- 1Division of Digital Health Sciences, Department of Health Sciences Research, Mayo Clinic, Rochester, MN, United States
- 2Division of Epidemiology, Department of Health Sciences Research, Mayo Clinic, Rochester, MN, United States
- 3Center for Individualized Medicine, Mayo Clinic, Rochester, MN, United States
- 4Division of Experimental Pathology, Department of Laboratory Medicine and Pathology, Mayo Clinic, Rochester, MN, United States
- 5Division of Biomedical Statistics and Informatics, Department of Health Sciences Research, Mayo Clinic, Rochester, MN, United States
Electronic health records (EHRs) are widely adopted with a great potential to serve as a rich, integrated source of phenotype information. Computational phenotyping, which extracts phenotypes from EHR data automatically, can accelerate the adoption and utilization of phenotype-driven efforts to advance scientific discovery and improve healthcare delivery. A list of computational phenotyping algorithms has been published but data fragmentation, i.e., incomplete data within one single data source, has been raised as an inherent limitation of computational phenotyping. In this study, we investigated the impact of diverse data sources on two published computational phenotyping algorithms, rheumatoid arthritis (RA) and type 2 diabetes mellitus (T2DM), using Mayo EHRs and Rochester Epidemiology Project (REP) which links medical records from multiple health care systems. Results showed that both RA (less prevalent) and T2DM (more prevalent) case selections were markedly impacted by data fragmentation, with positive predictive value (PPV) of 91.4 and 92.4%, false-negative rate (FNR) of 26.6 and 14% in Mayo data, respectively, PPV of 97.2 and 98.3%, FNR of 5.2 and 3.3% in REP. T2DM controls also contain biases, with PPV of 91.2% and FNR of 1.2% for Mayo. We further elaborated underlying reasons impacting the performance.
Introduction
The increased availability of EHRs fostered by the HITECH Act has a great potential to serve as a rich, integrated source of phenotype information (Denny et al., 2011; Crawford et al., 2014). Critical to this effort is computational phenotyping, which identifies patients with certain conditions of interest from EHR data (Gunasekar et al., 2016). A list of computational phenotyping algorithms covering over fifty diseases (PheKB, 2019) including RA (Liao et al., 2010; Partners Phenotyping Group, 2016) and T2DM are available at the PheKB, primarily developed through the eMERGE Network. The majority of the eMERGE phenotyping algorithms were developed to identify cases and controls of specific medical conditions for use in genome and phenome-wide association studies (Ritchie et al., 2010). However, developing research quality EHR-based computational phenotyping algorithms that categorize disease or traits in complete populations is not an easy task, as the primary purpose of EHR data is for healthcare delivery and reimbursement practices (Wei and Denny, 2015). Case/control EHR algorithms are powerful tools in research, however, the ability to characterize real-world clinical patient populations that are comprised of a mix of primary care patients (i.e., medical home), transient patients, and referral patients resulting in varying patterns of depth and detail in EMR data is more challenging. Thus, data fragmentation, or incomplete data due to patient movement across healthcare institutions, is an inherent limitation of using EHR data for research (Robinson et al., 2018), and creates challenges in validating EHR-based phenotypes in populations (Newton et al., 2013). While extensive investigations on computational phenotyping have been performed to improve algorithm performance and portability (Kullo et al., 2010; Kurreeman et al., 2011; Newton et al., 2013; Wei and Denny, 2015; Teixeira et al., 2016), the impact of data fragmentation on computational phenotyping is under investigated. We identified only one study which evaluated the impact of data fragmentation on algorithm performance. Using data from two healthcare institutions, the study demonstrated that running a T2DM phenotyping algorithm, developed by researchers from Northwestern University, on data from a single institution missed almost one third of the T2DM cases (Wei et al., 2012).
Using the Mayo Biobank cohort, we assessed the impact of data fragmentation on two popular eMERGE phenotyping algorithms, RA and T2DM, as these two diseases differ greatly in prevalence. Specifically, the overall prevalence of RA is estimated at 0.5%(Hunter et al., 2017) while T2DM is a common disease affecting approximately 8.6% adults in the United States (Bullard et al., 2018). Additionally, the RA algorithm is regression-based while the T2DM algorithm is rule-based.
Materials and Methods
Data Sources
In this study, we used the Mayo Clinic Biobank cohort (Olson et al., 2013) with the following self-reported data collected at the time of consent into the Biobank: general health, self and first-degree relative family disease history, and demographic characteristics. The clinical data for the cohort can be retrieved from two sources: Mayo Clinic EHRs and the REP (Rocca et al., 2018). The REP is a record linkage system which links and archives medical records from multiple healthcare providers in Minnesota and Wisconsin including Mayo Clinic since 2010 (Rocca et al., 2018). All analyses were based on a subset of the Biobank cohort consisting of 45,183 patients who have at least one diagnosis code in Mayo Clinic EHRs and at least one diagnosis code in the REP during 2010 and 2017. We used diabetes family history from the Biobank self-reported data to run the T2DM phenotyping algorithm. All patients in the chosen Biobank cohort consented to have their EHR data used for research. This study was approved by the Institutional Review Board of Mayo Clinic.
Phenotyping Algorithms
The eMERGE RA algorithm was created using a machine-learning penalized logistic regression model trained on a screen-positive data set with at least one RA diagnosis code (inclusion cohort) (Figure 1). Among the training set, the gold standard for model development was built up based on the 2010 American College of Rheumatology criteria for classification of RA (Aletaha et al., 2010). In the model, relative weights for features significantly associated with RA, including diagnosis of RA, SLE, PA, lab tests for rheumatoid factor, and total number of encounters (visits) per subject were assigned. Once the model was created, a threshold value based on a specificity of 97% was selected to identify cases. We used an updated version of the algorithm (the Harvard eMERGE RA Algorithm Document downloaded from https://phekb.org/phenotype/rheumatoid-arthritis-ra) which incorporates ICD 9 and 10 codes. Comparing to the previous version using only ICD 9 (Carroll et al., 2012), the updated version achieved sensitivity, PPV, and overall area under the curve of 87, 95, and 95%, respectively, compared to 65, 90, and 95% previously. Controls were selected from persons without any RA diagnosis codes and without any exclusion codes.
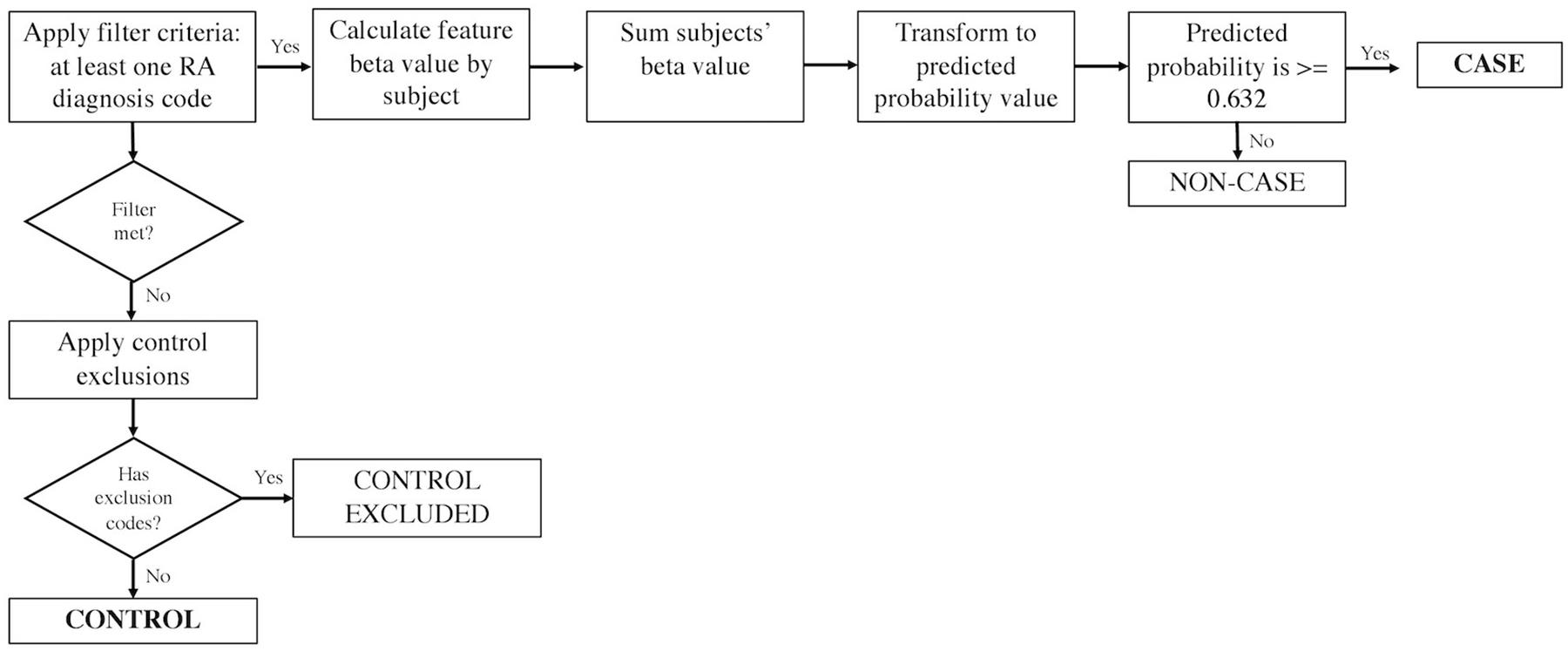
Figure 1. The eMERGE algorithm for identifying RA cases and controls. Adapted from Partners Phenotyping Group (2016).
As stated above, the eMERGE T2DM algorithm1 was developed by researchers from Northwestern University in 2012 (Kho et al., 2011). It is a rule-based algorithm based on diabetes related diagnosis, lab, and medication information, achieving 98 and 100% PPV for T2DM case and control identification, respectively. In addition to structured EHR data, in this study, we also extracted physician entered diagnoses from clinical notes of both Mayo and REP sources leveraging natural language processing (NLP) techniques. We updated the algorithm to incorporate ICD 10 codes which have been adopted in late 2015 in the United States (Hirsch et al., 2016). To collect more complete data, we also added medication extracted by NLP from clinical notes in both Mayo and REP sources. Figure 2 shows the flowchart of T2DM case phenotyping algorithm and Figure 3 shows the flowchart of T2DM control phenotyping algorithm.
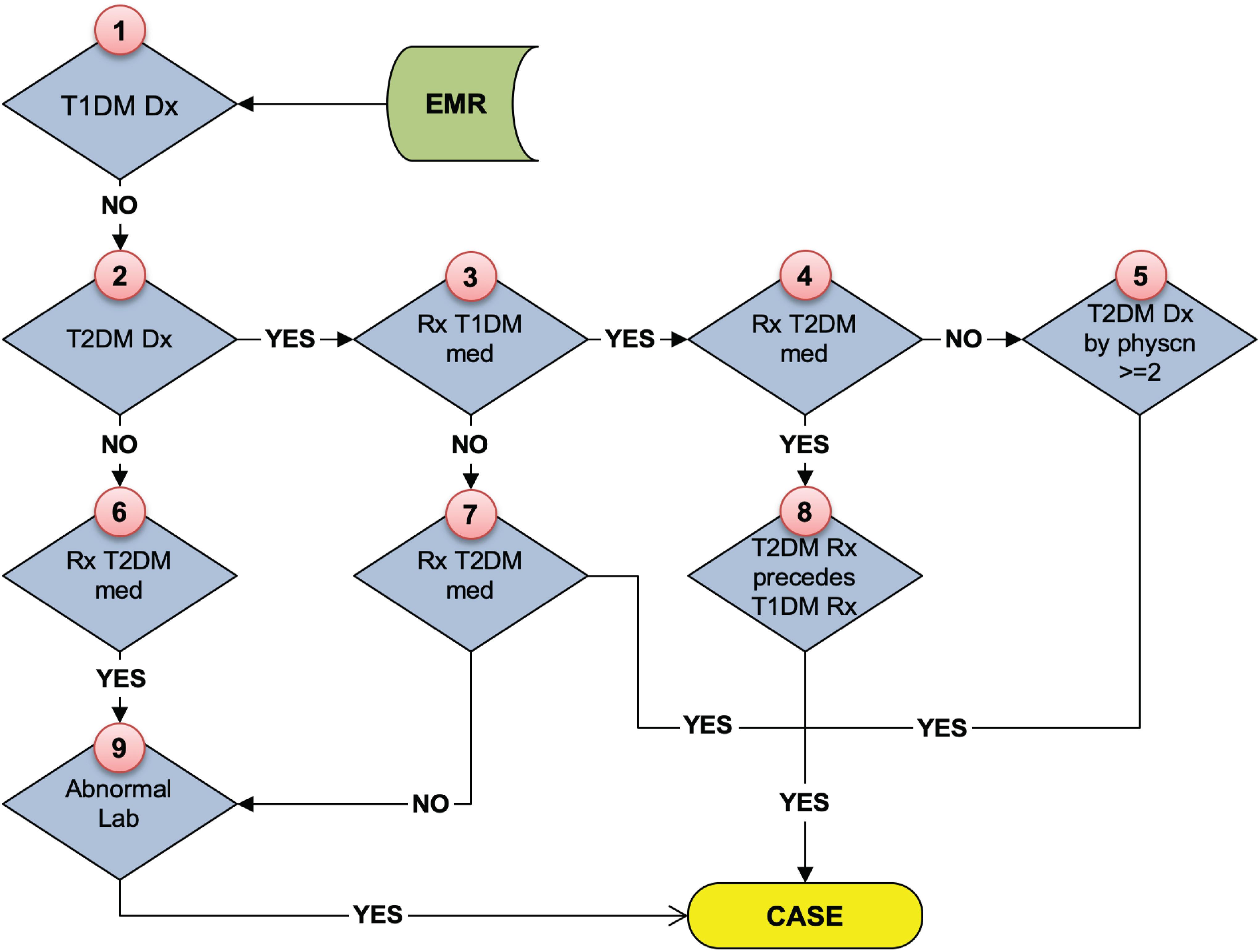
Figure 2. The eMERGE algorithm for identifying T2DM cases. Adapted from Pacheco and Thompson (2012).
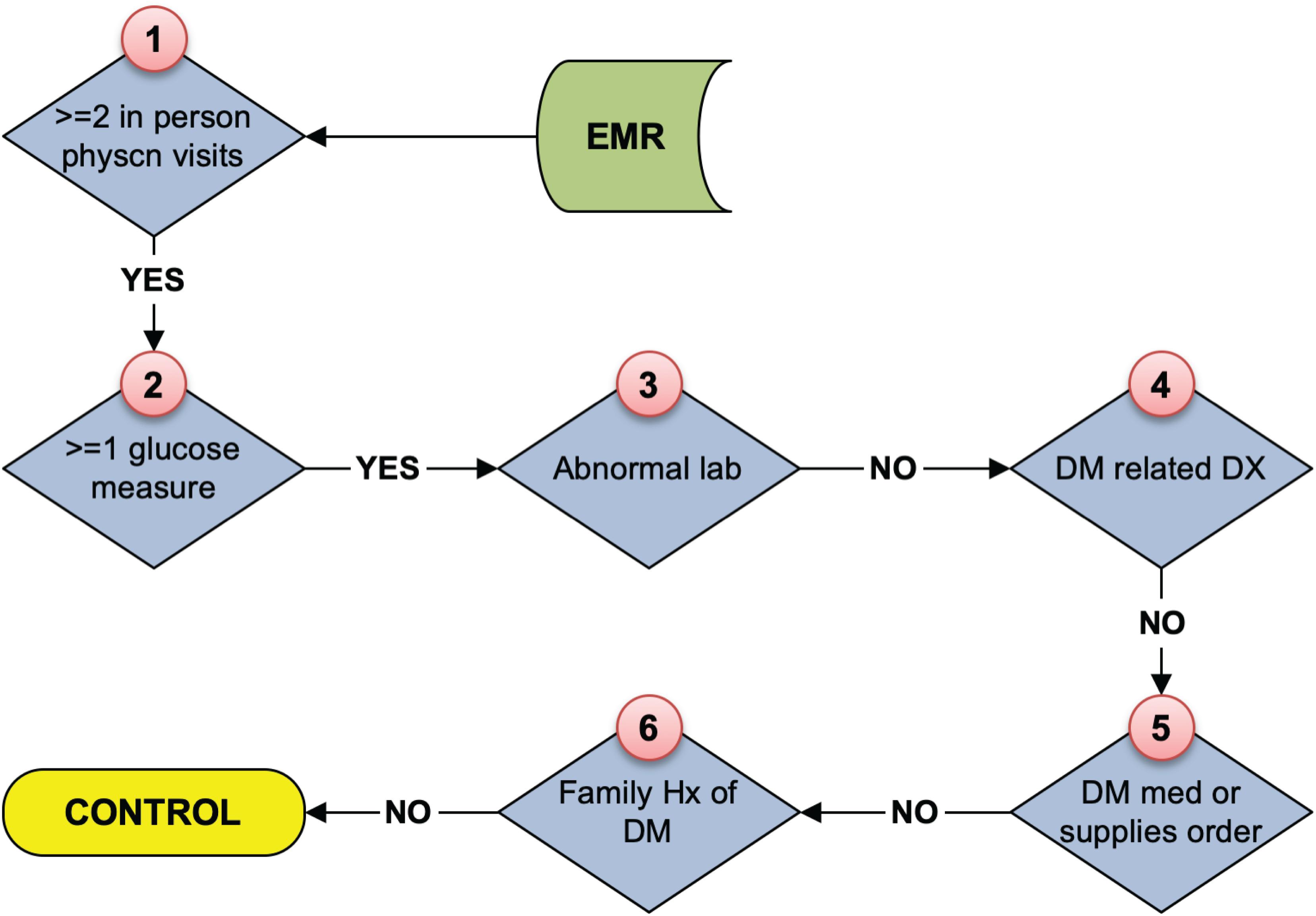
Figure 3. The eMERGE algorithm for identifying T2DM controls. Adapted from Pacheco and Thompson (2012).
Analysis
We assessed data fragmentation by evaluating the performance of the phenotyping algorithms in the cohort. To evaluate phenotyping errors caused by data fragmentation across health institutions, we used subjects identified from the combination of Mayo and REP data as the benchmark. To evaluate the performance of the phenotyping algorithms, we randomly selected 50 cases and 50 controls for each algorithm from the benchmark to perform manual review. We calculated sensitivity, specificity, PPV and FNR based on the number of TP, FP, TN, and FN against the benchmark (Wei et al., 2012). The impact of various data sources on quantitative change of features contributing to phenotyping was analyzed.
Results
Table 1 shows the number of cases and controls identified by each data source as well as the combination of sources. Using both Mayo Clinic EHRs and REP data, we identified 620 RA cases (42,319 controls) and 5,215 T2DM cases (6,293 controls) to serve as our benchmark for the analyses. Table 2 shows performance of RA and T2DM phenotyping algorithms in benchmark against chart reviewed gold standards. PPV of RA case was 90% compared to 95% in the updated version of the algorithm. PPVs of T2DM case and control were 82 and 100% compared to 98 and 100% in the publication (Kho et al., 2011).

Table 2. Performances of RA and T2DM phenotyping algorithms in benchmark (against chart reviewed gold standard).
Table 3 shows benchmark performance of RA and T2DM cases and controls using various data sources. Using Mayo only data, the RA phenotyping algorithm identified 43 FP RA case subjects, missed 165 FN RA case subjects (FNR, 26.6%), and identified 751 FP RA control subjects because of data fragmentation across healthcare institutions. The T2DM phenotyping algorithm identified 368 FP T2DM case subjects, missed 733 FN T2DM case subjects (FNR, 14%), identified 597 FP T2DM control subjects and missed 75 FN T2DM control subjects (FNR, 1.2%) because of data fragmentation across healthcare institutions. Fewer errors were resulted using REP only data, the RA phenotyping algorithm identified 17 FP RA case subjects, missed 32 FN RA case subjects (FNR, 5.2%), and identified 79 FP RA control subjects because of data fragmentation across healthcare institutions. The T2DM phenotyping algorithm identified 91 FP T2DM case subjects, missed 173 FN T2DM case subjects (FNR, 3.3%), identified 245 FP T2DM control subjects and missed 56 FN RA control subjects (FNR, 0.9%) because of data fragmentation across healthcare institutions.
Further analysis showed that the 150 out of 165 RA false negative cases obtained from the Mayo only data source were in the Mayo Clinic inclusion cohort (positive-screening set; Table 3). Among the 32 RA false negative cases in REP data source, all were in the REP inclusion cohort. Figure 4 presents the RA probabilities of all benchmark cases (620) derived from Mayo Clinic, REP, and the combination of Mayo and REP data. Probability estimated from Mayo and REP data show multiple regression lines, reflecting heterogenicity among healthcare providers. The red line intercepts the cutoff of 0.632; probabilities above the red line indicate RA cases. Figure 4 shows that probabilities of the FN in Mayo or REP sources improved after combining Mayo data with REP data.
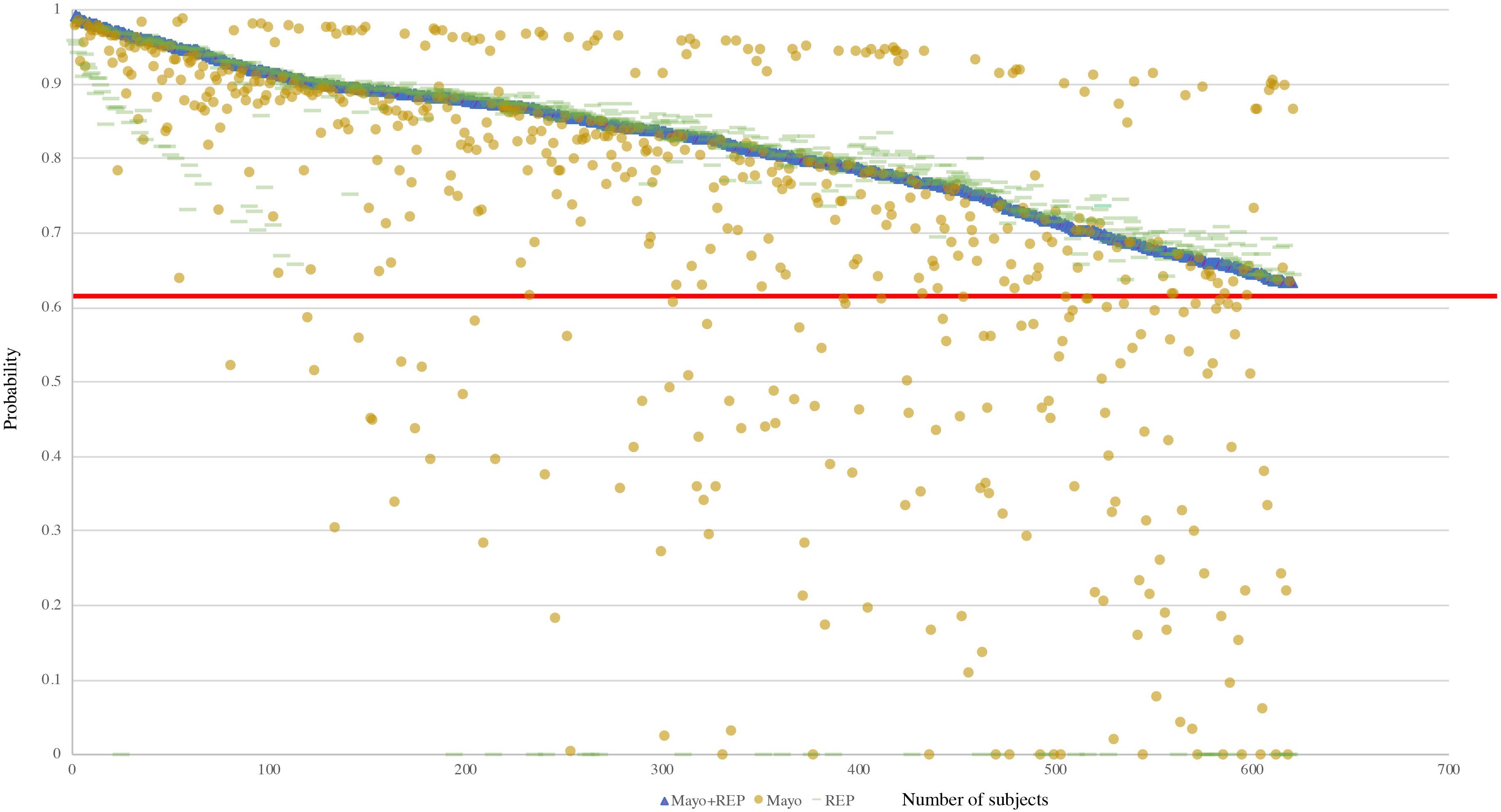
Figure 4. Probability of all benchmark cases based on various data sources. The red line intercepts the cutoff of 0.632, probabilities above the red line are classified as RA cases.
Table 4 shows statistics of features associated with RA phenotyping in various data sources between 2010 and 2017, specifically the number of patients with RA, PA, and SLE diagnosis codes, laboratory tests for rheumatoid factor as well as total number of encounters for the Mayo Biobank cohort. The inclusion cohort increased to 2,127 using both Mayo and REP data, with an increase of 524 compared to Mayo and 69 compared to REP. 15 of the 524 was identified as an RA case in the combined Mayo and REP data. Supplementary Figure S1 shows RA case probabilities of the false negative Mayo inclusion cohort (524 subjects) in the combination of Mayo and REP data, only several subjects had values close to the cutoff (0.632). Supplementary Figure S2 shows RA case probabilities of the false negative REP inclusion cohort (69 subjects) in the combination of Mayo and REP data, no subject had value above the cutoff.
The flowcharts (Figures 5, 6) show the comparison of each step of T2DM case and control phenotyping algorithms among various data sources, where the number denoting each step is from Figures 2, 3, respectively. The detailed statistics are provided in Supplementary Tables S1, S2. Using only Mayo data, 1 of the 733 FN T2DM cases have been falsely identified as a control, and 1 benchmark case was falsely identified as a control among the 597 FP T2DM controls. Table 5 shows factors that contribute to FP and FN RA case subjects. There are 751 FP controls from Mayo and 79 FP controls from REP due to incomplete diagnosis codes in each institution. Table 6 shows factors that contribute to FP and FN T2DM case subjects. Table 7 shows factors that contribute to FP and FN T2DM control subjects.
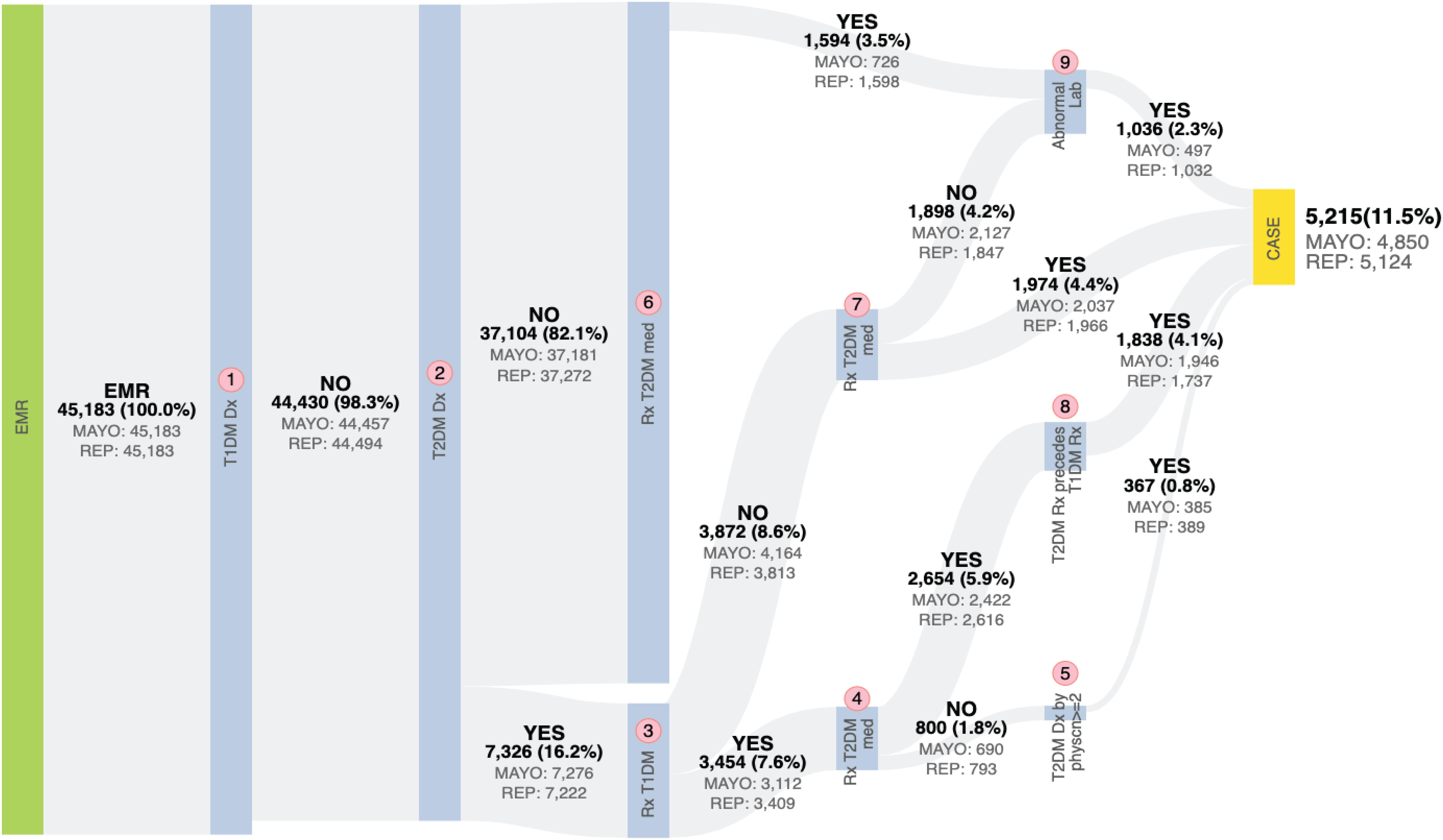
Figure 5. Quantitative comparison of each step in T2DM case phenotyping among various data sources. The number of each step corresponds to Figure 2, bold numbers are derived from the combination of Mayo of REP data.
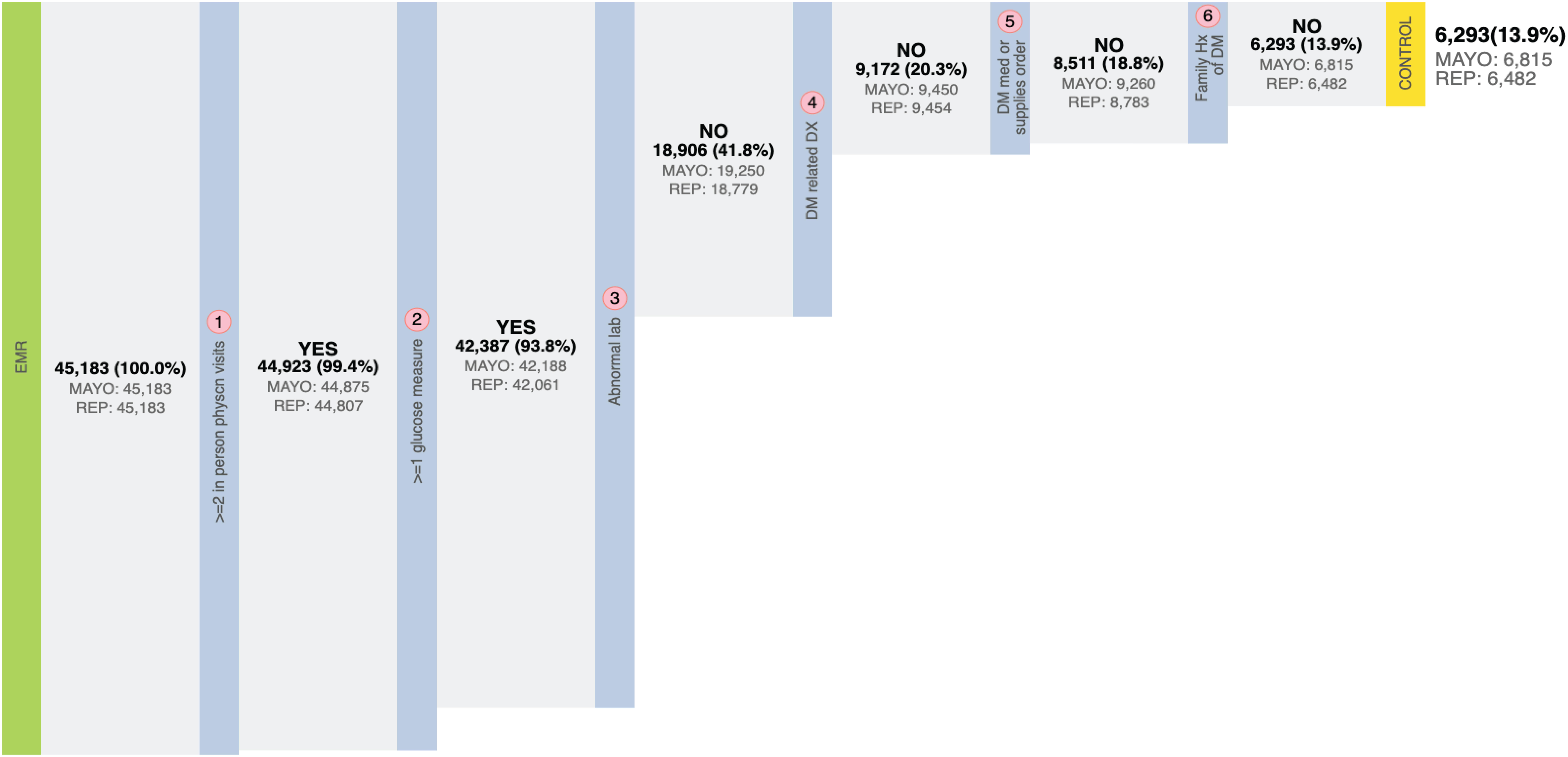
Figure 6. Quantitative comparison of each step in T2DM control phenotyping among various data sources. The number of each step corresponds to Figure 3, bold numbers are derived from the combination of Mayo of REP data.
Discussion
Electronic health records have been an asset for studies whose goal is to define cases and controls with high accuracy. However, leveraging EHRs for population studies is more challenging. Inherent data issues and bias (e.g., data fragmentation and referral bias) of EHRs may result in inaccurate and biased phenotyping results. RA and T2DM phenotyping algorithms to define cases and controls have been well studied by researchers with high performances within separate institutions (Carroll et al., 2012; Wei et al., 2012). These two diseases have also been fertile ground for secondary use of EHRs in combination with DNA samples (Wood et al., 2008; Kurreeman et al., 2011; Carroll et al., 2015). Therefore, it is worthwhile to investigate and evaluate the impact of data fragmentation on completeness and validity of algorithm results. To the best of our knowledge, this study is the first to evaluate the impact of data fragmentation on RA phenotyping algorithm. When assessing the impact of data fragmentation on T2DM, the FNR is 14% in our current study in comparison to 33% reported previously (Wei et al., 2012) as we leveraged NLP to extract more complete diagnosis and medication information from clinical notes. Our performance metrics of the benchmark against chart reviewed gold standard are slightly inferior to those metrics published previously, which is consistent with other studies, primarily due to the heterogenicity of healthcare systems impacting algorithm portability (Ostropolets et al., 2020).
As a regression-based algorithm, the sensitivity of the RA phenotyping depends on the comprehensiveness of the data, partially due to the complex relationships between autoimmune disorders. Systemic lupus erythematosus and PA both interfere with RA diagnosis. Total encounter numbers also exert adverse effects on RA case identification. Though RA diagnosis and positive RA lab results were assigned with relatively high positive weights, SLE, PA, and all encounter numbers were assigned with negative weights in the algorithm. In our study, 150 out of 165 FN cases in Mayo were already in the Mayo inclusion cohort and all FN cases in REP were in the REP inclusion cohort.
As a medical record-linkage system, REP captures more comprehensive diagnosis codes than Mayo EHRs resulting in fewer benchmark phenotyping errors. Meanwhile, not all information in Mayo EHRs is included in REP. For example, REP misses the diagnosis information coming from the Problem List in Mayo EHRs. Using Mayo only data, the RA phenotyping algorithm missed 165 FN RA case subjects (FNR = 26.6%) and the T2DM phenotyping algorithm missed 733 FN T2DM case subjects (FNR = 14%). All FN and FP cases, FN and FP controls defined using only Mayo data or only REP data were masked or falsely identified due to missing or insufficient information.
Although we investigated only two phenotyping algorithms in this study, the findings would also have implications for other research that relies on case and control identification using EMERGE algorithms. Considering the potential impact of data fragmentation on data quality for genomic research, clinical researchers should always keep this caveat in mind when employing a phenotyping algorithm for such studies. Ideally, adding data from a medical record linkage system or a comprehensive claims data source (such as the Centers for Medicare and Medicaid Services) can capture and reuse clinical information more efficiently. In addition, unstructured data extracted using NLP techniques could help to decrease data fragmentation because unstructured clinical notes in EHRs often record patients’ history of past diseases which may have been originally diagnosed and treated in other health institutions. Finally, besides the data fragmentation issue, temporal issues could also result in incomplete phenotyping results. Researchers have conducted a related study on T2DM (Wei et al., 2013), and we also investigated the dependence of the eMERGE RA algorithm on both RA and electronic health record (EHR) duration in another manuscript (Journal of the American Medical Informatics Association, in press).
The limitation of the study is that we didn’t have fully-annotated gold standards. Because of the high PPV seen in previous studies (Kho et al., 2011; Carroll et al., 2012), (95% for RA case, 98% and 100% for T2DM case and control), we set the benchmark for evaluation to be the phenotyping results based on the combination of data from both Mayo and REP, and only manually reviewed 50 cases and 50 controls for each algorithm to validate. In addition, the study would have been more generalizable if data from multiple sites had been independently collected.
Conclusion
In this study, we demonstrated that various data sources may result in different phenotyping results through two case studies. We also identified underlying reasons for variation in the algorithm performance. The completeness and validity of phenotypic and exposure information derived from EHRs are the foundations for precision medicine and patient care. As more genomic research makes use of EHR-derived phenotypes, it is important to understand that data fragmentation may significantly affect algorithm performance.
Data Availability Statement
The datasets for this article are not publicly available because: The Ethical approval is contingent on the data remaining private. Requests to access the datasets should be directed to HL, liu.hongfang@mayo.edu.
Ethics Statement
The studies involving human participants were reviewed and approved by the Institutional Review Board (IRB) of Mayo Clinic. The Ethics Committee waived the requirement of written informed consent for participation.
Author Contributions
All co-authors are justifiably credited with authorship, according to the authorship criteria. All authors read and approved the final manuscript. LW: design, development, data collection, analysis of data, interpretation of results, drafting and revision of the manuscript. MH, SF, and HH: data collection. JO, SB, JS, MC, and JC: critical revision of the manuscript. HL: conception, design, analysis of data, interpretation of results, critical revision of the manuscript.
Funding
This work was supported by the Mayo internal funding. This work was also supported by the National Center for Advancing Translational Sciences (U01TR02062) and National Library of Medicine of the National Institutes of Health under Award Number R01LM011829.
Conflict of Interest
The authors declare that the research was conducted in the absence of any commercial or financial relationships that could be construed as a potential conflict of interest.
Acknowledgments
We acknowledge the Mayo Clinic Center for Individualized Medicine. We acknowledge Donna M. Ihrke for manual chart review.
Supplementary Material
The Supplementary Material for this article can be found online at: https://www.frontiersin.org/articles/10.3389/fgene.2020.00556/full#supplementary-material
Abbreviations
EHRs, electronic health records; eMERGE, electronic medical records and genomics; FN, false negatives; FNR, false-negative rate; FP, false positives; HITECH, health information technology for economic and clinical health; PA, psoriatic arthritis; PheKB, phenotype knowledgebase; PPV, positive predictive value; RA, rheumatoid arthritis; REP, rochester epidemiology project; SLE, systemic lupus erythematosus; T2DM, type 2 diabetes mellitus; TN, true negatives; TP, true positives
Footnotes
References
Aletaha, D., Neogi, T., Silman, A. J., Funovits, J., Felson, D. T., Bingham, C. O., et al. (2010). 2010 Rheumatoid arthritis classification criteria: an American College of Rheumatology/European League Against Rheumatism collaborative initiative. Arthr. Rheum 62, 2569–2581. doi: 10.1002/art.27584
Bullard, K. M., Cowie, C. C., and Lessem, S. E. (2018). Prevalence of diagnosed diabetes in adults by diabetes type—United States, 2016. Morb. Mortal. Wkly Rep. 67:359. doi: 10.15585/mmwr.mm6712a2
Carroll, R. J., Eyler, A. E., and Denny, J. C. (2015). Intelligent use and clinical benefits of electronic health records in rheumatoid arthritis. Expert Rev. Clin. Immunol. 11, 329–337. doi: 10.1586/1744666X.2015.1009895
Carroll, R. J., Thompson, W. K., Eyler, A. E., Mandelin, A. M., Cai, T., Zink, R. M., et al. (2012). Portability of an algorithm to identify rheumatoid arthritis in electronic health records. J. Am. Med. Inform. Assoc. 19, e162–e169. doi: 10.1136/amiajnl-2011-000583
Crawford, D. C., Crosslin, D. R., Tromp, G., Kullo, I. J., Kuivaniemi, H., and Hayes, M. G. (2014). eMERGEing progress in genomics—the first seven years. Front. Genet. 5:184. doi: 10.3389/fgene.2014.00184
Denny, J. C., Crawford, D. C., Ritchie, M. D., Bielinski, S. J., Basford, M. A., Bradford, Y., et al. (2011). Variants near FOXE1 are associated with hypothyroidism and other thyroid conditions: using electronic medical records for genome-and phenome-wide studies. Am. J. Hum. Genet. 89, 529–542. doi: 10.1016/j.ajhg.2011.09.008
Gunasekar, S., Ho, J. C., Ghosh, J., Kreml, S., Kho, A. N., Denny, J. C., et al. (2016). Phenotyping using Structured Collective Matrix Factorization of Multi–source EHR Data. arXiv [preprint]. Available online at: https://arxiv.org/abs/1609.04466 (accessed January 20, 2020).
Hirsch, J., Nicola, G., McGinty, G., Liu, R. W., Barr, R. M., Chittle, M. D., et al. (2016). ICD-10: history and context. Am. J. Neuroradiol. 37, 596–599. doi: 10.3174/ajnr.A4696
Hunter, T. M., Boytsov, N. N., Zhang, X., Schroeder, K., Michaud, K., and Araujo, A. B. (2017). Prevalence of rheumatoid arthritis in the United States adult population in healthcare claims databases, 2004–2014. Rheumatol. Int. 37, 1551–1557. doi: 10.1007/s00296-017-3726-1
Kho, A. N., Hayes, M. G., Rasmussen-Torvik, L., Pacheco, J. A., Thompson, W. K., Armstrong, L. L., et al. (2011). Use of diverse electronic medical record systems to identify genetic risk for type 2 diabetes within a genome-wide association study. J. Am. Med. Inform. Assoc. 19, 212–218. doi: 10.1136/amiajnl-2011-000439
Kullo, I. J., Ding, K., Jouni, H., Smith, C. Y., and Chute, C. G. (2010). A genome-wide association study of red blood cell traits using the electronic medical record. PLoS One 5:e13011. doi: 10.1371/journal.pone.0013011
Kurreeman, F., Liao, K., Chibnik, L., Hickey, B., Stahl, E., Gainer, V., et al. (2011). Genetic basis of autoantibody positive and negative rheumatoid arthritis risk in a multi-ethnic cohort derived from electronic health records. Am. J. Hum. Genet. 88, 57–69. doi: 10.1016/j.ajhg.2010.12.007
Liao, K. P., Cai, T., and Gainer, V. (2010). Electronic medical records for discovery research in rheumatoid arthritis. Arthr. Care Res. 62, 1120–1127. doi: 10.1002/acr.20184
Newton, K. M., Peissig, P. L., Kho, A. N., Bielinski, S. J., Berg, R. L., Choudhary, V., et al. (2013). Validation of electronic medical record-based phenotyping algorithms: results and lessons learned from the eMERGE network. J. Am. Med. Inform. Assoc. 20, e147–e154. doi: 10.1136/amiajnl-2012-000896
Olson, J. E., Ryu, E., and Johnson, K. J. (2013). The mayo clinic biobank: a building block for individualized medicine. Mayo Clin Proc. 88, 952–962. doi: 10.1016/j.mayocp.2013.06.006
Ostropolets, A., Christian, R., Patrick, R., Ning, S., George, H., and Chunhua, W. (2020). Adapting electronic health records-derived phenotypes to claims data: Lessons learned in using limited clinical data for phenotyping. J. Biomed. Inform. 102:103363. doi: 10.1016/j.jbi.2019.103363
Pacheco, J., and Thompson, W. (2012). Type 2 Diabetes Mellitus. Northwestern University. PheKB. Available online at: https://phekb.org/phenotype/18
Partners Phenotyping Group (2016). Partners Healthcare. Rheumatoid Arthritis (RA). Available online at: https://phekb.org/phenotype/585 (accessed July 29, 2019).
PheKB (2019). PheKB: A Knowledgebase for Discovering Phenotypes from Electronic Medical Records. Available online at: https://phekb.org/ (accessed August 7, 2019).
Ritchie, M. D., Denny, J. C., Crawford, D. C., Ramirez, A. H., Weiner, J. B., Pulley, J. M., et al. (2010). Robust replication of genotype-phenotype associations across multiple diseases in an electronic medical record. Am. J. Hum. Genet. 86, 560–572. doi: 10.1016/j.ajhg.2010.03.003
Robinson, J. R., Wei, W.-Q., Roden, D. M., and Denny, J. C. (2018). Defining phenotypes from clinical data to drive genomic research. Annu. Rev. Biomed. Data Sci. 1, 69–92.
Rocca, W. A., Grossardt, B. R., Brue, S. M., Bock-Goodner, C. M., Chamberlain, A. M., Wilson, P. M., et al. (2018). Data resource profile: expansion of the Rochester epidemiology project medical records-linkage system (E-REP). Int. J. Epidemiol. 47, 368–368j. doi: 10.1093/ije/dyx268
Teixeira, P. L., Wei, W.-Q., Cronin, R. M., Mo, H., VanHouten, J. P., Carroll, R. J., et al. (2016). Evaluating electronic health record data sources and algorithmic approaches to identify hypertensive individuals. J. Am. Med. Inform. Assoc. 24, 162–171. doi: 10.1093/jamia/ocw071
Wei, W.-Q., and Denny, J. C. (2015). Extracting research-quality phenotypes from electronic health records to support precision medicine. Genome Med. 7:41. doi: 10.1186/s13073-015-0166-y
Wei, W.-Q., Leibson, C. L., Ransom, J. E., Kho, A. N., Caraballo, P. J., Chai, H. S., et al. (2012). Impact of data fragmentation across healthcare centers on the accuracy of a high-throughput clinical phenotyping algorithm for specifying subjects with type 2 diabetes mellitus. J. Am. Med. Inform. Assoc. 19, 219–224. doi: 10.1136/amiajnl-2011-000597
Wei, W.-Q., Leibson, C. L., Ransom, J. E., Kho, A. N., and Chute, C. G. (2013). The absence of longitudinal data limits the accuracy of high-throughput clinical phenotyping for identifying type 2 diabetes mellitus subjects. Int. J. Med. Inform. 82, 239–247. doi: 10.1016/j.ijmedinf.2012.05.015
Keywords: phenotyping algorithms, computational phenotyping, rheumatoid arthritis, type 2 diabetes mellitus, diverse data sources
Citation: Wang L, Olson JE, Bielinski SJ, St. Sauver JL, Fu S, He H, Cicek MS, Hathcock MA, Cerhan JR and Liu H (2020) Impact of Diverse Data Sources on Computational Phenotyping. Front. Genet. 11:556. doi: 10.3389/fgene.2020.00556
Received: 31 October 2019; Accepted: 07 May 2020;
Published: 03 June 2020.
Edited by:
Ronald M. Przygodzki, United States Department of Veterans Affairs, United StatesReviewed by:
Xiaowei Sherry Yan, Sutter Health, United StatesChristopher G. Chute, Johns Hopkins University, United States
Quan Long, University of Calgary, Canada
Copyright © 2020 Wang, Olson, Bielinski, St. Sauver, Fu, He, Cicek, Hathcock, Cerhan and Liu. This is an open-access article distributed under the terms of the Creative Commons Attribution License (CC BY). The use, distribution or reproduction in other forums is permitted, provided the original author(s) and the copyright owner(s) are credited and that the original publication in this journal is cited, in accordance with accepted academic practice. No use, distribution or reproduction is permitted which does not comply with these terms.
*Correspondence: Hongfang Liu, liu.hongfang@mayo.edu