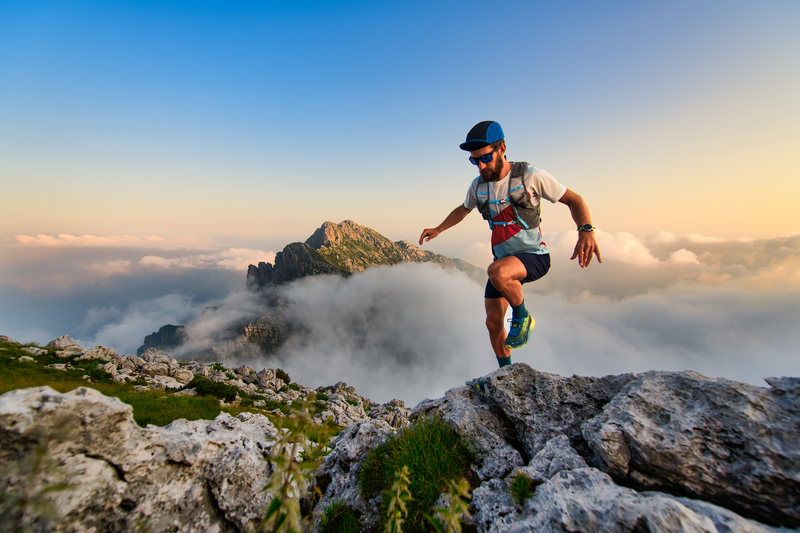
95% of researchers rate our articles as excellent or good
Learn more about the work of our research integrity team to safeguard the quality of each article we publish.
Find out more
ORIGINAL RESEARCH article
Front. Genet. , 24 July 2020
Sec. Computational Genomics
Volume 11 - 2020 | https://doi.org/10.3389/fgene.2020.00673
This article is part of the Research Topic Predicting High-Risk Individuals for Common Diseases Using Multi-Omics and Epidemiological Data View all 16 articles
Breast cancer (BRCA) is the most common cancer and a major cause of death in women. Long non-coding RNAs (lncRNAs) are emerging as key regulators and have been implicated in carcinogenesis and prognosis. In this study, we aimed to develop a lncRNA signature of BRCA patients to improve risk stratification. In the training cohort (GSE21653, n = 232), 17 lncRNAs were identified by univariate Cox proportional hazards regression, which were significantly associated with patients’ survival. The least absolute shrinkage and selection operator-penalized Cox proportional hazards regression analysis was used to identify a six-lncRNA signature. According to the median of the signature risk score, patients were divided into a high-risk group and a low-risk group with significant disease-free survival differences in the training cohort. A similar phenomenon was observed in validation cohorts (GSE42568, n = 101; GSE20711, n = 87). The six-lncRNA signature remained as independent prognostic factors after adjusting for clinical factors in these two cohorts. Furthermore, this signature significantly predicted the survival of grade III patients and estrogen receptor-positive patients. Furthermore, in another cohort (GSE19615, n = 115), the low-risk patients that were treated with tamoxifen therapy had longer disease-free survival than those who underwent no therapy. Overall, the six-lncRNA signature can be a potential prognostic tool used to predict disease-free survival of patients and to predict the benefits of tamoxifen treatment in BRCA, which will be helpful in guiding individualized treatments for BRCA patients.
Breast cancer (BRCA) is the second leading cause of cancer death among women. More than 268,000 new patients are diagnosed with BRCA each year and 41,760 patients will die from BRCA (DeSantis et al., 2019; Siegel et al., 2019). The current treatment for BRCA, which can improve survival of BRCA patients, includes mastectomy, hormone therapy (Early Breast Cancer Trialists Collaborative et al., 2011), surgery with adjuvant radiation therapy (Bradley and Mendenhall, 2018; Chargari et al., 2019), and chemotherapy (Oikonomou et al., 2019). Immunotherapy of BRCA patients is a recent emerging area of treatment (Greenlee et al., 2017; Jia et al., 2017; Adams et al., 2019). Although the TNM stage system is a valuable resource for the classification of BRCA patients, it does not predict the prognosis of patients. Therefore, the molecular markers need to be identified so that the survival of BRCA patients can be evaluated (Giuliano et al., 2017; Zhang et al., 2017).
Long non-coding RNAs (lncRNA, >200 nucleotides in length) are a class of non-coding RNAs transcribed from mammalian genomes (Yu et al., 2018). Some lncRNAs are found to be deregulated between cancer and normal tissues, such as BRCA (Liu et al., 2015), lung cancer (Jen et al., 2017), gastric cancer (Liu et al., 2017), and prostate cancer (Xu et al., 2018). Furthermore, lncRNAs have been confirmed to participate in diverse biological processes by acting as key regulators in cancers. Gupta et al. (2010) found that dysregulated HOTAIR increased cancer invasiveness and metastasis through dependence on PRC2, and lncRNA HOXD-AS1 regulated the Rho GTPase activating protein 11A (ARHGAP11A), which resulted in induced metastasis (Lu et al., 2017). In recent years, some lncRNAs have been found to be biomarkers of predicting BRCA patient outcomes, such as lncRNA BCYRN1 (Booy et al., 2017) and HOTAIR (Zhang et al., 2013a), which has attracted increasing attention.
In this study, we developed a six-lncRNA signature based on lncRNA expression, with the ability to predict disease-free survival of patients with BRCA, and we assessed its prognostic value in the training and validation cohorts. This signature had an independent prognostic value after adjusting for clinical factors. Furthermore, the lncRNA signature also significantly predicted survival of grade III and estrogen receptor (ER)-positive BRCA patients. Moreover, the signature predicted survival benefits of tamoxifen therapy in BRCA patients.
Breast cancer gene expression data generated by the Affymetrix HG-U133 Plus 2.0 microarray platform and corresponding clinical information were obtained from the publicly available GEO database1. To analyze the correlation of lncRNA expression with disease-free survival (DFS) for BRCA, we selected those data sets that included patients with survival status information. In total, 232 samples from GSE21653 (Sabatier et al., 2011a, b), 101 samples from GSE42568 (Clarke et al., 2013), and 87 samples from GSE20711 (Dedeurwaerder et al., 2011) were obtained. The GSE21653 data set was defined as the training cohort, and the GSE42568 and GSE20711 data sets were treated as the validation cohort. Another dataset, GSE19615 (n = 115) (Li et al., 2010), which contained 62 patients treated with tamoxifen, was obtained to validate the prognostic value of the signature for patients after hormone treatment. Detailed clinical information of patients with BRCA in this study is shown in Table 1.
All the raw microarray data (CEL files) of BRCA patients were downloaded from the GEO database and background adjusted and normalized using the Robust Multichip Average (RMA) algorithm (Irizarry et al., 2003a, b) and “Affy” package (Gautier et al., 2004). The probe sequences of Affymetrix HG-U133 Plus 2.0 array were downloaded from the Affymetrix website2 and uniquely mapped to the human genome (hg19). Specific probes of lncRNAs were obtained by matching the chromosomal position of probes to the chromosomal position of lncRNA genes based on the annotations from GENCODE (release 23) according to the previous studies (Du et al., 2013; Zhou et al., 2015). When multiple probes were mapped to the same lncRNA, expression values of these probes were integrated using the median value to represent the expression value of the single lncRNA. As a result, 2,673 lncRNAs were obtained for further analysis.
A univariate Cox proportional hazards regression analysis was carried out to evaluate the association between expression levels of lncRNAs and patients’ disease-free survival in the training cohort. Only those lncRNAs with a p-value of <0.01 were considered statistically significant. We then conducted the least absolute shrinkage and selection operator (LASSO) penalized Cox proportional hazards regression analysis to select the prognostic markers of the above lncRNAs (Tibshirani, 1997; Zhang et al., 2013b). We created a risk-score formula by a linear combination of the expressions of these six lncRNAs, weighted by their respective Cox regression coefficients as follows (Zhang et al., 2012, 2013c):
where N is the number of prognostic genes, Expi is the expression value of the i gene, and Coefi is the estimated regression coefficient of the i gene in the univariate Cox regression analysis. Using the median signature risk score in each cohort as the cutoff point, BRCA patients in every cohort were divided into low- and high-risk groups.
The association between the lncRNA gene expression and the patient’s survival was assessed by univariable Cox regression analysis. LASSO logistic regression analysis was used to identify the lncRNAs comprising the prognostic signature with non-zero coefficients in the training cohort using “glmnet” package (Friedman et al., 2010). Kaplan–Meier survival analysis and the log-rank test were used to compare the difference in disease-free survival between the high-risk group and low-risk group using the R package “survival.” Furthermore, we used Cox multivariate analysis to test whether the lncRNA signature was independent of patient age and histological grade. Hazard ratio (HR) and 95% confidence intervals (CI) were estimated by the Cox proportional hazards regression model. The time-dependent receiver operating characteristic (ROC) curves were used to compare the prognostic accuracy of the six-lncRNA signature for survival. Statistical significance was defined as two-tailed p-values being less than 0.05. All of the statistical analyses were performed using R program 3.5.23 and Bioconductor.
As summarized in the workflow (Figure 1), we first performed an univariable Cox proportional hazards regression analysis to assess the association between lncRNA expression and disease-free survival of patients with BRCA in the training cohort. A set of 17 lncRNAs that were significantly correlated with patients’ survival (p ≤ 0.01, Table 2) was identified. We found six lncRNAs (LINC00917, AL391840.1, TRIM52-AS1, AL355075.4, AC093802.2, and AC091544.4) to comprise a prognostic signature using a LASSO-penalized Cox proportional hazards regression analysis for the above 17 lncRNAs with optimal tuning parameters. All six lncRNAs have positive coefficients, which indicates that their high expressions are associated with shorter survival. Finally, we calculated the signature risk score based on a linear combination of the expression levels of six prognostic lncRNAs, weighted by the coefficients derived from the univariable Cox regression analysis as follows: Risk Score = (1.6348 × expression value of LINC00917) + (1.7487 × expression value of AL391840.1) + (0.6661 × expression value of TRIM52-AS1) + (0.9439 × expression value of AL355075.4) + (1.1742 × expression value of AC093802.2) + (0.4818 × expression value of AC091544.4).
Table 2. The 17 lncRNAs that are significantly associated with the disease-free survival in the training cohort (n = 232).
We calculated the six-lncRNA signature risk score for each patient in the training cohort (GSE21653, n = 232). The patients were divided into a high-risk group (n = 116) and a low-risk group (n = 116) using the median risk score as the cutoff. Compared with the low-risk patients, the high-risk patients had shorter disease-free survival (median survival 62.4 months vs greater than 200 months, HR = 1.67, 95% CI = 1.05–2.66, p = 0.028, Figure 2A). The prognostic value of the six-lncRNA signature was then evaluated in the validation cohort (GSE42568, n = 101). The signature classified patients into two groups, including a high-risk group (n = 50) and a low-risk group (n = 51), based on the median risk score. The disease-free survival of the high-risk group was significantly shorter than that of the low-risk group (median survival 69.7 months vs greater than 100 months, HR = 2, 95% CI = 1.09–3.66, p = 0.022, Figure 2B). Similarly, in another validated cohort (GSE20711, n = 87), the high-risk group still had a poorer prognosis than the low-risk group (median survival 77.8 months vs 122.5 months, HR = 1.54, 95% CI = 1.02–2.91, p = 0.040, Figure 2C).
Figure 2. Kaplan–Meier survival curves of disease-free survival between high-risk (red) and low-risk (blue) patients in the (A) training cohort (GSE21653, n = 232), and the (B,C) validation cohort (GSE42568, n = 101; GSE20711, n = 87). The differences between the two curves were determined by the two-sided log-rank test. The number of patients at risk is listed below the survival curves. HR, hazard ratio.
Next, we assessed whether the prognostic value of the six-lncRNA signature was independent of other clinical factors. We performed univariate and multivariate Cox proportional hazards regression analysis for factors, including age, ER status, histological grade, and the signature. In the training cohort, the high-risk six-lncRNA signature (HR = 1.789, 95% CI = 1.122–2.852, p = 0.015), grade III (HR = 3.174, 95% CI = 1.314–7.666, p = 0.010) and grade II (HR = 2.881, 95% CI = 1.181–7.028, p = 0.020) were significantly correlated with DFS of patients (Table 3). We found that the signature (HR = 2.327, 95% CI = 1.256–4.311, p = 0.007) and ER status (HR = 0.472, 95% CI = 0.234–0.877, p = 0.017) significantly independently predicted patients’ disease-free survival in the validation cohort GSE42568 (Table 3). Moreover, the six-lncRNA signature was also an independent prognostic factor associated with disease-free survival in the GSE20711 dataset (HR = 1.631, 95% CI = 1.037–3.105, p = 0.043). These results indicate that the six-lncRNA signature is an independent prognostic factor for BRCA patients’ disease-free survival.
We explored whether the six-lncRNA signature was effective for patients within different histological grades using a Kaplan–Meier survival analysis. For grade III patients, the signature significantly classified patients into two groups with distinctively different survival times (median survival 55.2 months vs greater than 150 months, HR = 2.39, 95% CI = 1.26–4.51, p = 0.0057, Figure 3A), including the high-risk group (n = 56) and the low-risk group (n = 61) in the training cohort. The signature showed a similar prognostic value for grade III patients in the validation cohort (median survival 25.2 months vs greater than 69.3 months, HR = 3.01 95% CI = 0.96–9.46, p = 0.048, Figure 3B). In grade I patients, there were no significant survival differences among the high-risk groups and the low-risk groups in two cohorts (Supplementary Figure S1A,B). A similar phenomenon was observed in grade II patients from the GSE21653 data set (Supplementary Figure S1C). However, in grade II patients from the GSE42568 data set, the high-risk and low-risk groups had significant survival differences (HR = 5.29, 95% CI = 1.17–23.9, p = 0.015, Supplementary Figure S1D).
Figure 3. Survival analysis of grade III patients based on the six-lncRNA signature. Kaplan–Meier survival curves for grade III patients in (A) GSE21653 (n = 117) and (B) GSE42568 (n = 51).
Furthermore, Kaplan–Meier survival analysis was performed after patient stratification according to ER status. The ER-positive patients were divided into high-risk and low-risk groups. The high-risk ER-positive patients had shorter disease-free survival than low-risk ER-positive patients in the training cohort (HR = 1.77, 95% CI = 0.93–3.38, p = 0.078, Figure 4A) and the validation cohort (HR = 3.32, 95% CI = 1.31–8.38, p = 0.0072, Figure 4B). There were no significant survival differences between the high-risk and low-risk ER-negative patients in these two cohorts when using the same risk formula (Supplementary Figure S2).
Figure 4. Survival analysis of ER-positive patients based on the six-lncRNA signature. Kaplan–Meier survival curves for ER-positive patients in (A) GSE21653 (n = 128) and (B) GSE42568 (n = 67).
We further tested whether the six-lncRNA was useful to guide therapy in an independent cohort (GSE19615). In this cohort, there were 62 patients who received tamoxifen therapy and 47 who did not. We classified each patient into high- and low-risk groups based on the lncRNA signature risk score. Among the 58 low-risk patients, tamoxifen treatment could prolong the disease-free survival of these patients (HR = 0.08, 95% CI = 0.01–0.62, p = 0.0018, Figure 5A), while there were no significant survival differences between patients with and without tamoxifen therapy in the high-risk group (Figure 5B). This result revealed that tamoxifen treatment was only beneficial for low-risk BRCA patients.
Figure 5. Kaplan–Meier curves of the disease-free survival according to low-risk or high-risk scores, stratified by tamoxifen therapy in an independent cohort (GSE19615, n = 115). (A) Patients with tamoxifen therapy (red) had significantly longer disease-free survival than patients without treatment (blue) in the low-risk group. (B) Patients who received tamoxifen therapy (red) and those who did not (blue) showed no survival differences in the high-risk group.
To compare the sensitivity and specificity in survival prediction between clinical factors (histological grade and ER status) and the six-lncRNA signature, we performed a time-dependent ROC analysis in the training cohort. We also constructed a prognostic model by combining our signature with histological grade or ER status. There were no significant differences between histological grade and the lncRNA signature (p = 0.171). A similar result was found between the signature and ER status (p = 0.997). Moreover, for the histological grade, we observed that the histologic grade combined with the six-lncRNA signature (AUC = 0.73) had a higher area under the ROC curve than the histological grade alone (AUC = 0.68, Figure 6A). The six-lncRNA signature could also improve the prognostic accuracy of the ER status (0.63 vs 0.59, Figure 6B). In addition, for further clinical utility, we constructed a full clinical prognostic model by combining all clinical factors including age, histological grade, and ER status. After adding the six-lncRNA signature into the clinical prognostic model, the prediction accuracy of the model was effectively improved (0.74 vs 0.69, Figure 6C). These results suggest that our six-lncRNA signature can add a complementary value to known clinical factors.
Figure 6. Comparison of sensitivity and specificity for survival prediction by the six-lncRNA signature, histological grade, and ER status. (A) The receiver operating characteristics (ROC) curves of the six-lncRNA signature, histological grade, and the combination of the two factors. (B) The ROC curves of the six-lncRNA signature, ER status, and the combination of the two factors. (C) The ROC curves of the clinical model and the clinical model combined with the six-lncRNA signature. AUC, the area under the curve.
In the current study, we developed and validated a prognostic six-lncRNA signature based on lncRNA expression, which stratified BRCA patients into two groups (high-risk group and low-risk group) with different disease-free survival. We demonstrated that this signature could predict the survival of grade III BRCA patients. The ER-positive patients who were classified as the low-risk group achieved better survival benefits. Furthermore, by using this signature, we can find a subgroup of patients who are likely to benefit from tamoxifen therapy. In sum, the six-lncRNA signature for BRCA patients may be a prognostic tool that is helpful in guiding individualized treatment of patients.
Histological classification of BRCA into grades I, II, and III, determines the treatment of BRCA patients (Cortes et al., 2012; Harris et al., 2016; Waks and Winer, 2019). The tumor cells of grade III cancer tend to grow more quickly and look different from normal breast cells (Wani et al., 2010). We observed that the six-lncRNA signature significantly predicted the survival of grade III BRCA patients. This finding suggests that this lncRNA signature predicted survival in patients with invasive cancer. In addition, we found that high-risk ER-positive BRCA patients had shorter disease-free survival than low-risk ER-negative patients. Some studies have confirmed that ER is an essential predictor for responding to therapy, such as tamoxifen therapy, in metastatic BRCA (Fisher et al., 1997).
Given the heterogeneity of cancer, reliable prognostic biomarkers are needed to identify patients who can benefit from therapy (Li et al., 2017; Zhang et al., 2018). There is growing research on several gene signatures to improve decision-making and individualization of BRCA therapy (Cronin et al., 2007; Cardoso et al., 2016; Yu et al., 2019). However, it is difficult to apply all of them for clinical management. Our prognostic signature could identify a group of patients at low risk, where the use of tamoxifen therapy led to significantly extended disease-free survival. This suggests that our signature may hold special clinical value by separating responders to tamoxifen treatment, from non-responders, independent of pathological stage. Such separation could spare non-responders from therapy that is not beneficial and could promote the exploration of more effective therapeutic regimens.
The six-lncRNA (LINC00917, AL391840.1, TRIM52-AS1, AL355075.4, AC093802.2, and AC091544.4) signature in BRCA suggests that lncRNAs can be used as prognostic factors for the survival of patients. To avoid the influence of protein-coding genes, we annotated these probes with protein-coding genes, and found that only one lncRNA overlapped with protein-coding gene RPPH1. This gene had no predictive performance for survival, whether by itself or in combination with other lncRNAs (p = 0.39 and 0.16 respectively, Supplementary Figure S3). In addition, among these lncRNAs, TRIM52-AS1 was dominantly up-regulated in triple-negative breast cancer (TNBC) tissues compared to non-TNBC tissues by a RT-PCR (Lv et al., 2016). Moreover, another study found that the overexpression of TRIM52-AS1 suppressed cell migration and proliferation and induced cell apoptosis in renal cell carcinoma (Liu et al., 2016). However, these six lncRNAs have not been studied in BRCA. Thus, this is a novel study on the association between lncRNA expression and the disease-free survival of patients with BRCA.
Although the signature demonstrated an accurate survival prediction, several limitations should be noted. Because the sample size of our study was limited, large-scale cohort studies should be performed to investigate the prognostic value of this six-lncRNA signature. In addition, we only used a bioinformatics method to predict the six-lncRNA signature in BRCA, thus, further in vitro or in vivo experiments need to be conducted. Third, we investigated the efficacy of tamoxifen therapy in a low-risk BRCA group, thus more examinations are required to evaluate its efficacy and safety.
In conclusion, the six-lncRNA signature that we identified predicted the disease-free survival of patients with BRCA. This signature also predicted the survival of grade III and ER-positive patients. Furthermore, our findings revealed that the six-lncRNA signature could predict the benefits to patients treated with tamoxifen therapy. Further validation studies are needed to test the prognostic power of this signature before it is used clinically.
All datasets used in this study were publicly available from the GEO database (https://www.ncbi.nlm.nih.gov/geo) at accession numbers GSE21653, GSE42568, GSE20711, and GSE19615.
JH conceived the idea and conceptualized the study. EZ, YL, FQ, and JX conducted the bioinformatics analysis and interpreted the results. EZ and JX collected and pre-processed data. EZ, YL, SA, and LW generated the figures and tables. EZ and YL wrote the manuscript. JH and JX supervised the whole study process and revised the manuscript. All authors have read and approved the final version of manuscript.
This work was supported in part by the National Natural Science Foundation of China (Grant No. 31900478), the Heilongjiang Postdoctoral Foundation (LBH-Z17157), and the Fundamental Research Funds for the Provincial Universities (Grant Nos. 2018-KYYWF-0454 and 2018-KYYWF-0455).
The authors declare that the research was conducted in the absence of any commercial or financial relationships that could be construed as a potential conflict of interest.
The Supplementary Material for this article can be found online at: https://www.frontiersin.org/articles/10.3389/fgene.2020.00673/full#supplementary-material
Adams, S., Gatti-Mays, M. E., Kalinsky, K., Korde, L. A., Sharon, E., Amiri-Kordestani, L., et al. (2019). Current landscape of immunotherapy in breast cancer: a review. JAMA Oncol. doi: 10.1001/jamaoncol.2018.7147 [Online ahead of print].
Booy, E. P., McRae, E. K., Koul, A., Lin, F., and McKenna, S. A. (2017). The long non-coding RNA BC200 (BCYRN1) is critical for cancer cell survival and proliferation. Mol. Cancer 16:109. doi: 10.1186/s12943-017-0679-7
Bradley, J. A., and Mendenhall, N. P. (2018). Novel radiotherapy techniques for breast cancer. Annu. Rev. Med. 69, 277–288. doi: 10.1146/annurev-med-042716-103422
Cardoso, F., van’t Veer, L. J., Bogaerts, J., Slaets, L., Viale, G., Delaloge, S., et al. (2016). 70-Gene signature as an aid to treatment decisions in early-stage breast cancer. N. Engl. J. Med. 375, 717–729. doi: 10.1056/NEJMoa1602253
Chargari, C., Deutsch, E., Blanchard, P., Gouy, S., Martelli, H., Guerin, F., et al. (2019). Brachytherapy: an overview for clinicians. CA Cancer J. Clin. 69, 386–401. doi: 10.3322/caac.21578
Clarke, C., Madden, S. F., Doolan, P., Aherne, S. T., Joyce, H., O’Driscoll, L., et al. (2013). Correlating transcriptional networks to breast cancer survival: a large-scale coexpression analysis. Carcinogenesis 34, 2300–2308. doi: 10.1093/carcin/bgt208
Cortes, J., Calvo, V., Ramirez-Merino, N., O’Shaughnessy, J., Brufsky, A., Robert, N., et al. (2012). Adverse events risk associated with bevacizumab addition to breast cancer chemotherapy: a meta-analysis. Ann. Oncol. 23, 1130–1137. doi: 10.1093/annonc/mdr432
Cronin, M., Sangli, C., Liu, M. L., Pho, M., Dutta, D., Nguyen, A., et al. (2007). Analytical validation of the Oncotype DX genomic diagnostic test for recurrence prognosis and therapeutic response prediction in node-negative, estrogen receptor-positive breast cancer. Clin. Chem. 53, 1084–1091. doi: 10.1373/clinchem.2006.076497
Dedeurwaerder, S., Desmedt, C., Calonne, E., Singhal, S. K., Haibe-Kains, B., Defrance, M., et al. (2011). DNA methylation profiling reveals a predominant immune component in breast cancers. EMBO Mol. Med. 3, 726–741. doi: 10.1002/emmm.201100801
DeSantis, C. E., Ma, J., Gaudet, M. M., Newman, L. A., Miller, K. D., Goding Sauer, A., et al. (2019). Breast cancer statistics, 2019. CA Cancer J. Clin. 69, 438–451. doi: 10.3322/caac.21583
Du, Z., Fei, T., Verhaak, R. G., Su, Z., Zhang, Y., Brown, M., et al. (2013). Integrative genomic analyses reveal clinically relevant long noncoding RNAs in human cancer. Nat. Struct. Mol. Biol. 20, 908–913. doi: 10.1038/nsmb.2591
Early Breast, Cancer Trialists, Collaborative, G., Davies, C., Godwin, J., Gray, R., et al. (2011). Relevance of breast cancer hormone receptors and other factors to the efficacy of adjuvant tamoxifen: patient-level meta-analysis of randomised trials. Lancet 378, 771–784. doi: 10.1016/S0140-6736(11)60993-8
Fisher, B., Dignam, J., Wolmark, N., DeCillis, A., Emir, B., Wickerham, D. L., et al. (1997). Tamoxifen and chemotherapy for lymph node-negative, estrogen receptor-positive breast cancer. J. Natl. Cancer Inst. 89, 1673–1682. doi: 10.1093/jnci/89.22.1673
Friedman, J., Hastie, T., and Tibshirani, R. (2010). Regularization paths for generalized linear models via coordinate descent. J. Stat. Softw. 33, 1–22.
Gautier, L., Cope, L., Bolstad, B. M., and Irizarry, R. A. (2004). affy–analysis of Affymetrix GeneChip data at the probe level. Bioinformatics 20, 307–315. doi: 10.1093/bioinformatics/btg405
Giuliano, A. E., Connolly, J. L., Edge, S. B., Mittendorf, E. A., Rugo, H. S., Solin, L. J., et al. (2017). Breast Cancer-Major changes in the American Joint Committee on Cancer eighth edition cancer staging manual. CA Cancer J. Clin. 67, 290–303. doi: 10.3322/caac.21393
Greenlee, H., DuPont-Reyes, M. J., Balneaves, L. G., Carlson, L. E., Cohen, M. R., Deng, G., et al. (2017). Clinical practice guidelines on the evidence-based use of integrative therapies during and after breast cancer treatment. CA Cancer J. Clin. 67, 194–232. doi: 10.3322/caac.21397
Gupta, R. A., Shah, N., Wang, K. C., Kim, J., Horlings, H. M., Wong, D. J., et al. (2010). Long non-coding RNA HOTAIR reprograms chromatin state to promote cancer metastasis. Nature 464, 1071–1076. doi: 10.1038/nature08975
Harris, L. N., Ismaila, N., McShane, L. M., Andre, F., Collyar, D. E., Gonzalez-Angulo, A. M., et al. (2016). Use of biomarkers to guide decisions on adjuvant systemic therapy for women with early-stage invasive breast cancer: American Society of clinical oncology clinical practice guideline. J. Clin. Oncol. 34, 1134–1150. doi: 10.1200/JCO.2015.65.2289
Irizarry, R. A., Bolstad, B. M., Collin, F., Cope, L. M., Hobbs, B., and Speed, T. P. (2003a). Summaries of Affymetrix GeneChip probe level data. Nucleic Acids Res. 31, e15. doi: 10.1093/nar/gng015
Irizarry, R. A., Hobbs, B., Collin, F., Beazer-Barclay, Y. D., Antonellis, K. J., Scherf, U., et al. (2003b). Exploration, normalization, and summaries of high density oligonucleotide array probe level data. Biostatistics 4, 249–264. doi: 10.1093/biostatistics/4.2.249
Jen, J., Tang, Y. A., Lu, Y. H., Lin, C. C., Lai, W. W., and Wang, Y. C. (2017). Oct4 transcriptionally regulates the expression of long non-coding RNAs NEAT1 and MALAT1 to promote lung cancer progression. Mol. Cancer 16:104. doi: 10.1186/s12943-017-0674-z
Jia, H., Truica, C. I., Wang, B., Wang, Y., Ren, X., Harvey, H. A., et al. (2017). Immunotherapy for triple-negative breast cancer: existing challenges and exciting prospects. Drug Resist. Updat. 32, 1–15. doi: 10.1016/j.drup.2017.07.002
Li, B., Cui, Y., Diehn, M., and Li, R. (2017). Development and validation of an individualized immune prognostic signature in early-stage nonsquamous non-small cell lung cancer. JAMA Oncol. 3, 1529–1537. doi: 10.1001/jamaoncol.2017.1609
Li, Y., Zou, L., Li, Q., Haibe-Kains, B., Tian, R., Li, Y., et al. (2010). Amplification of LAPTM4B and YWHAZ contributes to chemotherapy resistance and recurrence of breast cancer. Nat. Med. 16, 214–218. doi: 10.1038/nm.2090
Liu, B., Sun, L., Liu, Q., Gong, C., Yao, Y., Lv, X., et al. (2015). A cytoplasmic NF-kappaB interacting long noncoding RNA blocks IkappaB phosphorylation and suppresses breast cancer metastasis. Cancer Cell 27, 370–381. doi: 10.1016/j.ccell.2015.02.004
Liu, Z., Chen, Z., Fan, R., Jiang, B., Chen, X., Chen, Q., et al. (2017). Over-expressed long noncoding RNA HOXA11-AS promotes cell cycle progression and metastasis in gastric cancer. Mol. Cancer 16:82. doi: 10.1186/s12943-017-0651-6
Liu, Z., Yan, H. Y., Xia, S. Y., Zhang, C., and Xiu, Y. C. (2016). Downregulation of long non-coding RNA TRIM52-AS1 functions as a tumor suppressor in renal cell carcinoma. Mol. Med. Rep. 13, 3206–3212. doi: 10.3892/mmr.2016.4908
Lu, S., Zhou, J., Sun, Y., Li, N., Miao, M., Jiao, B., et al. (2017). The noncoding RNA HOXD-AS1 is a critical regulator of the metastasis and apoptosis phenotype in human hepatocellular carcinoma. Mol. Cancer 16:125. doi: 10.1186/s12943-017-0676-x
Lv, M., Xu, P., Wu, Y., Huang, L., Li, W., Lv, S., et al. (2016). LncRNAs as new biomarkers to differentiate triple negative breast cancer from non-triple negative breast cancer. Oncotarget 7, 13047–13059. doi: 10.18632/oncotarget.7509
Oikonomou, E. K., Kokkinidis, D. G., Kampaktsis, P. N., Amir, E. A., Marwick, T. H., Gupta, D., et al. (2019). Assessment of prognostic value of left ventricular global longitudinal strain for early prediction of chemotherapy-induced cardiotoxicity: a systematic review and meta-analysis. JAMA Cardiol. 4, 1007–1018. doi: 10.1001/jamacardio.2019.2952
Sabatier, R., Finetti, P., Adelaide, J., Guille, A., Borg, J. P., Chaffanet, M., et al. (2011a). Down-regulation of ECRG4, a candidate tumor suppressor gene, in human breast cancer. PLoS One 6:e27656. doi: 10.1371/journal.pone.0027656
Sabatier, R., Finetti, P., Cervera, N., Lambaudie, E., Esterni, B., Mamessier, E., et al. (2011b). A gene expression signature identifies two prognostic subgroups of basal breast cancer. Breast Cancer Res. Treat. 126, 407–420. doi: 10.1007/s10549-010-0897-9
Siegel, R. L., Miller, K. D., and Jemal, A. (2019). Cancer statistics, 2019. CA Cancer J. Clin. 69, 7–34. doi: 10.3322/caac.21551
Tibshirani, R. (1997). The lasso method for variable selection in the Cox model. Stat. Med. 16, 385–395. doi: 10.1002/(sici)1097-0258(19970228)16:4<385:aid-sim380<3.0.co;2-3
Waks, A. G., and Winer, E. P. (2019). Breast cancer treatment: a review. JAMA 321, 288–300. doi: 10.1001/jama.2018.19323
Wani, F. A., Bhardwaj, S., Kumar, D., and Katoch, P. (2010). Cytological grading of breast cancers and comparative evaluation of two grading systems. J. Cytol. 27, 55–58. doi: 10.4103/0970-9371.70738
Xu, J., Lan, Y., Yu, F., Zhu, S., Ran, J., Zhu, J., et al. (2018). Transcriptome analysis reveals a long non-coding RNA signature to improve biochemical recurrence prediction in prostate cancer. Oncotarget 9, 24936–24949. doi: 10.18632/oncotarget.25048
Yu, F., Quan, F., Xu, J., Zhang, Y., Xie, Y., Zhang, J., et al. (2019). Breast cancer prognosis signature: linking risk stratification to disease subtypes. Brief. Bioinform. 20, 2130–2140. doi: 10.1093/bib/bby073
Yu, F., Zhang, G., Shi, A., Hu, J., Li, F., Zhang, X., et al. (2018). LnChrom: a resource of experimentally validated lncRNA-chromatin interactions in human and mouse. Database 2018:bay039. doi: 10.1093/database/bay039
Zhang, H., Deng, Y., Zhang, Y., Ping, Y., Zhao, H., Pang, L., et al. (2017). Cooperative genomic alteration network reveals molecular classification across 12 major cancer types. Nucleic Acids Res. 45, 567–582. doi: 10.1093/nar/gkw1087
Zhang, J. X., Han, L., Bao, Z. S., Wang, Y. Y., Chen, L. Y., Yan, W., et al. (2013a). HOTAIR, a cell cycle-associated long noncoding RNA and a strong predictor of survival, is preferentially expressed in classical and mesenchymal glioma. Neuro Oncol. 15, 1595–1603. doi: 10.1093/neuonc/not131
Zhang, J. X., Song, W., Chen, Z. H., Wei, J. H., Liao, Y. J., Lei, J., et al. (2013b). Prognostic and predictive value of a microRNA signature in stage II colon cancer: a microRNA expression analysis. Lancet Oncol. 14, 1295–1306. doi: 10.1016/S1470-2045(13)70491-1
Zhang, X. Q., Sun, S., Lam, K. F., Kiang, K. M., Pu, J. K., Ho, A. S., et al. (2013c). A long non-coding RNA signature in glioblastoma multiforme predicts survival. Neurobiol. Dis. 58, 123–131. doi: 10.1016/j.nbd.2013.05.011
Zhang, X., Sun, S., Pu, J. K., Tsang, A. C., Lee, D., Man, V. O., et al. (2012). Long non-coding RNA expression profiles predict clinical phenotypes in glioma. Neurobiol. Dis. 48, 1–8. doi: 10.1016/j.nbd.2012.06.004
Zhang, Y., Li, X., Zhou, D., Zhi, H., Wang, P., Gao, Y., et al. (2018). Inferences of individual drug responses across diverse cancer types using a novel competing endogenous RNA network. Mol. Oncol. 12, 1429–1446. doi: 10.1002/1878-0261.12181
Keywords: long non-coding RNA, signature, prognosis, disease-free survival, breast cancer
Citation: Zhao E, Lan Y, Quan F, Zhu X, A S, Wan L, Xu J and Hu J (2020) Identification of a Six-lncRNA Signature With Prognostic Value for Breast Cancer Patients. Front. Genet. 11:673. doi: 10.3389/fgene.2020.00673
Received: 04 March 2020; Accepted: 02 June 2020;
Published: 24 July 2020.
Edited by:
Bailiang Li, Stanford University, United StatesReviewed by:
Hongyi Zhang, University of Texas Southwestern Medical Center, United StatesCopyright © 2020 Zhao, Lan, Quan, Zhu, A, Wan, Xu and Hu. This is an open-access article distributed under the terms of the Creative Commons Attribution License (CC BY). The use, distribution or reproduction in other forums is permitted, provided the original author(s) and the copyright owner(s) are credited and that the original publication in this journal is cited, in accordance with accepted academic practice. No use, distribution or reproduction is permitted which does not comply with these terms.
*Correspondence: Jing Hu, aGppc3RiQGdtYWlsLmNvbQ==; Jinyuan Xu, eHVqaW55dWFuQGhyYm11LmVkdS5jbg==
†These authors have contributed equally to this work
Disclaimer: All claims expressed in this article are solely those of the authors and do not necessarily represent those of their affiliated organizations, or those of the publisher, the editors and the reviewers. Any product that may be evaluated in this article or claim that may be made by its manufacturer is not guaranteed or endorsed by the publisher.
Research integrity at Frontiers
Learn more about the work of our research integrity team to safeguard the quality of each article we publish.