- 1College of Physics, Jilin University, Changchun, China
- 2Department of Biochemistry and Molecular Biology, Institute of Bioinformatics, The University of Georgia, Athens, GA, United States
- 3Cancer Systems Biology Center, The China-Japan Union Hospital, Jilin University, Changchun, China
Quantitative assessment of the intracellular oxidative stress level is a very important problem since it is the basis for elucidation of the fundamental causes of metabolic changes in diseased human cells, particularly cancer. However, the problem proves to be very challenging to solve in vivo because of the complex nature of the problem. Here a computational method is presented for predicting the quantitative level of the intracellular oxidative stress in cancer tissue cells. The basic premise of the predictor is that the genomic mutation level is strongly associated with the intracellular oxidative stress level. Based on this, a statistical analysis is conducted to identify a set of enzyme-encoding genes, whose combined expression levels can well explain the mutation rates in individual cancer tissues in the TCGA database. We have assessed the validity of the predictor by assessing it against genes that are known to have anti-oxidative functions for specific types of oxidative stressors. Then the applications of the predictor are conducted to illustrate its utility.
Introduction
Oxidative stress has long been recognized as a cellular stress associated with cancer formation and development (Cerutti and Trump, 1993; Wangpaichitr et al., 2009; Bellezza et al., 2010; Chan et al., 2010; Conti et al., 2010; Dayem et al., 2010; Gibellini et al., 2010; Henkler et al., 2010; Karlenius and Tonissen, 2010; Massi et al., 2010; Ortega et al., 2010; Pizzimenti et al., 2010; Reuter et al., 2010; Schultz et al., 2010; Soory, 2010; Sun et al., 2010; Valle et al., 2010; Gorrini et al., 2013; Sosa et al., 2013; Leone et al., 2017). It refers to the gap between the intracellular oxidizing power and the anti-oxidation capacity in a human cell. Numerous authors have pointed out that oxidative stress may be responsible for the induction of a variety of altered metabolisms in cancer, which include (i) considerably increased lipid metabolism (Santos and Schulze, 2012; Zhang and Du, 2012; Alfaradhi et al., 2014; Zhang et al., 2014), (ii) altered metabolisms of sulfur-containing amino acids (Schulz et al., 2000; Zhang et al., 2012; Campbell et al., 2016; Martínez et al., 2017), (iii) reprogrammed sugar metabolisms (Takeyama et al., 2000; Wu et al., 2009; Dewald et al., 2016), among other reprogrammed metabolisms. Increased genomic mutations in cancer have also been attributed to oxidative stress (Limoli and Giedzinski, 2003; Doudican et al., 2005; Slane et al., 2006; Xu et al., 2014; Fitzgerald et al., 2017; Markkanen, 2017; Rao et al., 2017; Tubbs and Nussenzweig, 2017). However, it has proven to be very challenging to accurately estimate the level of intracellular oxidative stress (Selvaraj et al., 2002; Farah, 2005). Because existing techniques are mostly designed to detect quantitatively specific oxidizing molecular species (e.g., H2O2 and O2) rather than detecting the overall level of oxidative stress. Computational techniques could potentially play a key role in estimating the quantitative level of oxidative stress.
Unlike hypoxia or acid-base imbalance whose related stress can be measured/estimated in terms of the concentration of one or a few molecular species such as O2 or H+, there are many types of oxidizing molecules such as reactive oxygen species (Barash et al., 2010; Reczek and Chandel, 2017), reactive nitrogen species (Fionda et al., 2016; Kruk and Aboul-Enein, 2017), reactive lipid species (Higdon et al., 2012; Graham, 2016) among others. Furthermore, to estimate the stress level, it also requires to know the total cellular reducing capacity. This is also challenging since human cells not only have a basic set of anti-oxidation (or reducing) capacities consisting of (1) glutathione and associated enzymes (e.g., glutathione transferase and peroxidase), (2) vitamin A, C, E and derivatives like beta-carotene, and (3) anti-oxidation enzymes like SOD (superoxide dismutase), PRX (periaxin), and TRX (thioredoxin) (Chang et al., 2008; Mantovani et al., 2010), but also rely on anti-oxidation capacities of various other molecules including some enzymes and fatty acids. Among them, the main functions of the enzymes may not be for anti-oxidation (Osmundsen et al., 1982; Wieczorek et al., 2017), while the anti-oxidative properties of fatty acids have long been well-established (Richard et al., 2008; Freitas et al., 2017). All these make it very difficult to pin down on what molecular species should be used when assessing the level of oxidative stress.
A few proposals have been made regarding possible biomarkers for intracellular level of oxidative stress such as the carbonylation level (aldehydes and ketones) of proteins (Dalle-Donne et al., 2003; Hacişevki et al., 2012; Fernando et al., 2016), the level of oxidized low-density lipoprotein (Itabe, 2012; Osman et al., 2016), oxidized products of lipids such as 4-HNE (4-hydroxynonenal) and MDA (malondialdehyde) (Niki, 2008; Halder and Bhattacharyya, 2014; Teppner et al., 2015), and protein thiols (Giustarini et al., 2012, 2017). There are two general issues with these biomarkers: (1) they tend to reflect the level of oxidation by specific oxidizing molecules; and (2) more importantly, they are not high-throughput, hence it is impractical for large-scale analyses, such as analyses of TCGA tissue samples (https://portal.gdc.cancer.gov/) to elucidate possible causes of specific metabolic changes in such tissues.
The goal here is to identify a set of genes whose mRNA expression levels can collectively reflect the overall level of intracellular oxidative stress present in a cancer tissue. The strategy is: (1) the somatic point-mutation rate in each cancer tissue sample is used as an indicator for the level of intracellular oxidative stress, an idea that has been well-established and applied (Doudican et al., 2005; Slane et al., 2006; Xu et al., 2014; Fitzgerald et al., 2017; Markkanen, 2017; Rao et al., 2017; Tubbs and Nussenzweig, 2017); (2) a few enzyme classes are selected, which are known to have anti-oxidation activities such as EC 3.1.-, EC 3.6.-, EC 2.4.-, and EC 2.7.- (Kato et al., 1995; Kong et al., 2007); and (3) a subset of genes are selected from these enzyme classes, whose combined expressions correlate strongly with the mutation rates in the matching genomes, determined through regression analyses. One assumption used in the analysis is that the oxidative stress levels in cytosol and nucleus are the same, which is reasonable knowing that the nuclear pores are large enough to allow most of the oxidizing molecules to go through freely between the two compartments; and increased mutation rates in cancer are known to be related to the nucleus oxidative stress level (Chung et al., 2014; Markkanen, 2017).
By applying this strategy to gene-expression data and matching genomic mutation data of cancer tissues of 14 cancer types in TCGA, representing all those with sufficiently large sample sizes, we have trained a predictor for the intracellular level of oxidative stress for each of the 14 cancer types. They are BLCA (bladder urothelial carcinoma), BRCA (breast invasive carcinoma), COAD (colon adenocarcinoma), ESCA (esophageal carcinoma), HNSC (head and neck squamous cell carcinoma), KICH (kidney renal papillary cell carcinoma), KIRC (kidney chromophobe), KIRP (kidney renal clear cell carcinoma), LICH (liver hepatocellular carcinoma), LUAD (lung squamous cell carcinoma), LUSC (lung adenocarcinoma), PRAD (prostate adenocarcinoma), STAD (stomach adenocarcinoma), and THCA (thyroid carcinoma). We have then validated the predictor against data with known oxidative stress related information. A key advantage in having a gene-expression data-based predictor is that RNA-seq data tend to be collected in general for cancer research; and such a predictor does not require a user to know the mutation rate distribution of the cancer type under study since such information is already encoded in the predictor for each cancer type.
Results
Genomic Mutation Profiles
We have calculated and plotted the distribution of the number of point-mutations in coding regions per genome across all cancer genomes for each of the 14 cancer types, as shown in Figure 1. From the figure, LUAD has the highest average mutation rate at 347.20 per genome, and KICH has the lowest one at 19.75 per genome. The following lists the average numbers of the remaining 12 cancer types in the descending order: 288.95 mutations in LUSC, 272.02 in COAD, 257.50 in STAD, 234.71 in BLCA, 213.26 in HNSC, 124.59 in ESCA, 147.65 in LIHC, 303.75 in BRCA, 111.14 in KIPR, 113.94 in KIRC, 138.75 in PRAD, and 134.18 in THCA.
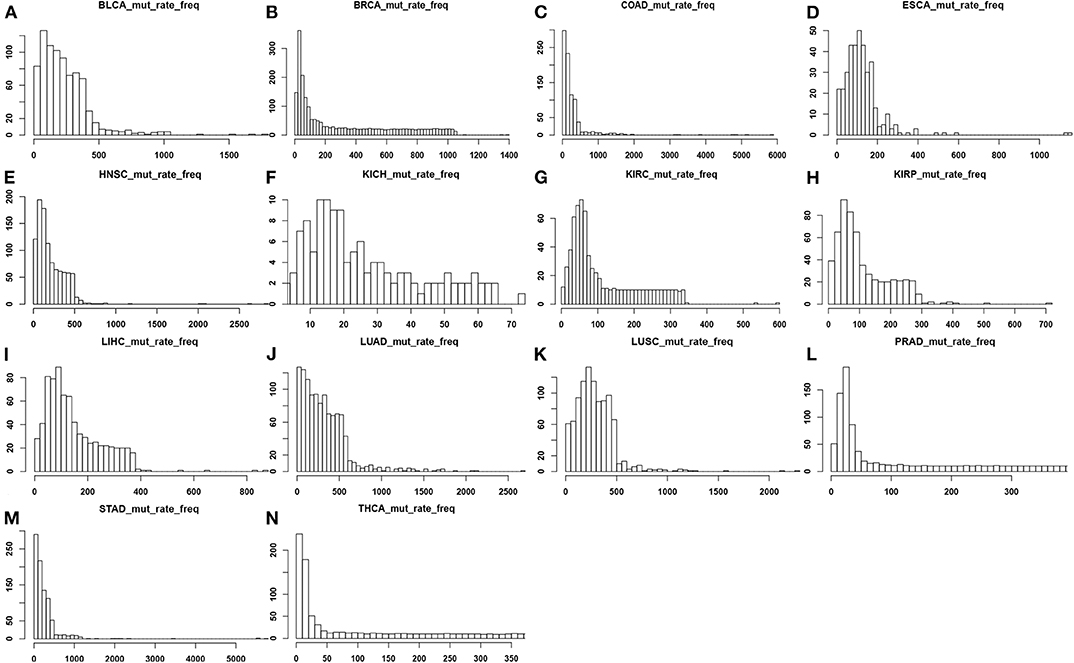
Figure 1. Distributions of the number of point mutations across all samples for each of the 14 cancer types, where the x-axis represents the mutation rate and the y-axis denotes the frequency of mutation rate across the tissue samples in each cancer type. (A) BLCA; (B) BRCA; (C) COAD; (D) ESCA; (E) HNSC; (F) KICH; (G) KIRC; (H) KIRP; (I) LIHC; (J) LUAD; (K) LUSC; (L) PRAD; (M) STAD; (N) THCA.
Selection of Antioxidant Enzyme-Encoding Genes
Some classes of enzymes have long been known to have anti-oxidative functions as their main function such as the antioxidant enzymes mentioned earlier while some others have anti-oxidation as their secondary function such as cytochromes P450 and mitogen activated protein kinases (Limón-Pacheco and Gonsebatt, 2009). And yet, increasingly more proteins have been found to have antioxidant roles in addition to their main functions such as translocases (Tang et al., 2017), hydrolase (Liu et al., 2018), and hexokinase (Heneberg, 2019).
Based on such information, we have conducted a preliminary regression analysis of the mutation rates against the expression data of all the enzyme-encoding genes in the same samples for each of the 14 cancer types (with a Lasso penalty). Interestingly, genes that give rise to good regression results across all 14 cancer types generally fall into a small set of enzyme subclasses, particularly EC 3.- (hydrolases) and EC 2.- (transferases). Hence, we have conducted a second round of regression against expression data of genes only in these two EC classes.
From genes selected for each of the 14 regression models, we note the following: (1) in all 14 cancer types, genes in each regression model fall into exactly four sub-subclasses of EC 2.- and EC 3.-; (2) in five cancer types: BLCA, KIRC, KIRP, LUAD, and LUSC, all the genes fall into four sub-subclasses of EC 3.-; in three cancer types, BRCA, ESCA and KICH, all genes fall into four sub-subclasses of EC 2.-; and in the remaining six cancer types: COAD, HNSC, LIHC, PRAD, STAD, and THCA, all genes fall into two subclasses of EC 2.- and two subclasses of EC 3.-; (3) the two most commonly used EC 3.- subclasses are EC 3.4.21.- and EC 3.1.3.- while the two mostly used EC 2.- subclasses are EC 2.4.1- and EC 2.7.1.-. Table S1 gives the gene names selected in the regression model for each cancer type.
Linear Regression Analyses
For each cancer type, a linear regression model is trained to predict the mutation rate using expression data in the same cancer sample, of selected genes from some EC subclasses as discussed above. The detailed objective function is described in the Methods section. To ensure the quality of each trained model, we have randomly selected 2/3 of the samples as the training data, and used the remaining 1/3 as the test data. Figure 2 shows the predicted values by the best trained model vs. the actual mutation rates across all samples for each cancer type. Similar plots for the test samples are given in Figure S1. Table 1 summarizes the level of contribution by genes of each EC subclass to the regression result for each cancer type, with the detailed information of the 14 models being given in Table S2.
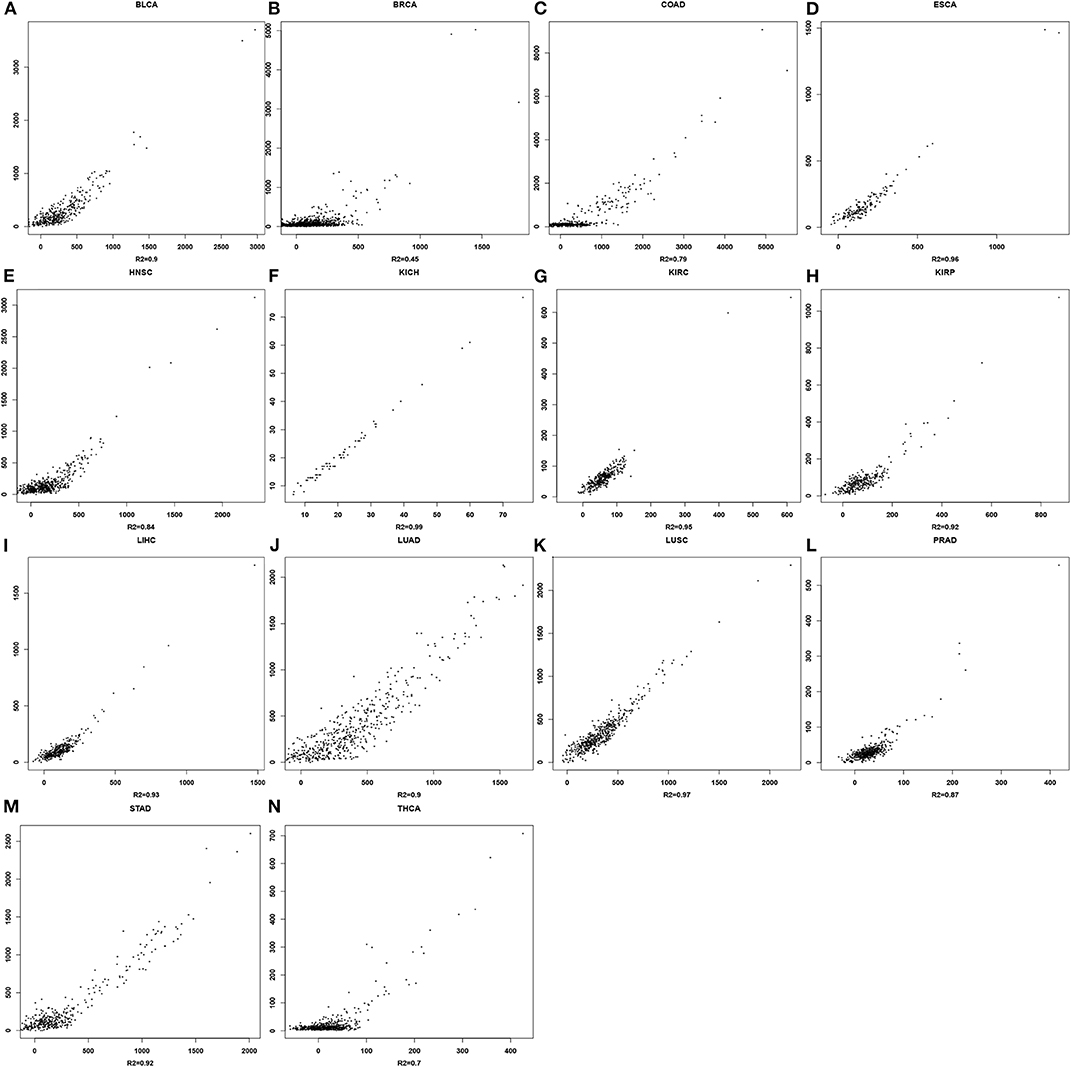
Figure 2. Scatter plots for mutation rates vs. predicted values in each of the 14 cancer types. For each panel, the x-axis represents the predicted mutation rates and the y-axis denotes the actual mutation rates. (A) BLCA; (B) BRCA; (C) COAD; (D) ESCA; (E) HNSC; (F) KICH; (G) KIRC; (H) KIRP; (I) LIHC; (J) LUAD; (K) LUSC; (L) PRAD; (M) STAD; (N) THCA.
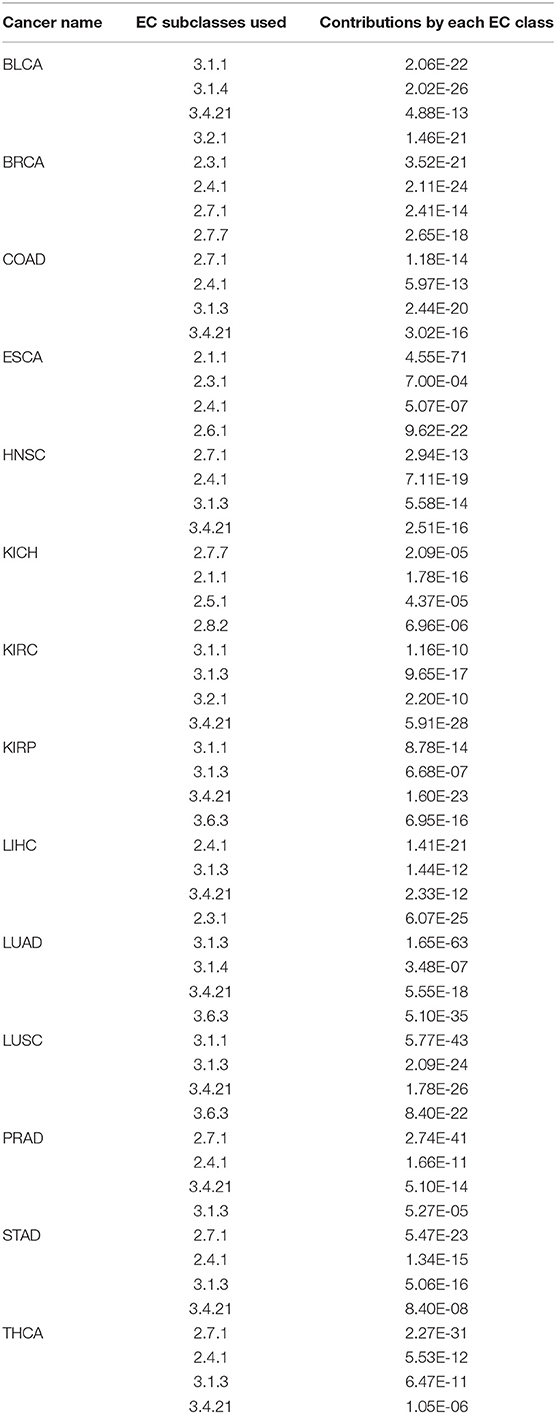
Table 1. Estimated contribution by genes in each EC subclass to the linear model for each of the 14 cancer types.
Prediction Validation
We have conducted the following analyses to provide supporting data to our prediction model.
Validation Against Fatty Acid Synthesis Genes
It is well-known that fatty acids serve as antioxidants in cancer and other disease cells (Richard et al., 2008; Freitas et al., 2017). Hence, we anticipate that our predictor should have some level of correlation with the fatty acid synthesis process. Table 2 shows the best correlation coefficient between our predictor and one of the four fatty acid synthesis genes (see Methods) across the 14 cancer types, with Table 3 giving the statistical significance of the observed correlations.
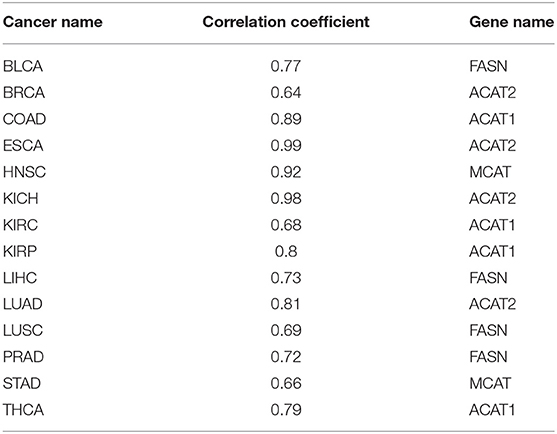
Table 2. Correlation coefficients between our oxidative-stress predictor and the fatty acid synthesis gene across 14 cancer types.
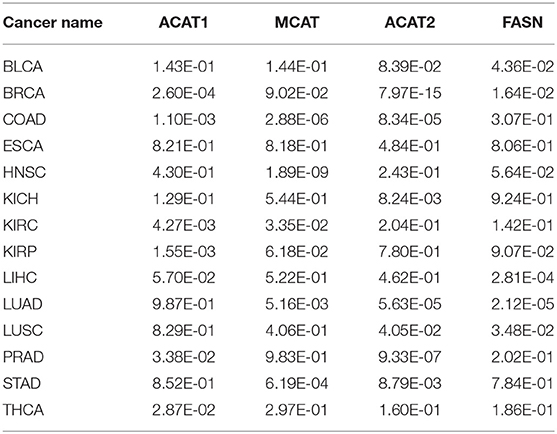
Table 3. Statistical significance for the observed correlation coefficient in Table 2 across 14 cancer types.
Validation Against Mucin Genes
Mucins have been found to have elevated levels across numerous cancer types and are known to have anti-oxidation roles (Takeyama et al., 2000; Wu et al., 2009; Dewald et al., 2016). We have examined their expression levels and our predictor. Table 4 shows the best correlation coefficient between our predictor and one of the mucin genes across the 14 cancer types, with Table 5 giving the statistical significance of the observed correlations. And the statistical significance of the observed correlations for each Mucin genes is given in Table S3 in detail.
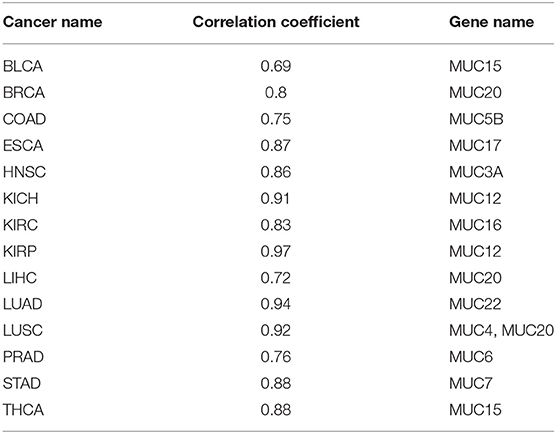
Table 4. Correlation coefficients between our oxidative-stress predictor and mucin genes across 14 cancer types.
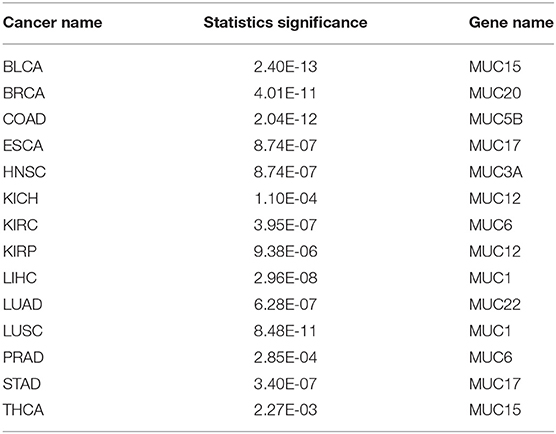
Table 5. Statistical significance for the observed correlation coefficients in Table 4 across 14 cancer types.
Validation Against Glutathione Synthesis Genes
Glutathione is a molecule human cells use as a key antioxidant. We have examined their expression levels and our predictor. Table 6 shows the R2 values between our predictor and the glutathione synthesis genes across the 14 cancer types, with Table 7 giving the statistical significance of the observed correlations.
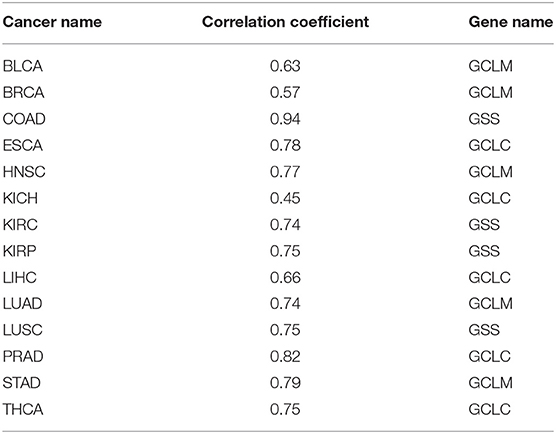
Table 6. Correlation coefficients between our predictor and the glutathione synthesis genes across 14 cancer types.
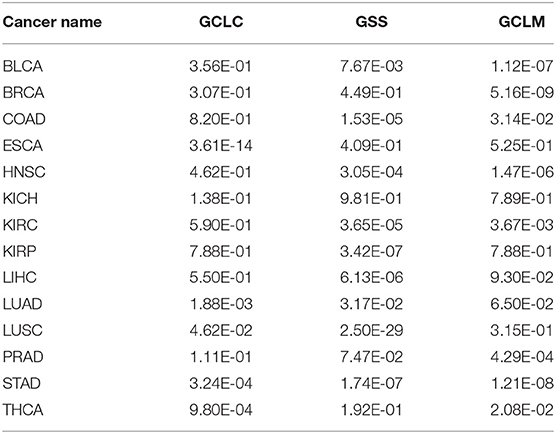
Table 7. Statistical significance for observed correlation coefficient in Table 6 across 14 cancer types.
It is noteworthy that our predictor is designed to predict the level of oxidative stress; and each of the above three groups of genes is known to be associated with the level of oxidative stress and not involved in the training dataset. The observed strong correlations between our predicted oxidative stress levels and the expression levels of each such group, along with significant p-values, provide strong support for that our predictor captures the anti-oxidative level from different independent aspects, hence indicating the validity of our trained predictor as an indicator for the level of oxidative stress.
Application
To demonstrate the utility of our predictor, we have calculated the average oxidative stress levels for each of the four stages vs. the matching controls in each of the 14 cancer types, as detailed in Figure 3. From the figure, we can see: (1) cancer tissue cells have elevated oxidative-stress levels than matching controls in all of 14 cancer types; and (2) the oxidative stress level tends to progressively increase as a cancer advances from stage 1 through stage 4, in 10 out of 14 cancer types with at most one predicted level of oxidative stress being out of order. This is clearly consistent with our general understanding about cancer progression.
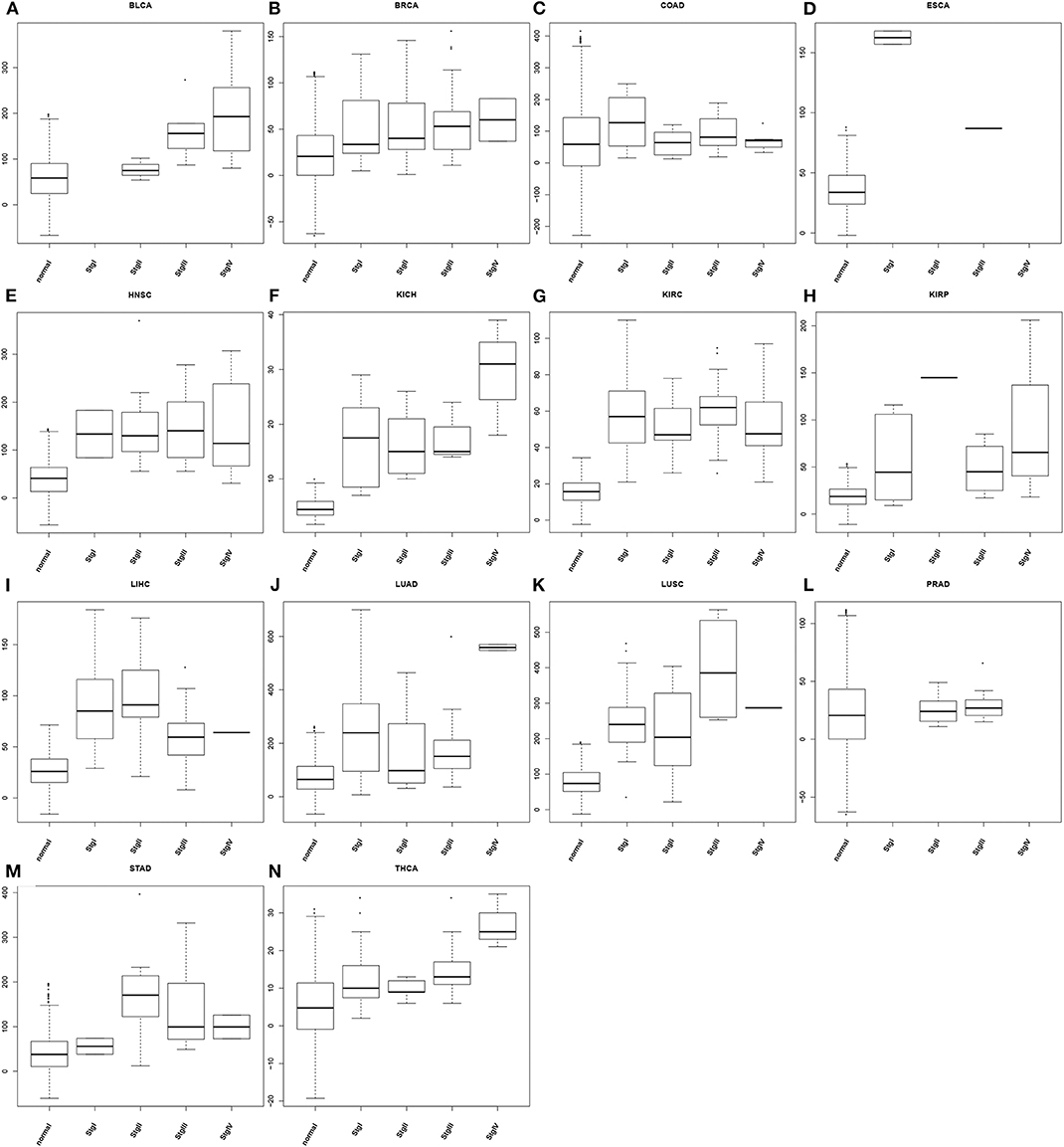
Figure 3. Boxplots of predicted oxidative stress levels across 4 cancer stages (when data are available) along with matching controls for each of the 14 cancer types. (A) BLCA; (B) BRCA; (C) COAD; (D) ESCA; (E) HNSC; (F) KICH; (G) KIRC; (H) KIRP; (I) LIHC; (J) LUAD; (K) LUSC; (L) PRAD; (M) STAD; (N) THCA.
We have also conducted co-expression analyses to find genes that strongly correlate with our predictor in each cancer type and analyzed the pathways enriched by such genes. Table S4 gives the up-to top 100 enriched pathways with p < 0.05 in each cancer. We note that the enriched pathways are highly consistent across different cancer types. Furthermore, we note based on extensive literature search that a majority of the enriched pathways, marked in bold letters, is: (1) involved in anti-oxidation activities, (2) induced by oxidative stress, (3) induces oxidative stress, (4) involved in reactive oxidative stress signaling, and/or (5) is altered by oxidative stress. While these data provide supporting data to our predictor, pathways not known to be oxidative stress related may provide useful candidates for elucidation of the overall oxidative-stress responding mechanisms in cancer cells.
Discussion
Quantitative assessment of the intracellular oxidative stress will prove to be an invaluable tool for elucidation of the possible causes of various changes in cancer cells, including extensive metabolic reprogramming. However, the problem proves to be very challenging because there are large numbers of contributors to both the total oxidizing power and the anti-oxidizing capacity in human cells. Previous studies tend to focus on oxidative stress induced by specific molecular species such as H2O2 or lipid radicals rather than the overall oxidative stress level. To the best our knowledge, our work is the first computational tool for estimating the oxidative stress level. The basic premise of our tool is that the genomic mutation level is strongly associated with the overall intracellular oxidative stress level. The second premise is that many enzymes have anti-oxidation capacity as reported by numerous authors (Sies, 1997; Rajput et al., 2013).
One surprising result in our regression analyses is that genes in two EC classes, EC 2.- (transferases) and EC 3.- (hydrolases), specifically four subclasses of these two, can be used to well interpret the mutation rate in each cancer sample by a linear combination of their expressions with high statistical significance. This strongly suggests that all the enzymes encoded by these genes play roles in cellular anti-oxidation, which is clearly a novel discovery and warrants further investigation. Furthermore, different cancer types tend to use a distinct combination of genes from four subclasses of enzymes strongly suggest that these cancer types may encounter different types of oxidative stress, hence using different combinations of anti-oxidation enzymes. This also warrants further study regarding why genes in different enzyme classes show strong correlations with mutation rates in different cancer types, therefore to understand the detailed mechanisms of their anti-oxidative functions possibly for different types of oxidants.
As mentioned in the Introduction, oxidative stress may arise from different molecular species such reactive oxygen species, reactive nitrogen species, reactive lipid species and various free radicals. Different molecular species might be utilized to consume specific types of oxidizing molecules. By showing that three independent classes of molecules all have statistically significant correlation coefficients with our general-purpose predictor for oxidative stress, we clearly have strong support for the validity of our predictor.
The availability of this new tool make open new doors for studying impact of oxidative stress on various chronic inflamed diseases, including cancer, including (i) elucidation of all metabolic processes, particularly reprogrammed metabolisms observed in cancer and other diseases, that are statistically associated with the level of oxidative stress, hence providing a new capability for detection of the possible causes of various altered metabolisms, and (ii) systematic analyses of different classes of enzymes in terms of their anti-oxidative roles, which could provide potentially powerful and new targets for treating various chronic diseases, including cancer.
We anticipate that our predictor will prove a powerful tool useful for elucidation of causes of variety of systematic changes, including metabolic reprogramming to gain in-depth understanding of why specific metabolic pathways are reprogrammed and certain cellular functions tend to be repressed or hyper-activated in cancer.
Data and Methods
Gene-Expression Data and Genomic Mutation Data
Gene expression and genomic mutation data of 14 cancer types: BLCA, BRCA, COAD, ESCA, HNSC, KICH, KIRC, KIRP, LIHC, LUAD, LUSC, PRAD, STAD, and THCA were downloaded from the TCGA data portal1. The detailed information of these data is summarized in Table 8.
Genomic mutation data were derived from the whole-exome sequencing data. Specifically, somatic changes are identified through comparing allele frequencies in the aligned DNA sequences of each cancer and the matching normal samples, using the GDC DNA-Seq analysis pipeline (GDC DNA-Seq analysis pipeline: https://docs.gdc.cancer.gov/Data/Bioinformatics_Pipelines/DNA_Seq_Variant_Calling_Pipeline). MuTect2 (GDC MuTect2: https://docs.gdc.cancer.gov/Data/Bioinformatics_Pipelines/DNA_Seq_Variant_Calling_Pipeline/#somatic-variant-calling-workflow) is used to call each mutation using the default parameters.
Human enzyme information, including gene names and the relevant enzyme classes, is downloaded from BRENDA (BRENDA links: https://www.brenda-enzymes.org/).
Synthesis of Fatty Acids
We have used the four fatty-acid synthesis genes: FASN (Fatty Acid Synthase), ACAT1 (Acetyl-CoA Acetyltransferase 1), ACAT2 (Acetyl-CoA Acetyltransferase 2), and MCAT (Malonyl-CoA-Acyl Carrier Protein Transacylase) to reflect the level of fatty acid synthesis, and have calculated the Pearson correlation coefficient between the expressions of one of them and our predictor.
Mucin Genes
Human has 20 mucin genes, namely MUC1, MUC2, MUC8, MUC12, MUC13, MUC15, MUC16, MUC17, MUC19, MUC20, MUC21, MUC22, MUC3A, MUC3B, MUC4, MUC5AC, MUC5B, MUC6, MUC7, and MUCL1. To assess their overall correlation with our predictor, we have conducted the Pearson correlation coefficient between the expression of each mucin gene and our oxidative stress predictor.
Glutathione Synthesis
We have used the three biosynthesis genes: GCLC (glutamate-cysteine ligase catalytic subunit), GCLM (glutamate-cysteine ligase modifier subunit) and GSS (glutathione synthase) to reflect the level of glutathione synthesis. As above, we have calculated the Pearson correlation coefficient between the expressions of the glutathione synthesis genes and our oxidative stress predictor.
Differential Expression Analyses
We have used edgeR in the R package (edgeR package: https://www.r-project.org/) to determine if a gene is differentially expressed in cancer vs. control samples of the same cancer type. T-test is applied to estimate the statistical significance of each gene considered as differentially expressed, using 0.05 as the cut-off.
Linear Regression Analysis
We have conducted a linear regression analysis of the observed mutation rates, Yn, in n samples of a specified cancer type against the expressions of m selected genes across n samples, Xn, m, so that residual ||ε|| is as small as possible as defined below:
where Bm is a coefficient vector with its m values to be determined through solving this optimization problem. To avoid using too many genes in the regression analysis, we have included a penalty for penalizing using more variables than necessary.
where λ is an (adjustable) constant. This problem can be solved using a least squared regression analyses in the following form:
For each Y, we have retrieved the number of point mutations per cancer genome. Then for the n cancer genomes of each of the 14 cancer types, we have n numbers, namely the mutation number divided by the number of genes (20,000), which gives rise to the Y. For the ith row of X, we have retrieved the expression data of m selected genes in the ith sample of the same cancer type. For each regression analysis, we have used the R package to solve the minimization problem. The regression result has a p-value associated with each of the m values of B. We then remove those genes with insignificant p-values, i.e., > 0.05, for the second round of regression analyses mentioned in the Results section.
Correlation Analyses
We have used Pearson correlation coefficient to calculate the linear correlation between two lists of numbers, with one being the combined gene-expression data and the other being mutation rates of the same samples.
Pathway Enrichment Analysis
We have conducted a pathway enrichment analysis over a given set of genes found to be strongly correlated with our predictor for oxidative stress using DAVID tool (David links: https://david.ncifcrf.gov/) against the combined database of GO/Biological Process, KEGG and Reactome pathways. A pathway is considered enriched if tits adjusted p < 0.05.
Assessment of the Level of Contribution by Each Enzyme Subclass in Regression Model
For each derived regression model against selected enzymes, the following is used to estimate the level of contribution by each subclass of enzymes. The package used for linear repression construction provides a p-value for each selected gene, indicating the level of the gene's contribution to the regression result with smaller p-value representing higher level of contribution. We then use the product of the p-values of the selected genes from the same enzyme subclass as the p-value of the subclass.
Software Availability
Our predictor is written in R language and is available upon request.
Data Availability Statement
BLCA, BRCA, COAD, ESCA, HNSC, KICH, KIRC, KIRP, LIHC, LUAD, LUSC, PRAD, STAD, and THCA were downloaded from the TCGA data portal (TCGA data portal: https://portal.gdc.cancer.gov/). Human enzyme information, including gene names and the relevant enzyme classes, is downloaded from BRENDA (BRENDA links: https://www.brenda-enzymes.org/).
Author Contributions
LL designed the method, carried out computational analyses, analyzed computational results, and wrote the article. HC supported in data analysis. YX conceived the project, reviewed computational analyses, and revised the article.
Conflict of Interest
The authors declare that the research was conducted in the absence of any commercial or financial relationships that could be construed as a potential conflict of interest.
Acknowledgments
LL wants to extend her thanks to members of the Computational Systems Biology Lab (CSBL) in the University of Georgia, for their helpful discussions related to the study here.
Supplementary Material
The Supplementary Material for this article can be found online at: https://www.frontiersin.org/articles/10.3389/fgene.2020.00494/full#supplementary-material
Footnote
1. ^TCGA data portal: https://portal.gdc.cancer.gov/
References
Alfaradhi, M. Z., Fernandez-Twinn, D. S., Martin-Gronert, M. S., Musial, B., Fowden, A., and Ozanne, S. E. (2014). Oxidative stress and altered lipid homeostasis in the programming of offspring fatty liver by maternal obesity. Am. J. Physiol. Regul. Integr. Comp. Physiol. 307, R26–R34. doi: 10.1152/ajpregu.00049.2014
Barash, H. R., Gross, E., Edrei, Y., Ella, E., Israel, A., Cohen, I., et al. (2010). Accelerated carcinogenesis following liver regeneration is associated with chronic inflammation-induced double-strand DNA breaks. Proc Natl Acad Sci USA. 107, 2207–2212. doi: 10.1073/pnas.0908867107
Bellezza, I., Mierla, A. L., and Minelli, A. (2010). Nrf2 and NF-κB and their concerted modulation in cancer pathogenesis and progression. Cancers 2, 483–497. doi: 10.3390/cancers2020483
Campbell, K., Vowinckel, J., Keller, M. A., and Ralser, M. (2016). Methionine metabolism alters oxidative stress resistance via the pentose phosphate pathway. Antioxid. Redox Signal. 24, 543–547. doi: 10.1089/ars.2015.6516
Cerutti, P. A., and Trump, B. F. (1993). Inflammation and oxidative stress in carcinogenesis. Cancer Cells 3, 1–7. doi: 10.1007/978-1-4615-3520-1_75
Chan, H. P., Lewis, C., and Thomas, P. S. (2010). Oxidative stress and exhaled breath analysis: a promising tool for detection of lung cancer. Cancers 2, 32–42. doi: 10.3390/cancers2010032
Chang, D., Wang, F., Zhao, Y. S., and Pan, H. Z. (2008). Evaluation of oxidative stress in colorectal cancer patients. Biomed. Environ. Sci. 21, 286–289. doi: 10.1016/S0895-3988(08)60043-4
Chung, Y. J., Robert, C., Gough, S. M., Rassool, F. V., and Aplan, P. D. (2014). Oxidative stress leads to increased mutation frequency in a murine model of myelodysplastic syndrome. Leuk. Res. 38, 95–102. doi: 10.1016/j.leukres.2013.07.008
Conti, A., Gulì, C., La Torre, D., Tomasello, C., Angileri, F. F, and M'Hammed, A. (2010). Role of inflammation and oxidative stress mediators in gliomas. Cancers 2, 693–712. doi: 10.3390/cancers2020693
Dalle-Donne, I., Rossi, R., Giustarini, D., Milzani, A., and Colombo, R. (2003). Protein carbonyl groups as biomarkers of oxidative stress. Clin. Chim. Acta 329, 23–38. doi: 10.1016/S0009-8981(03)00003-2
Dayem, A. A., Choi, H. Y., Kim, J. H., and Cho, S. G. (2010). Role of oxidative stress in stem, cancer, and cancer stem cells. Cancers 2, 859–884. doi: 10.3390/cancers2020859
Dewald, J. H., Colomb, F., Bobowski-Gerard, M., Groux-Degroote, S., and Delannoy, P. (2016). Role of cytokine-induced glycosylation changes in regulating cell interactions and cell signaling in inflammatory diseases and cancer. Cells 5:43. doi: 10.3390/cells5040043
Doudican, N. A., Song, B., Shadel, G. S., and Doetsch, P. W. (2005). Oxidative DNA damage causes mitochondrial genomic instability in Saccharomyces cerevisiae. Mol. Cell. Biol. 25, 5196–5204. doi: 10.1128/MCB.25.12.5196-5204.2005
Farah, I. O. (2005). Assessment of cellular responses to oxidative stress using MCF-7 breast cancer cells. black seed (N. Sativa L.) extracts and H2O2. Int. J. Environ. Res. Public Health. 2, 411–419. doi: 10.3390/ijerph2005030005
Fernando, N., Wickremesinghe, S., Niloofa, R., Rodrigo, C., Karunanayake, L., de Silva, H. J., et al. (2016). Protein carbonyl as a biomarker of oxidative stress in severe leptospirosis, and its usefulness in differentiating leptospirosis from dengue infections. PLoS ONE 11:e0156085. doi: 10.1371/journal.pone.0156085
Fionda, C., Abruzzese, M. P., Santoni, A., and Cippitelli, M. (2016). Immunoregulatory and effector activities of nitric oxide and reactive nitrogen species in cancer. Curr. Med. Chem. 23, 2618–2636. doi: 10.2174/0929867323666160727105101
Fitzgerald, D. M., Hastings, P. J., and Rosenberg, S. M. (2017). Stress-induced mutagenesis: implications in cancer and drug resistance. Ann. Rev. Cancer Biol. 1, 119–140. doi: 10.1146/annurev-cancerbio-050216-121919
Freitas, H. R., Ferreira, G. D. C., Trevenzoli, I. H., Oliveira, K. J., and de Melo Reis, R. A. (2017). Fatty acids, antioxidants and physical activity in brain aging. Nutrients 9:1263. doi: 10.3390/nu9111263
Gibellini, L., Pinti, M., Nasi, M., De Biasi, S., Roat, E., Bertoncelli, L., et al. (2010). Interfering with ROS metabolism in cancer cells: the potential role of quercetin. Cancers 2, 1288–1311. doi: 10.3390/cancers2021288
Giustarini, D., Dalle-Donne, I., Lorenzini, S., Selvi, E., Colombo, G., Milzani, A., et al. (2012). Protein thiolation index (PTI) as a biomarker of oxidative stress. Free Radic. Biol. Med. 53, 907–915. doi: 10.1016/j.freeradbiomed.2012.06.022
Giustarini, D., Galvagni, F., Colombo, G., Dalle-Donne, I., Milzani, A., Aloisi, A. M., et al. (2017). Determination of protein thiolation index (PTI) as a biomarker of oxidative stress in human serum. Anal. Biochem. 538, 38–41. doi: 10.1016/j.ab.2017.09.010
Gorrini, C., Harris, I. S., and Mak, T. W. (2013). Modulation of oxidative stress as an anticancer strategy. Nat. Rev. Drug Discov. 12, 931–947. doi: 10.1038/nrd4002
Graham, S. H. (2016). Modification of ubiquitin C-terminal hydrolase L1 by reactive lipid species: role in neural regeneration and diseases of aging. Neural Regen. Res. 11, 908–909. doi: 10.4103/1673-5374.184482
Hacişevki, A., Baba, B., Gonenc, A., and Aslan, S. (2012). Protein carbonyl content as the most general and well-used biomarker of severe oxidative stress. Oxid. Commun. 35, 413–422. Available online at: https://www.researchgate.net/publication/281595280_Protein_carbonyl_content_as_the_most_general_and_well-used_biomarker_of_severe_oxidative_stress
Halder, S. R., and Bhattacharyya, M. (2014). Oxidative stress: lipid peroxidation products as predictors in disease progression. J. Exp. Integr. Med. 4, 151–164. doi: 10.5455/jeim.210414.rw.007
Heneberg, P. (2019). Redox regulation of hexokinases. Antioxid. Redox Signal. 30, 415–442. doi: 10.1089/ars.2017.7255
Henkler, F., Brinkmann, J., and Luch, A. (2010). The role of oxidative stress in carcinogenesis induced by metals and xenobiotics. Cancers 2, 376–396. doi: 10.3390/cancers2020376
Higdon, A., Diers, A. R., Oh, J. Y., Landar, A., and Darley-Usmar, V. M. (2012). Cell signalling by reactive lipid species: new concepts and molecular mechanisms. Biochem. J. 442, 453–464. doi: 10.1042/BJ20111752
Itabe, H. (2012). Oxidized low-density lipoprotein as a biomarker of in vivo oxidative stress: from atherosclerosis to periodontitis. J. Clin. Biochem. Nutr. 51, 1–8. doi: 10.3164/jcbn.11-00020R1
Karlenius, T. C., and Tonissen, K. F. (2010). Thioredoxin and cancer: a role for thioredoxin in all states of tumor oxygenation. Cancers 2, 209–232. doi: 10.3390/cancers2020209
Kato, S., Karino, K., Hasegawa, S., Nagasawa, J., Nagasaki, A., Eguchi, M., et al. (1995). Octacosanol affects lipid metabolism in rats fed on a high-fat diet. Br. J. Nutr. 73, 433–441. doi: 10.1079/BJN19950045
Kong, X., Wang, G., and Li, S. (2007). Antioxidation and ATPase activity in the gill of mud crabScylla serrataunder cold stress. Chin. J. Oceanol. Limnol. 25, 221–226. doi: 10.1007/s00343-007-0221-7
Kruk, J., and Aboul-Enein, H. Y. (2017). Reactive oxygen and nitrogen species in carcinogenesis: implications of oxidative stress on the progression and development of severalcancer types. Mini Rev. Med. Chem. 17, 904–919. doi: 10.2174/1389557517666170228115324
Leone, A., Roca, M. S., Ciardiello, C., Costantini, S., and Budillon, A. (2017). Oxidative stress gene expression profile correlates with cancer patient poor prognosis: identification of crucial pathways might select novel therapeutic approaches. Oxid. Med. Cell. Longev. 2017, 1–18. doi: 10.1155/2017/2597581
Limoli, C. L., and Giedzinski, E. (2003). Induction of chromosomal instability by chronic oxidative stress. Neoplasia 5, 339–346. doi: 10.1016/S1476-5586(03)80027-1
Limón-Pacheco, J., and Gonsebatt, M. E. (2009). The role of antioxidants and antioxidant-related enzymes in protective responses to environmentally induced oxidative stress. Mutat. Res. Genet. Toxicol. Environ. Mutagen. 674, 137–147. doi: 10.1016/j.mrgentox.2008.09.015
Liu, L. P., Li, B., Shuai, T. K., Zhu, L., and Li, Y.-M. (2018). Deletion of soluble epoxide hydrolase attenuates mice Hyperoxic acute lung injury. BMC Anesthesiol. 18,1–7. doi: 10.1186/s12871-018-0490-z
Mantovani, G., Macciò, A., Madeddu, C., Mura, L., Gramignano, G., Lusso, M. R., et al. (2010). Quantitative evaluation of oxidative stress, chronic inflammatory indices and leptin in cancer patients: correlation with stage and performance status. Int. J. Cancer 98, 84–91. doi: 10.1002/ijc.10143
Markkanen, E. (2017). Not breathing is not an option: how to deal with oxidative DNA damage. DNA Repair 59, 82–105. doi: 10.1016/j.dnarep.2017.09.007
Martínez, Y., Li, X., Liu, G., Bin, P., Yan, W., Más, D., et al. (2017). The role of methionine on metabolism, oxidative stress, and diseases. Amino Acids 49, 1–8. doi: 10.1007/s00726-017-2494-2
Massi, P., Valenti, M., Solinas, M., and Parolaro, D. (2010). Molecular mechanisms involved in the antitumor activity of cannabinoids on gliomas: role for oxidative stress. Cancers 2, 1013–1026. doi: 10.3390/cancers2021013
Niki, E. (2008). Lipid peroxidation products as oxidative stress biomarkers. Biofactors 34, 171–180. doi: 10.1002/biof.5520340208
Ortega, A. L., Mena, S., and Estrela, J. M. (2010). Oxidative and nitrosative stress in the metastatic microenvironment. Cancers 2, 274–304. doi: 10.3390/cancers2020274
Osman, M. Y., Rahman, T., Ismail, T. S., Azlina, A. R., and Nawawi, H. (2016). Investigation of oxidative stress status in metabolic syndrome patients using lipid peroxidation biomarkers. Int. Arch. Med. 9, 1–10. doi: 10.3823/1879
Osmundsen, H., Cervenka, J., and Bremer, J. (1982). A role for 2,4-enoyl-CoA reductase in mitochondrial beta-oxidation of polyunsaturated fatty acids. Effects of treatment with clofibrate on oxidation of polyunsaturated acylcarnitines by isolated rat liver mitochondria. Biochem. J. 208, 749–757. doi: 10.1042/bj2080749
Pizzimenti, S., Toaldo, C., Pettazzoni, P., Dianzani, M. U., and Barrera, G. (2010). Effects of reactive oxygen species and the lipid peroxidation product 4-hydroxynonenal in the hallmarks of cancer. Cancers 2, 338–363. doi: 10.3390/cancers2020338
Rajput, I. R., Li, Y. L., Xu, X., Huang, Y., Zhi, W. C., Yu, D. Y., et al. (2013). Supplementary effects of Saccharomyces boulardii and Bacillus subtilis B10 on digestive enzyme activities, antioxidation capacity and blood homeostasis in broiler. Int. J. Agric. Biol. 15, 1560–8530. Available online at: https://www.researchgate.net/publication/236872023_Supplementary_Effects_of_Saccharomyces_boulardii_and_Bacillus_subtilis_B10_on_Digestive_Enzyme_Activities_Antioxidation_Capacity_and_Blood_Homeostasis_in_Broiler
Rao, C. V., Asch, A. S., and Yamada, H. Y. (2017). Frequently mutated genes/pathways and genomic instability as prevention targets in liver cancer. Carcinogenesis 38, 2–11. doi: 10.1093/carcin/bgw118
Reczek, C. R., and Chandel, N. S. (2017). The two faces of reactive oxygen species in cancer. Ann. Rev. Cancer Biol. 1, 79–98. doi: 10.1146/annurev-cancerbio-041916-065808
Reuter, S., Gupta, S. C., Chaturvedi, M. M., and Aggarwal, B. B. (2010). Oxidative stress, inflammation, and cancer: how are they linked? Free Radic. Biol. Med. 49, 1603–1616. doi: 10.1016/j.freeradbiomed.2010.09.006
Richard, D., Kefi, K., Barbe, U., Bausero, P., and Visioli, F. (2008). Polyunsaturated fatty acids as antioxidants. Pharmacol. Res. 57, 451–455. doi: 10.1016/j.phrs.2008.05.002
Santos, C. R., and Schulze, A. (2012). Lipid metabolism in cancer. FEBS J. 279, 2610–2623. doi: 10.1111/j.1742-4658.2012.08644.x
Schultz, M. A., Abdel-Mageed, A. B., and Mondal, D. (2010). The Nrf1 and Nrf2 balance in oxidative stress regulation and androgen signaling in prostate cancer cells. Cancers 2, 1354–1378. doi: 10.3390/cancers2021354
Schulz, J. B., Lindenau, J., Seyfried, J., and Dichgans, J. (2000). Glutathione, oxidative stress and neurodegeneration. Eur. J. Biochem. 267, 4904–4911. doi: 10.1046/j.1432-1327.2000.01595.x
Selvaraj, N., Bobby, Z., Das, A. K., Ramesh, R., and Koner, B. C. (2002). An evaluation of level of oxidative stress and protein glycation in nondiabetic Undialyzed chronic renal failure patients. Clin. Chim. Acta 324, 45–50. doi: 10.1016/S0009-8981(02)00211-5
Sies, H. (1997). Oxidative stress: oxidants and antioxidants. Exp. Physiol. Transl. Integr. 82, 291–295. doi: 10.1113/expphysiol.1997.sp004024
Slane, B. G., Aykin-Burns, N., Smith, B. J., Kalen, A. L., Goswami, P. C., Domann, F. E., et al. (2006). Mutation of succinate dehydrogenase subunit C results in increased O2·-oxidative stress, and genomic instability. Cancer Res. 66, 7615–7620. doi: 10.1158/0008-5472.CAN-06-0833
Soory, M. Oxidative Stress Induced Mechanisms in the Progression of Periodontal Diseases and Cancer: A Common Approach to Redox Homeostasis? Cancers. (2010). 2, 670–692. doi: 10.3390/cancers2020670
Sosa, V., Moliné, T., Somoza, R., Paciucci, R., Kondoh, H., and LLeonart, M. E. (2013). Oxidative stress and cancer: an overview. Ageing Res. Rev. 12, 376–390. doi: 10.1016/j.arr.2012.10.004
Sun, W. G., Weydert, C. J., Zhang, Y., Yu, L., Liu, J., Spitz, D. R., et al. (2010). Superoxide enhances the antitumor combination of AdMnSOD plus BCNU in breast cancer. Cancers 2, 68–87. doi: 10.3390/cancers2010068
Takeyama, K., Dabbagh, K., Jeong Shim, J., Dao-Pick, T., Ueki, I. F., and Nadel, J. A. (2000). Oxidative stress causes mucin synthesis via transactivation of epidermal growth factor receptor: role of neutrophils. J. Immunol. 164, 1546–1552. doi: 10.4049/jimmunol.164.3.1546
Tang, K., Zhao, Y., Li, H., Zhu, M., Li, W., Liu, W., et al. (2017). Translocase of inner membrane 50 functions as a novel protective regulator of pathological cardiac hypertrophy. J. Am. Heart Assoc. 6:e004346. doi: 10.1161/JAHA.116.004346
Teppner, M., Böss, F., Ernst, B., and Pähler, A. (2015). Application of lipid peroxidation products as biomarkers for flutamide-induced oxidative stress in vitro. Toxicol. Lett. 238, 53–59. doi: 10.1016/j.toxlet.2015.08.005
Tubbs, A., and Nussenzweig, A. (2017). Endogenous DNA damage as a source of genomic instability in cancer. Cell 168, 644–656. doi: 10.1016/j.cell.2017.01.002
Valle, A., Oliver, J., and Roca, P. (2010). Role of uncoupling proteins in cancer. Cancers 2, 567–591. doi: 10.3390/cancers2020567
Wangpaichitr, M., Wu, C., You, M., Maher, J. C., Dinh, V., Feun, L. G., et al. (2009). N′1, N′3-dimethyl-N′1, N′3-bis (phenylcarbonothioyl) propanedihydrazide (Elesclomol) selectively kills cisplatin resistant lung cancer cells through reactive oxygen species (ROS). Cancers 1, 23–38. doi: 10.3390/cancers1010023
Wieczorek, E., Jablonowski, Z., Tomasik, B., Gromadzinska, J., Jablonska, E., Konecki, T., et al. (2017). Different gene expression and activity pattern of antioxidant enzymes in bladder cancer. Anticancer Res. 37, 841–848. doi: 10.21873/anticanres.11387
Wu, Y. M., Nowack, D. D., Omenn, G. S., and Haab, B. B. (2009). Mucin glycosylation is altered by pro-inflammatory signaling in pancreatic-cancer cells. J. Proteome Res. 8, 1876–1886. doi: 10.1021/pr8008379
Xu, B., Wang, W., Guo, H., Sun, Z., Wei, Z., Zhang, X., et al. (2014). Oxidative stress preferentially induces a subtype of micronuclei and mediates the genomic instability caused by p53 dysfunction. Mutat. Res. 770, 1–8. doi: 10.1016/j.mrfmmm.2014.08.004
Zhang, F., and Du, G. (2012). Dysregulated lipid metabolism in cancer. World J. Biol. Chem. 3, 167–174. doi: 10.4331/wjbc.v3.i8.167
Zhang, H., Lei, Y., Yuan, P, Li, L., Luo, C., Gao, R., et al. (2014). ROS-mediated autophagy induced by dysregulation of lipid metabolism plays a protective role in colorectal cancer cells treated with gambogic acid. PLoS ONE 9:e96418. doi: 10.1371/journal.pone.0096418
Keywords: oxidative stress, genomic mutation, transcriptomic data, cancer, TCGA data analysis, computational prediction
Citation: Liu L, Cui H and Xu Y (2020) Quantitative Estimation of Oxidative Stress in Cancer Tissue Cells Through Gene Expression Data Analyses. Front. Genet. 11:494. doi: 10.3389/fgene.2020.00494
Received: 24 February 2020; Accepted: 20 April 2020;
Published: 19 May 2020.
Edited by:
Yi Zhao, Beijing University of Chinese Medicine, ChinaReviewed by:
Jie Sun, Harbin Medical University, ChinaFei He, University of Missouri, United States
Meng Zhou, Wenzhou Medical University, China
Copyright © 2020 Liu, Cui and Xu. This is an open-access article distributed under the terms of the Creative Commons Attribution License (CC BY). The use, distribution or reproduction in other forums is permitted, provided the original author(s) and the copyright owner(s) are credited and that the original publication in this journal is cited, in accordance with accepted academic practice. No use, distribution or reproduction is permitted which does not comply with these terms.
*Correspondence: Haining Cui, cuihn@jlu.edu.cn; Ying Xu, xyn@uga.edu