- 1Hunan Cancer Hospital/The Affiliated Cancer Hospital of Xiangya School of Medicine, Central South University, Changsha, China
- 2Department of Medicine, University of South China, Hengyang, China
In developing countries, cervical cancer is still the major cause of cancer-related death among women. To better understand the correlation between tumor microenvironment (TME) and prognosis of cervical cancer, we screened 1367 differentially expressed genes (DEGs) of cervical cancer samples in The Cancer Genome Atlas (TCGA) database using Estimation of STromal and Immune cells in MAlignant Tumor tissues using Expression data (ESTIMATE) algorithm-derived immune scores. Then, we extracted 401 tumor immune microenvironment (TIME)-related DEGs that related to patients’ survival outcomes. Protein-protein interaction (PPI) network and functional enrichment analysis revealed that the prognostic genes mainly participated in myeloid leukocyte activation, adaptive immune response regulation, and receptor signaling pathways. A total of 79 key prognostic DEGs were obtained through PPI network. A TF-lncRNA-miRNA-mRNA regulatory network was constructed to explore the potential regulatory mechanism. 4 genes (CCR7, PD-1, ZAP70, and CD28) were validated in another independent cohort of cervical cancer from the Gene Expression Omnibus (GEO) database. Finally, potential drugs for key prognostics DEGs were predicted using DrugBank. In conclusion, we obtained a list of potential prognostic TIME-related genes and potential predicted drugs by integrative bioinformatics approaches. A comprehensive understanding of prognostic genes within the TIME may provide new strategies for cervical cancer treatment.
Introduction
In developing countries, cervical cancer is still the major cause of cancer-related death among women (Arbyn et al., 2020). Nearly all cervical cancers are associated with human papillomavirus (HPV) infection (Berman and Schiller, 2017). Although significant progress has been achieved in screening and prevention, the 5-year overall survival (OS) rate for cervical cancer remains around 60% (McLachlan et al., 2017). Radiotherapy and chemotherapy are standard therapies for advanced-stage patients (Wui-Jin et al., 2019), but with limited success. Recently, remarkable progress in cervical cancer immunotherapy has been made, but positive responses only occur in a small fraction of patients. Such responses are usually dependent on dynamic interactions between tumor cells and other factors within the tumor microenvironment (TME).
Tumor microenvironment contains tumor cells and the surrounding blood vessels, signaling molecules, immune cells, and fibroblasts (Joyce and Fearon, 2015; Spill et al., 2016), etc. The TME can critically influence gene expression in cancer tissues, and is gradually recognized as a key contributor to cancer progression and drug resistance (Piersma, 2011; Pasini et al., 2014; Kim et al., 2016; Petitprez et al., 2018; Li et al., 2019; Looi et al., 2019). Cancer cells can create a full range of immunosuppression in the TME to counter the body’s anti-tumor immunity and achieve immune escape (Teng et al., 2015). Several studies have suggested that tumor-associated macrophages (TAMs), matrix metalloproteinase, transforming growth factor-beta, and interleukin (IL)-2 play key roles in cervical cancer progression and are associated with cancer cell invasion and dissemination ability (Valle-Mendiola et al., 2016; Zhu et al., 2016; Ng et al., 2019; Wang et al., 2019a). A deep understanding of the correlation between TME and prognosis, and exploring new strategies for the treatment are urgently needed for precise therapy improvement of cervical cancer.
With the rapid development of public databases and second-generation sequencing technologies, comprehensive analysis for TME-related prognostic genes has become possible. The Estimation of STromal and Immune cells in MAlignant Tumor tissues using Expression data (ESTIMATE) algorithm (Yoshihara et al., 2013) was developed to predict infiltrating immune and stromal cells within tumor tissues using gene expression data in The Cancer Genome Atlas (TCGA) database. Subsequent studies have involved the ESTIMATE algorithm to glioblastomas (Jia et al., 2018), renal cell carcinomas (Xu et al., 2019), and colon cancers (Alonso et al., 2017). However, the utility of the ESTIMATE algorithm in cervical cancer has not been previously investigated. In this study, we screened the expression and interaction of TME-related differentially expressed genes (DEGs) in cervical cancer, predicted their regulatory network, and evaluated the potential therapeutic drugs based on several large public databases (Figure 1). The results might provide useful clues for prospective treatment strategies of cervical cancer.
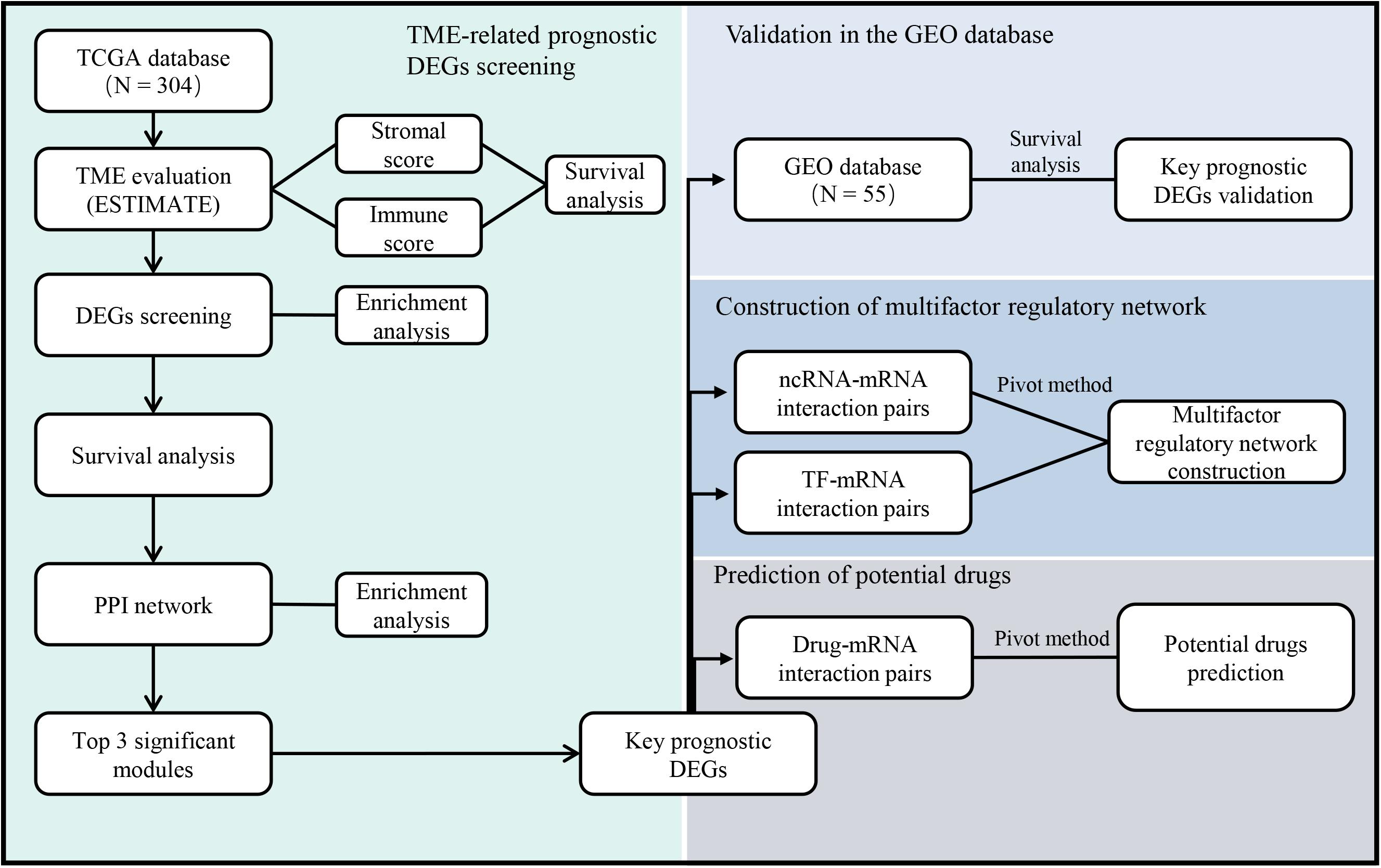
Figure 1. The workflow of the current study. TCGA = The Cancer Genome Atlas, GEO = Gene Expression Omnibus, TME = Tumor microenvironment, DEGs = Differentially expressed genes, PPI = Protein-protein interaction, ncRNA = non-coding RNA, TF = Transcription factor.
Materials and Methods
Data
Gene expression profiles and related clinical materials for cervical cancer were downloaded from the TCGA data portal (Tomczak et al., 2015). The inclusion criteria were (Arbyn et al., 2020) pathologically confirmed cervical cancer, (Berman and Schiller, 2017) complete RNA expression data from the patients, and (McLachlan et al., 2017) complete ESTIMATE score, immune score, and stromal score (Yoshihara et al., 2013).
For further verification, gene expression profiles and clinical materials of another cohort of cervical cancer patients were downloaded from the Gene Expression Omnibus (GEO) database (GSE52903) (Medina-Martinez et al., 2014). In addition, we also used an online web server (OScc) to verify the prognostic value of targeted genes (Wang et al., 2019b).
Identification of DEGs and Functional Enrichment Analysis
Data analysis was conducted using package limma in R language (version 3.4.0) (Ritchie et al., 2015). A fold change (FC) > 2 and adjusted p-value < 0.05 were set up to screen DEGs. Heat maps were generated by pheatmap package in R (Kolde and Kolde, 2015).
Through the Search Tool for Retrieval of Interacting Genes/Proteins (STRING) database (version 11.0), functional enrichment analysis was conducted to identify gene ontology (GO) annotation (Szklarczyk et al., 2019) and Kyoto Encyclopedia of Genes and Genomes (KEGG) pathways. P < 0.05 was considered to be statistically significant.
Survival Analysis
Using the survival and survminer package in R, Kaplan-Meier plots and log-rank tests were performed to elucidate the relationship between 5-year overall survival (OS) rates and DEGs expression levels. Univariate Cox regression was used to assess the effect of clinical parameters and mRNA expression on the survival of cervical cancer patients. P < 0.05 was considered to be statistically significant.
Protein-Protein Interaction (PPI) Network Building and Gene Set Enrichment Analysis (GSEA)
The PPI network was extracted from the STRING database and visualized by Cytoscape software (version 3.4.0) (Shannon et al., 2003). To identify densely connected regions, Molecular COmplex Detection (MCODE) in Cytoscape was then involved to extract topology-based clusters.
Using the STRING database and GSEA method, we further retrieved a functional profile of the gene set derived from the PPI network (Mootha et al., 2003; Subramanian et al., 2005). P < 0.05 was considered to be statistically significant.
Extraction of microRNA (miRNA), Long Non-coding RNA (lncRNA), Transcription Factor (TF), and Drug Interactions
We obtained the miRNA – mRNA and lncRNA-mRNA interactions from the RNA Interactome (RNAInter) database (version RNAInter in 2020) (Lin et al., 2019), TF-mRNA interactions from the Transcriptional Regulatory Relationships Unraveled by Sentence-based Text mining (TRRUST) database (version 2.0) (Han et al., 2017), and drug-mRNA interactions from the DrugBank database (version 5.1.1) (Law et al., 2014). RNAInter, TRRUST V2 and DrugBank include the curated confirmed interactions from the literatures.
To construct a muti-factor regulator network, we extracted miRNAs, lncRNAs, TFs, and drugs that had interactions with obtained genes.
Pivot Method
We further screened pivot nodes from obtained interaction pairs using the phyper() function in R. The pivot node refers to at least two interacting pairs between the node and a gene, and the significance analysis p-value of the interaction between the node and the gene set should be <0.05 by the hypergeometric test (Wu et al., 2015). The obtained pivot miRNAs-mRNAs, lncRNAs-mRNAs, and TFs-mRNAs interactions were visualized by Cytoscape. Pivot drugs-mRNA interactions pairs were also analyzed.
Results
Immune Scores Are Significantly Associated With HPV Infection, Histological Type, and Patients’ Survival
Among 304 cases in TCGA, 253 (83.2%) were squamous carcinomas, 47 (15.5%) adenocarcinomas, and 4 (1.3%) adenosquamous carcinomas (Supplementary Table 1). Based on the ESTIMATE algorithm, the median of stromal scores was -1047.855 (-2586.99 to 778.01), and the median of immune scores was -246.78 (-1645.63 to 3295.3) (Figure 2A). HPV-positive cases had higher immune scores than HPV-negative cases (p < 0.001) (Figure 2B). Cases of squamous carcinoma had significantly higher immune scores and stromal scores than cases of adenocarcinoma (p < 0.01) (Figures 2C,D).
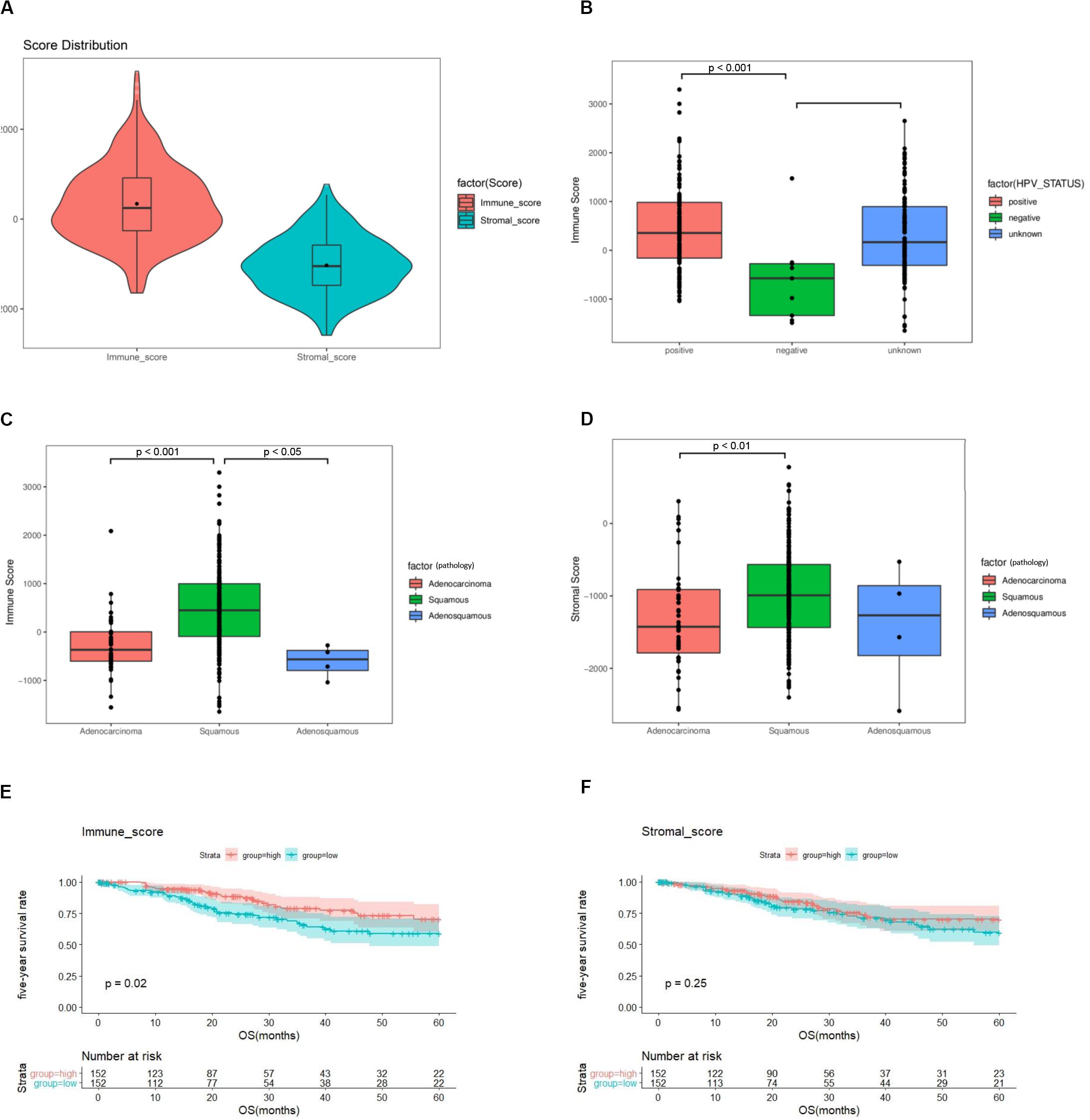
Figure 2. The immune score and stromal score are associated with clinicopathologic characteristics and overall survival of cervical cancer patients. (A) Distribution of immune scores and stromal scores among 304 cervical cancer samples in TCGA. (B) Distribution of immune scores among HPV-negative and HPV-positive cases. (C) Distribution of immune scores among cervical cancer subtypes. (D) Distribution of stromal scores among cervical cancer subtypes. (E) A higher immune score is associated with better overall survival (p = 0.02). (F) Stromal score is not associated with overall survival (p = 0.25). TCGA = The Cancer Genome Atlas, GEO = Gene Expression Omnibus, HPV = Human papillomavirus.
To assess the potential relationship of stromal and immune scores with patients’ outcome, a total of 304 cervical cancer cases were categorized into high-score and low-score groups by the median expression value. The results revealed that patients with high immune scores had a better survival outcome than those with low scores (p = 0.02) (Figure 2E). There was no difference in survival outcomes between the two stromal-score groups (p = 0.25) (Figure 2F).
DEG Screening and Functional Analysis Between Low- and High-Immune Score Groups
To determine the relationship between global gene expression profiles and immune scores, 1367 DEGs between the two immune-score groups were identified, including 488 downregulated genes and 879 upregulated genes (Figure 3A).
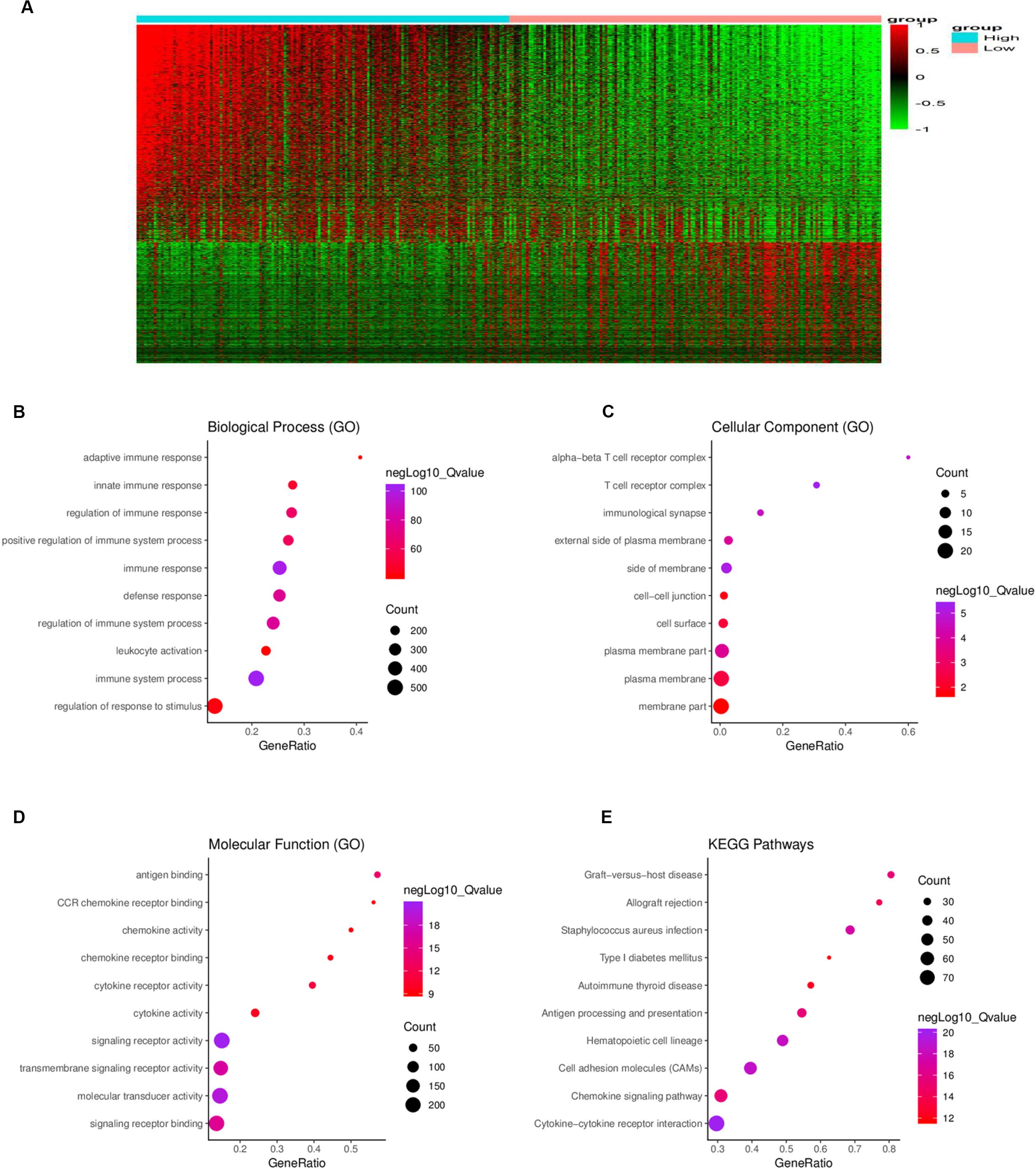
Figure 3. Comparison of gene expression profiles between the high- and low-immune score groups. (A) In the heat maps, genes with higher expression are shown in red, and genes with lower expression are shown in green; genes expressed at the same level are in black. A total of 879 genes were upregulated and 488 genes downregulated in the high-score group as compared to the low-score group. Biological process (B), cellular component (C), and molecular function (D) in gene ontology (GO) analysis for 1367 DEGs. (E) Kyoto Encyclopedia of Genes and Genomes (KEGG) analysis for 1367 DEGs. DEG = differentially expressed gene.
To further understand the potential biological function of the DEGs, GO annotation and KEGG pathway were analyzed. GO analysis showed that the DEGs were mainly enriched in ingredients such as immunological synapse and T cell receptor complex, and mainly enriched in processes such as immune system process and regulation of immune system process, signaling receptor binding, and leukocyte activation (Figures 3B–D). KEGG pathway enrichment analysis demonstrated that the DEGs were mainly associated with antigen processing and presentation, cytokine-cytokine receptor interaction, chemokine signaling pathway and cell adhesion molecules, etc. (Figure 3E).
Kaplan–Meier plots were further performed for 1367 DEGs. A total of 401 DEGs were significantly related to the 5-year OS (Figure 4) (Supplementary Table 2).
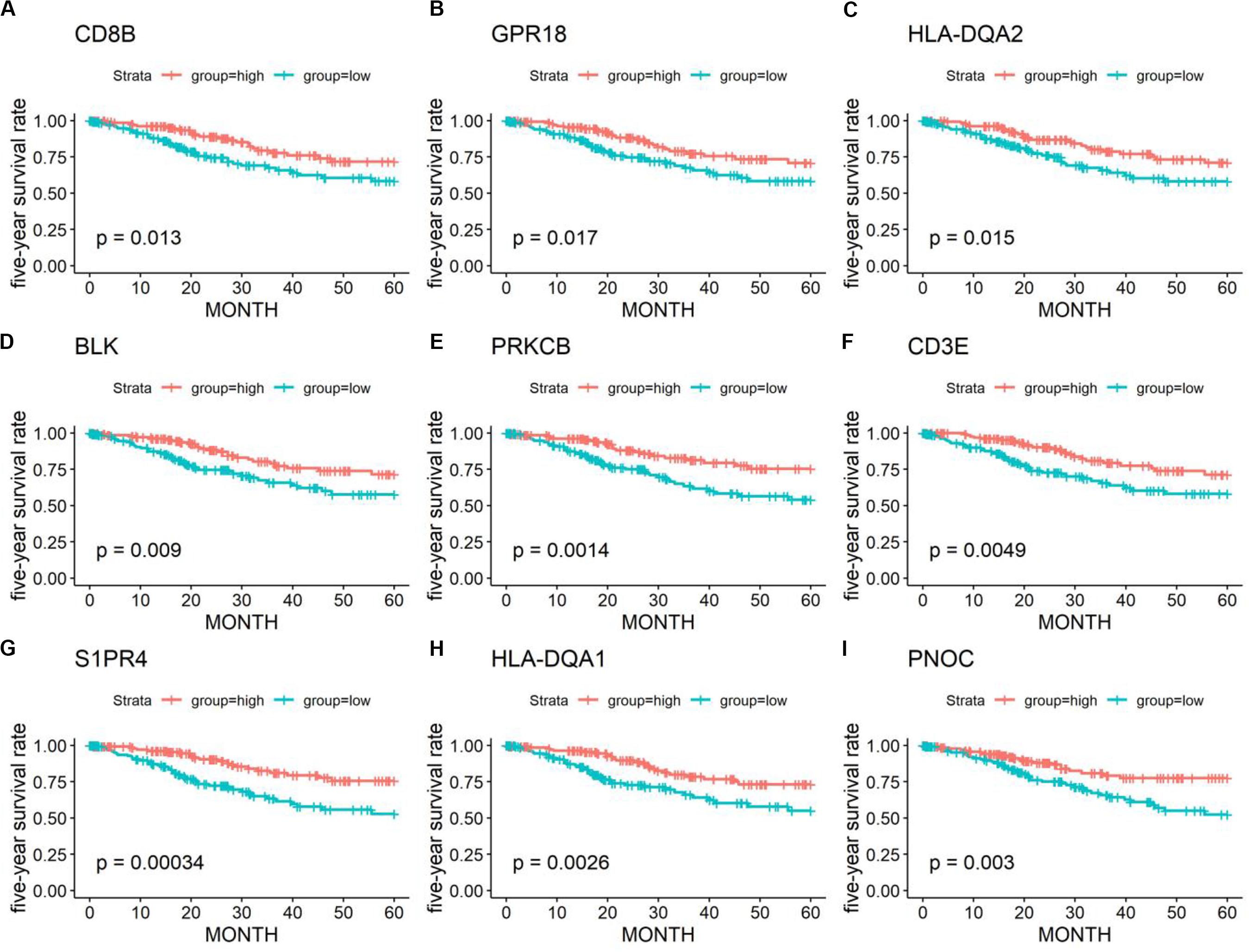
Figure 4. Kaplan–Meier survival curves and log-rank tests showing the correlation of partial immune-related genes (A–I) with the 5-year survival rates. High gene expression (red line) was correlated with better outcomes in cervical cancer patients.
PPI Networks Construction and Functional Enrichment Analysis
To examine the interplay among the prognostic DEGs, we built a PPI network, which was made up of 15 modules and comprised 228 nodes and 1041 edges (Figure 5A). GSEA was used to clarify the main biological functions of 228 node genes. The results showed that they were mainly associated with myeloid leukocyte activation, adaptive immune response regulation, and receptor signaling pathways (Figure 5B).
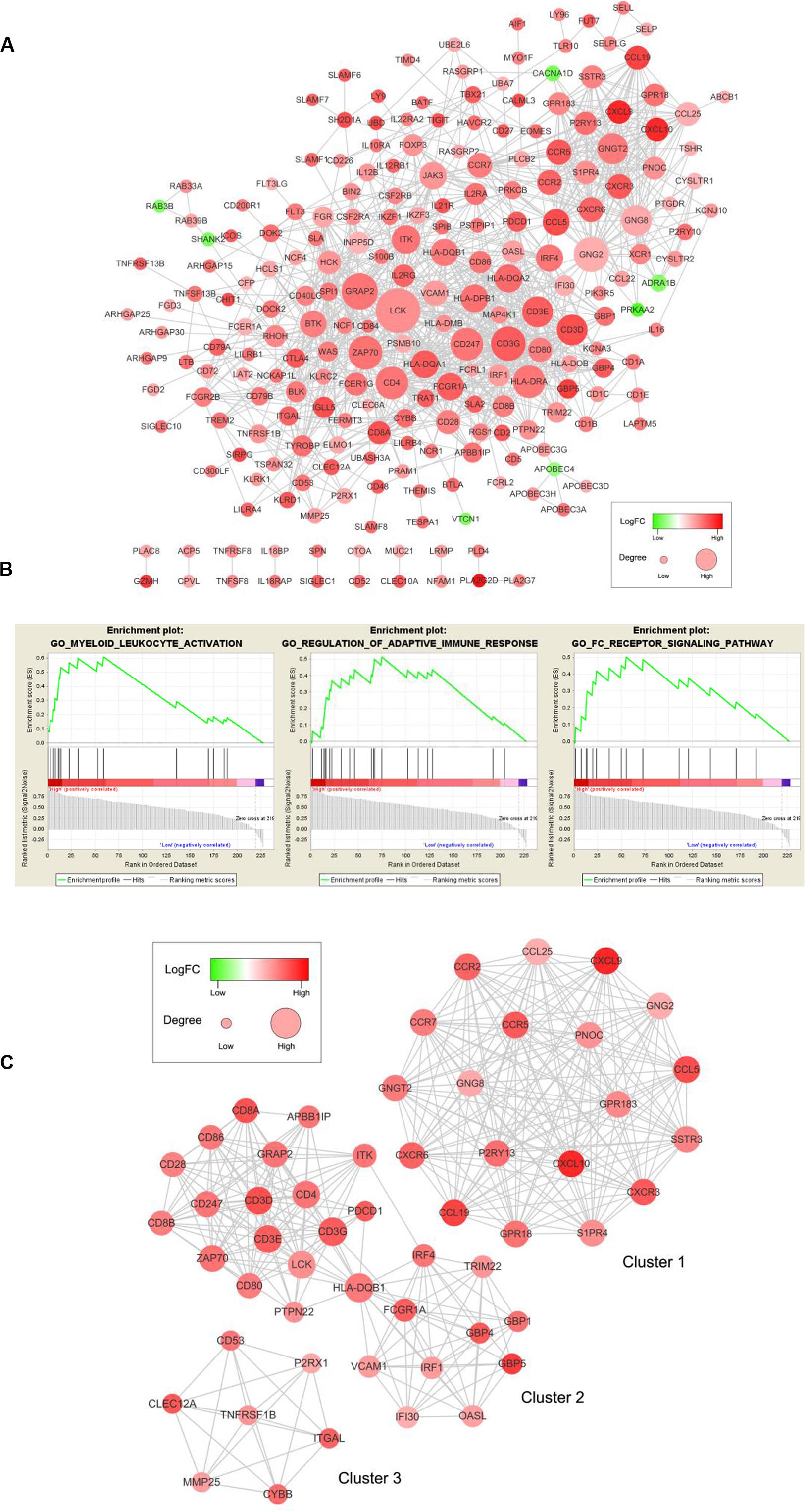
Figure 5. PPI network among prognostic genes and functional enrichment analysis. (A) The PPI network was made up of 15 modules and included 228 nodes and 1041 edges. The color of a node in the PPI network reflects the log (FC) value of the Z score of gene expression, and the size of the node indicates the number of proteins interacting with the designated protein. (B) Enrichment profiles generated with GSEA for the gene set in the PPI network. (C) Top three modules in the PPI network. PPI = protein-protein interaction, FC = Fold change, GSEA = gene set enrichment analysis.
We selected the top three significant modules for further analysis and named these modules cluster 1, cluster 2, and cluster 3 (Figure 5C). Cluster 1 had 171 edges and 19 nodes in the network. In cluster 2, HLA-DQB1, CD3G, CD3D, CD4, CD3E, LCK, and ZAP70, which are critical to the immune response, had higher degree values. In cluster 3, TNFRSF1B, which is crucial to immune and inflammatory procession (Croft, 2009), occupied the module center.
After selecting from the three modules and PPI networks with ≥10 node degrees, we obtained 79 key prognostic DEGs (Supplementary Table 3).
Validation of Key Prognostic DEGs in the GEO Database
We further validated 79 key DEGs in another cohort of 55 cervical cancer patients from the GEO database. Finally, high expressions of four genes (CCR7, programmed cell death-1 [PD-1], ZAP70, and CD28) was found to be associated with better 5-year OS in both GEO (Figures 6A,B) and OScc (Supplementary Figure 1). In univariate analysis, high expression of CCR7, PD-1, and ZAP70 were related to better survival outcome in both TCGA and GEO (Supplementary Table 4).
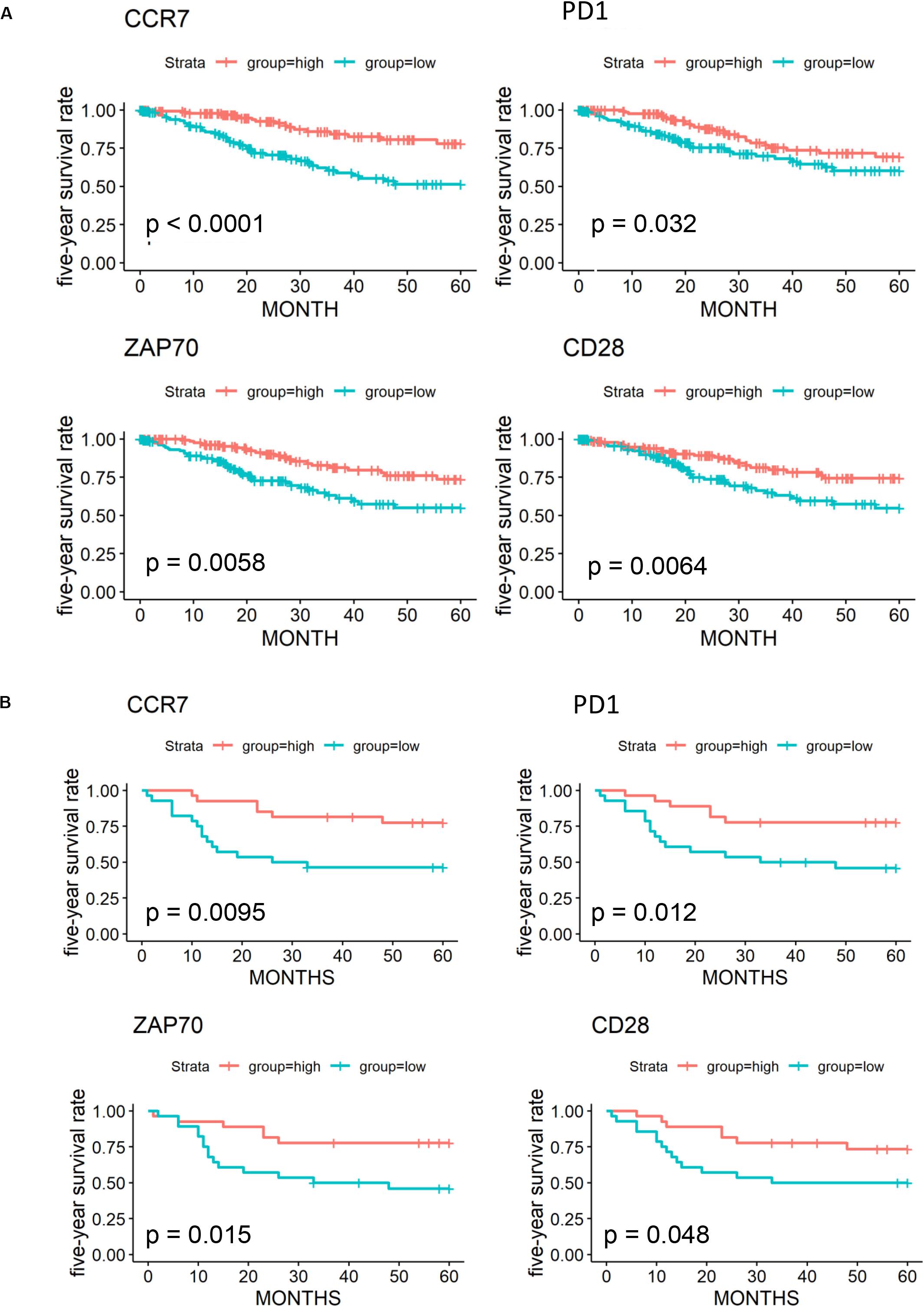
Figure 6. Validation of 79 key prognostic DEGs in an independent GEO cohort. (A) Kaplan-Meier plots and log-rank tests in the TCGA cohort were performed for 4 validated prognostic DEGs based on high (red line) and low (blue line) gene expression. (B) Kaplan–Meier plots and log-rank tests in the GEO cohort were performed for 4 validated prognostic DEGs.
Construction of a Multifactor Regulatory Network Based on Key Prognostic DEGs
We extracted interaction pairs of miRNAs, lncRNAs, and TFs with 79 key DEGs and constructed a multifactor regulatory network. The network contained 2295 nodes and 7678 edges (2058 miRNA nodes, 79 lncRNA nodes, 192 TF nodes, and 76 mRNA nodes). To acquire nodes with greater influence on the network, the network was pruned using the pivot method and visualized with Cytoscape. The final network including 31 pivot lncRNAs, 148 pivot miRNAs, 21 pivot TFs, and 75 pivot mRNAs, was identified (Figure 7).
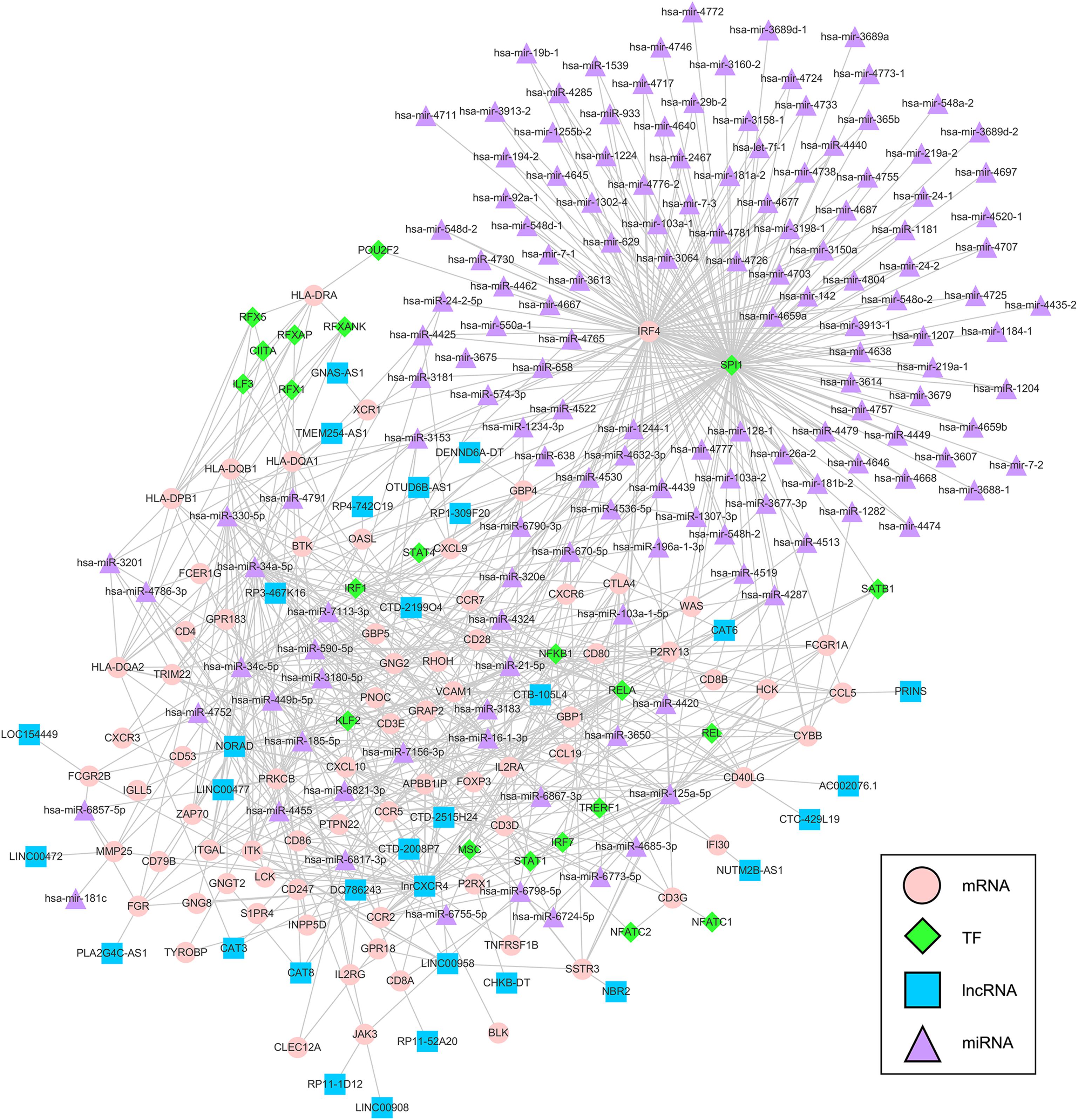
Figure 7. Multifactor regulatory network of key prognostic DEGs. The network was composed of 148 pivot miRNAs, 31 pivot lncRNAs, 21 pivot TFs, and 75 pivot mRNAs. TF = transcription factor, lncRNA = long non-coding RNAs, miRNA = microRNA.
Identification of Potential Predictive Drugs
From DrugBank, we obtained 25020 drug-mRNA interaction pairs. A total of 79 key DEGs were then inputted into the database to predict the potential drugs of the genes, and 149 drug-mRNA interactions were extracted. Pivot method was used to simplify the obtained drugs and a total of 39 pivot drugs were predicted (Table 1). For example, Bevacizumab and cetuximab has been reported to target FCGR1A and FCGR2B (Imming et al., 2006; Bogdanovich et al., 2016), and these 2 drugs (Moore et al., 2012; Zighelboim et al., 2013; Tewari et al., 2014; Penson et al., 2015) have already been approved for clinical treatment of cervical cancer.
Discussion
Cervical cancer treatment has suffered rapid progress in the past decade. It moves away from drugs that attack tumors broadly toward precise immunotherapy that regulates immune responses against tumors. Identifying effective biomarkers related to tumor immune microenvironment (TIME) and prognosis are urgently needed for better patient management.
By ESTIMATE algorithm, we first found that high immune scores were related to better OS, which is consistent with the results of previous studies showing that immune cells infiltrating the tumor tissue may inhibit cancer cells (Cho et al., 2014; Gorter et al., 2015). The study also found HPV-positive cases had higher immune scores than HPV-negative cases, which might be associated with HPV-related microenvironment components regulation, such as increase of regulatory immune responses and decrease of effector immune responses (Zhou et al., 2019). A total of 1367 DEGs between the low- and high-immune score groups were identified, and 401 DEGs among them were related to survival outcomes of cervical cancer patients. These genes affect the outcomes of patients mainly by regulating TIME-related biological functions, including immune response regulation, leukocyte activation, chemokine activities, and integrin binding. These processes may shape tumor development and anti-cancer immunity, thus improving prognosis (Jochems and Schlom, 2011; Engblom et al., 2016; Böttcher et al., 2018).
A PPI network for 401 prognostic DEGs was constructed to reveal the interplay between DEGs, and 228 node genes were confirmed. The top modules that we selected from the PPI network have been reported to influence angiogenesis, proliferation, invasiveness, and therapeutic efficacy in cervical cancer (Yang et al., 2012; Zhang et al., 2015, 2018; Zhao et al., 2015; Che et al., 2016). The GSEA results showed that 228 node genes were highly associated with myeloid leukocyte activation, adaptive immune response regulation, and receptor signaling pathways. Silveira et al. reported that proliferation and accumulation of myeloid-derived suppressor cells might worsen cervical cancer progression and strong infiltration of CD14-positive myeloid cells might prolong survival in cervical cancer patients (Garcia et al., 2004; de Vos van Steenwijk et al., 2013).
By cross-validation with an independent GEO cohort, we identified four prognostic immune-related genes (CCR7, CD28, PD-1, and ZAP70). In previous studies, PD-1 expression was only found on the surface of immune cells, while programmed death receptor ligand-1 (PD-L1) was on cervical cancer cells. Their interaction played critical a role in tumor immune escape (Antoni, 2012). Monoclonal antibodies targeting PD-1/programmed death ligand, such as pembrolizumab, have already been widely assessed in clinical trials and are currently approved for the treatment of advanced cervical cancer (Borcoman and Le Tourneau, 2017; Chen et al., 2017; Chung et al., 2019; Dyer et al., 2019). Interestingly, in our results, higher expression of PD-1 was associated with better clinical outcomes. However, recent studies revealed a high intrinsic expression of PD-1 in most tumor cell lines (Yao et al., 2018). Combined with our functional enrichment analysis results of DEGs (myeloid leukocyte activation, adaptive immune response regulation, and receptor signaling pathways), we speculated that PD-1 expressed on tumor cells might have different functions, such as immune activation, other than that on immune cells. CCR7 has been reported to influence the lymph node metastasis of cervical cancer, prostate cancer cell migration, and mammary cancer cell stemness (Boyle et al., 2017; Dai et al., 2017; Maolake et al., 2018). Tyrosine kinase ZAP70 has been identified to play a key role in T cell activation and the immune response (Fu et al., 2016; Alsadeq et al., 2017; Laufer et al., 2018).
To explore the molecular mechanisms underlying the differential expression of these genes, we further constructed a TF-lncRNA-miRNA-mRNA regulatory network. We identified 148 pivot miRNAs, 31 pivot lncRNAs, 21 pivot TFs, and 75 pivot mRNAs. In addition, a total of 39 potential drugs for key prognostic DEGs were predicted. Bevacizumab was the first molecular antibody to show survival benefit in advanced cervical cancer, and it improved progression-free survival more than 3.7 months (Tewari et al., 2017). Cetuximab, an anti-epidermal growth factor receptor monoclonal antibody, is a standard option for the treatment of advanced cervical cancer (Meira et al., 2009). Fourteen drugs were identified, including catumaxomab, aldesleukin, trastuzumab, and ibritumomab tiuxetan, all of which have been confirmed for various cancers, including malignant ascites (Kietpeerakool et al., 2019), renal cell carcinoma (Fishman et al., 2019), gastric cancer (Kimura et al., 2018), and lymphoma (Lansigan et al., 2019), respectively. Among drug-interactions obtained, Staurosporine has been reported to target ZAP70 (Overington et al., 2006), but their interaction in cancer research is still blank.
One limitation of our study is that our predictions were based on analyses of online databases, so further experimental validation is needed. In future research, we will further explore the potential functions and signal pathways of the 79 DEGs (especially CCR7, CD28, PD-1, and ZAP70) within cervical cancer TIME. A deeper understanding of the complex molecular mechanism of TIME in cervical cancer may help explain the individual difference in immunotherapy efficiency and help explore new treatment strategies.
Conclusion
We identified 79 prognostic TIME-related genes in cervical cancer and validated 4 genes (CCR7, CD28, PD-1, and ZAP70). Additionally, a total of 39 potential predicted drugs targeting key prognostic genes were obtained, and they might provide new clues for future treatment management. Further investigation of these genes and related regulatory network might put novel insights into the cervical cancer immunotherapy and prognosis improvement in a comprehensive manner.
Data Availability Statement
Publicly available datasets were analyzed in this study, these can be found in The Cancer Genome Atlas (https://portal.gdc.cancer.gov/); the NCBI Gene Expression Omnibus (GSE52903).
Author Contributions
ZZ, N-YY, JC, and JW performed the data analysis work and aided in writing the manuscript. ZZ and JW designed the study and edited the manuscript. JL, HL, PO-Y, and SL assisted in writing the manuscript. All authors read and approved the final manuscript.
Funding
This work was supported by grants from the National Natural Science Foundation of China (81972636 and 2016YF103703) and the 13th Five-Year National Key R&D Program of the Ministry of Science and Technology (2016YFC1303703).
Conflict of Interest
The authors declare that the research was conducted in the absence of any commercial or financial relationships that could be construed as a potential conflict of interest.
Supplementary Material
The Supplementary Material for this article can be found online at: https://www.frontiersin.org/articles/10.3389/fgene.2020.00727/full#supplementary-material
Abbreviations
DEG, differentially expressed gene; ESTIMATE, Estimation of STromal and Immune cells in MAlignant Tumor tissues using Expression data; GEO, Gene Expression Omnibus; HPV, human papillomavirus; lncRNA, long non-coding RNAs; miRNA, microRNA; OS, overall survival; PPI, protein-protein interaction; TCGA, The Cancer Genome Atlas; TF, transcription factor; TIME, tumor immune microenvironment; TME, tumor microenvironment.
References
Alonso, M., Aussó, S., Lopez-Doriga, A., Cordero, D., Guinó, E., Solé, X., et al. (2017). Comprehensive analysis of copy number aberrations in microsatellite stable colon cancer in view of stromal component. J. Br. J. Cancer 117, 421–431. doi: 10.1038/bjc.2017.208
Alsadeq, A., Fedders, H., Vokuhl, C., Belau, N., Zimmermann, M., Wirbelauer, T., et al. (2017). The role of ZAP70 kinase in acute lymphoblastic leukemia infiltration into the central nervous system. J. Haematol. 102, 346–355. doi: 10.3324/haematol.2016.147744
Antoni, R. (2012). Tumor immunotherapy directed at PD-1. Cell 366, 2517–2519. doi: 10.1056/nejme1205943
Arbyn, M., Weiderpass, E., Bruni, L., de Sanjosé, S., Saraiya, M., Ferlay, J., et al. (2020). Estimates of incidence and mortality of cervical cancer in 2018: a worldwide analysis. Lancet Glob. Health 8, e191–e203. doi: 10.1016/s2214-109x(19)30482-6
Berman, T., and Schiller, J. (2017). Human papillomavirus in cervical cancer and oropharyngeal cancer: one cause, two diseases. J. Cancer 123, 2219–2229. doi: 10.1002/cncr.30588
Bogdanovich, S., Kim, Y., Mizutani, T., Yasuma, R., Tudisco, L., Cicatiello, V., et al. (2016). Human IgG1 antibodies suppress angiogenesis in a target-independent manner. Signal. Transduct. Target Ther. 1: 15001.
Borcoman, E., and Le Tourneau, C. (2017). Pembrolizumab in cervical cancer: latest evidence and clinical usefulness. J. Therap. Adv. Med. Oncol. 9, 431–439. doi: 10.1177/1758834017708742
Böttcher, J., Bonavita, E., Chakravarty, P., Blees, H., Cabeza-Cabrerizo, M., Sammicheli, S., et al. (2018). NK Cells stimulate recruitment of cDC1 into the tumor microenvironment promoting cancer immune control. J. Cell 172, 1022–1037.
Boyle, S., Gieniec, K., Gregor, C., Faulkner, J., McColl, S., and Kochetkova, M. (2017). Interplay between CCR7 and Notch1 axes promotes stemness in MMTV-PyMT mammary cancer cells. J. Mol. Cancer 16:19.
Che, L., Shao, S., and Wang, L. (2016). Downregulation of CCR5 inhibits the proliferation and invasion of cervical cancer cells and is regulated by microRNA-107. J. Exp. Therap. Med. 11, 503–509. doi: 10.3892/etm.2015.2911
Chen, Y., Zhang, Y., Lv, J., Li, Y., Wang, Y., He, Q., et al. (2017). genomic analysis of tumor microenvironment immune types across 14 solid cancer types: immunotherapeutic implications. J. Theranost. 7, 3585–3594. doi: 10.7150/thno.21471
Cho, H., Chung, J., Kim, S., Braunschweig, T., Kang, T., Kim, J., et al. (2014). MICA/B and ULBP1 NKG2D ligands are independent predictors of good prognosis in cervical cancer. BMC Cancer 14:957. doi: 10.1186/1471-2407-14-957
Chung, H., Ros, W., Delord, J., Perets, R., Italiano, A., Shapira-Frommer, R., et al. (2019). Efficacy and safety of pembrolizumab in previously treated advanced cervical cancer: results from the phase II KEYNOTE-158 study. J. Clin. Oncol. 37, 1470–1478. doi: 10.1200/jco.18.01265
Croft, M. (2009). The role of TNF superfamily members in T-cell function and diseases. J. Nat. Rev. Immunol. 9, 271–285. doi: 10.1038/nri2526
Dai, Y., Tong, R., Guo, H., Yu, T., and Wang, C. (2017). Association of CXCR4, CCR7, VEGF-C and VEGF-D expression with lymph node metastasis in patients with cervical cancer. J. Eur. J. Obstet. Gynecol. Reprod. Biol. 214, 178–183. doi: 10.1016/j.ejogrb.2017.04.043
de Vos van Steenwijk, P., Ramwadhdoebe, T., Goedemans, R., Doorduijn, E., van Ham, J., Gorter, A., et al. (2013). Tumor-infiltrating CD14-positive myeloid cells and CD8-positive T-cells prolong survival in patients with cervical carcinoma. J. Intern. J. Cancer 133, 2884–2894.
Dyer, B., Zamarin, D., Eskandar, R., and Mayadev, J. (2019). Role of immunotherapy in the management of locally advanced and recurrent/metastatic cervical cancer. J. Natl. Comprehens. Cancer Netw. 17, 91–97. doi: 10.6004/jnccn.2018.7108
Engblom, C., Pfirschke, C., and Pittet, M. (2016). The role of myeloid cells in cancer therapies. J. Nat. Rev. Cancer 16, 447–462. doi: 10.1038/nrc.2016.54
Fishman, M., Dutcher, J., Clark, J., Alva, A., Miletello, G., Curti, B., et al. (2019). Overall survival by clinical risk category for high dose interleukin-2 (HD IL-2) treated patients with metastatic renal cell cancer (mRCC): data from the PROCLAIM registry. J. Immunother. Cancer 7:84.
Fu, D., Liu, B., Zang, L., and Jiang, H. (2016). MiR-631/ZAP70: a novel axis in the migration and invasion of prostate cancer cells. J. Biochem. Biophys. Res. Commun. 469, 345–351. doi: 10.1016/j.bbrc.2015.11.093
Garcia, F., Petry, K., Muderspach, L., Gold, M., Braly, P., Crum, C., et al. (2004). ZYC101a for treatment of high-grade cervical intraepithelial neoplasia: a randomized controlled trial. J. Obstetr. Gynecol. 103, 317–326. doi: 10.1097/01.aog.0000110246.93627.17
Gorter, A., Prins, F., van Diepen, M., Punt, S., and van der Burg, S. (2015). The tumor area occupied by Tbet+ cells in deeply invading cervical cancer predicts clinical outcome. J. Transl. Med. 13:295.
Han, H., Jae-Won, C., Lee, S., Yun, A., Kim, H., Bae, D., et al. (2017). TRRUST v2: an expanded reference database of human and mouse transcriptional regulatory interactions. J. Nucleic Acids Res. 46(D1), D380–D386. doi: 10.1093/nar/gkx1013
Imming, P., Sinning, C., and Meyer, A. (2006). Drugs, their targets and the nature and number of drug targets. Nat. Rev. Drug Discov. 5, 821–834. doi: 10.1038/nrd2132
Jia, D., Li, S., Li, D., Xue, H., Yang, D., and Liu, Y. (2018). Mining TCGA database for genes of prognostic value in glioblastoma microenvironment. J. Aging 10, 592–605. doi: 10.18632/aging.101415
Jochems, C., and Schlom, J. (2011). Tumor-infiltrating immune cells and prognosis: the potential link between conventional cancer therapy and immunity. J. Exp. Biol. 236, 567–579. doi: 10.1258/ebm.2011.011007
Joyce, J., and Fearon, D. (2015). T cell exclusion, immune privilege, and the tumor microenvironment. J. Sci. 348, 74–80. doi: 10.1126/science.aaa6204
Kietpeerakool, C., Rattanakanokchai, S., Jampathong, N., Srisomboon, J., and Lumbiganon, P. (2019). Management of drainage for malignant ascites in gynaecological cancer. J. Cochrane Database Syst. Rev. 12:CD007794.
Kim, J., Nam, K., Ahn, S., Park, D., Kim, H., Kim, S., et al. (2016). Prognostic implications of immunosuppressive protein expression in tumors as well as immune cell infiltration within the tumor microenvironment in gastric cancer. J. Gastr. Cancer 19, 42–52. doi: 10.1007/s10120-014-0440-5
Kimura, Y., Fujii, M., Masuishi, T., Nishikawa, K., Kunisaki, C., Matsusaka, S., et al. (2018). Multicenter phase II study of trastuzumab plus S-1 alone in elderly patients with HER2-positive advanced gastric cancer (JACCRO GC-06). J. Gastr. Cancer 21, 421–427. doi: 10.1007/s10120-017-0766-x
Lansigan, F., Costa, C., Zaki, B., Yen, S., Winer, E., Ryan, H., et al. (2019). Multicenter, open-label, phase II study of bendamustine and rituximab followed by 90-Yttrium (Y) Ibritumomab tiuxetan for untreated follicular lymphoma (Fol-BRITe). J. Clin. Cancer Res. 25, 6073–6079. doi: 10.1158/1078-0432.ccr-18-3755
Laufer, J., Lyck, R., and Legler, D. (2018). ZAP70 expression enhances chemokine-driven chronic lymphocytic leukemia cell migration and arrest by valency regulation of integrins. FASEB J. 32, 4824–4835. doi: 10.1096/fj.201701452rr
Law, V., Knox, C., Djoumbou, Y., Jewison, T., Guo, A. C., Liu, Y., et al. (2014). DrugBank 4.0: shedding new light on drug metabolism. Nucleic Acids Res. 42, D1091–D1097.
Li, Q., Ma, L., Shen, S., Guo, Y., Cao, Q., Cai, X., et al. (2019). Intestinal dysbacteriosis-induced IL-25 promotes development of HCC via alternative activation of macrophages in tumor microenvironment. J. Exp. Clin. Cancer Res. 38:303.
Lin, Y., Liu, T., Cui, T., Wang, Z., Zhang, Y., Tan, P., et al. (2019). RNAInter in 2020: RNA interactome repository with increased coverage and annotation. J. Nucleic Acids Res. 48, D189–D197.
Looi, C., Chung, F., Leong, C., Wong, S., Rosli, R., and Mai, C. (2019). Therapeutic challenges and current immunomodulatory strategies in targeting the immunosuppressive pancreatic tumor microenvironment. J. Exp. Clin. Cancer Res. 38:162.
Maolake, A., Izumi, K., Natsagdorj, A., Iwamoto, H., Kadomoto, S., Makino, T., et al. (2018). Tumor necrosis factor-α induces prostate cancer cell migration in lymphatic metastasis through CCR7 upregulation. J. Cancer Sci. 109, 1524–1531. doi: 10.1111/cas.13586
McLachlan, J., Boussios, S., Okines, A., Glaessgen, D., Bodlar, S., Kalaitzaki, R., et al. (2017). The impact of systemic therapy beyond first-line treatment for advanced cervical cancer. J. Clin. Oncol. 29, 153–160. doi: 10.1016/j.clon.2016.10.002
Medina-Martinez, I., Barrón, V., Roman-Bassaure, E., Juárez-Torres, E., Guardado-Estrada, M., Espinosa, A., et al. (2014). Impact of gene dosage on gene expression, biological processes and survival in cervical cancer: a genome-wide follow-up study. PLoS One 9:e97842. doi: 10.1371/journal.pone.0097842
Meira, D., de Almeida, V., Mororó, J., Nóbrega, I., Bardella, L., Silva, R., et al. (2009). Combination of cetuximab with chemoradiation, trastuzumab or MAPK inhibitors: mechanisms of sensitisation of cervical cancer cells. Br. J. Cancer 101, 782–791. doi: 10.1038/sj.bjc.6605216
Moore, K., Sill, M., Miller, D., McCourt, C., De Geest, K., Rose, P., et al. (2012). A phase I trial of tailored radiation therapy with concomitant cetuximab and cisplatin in the treatment of patients with cervical cancer: a gynecologic oncology group study. J. Gynecol. Oncol. 127, 456–461. doi: 10.1016/j.ygyno.2012.08.030
Mootha, V., Lindgren, C., Eriksson, K., Subramanian, A., Sihag, S., Lehar, J., et al. (2003). PGC-1alpha-responsive genes involved in oxidative phosphorylation are coordinately downregulated in human diabetes. J. Nat. Genet. 34, 267–273. doi: 10.1038/ng1180
Ng, S., Wang, P., Lee, Y., Lee, C., Yang, S., Shen, H., et al. (2019). Impact of matrix metalloproteinase-11 gene polymorphisms on development and clinicopathologcial variables of uterine cervical cancer in Taiwanese women. Intern. J. Med. Sci. 16, 774–782. doi: 10.7150/ijms.33195
Overington, J. P., Al-Lazikani, B., and Hopkins, A. L. (2006). How many drug targets are there? Nat. Rev. Drug Discov. 5, 993–996. doi: 10.1038/nrd2199
Pasini, F., Zilberstein, B., Snitcovsky, I., Roela, R., Mangone, F., Ribeiro, U., et al. (2014). A gene expression profile related to immune dampening in the tumor microenvironment is associated with poor prognosis in gastric adenocarcinoma. J. Gastroenterol. 49, 1453–1466. doi: 10.1007/s00535-013-0904-0
Penson, R., Huang, H., Wenzel, L., Monk, B., Stockman, S., Long, H., et al. (2015). Bevacizumab for advanced cervical cancer: patient-reported outcomes of a randomised, phase 3 trial (NRG oncology-gynecologic oncology group protocol 240). J. Lancet Oncol. 16, 301–311. doi: 10.1016/s1470-2045(15)70004-5
Petitprez, F., Vano, Y., Becht, E., Giraldo, N., de Reyniès, A., Sautès-Fridman, C., et al. (2018). Transcriptomic analysis of the tumor microenvironment to guide prognosis and immunotherapies. J. Cancer Immunol. Immunother. 67, 981–988. doi: 10.1007/s00262-017-2058-z
Piersma, S. (2011). Immunosuppressive tumor microenvironment in cervical cancer patients. J. Cancer Microenviron. 4, 361–375. doi: 10.1007/s12307-011-0066-7
Ritchie, M. E., Phipson, B., Wu, D., Hu, Y., Law, C. W., Shi, W., et al. (2015). limma powers differential expression analyses for RNA-sequencing and microarray studies. Nucleic Acids Res. 43:e47. doi: 10.1093/nar/gkv007
Shannon, P., Markiel, A., Ozier, O., Baliga, N., Wang, J., Ramage, D., et al. (2003). Cytoscape: a software environment for integrated models of biomolecular interaction networks. J. Genome Res. 13, 2498–2504. doi: 10.1101/gr.1239303
Spill, F., Reynolds, D., Kamm, R., and Zaman, M. (2016). Impact of the physical microenvironment on tumor progression and metastasis. J. Curr. Opin. Biotechnol. 40, 41–48. doi: 10.1016/j.copbio.2016.02.007
Subramanian, A., Tamayo, P., Mootha, V., Mukherjee, S., Ebert, B., Gillette, M., et al. (2005). Gene set enrichment analysis: a knowledge-based approach for interpreting genome-wide expression profiles. J. Proc. Natl. Acad. Sci. U.S.A. 102, 15545–15550. doi: 10.1073/pnas.0506580102
Szklarczyk, D., Gable, A., Lyon, D., Junge, A., Wyder, S., Huerta-Cepas, J., et al. (2019). STRING v11: protein-protein association networks with increased coverage, supporting functional discovery in genome-wide experimental datasets. J. Nucleic Acids Res. 47, D607–D613.
Teng, M. W., Galon, J., Fridman, W.-H., and Smyth, M. J. (2015). From mice to humans: developments in cancer immunoediting. J. Clin. Invest. 125, 3338–3346. doi: 10.1172/jci80004
Tewari, K., Sill, M., Long, H., Penson, R., Huang, H., Ramondetta, L., et al. (2014). Improved survival with bevacizumab in advanced cervical cancer. N. Engl. J. Med. 370, 734–743.
Tewari, K., Sill, M., Penson, R., Huang, H., Ramondetta, L., Landrum, L., et al. (2017). Bevacizumab for advanced cervical cancer: final overall survival and adverse event analysis of a randomised, controlled, open-label, phase 3 trial (Gynecologic Oncology Group 240). J. Lancet 390, 1654–1663. doi: 10.1016/s0140-6736(17)31607-0
Tomczak, K., Czerwinska, P., and Wiznerowicz, M. (2015). The cancer genome atlas (TCGA): an immeasurable source of knowledge. Contemp. Oncol. 19, A68–A77.
Valle-Mendiola, A., Gutiérrez-Hoya, A., Lagunas-Cruz, M. C., Weiss-Steider, B., and Soto-Cruz, I. (2016). Pleiotropic effects of IL-2 on cancer: its role in cervical cancer. J. Med. Inflamm. 2016:2849523.
Wang, Q., Steger, A., Mahner, S., Jeschke, U., and Heidegger, H. (2019a). The formation and therapeutic update of tumor-associated macrophages in cervical cancer. Intern. J. Mol. Sci. 20:3310. doi: 10.3390/ijms20133310
Wang, Q., Zhang, L., Yan, Z., Xie, L., An, Y., Li, H., et al. (2019b). OScc: an online survival analysis web server to evaluate the prognostic value of biomarkers in cervical cancer. J. Future Oncol. 15, 3693–3699. doi: 10.2217/fon-2019-0412
Wu, D., Huang, Y., Kang, J., Li, K., Bi, X., Zhang, T., et al. (2015). ncRDeathDB: a comprehensive bioinformatics resource for deciphering network organization of the ncRNA-mediated cell death system. J. Autophagy 11, 1917–1926. doi: 10.1080/15548627.2015.1089375
Wui-Jin, K., Nadeem, R. A.-R., Sarah, B., Kristin, B., Susana, M. C., Kathleen, R. C., et al. (2019). Cervical cancer, version 3.2019, NCCN clinical practice guidelines in oncology. J. Natl. Compr. Canc. Netw. 17:64. doi: 10.1097/01.cot.0000365310.49708.ac
Xu, W., Xu, Y., Wang, J., Wan, F., Wang, H., Cao, D., et al. (2019). Prognostic value and immune infiltration of novel signatures in clear cell renal cell carcinoma microenvironment. J. Aging 11, 6999–7020.
Yang, L., Wang, B., Chen, L., Luo, H., and Wu, J. (2012). CXCL10 enhances radiotherapy effects in HeLa cells through cell cycle redistribution. J. Oncol. Lett. 3, 383–386. doi: 10.3892/ol.2011.472
Yao, H., Wang, H., Li, C., Fang, J.-Y., and Xu, J. (2018). Cancer cell-intrinsic PD-1 and implications in combinatorial immunotherapy. Front. Immunol. 9:1774. doi: 10.3389/fimmu.2018.01774
Yoshihara, K., Shahmoradgoli, M., Martínez, E., Vegesna, R., Kim, H., Torres-Garcia, W., et al. (2013). Inferring tumour purity and stromal and immune cell admixture from expression data. J. Nat. Commun. 4:2612.
Zhang, W., Chen, M., Cheng, H., Shen, Q., Wang, Y., and Zhu, X. (2018). The role of gene on the biological behavior of squamous cervical cancer in vitro and in vivo. J. Cancer Manag. Res. 10, 323–338. doi: 10.2147/cmar.s153036
Zhang, X., Lv, Z., Yu, H., Wang, F., and Zhu, J. (2015). The HLA-DQB1 gene polymorphisms associated with cervical cancer risk: a meta-analysis. J. Biomed. Pharmacother. 73, 58–64. doi: 10.1016/j.biopha.2015.06.002
Zhao, M., Ma, Q., Xu, J., Fu, S., Chen, L., Wang, B., et al. (2015). Combining CXCL10 gene therapy and radiotherapy improved therapeutic efficacy in cervical cancer HeLa cell xenograft tumor models. J. Oncol. Lett. 10, 768–772. doi: 10.3892/ol.2015.3281
Zhou, C., Tuong, Z. K., and Frazer, I. H. (2019). Papillomavirus immune evasion strategies target the infected cell and the local immune system. Front. Oncol. 9:682. doi: 10.3389/fimmu.2018.0682
Zhu, H., Luo, H., Shen, Z., Hu, X., Sun, L., and Zhu, X. (2016). Transforming growth factor-β1 in carcinogenesis, progression, and therapy in cervical cancer. J. Tumour Biol. 37, 7075–7083. doi: 10.1007/s13277-016-5028-8
Keywords: cervical cancer, tumor microenvironment, TCGA, GEO, multifactor, drug
Citation: Zhao Z, Li J, Li H, Yuan Wu N-Y, Ou-Yang P, Liu S, Cai J and Wang J (2020) Integrative Bioinformatics Approaches to Screen Potential Prognostic Immune-Related Genes and Drugs in the Cervical Cancer Microenvironment. Front. Genet. 11:727. doi: 10.3389/fgene.2020.00727
Received: 14 February 2020; Accepted: 15 June 2020;
Published: 07 July 2020.
Edited by:
Andrei Rodin, City of Hope National Medical Center, United StatesCopyright © 2020 Zhao, Li, Li, Yuan Wu, Ou-Yang, Liu, Cai and Wang. This is an open-access article distributed under the terms of the Creative Commons Attribution License (CC BY). The use, distribution or reproduction in other forums is permitted, provided the original author(s) and the copyright owner(s) are credited and that the original publication in this journal is cited, in accordance with accepted academic practice. No use, distribution or reproduction is permitted which does not comply with these terms.
*Correspondence: Jingting Cai, Y2FpamluZ3RpbmdAaG5jYS5vcmcuY24=; Jing Wang, d2FuZ2ppbmcwMDgxQGhuY2Eub3JnLmNu