- Respiratory Hospital of Angang General Hospital, Anshan, China
Evidence from clinical and epidemiological studies indicates that asthma is associated with allergic diseases including hay fever, allergic rhinitis, and eczema. Genetic analysis demonstrated that asthma had a positive genetic correlation with allergic diseases. A Mendelian randomization (MR) analysis using the rs16969968 single-nucleotide variant as the instrumental variable indicated that smoking was associated with increased risk of asthma. However, in a different MR analysis, smoking was significantly associated with reduced hay fever and reduced allergic sensitization risk. These findings revealed inconsistencies in the association of smoking with asthma and allergic diseases. Hence, we conducted an updated MR analysis to investigate the causal association between lifetime smoking and asthma risk by using 124 genetic variants as the instrumental variables. No significant pleiotropy was detected using the MR–Egger intercept test. We found that increased lifetime smoking was significantly associated with decreased asthma risk by using the inverse variance weighted (IVW) method (OR = 0.97, 95% CI 0.956–0.986, and P = 1.77E-04), the weighted median regression method (OR = 0.976, 95% CI 0.96–0.994, and P = 8.00E-03), and the MR–Egger method (OR = 0.919, 95% CI 0.847–0.998, and P = 4.5E-02). Importantly, MR pleiotropy residual sum and outlier (MR-PRESSO) MR analysis also indicated a significant association between increased lifetime smoking and decreased asthma risk with OR = 0.971, 95% CI 0.956–0.986, and P = 2.69E-04. After the outlier was removed, MR-PRESSO outlier test further supported the significant association with OR = 0.971, 95% CI 0.959–0.984, P = 1.57E-05.
Introduction
Evidence from clinical and epidemiological studies indicates that asthma is associated with allergic diseases including hay fever, allergic rhinitis, and eczema (Zhu et al., 2018). In 2018, Zhu et al. (2018) used the asthma and allergic disease genome-wide association study (GWAS) datasets including 33,593 cases and 76,768 controls of European ancestry from the UK Biobank and conducted a genetic correlation analysis using the linkage disequilibrium score regression (LDSC) method. They found that asthma had a positive genetic correlation with allergic diseases, which indicated shared genetic etiology (Zhu et al., 2018). In 2017, Ferreira et al. conducted a GWAS analysis of 360,838 samples with kinds of allergic disease phenotypes (Ferreira et al., 2017). They demonstrated that asthma, hay fever, and eczema share many common genetic variants (Ferreira et al., 2017). Importantly, these risk variants could regulate and dysregulate the expression of immune-related genes (Ferreira et al., 2017).
Many observational studies have been conducted to evaluate the association between smoking and asthma risk, but these studies have produced inconsistent findings for this association (Skaaby et al., 2017). Mendelian randomization (MR) analysis methods have been used widely to infer causal associations (Liu et al., 2018; Luo et al., 2019; Zhao and Schooling, 2019; Zhao et al., 2019; Zhuang et al., 2019a, b). Skaaby et al. (2017) used the rs16969968 and rs1051730 single-nucleotide variants, which were in linkage disequilibrium with each other, to genotype 231,020 individuals by observational and MR analyses. The observational analysis revealed similar asthma risk in current smokers and never smokers, and the MR analysis showed that current smokers had higher risk of asthma than never smokers (Skaaby et al., 2017).
Other MR studies using rs16969968 as an instrumental variable for smoking heaviness have reported its association with other human habits, such as coffee consumption (Bjorngaard et al., 2017) and alcohol use (Taylor et al., 2018), and factors such as depression and anxiety (Taylor et al., 2014), and blood pressure and resting heart rate (Linneberg et al., 2015). MR studies that use a single genetic variant as the instrumental variable have some limitations (Rosa et al., 2019). Therefore, an MR analysis using multiple significant and independent genetic variants as potential instrumental variables may increase the statistical power and precision, as was found in some recent studies (Relton and Davey Smith, 2015; Davies et al., 2018; Rosa et al., 2019).
Wootton et al. (2019) performed a GWAS of lifetime smoking behavior (including smoking duration, heaviness, and cessation) by analyzing a total of 462,690 samples from the UK Biobank. They identified 124 genetic variants associated with lifetime smoking that met a genome-wide level of significance of P < 5 × 10–8 (Wootton et al., 2019). Zhu et al. (2018) conducted a GWAS of asthma with 14,085 asthma subjects and 76,768 controls of European ancestry. We used these lifetime smoking and asthma GWAS datasets to perform an updated MR analysis to evaluate the causal association between lifetime smoking and asthma risk.
Materials and Methods
Lifetime Smoking Genome-Wide Association Study Dataset
The lifetime smoking GWAS dataset from the UK Biobank included 462,690 samples from individuals of European ancestry (Wootton et al., 2019). The genetic variant genotype dataset met the genotype quality control criteria (Wootton et al., 2019). The 462,690 samples were from 138,807 smokers and 323,883 controls. Among the 138,807 samples from smokers, 8% were from current smokers and 22% were from former smokers (Wootton et al., 2019). A lifetime smoking measure was used to evaluate smoking initiation, smoking heaviness, and smoking duration (Wootton et al., 2019). We selected the 124 genetic variants associated with lifetime smoking reported by Wootton et al. that met the genome-wide level of significance as the potential instrumental variables (Table 1; Wootton et al., 2019).
Asthma Genome-Wide Association Study Dataset
The asthma GWAS dataset from the UK Biobank included 487,409 participants. Before the GWAS analysis, the data were filtered by excluding non-white individuals, excluding individuals with missing genotype information, limiting to doctor-diagnosed asthma cases, and excluding individuals without complete phenotype or covariate information (Zhu et al., 2018). Finally, a total of 14,085 asthma participants and 76,768 controls were selected for the GWAS analysis (Zhu et al., 2018). The detailed information about the age and sex characteristics is provided in Table 2, as described in the original study (Zhu et al., 2018).
Pleiotropy Analysis
We conducted a pleiotropy analysis to assure that all the selected 124 genetic variants associated with lifetime smoking met the MR assumptions, as has been done in previous studies (Cheng et al., 2018, 2019; Liu et al., 2018; Luo et al., 2019; Zhao and Schooling, 2019; Zhao et al., 2019). Here, we selected two different methods. First, we used the MR–Egger intercept test to detect the presence of potential pleiotropy, and more detailed information about MR–Egger intercept test has been provided in previous studies (Cheng et al., 2018, 2019; Liu et al., 2018). Meanwhile, we selected MR pleiotropy residual sum and outlier (MR-PRESSO) test to perform the pleiotropy analysis (Verbanck et al., 2018). There are three different tests in MR-PRESSO. MR-PRESSO global test could detect horizontal pleiotropy (Verbanck et al., 2018). The significance level for the pleiotropy analysis was set as P < 0.05.
Mendelian Randomization Analysis
For the MR analysis, we used the inverse variance weighted (IVW) meta-analysis; and for the sensitivity analysis, we used both the weighted median regression method and MR–Egger method, as has been done in previous studies (Liu et al., 2018; Luo et al., 2019; Zhao and Schooling, 2019; Zhao et al., 2019). Even if half of the 124 genetic variants did not meet the MR assumptions, the weighted median regression method can still produce a consistent estimate of the causal association (Emdin et al., 2017). If there is significant pleiotropy, the MR–Egger method can adjust the pleiotropy and produce a corrected estimate of the causal association (Bowden et al., 2015, 2016; Dale et al., 2017; Tillmann et al., 2017; Yavorska and Burgess, 2017). Meanwhile, MR-PRESSO method was also selected as the sensitivity analysis. MR-PRESSO outlier test could correct for horizontal pleiotropy via outlier removal. MR-PRESSO distortion test could evaluate the differences in the causal estimates before and after correction for outliers (Verbanck et al., 2018). All the statistical analyses were conducted using R (v3.2.4) and R package “MendelianRandomization” and MR-PRESSO (Cheng et al., 2018, 2019; Liu et al., 2018). The significance threshold for the MR analysis was set as P < 0.05. Finally, a leave-one-out permutation analysis was used to evaluate the influence of each instrumental variable on the MR estimate (Liu et al., 2018).
Results
Genome-Wide Association Study Summary Statistics
Of the 124 genetic variants from the lifetime smoking GWAS dataset, only 117 were included in the asthma GWAS dataset. The missing variants were rs2867112, rs3896224, rs11768481, rs35169606, rs13016665, rs62098013, and rs35343344. Summary statistics of the 117 genetic variants in the lifetime smoking and asthma GWAS datasets are provided in Table 1. These 117 genetic variants were used in the subsequent analysis.
Pleiotropy Analysis
The pleiotropy analysis using the MR–Egger intercept test showed that all 117 genetic variants had no significant pleiotropy (intercept = 0.001, P = 0.187). Hence, evidence from MR–Egger intercept test supported that all 117 genetic variants could be taken as the potential instrumental variables. However, MR-PRESSO global test indicated significant horizontal pleiotropy P = 0.003. Hence, we further correct for the horizontal pleiotropy via outlier removal using MR-PRESSO outlier test.
Mendelian Randomization Analysis
Using all 117 genetic variants, we found that increased lifetime smoking was significantly associated with decreased asthma risk using both the IVW method (OR = 0.971, 95% CI 0.956–0.986, and P = 1.77E-04) and the weighted median regression method (OR = 0.976, 95% CI 0.96–0.994, and P = 8.00E-03). The MR–Egger method also showed that lifetime smoking reduced the risk of asthma (OR = 0.919, 95% CI 0.847–0.998, P = 4.5E-02). Importantly, all three methods produced consistent results. Figure 1 shows the individual causal estimates from each of the 117 genetic variants using the different methods.
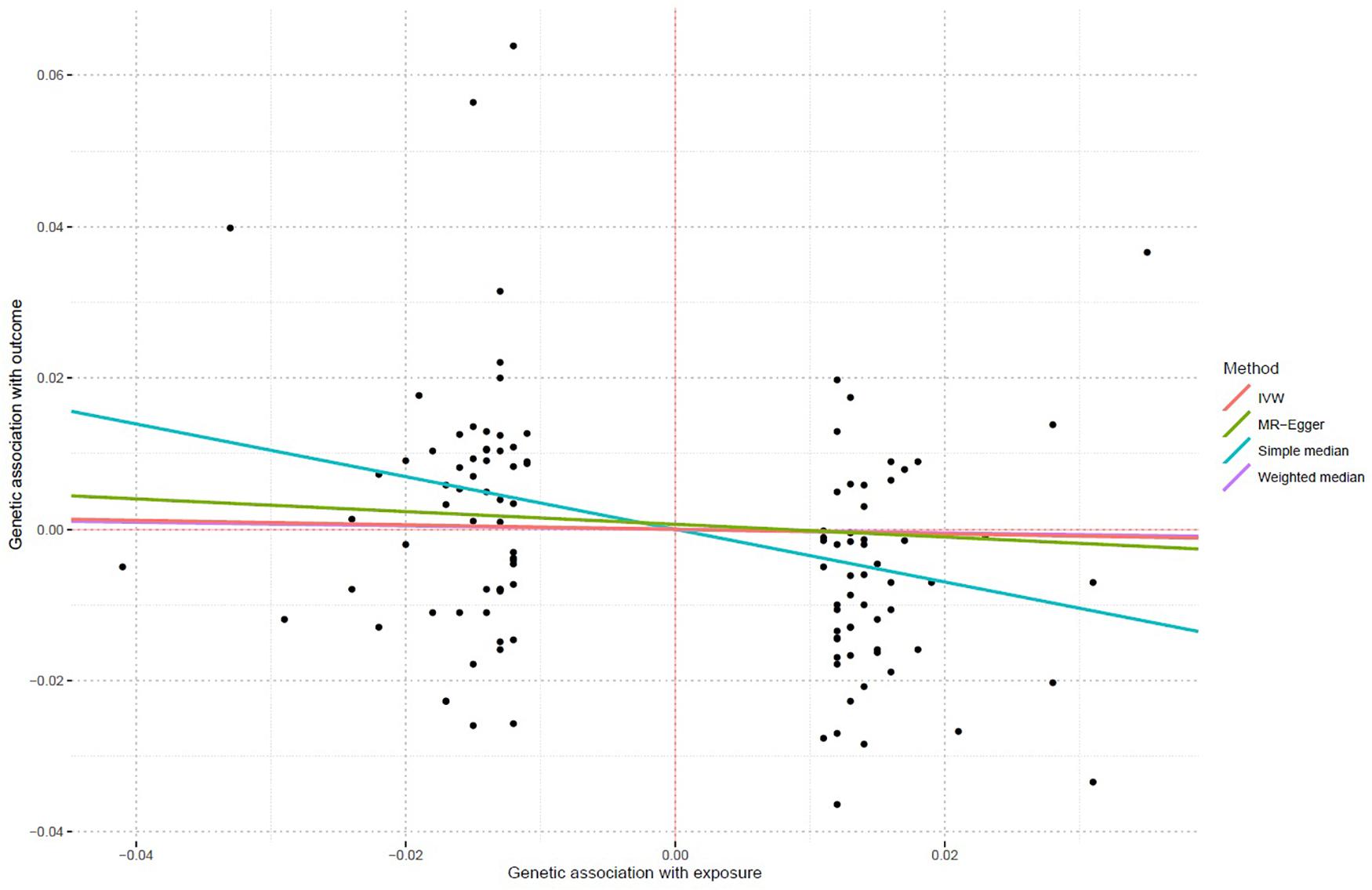
Figure 1. Individual causal estimates from each of 117 genetic variants using different methods. This scatter plot shows individual causal estimates from each of 117 genetic variants associated with lifetime smoking on the x-axis and asthma risk on the y-axis. The continuous line represents the causal estimate of lifetime smoking on asthma risk.
With the use of all 117 genetic variants, MR-PRESSO MR analysis also indicated a significant association between increased lifetime smoking and decreased asthma risk with OR = 0.971, 95% CI 0.956–0.986, and P = 2.69E-04. After the outlier was removed, MR-PRESSO outlier test further supported the significant association with OR = 0.971, 95% CI 0.959–0.984, and P = 1.57E-05. Importantly, there is no significant difference in the causal estimates before and after correction for outliers (P = 0.925).
The leave-one-out permutation showed that only one single genetic variant rs1050847 could significantly affect the estimates between increased lifetime smoking and decreased asthma risk. Figures 2–4 show the influence of each instrumental variable on the MR estimate using IVW method, weighted median regression method, and MR–Egger method, respectively.
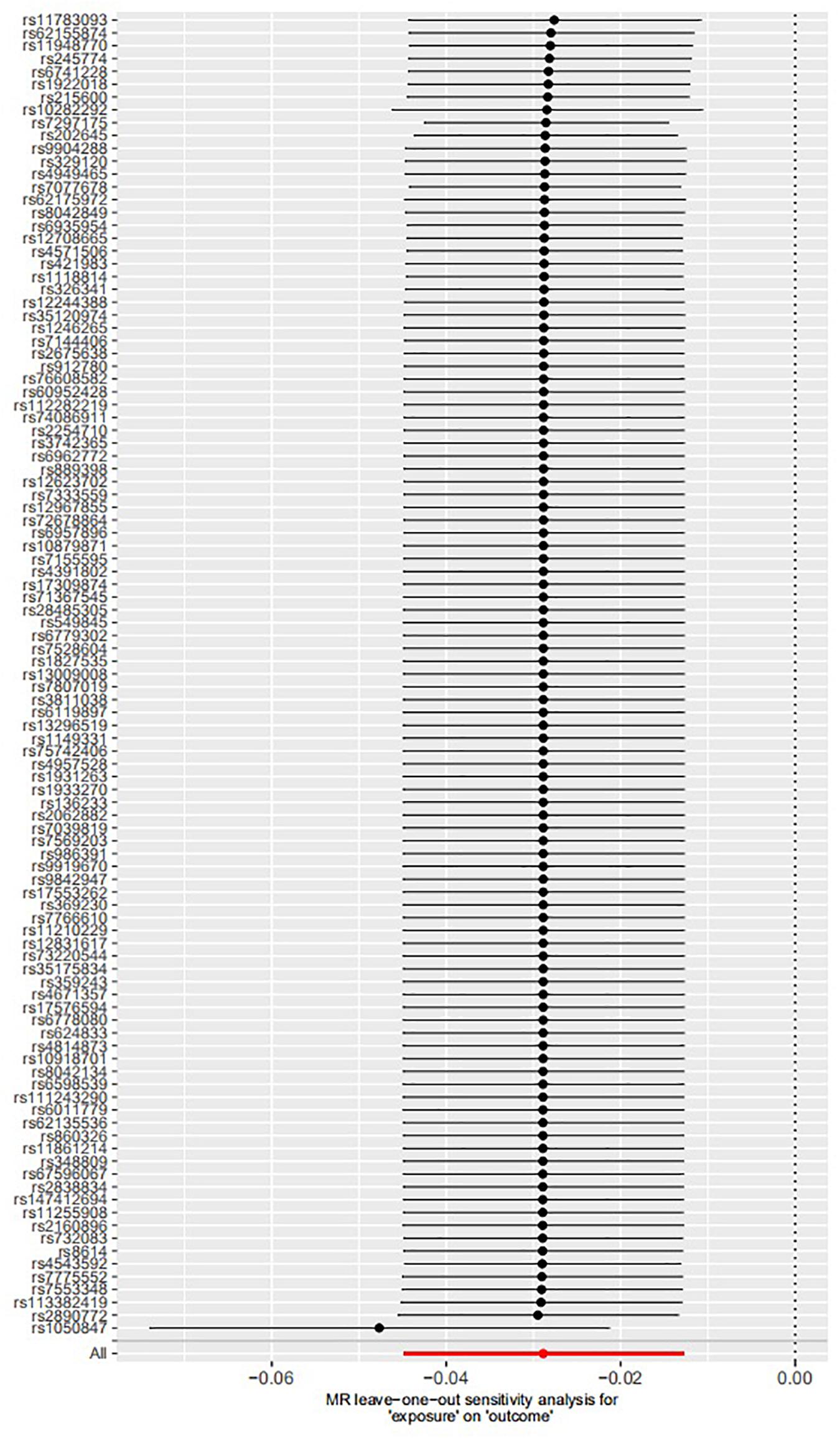
Figure 2. Influence of each instrumental variable on the Mendelian randomization (MR) estimate using inverse variance weighted (IVW) method. Only one single genetic variant rs1050847 could significantly affect the estimates between increased lifetime smoking and decreased asthma risk using IVW method.
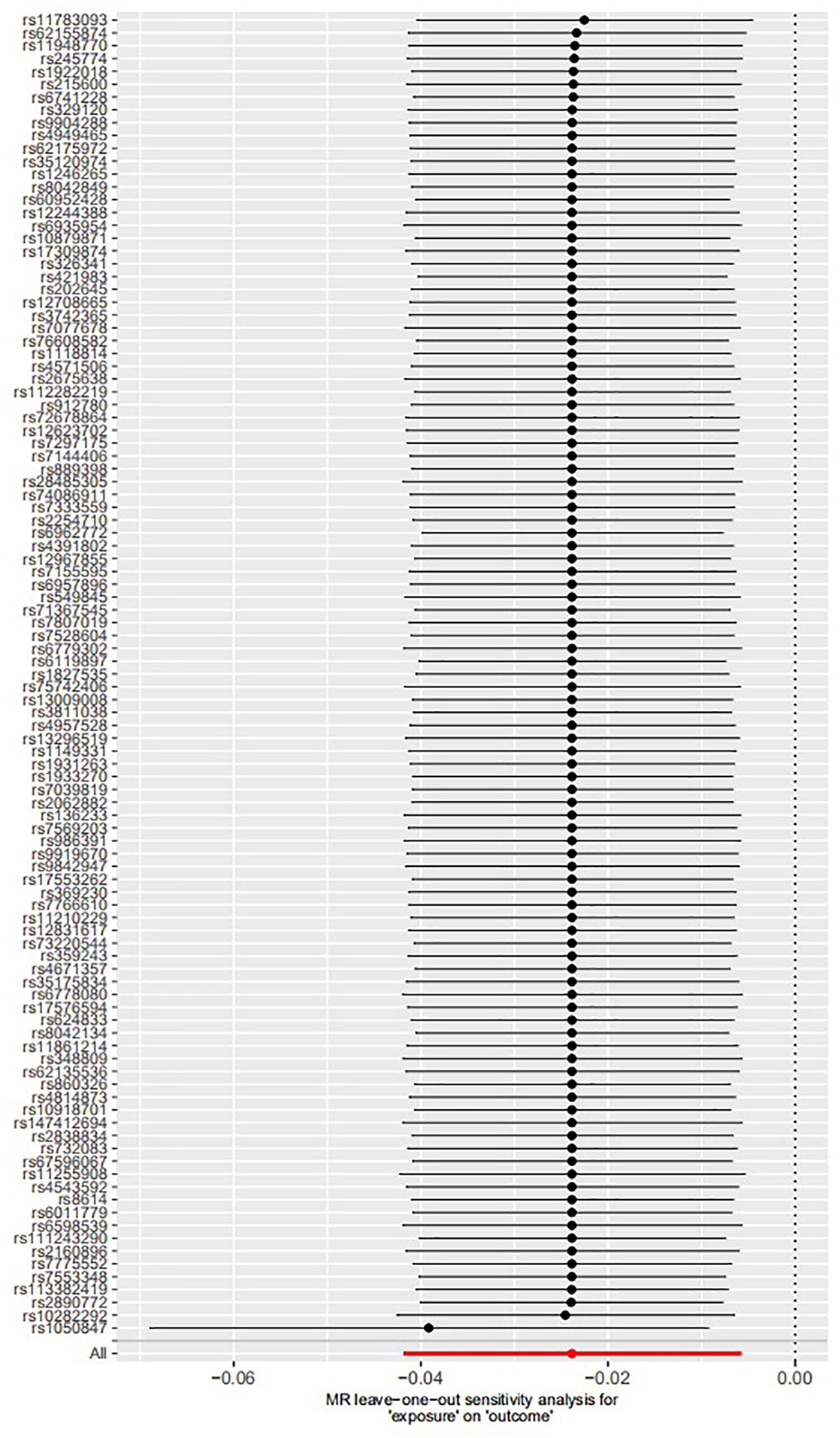
Figure 3. Influence of each instrumental variable on the Mendelian randomization (MR) estimate using weighted median regression method. Only one single genetic variant rs1050847 could significantly affect the estimates between increased lifetime smoking and decreased asthma risk using weighted median regression method.
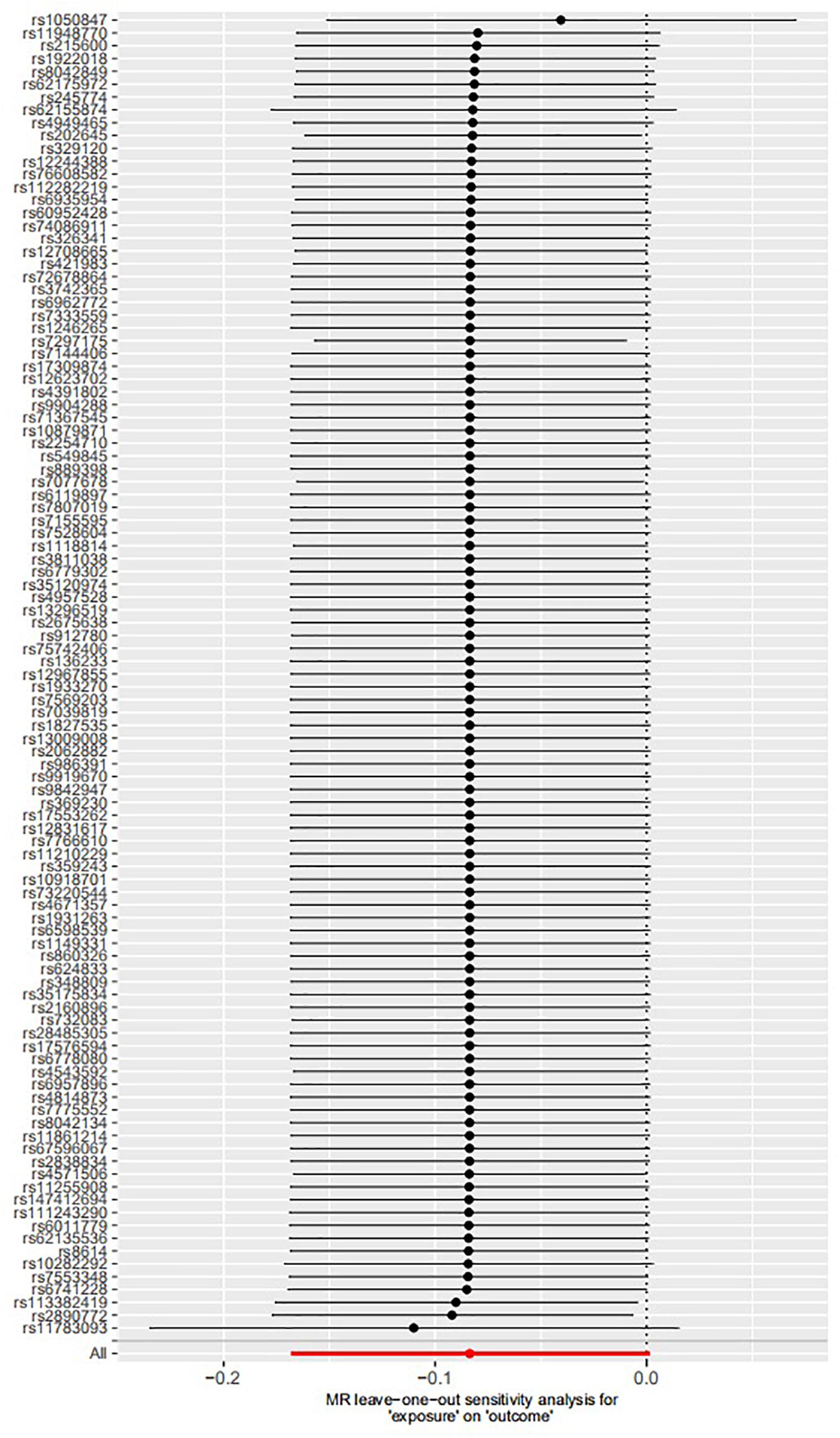
Figure 4. Influence of each instrumental variable on the Mendelian randomization (MR) estimate using MR–Egger method. Only one single genetic variant rs1050847 could significantly affect the estimates between increased lifetime smoking and decreased asthma risk using MR–Egger method.
Discussion
Epidemiological studies indicate that asthma and allergic disease co-occur and share a similar and very close course especially in the children (Ober and Yao, 2011; Guibas et al., 2017; Schoettler et al., 2019). It is reported that allergic asthma is most common in kinds of diseases in childhood (Cookson and Moffatt, 2000). It is known that both asthma and allergic disease are complex human diseases, which are caused by both genetic and environmental contributions (Ober and Yao, 2011; Guibas et al., 2017).
Until recently, large-scale GWAS and meta-analyses of GWAS have been conducted and identified common genetic variant, risk genes, or pathways that contribute to both diseases (Ferreira et al., 2017; Zhu et al., 2018). In a recent GWAS, Ferreira et al. analyzed 360,838 samples and demonstrated common genetic variants to be shared by asthma, hay fever, and eczema (Ferreira et al., 2017). Importantly, these risk variants could regulate and dysregulate the expression of immune-related genes (Ferreira et al., 2017). In 2018, Zhu et al. (2018) analyzed 33,593 cases and 76,768 controls and identified a positive genetic correlation between asthma and allergic diseases.
However, Skaaby et al. (2017) found that smoking was significantly associated with increased risk of asthma, reduced hay fever, and reduced allergic sensitization risk by an MR analysis. These findings are inconsistent, and currently, no consensus exists. In their MR analysis, Skaaby et al. (2017) selected only one single genetic variant rs16969968 as the instrumental variable. It is reported that MR studies that use a single genetic variant as the instrumental variable have some limitations (Relton and Davey Smith, 2015; Davies et al., 2018; Rosa et al., 2019). Hence, an MR analysis using multiple significant and independent genetic variants as potential instrumental variables may increase the statistical power and precision (Relton and Davey Smith, 2015; Davies et al., 2018; Rosa et al., 2019).
Here, we conducted an updated MR analysis to investigate the causal association between lifetime smoking and asthma risk using the 124 genetic variants from the lifetime smoking GWAS dataset as the instrumental variables (Wootton et al., 2019). We first performed a pleiotropy analysis using the MR–Egger intercept test, and we found no significant pleiotropy. We further conducted a pleiotropy analysis using MR-PRESSO global test, and we found significant pleiotropy (Verbanck et al., 2018). In the MR analysis, we first selected three methods including IVW, weighted median regression, and MR–Egger methods, and we clearly demonstrated that increased lifetime smoking was significantly associated with decreased asthma risk. We further conducted an MR analysis using MR-PRESSO method and verified the significant association between increased lifetime smoking and decreased asthma risk. MR-PRESSO outlier test further supported the significant association after removing the outlier. Importantly, no significant difference was observed in the causal estimates before and after correction for outliers. Finally, the leave-one-out permutation showed that only one single genetic variant rs1050847 could significantly affect the estimates between increased lifetime smoking and decreased asthma risk.
Our results are consistent with the results from a large-scale population-based international cohort study by Cerveri et al. (2012) who analyzed 9,092 non-asthma and 1,045 asthma samples. They found that the proportion (26%) of smokers in the asthma group was significantly less than the proportion (31%) of smokers in the non-asthma group (P < 0.001; Cerveri et al., 2012). In the asthma group, smoking increased the mean asthma score (Cerveri et al., 2012). A study using mouse mast cells showed that cigarette smoke prevented allergies by decreasing the reaction of immune cells to allergens (Mortaz et al., 2009).
Data Availability Statement
All datasets presented in this study are included in the article/supplementary material.
Author Contributions
HZ designed the project. MS analyzed the data. All authors contributed to the first draft of the manuscript and read and approved the final manuscript.
Conflict of Interest
The authors declare that the research was conducted in the absence of any commercial or financial relationships that could be construed as a potential conflict of interest.
Acknowledgments
We thank the UK Biobank for providing the lifetime smoking and asthma GWAS datasets.
References
Bjorngaard, J. H., Nordestgaard, A. T., Taylor, A. E., Treur, J. L., Gabrielsen, M. E., Munafo, M. R., et al. (2017). Heavier smoking increases coffee consumption: findings from a Mendelian randomization analysis. Int. J. Epidemiol. 46, 1958–1967. doi: 10.1093/ije/dyx147
Bowden, J., Davey Smith, G., and Burgess, S. (2015). Mendelian randomization with invalid instruments: effect estimation and bias detection through Egger regression. Int. J. Epidemiol. 44, 512–525. doi: 10.1093/ije/dyv080
Bowden, J., Davey Smith, G., Haycock, P. C., and Burgess, S. (2016). Consistent estimation in mendelian randomization with some invalid instruments using a weighted median estimator. Genet. Epidemiol. 40, 304–314. doi: 10.1002/gepi.21965
Cerveri, I., Cazzoletti, L., Corsico, A. G., Marcon, A., Niniano, R., Grosso, A., et al. (2012). The impact of cigarette smoking on asthma: a population-based international cohort study. Int. Arch. Allergy Immunol. 158, 175–183. doi: 10.1159/000330900
Cheng, L., Zhuang, H., Ju, H., Yang, S., Han, J., Tan, R., et al. (2019). Exposing the causal effect of body mass index on the risk of type 2 diabetes mellitus: a mendelian randomization study. Front. Genet. 10:94.
Cheng, L., Zhuang, H., Yang, S., Jiang, H., Wang, S., and Zhang, J. (2018). Exposing the causal effect of C-reactive protein on the risk of type 2 diabetes mellitus: a mendelian randomization study. Front. Genet. 9:657.
Cookson, W. O., and Moffatt, M. F. (2000). Genetics of asthma and allergic disease. Hum. Mol. Genet. 9, 2359–2364. doi: 10.1093/hmg/9.16.2359
Dale, C. E., Fatemifar, G., Palmer, T. M., White, J., Prieto-Merino, D., Zabaneh, D., et al. (2017). Causal associations of adiposity and body fat distribution with coronary heart disease, stroke subtypes, and type 2 diabetes mellitus: a mendelian randomization analysis. Circulation 135, 2373–2388. doi: 10.1161/circulationaha.116.026560
Davies, N. M., Holmes, M. V., and Davey Smith, G. (2018). Reading Mendelian randomisation studies: a guide, glossary, and checklist for clinicians. BMJ 362:k601. doi: 10.1136/bmj.k601
Emdin, C. A., Khera, A. V., Natarajan, P., Klarin, D., Zekavat, S. M., Hsiao, A. J., et al. (2017). Genetic association of waist-to-hip ratio with cardiometabolic traits, type 2 diabetes, and coronary heart disease. JAMA 317, 626–634.
Ferreira, M. A., Vonk, J. M., Baurecht, H., Marenholz, I., Tian, C., Hoffman, J. D., et al. (2017). Shared genetic origin of asthma, hay fever and eczema elucidates allergic disease biology. Nat. Genet. 49, 1752–1757.
Guibas, G. V., Mathioudakis, A. G., Tsoumani, M., and Tsabouri, S. (2017). Relationship of allergy with asthma: there are more than the allergy “eggs” in the asthma “basket”. Front. Pediatr. 5:92.
Linneberg, A., Jacobsen, R. K., Skaaby, T., Taylor, A. E., Fluharty, M. E., Jeppesen, J. L., et al. (2015). Effect of smoking on blood pressure and resting heart rate: a mendelian randomization meta-analysis in the CARTA consortium. Circ. Cardiovasc. Genet. 8, 832–841.
Liu, G., Zhao, Y., Jin, S., Hu, Y., Wang, T., Tian, R., et al. (2018). Circulating vitamin E levels and Alzheimer’s disease: a mendelian randomization study. Neurobiol. Aging 72, 189.e1–189.e9. doi: 10.1016/j.neurobiolaging.2018.08.008
Luo, S., Au Yeung, S. L., Zhao, J. V., Burgess, S., and Schooling, C. M. (2019). Association of genetically predicted testosterone with thromboembolism, heart failure, and myocardial infarction: mendelian randomisation study in UK Biobank. BMJ 364, l476. doi: 10.1136/bmj.l476
Mortaz, E., Folkerts, G., Engels, F., Nijkamp, F. P., and Redegeld, F. A. (2009). Cigarette smoke suppresses in vitro allergic activation of mouse mast cells. Clin. Exp. Allergy 39, 679–687. doi: 10.1111/j.1365-2222.2009.03209.x
Ober, C., and Yao, T. C. (2011). The genetics of asthma and allergic disease: a 21st century perspective. Immunol. Rev. 242, 10–30. doi: 10.1111/j.1600-065x.2011.01029.x
Relton, C. L., and Davey Smith, G. (2015). Mendelian randomization: applications and limitations in epigenetic studies. Epigenomics 7, 1239–1243. doi: 10.2217/epi.15.88
Rosa, M., Chignon, A., Li, Z., Boulanger, M. C., Arsenault, B. J., Bosse, Y., et al. (2019). A Mendelian randomization study of IL6 signaling in cardiovascular diseases, immune-related disorders and longevity. NPJ Genom. Med. 4:23.
Schoettler, N., Rodriguez, E., Weidinger, S., and Ober, C. (2019). Advances in asthma and allergic disease genetics: is bigger always better? J. Allergy Clin. Immunol. 144, 1495–1506. doi: 10.1016/j.jaci.2019.10.023
Skaaby, T., Taylor, A. E., Jacobsen, R. K., Paternoster, L., Thuesen, B. H., Ahluwalia, T. S., et al. (2017). Investigating the causal effect of smoking on hay fever and asthma: a Mendelian randomization meta-analysis in the CARTA consortium. Sci. Rep. 7:2224.
Taylor, A. E., Fluharty, M. E., Bjorngaard, J. H., Gabrielsen, M. E., Skorpen, F., Marioni, R. E., et al. (2014). Investigating the possible causal association of smoking with depression and anxiety using Mendelian randomisation meta-analysis: the CARTA consortium. BMJ Open 4:e006141.
Taylor, M., Rode, L., Bjorngaard, J., Taylor, A. E., Bojesen, S. E., Asvold, B. O., et al. (2018). Is smoking heaviness causally associated with alcohol use? A mendelian randomization study in four european cohorts. Int. J. Epidemiol. 47, 1098–1105. doi: 10.1093/ije/dyy027
Tillmann, T., Vaucher, J., Okbay, A., Pikhart, H., Peasey, A., Kubinova, R., et al. (2017). Education and coronary heart disease: mendelian randomisation study. BMJ 358:j3542. doi: 10.1136/bmj.j3542
Verbanck, M., Chen, C. Y., Neale, B., and Do, R. (2018). Detection of widespread horizontal pleiotropy in causal relationships inferred from Mendelian randomization between complex traits and diseases. Nat. Genet. 50, 693–698. doi: 10.1038/s41588-018-0099-7
Wootton, R. E., Richmond, R. C., Stuijfzand, B. G., Lawn, R. B., Sallis, H. M., Taylor, G. M. J., et al. (2019). Evidence for causal effects of lifetime smoking on risk for depression and schizophrenia: a Mendelian randomisation study. Psychol. Med. [Epub ahead of print].
Yavorska, O. O., and Burgess, S. (2017). MendelianRandomization: an R package for performing Mendelian randomization analyses using summarized data. Int. J. Epidemiol. 46, 1734–1739. doi: 10.1093/ije/dyx034
Zhao, J. V., Kwok, M. K., and Schooling, C. M. (2019). Effect of glutamate and aspartate on ischemic heart disease, blood pressure, and diabetes: a Mendelian randomization study. Am. J. Clin. Nutr. 109, 1197–1206. doi: 10.1093/ajcn/nqy362
Zhao, J. V., and Schooling, C. M. (2019). Effect of linoleic acid on ischemic heart disease and its risk factors: a Mendelian randomization study. BMC Med. 17:61. doi: 10.1186/s12916-019-1293-x
Zhu, Z., Lee, P. H., Chaffin, M. D., Chung, W., Loh, P. R., Lu, Q., et al. (2018). A genome-wide cross-trait analysis from UK Biobank highlights the shared genetic architecture of asthma and allergic diseases. Nat. Genet. 50, 857–864. doi: 10.1038/s41588-018-0121-0
Zhuang, H., Han, J., Cheng, L., and Liu, S. L. (2019a). A positive causal influence of IL-18 levels on the risk of T2DM: a mendelian randomization study. Front. Genet. 10:295.
Keywords: asthma, allergic diseases, genome-wide association study, Mendelian randomization, inverse-variance weighted meta-analysis
Citation: Shen M, Liu X, Li G, Li Z and Zhou H (2020) Lifetime Smoking and Asthma: A Mendelian Randomization Study. Front. Genet. 11:769. doi: 10.3389/fgene.2020.00769
Received: 12 May 2020; Accepted: 29 June 2020;
Published: 04 August 2020.
Edited by:
Guiyou Liu, Tianjin Institute of Industrial Biotechnology (CAS), ChinaReviewed by:
Tianyi Zhao, Harvard University, United StatesLiang Cheng, Harbin Medical University, China
Copyright © 2020 Shen, Liu, Li, Li and Zhou. This is an open-access article distributed under the terms of the Creative Commons Attribution License (CC BY). The use, distribution or reproduction in other forums is permitted, provided the original author(s) and the copyright owner(s) are credited and that the original publication in this journal is cited, in accordance with accepted academic practice. No use, distribution or reproduction is permitted which does not comply with these terms.
*Correspondence: Hongyu Zhou, enp4eTEzNUB5ZWFoLm5ldA==