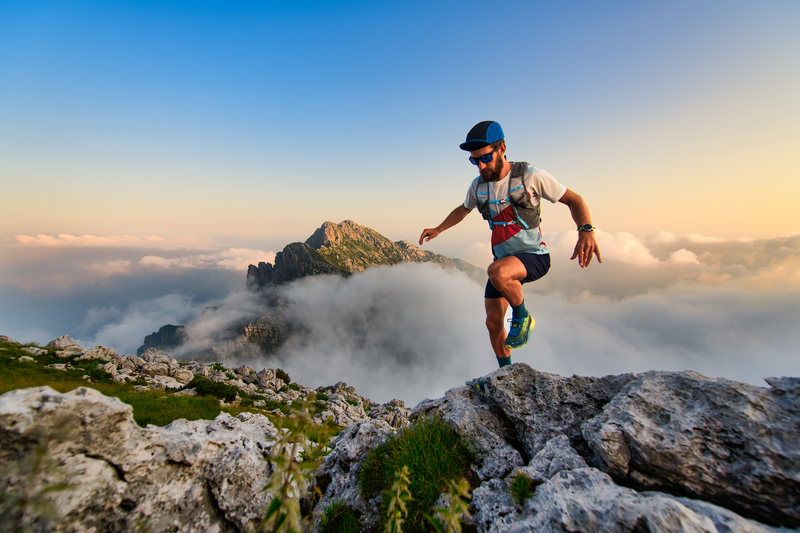
94% of researchers rate our articles as excellent or good
Learn more about the work of our research integrity team to safeguard the quality of each article we publish.
Find out more
ORIGINAL RESEARCH article
Front. Genet. , 04 June 2020
Sec. Computational Genomics
Volume 11 - 2020 | https://doi.org/10.3389/fgene.2020.00535
Jellyfish, such as Chrysaora quinquecirrha, hold an important evolutionary position and have great ecological value. However, limited genomic resources are currently available for studying their basic genetic and development processes. Here, we de novo assembled the first high-quality reference genome of C. quinquecirrha, and successfully annotated 21,606 protein-coding genes. Codon usage analysis identified the frequent use of low-GC-content codons during protein-coding gene translation. Analysis of the relative evolution rate indicated that jellyfish had a faster evolution rate than sea anemones but slower rate than the species in Hydra. Phylogenetic analysis with two other species of jellyfish indicated that Aurelia aurita and Nemopilema nomurai have a closer relationship with each other than with C. quinquecirrha, with divergence from their common ancestor occurring ≈475.7 million years ago. Our study not only showed the genomic characteristics and molecular adaptive evolution of C. quinquecirrha, but also provides valuable genomic resources for further study on complex developmental processes and environmental adaptations.
Jellyfish of the phylum Cnidaria are lower invertebrate gelatinous zooplankton. They are considered one of the most primitive branches of the “tree of life” and thus one of the earliest ancestral species (Lucas and Dawson, 2014; Ou et al., 2015). Jellyfish are generally regarded as diploblastic animals which has endoderm and ectoderm but lack of mesoderm. They further possess a primitive reticular nervous system that controls muscle contractions and that consists of nerve cells connected through nerve projections to form a neural network (Kellie et al., 1980; Ou et al., 2015). In addition, several jellyfishes have no real eyes, but a relatively unsophisticated visual organ made up of fibrous photoreceptors that sense changes in external light (Martin, 2002; Nilsson, 2004; Suga et al., 2008).
The increase in the frequency of jellyfish blooms in recent years has also drawn attention due to their potential to transform marine ecosystems (Brotz et al., 2012; Duarte et al., 2013; Pitt and Lucas, 2014). Jellyfish are carnivores that feed on zooplankton, fish eggs, and larvae, and can greatly impact plankton populations when blooms occur (Lilley et al., 2009; Acuña et al., 2011; Marques et al., 2015; Nagata and Morandini, 2018). However, very few animals feed on jellyfish, and thus the large amounts of carbon sequestered within their bodies is not transferred within the food web. As such, jellyfish are often considered to be at the energy terminal and can seriously affect the marine environment and ecological processes (Pitt et al., 2005; Turk et al., 2008; Condon et al., 2011; Oguz et al., 2012). Several studies have reported that jellyfish blooms have an important relationship with their own physiological reproduction (Hamner and Dawson, 2009; Kawahara et al., 2012; Schiariti et al., 2014). The life cycle of most jellyfish species consists of alternating modes of reproduction between generations, i.e., polyp and medusa (Schiariti et al., 2014; Lee et al., 2017). Under suitable environments, medusae reproduce sexually to produce hydroids, which, in turn, reproduce asexually to produce ephyrae. Finally, ephyrae develop into new medusae, allowing jellyfish to bloom in a cycle of reproduction and growth (Johnson et al., 2001; Fuchs et al., 2014; Schiariti et al., 2014).
There are about 2,000 kinds of jellyfish worldwide, including ∼200 species in the phylum Scyphozoa (Daly et al., 2007; Chang E. S. et al., 2015; Costa et al., 2015). As a representative of Scyphozoa, Chrysaora quinquecirrha is one of the most well-known and well-studied jellyfish species, with high ecological value along the Atlantic coast of the USA and Gulf of Mexico (Cones and Haven, 1969; Calder, 1974; Johnson et al., 2001; Meredith et al., 2016; Bayha et al., 2017). Genomics analysis can help clarify the genetic information and evolutionary origin of different species (Chang E. S. et al., 2015; Kim et al., 2019). Although several jellyfish species’ genome has been studied (Ryan et al., 2013; Gold et al., 2019; Jiang et al., 2019; Kim et al., 2019; Leclère et al., 2019; Ohdera et al., 2019), the genome of C. quinquecirrha has not yet been assembled or analyzed. In this study, we de novo assembled the first high-quality reference genome of C. quinquecirrha, and successfully obtained 21,606 protein-coding genes. Furthermore, based on genomics analysis, we elucidated the evolutionary history and genetic changes of C. quinquecirrha relative to closely related species. This study not only provides valuable information on the evolutionary status and genetic changes of jellyfish, but also provides a foundation for future studies on the development and evolutionary origin of multi-cellular animals.
Fresh muscle samples of jellyfish (Chrysaora quinquecirrha) were dissected and prepared for DNA and RNA extraction. For genome sequencing, we extracted high-quality DNA using a Qiagen Blood & Cell Culture DNA Mini Kit for Nanopore long-read (Oxford Nanopore, United Kingdom) and Illumina short-read (insert size: ∼250 bp; Pair-end 150 bp) sequencing. For RNA sequencing, RNA was extracted from muscle samples by Trizol (Invitrogen) according to the manufacturer’s instructions and sequenced on the Illumina platform (insert size: ∼250 bp; Pair-end 150 bp).
Nanopore long reads were filtered by the mean quality value of each read with in-house Perl scripts, and only reads with a quality value > seven were retained. For Illumina short reads, including genome and transcriptome sequencing data, we used the same standards for quality control. Specifically, any read with more than 50% low-quality bases or 10% unknown bases were filtered, and adaptor sequences and duplicated reads produced during polymerase chain reaction (PCR) were also removed. Then, all the remaining sequencing reads were used for further analysis.
Genome characteristics were evaluated using the genomic short reads based on the k-mer method. The reads were divided into a 17-bp length with 1-bp walking length. The k-mer frequency/number in each k-mer depth was then calculated, and genome size was estimated by the total k-mer number and peak k-mer frequency of 17-mer.
Although the Nanopore sequencing reads have a length advantage over the Illumina sequencing reads, they show low accuracy. In this study, we corrected the sequencing errors in the Nanopore reads using NextDenovo.1 The corrected Nanopore long reads were then used for genome assembly with WTDBG (v2.1) (Ruan and Li, 2019) and parameters: -p 15 –k 7 –AS 2 –E 1 –s 0.05 –L 5000. We further corrected the sequencing errors in the genome assembly with Racon (v1.2.1) and Pilon (v1.21) (Walker et al., 2014). Then, we mapped the corrected Nanopore reads to the assembled contigs by Minimap (v2.9) with parameters: -a –x map-ont –k 17. The haplotigs and low coverage contigs were removed by Purge_haplotigs (v1.1.1). The SSPACE-LongRead (v1.1) was used anchor the contigs to scaffolds, and Gapcloser (v1.10) was used to fill the gaps in the scaffold assembly. To evaluate the integrity of the assembled genome, we aligned all high-quality Illumina sequencing reads to the genome using BWA (v0.7.12) (Li and Durbin, 2009). The integrity of the protein-coding regions in the genome was evaluated by the mapping ratio of transcripts using BLAT (v34) (Kent, 2002).
To identify more complete repetitive sequences in the genome, we used RepeatModeler (v1.0.4)2 for de novo prediction of repetitive sequences, and RepeatMasker (open-4.0.7) (Bedell et al., 2000) for repetitive sequence prediction using both the RepeatModeler results and the public repbase library. RepeatProteinMask (open-4.0.7) was used for predicting transposable elements (TEs) at the protein level, and tandem repeats were analyzed by Tandem Repeat Finder (v4.04) (Benson, 1999).
To exclude the influence of repetitive sequences in the assembled genome during the coding-gene annotation process, we masked all repetitive sequences and then employed coding-gene annotation using different strategies. We first de novo predicted the protein-coding genes using AUGUSTUS (v2.5.5) (Stanke and Waack, 2003). We next downloaded several published gene sets, including Hydra vulgaris (GCF_000004095.1), Stylophora pistillata (GCF_002571385.1), Acropora digitifera (GCF_000222465.1), Nematostella vectensis (GCA_000209225.1), Exaiptasia pallida (GCF_001417965.1), Aurelia aurita (GCA_004194415.1), and Renilla muelleri (GigaDB) (Putnam et al., 2007; Chapman et al., 2010; Shinzato et al., 2011; Baumgarten et al., 2015; Voolstra et al., 2017; Gold et al., 2019; Jiang et al., 2019). We then aligned all gene sets to the annotated C. quinquecirrha protein sequences by tblastn (e = 10e-5) and predicted the gene structure using Genewise (v2-2-0) (Birney et al., 2004). After this, we de novo assembled the transcripts using Bridger software (r2014-12-01) (Chang Z. et al., 2015) and used them for coding-region prediction. Lastly, we merged the above results using EvidenceModeler (v1.1.1) (Haas et al., 2008). To better understand the biological functions of the annotated genes, we aligned them to public databases, including the InterPro, Gene Ontology (GO), SwissProt, TrEMBL, and Kyoto Encyclopedia of Genes and Genomes (KEGG).
Orthologous gene identification was conducted among these 10 species, including H. vulgaris, S. pistillata, A. digitifera, N. vectensis, E. pallida, A. aurita, R. muelleri, Nemopilema nomurai, Echinococcus granulosus, and C. quinquecirrha. Protein-coding genes among these species were used for orthologous relationship determination with OrthoMCL (v2.0.9) (Li et al., 2003). From these results, the 1:1 single-copy genes among the 10 species were selected and used for specific analyses.
To determine the phylogenetic relationships among the 10 species, we aligned the 1:1 single-copy genes using MUSCLE (v3.8.31) (Edgar, 2004). We then conducted phylogenetic analysis in RAxML (v8.2.10) (Stamatakis, 2014), with E. granulosus as the outgroup. Divergence time analysis was conducted using the MCMCtree program in PAML (v4.8) (Yang, 2007), and fossil records downloaded from the TIMETREE website3 were used for result calibration.
The protein-coding genes were used for codon usage analysis with CodonW (1.4.4; -all_indices -c_type 2 -f_type 4 -nomenu -nowarn -totals) (Peden, 1999) and in-house Perl scripts. Relative evolution rates among different species were analyzed using LINTRE (njboot -d7; tpcv -d7 -o 1) (Takezaki et al., 1995) and MEGA software (Tajima’s Relative Rate Test) (Kumar et al., 2018), with C. quinquecirrhaas the reference species and E. granulosus as the outgroup.
Gene family expansion and contraction were analyzed using CAFÉ (v3.1) (De Bie et al., 2006) with three input files: i.e., (1) Phylogenetic relationships were determined by RAxML (Stamatakis, 2014), (2) Divergence time was determined by MCMCtree (Yang, 1997), and (3) Orthologous relationships were determined by OrthoMCL (Li et al., 2003). The expanded or contracted gene families in the three jellyfish species were selected for further analysis.
To acquire the C. quinquecirrha reference genome, we first extracted DNA from muscle tissue for genome sequencing. We then obtained 51.46 Gb of Illumina short reads (Supplementary Table S1) and determined the genome characteristics (e.g., genome size, repetitive sequence content, heterozygosity ratio). We used the 17-mer method and found the C. quinquecirrha genome is very complex, with high heterozygosity and repeat sequences, and has a genome size of 330.67 Mb (Figure 1). To better complete the assembly, we next sequenced the genome on the Nanopore platform (Promethion, Oxford Nanopore Technology) and acquired 81.12 Gb of high-quality reads, accounting for ∼245 genome coverage (Supplementary Table S2). We then corrected the potential sequencing errors in the Nanopore long reads with Nextdenovo1 and assembled the genome with WTDBG (Ruan and Li, 2019). To further improve the base accuracy of the acquired genome, we polished the assembly by the Nanopore and Illumina sequencing data with Racon and Pilon, respectively. Then, the haplotigs and low coverage contigs were removed by Purge_haplotigs, scaffolded by SSPACE-LongRead, and gap-filled by Gapcloser software. Finally, we got a genome assembly with the contig N50 and scaffold N50 length of 230.04 and 733.65 Kb, respectively (Table 1). This could comparable with previously published high-quality genomes of closely related species (Supplementary Table S3). We then aligned the genome assembly with the core gene set in BUSCO (Simão et al., 2015), with nearly 80% of the conserved gene set among eukaryotes were found in the genome (Supplementary Table S4). We also mapped the Illumina short reads (Supplementary Table S1) and de novo assembled transcripts (with 99.6% BUSCO values) (Supplementary Tables S5–S7) and found that most could be successfully aligned to the genome assembly (Supplementary Tables S8, S9). Thus, we obtained a high-quality (high accuracy and connectivity) reference genome for C. quinquecirrha.
Genome annotation, which can greatly help to improve our understanding of species, was used in the current study. By combining the repetitive annotation results from several repeat annotation software, we successfully acquired 149.86 Mb of repetitive sequences, accounting for 44.49% of the assembled genome (Supplementary Table S10). Furthermore, 40.78% of the genome consisted of transposable elements (TEs) (Table 2), which could be divided into long terminal repeats (LTR, 4.07%), DNA elements (6.27%), short interspersed nuclear elements (SINE, 0.46%), and long interspersed nuclear elements (LINE, 5.70%). After repetitive sequence annotation, we first masked all repetitive sequences in the genome and employed protein-coding gene annotation by combining de novo prediction, homolog-based annotation, and transcript-based annotation. Finally, we merged the gene sets from the different strategies using EvidenceModeler software (Haas et al., 2008) and acquired 21,606 high-quality protein-coding genes in the C. quinquecirrha genome (Supplementary Table S11). We then compared and evaluated the annotation quality of the gene sets and found the quality to be comparable to that of closely related species (Figure 2). To better understand the biological functions of these genes, we performed functional annotation by aligning the protein sequences to the public databases, including GO, KEGG, InterPro, SwissProt, and TrEMBL. Most protein-coding genes could be found in the databases (Table 3), suggesting that we acquired a high-quality protein-coding gene set of the C. quinquecirrha genome. In addition to the coding-genes have key roles in biological processes, studies have shown that many non-coding RNAs (ncRNAs) also participate in and regulate many important physiological processes (Wilusz et al., 2009; Rinn and Guttman, 2011; Wang and Chang, 2011; Ulitsky and Bartel, 2013). Therefore, we systematically annotated and identified the ncRNAs in the C. quinquecirrha genome, including 7,833 tRNAs, 857 rRNAs, 745 snRNAs, and 50 miRNAs. These results could help clarify the functions of ncRNA in C. quinquecirrha (Table 4).
The genome sizes varied widely among Cnidaria species, especially in jellyfish (Figure 3), but the reasons of the genome expansion remain unclear. Thus, we compared the content of TEs (including LINE, SINE, LTR, and DNA elements) and coding regions among these species, and found that the main contributors to jellyfish genome expansion were non-coding regions (e.g., TEs), rather than coding regions (Figure 3). Further analysis demonstrated that the largest expansion of TEs in the C. quinquecirrha genome was that of SINE, with 5.40 times more SINE than that found in N. nomurai (Figure 3). To clarify the insertion history of TEs in jellyfish, we further analyzed the expansion history and found that TE expansion occurred within ∼235 million years in the three jellyfish species studied, and that the different insertion/expansion rates caused the differences in genome size (Figure 4).
For comparative genomics analysis, we first downloaded the protein-coding genes of several species, including N. vectensis, A. digitifera, N. nomurai, E. pallid, S. pistillata, A. aurita, R. muelleri, E. granulosus, and H. vulgaris. Cluster relationships among these protein-coding genes were then determined by OrthoMCL (Li et al., 2003). We identified 26,613 gene families among the 10 species and 459 1:1 single-copy genes (Figure 5A and Supplementary Table S12). To identify gene families that may contribute to their unique characteristics, we conducted gene family analysis for the three jellyfish species relative to the other seven species, and found 728 gene families that specifically existed in jellyfish (Figure 5B), suggesting the possible unique functions of these genes in jellyfish. We further conducted enrichment analysis of the specific gene families. Results showed enrichment in several biological processes, including Hedgehog signaling pathway (P = 0.001342) and TGF-beta signaling pathway (P = 0.012341; Supplementary Table S13), thus suggesting that these genes contributed to unique development and adaptive evolution.
Figure 5. Gene family analysis of these species. (A) Gene family statistics among species. (B) Shared or specific gene families among species.
Although the phylogenetic relationships of jellyfishes and their closely related species have been investigated (Kayal et al., 2018), the whole-genome level phylogenetic tree of C. quinquecirrha and other species have not been studied. Here, we analyzed their phylogenetic relationships using RAxML software (PROTGAMMAJTT model; 100 bootstrap replicates) (Stamatakis, 2014). Results showed that A. aurita and N. nomurai has a close relationship than with C. quinquecirrha (Figure 6A). Divergence time analysis indicated that A. aurita and N. nomurai diverged 403.6 million years ago (Mya), and C. quinquecirrha diverged with the common ancestor of A. aurita and N. nomurai 475.7 Mya (Figure 6A and Supplementary Table S14). Furthermore, we conducted gene family analysis and identified 85 expanded and 64 contracted gene families (P < 0.05) in jellyfish. Functional analysis identified 2 GO and 30 KEGG terms were enriched in expansion, respectively (Supplementary Tables S15, S16). We found that biological processes, such as dorso-ventral axis formation (P = 0.000264635), fatty acid degradation (P = 0.002908315), and Notch signaling pathway (P = 0.005299582), were expanded in jellyfish relative to closely related species (Supplementary Table S16), suggesting genes in these families may have important functions in jellyfish.
Figure 6. Phylogenetic relationship and relative evolution rate analyses of these species. (A) Phylogenetic relationship among these species. (B) Relative evolution rate among these species.
Jellyfishes are relatively ancient and simple multi-cellular organisms. Whether they exhibit similar codon usage with other species remains unclear. Here, we performed codon usage analysis in jellyfish and found it has no obvious differences with closely related species (Supplementary Table S17). Based on manually checking, we identified the frequent low-GC-content codons usage in C. quinquecirrha, including Glu and Asp (Supplementary Table S18), suggesting that low-energy codons are more commonly used in C. quinquecirrha. We next analyzed the relative evolution rate of species, with C. quinquecirrha as the reference and E. granulosus as the outgroup. Results indicated that jellyfish have a faster evolution rate than sea anemones but a slower rate than the species in Hydra (Figure 6B and Supplementary Tables S19, S20), suggesting different survival pressures and environmental adaptations during their evolutionary history.
We de novo assembled the first high-quality reference genome of C. quinquecirrha, with a scaffold N50 length of 733.65 Kb, and annotated 21,606 protein-coding genes. The ncRNAs annotation, especially for miRNA, could help us study the expression regulation of coding genes in the future. Comparative genomics analysis indicated that the large C. quinquecirrha genome was mainly due to non-coding region expansion. Codon usage analysis indicated that C. quinquecirrha tends to use low-energy codons in the protein-coding genes. Furthermore, results demonstrated that C. quinquecirrha has a relatively faster evolution rate than sea anemones but slower evolution rate than the species in Hydra. Phylogenetic results indicated that A. aurita and N. nomurai are more closely related to each other than to C. quinquecirrha, with divergence between their common ancestor and C. quinquecirrha occurring 475.7 Mya. Simple morphological considerations, several previous studies got different phylogenetic relationships among these species. Our study analyzed the phylogeny by the whole-genome data could help us better understand the evolution and their relationships in Cnidaria.
All raw sequencing reads were deposited in the Sequence Read Archive (SRA) database of National Center for Biotechnology Information (NCBI) with accession number of PRJNA555711. Besides, the genome assembly (https://www.ncbi.nlm.nih.gov/assembly/GCA_012295145.1) and transcript assembly (https://www.ncbi.nlm.nih.gov/nuccore/GILX00000000) were also uploaded to the NCBI database. All the other data, including genome assembly file, gff file, protein-coding genes, and the de novo assembled transcripts with the RNA-seq data, were uploaded to the DRYAD database (https://doi.org/10.5061/dryad.brv15dv6c).
YL and XG conceived and supervised the project and revised the manuscript. WX and YL collected samples. WX and Hao Li performed bioinformatics analyses. WX and Hao Li wrote the manuscript. WC, Hon Li, and YM revised the manuscript. All authors have read and approved the final manuscript.
This work was supported by the Youth Innovation Team of Shaanxi Universities and the Science and Technology Innovation Base-Open and Sharing Platform of Science and Technology Resources Project of Shaanxi Province (2019PT-26). This work was also supported by Grant No. 81971330, National Natural Science Foundation of China (31660656) and Grant No. 81873740 from the National Natural Science Foundation of China, and the Yunnan Provincial Department of Education Research Fund (2019J0150).
The authors declare that the research was conducted in the absence of any commercial or financial relationships that could be construed as a potential conflict of interest.
We thank Christine Watts for suggestions in modifying the manuscript.
The Supplementary Material for this article can be found online at: https://www.frontiersin.org/articles/10.3389/fgene.2020.00535/full#supplementary-material
Acuña, J. L., López-Urrutia, Á., and Colin, S. (2011). Faking giants: the evolution of high prey clearance rates in jellyfishes. Science 333, 1627–1629. doi: 10.1126/science.1205134
Baumgarten, S., Simakov, O., Esherick, L. Y., Liew, Y. J., Lehnert, E. M., Michell, C. T., et al. (2015). The genome of Aiptasia, a sea anemone model for coral symbiosis. Proc. Natl. Acad. Sci. U.S.A. 112, 11893–11898. doi: 10.1073/pnas.1513318112
Bayha, K. M., Collins, A. G., and Gaffney, P. M. (2017). Multigene phylogeny of the scyphozoan jellyfish family pelagiidae reveals that the common US Atlantic sea nettle comprises two distinct species (Chrysaora quinquecirrha and C. chesapeakei). PeerJ 5:e3863. doi: 10.7717/peerj.3863
Bedell, J. A., Korf, I., and Gish, W. (2000). MaskerAid: a performance enhancement to RepeatMasker. Bioinformatics 16, 1040–1041. doi: 10.1093/bioinformatics/16.11.1040
Benson, G. (1999). Tandem repeats finder: a program to analyze DNA sequences. Nucleic Acids Res. 27, 573–580. doi: 10.1093/nar/27.2.573
Brotz, L., Cheung, W. W., Kleisner, K., Pakhomov, E., and Pauly, D. (2012). Increasing jellyfish populations: trends in large marine ecosystems. Hydrobiologia 690, 3–20.
Calder, D. R. (1974). Strobilation of the sea nettle, Chrysaora quinquecirrha, under field conditions. Biol. Bull. 146, 326–334.
Chang, E. S., Neuhof, M., Rubinstein, N. D., Diamant, A., Philippe, H., Huchon, D., et al. (2015). Genomic insights into the evolutionary origin of Myxozoa within Cnidaria. Proc. Natl. Acad. Sci. U.S.A. 112, 14912–14917. doi: 10.1073/pnas.1511468112
Chang, Z., Li, G., Liu, J., Zhang, Y., Ashby, C., Liu, D., et al. (2015). Bridger: a new framework for de novo transcriptome assembly using RNA-seq data. Genome Biol. 16:30. doi: 10.1186/s13059-015-0596-2
Chapman, J. A., Kirkness, E. F., Simakov, O., Hampson, S. E., Mitros, T., Weinmaier, T., et al. (2010). The dynamic genome of Hydra. Nature 464, 592–596. doi: 10.1038/nature08830
Condon, R. H., Steinberg, D. K., Del Giorgio, P. A., Bouvier, T. C., Bronk, D. A., Graham, W. M., et al. (2011). Jellyfish blooms result in a major microbial respiratory sink of carbon in marine systems. Proc. Natl. Acad. Sci. U.S.A. 108, 10225–10230. doi: 10.1073/pnas.1015782108
Cones, H. N., and Haven, D. S. (1969). Distribution of Chrysaora quinquecirrha in the York river. Chesapeake Sci. 10, 75–84.
Costa, E., Gambardella, C., Piazza, V., Greco, G., Lavorano, S., Beltrandi, M., et al. (2015). Effect of neurotoxic compounds on ephyrae of Aurelia aurita jellyfish. Hydrobiologia 759, 75–84.
Daly, M., Brugler, M. R., Cartwright, P., Collins, A. G., Dawson, M. N., Fautin, D. G., et al. (2007). The phylum Cnidaria: a review of phylogenetic patterns and diversity 300 years after Linnaeus. Zootaxa 1668, 127–182.
De Bie, T., Cristianini, N., Demuth, J. P., and Hahn, M. W. (2006). CAFE: a computational tool for the study of gene family evolution. Bioinformatics 22, 1269–1271. doi: 10.1093/bioinformatics/btl097
Duarte, C. M., Pitt, K. A., Lucas, C. H., Purcell, J. E., Uye, S.-I., Robinson, K., et al. (2013). Is global ocean sprawl a cause of jellyfish blooms? Front. Ecol. Environ. 11, 91–97.
Edgar, R. C. (2004). MUSCLE: a multiple sequence alignment method with reduced time and space complexity. BMC Bioinformatics 5:113. doi: 10.1186/1471-2105-5-113
Fuchs, B., Wang, W., Graspeuntner, S., Li, Y., Insua, S., Herbst, E.-M., et al. (2014). Regulation of polyp-to-jellyfish transition in Aurelia aurita. Curr. Biol. 24, 263–273. doi: 10.1016/j.cub.2013.12.003
Gold, D. A., Katsuki, T., Li, Y., Yan, X., Regulski, M., Ibberson, D., et al. (2019). The genome of the jellyfish Aurelia and the evolution of animal complexity. Nat. Ecol. Evol. 3, 96–104. doi: 10.1038/s41559-018-0719-8
Haas, B. J., Salzberg, S. L., Zhu, W., Pertea, M., Allen, J. E., Orvis, J., et al. (2008). Automated eukaryotic gene structure annotation using EVidenceModeler and the Program to Assemble Spliced Alignments. Genome Biol. 9:R7. doi: 10.1186/gb-2008-9-1-r7
Hamner, W. M., and Dawson, M. N. (2009). A review and synthesis on the systematics and evolution of jellyfish blooms: advantageous aggregations and adaptive assemblages. Hydrobiologia 616, 161–191.
Jiang, J. B., Quattrini, A. M., Francis, W. R., Ryan, J. F., Rodríguez, E., and McFadden, C. S. (2019). A hybrid de novo assembly of the sea pansy (Renilla muelleri) genome. Gigascience 8:giz026. doi: 10.1093/gigascience/giz026
Johnson, D. R., Perry, H. M., and Burke, W. D. (2001). Developing jellyfish strategy hypotheses using circulation models. Hydrobiologia 451, 213–221.
Kawahara, M., Ohtsu, K., and Uye, S.-I. (2012). Bloom or non-bloom in the giant jellyfish Nemopilema nomurai (Scyphozoa: Rhizostomeae): roles of dormant podocysts. J. Plankton Res. 35, 213–217.
Kayal, E., Bentlage, B., Pankey, M. S., Ohdera, A. H., Medina, M., Plachetzki, D. C., et al. (2018). Phylogenomics provides a robust topology of the major cnidarian lineages and insights on the origins of key organismal traits. BMC Evol. Biol. 18:68. doi: 10.1186/s12862-018-1142-0
Kellie, S., Evans, C. W., and Kemp, G. (1980). The effects of serum-proteins on the adhesion of lymphocytes. Cell Biol. Int. Rep. 4:777.
Kim, H.-M., Weber, J. A., Lee, N., Park, S. G., Cho, Y. S., Bhak, Y., et al. (2019). The genome of the giant Nomura’s jellyfish sheds light on the early evolution of active predation. BMC Biol. 17:28. doi: 10.1186/s12915-019-0643-7
Kumar, S., Stecher, G., Li, M., Knyaz, C., and Tamura, K. (2018). MEGA X: molecular evolutionary genetics analysis across computing platforms. Mol. Biol. Evol. 35, 1547–1549. doi: 10.1093/molbev/msy096
Leclère, L., Horin, C., Chevalier, S., Lapébie, P., Dru, P., Péron, S., et al. (2019). The genome of the jellyfish Clytia hemisphaerica and the evolution of the cnidarian life-cycle. Nat. Ecol. Evol. 3, 801–810. doi: 10.1038/s41559-019-0833-2
Lee, H. E., Han, C. H., Kim, B., and Yoon, W. D. (2017). Effects of temperature and salinity on the asexual reproduction of Nemopilema nomurai (Scyphozoa: Rhizostomeae). Ocean Sci. J. 52, 573–579.
Li, H., and Durbin, R. (2009). Fast and accurate short read alignment with Burrows–Wheeler transform. Bioinformatics 25, 1754–1760. doi: 10.1093/bioinformatics/btp324
Li, L., Stoeckert, C. J., and Roos, D. S. (2003). OrthoMCL: identification of ortholog groups for eukaryotic genomes. Genome Res. 13, 2178–2189. doi: 10.1101/gr.1224503
Lilley, M., Houghton, J., and Hays, G. (2009). Distribution, extent of inter-annual variability and diet of the bloom-forming jellyfish Rhizostoma in European waters. J. Mar. Biol. Assoc. U.K. 89, 39–48.
Lucas, C. H., and Dawson, M. N. (2014). “What are jellyfishes and thaliaceans and why do they bloom?,” in Jellyfish Blooms, eds K. Pitt and C. Lucas (Dordrecht: Springer), 9–44.
Marques, F., Chainho, P., Costa, J., Domingos, I., and Angélico, M. (2015). Abundance, seasonal patterns and diet of the non-native jellyfish Blackfordia virginica in a Portuguese estuary. Estuar. Coast. Shelf Sci. 167, 212–219.
Meredith, R. W., Gaynor, J. J., and Bologna, P. A. (2016). Diet assessment of the Atlantic Sea Nettle Chrysaora quinquecirrha in Barnegat Bay, New Jersey, using next-generation sequencing. Mol. Ecol. 25, 6248–6266. doi: 10.1111/mec.13918
Nagata, R. M., and Morandini, A. C. (2018). Diet, prey selection, and individual feeding rates of the jellyfish Lychnorhiza lucerna (Scyphozoa, Rhizostomeae). Mar. Biol. 165:187.
Nilsson, D.-E. (2004). Eye evolution: a question of genetic promiscuity. Curr. Opin. Neurobiol. 14, 407–414. doi: 10.1016/j.conb.2004.07.004
Oguz, T., Salihoglu, B., Moncheva, S., and Abaza, V. (2012). Regional peculiarities of community-wide trophic cascades in strongly degraded Black Sea food web. J. Plankton Res. 34, 338–343.
Ohdera, A., Ames, C. L., Dikow, R. B., Kayal, E., Chiodin, M., Busby, B., et al. (2019). Box, stalked, and upside-down? Draft genomes from diverse jellyfish (Cnidaria, Acraspeda) lineages: Alatina alata (Cubozoa), Calvadosia cruxmelitensis (Staurozoa), and Cassiopea xamachana (Scyphozoa). Gigascience 8:giz069. doi: 10.1093/gigascience/giz069
Ou, Q., Xiao, S., Han, J., Sun, G., Zhang, F., Zhang, Z., et al. (2015). A vanished history of skeletonization in Cambrian comb jellies. Sci. Adv. 1:e1500092. doi: 10.1126/sciadv.1500092
Pitt, K., Koop, K., and Rissik, D. (2005). Contrasting contributions to inorganic nutrient recycling by the co-occurring jellyfishes, Catostylus mosaicus and Phyllorhiza punctata (Scyphozoa, Rhizostomeae). J. Exp. Mar. Biol. Ecol. 315, 71–86.
Putnam, N. H., Srivastava, M., Hellsten, U., Dirks, B., Chapman, J., Salamov, A., et al. (2007). Sea anemone genome reveals ancestral eumetazoan gene repertoire and genomic organization. Science 317, 86–94. doi: 10.1126/science.1139158
Rinn, J., and Guttman, M. (2011). RNA and dynamic nuclear organization: long noncoding RNAs may function as organizing factors that shape the cell nucleus. Science 345, 1240–1241.
Ruan, J., and Li, H. (2019). Fast and accurate long-read assembly with wtdbg2. BioRxiv [Preprint] doi: 10.1101/530972
Ryan, J. F., Pang, K., Schnitzler, C. E., Nguyen, A.-D., Moreland, R. T., Simmons, D. K., et al. (2013). The genome of the ctenophore Mnemiopsis leidyi and its implications for cell type evolution. Science 342:1242592. doi: 10.1126/science.1242592
Schiariti, A., Morandini, A. C., Jarms, G., von Glehn Paes, R., Franke, S., and Mianzan, H. (2014). Asexual reproduction strategies and blooming potential in Scyphozoa. Mar. Ecol. Prog. Ser. 510, 241–253.
Shinzato, C., Shoguchi, E., Kawashima, T., Hamada, M., Hisata, K., Tanaka, M., et al. (2011). Using the Acropora digitifera genome to understand coral responses to environmental change. Nature 476, 320–323. doi: 10.1038/nature10249
Simão, F. A., Waterhouse, R. M., Ioannidis, P., Kriventseva, E. V., and Zdobnov, E. M. (2015). BUSCO: assessing genome assembly and annotation completeness with single-copy orthologs. Bioinformatics 31, 3210–3212. doi: 10.1093/bioinformatics/btv351
Stamatakis, A. (2014). RAxML version 8: a tool for phylogenetic analysis and post-analysis of large phylogenies. Bioinformatics 30, 1312–1313. doi: 10.1093/bioinformatics/btu033
Stanke, M., and Waack, S. (2003). Gene prediction with a hidden Markov model and a new intron submodel. Bioinformatics 19(Suppl._2), ii215–ii225. doi: 10.1093/bioinformatics/btg1080
Suga, H., Schmid, V., and Gehring, W. J. (2008). Evolution and functional diversity of jellyfish opsins. Curr. Biol. 18, 51–55. doi: 10.1016/j.cub.2007.11.059
Takezaki, N., Rzhetsky, A., and Nei, M. (1995). Phylogenetic test of the molecular clock and linearized trees. Mol. Biol. Evol. 12, 823–833. doi: 10.1093/oxfordjournals.molbev.a040259
Turk, V., Lučić, D., Flander-Putrle, V., and Malej, A. (2008). Feeding of Aurelia sp.(Scyphozoa) and links to the microbial food web. Mar. Ecol. 29, 495–505.
Ulitsky, I., and Bartel, D. P. (2013). lincRNAs: genomics, evolution, and mechanisms. Cell 154, 26–46.
Voolstra, C. R., Li, Y., Liew, Y. J., Baumgarten, S., Zoccola, D., Flot, J.-F., et al. (2017). Comparative analysis of the genomes of Stylophora pistillata and Acropora digitifera provides evidence for extensive differences between species of corals. Sci. Rep. 7:17583. doi: 10.1038/s41598-017-17484-x
Walker, B. J., Abeel, T., Shea, T., Priest, M., Abouelliel, A., Sakthikumar, S., et al. (2014). Pilon: an integrated tool for comprehensive microbial variant detection and genome assembly improvement. PLoS One 9:e112963. doi: 10.1371/journal.pone.0112963
Wang, K. C., and Chang, H. Y. (2011). Molecular mechanisms of long noncoding RNAs. Mol. Cell 43, 904–914. doi: 10.1016/j.molcel.2011.08.018
Wilusz, J. E., Sunwoo, H., and Spector, D. L. (2009). Long noncoding RNAs: functional surprises from the RNA world. Genes Dev. 23, 1494–1504. doi: 10.1101/gad.1800909
Yang, Z. (1997). PAML: a program package for phylogenetic analysis by maximum likelihood. Comput. Appl. In Biosci. Cabios 13, 555–556. doi: 10.1093/bioinformatics/13.5.555
Keywords: jellyfish, genome, evolution, assembly, adaptation
Citation: Xia W, Li H, Cheng W, Li H, Mi Y, Gou X and Liu Y (2020) High-Quality Genome Assembly of Chrysaora quinquecirrha Provides Insights Into the Adaptive Evolution of Jellyfish. Front. Genet. 11:535. doi: 10.3389/fgene.2020.00535
Received: 12 January 2020; Accepted: 04 May 2020;
Published: 04 June 2020.
Edited by:
Nunzio D’Agostino, Università degli Studi di Napoli Federico II, ItalyReviewed by:
Konstantin Khalturin, Okinawa Institute of Science and Technology Graduate University, JapanCopyright © 2020 Xia, Li, Cheng, Li, Mi, Gou and Liu. This is an open-access article distributed under the terms of the Creative Commons Attribution License (CC BY). The use, distribution or reproduction in other forums is permitted, provided the original author(s) and the copyright owner(s) are credited and that the original publication in this journal is cited, in accordance with accepted academic practice. No use, distribution or reproduction is permitted which does not comply with these terms.
*Correspondence: Xingchun Gou, Z291eGluZ2NodW5AMTg5LmNu; Yaowen Liu, eWFvd2VubGl1QGZveG1haWwuY29t
†These authors have contributed equally to this work
Disclaimer: All claims expressed in this article are solely those of the authors and do not necessarily represent those of their affiliated organizations, or those of the publisher, the editors and the reviewers. Any product that may be evaluated in this article or claim that may be made by its manufacturer is not guaranteed or endorsed by the publisher.
Research integrity at Frontiers
Learn more about the work of our research integrity team to safeguard the quality of each article we publish.