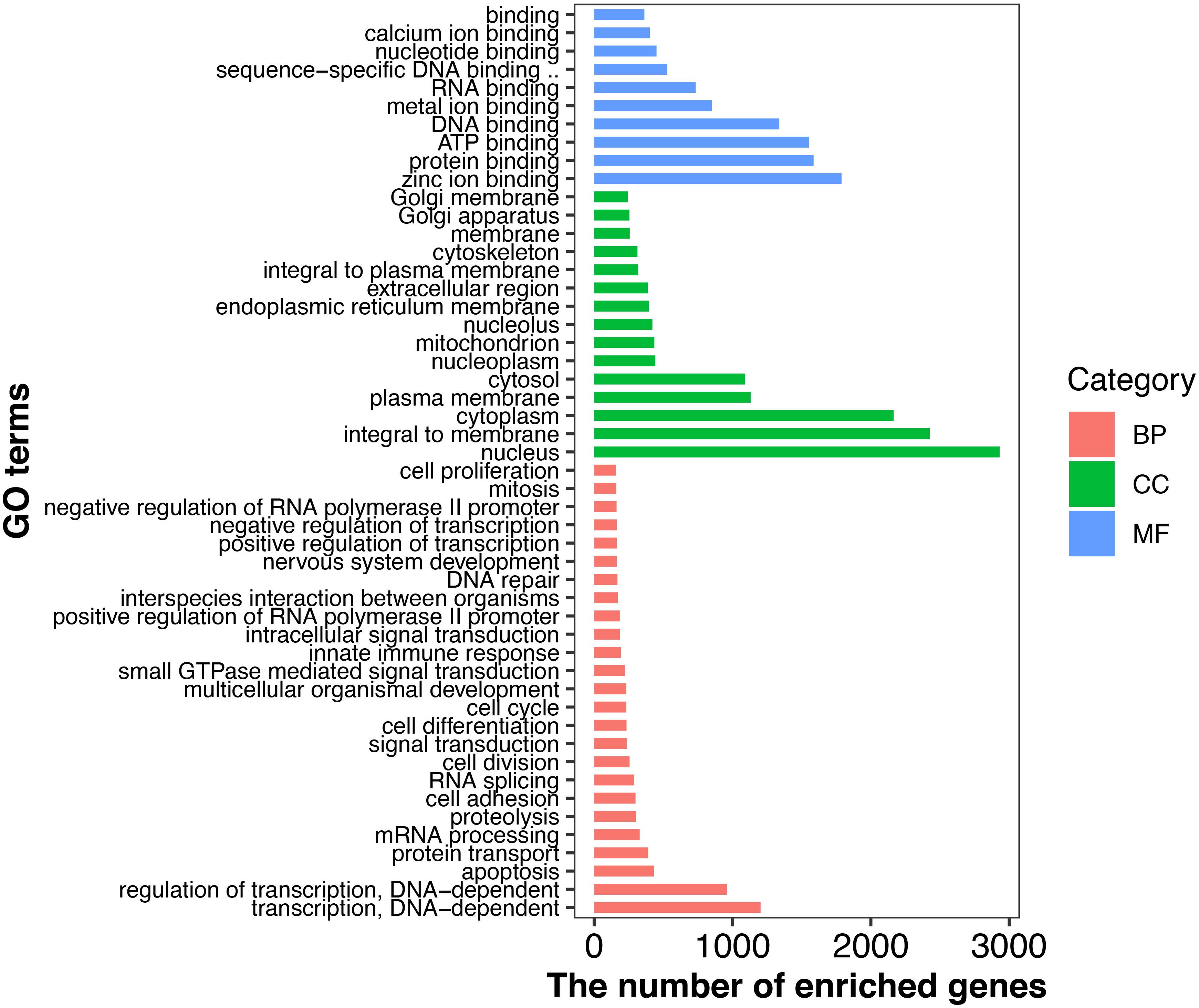
Figure 3. GO classifications of DEGs in goat mammary gland. Distribution of the GO categories assigned to the goat mammary gland transcriptome. DEGs were classified into three categories: biological processes, cellular components, and molecular functions. L, peak lactation period; D, dry-off period; NP, non-lactating/non-pregnant period.
The most abundant DEGs involved in fatty acid biosynthesis and metabolism processes, lipid metabolism, protein metabolism, and lactose metabolism were used to mine within GO and KEGG categories for pathways or terms across lactation stages (Supplementary Tables S7–S10). A total of 48 DEGs were identified in terms of fatty acid metabolic processes/pathways, whereas 18 DEGs including ARL5A, MARK, STAM, and EPHA1 were simultaneously revealed by GO and KEGG (Supplementary Table S7). Among a total of 36 identified DEGs associated with fatty acid biosynthetic processes/pathways, SEMA6, RUNX1T1, and LEF1 were detected by both GO and KEGG (Supplementary Table S7). Within GO and KEGG, the well-known genes GPAT3, ADH and ALDH involved in lipid metabolic processes/pathways were among a total of 29 most abundant DEGs (Supplementary Table S8). Highly expressed DEGs such as NR1F1, EIF3S5, MYH, and ABCF2 were in the lipid biosynthetic processes/pathways (Supplementary Table S8).
For protein metabolism, 4 DEGs were identified in protein biosynthetic processes, 21 DEGs were grouped in protein metabolic process, including STAT5A, IGF1, IGFR2, and MARCH2. Additionally, 15 DEGs were identified within protein export pathway, including GPAT3, NR1F1, and IGFR2 (Supplementary Table S9). B4GALT5, SERPINE1, and MDH2 were identified as the top-most abundant DEGs in the lactose metabolic process/pathway (Supplementary Table S10).
A total of 16 possible expression patterns were detected among the 9,131 DEGs (Figure 4 and Supplementary File S2D). Of these, seven patterns (9, 10, 11, 12, 13, 14, and 15) containing 8,179 DEGs were upregulated in stage of lactation “D” compared with “L” (P < 0.05). In contrast, seven patterns (0, 1, 2, 3, 4, 5, and 6) representing 170 DEGs were downregulated in lactation stage “D” (P < 0.05). The other two patterns (7 and 8) representing 47 DEGs had a similar expression level in the “D” and “L” groups. A total of seven patterns (1, 5, 6, 8, 12, 13, and 15) representing 351 DEGs were upregulated in lactation stage “NP” compared with “D” (P < 0.05), whereas, another seven patterns (0, 2, 3, 7, 9, 10, and 14) representing 5,409 DEGs were significantly downregulated in the “NP” group compared with “D” group. The patterns 11 and 4 containing 2,633 DEGs had the similar expression levels between the “D” and “NP” group.
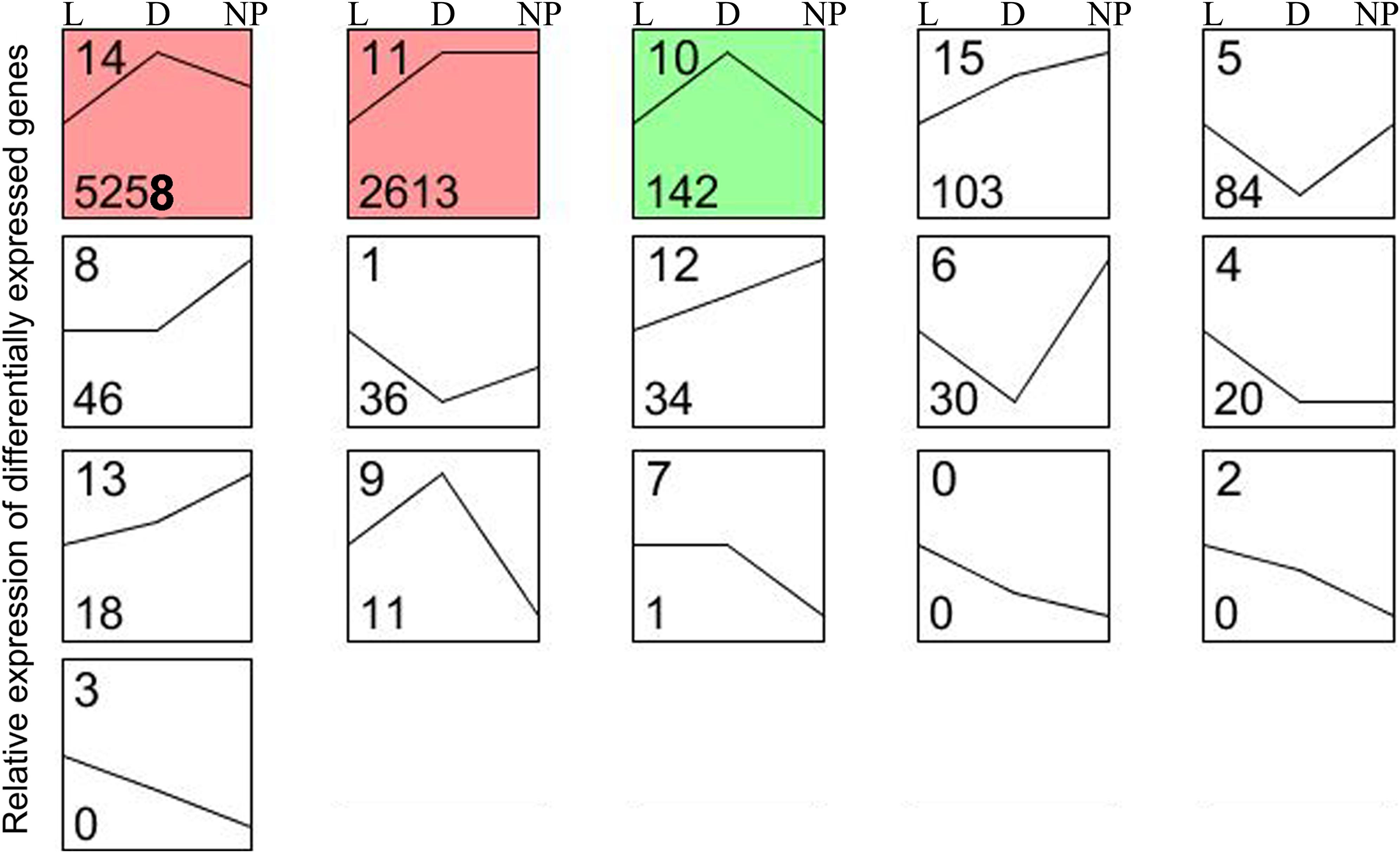
Figure 4. Sixteen patterns encompassing the DEGs during different stages of lactation using STEM software. L, peak lactation period; D, dry-off period; NP, non-lactating/non-pregnant period. Clusters ordered based on number of genes, and profiles ordered by significance. The number in the top left-hand corner of a profile box is the profile ID number, and the number in the bottom left-hand corner of a profile box is the profile gene number. The colored profiles had a statistically significant number of genes assigned. Non-white profiles of the same color represent profiles grouped into a single cluster.
Co-expression analysis revealed that 13 DEGs interacted with 8,028 correlated background genes including POLG, SPTA1, KLC, GIT2, COPS3, PDP, CD31, USP 16/29/37, TLL1, NCAPH, ABI2, DNAJC4, and MAPK8IP3 (Table 2). Specifically, three genes involved in lipid transport and metabolism (PLA2, CPT1, and PLD) had the highest number of correlated genes (Table 3). In addition, threegenes had associations with intracellular trafficking, secretion, and vesicular transport with GGA, SRPRB, AP4S1 having the greatest number of correlated genes (Table 4). The correlated genes for node gene selection are reported in Supplementary File S2E.
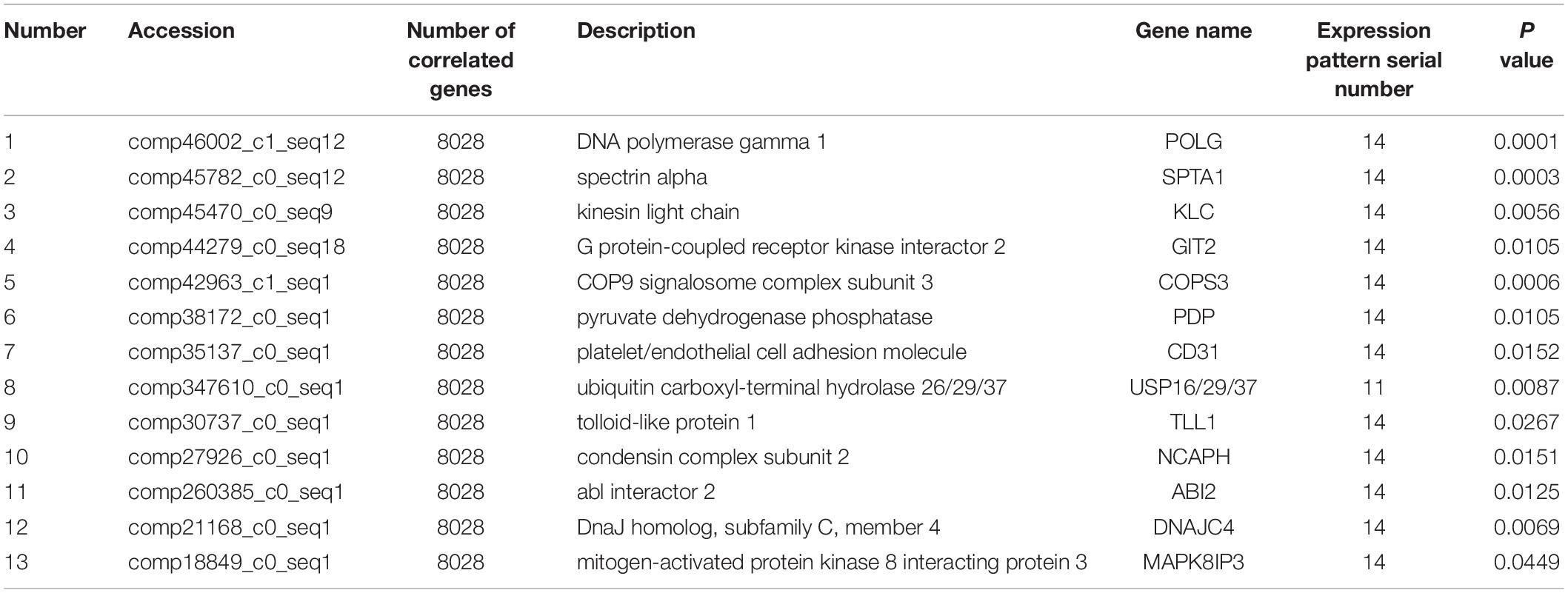
Table 2. Detailed information of 13 novel candidate genes that are putative regulators of lactation.
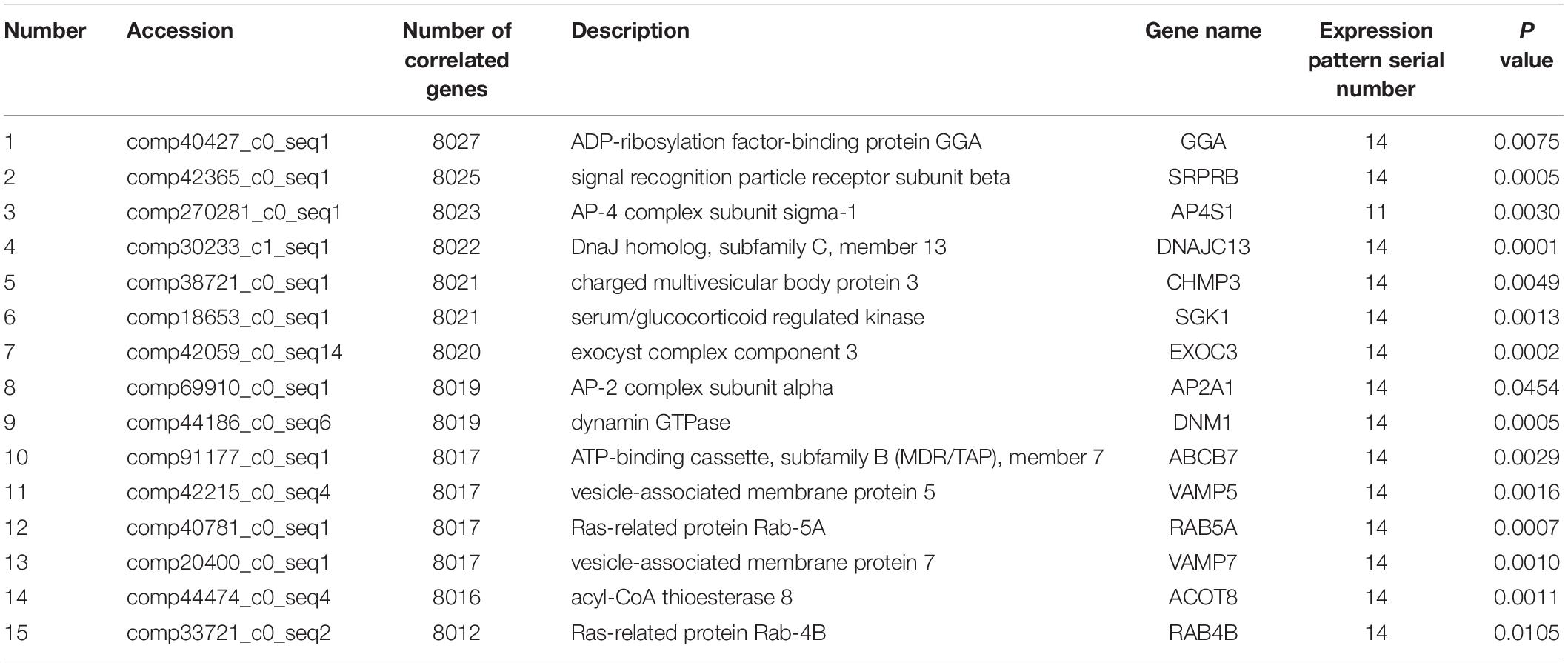
Table 4. Significant node DEGs involved in intracellular trafficking, secretion, and vesicular transport in the KOG database.
DIA Analysis of DEGs
The DIA provides a summary of the KEGG pathways in the form of categories and sub-categories (Figure 5A) that are altered across a given comparison. Details of each pathway are presented in Supplementary File S3. As shown in Figure 5A, KEGG pathway categories were more impacted in stage of lactation “L” versus “NP.” Among these pathways, the category “Metabolism” was the most impacted one, followed by the category “Genetic Information Processing.” With the exception of the subcategory of pathways within “Metabolism of Terpenoids and Polyketides,” all other subcategories within Metabolism had an impact value >50 in the comparison of “L” versus “NP,” with highest impact value (880) in “Biosynthesis of Other Secondary Metabolites.” Except for inhibition of “Glycan Biosynthesis and Metabolism” and “Nucleotide Metabolism,” compared with “NP,” most of the metabolic pathways were markedly activated in the “L” group including “Carbohydrate Metabolism,” “Energy Metabolism,” “Lipid Metabolism,” “Amino Acid Metabolism,” “Metabolism of other Amino Acid,” “Metabolism of Cofactors and Vitamins,” and “Xenobiotics Biodegradation and Metabolism.” According to the impact value, the categories “Environment Information Processing,” “Cellular Process,” “Organismal System” were also altered in the comparison of “L” versus “NP.” However, most of their flux values were slightly activated or did not change in the comparisons of “D” versus “NP” or “L” versus “NP.”
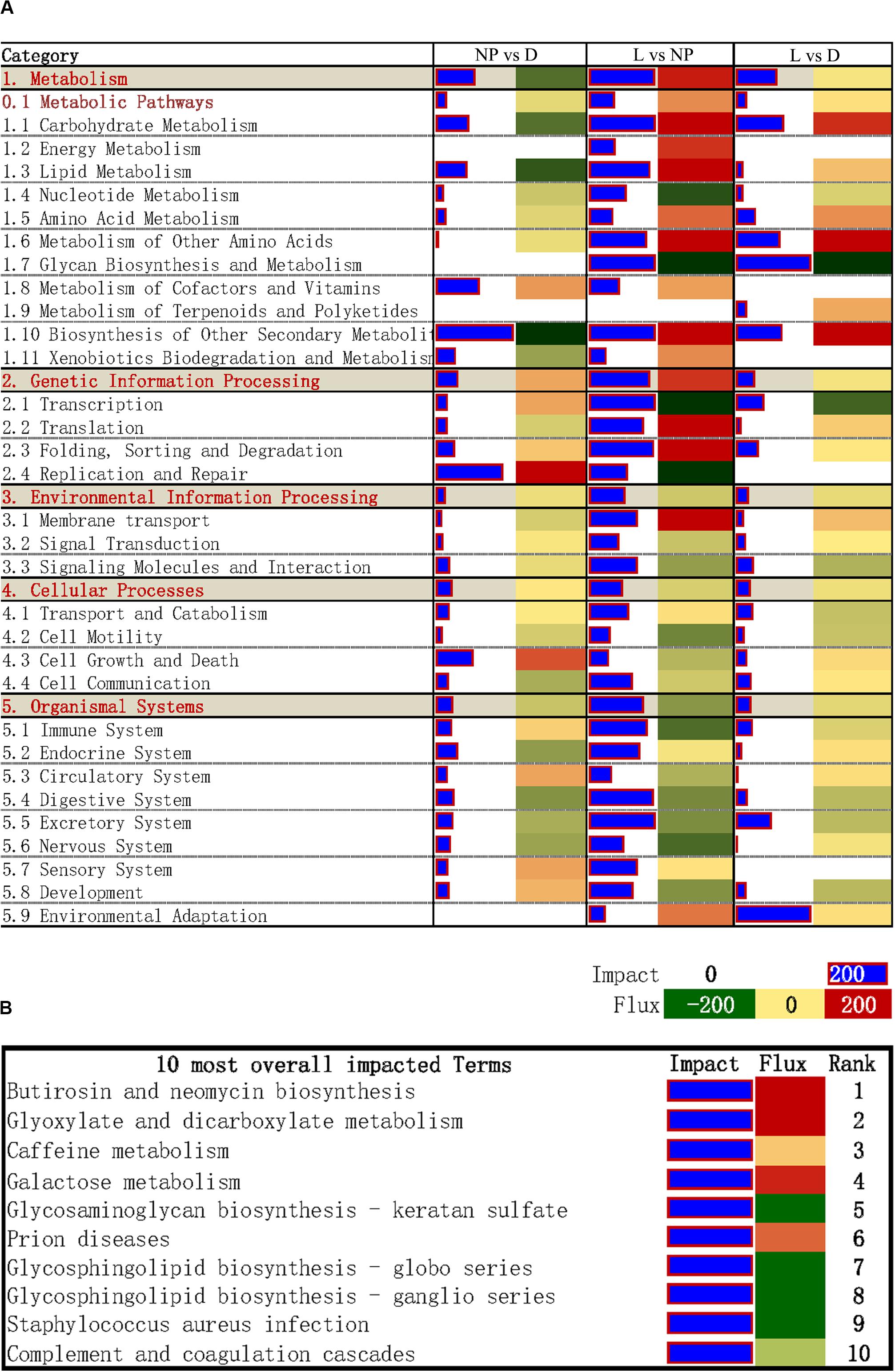
Figure 5. DIA analysis of DEGs during different stages of the lactation cycle. (A) The summary of KEGG pathways provided by DIA. The “impact” is represented by the horizontal blue bars (the larger the bar, the larger the impact) and the “flux” (Direction of the Impact) is represented by green (more inhibited) to red (more activated) rectangles. (B) DIA results for the 10 most impacted KEGG pathways.
The top 10 overall most-impacted terms are shown in Figure 5B. The most impacted pathway was “Butirosin and neomycin biosynthesis” followed by “Glyoxylate and dicarboxylate metabolism” with flux >200 (Figure 5B). The categories “Galactose metabolism” were highly activated. In contrast, the pathways “Glycosaminoglycan biosynthesis-keratan sulfate,” “Glycosphingolipid biosynthesis - globo series,” and “Glycosphingolipid biosynthesis-ganglio series” were inhibited.
Discussion
The development of next-generation sequencing has made it feasible to explore patterns of DEGs in goat mammary glands across stages of lactation. Similar to bovine, this information will help us better understand fatty acid metabolisms and tissue function at the transcriptional level (Bionaz et al., 2012a). One limitation of the current experiment was that mammary gland tissue harvested at the three stages of lactation was not from the same goats, which might overshadow the specific effects associated with lactation. A similar experimental approach for tissue collection and analyses has been utilized in previous work with dairy cows (Li et al., 2016; Cai et al., 2018). However, unlike dairy cows with a large udder mass, repeated mammary biopsies in small ruminants is riskier and prone to inducing cessation of lactogenesis. The fact that peak lactation in the goat occurs at ∼100 days postpartum meant that biopsies collected at dry-off would have taken place roughly 200 days afterward, which was deemed problematic due to the uncertainty about recovery from a biopsy. From a technical standpoint, sampling different animals at the target stages of lactation within the same day increased accuracy of sequencing partly because of the characteristics of temporality and spatiality of mRNA expression.
In the present study, we identified DEGs across three important stages of the lactation period, i.e., established lactation, dry-off, and the non-lactating/non-pregnant period. Data mining revealed key factors involved in the control of mammary gland function, as well as improved our understanding about the role of transcriptional regulation in coordinating lactation. The assembly of 98,864 transcripts was greater than that in macaque (42,702 transcripts) (Gao et al., 2013), bovine (35,945 transcripts) (Shen et al., 2015), and pig (21,331 transcripts) (Zhu et al., 2014). The fact that the latest reference genome was not used in the present study may be a potential reason for the greater number of transcripts detected. However, an advantage of the approach we used is that we could perform a better mapping of the sequences with a more robust unigene annotation. The sequences in this study were assembled according to the goat transcriptome sequences published in our previous study (Shi et al., 2015), i.e., the first study to characterize the complete transcriptome of goat mammary gland, constituting a comprehensive genomic resource available for studies of ruminant lactation. An N50 length is commonly used for assembly evaluation, and a higher number suggests higher-quality assembly (Lander et al., 2001). The N50 length of assembly in our previously published transcriptome sequences (Shi et al., 2015) was higher than other ruminant transcriptome data with a higher overall number of unique transcripts (Wickramasinghe et al., 2011, 2012), suggesting it could provide a comprehensive reference dataset of gene expression profiling for future goat mammary gland research.
It is well known that FASN is a crucial gene for de novo fatty acid synthesis in mammary cells (Zhu et al., 2014). Consistent with our previous qRT-PCR analysis, the expression of FASN in mammary tissue of goats was higher during lactation than dry-off (Zhu et al., 2014). This may partly explain the enrichment of short- and medium-chain fatty acids in goat milk (Morris et al., 2007; Silanikove et al., 2010). However, the up-regulation of FASN in the non-lactating/non-pregnant period, despite the shutting down of lactogenesis within mammary cells, was somewhat unexpected. It is possible that this response was related to an additional need of fatty acids for cell proliferation (Mellenberger et al., 1973) associated with mammary gland development during pregnancy (Faucon et al., 2009), which would be important for the turnover of mammary gland (Norgaard et al., 2008).
Although determining differences between lactating and non-lactating periods has helped elucidate regulatory mechanisms that are controlling lactation (Bionaz and Loor, 2008; Li et al., 2012; Wickramasinghe et al., 2012), it is also important to uncover the DEGs that are specifically induced during pregnancy (Faucon et al., 2009). In the present study, in order to avoid the effects of pregnancy (e.g., endocrine status) on gene expression, we examined expression trends of DEGs among the three periods and detected 20 DEGs with lower and stable expression during the non-lactating and non-pregnant period compared with lactation (Figure 4, pattern 4). Although some milk fat-related genes might be important during pregnancy (Linzell, 1959), most of the genes related to milk fat formation had expression levels that were between those observed in the dry-off (pregnant) and the non-lactating (non-pregnant) periods. More functional research is needed for identifying the role of these genes.
A total of two and six DEGs were determined as uniquely expressed in the dry-off and non-lactating periods. In the non-lactating group, bone morphogenetic protein 3/3B (BMP-3B) is a cytokine that belongs to the transforming growth factor β (TGF-β) superfamily, and plays an important role in the antral nervous system as well as in bone formation and remodeling (Takao et al., 1996). The G protein-coupled receptor 64 (GPR64) is well-known as a member of G protein-coupled receptor superfamily, and is crucial for neuronal development regulation (Richter et al., 2013). Despite putative functions in development, their expression in the non-lactating period was relatively low (RPKM were 0.415 and 0.386, respectively). These results are in close agreement with the extensive remodeling of the mammary gland during the non-lactating period (McDaniel et al., 2006; Safayi et al., 2010).
Among genes with higher expression in the dry-off group, protein Myb-Like, SWIRM and MPN domains 1 (MYSM1) are important for the control of B cell development (Jiang et al., 2011). Myb proto-oncogene protein (MYB) promotes proliferation and inhibits differentiation (Hugo et al., 2013) and also has a key role in regulating stem and progenitor cells in the bone marrow, colonic crypts and a neurogenic region of the adult brain (Ramsay and Gonda, 2008). The gap junction protein beta 2 (GJB2) is important for the development of the auditory system (Bozdogan et al., 2015). All these genes had a relatively higher expression level (the RPKM was 0.958, 1.720, and 2.511, respectively) and most of them are beneficial for embryo development, probably playing a role in mammary cells during dry-off to prepare well in preparation for the impending parturition.
Co-expression analysis is a suitable method for regulatory gene selection (Xu et al., 2013). A node gene correlated with the greatest numbers of genes was considered crucial for the regulation of lactation. Our analysis revealed that POLG, SPTA1, KLC, GIT2, COPS3, PDP, CD31, USP 16/29/37, TLL1, NCAPH, ABI2, DNAJC4, and MAPK8IP3 correlated with most genes. In turn, these genes may play roles in regulating mammary cell function in the lactation stages that were examined in the current study.
Replication of DNA and repair in mitochondria is a crucial function of POLG. SPTA1 is the major protein component of the erythrocyte membrane skeleton (Huebner et al., 1985). KLC is a component of Kinesin heterotetramer and acts in binding motor protein to cargo (Hanks et al., 1988). GIT2 plays an important role in cell attachment, spreading and motility (Pahlich et al., 2006). COPS3 is essential for maintenance of cell proliferation in the mouse embryonic epiblast (Buhr et al., 2008). PDP is important for dephosphorylating pyruvate dehydrogenase in the mammalian pyruvate dehydrogenase complex which catalyzes the conversion of pyruvate to acetyl-CoA, a substrate for de novo fatty acid synthesis (Gonsalvez et al., 2007). CD31 is associated with the vascular compartment and is uniquely-positioned to mediate multiple and important cell-cell interactions involving platelets, leukocytes and endothelial cells (Zheng et al., 2005). USP is essential for maintaining the structure and function of neuromuscular junction (Verbiest et al., 2008). TLL1 is necessary for normal septation and positioning of the heart (Thomassen et al., 2009). NCAPH plays an important in regulating cell cycle activity and proliferation (Hardwick, 2008). ABI2 is involved in abscisic acid signal transduction in Arabidopsis (Dean et al., 2001). DNAJC4 is a sterol-regulatory element binding protein (SREBP)-regulated chaperone involved in the cholesterol biosynthesis pathway. MAPK8IP3 is involved in c-Jun N-terminal kinase (JNK) signaling pathway.
All the above genes have a wide number of functions associated with cellular regulation, consistent with the idea that the process of lactation is regulated and balanced by a complex network of genes (Bionaz and Loor, 2008). Consistent with previous studies, the node genes are central for lipid transport and metabolism along with intracellular trafficking, secretion, and vesicular transport. Specifically, PLA2 (Zhu et al., 2011; Shen et al., 2012; Zhang et al., 2013), CPT1 (Bovia et al., 1995), GGA (Gustavsson et al., 2014), and SRPRB (Hill et al., 1988) had the most number of correlated genes. Although the exact role of these genes in fatty acid metabolism during the various stages of lactation remains to be determined, they may represent candidate genes predicted to be beneficial for our greater understanding of milk fat synthesis.
By combining the proportion of DEGs with the log2 mean fold change and mean -log P-value of genes associated with the biological terms, the DIA is a useful approach to estimate the biological impacts of experimental conditions and the direction of impact (Shi et al., 2017). It has been successfully used for studying goat mammary lipid metabolism in isolated epithelial cells (Lin et al., 2013). In the present study, we determined “Glyoxylate and dicarboxylate metabolism” as one of the most-impacted metabolic pathways during lactation, and was closely associated with the biosynthesis of acetyl-CoA, the substrate for de novo fatty acid synthesis. Thus, the present results underscored alterations of milk lipid synthesis during lactation in goat mammary gland as one of the most-important functional aspects occurring in this organ.
Conclusion
The comprehensive transcriptome profiling of mammary gland across different stages of lactation in Xinong Saanen dairy goat revealed a total of 51,299 unigenes that were annotated to 12,763 genes. The most highly expressed genes were involved in milk fatty acid synthesis and metabolism. Eight out of 9,131 identified DEGs were uniquely expressed in the non-lactating or dry-off periods. We obtained 16 possible expression patterns among DEGs and detected 13 node genes potentially regulating functional adaptations across stages of lactation: POLG, SPTA1, KLC, GIT2, COPS3, PDP, CD31, USP16/29/37, TLL1, NCAPH, ABI2, DNAJC4, and MAPK8IP3. “Butirosin and neomycin biosynthesis” and “Glyoxylate and dicarboxylate metabolism” were the most impacted pathways, underscoring their importance for functional adaptations of the mammary gland. In addition, PLA2, CPT1, PLD, GGA, SRPRB, and AP4S1 were uncovered as node genes and participate in aspects of fatty acid metabolism. Our findings provide promising candidate genes for elucidating molecular mechanisms controlling mammary fatty acid metabolism during lactation in the dairy goat and should be explored further.
Data Availability Statement
The sequencing data have been submitted to the NCBI SRA, and are accessible through the accession number PRJNA637690.
Ethics Statement
The animal study was reviewed and approved by Animal Care and Use Committee of Northwest A&F University.
Author Contributions
JL was responsible for the experimental design. JZ, WZ, HX, HW, and HS collected the tissue samples and isolated RNA for sequencing. JZ and HS performed the analysis of sequencing data and bioinformatics analysis. CL, JZ, HS, JL, and JJL wrote the manuscript. CL, JL, and JJL supervised the entire experiment, participated in result interpretation, and manuscript revision. All authors read and approved the final manuscript.
Funding
This work was jointly supported by National Natural Science Foundation of China (31772575 and 31702098) and the Key Research and Development Plan of Shaanxi Province, China (2018ZDCX-NY-01-05).
Conflict of Interest
The authors declare that the research was conducted in the absence of any commercial or financial relationships that could be construed as a potential conflict of interest.
Acknowledgments
We thank Prof. Cal (Northwest A&F University) for surgical sampling of mammary gland tissue, the goat farm crew for taking care of the animals, and researchers of the Dairy Goat Lab of Northwest A&F University for their useful discussion.
Supplementary Material
The Supplementary Material for this article can be found online at: https://www.frontiersin.org/articles/10.3389/fgene.2020.00878/full#supplementary-material
FIGURE S1 | Analytical flowchart for digital gene expression sequencing in mammary gland of dairy goat. Nine mammary gland samples were collected in the stage of lactation, dry-off and non-lactation with three biological replicates per stage.
TABLE S1 | The 15 most abundant genes involved in lipid transport and metabolism category (KOG database) in mammary gland.
TABLE S2 | The 15 most abundant genes involved in intracellular trafficking, secretion, and vesicular transport category (KOG database) in mammary gland.
TABLE S3 | The 15 most abundant genes involved in fatty acid metabolic process category (GO database) in mammary gland.
TABLE S4 | The 15 most abundant genes involved in fatty acid biosynthetic process category (GO database) in mammary gland.
TABLE S5 | Most abundant genes involved in fatty acid metabolism signaling pathway in mammary gland (KEGG database).
TABLE S6 | Most abundant genes involved in fatty acid biosynthesis signaling pathway in mammary gland (KEGG database).
TABLE S7 | DEGs involved in fatty acid metabolism among different stages of lactation (GO and KEGG databases).
TABLE S8 | Most abundant DEGs involved in lipid metabolism among different stages of lactation (GO and KEGG databases).
TABLE S9 | DEGs involved in protein metabolism among different stages of lactation (GO and KEGG databases).
TABLE S10 | DEGs involved in lactose metabolism among different stages of lactation (GO and KEGG databases).
FILE S2 | (A) List of all 51,299 defined unigenes. (B) List of all 9,131 differentially expressed genes (DEG). (C) List of all 246 enriched KEGG pathways. (D) List of specific genes from all 16 expression patterns through series-cluster analysis. (E) List of all DEGs for node genes selection through co-expression analysis.
FILE S3 | Dynamic Impact Approach (DIA) analysis of differentially expressed genes during different stages of the lactation cycle. (A) General information during the process of DIA analysis using DEGs. (B) DEG data of the three comparison groups used in the DIA analysis. (C) Flux and impact generated by the DIA analysis. (D) Summary graph generated from the DIA analysis. (E) Overall pathway rank generated by the DIA analysis. (F) Pathway rank in each comparison generated by the DIA analysis.
Abbreviations
ABCF2, ATP-binding cassette sub-family F member 2; ABDH2, acylglycerol lipase; ABI2, abl interactor 2; ACAA, acetyl-CoA acyltransferase; ACAA2, acetyl-CoA acyltransferase 2; ACADL, very long chain acyl-CoA dehydrogenase; ACADM, acyl-CoA dehydrogenase; ACADS, butyryl-CoA dehydrogenase; ACADSB, short/branched chain acyl-CoA dehydrogenase; ACAT1, acetyl-CoA C-acetyltransferase; ACBP, acyl-CoA-binding protein; ACOX1, acyl-CoA oxidase; ACSL, long-chain acyl-CoA synthetase; ADH, alcohol dehydrogenase; ADH1, alcohol dehydrogenase; ALDH, aldehyde dehydrogenase (NAD+); ALDH1, aldehyde dehydrogenase (NAD+); AP2M1, AP-2 complex subunit mu-1; AP4S1, AP-4 complex subunit sigma-1; ARF1, ADP-ribosylation factor 1; ARL5A, ADP-ribosylation factor-like 5A; B4GALT5, Beta-1,4-galactosyltransferase 5; BMP-3B, bone morphogenetic protein 3/3B; CD31, platelet/endothelial cell adhesion molecule; CHMP2A, charged multivesicular body protein 2A; CLTA, clathrin, light polypeptide A; COPS3, COP9 signalosome complex subunit 3; CPT1, choline-phosphate cytidylyltransferase; CPT1B, carnitine O-palmitoyltransferase 2; CPTA1, carnitine O-palmitoyltransferase 1; DEGs, differentially expressed genes; DEGS1, sphingolipid delta-4 desaturase; DGE, digital gene expression profiling; DIA, dynamic impact approach; DNAJC4, DnaJ homolog subfamily C member 4; EBP, cholestenol delta-isomerase; EHHADH, enoyl-CoA hydratase/3-hydroxyacyl-CoA dehydrogenase; EIF3S5, translation initiation factor eIF-3 subunit 5; ELOVL1, elongation of very long chain fatty acids protein 1; EPHA1, Eph receptor A1; FABP4, fatty acid-binding protein 4; FASN, fatty acid synthase; GCDH, glutaryl-CoA dehydrogenase; GGA, ADP-ribosylation factor-binding protein; GIT2, G protein-coupled receptor kinase interactor 2; GO, Gene Ontology; GPAT3, glycerol-3-phosphate O-acyltransferase 1/2; GPR64, G protein-coupled receptor 64; HLCS, acetyl-CoA carboxylase/biotin carboxylase; IGF1, insulin-like growth factor 1; IGFR2, Fc receptor IgG low affinity IIa; JNK, c-JunN-terminal kinase. KEGG, Kyoto Encyclopedia of Genes and Genomes; KLC, kinesin light chain; KOG, clusters of euKaryotic Orthologous Groups; LEF1, lymphoid enhancer-binding factor 1; lepB, signal peptidase I; MAPK8IP3, mitogen-activated protein kinase 8 interacting protein 3; MARCH2, E3 ubiquitin-protein ligase MARCH2; MARK, MAP/microtubule affinity-regulating kinase; MCAT, [acyl-carrier-protein] S-malonyltransferase; MDH2, malate dehydrogenase (NADP+); MSMO1, methylsterol monooxygenase; MYB, Myb proto-oncogene protein;GJB2, gap junction protein beta 2; MYH, myosin heavy chain; MYSM1, protein Myb Like, SWIRM and MPN domains 1; NCAPH, condensin complex subunit 2; NDUFAB1, NADH dehydrogenase (ubiquinone) 1 alpha/beta subcomplex 1; NR1F1, nuclear receptor subfamily 1, group F member 1; OXSM, 3-oxoacyl-[acyl-carrier-protein] synthase II; PDP, pyruvate dehydrogenase phosphatase; PLA2, phospholipase A2; PLD, phospholipase D; PNK, polynucleotide kinase; POLG, DNA polymerase gamma 1; PPKAG1, 5’-AMP-activated protein kinase, regulatory gamma subunit; RAB11B, Ras-related protein Rab-11B; RAB1A, Ras-related protein Rab-1A; RNPEPL1, arginyl aminopeptidase-like 1; RUNX1T1, runt-related transcription factor 1 translocated to 1; SAPOSIN, saposin; SC5D, lathosterol oxidase; SCD, stearoyl-CoA desaturase; SDHD, succinate dehydrogenase (ubiquinone) membrane anchor subunit; SEC61, protein transport protein SEC61 subunit alpha; SEC61G, protein transport protein SEC61 subunit gamma and related proteins; SEMA6, semaphorin 6; SERPINE1, plasminogen activator inhibitor-1; SPAT1, spectrin alpha; SREBP, sterol-regulatory element binding proteins; SRPRB, signal recognition particle receptor subunit beta; SSR4, signal sequence receptor subunit 4; STAM, signal transducing adaptor molecule; STAT5A, signal transducer and activator of transcription 5A; STEM, Short Time-series Expression Miner; STK11, serine/threonine-protein kinase 11; TECR, enoyl reductase; TGF- β, growth factor β; TLL1, tolloid-like protein 1; USP16/29/37, ubiquitin carboxyl-terminal hydrolase 26/29/37.
Footnotes
References
Akers, R. M. (2017). A 100-year review: mammary development and lactation. J. Dairy Sci. 100, 10332–10352. doi: 10.3168/jds.2017-12983
Andres, A. C., and Djonov, V. (2010). The mammary gland vasculature revisited. J. Mamm. Gland Biol. Neoplasia 15, 319–328. doi: 10.1007/s10911-010-9186-9
Bionaz, M., and Loor, J. (2008). Gene networks driving bovine milk fat synthesis during the lactation cycle. BMC Genomics 9:366. doi: 10.1186/1471-2164-9-366
Bionaz, M., Periasamy, K., Rodriguez-Zas, S. L., Everts, R. E., Lewin, H. A., Hurley, W. L., et al. (2012a). Old and new stories: revelations from functional analysis of the bovine mammary transcriptome during the lactation cycle. PLoS One 7:e33268. doi: 10.1371/journal.pone.0033268
Bionaz, M., Periasamy, K., Rodriguez-Zas, S. L., Hurley, W. L., and Loor, J. J. (2012b). A novel dynamic impact approach (DIA) for functional analysis of time-course omics studies: validation using the bovine mammary transcriptome. PLoS One 7:e32455. doi: 10.1371/journal.pone.0032455
Bovia, F., Fornallaz, M., Leffers, H., and Strub, K. (1995). The SRP9/14 subunit of the signal recognition particle (SRP) is present in more than 20-fold excess over SRP in primate cells and exists primarily free but also in complex with small cytoplasmic Alu RNAs. Mol. Biol. Cell 6, 471–484. doi: 10.1091/mbc.6.4.471
Bozdogan, S. T., Kuran, G., Yuregir, O. O., Aslan, H., Haytoglu, S., Ayaz, A., et al. (2015). The prevalence of gap junction protein beta 2 (GJB2) mutations in non syndromic sensorineural hearing loss in cukurova region. J. Int. Adv. Otol. 11, 118–121. doi: 10.5152/iao.2015.1212
Buhr, N., Carapito, C., Schaeffer, C., Kieffer, E., Van Dorsselaer, A., and Viville, S. (2008). Nuclear proteome analysis of undifferentiated mouse embryonic stem and germ cells. Electrophoresis 29, 2381–2390. doi: 10.1002/elps.200700738
Cai, W., Li, C., Liu, S., Zhou, C., Yin, H., Song, J., et al. (2018). Genome wide identification of novel long Non-coding RNAs and their potential associations with milk proteins in chinese holstein cows. Front. Genet. 9:281. doi: 10.3389/fgene.2018.00281
Canovas, A., Rincon, G., Bevilacqua, C., Islas-Trejo, A., Brenaut, P., Hovey, R. C., et al. (2014). Comparison of five different RNA sources to examine the lactating bovine mammary gland transcriptome using RNA-sequencing. Sci. Rep. 4:5297. doi: 10.1038/srep05297
Casado, B., Affolter, M., and Kussmann, M. (2009). OMICS-rooted studies of milk proteins, oligosaccharides and lipids. J. Proteom. 73, 196–208. doi: 10.1016/j.jprot.2009.09.018
Clarke, R., Ressom, H. W., Wang, A., Xuan, J., Liu, M. C., Gehan, E. A., et al. (2008). The properties of high-dimensional data spaces: implications for exploring gene and protein expression data. Nat. Rev. Cancer 8, 37–49. doi: 10.1038/nrc2294
Dean, M., Hamon, Y., and Chimini, G. (2001). The human ATP-binding cassette (ABC) transporter superfamily. J. Lipid Res. 42, 1007–1017.
Ernst, J., and Bar-Joseph, Z. (2006). STEM: a tool for the analysis of short time series gene expression data. BMC Bioinformatics 7:191. doi: 10.1186/1471-2105-7-191
Faucon, F., Rebours, E., Bevilacqua, C., Helbling, J. C., Aubert, J., Makhzami, S., et al. (2009). Terminal differentiation of goat mammary tissue during pregnancy requires the expression of genes involved in immune functions. Physiol. Genom. 40, 61–82. doi: 10.1152/physiolgenomics.00032.2009
Gao, Y., Lin, X., Shi, K., Yan, Z., and Wang, Z. (2013). Bovine mammary gene expression profiling during the onset of lactation. PLoS One 8:e70393. doi: 10.1371/journal.pone.0070393
Georges, M., Charlier, C., and Hayes, B. (2019). Harnessing genomic information for livestock improvement. Nat. Rev. Genet. 20, 135–156. doi: 10.1038/s41576-018-0082-2
German, J. B., Dillard, C. J., and Ward, R. E. (2002). Bioactive components in milk. Curr. Opin. Clin. Nutr. Metab. Care 5, 653–658. doi: 10.1097/01.mco.0000038808.16540.7f
Goddard, M. E., and Hayes, B. J. (2009). Mapping genes for complex traits in domestic animals and their use in breeding programmes. Nat. Rev. Genet. 10, 381–391. doi: 10.1038/nrg2575
Gonsalvez, G. B., Tian, L., Ospina, J. K., Boisvert, F.-M., Lamond, A. I., and Matera, A. G. (2007). Two distinct arginine methyltransferases are required for biogenesis of Sm-class ribonucleoproteins. J. Cell Biol. 178, 733–740. doi: 10.1083/jcb.200702147
Gustavsson, E. K., Joanne Trinh, B., Guell, I., Vilari~no-Guell, C., Appel-Cresswell, S., Stoessl, A. J., et al. (2014). DNAJC13 genetic variants in parkinsonism. Mov. Disord. 30, 273–278.
Haas, B. J., Papanicolaou, A., Yassour, M., Grabherr, M., Blood, P. D., Bowden, J., et al. (2013). De novo transcript sequence reconstruction from RNA-seq using the Trinity platform for reference generation and analysis. Nat. Protoc. 8, 1494–1512. doi: 10.1038/nprot.2013.084
Hanks, S., Quinn, A., and Hunter, T. (1988). The protein kinase family: conserved features and deduced phylogeny of the catalytic domains. Science 241, 42–52. doi: 10.1126/science.3291115
Hardwick, J. P. (2008). Cytochrome P450 omega hydroxylase (CYP4) function in fatty acid metabolism and metabolic diseases. Biochem. Pharmacol. 75, 2263–2275. doi: 10.1016/j.bcp.2008.03.004
Hill, B. L., Drickamer, K., Brodsky, F. M., and Parham, P. (1988). Identification of the phosphorylation sites of clathrin light chain LCb. J. Biol. Chem. 263, 5499–5501.
Huebner, K., Palumbo, A. P., Isobe, M., Kozakt, C. A., Monaco, S., Rovera, G., et al. (1985). The alpha-spectrin gene is on chromosome 1 in mouse and man. Proc. Natl. Acad. Sci. U.S.A. 82, 3790–3793. doi: 10.1073/pnas.82.11.3790
Hugo, H. J., Pereira, L., Suryadinata, R., Drabsch, Y., Gonda, T. J., Gunasinghe, N. P., et al. (2013). Direct repression of MYB by ZEB1 suppresses proliferation and epithelial gene expression during epithelial-to-mesenchymal transition of breast cancer cells. Breast Cancer Res. 15:R113. doi: 10.1186/bcr3580
Jiang, X. X., Nguyen, Q., Chou, Y., Wang, T., Nandakumar, V., Yates, P., et al. (2011). Control of B cell development by the histone H2A deubiquitinase MYSM1. Immunity 35, 883–896. doi: 10.1016/j.immuni.2011.11.010
Lander, E. S., Linton, L. M., Birren, B., Nusbaum, C., Zody, M. C., Baldwin, J., et al. (2001). Initial sequencing and analysis of the human genome. Nature 409, 860–921. doi: 10.1038/35057062
Li, C., Cai, W., Zhou, C., Yin, H., Zhang, Z., Loor, J. J., et al. (2016). RNA-Seq reveals 10 novel promising candidate genes affecting milk protein concentration in the Chinese Holstein population. Sci. Rep. 6:26813. doi: 10.1038/srep26813
Li, C., Wang, M., Zhang, T., He, Q., Shi, H., Luo, J., et al. (2019). Insulin-induced gene 1 and 2 isoforms synergistically regulate triacylglycerol accumulation, lipid droplet formation, and lipogenic gene expression in goat mammary epithelial cells. J. Dairy Sci. 102, 1736–1746. doi: 10.3168/jds.2018-15492
Li, Z., Liu, H., Jin, X., Lo, L., and Liu, J. (2012). Expression profiles of microRNAs from lactating and non-lactating bovine mammary glands and identification of miRNA related to lactation. BMC Genomics 13:731. doi: 10.1186/1471-2164-13-731
Lin, X. Z., Luo, J., Zhang, L. P., Wang, W., Shi, H. B., and Zhu, J. J. (2013). MiR-27a suppresses triglyceride accumulation and affects gene mRNA expression associated with fat metabolism in dairy goat mammary gland epithelial cells. Gene 521, 15–23. doi: 10.1016/j.gene.2013.03.050
Linzell, J. L. (1959). Physiology of the mammary glands. Physiol. Rev. 39, 534–576. doi: 10.1152/physrev.1959.39.3.534
Lohse, M., Bolger, A. M., Nagel, A., Fernie, A. R., Lunn, J. E., Stitt, M., et al. (2012). RobiNA: a user-friendly, integrated software solution for RNA-Seq-based transcriptomics. Nucleic Acids Res. 40, W622–W627. doi: 10.1093/nar/gks540
Mardis, E. R. (2008). The impact of next-generation sequencing technology on genetics. Trends Genet. 24, 133–141. doi: 10.1016/j.tig.2007.12.007
McDaniel, S. M., Rumer, K. K., Biroc, S. L., Metz, R. P., Singh, M., Porter, W., et al. (2006). Remodeling of the mammary microenvironment after lactation promotes breast tumor cell metastasis. Am. J. Pathol. 168, 608–620. doi: 10.2353/ajpath.2006.050677
Mellenberger, R. W., Bauman, D. E., and Nelson, D. R. (1973). Metabolic adaptations during lactogenesis. Fatty acid and lactose synthesis in cow mammary tissue. Biochem. J. 136, 741–748. doi: 10.1042/bj1360741
Metzker, M. L. (2010). Applications of next-generation sequencing sequencing technologies - the next generation. Nat. Rev. Genet. 11, 31–46. doi: 10.1038/nrg2626
Morris, C. A., Cullen, N. G., Glass, B. C., Hyndman, D. L., Manley, T. R., Hickey, S. M., et al. (2007). Fatty acid synthase effects on bovine adipose fat and milk fat. Mamm. Genome 18, 64–74. doi: 10.1007/s00335-006-0102-y
Norgaard, J. V., Theil, P. K., Sorensen, M. T., and Sejrsen, K. (2008). Cellular mechanisms in regulating mammary cell turnover during lactation and dry period in dairy cows. J. Dairy Sci. 91, 2319–2327. doi: 10.3168/jds.2007-0767
Ogata, H., Goto, S., Sato, K., Fujibuchi, W., Bono, H., and Kanehisa, M. (1999). KEGG: kyoto encyclopedia of genes and genomes. Nucleic Acids Res. 27, 29–34.
Pahlich, S., Zakaryan, R. P., and Gehring, H. (2006). Protein arginine methylation, Cellular functions and methods of analysis. Biochim. Biophys. Acta 1764, 1980–1983.
Prieto, C., Risueno, A., Fontanillo, C., and De las Rivas, J. (2008). Human gene coexpression landscape: confident network derived from tissue transcriptomic profiles. PLoS One 3:e3911. doi: 10.1371/journal.pone.0003911
Pujana, M. A., Han, J. D., Starita, L. M., Stevens, K. N., Tewari, M., Ahn, J. S., et al. (2007). Network modeling links breast cancer susceptibility and centrosome dysfunction. Nat. Genet. 39, 1338–1349. doi: 10.1038/ng.2007.2
Ramsay, R. G., and Gonda, T. J. (2008). MYB function in normal and cancer cells. Nat. Rev. Cancer 8, 523–534. doi: 10.1038/nrc2439
Richter, G. H. S., Fasan, A., Hauer, K., Grunewald, T. G. P., Berns, C., Rössler, S., et al. (2013). G-Protein coupled receptor 64 promotes invasiveness and metastasis in Ewing sarcomas through PGF and MMP1. J. Pathol. 230, 70–81. doi: 10.1002/path.4170
Rudolph, M. C., McManaman, J. L., Hunter, L., Phang, T., and Neville, M. C. (2003). Functional development of the mammary gland: use of expression profiling and trajectory clustering to reveal changes in gene expression during pregnancy, lactation, and involution. J. Mammary Gland Biol. Neoplasia 8, 287–307. doi: 10.1023/b:jomg.0000010030.73983.57
Rudolph, M. C., Neville, M. C., and Anderson, S. M. (2007). Lipid synthesis in lactation: diet and the fatty acid switch. J. Mammary Gland Biol. Neoplasia 12, 269–281. doi: 10.1007/s10911-007-9061-5
Safayi, S., Theil, P. K., Hou, L., Engbaek, M., Norgaard, J. V., Sejrsen, K., et al. (2010). Continuous lactation effects on mammary remodeling during late gestation and lactation in dairy goats. J. Dairy Sci. 93, 203–217. doi: 10.3168/jds.2009-2507
Shen, L., Luo, J., Du, J., Liu, C., Wu, X., Pu, Q., et al. (2015). Transcriptome analysis of liangshan pig muscle development at the growth curve inflection point and asymptotic stages using digital gene expression profiling. PLoS One 10:e0135978. doi: 10.1371/journal.pone.0135978
Shen, Y. H., Song, G. X., Liu, Y. Q., Sun, W., Zhou, L. J., Liu, H. L., et al. (2012). Silencing of FABP3 promotes apoptosis and induces mitochondrion impairment in embryonic carcinoma cells. J. Bioenerg. Biomembr. 44, 317–323. doi: 10.1007/s10863-012-9439-y
Shennan, D. B., and Peaker, M. (2000). Transport of milk constituents by the mammary gland. Physiol. Rev. 80, 925–951. doi: 10.1152/physrev.2000.80.3.925
Shi, H., Wang, L., Luo, J., Liu, J., Loor, J. J., and Liu, H. (2019). Fatty acid elongase 7 (ELOVL7) plays a role in the synthesis of long-chain unsaturated fatty acids in goat mammary epithelial cells. Animals 9:389. doi: 10.3390/ani9060389
Shi, H., Zhu, J., Luo, J., Cao, W., Shi, H., Yao, D., et al. (2015). Genes regulating lipid and protein metabolism are highly expressed in mammary gland of lactating dairy goats. Funct. Integr. Genomics 15, 309–321. doi: 10.1007/s10142-014-0420-1
Shi, H. B., Zhang, C. H., Zhao, W., Luo, J., and Loor, J. J. (2017). Peroxisome proliferator-activated receptor delta facilitates lipid secretion and catabolism of fatty acids in dairy goat mammary epithelial cells. J. Dairy Sci. 100, 797–806. doi: 10.3168/jds.2016-11647
Silanikove, N., Leitner, G., Merin, U., and Prosser, C. G. (2010). Recent advances in exploiting goat’s milk: Quality, safety and production aspects. Small Ruminant Res. 89, 110–124. doi: 10.1016/j.smallrumres.2009.12.033
t Hoen, P. A., Ariyurek, Y., Thygesen, H. H., Vreugdenhil, E., Vossen, R. H., de Menezes, R. X., et al. (2008). Deep sequencing-based expression analysis shows major advances in robustness, resolution and inter-lab portability over five microarray platforms. Nucleic Acids Res. 36:e141. doi: 10.1093/nar/gkn705
Takao, M., Hino, J., Takeshita, N., Konno, Y., Nishizawa, T., Matsuo, H., et al. (1996). Identification of rat bone morphogenetic protein-3b (BMP-3b), a new member of BMP-3. Biochem. Biophys. Res. Commun. 219, 656–662. doi: 10.1006/bbrc.1996.0289
Thomassen, M., Tan, Q., and Kruse, T. (2009). Gene expression meta-analysis identifies chromosomal regions and candidate genes involved in breast cancer metastasis. Breast Cancer Res. Treat. 113, 239–249. doi: 10.1007/s10549-008-9927-2
Verbiest, V., Montaudon, D., Tautu, M. T., Moukarzel, J., Portail, J. P., Markovits, J., et al. (2008). Protein arginine (N)-methyltransferase 7 (PRMT7) as a potential target for the sensitization of tumor cells to camptothecins. FEBS Lett. 582, 1483–1489. doi: 10.1016/j.febslet.2008.03.031
Wickramasinghe, S., Hua, S., Rincon, G., Islas-Trejo, A., German, J. B., Lebrilla, C. B., et al. (2011). Transcriptome profiling of bovine milk oligosaccharide metabolism genes using RNA-sequencing. PLoS One 6:e18895. doi: 10.1371/journal.pone.0018895
Wickramasinghe, S., Rincon, G., Islas-Trejo, A., and Medrano, J. F. (2012). Transcriptional profiling of bovine milk using RNA sequencing. BMC Genomics 13:45. doi: 10.1186/1471-2164-13-45
Wright, G. W., and Simon, R. M. (2003). A random variance model for detection of differential gene expression in small microarray experiments. Bioinformatics 19, 2448–2455. doi: 10.1093/bioinformatics/btg345
Xu, H. F., Luo, J., Zhao, W. S., Yang, Y. C., Tian, H. B., Shi, H. B., et al. (2016). Overexpression of SREBP1 (sterol regulatory element binding protein 1) promotes de novo fatty acid synthesis and triacylglycerol accumulation in goat mammary epithelial cells. J. Dairy Sci. 99, 783–795. doi: 10.3168/jds.2015-9736
Xu, W. W., Han, M. J., Chen, D., Chen, L., Guo, Y., Willden, A., et al. (2013). Genome-wide search for the genes accountable for the induced resistance to HIV-1 infection in activated CD4+ T cells: apparent transcriptional signatures, co-expression networks and possible cellular processes. BMC Med. Genomics 6:1–30. doi: 10.1186/1755-8794-6-15
Yang, H., Crawford, N., Lukes, L., Finney, R., Lancaster, M., and Hunter, K. W. (2005). Metastasis predictive signature profiles pre-exist in normal tissues. Clin. Exp. Metastasis 22, 593–603. doi: 10.1007/s10585-005-6244-6
Yao, D., Luo, J., He, Q., Shi, H., Li, J., Wang, H., et al. (2017). SCD1 alters long-chain fatty acid (LCFA) composition and its expression is directly regulated by SREBP-1 and PPARgamma 1 in dairy goat mammary cells. J. Cell. Physiol. 232, 635–649. doi: 10.1002/jcp.25469
Zhang, Y., Kent, J. W., Lee, A., Cerjak, D., Ali, O., Diasio, R., et al. (2013). Fatty acid binding protein 3 (fabp3) is associated with insulin, lipids and cardiovascular phenotypes of the metabolic syndrome through epigenetic modifications in a northern european family population. BMC Med. Genomics 6:9. doi: 10.1186/1755-8794-6-9
Zheng, Z., Schmidt-Ott, K. M., Chua, S., Foster, K. A., Frankel, R. Z., Pavlidis, P., et al. (2005). A Mendelian locus on chromosome 16 determines susceptibility to doxorubicin nephropathy in the mouse. Proc. Natl. Acad. Sci. U.S.A. 102, 2502–2507. doi: 10.1073/pnas.0409786102
Zhu, C., Hu, D. L., Liu, Y. Q., Zhang, Q. J., Chen, F. K., Kong, X. Q., et al. (2011). Fabp3 inhibits proliferation and promotes apoptosis of embryonic myocardial cells. Cell Biochem. Biophys. 60, 259–266. doi: 10.1007/s12013-010-9148-2
Keywords: dairy goat, digital gene expression sequencing, lactation, mammary gland, fatty acid metabolism
Citation: Li C, Zhu J, Shi H, Luo J, Zhao W, Shi H, Xu H, Wang H and Loor JJ (2020) Comprehensive Transcriptome Profiling of Dairy Goat Mammary Gland Identifies Genes and Networks Crucial for Lactation and Fatty Acid Metabolism. Front. Genet. 11:878. doi: 10.3389/fgene.2020.00878
Received: 23 March 2020; Accepted: 17 July 2020;
Published: 25 September 2020.
Edited by:
Peter Dovc, University of Ljubljana, SloveniaReviewed by:
Eveline M. Ibeagha-Awemu, Agriculture and Agri-Food Canada, CanadaYachun Wang, China Agricultural University, China
Copyright © 2020 Li, Zhu, Shi, Luo, Zhao, Shi, Xu, Wang and Loor. This is an open-access article distributed under the terms of the Creative Commons Attribution License (CC BY). The use, distribution or reproduction in other forums is permitted, provided the original author(s) and the copyright owner(s) are credited and that the original publication in this journal is cited, in accordance with accepted academic practice. No use, distribution or reproduction is permitted which does not comply with these terms.
*Correspondence: Jun Luo, luojun@nwafu.edu.cn; Juan J. Loor, jloor@illinois.edu
†These authors have contributed equally to this work