- 1School of Biosciences and Technology, Vellore Institute of Technology, Vellore, India
- 2Department of Biotechnology, College of Biomedical Sciences Technology and Research, Sri Ramachandra Institute of Higher Education and Research (DU), Chennai, India
- 3Department of Biomedical Sciences, College of Health and Sciences, Qatar University, QU Health, Doha, Qatar
Background and Aims: Familial hypercholesterolemia (FH) is one of the major risk factor for the progression of atherosclerosis and coronary artery disease. This study focused on identifying the dysregulated molecular pathways and core genes that are differentially regulated in FH and to identify the possible genetic factors and potential underlying mechanisms that increase the risk to atherosclerosis in patients with FH.
Methods: The Affymetrix microarray dataset (GSE13985) from the GEO database and the GEO2R statistical tool were used to identify the differentially expressed genes (DEGs) from the white blood cells (WBCs) of five heterozygous FH patients and five healthy controls. The interaction between the DEGs was identified by applying the STRING tool and visualized using Cytoscape software. MCODE was used to determine the gene cluster in the interactive networks. The identified DEGs were subjected to the DAVID v6.8 webserver and ClueGo/CluePedia for functional annotation, such as gene ontology (GO) and enriched molecular pathway analysis of DEGs.
Results: We investigated the top 250 significant DEGs (p-value < 0.05; fold two change ≥ 1 or ≤ −1). The GO analysis of DEGs with significant differences revealed that they are involved in critical biological processes and molecular pathways, such as myeloid cell differentiation, peptidyl-lysine modification, signaling pathway of MyD88-dependent Toll-like receptor, and cell-cell adhesion. The analysis of enriched KEGG pathways revealed the association of the DEGs in ubiquitin-mediated proteolysis and cardiac muscle contraction. The genes involved in the molecular pathways were shown to be differentially regulated by either activating or inhibiting the genes that are essential for the canonical signaling pathways. Our study identified seven core genes (UQCR11, UBE2N, ADD1, TLN1, IRAK3, LY96, and MAP3K1) that are strongly linked to FH and lead to a higher risk of atherosclerosis.
Conclusion: We identified seven core genes that represent potential molecular biomarkers for the diagnosis of atherosclerosis and might serve as a platform for developing therapeutics against both FH and atherosclerosis. However, functional studies are further needed to validate their role in the pathogenesis of FH and atherosclerosis.
Introduction
Atherosclerosis is a chronic immune-inflammatory disease that is characterized by the progressive accumulation of lipids in the intimal space of the atrial walls, which results in such complications as chronic low-grade inflammation, endothelial dysfunction, and oxidative stress. A high level of low-density lipoprotein (LDL) in plasma induces atherosclerosis. In contrast, a decreasing level of LDL cholesterol is associated with a decreased frequency of severe cardiovascular events (Silverman et al., 2016). Elevated levels of blood cholesterol are caused by a group of genetic defects known as familial hypercholesterolemia (FH). FH is one of the known genetic causes of premature cardiovascular disease due to prolonged exposure to elevated LDL, with a prevalence of ∼1:220 being observed (Abul-Husn et al., 2016; Wald et al., 2016; Alhababi and Zayed, 2018). Recent studies estimated the prevalence of heterozygous FH (HeFH) to be significantly higher (1/220-250) than initially reported (1/500) (Hopkins et al., 2011; Genest et al., 2014; Perez-Calahorra et al., 2019). However, the homozygous FH (HoFH) prevalence has been estimated to be 1 in 300,000–1,000,000 (Austin et al., 2004; Sjouke et al., 2015; de Ferranti et al., 2016; Akioyamen et al., 2017; Alhababi and Zayed, 2018; Alonso et al., 2018). The prevalence of FH is higher due to founder effect that estimates to be upto 1 in 50–67 in some populations like Lebanese, Ashkenazi Jews, French Canadians, Finns, Afrikaners, and Tunisians (Leitersdorf et al., 1990; Nanchen et al., 2015; Amor-Salamanca et al., 2017; Alonso et al., 2018). In the past, the term FH was used to refer to defects in the LDL receptor (Goldberg et al., 2011). Among FH patients, the clinical phenotypes are distinctly versatile, even in patients who share the same disease-causing mutation. This finding suggests that FH is not a single disease but is a multifaceted syndrome (Hartgers et al., 2015; Di Resta and Ferrari, 2018; Masana et al., 2019). HeFH is mainly caused by mutations that occur in such genes as LDLR, less frequently, mutations in APOB and PCSK9 genes can be found in patients with phenotypic FH (Soutar and Naoumova, 2007; Gidding et al., 2015). Several studies reported that HoFH causes considerable premature ASCVD, and would result in early death if left untreated (males are at 50% risk, and females are at 30% risk) (Slack, 1969; Stone et al., 1974; Joseph et al., 2001; Naoumova et al., 2004; Vuorio et al., 2014). For the management of patients above 75 years of age with clinically evident atherosclerotic cardiovascular disease (ASCVD), the ACC/AHA standards endorse a moderate intensity (but not a high-intensity) statin (Vuorio et al., 2013, 2017; Stone et al., 2014). Microarray technology is a robust procedure that is widely used to compare genes that are differentially expressed in patients with different diseases. This technology is also beneficial in understanding gene association, mapping, expression, and linkage studies (Russo et al., 2003). However, studies that investigated the white blood cells (WBCs) transcriptome of patients with FH versus healthy controls are limited. Therefore, this study aimed to identify differentially expressed genes (DEGs), protein-protein interactions, and dysregulated pathways that might be involved in an increased risk of atherosclerosis due to FH.
Materials and Methods
Array Data Acquisition and Processing
The GEO database from NCBI1 was used to access the GSE13985 dataset that contains expression profiles by array. The datasets from various experiments are deposited in this database and enable users to download the gene expression profiles stored in GEO (Barrett et al., 2013). To seek GEO datasets for related gene expression profiles, we used the keywords “Familial Hypercholesterolemia” and “Microarray” and “Homo sapiens.” GSE13985 contains ten samples, including five patients with FH and five healthy control samples obtained with the help of platform GPL570 [HG-U133_Plus_2] Affymetrix Human Genome U133 Plus 2.0 Array (Režen et al., 2008). The five FH patients and five controls were matched by age, BMI, sex, and smoking status. The FH patients were free of clinical ASCVD. The Blood samples were provided from the ten samples, and RNA was extracted to be used for the array analysis. The gene expression data were downloaded from the public database, and in this study, there were no animal or human experiments assisted by any of the authors.
Data Preprocessing and Identification of DEGs
With the help of powerful multiarray technology, the preliminary data from the dataset were made susceptible to the correction of background, quantile normalization, and log transition (Irizarry et al., 2003). Initial processing of the data involved altering specific gene symbols from probe IDs with the help of a Entrez’s Gene ID converter (Alibés et al., 2007). When the same gene contribution was observed in several samples, their mean value was determined and considered as the eventual level of gene expression. To examine the raw gene expression data, the online statistical tool GEO2R was utilized, and the tool incorporated the R/Bioconductor and Limma package v3.26.8 (Smyth, 2005; Barrett et al., 2013; Ritchie et al., 2015). The GEO2R inbuilt methods, such as T-test and Benjamini and Hochberg (false discovery rate), were used to calculate the p-value and FDR in order to determine the DEGs among patients with FH and controls (Aubert et al., 2004). We set the principal standards of | log (fold change) | > 1 and p < 0.05 to acquire DEGs that are significant from the dataset, whereas the upregulated DEGs were considered if the logFC ≥ 1 and logFC ≤ −1 for downregulated DEGs. The RStudio (v1.2.5019) and library Calibrate package were used to create the volcano plot. The subsequent DEGs were attained from a dataset, and further investigation was performed with selected DEGs. The heat map for the gene expression data was generated using a heat mapper webserver.2 The inbuilt average linkage clustering method was used to compute the hierarchical clustering, and the Euclidean algorithm was used for computing the distance between rows and columns (Babicki et al., 2016). The flowchart diagram for this study is represented in Figure 1.
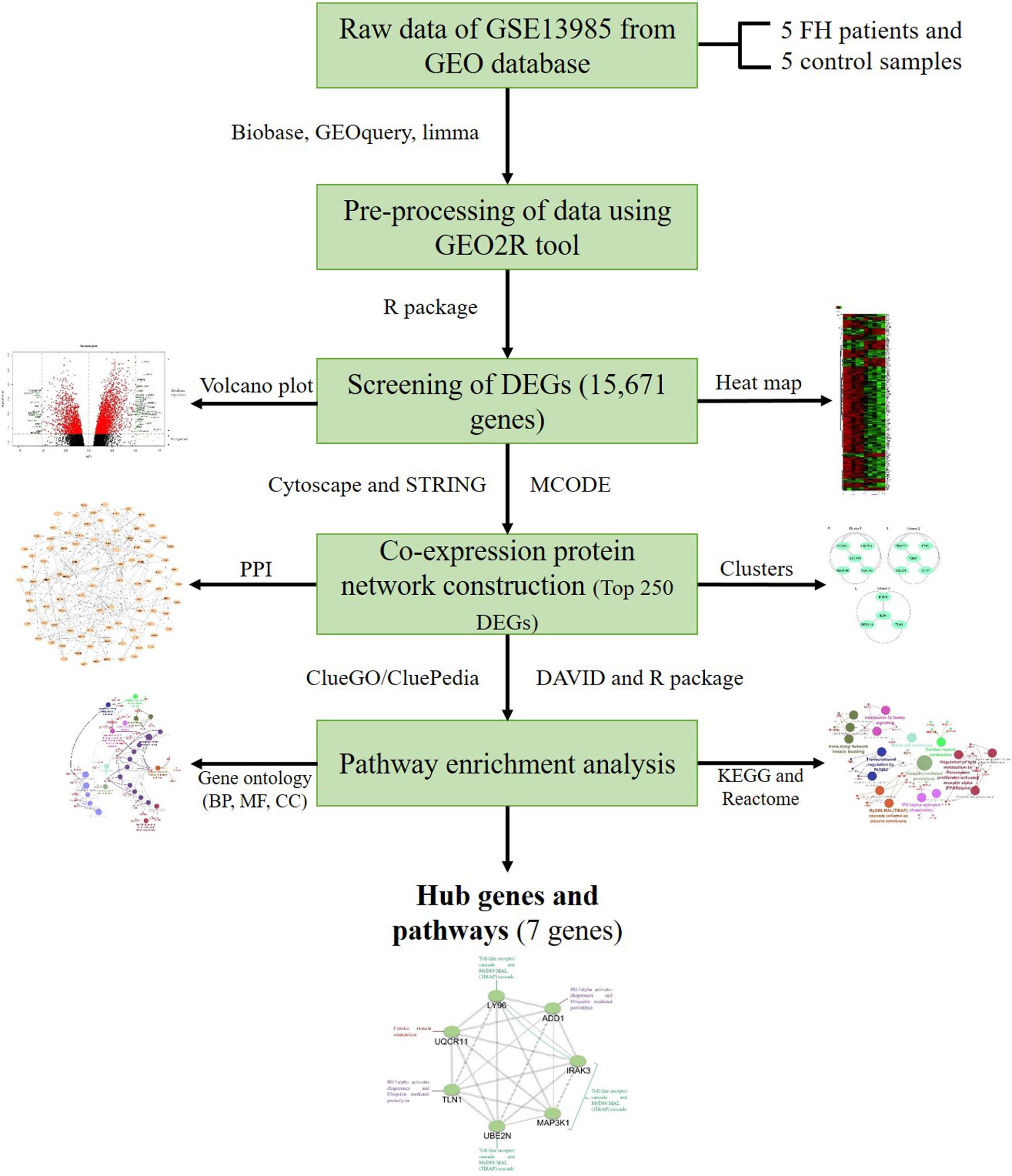
Figure 1. Outline of the workflow and expression data analysis in the present study using bioinformatic strategies.
Establishment of PPI Networks and Module Analysis
We framed a PPI (protein-protein interaction) network by utilizing the STRING web-based tool (v11.0,3) to evaluate the relationship among the DEGs from the attained datasets (Szklarczyk et al., 2017, 2019). To eradicate the PPI interactions that are in consistent from the dataset, we fixed the cutoff standard to a confident interaction score ≥0.4. Therefore, we attained a strong PPI network. Then, we combined the outcomes from the STRING tool to Cytoscape software (v3.7.1,4) to conceptualize the PPI interactions among the statistically appropriate DEGs (Shannon et al., 2003). To recognize the intersected clusters from the attained PPI network, we exploited the Cytoscape plugin Molecular Complex Detection plugin (MCODE). The group (cluster) determining extremities were charted, such as Kappa score (K-core) fixed to five, Degree Cutoff fixed to two, Max. Depth fixed to 100, and Node score Cutoff fixed to 0.2, which constraints the cluster size for coexpressing networks (Bader and Hogue, 2003). Further, we utilized the GeneMANIA web server to perform the inter-relation analysis and predict the function of the identified seven potential DEGs (Warde-Farley et al., 2010; Franz et al., 2018).
DAVID and ClueGO Enrichment Analysis
For functional annotation of GO and analysis of KEGG pathway enrichment, we used the web-based DAVID v6.8 tool.5 DAVID is a significant source for any functional evaluation of the high-throughput gene expression profiles (Huang et al., 2009a, b). The results from DAVID was further imported to GOplot in R Studio. The GOBubble and GOChord were used to visualize the functional enrichment of the top 250 DEGs, which facilitate the combination and integration of expression data with functional assessment results (Walter et al., 2015). For integrative analysis, we utilized both DAVID and ClueGO software to comprehensively observe the DEGs involved in the GO terms and pathways. The first DEGs from the GEO2R tool were exposed to ClueGO v2.5.5/CluePedia v1.5.5 to attain complete Gene Ontological terms (GO) and disease-related pathways from the DEG dataset. ClueGO syndicates KEGG or BioCarta pathways and GO, which delivers a fundamentally organized pathway network or GO from the DEG dataset (Bindea et al., 2009). Also, the study of molecular/biological function GO, and enrichment of pathways analysis was conducted for DEGs, and p-values < 0.05 were considered to be significant.
Results
Data Acquisition and Identification of DEGs
The GSE13985 dataset contained the gene expression profiles of five patient samples with FH, and five control groups (atherosclerotic markers in human blood - a study in patients with familial hypercholesterolemia) were obtained from the GEO database (Režen et al., 2008). The GPL570 platform (Affymetrix Human Genome U133 Plus 2.0 Array) was used in this work. Table 1 represents the original features of the patients and control samples involved in this study. By using the publicly available GEO2R tool, we identified the DEGs between FH patients and healthy controls according to the cutoff values of | log2FC| ≥ 1.0 and p-values < 0.05, which were calculated based on the inbuilt R/Bioconductor and limma package v3.26.8 from the GEO2R tool, the top 250 DEGs were accordingly identified. The R studio and library calibrate package was used to construct the volcano plot to compare the DEGs between FH patients and healthy controls. Figure 2 represents the volcano plot, and the significant genes with satisfying values (p-value < 0.05, logFC ≥ 1, and logFC ≤ −1) are labeled and shown in green dots. The top 250 DEGs identified between both groups were subjected to a heat mapper web server to determine the expression level of the genes. As a result, clustering based on hierarchy and heat maps was generated and is depicted in Figure 3.
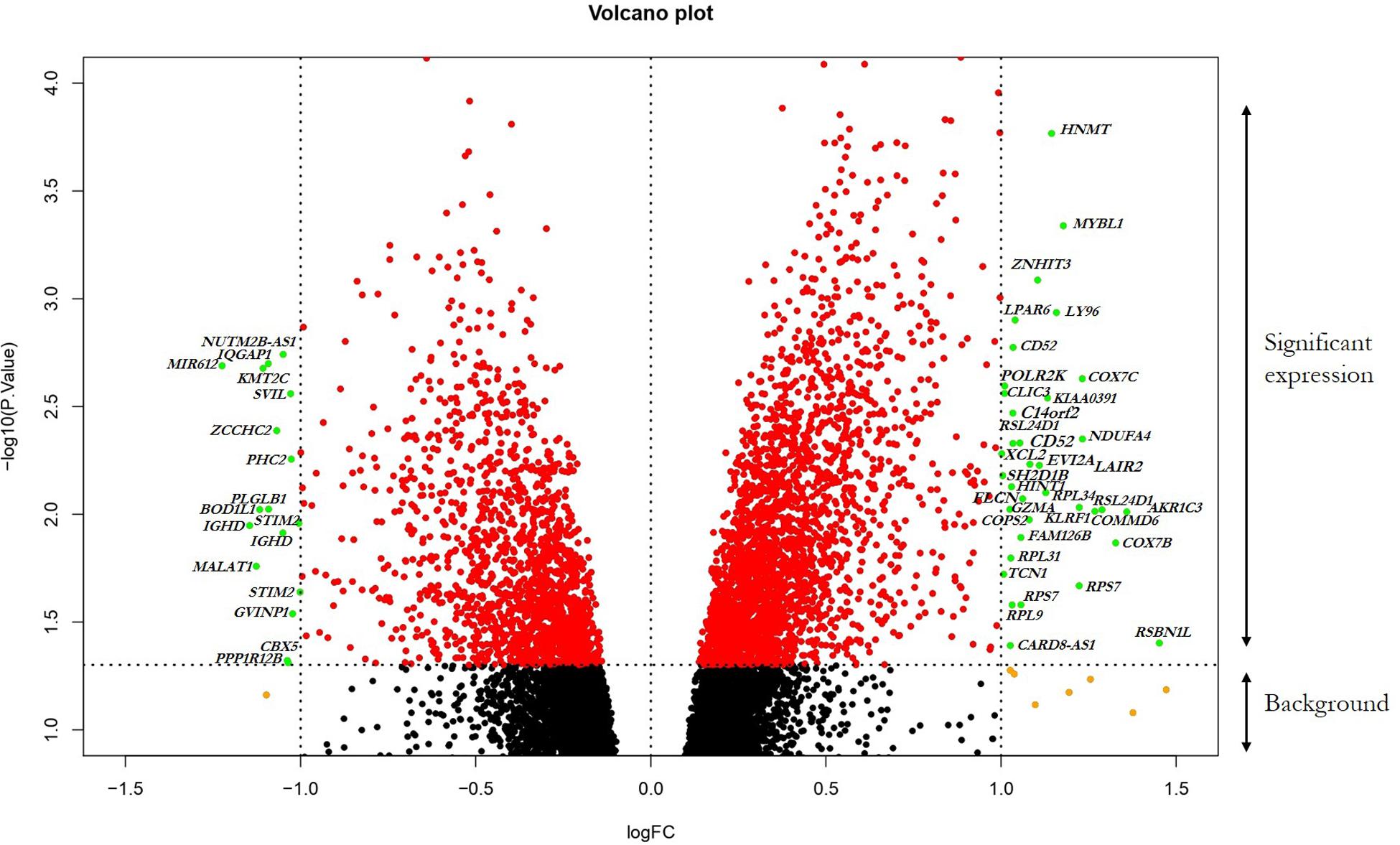
Figure 2. Visualization of DEGs volcano plots using R studio. The plot compared the DEGs between FH patients and controls from the dataset. The representations are as follows: x-axis, logFC; y-axis, -log10 of a p-value. The p-values < 0.05 are in red dots, and logFC ≥ 1 and logFC ≤ –1 are in yellow dots; the significant DEGs with both satisfying values are in green dots and indicated with gene names. Black dots indicate the remaining genes present in the array that were not significantly changed. The genes that are upregulated in the array are on the right panel, and downregulated ones are on the left panel of the plot.
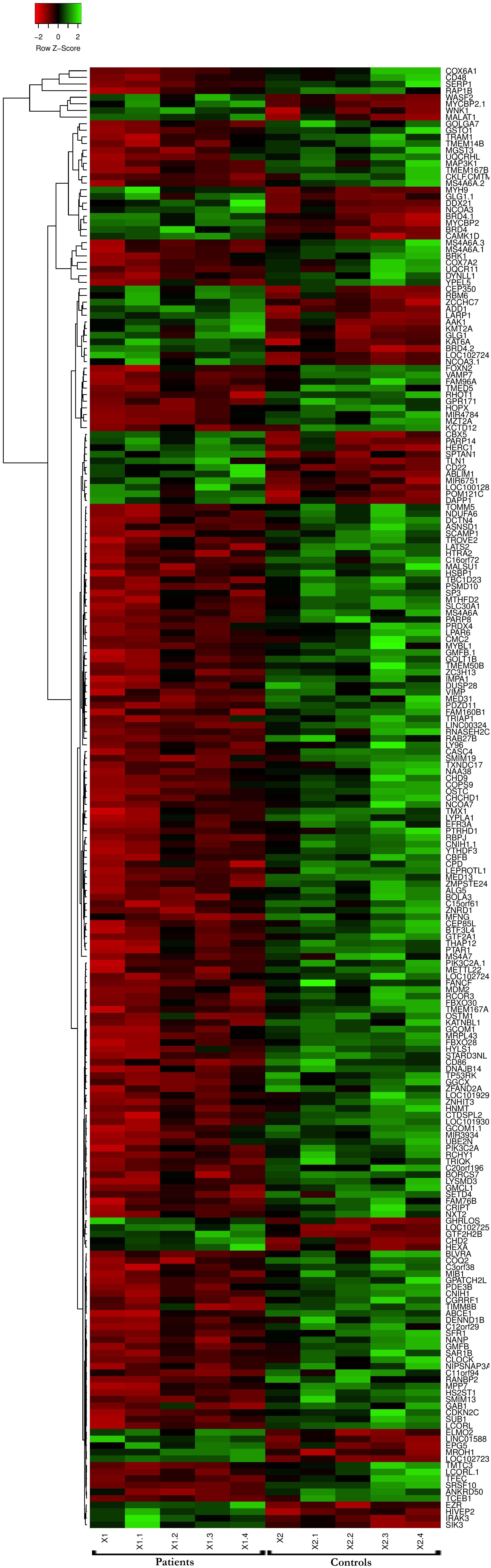
Figure 3. Microarray heat map and clustering based on hierarchy for the top 250 gene expression data from the datasets. n = 250 DEGs. Upregulated genes are represented in red, and downregulated genes are represented in green.
Establishment of PPI Networks and Module Analysis
The physical and functional associations among the proteins of DEGs of FH were assessed using the STRING tool. The minimum required interaction score was set to the confidence of 0.4. Simple tabular text output was generated from STRING. The interaction among the query proteins was further visualized using Cytoscape v3.7.1. Figure 4 represents the network with 96 nodes and 134 edges of PPI. The nodes denote the number of proteins while edges and their interactions. The Cytoscape plugin MCODE v1.5.1 interpreted the closely interlinked regions from the network of proteins in the form of clusters. The top three clusters that are significant from the PPI network with MCODE scores of 5, 5, and 4 were preferred. These clusters are represented graphically in Figure 5. Cluster 1 was derived from node UQCR11 (Figure 5A). Cluster 2 and Cluster 3 were derived from nodes TCEB1 and EZR, respectively (Figures 5B,C). The tabular column represents the detailed MCODE clusters of interlinked regions with their cluster number, MCODE scores, node IDs, node numbers, and edge numbers (Table 2).
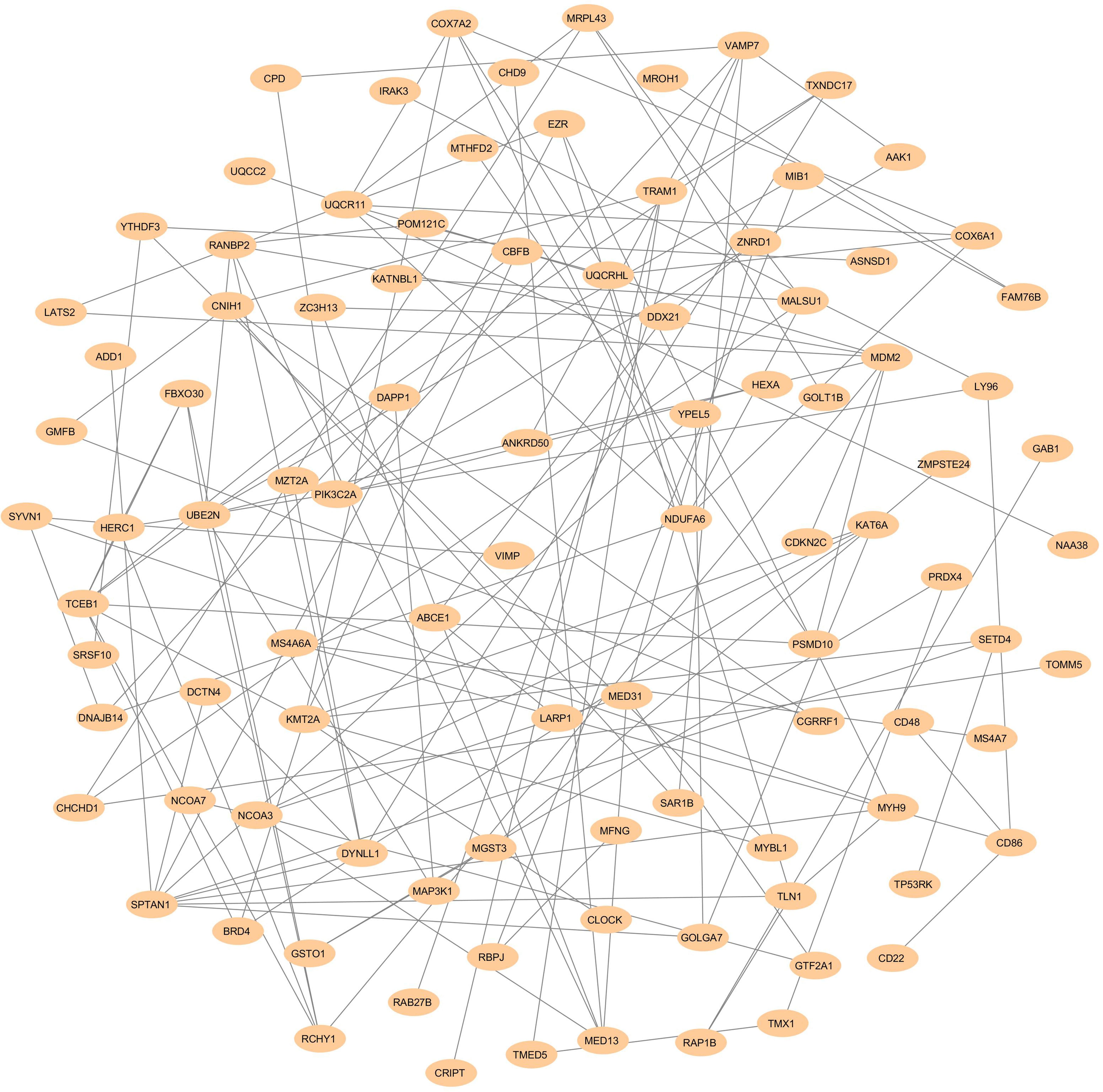
Figure 4. PPI networks show the interaction of DEGs from the GSE13985 dataset. The nodes and edges are retrieved from the STRING tool and plotted using Cytoscape software. The nodes are displayed as Navajo white, while edges are shown as gray.
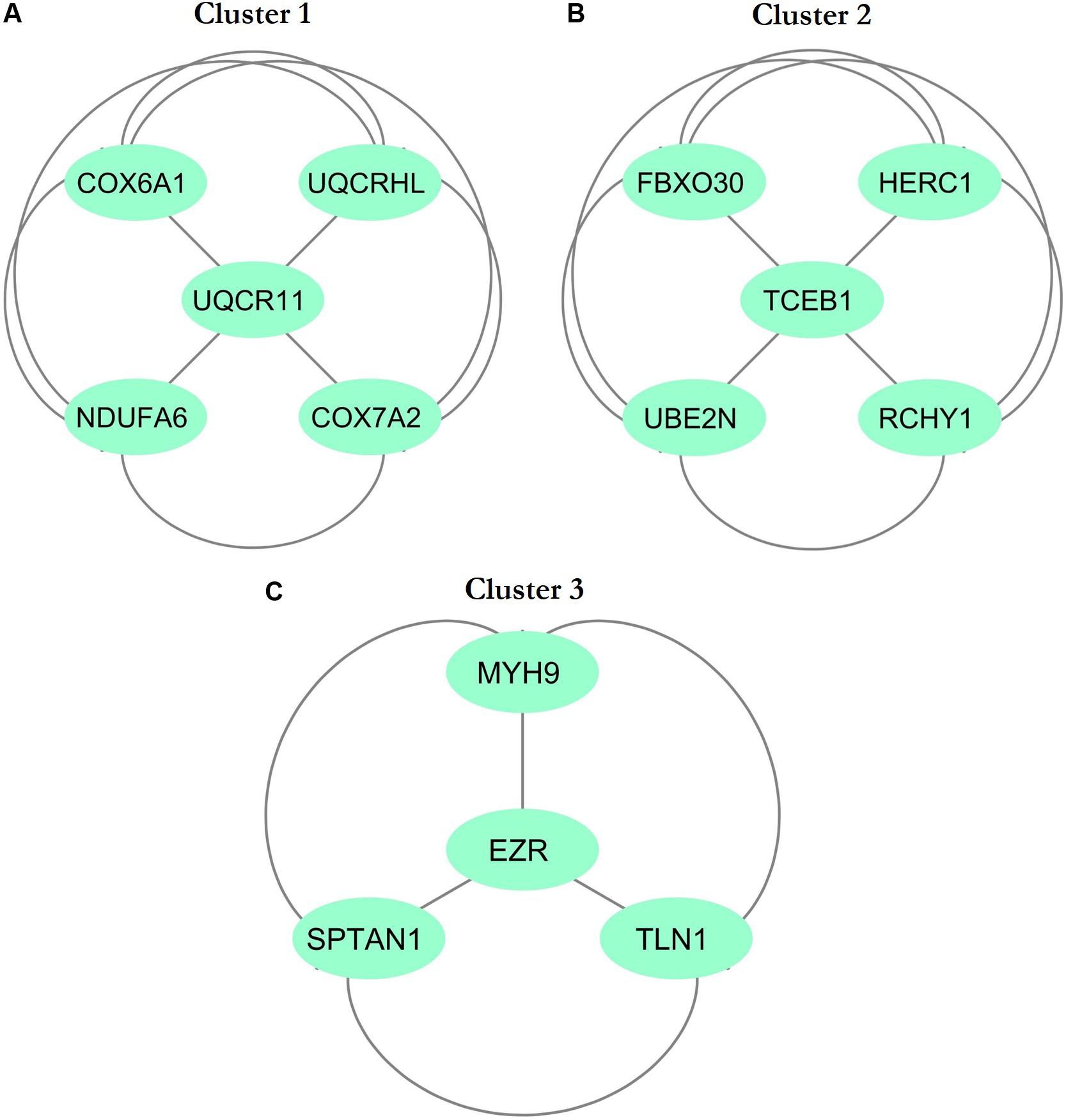
Figure 5. Top three cluster subnetworks were identified from the PPI network with the help of Cytoscape using the MCODE plugin with a cluster score above three. The aquamarine (ellipse) color indicates the nodes, while the edges are in gray. (A) Cluster 1; (B) Cluster 2; (C) Cluster 3.
DAVID Enrichment Analysis
For the functional annotation of DEGs, the DAVID v6.8 online server was used. To ascertain the KEGG pathway-enriched genes and the potential GO (Gene Ontology) classification, terms approximating biological process, molecular functions, and signaling pathways concerning KEGG pathways were used. The modified Fisher exact p-value (EASE score) ≤ 0.05 and FDR < 0.05 are considered strongly enriched. By analyzing BP, we found that the DEGs from the complex PPI network were enriched in myeloid cell differentiation (GO: 0030099), intracellular transport (GO: 0046907), negative regulation of response to DNA damage stimulus (GO: 2001021), peptidyl-lysine modification (GO: 0018205), negative regulation of signal transduction by p53 class mediator (GO: 1901797), and MyD88-dependent toll-like receptor signaling pathway (GO: 0002755). The Gene Ontology MF analysis revealed the involvement of DEGs in antioxidant activity (GO: 0016209), p53 binding (GO: 0002039), thyroid hormone receptor binding (GO: 0046966), cadherin binding involved in cell-cell adhesion (GO: 0098641), transcription factor activity, and transcription factor binding (GO: 0000989). In addition, we utilized the DAVID online method to classify the DEGs entailed in the different biological pathways based on the KEGG reference database (p < 0.05; FDR < 0.05). The KEGG pathway enrichment analysis revealed the association of the DEGs in ubiquitin-mediated proteolysis (hsa04120) and cardiac muscle contraction (hsa04260). The annotated results for the following terms were tabulated (Table 3). The GOBubble plots were constructed for the BP and MF of the top 250 DEGs from the dataset (Figures 6A,B), whereas the enriched cellular components of the identified DEGs were plotted with GOChord (Figure 6C).
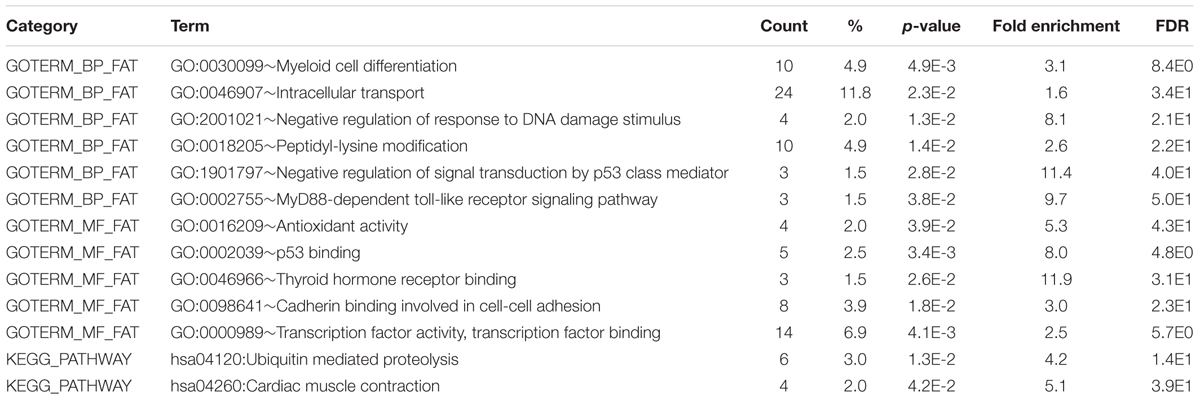
Table 3. Gene ontology (GO) terms such as biological process, molecular functions, and KEGG pathways of DEGs that are associated with familial hypercholesterolemia from DAVID.
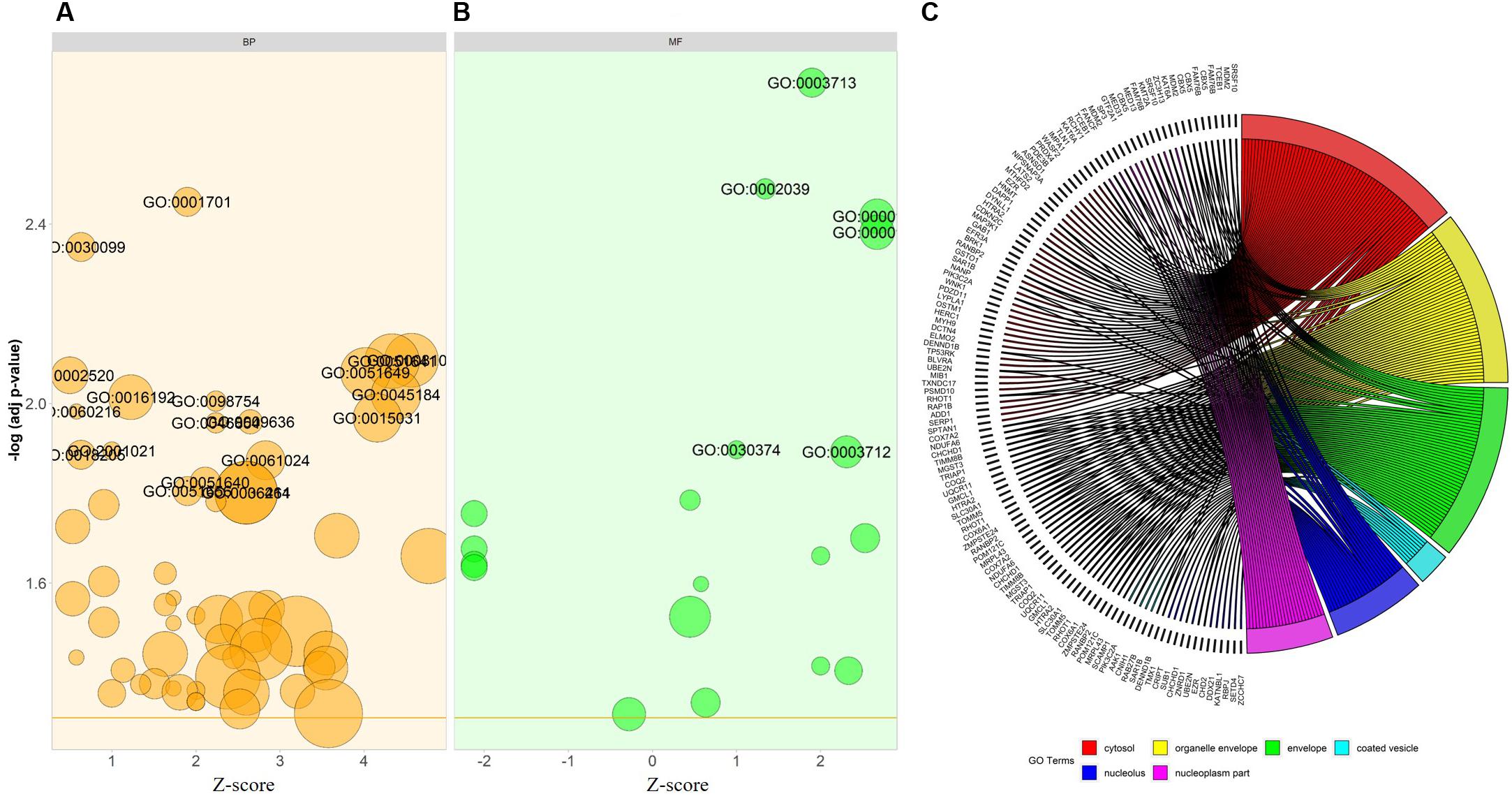
Figure 6. Bubble plot depicts the (A) biological process (BP-in orange), and (B) molecular function (MF-in green) of the GO terms from DAVID. The –log p-value allocated to the y-axis and a Z-score to x-axis. The region of bubbles are significantly proportional to the number of DEGs in a given GO term. The threshold implies the p-value standardized to 0.05 (orange line in BP and MF). (C) The association between the selected DEGs; their respective cellular component (CC) and GO terms, along with the gene’s log2FC are presented in GOChord plot. The plot’s left half showed the DEGs that involved in different cellular components and the right half displays the GO terms of cellular components in various colors. The colored bands connected a gene to a specific term GO.
ClueGO/CluePedia Enrichment Analysis
The Cytoscape plugin ClueGO/CluePedia was used to study the functional enrichment of the DEGs from the dataset. ClueGo helped visualize the GO terms of the identified PPI complex network. The MF and BF terms of the GO functional enrichment analysis of the complex PPI network are depicted in Figure 7. The statistical options for ClueGO enrichment analysis were set based on a hypergeometric test that is two-sided with p ≤ 0.05, Benjamini-Hochberg correction, and kappa score ≥ 0.4 as a primary criterion. The BF and MF of DEGs from the complex PPI network were predominantly enriched in the negative regulation of intracellular transport (GO: 0032387), endothelial cell development (GO: 0001885), scaffold protein binding (GO: 0097110), regulation of DNA damage response and signal transduction by p53 class mediator (GO: 0043516), p53 binding (GO: 0002039), peptidyl-lysine trimethylation (GO: 0018023), antioxidant activity (GO: 0016209), positive regulation of the Notch signaling pathway (GO: 0045747), and MyD88-dependent Toll-like receptor signaling pathway (GO: 0002755) (Figure 7). The KEGG and REACTOME pathway analysis from ClueGO showed that many DEGs were significantly enriched in cardiac muscle contraction (KEGG: 04260), regulation of lipid metabolism by peroxisome proliferator-activated receptor alpha (PPAR alpha) (R-HAS: 400206), transcriptional regulation by RUNX3 (R-HAS: 8878159), ubiquitin-mediated proteolysis (KEGG: 04120), renal cell carcinoma (KEGG: 05211), MyD88: MAL (TIRAP) cascade initiated on plasma membrane (R-HAS: 166058), and IRE1 alpha-activated chaperones (R-HAS: 381070) (Figure 8). Taken together, the results from ClueGO enrichment clearly illustrate that the DEGs change the metabolic behavior of the signaling pathways and are closely linked to FH, contributing to the progression of such complications as coronary artery disease and cardiovascular disease, which may lead to atherosclerosis. Additionally, the dysregulated pathways identified by our bioinformatics enrichment analysis could play important roles in FH pathogenesis. However, functional validations are needed to test our bioinformatics findings.
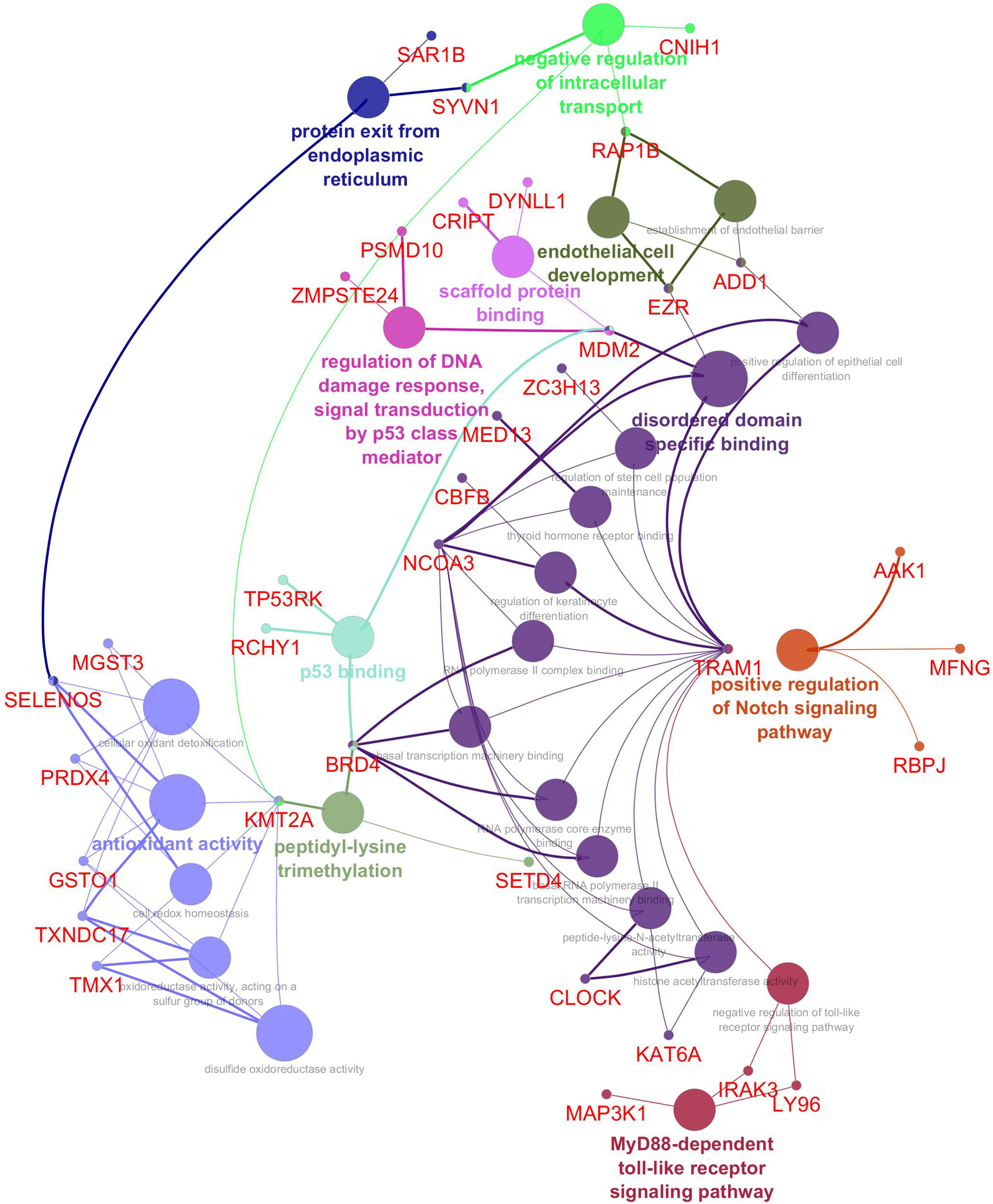
Figure 7. Enrichment by Gene Ontology (GO) terms was visualized using the ClueGO/CluePedia plugin from Cytoscape. Vital molecular functions (MF) and biological processes (BP) involved in the DEGs are shown with the specific gene interactions. The MF and BP enrichment analyses are inferred from the 250 top DEGs PPI network. The connectivity of the GO terms network described by functional nodes and edges that are shared between the DEGs with a kappa score of 0.4. The enrichment shows only significant GO terms (p-value ≤ 0.05). The values of p ≤ 0.05 indicate the node size. The node color code indicates the specific functional class that they are involved in. The color represents various molecular function and biological process involved in the enrichment analysis. The bold fonts indicate the most important functional GO terms that define the names of MF and BP of each group. The names of the DEGs involved in each group are displayed in red font.
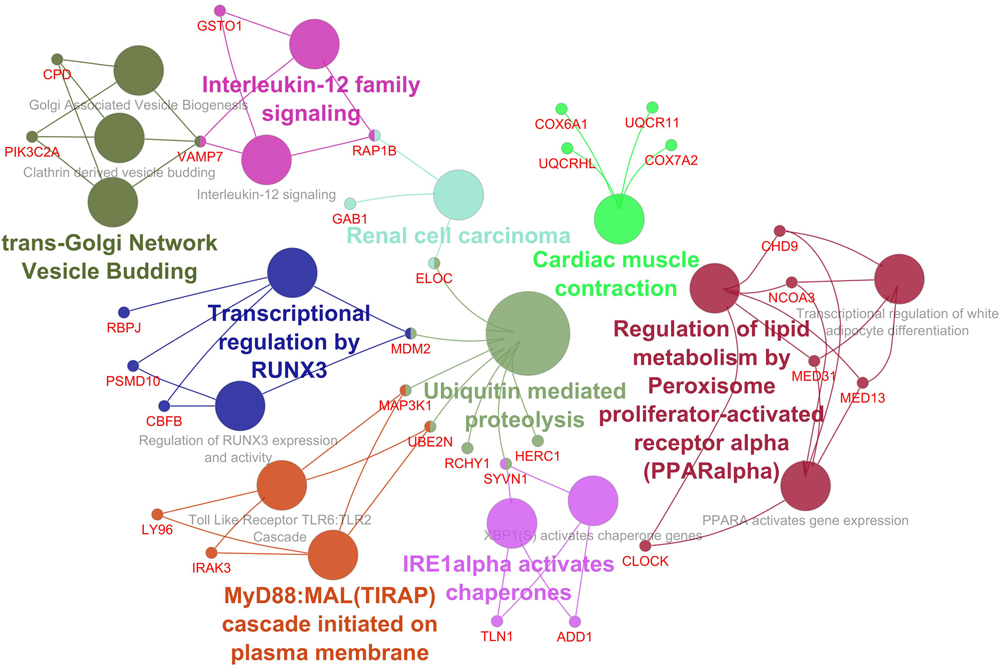
Figure 8. Enrichment by pathway terms is visualized using the ClueGo/CluePedia plugin from Cytoscape. The plugin delivers a comprehensive enrichment analysis for DEGs, including KEGG and REACTOME pathways. The connectivity of the pathways in the network described by functional nodes and edges that are shared between the DEGs with a kappa score of 0.4. The enrichment shows only significant pathways (p-value ≤ 0.05). The values of p ≤ 0.05 indicate the node size. The node color code indicates the specific functional class that they are involved in. The color represents various molecular pathways involved in the enrichment analysis of the identified DEGs. The bold fonts indicate the most critical functional pathways that define the names of the signaling pathway of each group. The names of the DEGs involved in each group are displayed in red font.
Discussion
In the present study, we investigated the DEGs between five patients with FH and five healthy controls with the GEO ID of GSE13985 (Režen et al., 2008). We examined a total of 13,057 DEGs, and the top 250 significant DEGs were considered for further studies (Supplementary Table S1). We found the top three significant clusters that are distinguished by high scores and closely interconnected regions from the networks of PPI (Figures 5A–C). Screening the gene cluster could help in determining the crucial genes and their interactions and how they are associated with the pathogenesis and progression of atherosclerosis. The clusters retrieved from MCODE were often shown in the PPI network mechanisms, and representation of clusters is crucial for a functional and comprehensive understanding of network properties (Krogan et al., 2006; Rahman et al., 2013). The densely interconnected nodes and the less connected vertices of the PPI network were weighed using the core clustering coefficient of the MCODE plugin. After computation, an algorithm examined the weighted graph to isolate the densely connected regions, which is considered as clusters and represents the molecular complexes that formed with DEGs (Sharan et al., 2007). From Table 2, we found three seed nodes, namely, the UQCR11, TCEB1, and EZR genes that might have been involved in the differential regulation of the pathway.
To explore the involvement of the 250 identified DEGs in BP, MF, and molecular pathways of FH, we used the built GO and KEGG enrichment to determine the functional annotation of these genes. We found that these DEGs were primarily enriched in myeloid cell differentiation, intracellular transport, negative regulation of response to DNA damage stimulus, peptidyl-lysine modification, negative regulation of signal transduction by p53 class mediator, and the MyD88-dependent Toll-like receptor signaling pathway. The analysis of MF from GO showed that the DEGs were significantly enriched in antioxidant activity, p53 binding, thyroid hormone receptor binding, cadherin binding involved in cell-cell adhesion, and transcription factor activity. Similarly, the analysis of KEGG pathway enrichment showed that the DEGs are involved in ubiquitin-mediated proteolysis and cardiac muscle contraction (Table 3). Interestingly, MyD88-mediated signaling plays a prominent role in the development of human atherosclerosis and matrix degradation (Monaco et al., 2009). In line with this finding, Yu et al. (2014) found that MyD88-deficient myeloid cells are involved in the inhibition of macrophage recruitment to adipose tissue and result in atherosclerosis and diet-induced systemic inflammation (Yu et al., 2014). In this context, our study identified the DEGs involved in the MyD88 signaling pathway, such as IRAK3 (interleukin 1 receptor-associated kinase 3), LY96 (lymphocyte antigen 96), and MAP3K1 (mitogen-activated protein kinase 1). Among these genes, LY96 and MAP3K1 were significantly downregulated in the FH patients, whereas IRAK3 was upregulated compared to the healthy controls (Supplementary Figure S1). IRAK3 prevents IRAK1 and IRAK4 from dissociating from MyD88 and inhibits the formation of IRAK-TRAF6 complexes (Lyu et al., 2018; Yu and Feng, 2018). The increased expression of IRAK3, as shown in Supplementary Figure S1A, typically coveys the dysregulation of MyD88 and the TLR cascade via IRAK3 expression. Similarly, the receptor complex resulting from the combination of LY96 and Toll-like receptor 4 (TLR4) ectodomain mediates transduction of the lipopolysaccharide (LPS) signal across the cell membrane (Gruber et al., 2004). A recent study found that LY96 can bind to cholesterol (Choi et al., 2016), and TLR4 activation involves agLDL, the predominant form of LDL found in atherosclerotic plaques (Singh et al., 2020). Thus, our examined DEGs are reliable with involvement in atherosclerosis-causing pathways.
To further refine the biological process, molecular functions, and pathways defined from the analysis of DAVID-GO terms (Figure 6), KEGG, and STRING, we implemented the ClueGO plugin from Cytoscape, an improved interpretation for biological terms, such as GO and KEGG pathway analysis/BioCarta, and constructed a functionally arranged network of terms GO/pathway. The plugin also helps to visualize the networks that are functionally grouped from more massive gene clusters (Bindea et al., 2009). To acquire a detailed picture of the DEGs involved in atherosclerosis, we utilized the ClueGO plugin to distinguish the molecular pathways that are differentially regulated and their significant gene interactions depending on the p-values and kappa statistics.
Among the enriched biological process and molecular pathways, we identified that ubiquitin-mediated proteolysis, cardiac muscle contraction, MyD88-dependent Toll-like receptor signaling pathway, and IRE1 alpha-activated chaperones were considered dysregulated and are significant to atherosclerosis progression in FH patients based on kappa statistics and p-values (Figures 7, 8). Additionally, we also identified the genes that are significant in the dysregulated molecular pathways and involved in FH progression. In line with this finding, UQCR11, UBE2N, ADD1, TLN1, IRAK3, LY96, and MAP3K1 were found to be associated with the risk of Atherosclerosis in FH patients.
This study identified DEGs that are involved in ubiquitin-mediated proteolysis, IRE1 alpha-activated chaperones, and cardiac muscle contraction, such as UQCR11, UBE2N, TLN1, and ADD1, respectively. As shown in Supplementary Figure S2, a significant gene expression level was shown between the FH patients and healthy control samples. In this study, the expression levels of the UQCR11 and UBE2N genes were significantly reduced, and the expression levels of the TLN1 and ADD1 genes were notably increased in the FH patients compared with the healthy controls. Our identified novel DEGs from the dataset indeed have high consistency with the risk of atherosclerosis and are involved in the dysregulation of such pathways as ubiquitin-mediated proteolysis, IRE1 alpha-activated chaperones, and cardiac muscle contraction. For instance, the transfer of ubiquitin from UBE2N to LDLR is required for its lysosomal degradation. The reduced gene expression level of UBE2N leads to skipping this event and might result in the accumulation of LDLR in the lysosome (Zhang et al., 2012).
Additionally, the polymorphism (p.G460W) present in ADD1 has reportedly contributed to the increased risk factor for coronary heart disease (Morrison et al., 2002). Our study clearly showed the increased expression of ADD1 from the FH patient dataset, which could alter the casual signaling of IRE1 alpha-activated chaperones (Supplementary Figure S2D). TLN1 functions as a molecular scaffolding protein and can contribute to the signaling of adhesion through its binding partners, translating mechanical signals into chemical signals (Hytönen and Wehrle-Haller, 2014). The TLN1 expression level in atherosclerotic plaques is significantly reduced, and it plays a central role in cell adhesion, indicating that tissue disintegration in atherosclerosis may be partly induced by TLN1 downregulation, leading to cell-ECM interaction loosening and tissue reorganization (Essen et al., 2016). However, our dataset showed increased expression of TLN1, which might differentially regulate and activate IRE1alpha chaperones. Yet, recent research has identified that proatherogenic gene expression is regulated by IRE1, which includes several essential chemokines and cytokines (Tufanli et al., 2017).
To establish the interrelationship between the core genes UQCR11, UBE2N, ADD1, TLN1, IRAK3, LY96, and MAP3K1, this interrelationship will help in understanding the coexpression and molecular pathways that pertain to FH. It is essential to comprehend the interactions between the core genes since these genes dysregulate the general molecular pathways in FH patients (Kumar et al., 2019; Udhaya Kumar et al., 2020). The differentially expressed genes might be responsible for the clinical phenotypes of the patients and the progression of atherosclerosis. We found that the Toll-like receptor and MyD88:MAL (TIRAP) cascades are activated by such DEGs as LY96, IRAK3, MAP3K1, and UBE2N (Monaco et al., 2009; Yu et al., 2014). Similarly, UQCR11 is directly involved in cardiac muscle contraction and has a physical interaction between LY96, TLN1, and UBE2N. The TLN1 and ADD1 genes directly associated with IRE1alpha-activated chaperones and ubiquitin-mediated proteolysis (Figure 9). Taken together, our observed findings suggest that the involvement of core genes related to the risk of atherosclerosis might be a critical metric in ubiquitin-mediated proteolysis, Toll-like receptor, and MyD88: MAL (TIRAP) cascades and a beneficial tool for diagnosis and targeted therapy. In addition, the FH patients were free of clinical ASCVD, and the patient 2 and 5 did not receive lipid-lowering treatment, whereas the patient 1, 3, and 4 received lovastatin, simvastatin, and atorvastatin, respectively. This drug is also given to the patients as primary and secondary prevention that develops the risk of ASCVD and for those who already developed ASCVD, respectively (Taylor et al., 2013; Vuorio et al., 2013). With the help of a clinical diagnostic strategy, approximately 50% of patients are identified in FH, which is a cost-effective process. Indeed, a diagnosis by screening the cascades is a systematic and useful tool for diagnosing FH patients before the development of Atherosclerosis (Marks et al., 2002; Leren et al., 2008).
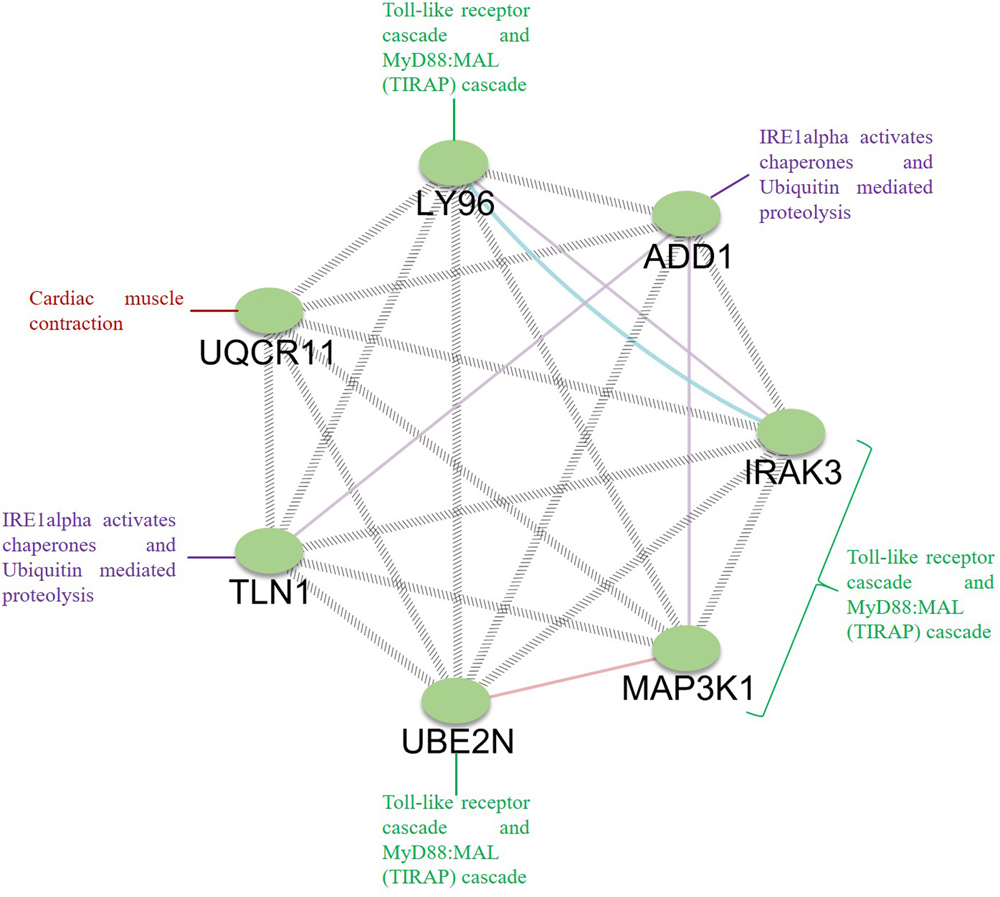
Figure 9. Analysis of the interrelation between the identified core genes. GeneMANIA was used to map the network, and Cytoscape was used for network visualization. The genes LY96, IRAK3, MAP3K1, and UBE2N are involved in the Toll-like receptor cascade and MyD88:MAL (TIRAP) cascade; ADD1 and TLN1 are involved in ubiquitin-mediated proteolysis, IRE1alpha activates chaperones, and UQCR11 is involved in cardiac muscle contraction.
In general, FH can be progressed through many cellular and molecular mechanisms, and pathways. A recent study claimed that the COX7B is a potential novel gene target for FH treatment (Li et al., 2015); however, authors did not provide supportive evidence for metabolic network and pathway links between the Atherosclerosis and FH. Later, a similar study claimed that the RPL17 and ribosome protein-related genes might increase the atherosclerotic risk. Although the study mentioned that these genes are downregulated in the FH patient’s blood cells, they are significantly upregulated in the reported dataset. In addition, they claimed that cytochrome-c oxidase genes could contribute to atherosclerosis development, yet there was no substantial evidence provided (Wang and Zhao, 2016). The study conducted by Smolock et al. (2012) suggested that RPL17 acts as an inhibitor for vascular smooth muscle cells (VSMC) and might be a therapeutic target to limit the thickening of carotid intima (Smolock et al., 2012). However, increased expression of RPL17 showed to inhibit the VSMC, reducing the progression of atherosclerosis, which could be a regular event that occurs in FH patients.
Interestingly, our functional enrichment analysis did not capture any biological/molecular functions related to inflammatory responses, as some patients with FH had shown inflammatory responses due to increased expression level of the molecules associated with tumor necrosis factor (TNF) (Fadini et al., 2014; Holven et al., 2014; Escate et al., 2018). The possible reason for this might be that the patients with FH in our study do not have any phenotypes characteristics to inflammation; consistent with the nature of FH, as it is a frequent disease (1/220-250) with a high variation in phenotypic expression associated with ASCVD (Alhababi and Zayed, 2018; Perez-Calahorra et al., 2019). Also, in era of personalized medicine, it is significant to identify the possible targeted therapy using advanced omics technologies (Prodan Žitnik et al., 2018).
Our study is the first to identify the association of core DEGs to the dysregulated pathways in FH patients. Our study recommends to screen WBCs from patients with FH to determine the metabolic and genetic factors that may help in identifying potential cardiovascular risks and might provide better diagnosis and improved therapy for the disease. The limitation of the present research is the small patient groups; therefore, investigating a larger sample size in different populations would help to confirm our data. The limitations of this study include; first, FH patients were free of clinical ASCVD and not possible to know if they have subclinical atherosclerosis by imaging. Second, the number is too small; therefore, it is important to study a larger cohort of patients that have clinical ASCVD that are compared with ones with no clinical ASCVD. Finally, no data were available for the LDL-C or TC of the FH patients in the GSE13985 dataset.
Conclusion
Most FH patients do not exhibit atherosclerotic symptoms in clinical diagnostic procedures. The findings using a transcriptome analysis from WBCs of FH patients and healthy controls to identify atherosclerotic markers are highly limited. Overall, our systematic interpretation demonstrated an essential role of DEGs and their essential role in the occurrence, development of FH, and increased risk of atherosclerosis. Our study identified a total of 250 genes that are differentially expressed and seven essential genes that are associated with FH patients compared to healthy controls. The study of expression from WBCs and the association with DEGs may help to elucidate the role played by these DEGs in FH progression and the development of atherosclerosis. Finally, we identified seven novel potential target genes (UQCR11, UBE2N, ADD1, TLN1, IRAK3, LY96, and MAP3K1), which might be valid targets for therapeutic development for FH, and might be used as diagnostic biomarkers for FH patients and prognostic indicators for atherosclerosis using WBCs from FH patients; however, functional studies are needed to validate their proposed role in FH and atherosclerosis.
Data Availability Statement
The GEO database from NCBI (Gene Expression Omnibus database, https://www.ncbi.nlm.nih.gov/geo/) was used to access the GSE13985 dataset.
Author Contributions
SU, DT, HZ, and CG were involved in the design of the study and the acquisition, analysis, and interpretation of the data. SU, DT, RB, SS, RM, MS, HZ, and CG were involved in the interpretation of the data and drafting the manuscript. CG and HZ supervised the entire study and were involved in study design, the acquisition, analysis, and understanding of the data, and drafting the manuscript. All authors contributed to the article and approved the submitted version.
Funding
This work was supported by Qatar University Grant# QUST-2-CHS-2019-3.
Conflict of Interest
The authors declare that the research was conducted in the absence of any commercial or financial relationships that could be construed as a potential conflict of interest.
Acknowledgments
The authors would like to take this opportunity to thank the management of VIT for providing the necessary facilities and encouragement to carry out this work.
Supplementary Material
The Supplementary Material for this article can be found online at: https://www.frontiersin.org/articles/10.3389/fgene.2020.00734/full#supplementary-material
Abbreviations
BP, biological process; FDR, false discovery rate; GO, gene ontology; KEGG, kyoto encyclopedia of genes and genomes; MF, molecular function.
Footnotes
- ^ https://www.ncbi.nlm.nih.gov/geo/
- ^ http://heatmapper.ca/expression/
- ^ http://www.string-db.org/
- ^ http://www.cytoscape.org/
- ^ https://david.ncifcrf.gov/
References
Abul-Husn, N. S., Manickam, K., Jones, L. K., Wright, E. A., Hartzel, D. N., Gonzaga-Jauregui, C., et al. (2016). Genetic identification of familial hypercholesterolemia within a single U.S. health care system. Science 354:aaf7000. doi: 10.1126/science.aaf7000
Akioyamen, L. E., Genest, J., Shan, S. D., Reel, R. L., Albaum, J. M., Chu, A., et al. (2017). Estimating the prevalence of heterozygous familial hypercholesterolaemia: a systematic review and meta-analysis. BMJ Open 7:e016461. doi: 10.1136/bmjopen-2017-016461
Alhababi, D., and Zayed, H. (2018). Spectrum of mutations of familial hypercholesterolemia in the 22 Arab countries. Atherosclerosis 279, 62–72. doi: 10.1016/j.atherosclerosis.2018.10.022
Alibés, A., Yankilevich, P., Cañada, A., and Díaz-Uriarte, R. (2007). IDconverter and IDClight: conversion and annotation of gene and protein IDs. BMC Bioinformatics 8:9. doi: 10.1186/1471-2105-8-9
Alonso, R., de Isla, L. P., Muñiz-Grijalvo, O., Diaz-Diaz, J. L., and Mata, P. (2018). Familial hypercholesterolaemia diagnosis and management. J. Fam. Hypercholester. Diagn. Manag. 13, 14–20.
Amor-Salamanca, A., Castillo, S., Gonzalez-Vioque, E., Dominguez, F., Quintana, L., Lluís-Ganella, C., et al. (2017). Genetically confirmed familial hypercholesterolemia in patients with acute coronary syndrome. J. Am. Coll. Cardiol. 70, 1732–1740. doi: 10.1016/j.jacc.2017.08.009
Aubert, J., Bar-Hen, A., Daudin, J. J., and Robin, S. (2004). Determination of the differentially expressed genes in microarray experiments using local FDR. BMC Bioinformatics 5:125. doi: 10.1186/1471-2105-5-125
Austin, M. A., Hutter, C. M., Zimmern, R. L., and Humphries, S. E. (2004). Genetic causes of monogenic heterozygous familial hypercholesterolemia: a huge prevalence review. Am. J. Epidemiol. 160, 407–420. doi: 10.1093/aje/kwh236
Babicki, S., Arndt, D., Marcu, A., Liang, Y., Grant, J. R., Maciejewski, A., et al. (2016). Heatmapper: web-enabled heat mapping for all. Nucleic Acids Res. 44, W147–W153. doi: 10.1093/nar/gkw419
Bader, G. D., and Hogue, C. W. (2003). An automated method for finding molecular complexes in large protein interaction networks. BMC Bioinformatics 4:2. doi: 10.1186/1471-2105-4-2
Barrett, T., Wilhite, S. E., Ledoux, P., Evangelista, C., Kim, I. F., Tomashevsky, M., et al. (2013). NCBI GEO: archive for functional genomics data sets–update. Nucleic Acids Res. 41, D991–D995. doi: 10.1093/nar/gks1193
Bindea, G., Mlecnik, B., Hackl, H., Charoentong, P., Tosolini, M., Kirilovsky, A., et al. (2009). ClueGO: a Cytoscape plugin to decipher functionally grouped gene ontology and pathway annotation networks. Bioinformatics 25, 1091–1093. doi: 10.1093/bioinformatics/btp101
Choi, S.-H., Kim, J., Gonen, A., Viriyakosol, S., and Miller, Y. I. (2016). MD-2 binds cholesterol. Biochem. Biophys. Res. Commun. 470, 877–880. doi: 10.1016/j.bbrc.2016.01.126
de Ferranti, S. D., Rodday, A. M., Mendelson, M. M., Wong, J. B., Leslie, L. K., and Sheldrick, R. C. (2016). Prevalence of Familial Hypercholesterolemia in the 1999 to 2012 United States National Health and nutrition examination surveys (NHANES). Circulation 133, 1067–1072. doi: 10.1161/CIRCULATIONAHA.115.018791
Di Resta, C., and Ferrari, M. (2018). Next generation sequencing: from research area to clinical practice. EJIFCC 29, 215–220.
Escate, R., Mata, P., Cepeda, J. M., Padreó, T., and Badimon, L. (2018). miR-505-3p controls chemokine receptor up-regulation in macrophages: role in familial hypercholesterolemia. FASEB J. 32, 601–612. doi: 10.1096/fj.201700476R
Essen, M. V., Rahikainen, R., Oksala, N., Raitoharju, E., Seppälä, I., Mennander, A., et al. (2016). Talin and vinculin are downregulated in atherosclerotic plaque; tampere Vascular Study. Atherosclerosis 255, 43–53. doi: 10.1016/j.atherosclerosis.2016.10.031
Fadini, G. P., Iori, E., Marescotti, M. C., de Kreutzenberg, S. V., and Avogaro, A. (2014). Insulin-induced glucose control improves HDL cholesterol levels but not reverse cholesterol transport in type 2 diabetic patients. Atherosclerosis 235, 415–417. doi: 10.1016/j.atherosclerosis.2014.05.942
Franz, M., Rodriguez, H., Lopes, C., Zuberi, K., Montojo, J., Bader, G. D., et al. (2018). GeneMANIA update 2018. Nucleic Acids Res. 46, W60–W64. doi: 10.1093/nar/gky311
Genest, J., Hegele, R. A., Bergeron, J., Brophy, J., Carpentier, A., Couture, P., et al. (2014). Canadian cardiovascular society position statement on familial hypercholesterolemia. Can. J. Cardiol. 30, 1471–1481. doi: 10.1016/j.cjca.2014.09.028
Gidding, S. S., Ann, C. M., de Ferranti, S. D., Defesche, J., Ito, M. K., Knowles, J. W., et al. (2015). The agenda for familial hypercholesterolemia. Circulation 132, 2167–2192. doi: 10.1161/CIR.0000000000000297
Goldberg, A. C., Hopkins, P. N., Toth, P. P., Ballantyne, C. M., Rader, D. J., Robinson, J. G., et al. (2011). Familial hypercholesterolemia: screening, diagnosis and management of pediatric and adult patients: clinical guidance from the national lipid association expert panel on familial hypercholesterolemia. J. Clin. Lipidol. 5, S1–S8. doi: 10.1016/j.jacl.2011.04.003
Gruber, A., Manèek, M., Wagner, H., Kirschning, C. J., and Jerala, R. (2004). Structural model of MD-2 and functional role of its basic amino acid clusters involved in cellular lipopolysaccharide recognition. J. Biol. Chem. 279, 28475–28482. doi: 10.1074/jbc.M400993200
Hartgers, M. L., Ray, K. K., and Hovingh, G. K. (2015). New approaches in detection and treatment of familial hypercholesterolemia. Curr. Cardiol. Rep. 17:109. doi: 10.1007/s11886-015-0665-x
Holven, K. B., Narverud, I., Lindvig, H. W., Halvorsen, B., Langslet, G., Nenseter, M. S., et al. (2014). Subjects with familial hypercholesterolemia are characterized by an inflammatory phenotype despite long-term intensive cholesterol lowering treatment. Atherosclerosis 233, 561–567. doi: 10.1016/j.atherosclerosis.2014.01.022
Hopkins, P. N., Toth, P. P., Ballantyne, C. M., and Rader, D. J. (2011). Familial hypercholesterolemias: prevalence, genetics, diagnosis and screening recommendations from the national lipid association expert panel on familial hypercholesterolemia. J. Clin. Lipidol. 5, S9–S17. doi: 10.1016/j.jacl.2011.03.452
Huang, D. W., Sherman, B. T., and Lempicki, R. A. (2009a). Bioinformatics enrichment tools: paths toward the comprehensive functional analysis of large gene lists. Nucleic Acids Res. 37, 1–13. doi: 10.1093/nar/gkn923
Huang, D. W., Sherman, B. T., and Lempicki, R. A. (2009b). Systematic and integrative analysis of large gene lists using DAVID bioinformatics resources. Nat. Protoc. 4, 44–57. doi: 10.1038/nprot.2008.211
Hytönen, V. P., and Wehrle-Haller, B. (2014). Protein conformation as a regulator of cell–matrix adhesion. Phys. Chem. Chem. Phys. 16, 6342–6357. doi: 10.1039/C3CP54884H
Irizarry, R. A., Bolstad, B. M., Collin, F., Cope, L. M., Hobbs, B., and Speed, T. P. (2003). Summaries of affymetrix GeneChip probe level data. Nucleic Acids Res. 31:e15. doi: 10.1093/nar/gng015
Joseph, L. G., Helen, H. H., and Michael, S. B. (2001). “Familial hypercholesterolemia,” in The Online Metabolic and Molecular Bases of Inherited Disease (OMMBID), eds D. L. Valle, S. Antonarakis, A. Ballabio, A. L. Beaudet, and G. A. Mitchell (New York, NY: McGraw-Hill), 2863–2913.
Krogan, N. J., Cagney, G., Yu, H., Zhong, G., Guo, X., Ignatchenko, A., et al. (2006). Global landscape of protein complexes in the yeast Saccharomyces cerevisiae. Nature 440, 637–643. doi: 10.1038/nature04670
Kumar, S. U., Kumar, D. T., Siva, R., Doss, C. G. P., and Zayed, H. (2019). Integrative bioinformatics approaches to map potential novel genes and pathways involved in ovarian cancer. Front. Bioeng. Biotechnol. 7:391. doi: 10.3389/fbioe.2019.00391
Leitersdorf, E., Tobin, E. J., Davignon, J., and Hobbs, H. H. (1990). Common low-density lipoprotein receptor mutations in the French Canadian population. J. Clin. Invest. 85, 1014–1023. doi: 10.1172/JCI114531
Leren, T. P., Finborud, T. H., Manshaus, T. E., Ose, L., and Berge, K. E. (2008). Diagnosis of familial hypercholesterolemia in general practice using clinical diagnostic criteria or genetic testing as part of cascade genetic screening. PHG 11, 26–35. doi: 10.1159/000111637
Li, G., Wu, X.-J., Kong, X.-Q., Wang, L., and Jin, X. (2015). Cytochrome c oxidase subunit VIIb as a potential target in familial hypercholesterolemia by bioinformatical analysis. Eur. Rev. Med. Pharmacol. Sci. 19, 4139–4145.
Lyu, C., Zhang, Y., Gu, M., Huang, Y., Liu, G., Wang, C., et al. (2018). IRAK-M deficiency exacerbates ischemic neurovascular injuries in experimental stroke mice. Front. Cell. Neurosci. 12:504. doi: 10.3389/fncel.2018.00504
Marks, D., Wonderling, D., Thorogood, M., Lambert, H., Humphries, S. E., and Neil, H. A. W. (2002). Cost effectiveness analysis of different approaches of screening for familial hypercholesterolaemia. BMJ 324:1303. doi: 10.1136/bmj.324.7349.1303
Masana, L., Ibarretxe, D., Rodríguez-Borjabad, C., Plana, N., Valdivielso, P., Pedro-Botet, J., et al. (2019). Toward a new clinical classification of patients with familial hypercholesterolemia: one perspective from Spain. Atherosclerosis 287, 89–92. doi: 10.1016/j.atherosclerosis.2019.06.905
Monaco, C., Gregan, S. M., Navin, T. J., Foxwell, B. M. J., Davies, A. H., and Feldmann, M. (2009). Toll-like receptor-2 mediates inflammation and matrix degradation in human atherosclerosis. Circulation 120, 2462–2469. doi: 10.1161/CIRCULATIONAHA.109.851881
Morrison, A. C., Bray, M. S., Folsom, A. R., and Boerwinkle, E. (2002). ADD1 460W allele associated with cardiovascular disease in hypertensive individuals. Hypertension 39, 1053–1057. doi: 10.1161/01.HYP.0000019128.94483.3A
Nanchen, D., Gencer, B., Auer, R., Räber, L., Stefanini, G. G., Klingenberg, R., et al. (2015). Prevalence and management of familial hypercholesterolaemia in patients with acute coronary syndromes. Eur. Heart J. 36, 2438–2445. doi: 10.1093/eurheartj/ehv289
Naoumova, R. P., Thompson, G. R., and Soutar, A. K. (2004). Current management of severe homozygous hypercholesterolaemias. Curr. Opin. Lipidol. 15:413. doi: 10.1097/01.mol.0000137222.23784.2a
Perez-Calahorra, S., Laclaustra, M., Marco-Benedí, V., Lamiquiz-Moneo, I., Pedro-Botet, J., Plana, N., et al. (2019). Effect of lipid-lowering treatment in cardiovascular disease prevalence in familial hypercholesterolemia. Atherosclerosis 284, 245–252. doi: 10.1016/j.atherosclerosis.2019.02.003
Prodan Žitnik, I., Černe, D., Mancini, I., Simi, L., Pazzagli, M., Di Resta, C., et al. (2018). Personalized laboratory medicine: a patient-centered future approach. Clin. Chem. Lab. Med. 56, 1981–1991. doi: 10.1515/cclm-2018-0181
Rahman, K. M. T., Islam, M. F., Banik, R. S., Honi, U., Diba, F. S., Sumi, S. S., et al. (2013). Changes in protein interaction networks between normal and cancer conditions: total chaos or ordered disorder? Netw. Biol. 3:15. doi: 10.1145/3064650.3064653
Režen, T., Cvikl, A., Brecelj, N., Rozman, D., Keber, I., and Fon Tacer, K. (2008). Atherosclerotic Markers in Human Blood - A Study in Patients With Familial Hypercholesterolemia. GEO Accession Viewer. Available at: https://www.ncbi.nlm.nih.gov/geo/query/acc.cgi?acc=GSE13985 (accessed November 10, 2019).
Ritchie, M. E., Phipson, B., Wu, D., Hu, Y., Law, C. W., Shi, W., et al. (2015). limma powers differential expression analyses for RNA-sequencing and microarray studies. Nucleic Acids Res. 43:e47. doi: 10.1093/nar/gkv007
Russo, G., Zegar, C., and Giordano, A. (2003). Advantages and limitations of microarray technology in human cancer. Oncogene 22:6497. doi: 10.1038/sj.onc.1206865
Shannon, P., Markiel, A., Ozier, O., Baliga, N. S., Wang, J. T., Ramage, D., et al. (2003). Cytoscape: a software environment for integrated models of biomolecular interaction networks. Genome Res. 13, 2498–2504. doi: 10.1101/gr.1239303
Sharan, R., Ulitsky, I., and Shamir, R. (2007). Network-based prediction of protein function. Mol. Syst. Biol. 3:88. doi: 10.1038/msb4100129
Silverman, M. G., Ference, B. A., Im, K., Wiviott, S. D., Giugliano, R. P., Grundy, S. M., et al. (2016). Association between lowering LDL-C and cardiovascular risk reduction among different therapeutic interventions: a systematic review and meta-analysis. JAMA 316, 1289–1297. doi: 10.1001/jama.2016.13985
Singh, R. K., Haka, A. S., Asmal, A., Barbosa-Lorenzi, V. C., Grosheva, I., Chin, H. F., et al. (2020). TLR4 (Toll-Like Receptor 4)-dependent signaling drives extracellular catabolism of LDL (Low-Density Lipoprotein) aggregates. Arterioscler. Thromb. Vasc. Biol. 40, 86–102. doi: 10.1161/ATVBAHA.119.313200
Sjouke, B., Kusters, D. M., Kindt, I., Besseling, J., Defesche, J. C., Sijbrands, E. J. G., et al. (2015). Homozygous autosomal dominant hypercholesterolaemia in the Netherlands: prevalence, genotype–phenotype relationship, and clinical outcome. Eur. Heart J. 36, 560–565. doi: 10.1093/eurheartj/ehu058
Slack, J. (1969). Risks of ischaemic heart-disease in familial hyperlipoproteinaemic states. Lancet 294, 1380–1382. doi: 10.1016/S0140-6736(69)90930-1
Smolock, E. M., Korshunov, V. A., Glazko, G., Qiu, X., Gerloff, J., and Berk, B. C. (2012). Ribosomal protein L17, RpL17, is an inhibitor of vascular smooth muscle growth and carotid intima formation. Circulation 126:2418. doi: 10.1161/CIRCULATIONAHA.112.125971
Smyth, G. K. (2005). “limma: linear models for microarray data,” in Bioinformatics and Computational Biology Solutions Using R and Bioconductor Statistics for Biology and Health, eds R. Gentleman, V. J. Carey, W. Huber, R. A. Irizarry, and S. Dudoit (New York, NY: Springer), 397–420. doi: 10.1007/0-387-29362-0_23
Soutar, A. K., and Naoumova, R. P. (2007). Mechanisms of disease: genetic causes of familial hypercholesterolemia. Nat. Clin. Pract. Cardiovasc. Med. 4, 214–225. doi: 10.1038/ncpcardio0836
Stone, N. J., Levy, R. I., Fredrickson, D. S., and Verter, J. (1974). Coronary artery disease in 116 kindred with familial type II hyperlipoproteinemia. Circulation 49, 476–488. doi: 10.1161/01.CIR.49.3.476
Stone, N. J., Robinson, J. G., Lichtenstein, A. H., Bairey Merz, C. N., Blum, C. B., Eckel, R. H., et al. (2014). 2013 ACC/AHA guideline on the treatment of blood cholesterol to reduce atherosclerotic cardiovascular risk in adults: a report of the american college of cardiology/american heart association task force on practice guidelines. J. Am. Coll. Cardiol. 63, 2889–2934. doi: 10.1016/j.jacc.2013.11.002
Szklarczyk, D., Gable, A. L., Lyon, D., Junge, A., Wyder, S., Huerta-Cepas, J., et al. (2019). STRING v11: protein–protein association networks with increased coverage, supporting functional discovery in genome-wide experimental datasets. Nucleic Acids Res. 47, D607–D613. doi: 10.1093/nar/gky1131
Szklarczyk, D., Morris, J. H., Cook, H., Kuhn, M., Wyder, S., Simonovic, M., et al. (2017). The STRING database in 2017: quality-controlled protein–protein association networks, made broadly accessible. Nucleic Acids Res. 45, D362–D368. doi: 10.1093/nar/gkw937
Taylor, F., Huffman, M. D., Macedo, A. F., Moore, T. H. M., Burke, M., Davey Smith, G., et al. (2013). Statins for the primary prevention of cardiovascular disease. Cochrane Database Syst. Rev. 1:CD004816. doi: 10.1002/14651858.CD004816.pub5
Tufanli, O., Telkoparan Akillilar, P., Acosta-Alvear, D., Kocaturk, B., Onat, U. I., Hamid, S. M., et al. (2017). Targeting IRE1 with small molecules counteracts progression of atherosclerosis. Proc. Natl. Acad. Sci. U.S.A. 114, E1395–E1404. doi: 10.1073/pnas.1621188114
Udhaya Kumar, S., Thirumal Kumar, D., Siva, R., George Priya Doss, C., Younes, S., Younes, N., et al. (2020). Dysregulation of signaling pathways due to differentially expressed genes from the B-cell transcriptomes of systemic lupus erythematosus patients – a bioinformatics approach. Front. Bioeng. Biotechnol. 8:276. doi: 10.3389/fbioe.2020.00276
Vuorio, A., Docherty, K. F., Humphries, S. E., Kuoppala, J., and Kovanen, P. T. (2013). Statin treatment of children with familial hypercholesterolemia – Trying to balance incomplete evidence of long-term safety and clinical accountability: are we approaching a consensus? Atherosclerosis 226, 315–320. doi: 10.1016/j.atherosclerosis.2012.10.032
Vuorio, A., Tikkanen, M. J., and Kovanen, P. T. (2014). Inhibition of hepatic microsomal triglyceride transfer protein – a novel therapeutic option for treatment of homozygous familial hypercholesterolemia. Vasc. Health Risk Manag. 10, 263–270. doi: 10.2147/VHRM.S36641
Vuorio, A., Watts, G. F., and Kovanen, P. T. (2017). Depicting new pharmacological strategies for familial hypercholesterolaemia involving lipoprotein (a). Eur. Heart J. 38, 3555–3559. doi: 10.1093/eurheartj/ehx546
Wald, D. S., Bestwick, J. P., Morris, J. K., Whyte, K., Jenkins, L., and Wald, N. J. (2016). Child–parent familial hypercholesterolemia screening in primary care. New Engl. J. Med. 375, 1628–1637. doi: 10.1056/NEJMoa1602777
Walter, W., Sánchez-Cabo, F., and Ricote, M. (2015). GOplot: an R package for visually combining expression data with functional analysis. Bioinformatics 31, 2912–2914. doi: 10.1093/bioinformatics/btv300
Wang, H. X., and Zhao, Y. X. (2016). Prediction of genetic risk factors of atherosclerosis using various bioinformatic tools. Genet. Mol. Res. 15:gmr7347. doi: 10.4238/gmr.15027347
Warde-Farley, D., Donaldson, S. L., Comes, O., Zuberi, K., Badrawi, R., Chao, P., et al. (2010). The GeneMANIA prediction server: biological network integration for gene prioritization and predicting gene function. Nucleic Acids Res. 38, W214–W220. doi: 10.1093/nar/gkq537
Yu, L., and Feng, Z. (2018). The role of toll-like receptor signaling in the progression of heart failure. Mediators Inflamm. 2018, 1–15. doi: 10.1155/2018/9874109
Yu, M., Zhou, H., Zhao, J., Xiao, N., Roychowdhury, S., Schmitt, D., et al. (2014). MyD88-dependent interplay between myeloid and endothelial cells in the initiation and progression of obesity-associated inflammatory diseasesMyD88 in obesity-associated inflammatory diseases. J. Exp. Med. 211, 887–907. doi: 10.1084/jem.20131314
Keywords: familial hypercholesterolemia, atherosclerosis, coronary artery disease, functional enrichment analysis, expression profiling data, gene expression arrays
Citation: Udhaya Kumar S, Thirumal Kumar D, Bithia R, Sankar S, Magesh R, Sidenna M, George Priya Doss C and Zayed H (2020) Analysis of Differentially Expressed Genes and Molecular Pathways in Familial Hypercholesterolemia Involved in Atherosclerosis: A Systematic and Bioinformatics Approach. Front. Genet. 11:734. doi: 10.3389/fgene.2020.00734
Received: 04 March 2020; Accepted: 17 June 2020;
Published: 15 July 2020.
Edited by:
Alpo Juhani Vuorio, University of Helsinki, FinlandReviewed by:
Rodrigo Alonso, Las Condes Clinic, ChileTeresa Padro, Sant Pau Institute for Biomedical Research, Spain
Chiara Di Resta, Vita-Salute San Raffaele University, Italy
Copyright © 2020 Udhaya Kumar, Thirumal Kumar, Bithia, Sankar, Magesh, Sidenna, George Priya Doss and Zayed. This is an open-access article distributed under the terms of the Creative Commons Attribution License (CC BY). The use, distribution or reproduction in other forums is permitted, provided the original author(s) and the copyright owner(s) are credited and that the original publication in this journal is cited, in accordance with accepted academic practice. No use, distribution or reproduction is permitted which does not comply with these terms.
*Correspondence: C. George Priya Doss, Z2VvcmdlcHJpeWFkb3NzQHZpdC5hYy5pbg==; Hatem Zayed, aGF0ZW0uemF5ZWRAcXUuZWR1LnFh