- 1Department of Soil, Plant and Food Science, Section of Genetics and Plant Breeding, University of Bari Aldo Moro, Bari, Italy
- 2Institute of Biomedical Technologies, National Research Council (CNR), Bari, Italy
- 3Department of Agricultural, Food and Environmental Sciences, University of Foggia, Foggia, Italy
- 4Department of Biosciences, Biotechnologies and Biopharmaceutics, University of Bari Aldo Moro, Bari, Italy
- 5Department of Agricultural Sciences, University of Naples Federico II, Naples, Italy
High-throughput genotyping boosts genome-wide association studies (GWAS) in crop species, leading to the identification of single-nucleotide polymorphisms (SNPs) associated with economically important traits. Choosing a cost-effective genotyping method for crop GWAS requires careful examination of several aspects, namely, the purpose and the scale of the study, crop-specific genomic features, and technical and economic matters associated with each genotyping option. Once genotypic data have been obtained, quality control (QC) procedures must be applied to avoid bias and false signals in genotype–phenotype association tests. QC for human GWAS has been extensively reviewed; however, QC for crop GWAS may require different actions, depending on the GWAS population type. Here, we review most popular genotyping methods based on next-generation sequencing (NGS) and array hybridization, and report observations that should guide the investigator in the choice of the genotyping method for crop GWAS. We provide recommendations to perform QC in crop species, and deliver an overview of bioinformatics tools that can be used to accomplish all needed tasks. Overall, this work aims to provide guidelines to harmonize those procedures leading to SNP datasets ready for crop GWAS.
Introduction
High-throughput genotyping, which leads to the identification of a large number of single-nucleotide polymorphisms (SNPs) is boosting the implementation of genome-wide association studies (GWAS), linking DNA variants to phenotypes of interest (Taranto et al., 2018). In crop species, GWAS enabled the mapping of genomic loci associated with economically important traits, including yield, resistance to biotic and abiotic stresses, and quality (Boyles et al., 2016; Pavan et al., 2017; Hou et al., 2018; Liu et al., 2018; He et al., 2019). This information has been further used to perform marker-assisted selection (MAS) in breeding programs and discover genes underlying phenotypic variation (Liu and Yan, 2019).
Several genotyping methods are available (reviewed by Scheben et al., 2017), which are usually performed by commercial parties upon the receipt of DNA samples. For application in GWAS, widely adopted genotyping options fall into three categories: whole genome resequencing (WGR), reduced representation sequencing (RRS), and SNP arrays. WGR and RRS are based on next-generation sequencing (NGS) technologies and bioinformatics pipelines that align reads to a reference genome and call both SNPs and genotypes (Nielsen et al., 2011). SNP arrays rely on allele-specific oligonucleotide (ASO) probes (including target SNP loci plus their flanking regions) fixed on a solid support, which are used to interrogate complementary fragments from DNA samples and infer genotypes based on the interpretation of the hybridization signal. Choosing the most appropriate (cost-effective) genotyping method for crop GWAS requires careful examination of several aspects, namely, the purpose and the scale of the study, crop-specific genomic features, and technical and economic matters associated with each genotyping method.
Raw SNP datasets resulting from genotyping experiments are typically inaccurate and incomplete. In addition, genes associated with phenotypes can have a small effect on genetic variance. In this scenario, quality control (QC) procedures are of pivotal importance to minimize false-positive or false-negative associations, referred to as type I and type II errors, respectively. QC includes filtering out poor-quality or suspected artifactual SNP loci, filtering out individuals in relation to missing data, anomalous genotype call and genetic synonymies, and the characterization of ancestral relationships among individuals of the GWAS population. Excellent reviews focused on QC of human SNP data (Turner et al., 2011; Marees et al., 2018); however, the QC procedure may be quite different for crop species. In this case, variables that need to be considered include the crop prevailing mating system (self- or open-pollinating) and the breeding history of the specific GWAS population.
This review aims to provide recommendations on how to plan genotyping experiments and best practices on how to perform QC in crop species.
Choosing the Correct Genotyping Method
Genotyping methods differ with respect to the number of identifiable SNPs and the cost of the analysis per sample, and these two parameters are directly proportional. Given this premise, choosing the correct option for GWAS requires to have a clear idea on two key aspects, i.e., the number of SNPs that is sufficient/desirable to fulfill the GWAS goals and the cost associated with each genotyping alternative. In addition, genotyping methods come with different technical specifications that should be evaluated in relation to the particular GWAS experiment.
Whole Genome Resequencing
WGR allows the highest number of SNP calls, up to several millions as reported in peach (Cao et al., 2016) and cotton (Du et al., 2018). This is a clear advantage when, rather than MAS, gene isolation is the main aim of the GWAS project (Wang et al., 2016; Happ et al., 2019). Indeed, in high-resolution GWAS, SNP loci showing the highest evidence of association are usually in tight linkage, or may even coincide, with loci underlying phenotypic variation (e.g., Shang et al., 2014; Yano et al., 2016). However, it should be pointed out that, even with a very high marker density, the identification of causal polymorphisms can be difficult in the case of GWAS populations displaying slow decay of linkage disequilibrium (LD) (i.e., populations in which the allelic state at two loci on the same chromosome tends to be correlated even at high physical distance) (Korte and Farlow, 2013). As shown in Table 1, in populations of self-pollinating crops, such as wheat or soybean, the average square correlation coefficient (r2) between pairs of loci may take several Mb to decay to values indicating substantial linkage equilibrium (0.2 or 0.1) (Vos et al., 2017).
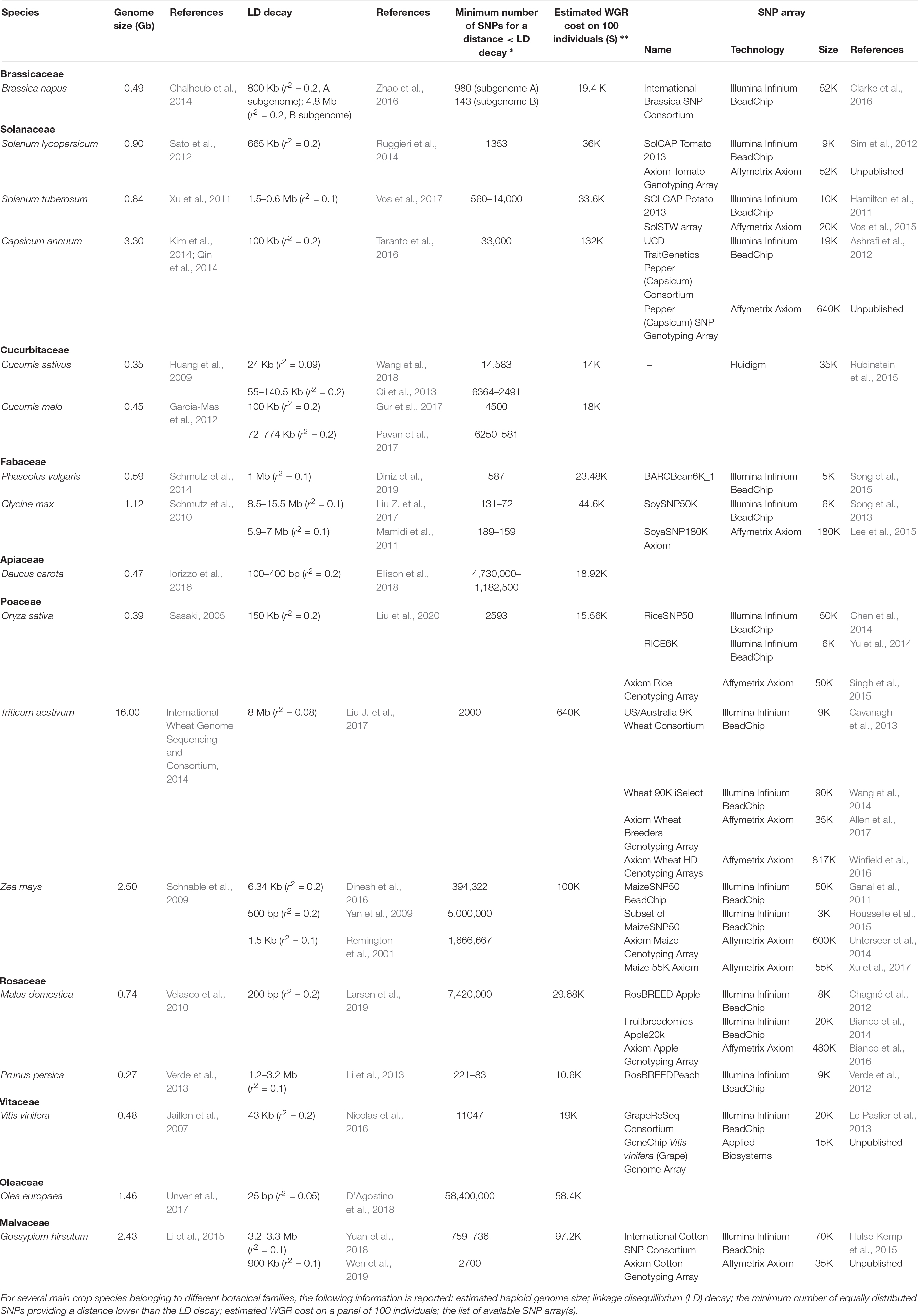
Table 1. List of some genomic and economic aspects that should be taken into consideration when planning GWAS in crops.
WGR is especially desirable for GWAS populations displaying rapid LD decay. Indeed, in this case, low marker density may result in missing genomic regions associated with phenotypic traits. Extremely rapid LD decay (in the range of a few base pairs) has been reported for highly heterozygous populations of open-pollinating species (e.g., maize, carrot, olive), in which recombination is effective in breaking up haplotypes (Table 1). In this situation, even in the ideal case of equally spaced SNPs, millions of markers would be required to have a SNP distance lower than the LD decay distance. This is exactly the condition that enables one to detect associations for most genomic regions (Table 1).
WGR genotyping has been so far generally performed using paired-end Illumina technology (e.g., Zhou et al., 2015; Cao et al., 2016; Liang et al., 2019), which, according to our survey, roughly costs $400 per sample for a genome of 1 Gb and 10× average sequencing depth (this term indicating the number of times a base is sequenced on average). This implies that WGR-based GWAS, typically involving a few hundred individuals, may cost several hundred thousand dollars for crops with large genomes, as shown in Table 1. Decreasing the average sequencing depth can lower the cost of WGR; however, this may result in an unacceptable number of genotyping errors. This is especially the case of heterozygous loci, which are associated with a larger number of genotypic combinations (Kishikawa et al., 2019). In practice, WGR in crops has been usually performed with average sequencing depth ranging from ∼5×, as for cotton (Du et al., 2018), tomato (Lin et al., 2014), and peach (Cao et al., 2019), to ∼15×, as for watermelon (Guo et al., 2019) and grapevine (Liang et al., 2019). A notable exception is represented by strict self-pollinating species, such as rice and soybean, for which very low average sequencing depth (1× or lower) has been successfully applied (Wang et al., 2016; Happ et al., 2019). Indeed, homozygous populations of pure lines are effectively haploid, thus allowing easy reconstruction of haplotypes and, consequently, accurate imputation of missing data (Wang et al., 2016).
Reduced Representation Sequencing
RRS consists in sequencing only a small fraction of the genome, thus reducing the cost of the analysis with respect to WGR (Hirsch et al., 2014). Genotyping by sequencing (GBS) (Elshire et al., 2011), restriction site-associated DNA sequencing (RADseq) (Davey and Blaxter, 2011), and double digest RAD sequencing (ddRAD-seq) (Truong et al., 2012), which use restriction enzymes (REs) for the reduction of genome complexity, are currently the most popular RRS methods used to perform GWAS in crops, mainly due to their moderate cost. At a minimum, this is approximately $35 per sample independently from the genome size and including the application of bioinformatics pipelines for SNP and genotype calling (You et al., 2018). Another advantage of these RRS methods is their scalability, meaning that different combinations of restriction enzymes may be used to customize the percentage of the genome captured.
The number of SNPs identified by RRS genotyping typically varies from a few to several thousands (Pavan et al., 2018, 2019; Colonna et al., 2019), depending on the amount of genome sequenced and population diversity. As discussed above, this output can be largely sufficient in GWAS experiments whose main aim is to implement marker-assisted selection, and for crops displaying slow LD decay (Table 1).
A major technical limitation of RSS is that the genomic distribution of SNPs depends on the specific combination of REs used (D’Agostino and Tripodi, 2017). In addition, sequencing depth at individual SNP loci identified by RRS is typically uneven, leading to under-calling of heterozygous loci and many missing data. The latter issue can be mitigated by genotype imputation strategies; however, we highlight that the success of genotype imputation depends on the genetic makeup of the GWAS population, which influences, among other things, the occurrence of long homozygous segments useful to reconstruct haplotypes (Glaubitz et al., 2014).
SNP Arrays
SNP arrays for agrigenomics have been developed for over a decade to meet the needs for single research groups or consortia and are still widely used for GWAS in crops despite the decreasing cost of NGS-based technologies (LaFramboise, 2009; Rasheed et al., 2017; Table 1). In 2017, the two leader manufacturers Affymetrix and Illumina had developed 46 SNP array platforms for 25 crop species, associated with a number of markers ranging from 3K to 820K (Rasheed et al., 2017). Pricing of array genotyping is widely considered to exceed that of RRS; however, this is subject to fluctuations over time and is volume-dependent, as it varies with the number of samples and the array SNP density. Indeed, Darrier et al. (2019), considering a set of 1000 barley accessions, found that genotyping with the Illumina 50K iSelect SNP array was cheaper than GBS, with respect to both the cost per sample (£40 vs. £60.50) and the cost per marker (£0.001 vs. £0.003).
From a technical standpoint, SNP array genotyping has a series of advantages. First, genotype calls are generally accurate, even for highly heterozygous species (Bourke et al., 2018). In addition, polyploid crops represent an advantageous field of application of SNP genotyping arrays, as: for allopolyploids, NGS genotyping is complicated by sequence similarity among subgenomes, which hinders the alignment of reads to the reference genome; for autopolyploids, NGS genotyping requires very high sequencing depth and specific polyploid haplotyping algorithms, which make use of the sequence reads to determine the sequence of alleles along the same chromosomes (Motazedi et al., 2018). To date, array providers developed platforms for nine polyploid species ranging from the tetraploid potato to the dodecaploid sugarcane (reviewed by You et al., 2018), together with software solutions suitable to genotype polyploid datasets [i.e., Affymetrix’s Power Tools (APT) and the Polyploid Genotyping Module within Illumina’s GenomeStudio]. We highlight that while GWAS are commonly performed in allopolyploids, GWAS in autopolyploids are complicated by difficulties in the assessment of population structure and allele dosage (Rosyara et al., 2016).
A main disadvantage of SNP arrays is that they suffer from ascertainment bias (Lachance and Tishkoff, 2013), i.e., they cannot identify marker-trait associations in the case of SNPs that were not present in the population used for the development of the array. In addition, a typical drawback in the use of SNP arrays is the possibility that information (e.g., SNP chromosomal location) used for the design of the array is outdated and that there is no consistency in the use of SNPs among different genotyping array formats.
Recommendations for Quality Control
Genotyping companies apply QC procedures depending on the method used. For NGS genotyping, these consist in removing loci with low sequencing depth (i.e., loci only supported by a few reads) and loci with low PHRED-like quality score (Q) (where Q indicates the probability that the base call is incorrect). As for array genotyping, these mainly consist in applying a clustering algorithm on fluorescence measurement data of ASO probes to distinguish samples into genotype clusters (allelic discrimination plot), and in assessing a set of QC scores on the goodness of cluster separation and signal-to-background ratio.
It should be clear that, in order to avoid bias and false signals in genotype-trait association tests, the QC procedures above mentioned are not enough and need to be complemented by others performed by the investigator, which are the focus of this paragraph. These include filtering procedures that are either common to any GWAS experiment or depend on the specific GWAS population type, as well as the characterization of the GWAS population for duplicated samples and ancestral relationships (Figure 1).
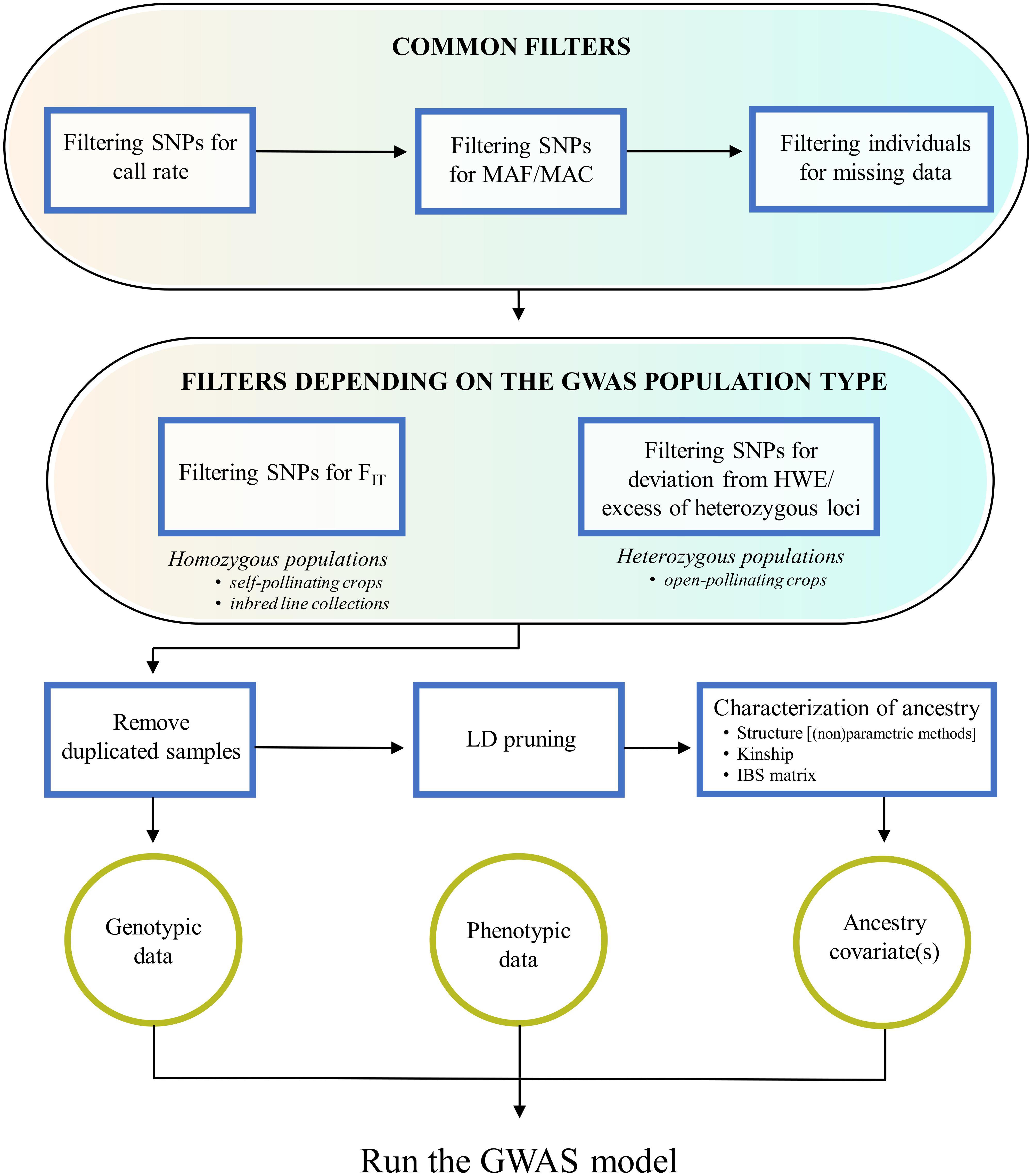
Figure 1. Overview of quality control procedures for crop GWAS. These include: filtering steps that are common to any GWAS experiment; filtering steps depending on the GWAS population structure (homozygous or heterozygous); the removal of duplicated samples; the characterization of ancestral relationships, starting from a SNP dataset pruned for markers in linkage disequilibrium.
Application of Common Filters
A high rate of missing data at a SNP locus is considered an indication of inaccurate genotype calls (Turner et al., 2011). Therefore, filtering SNPs for call rate is typically the first step in QC. A standard rule is filtering for SNPs with call rates ≥95 or 99% (Anderson et al., 2010); however, a lower threshold might be chosen, especially in the case of NGS genotyping with low sequencing depth. For example, GBS-derived SNP data in crops have been filtered using call rate thresholds of 90% or lower (e.g., Nimmakayala et al., 2014; Pavan et al., 2016, 2017). The overall distribution of call rates may be examined in order to set up a threshold value that eliminates classes occurring at suspiciously low frequency (Figure 2A).
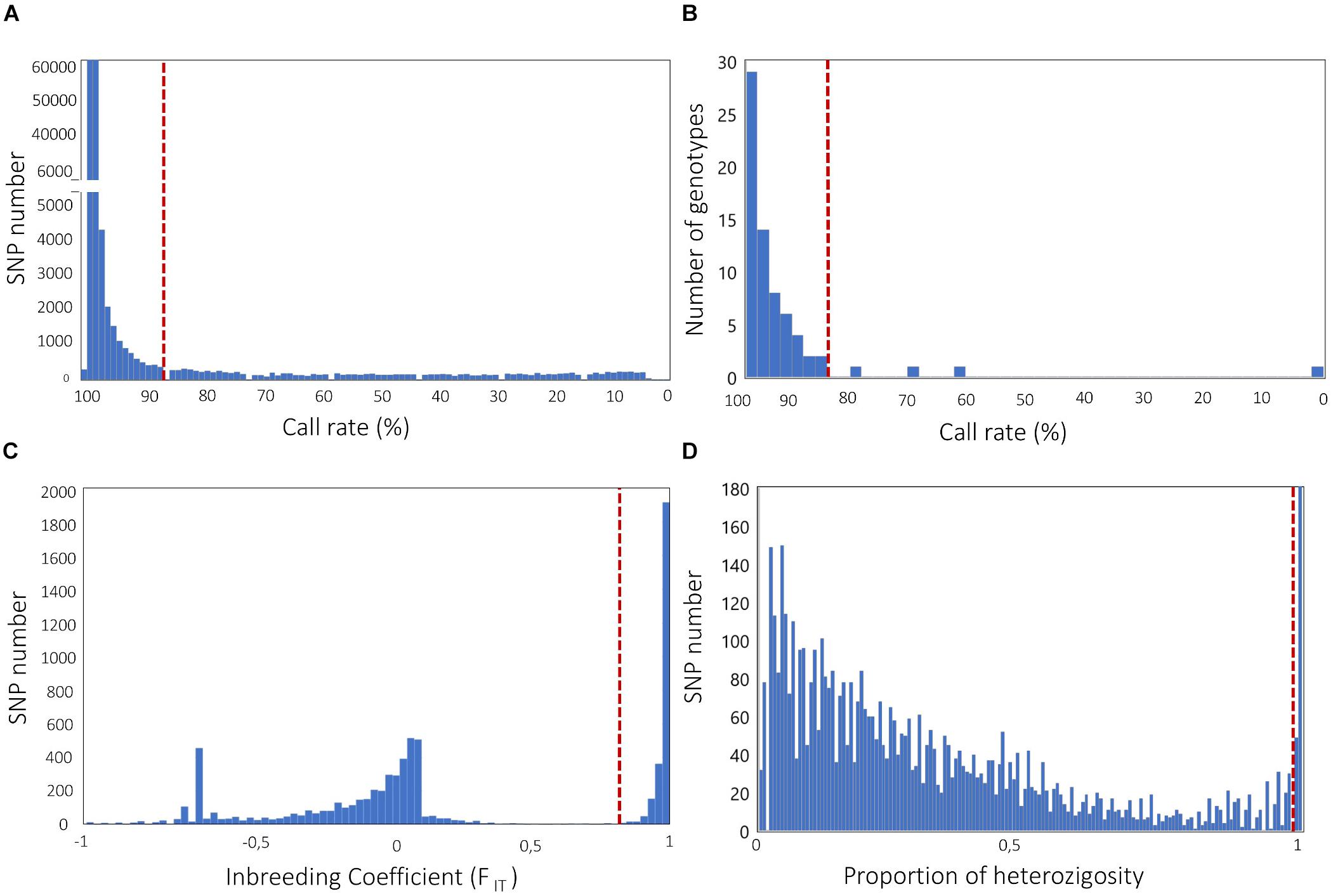
Figure 2. Frequency distribution analysis to define filtering solutions for (A) SNP call rate; (B) genotype call rate; (C) SNP inbreeding coefficient (FIT); (D) SNP proportion of heterozygosity. Dashed lines indicate possible filtering thresholds, based on classes occurring at suspiciously low (A,B) or high (D) frequency, and distribution gaps (C). Genotypic data used to build histograms are all relative to published genotyping-by-sequencing experiments, carried out in the self-pollinated crops Cicer arietinum L. (Pavan et al., 2017, A,C) and Lens culinaris Medik (Pavan et al., 2019, B), and the open-pollinated crop Cynara cardunculus L. (Pavan et al., 2018, D).
SNP loci displaying rare variants may arise from genotyping errors and, in addition, have low statistical power to reveal association with phenotypic traits, thus they are commonly excluded by QC procedures. In this sense, a widely adopted solution is filtering for minor allele frequency (MAF). Filtering for MAF ≥1–5% has been commonly applied for crop GWAS involving populations of a few hundred individuals (Pavan et al., 2017; Yu et al., 2018), however the same thresholds might be too stringent for larger GWAS populations. Filtering for minor allele count (MAC) allows to set-up thresholds independent from the GWAS population size, commonly ranging from 5 to 10 (e.g., Taranto et al., 2016; Thomson et al., 2017).
As for loci, the presence of individuals with high rates of missing data is also suggestive of technical issues, often related with poor quality and/or quantity of DNA samples. This can generate inaccuracies and bias in downstream analyses. We emphasize that filtering for SNP missingness should normally precede filtering for individual missingness, as the opposite procedure may result in unnecessary removal of individuals. In literature, very different cutoff thresholds for individual missingness have been reported (Begum et al., 2015; Pavan et al., 2018). Our suggestion is to inspect the distribution of missing data across individuals and select a threshold that allows the elimination of classes occurring at suspiciously low frequency (Figure 2B). In addition, for binary traits (e.g., the response to a pathogen, for which individuals of the GWAS population can be classified in either resistant or susceptible), it is of main importance that there are no systematic differences of call rate between the two groups, in order to avoid bias in association tests.
Application of Filters Depending on the GWAS Population Type
SNP loci characterized by excessive heterozygosity should be filtered out, as they are indicative of technical artifacts or paralogous/repetitive regions that could not be distinguished through the genotyping procedure (Glaubitz et al., 2014). Therefore, specific SNP filters are applied based on the extent of heterozygosity expected in the GWAS population. For crops, this depends on the natural mating system, which may favor self-pollination or open-pollination, and anthropic interventions, such as artificial inbreeding.
Natural populations of self-pollinating crops, as well as populations of inbred lines, are highly homozygous. Therefore, in these cases, even loci with modest heterozygosity rates are suspicious. Glaubitz et al. (2014) suggested the use of the FIT inbreeding coefficient (given by 1-Ho/HE, with Ho and HE being the observed heterozygosity and the expected heterozygosity at Hardy–Weinberg equilibrium, respectively) to filter SNPs in homozygous populations, and applied a minimal FIT threshold of 0.8 in case of a large population of maize inbred lines. The identification of gaps in the distribution of FIT across all loci may help to set up a threshold that allows the elimination of most of the genotyping errors while retaining the highest possible number of loci (Figure 2C).
For natural populations of open-pollinating crops, filtering SNPs that significantly deviate from the Hardy–Weinberg equilibrium (HWE) (e.g., through chi-square or exact tests) can be performed to remove excessively heterozygous loci. In accordance with GWAS on human genotypic data, the HWE filter in open-pollinating crops has been generally applied using a threshold p-value of 10–4, e.g., in, cassava, olive and watermelon (Anderson et al., 2010; Nimmakayala et al., 2014; D’Agostino et al., 2018; Zhang et al., 2018). We stress here that, in crops, the HWE filter should be used with care, as there is the risk of a significant and unnecessary loss of the GWAS resolution power. Indeed, it should be firstly noticed that the HWE assumption of random mating is not respected when the population has strong genetic structure (see next paragraph) and contains some inbred individuals. Secondly, loci under selection violate by definition the HWE, thus the HWE filter might exclude loci associated with important traits under investigation. All of this considered, solutions might be to (i) adopt a relaxed threshold to eliminate markers, e.g., p < 10–6, as previously performed on apple and globe artichoke (Bianco et al., 2016; Pavan et al., 2018); (ii) apply the HWE filter separately to each sub-population identified by the analysis of genetic structure; (iii) apply the HWE filter only to individuals not showing the phenotype supposedly under selection, in case of GWAS on binary traits. In other circumstances, including that of partially outbreeding crops, it might be advisable to avoid the HWE filter and, as a possible alternative, to eliminate SNPs with unexpected high levels of heterozygosity (Figure 2D).
Checking for Sample Duplication and Ancestral Relationships
In the case of crops, GWAS populations might contain several genetically identical samples. This is often caused by the occurrence, in germplasm collections, of unintended duplication of anonymous accessions and/or the occurrence of synonymous accessions. For example, genotyping with the 9K SNP array of the USDA grapevine collection revealed that 568 out of 950 accessions (58%) were genetically identical to at least another accession (Myles et al., 2011).
The identification and removal of duplicated samples is usually performed on the basis of pairwise identity-by-state (IBS) or identity-by-descent (IBD). Pairwise IBS refers to the proportion of alleles shared by two individuals, whereas pairwise IBD refers to the proportion of two individuals’ genome tracing back to the same recent common ancestor (Purcell et al., 2007; Manichaikul et al., 2010). The latter is commonly estimated from pairwise IBS and allele frequency using a method-of-moment algorithm (Purcell et al., 2007). Many studies have used IBS/IBD thresholds of 95 or 99% to declare samples as identical (Myles et al., 2011). The examination of the IBS/IBD distribution associated with a few known identical samples, included on purpose in the GWAS population, might also be used to set up a threshold to estimate identity (Pavan et al., 2019).
Ancestral relationships generate LD between unlinked loci, so they are considered in the GWAS model to limit spurious associations (Astle and Balding, 2009). Therefore, a crucial step in the QC procedure is the characterization of ancestry within the GWAS population. Genetic structure (i.e., the occurrence of sub-populations with different allele frequencies) reflects remote differences in ancestry; in crops, it often originates from physical barriers to random mating and anthropic selection for specific traits, such as seed/fruit size and phenological features (Pavan et al., 2017, 2019; Siol et al., 2017). Instead, kinship reflects recent ancestry, often related to pedigree connections among modern cultivars (Taranto et al., 2020).
Starting from genotypic data, the analysis of population structure can be carried out through different approaches. Parametric methods, such as those implemented in the popular software STRUCTURE (Pritchard et al., 2000) and ADMIXTURE (Alexander et al., 2009), typically estimate the allele frequency of each sub-population jointly with the membership of individuals to each sub-population, using maximum-likelihood or Bayesian statistics. The resulting matrix (known as Q-matrix), which indicates, for each individual, the proportion of the genome referable to various sub-populations, can be conveniently incorporated in GWAS models. However, it should be noticed that parametric methods are based on several genetic assumptions, including those of linkage equilibrium (LE) among markers and HWE within sub-populations. Approximate LE from the original SNP dataset can be obtained by removing markers through LD pruning algorithms (Joiret et al., 2019); on the other hand, HWE may not be met even in populations of open-pollinating crops, due to displacements, breeding activities, and clonal propagation (Campoy et al., 2016).
Non-parametric methods such as principal component analysis (PCA) and multidimensional scaling (MDS) can be used to account for population structure, using coordinates of each individual along the main PCA/MDS axes as covariates in association models (Wang et al., 2009). While non-parametric methods have the advantage of being independent on genetic assumptions, they also come with a number of issues that need to be considered. Importantly, the top PCA/MDS axes may not adequately capture variation due to population structure in the presence of other strong sources of variation, such as outlier sub-populations/individuals or family groups (Price et al., 2010; Liu et al., 2013). These latter may be frequent when the GWAS population contains many cultivars with similar pedigrees. Finally, as for parametric models, it is advisable to perform LD pruning prior to non-parametric analysis, in order to avoid noise from correlated marker data (Liu et al., 2013).
Kinship ultimately depends on the proportion of the genome that is identical-by-descent. Therefore, in order to account for kinship, the GWAS model can use IBD estimates from pedigree notes. However, it is clear that pedigrees of crop species might be in several cases unknown or inaccurate. As mentioned above in this paragraph, methods to estimate pairwise IBD from genotypic data have been also developed. These yield a kinship matrix, also referred to as K-matrix, which has been widely used together with the Q-matrix or PCA/MDS covariates to implement the so-called Q + K and P + K GWAS models (Yu et al., 2006; Zhao et al., 2007).
We finally highlight that several works showed that a simple pairwise IBS matrix could efficiently capture both remote and recent ancestry (Zhao et al., 2007). Therefore, many GWAS models today accommodate the IBS matrix in the framework of linear mixed models, under the assumption that phenotypic variation is positively correlated with genetic distance (e.g., Kang et al., 2008, 2010).
Bioinformatics Tools to Perform QC
QC can be carried out using several bioinformatics tools, which may differ with respect to the specific action(s) performed and the file requested as input. Therefore, the investigator is often called to the conversion of genotypic data among different formats, the most common being variant call format (VCF), haplotype map (hapmap), pedigree/map (ped/map), binary (bed/bim/fam), Affymetrix chip (chp), Illumina sample map and final report, and structure. PGDSpider1 (Lischer and Excoffier, 2012) is a dedicated tool for the conversion of genotypic data among a wide range of formats. Among other powerful conversion tools, we mention the one implemented in the software suite TASSEL (Bradbury et al., 2007), which deals with the most common formats associated with NGS genotyping, and the gene_converter function within the R package radiator (Gosselin, 2017), accepting and delivering 13 and 29 file formats, respectively.
Several open-source software suites are available for QC. Among the most widely used, PLINK (Purcell et al., 2007), starting from common genotypic data file formats (ped/map, bed/bim/fam and VCF), enables the application of all the SNP and individual filters presented in Sections “Application of Common Filters” and “Application of Filters Depending on the GWAS Population Type,” with the exception of the FIT filter. In relation to the study of genetic ancestry, it has options for LD pruning and MDS, and for the estimation of pairwise IBS and IBD.
Compared with PLINK, the abovementioned TASSEL (Bradbury et al., 2007) accepts a wider range of file formats (also including hapmap) and does not perform filtering for HWE departure. On the other hand, having been developed for GWAS on maize inbred lines, TASSEL provides the possibility to perform the FIT filter. As for the genetic ancestry options, it can perform PCA/MDS and estimate pairwise IBS. While PLINK is based on command lines, thus requiring specific training by the user, TASSEL also implements a graphical user interface. Another important feature of TASSEL is the possibility to easily build histograms for SNP and individual missingness and SNP heterozygosity, which, as discussed above, are useful to set up cutoff thresholds specific for each GWAS experiment.
Investigators with some bioinformatics skills may be interested in QC tools also enabling filtering procedures depending on the genotyping method, which, as stated above, are commonly performed through external services. For NGS genotyping, we cite VCFtools (Danecek et al., 2011), a command line software suite developed for the VCF format, which allows, among other options, filtering SNP sites and individuals based on sequencing depth and PHRED-quality score. For array genotyping, we cite the following: (i) the proprietary packages GenomeStudio and Axiom Analysis Suite, for data generated on Illumina or Affymetrix SNP array platforms, respectively; (ii) freeware tools that directly accept raw data in the original format generated by array genotyping platforms, including fluorescence intensity data necessary for QC of genotype calls. Among the many available options, we cite here the R packages argyle (Morgan, 2016) and SNPQC (Gondro et al., 2014), and the Python package ASSIsT (Di Guardo et al., 2015), for data generated on Illumina SNP array platforms, and AffyPipe (Nicolazzi et al., 2014), for data generated on Affymetrix SNP array platforms.
Finally, concerning the study of genetic structure, besides the above mentioned STRUCTURE (Pritchard et al., 2000) and ADMIXTURE (Alexander et al., 2009), the EIGENSOFT utilities SMARTPCA and SMARTEIGENSTRAT are popular bioinformatics tools for, respectively, detecting and analyzing population structure via PCA, and correcting for population stratification in association studies (Price et al., 2006).
Conclusion
This work is thought to provide researchers, who mainly focus on the biology and breeding of crop species, with essential technical and economic aspects required to plan and carry out cost-effective and accurate GWAS. To the best of our knowledge, this is the first work specifically addressing the issue of QC in crop species, so we expect that it may contribute to the future harmonization of the procedures leading to the obtainment of high-quality SNP datasets ready for GWAS.
Author Contributions
SP, ND’A, and EC conceived the review. SP, ND’A, EC, and CD wrote the manuscript. CL and LR critically revised the manuscript.
Funding
This research has been performed within the project “LEgume GEnetic REsources as a tool for the development of innovative and sustainable food TEchnological system” supported under the “Thought for Food” Initiative by Agropolis Fondation (through the “Investissements d’avenir” programme with reference number ANR-10-LABX-0001-01), Fondazione Cariplo, and Daniel & Nina Carasso Foundation.
Conflict of Interest
The authors declare that the research was conducted in the absence of any commercial or financial relationships that could be construed as a potential conflict of interest.
Footnotes
References
Alexander, D. H., Novembre, J., and Lange, K. (2009). Fast model-based estimation of ancestry in unrelated individuals. Genome Res. 19, 1655–1664. doi: 10.1101/gr.094052.109
Allen, A. M., Winfield, M. O., Burridge, A. J., Downie, R. C., Benbow, H. R., Barker, G. L. A., et al. (2017). Characterization of a wheat breeders’ array suitable for high-throughput SNP genotyping of global accessions of hexaploid bread wheat (Triticum aestivum). Plant Biotechnol. J. 15, 390–401. doi: 10.1111/pbi.12635
Anderson, C. A., Pettersson, F. H., Clarke, G. M., Cardon, L. R., Morris, A. P., and Zondervan, K. T. (2010). Data quality control in genetic case-control association studies. Nat. Protoc. 5, 1564–1573. doi: 10.1038/nprot.2010.116
Ashrafi, H., Hill, T., Stoffel, K., Kozik, A., Yao, J., Chin-Wo, S. R., et al. (2012). De novo assembly of the pepper transcriptome (Capsicum annuum): a benchmark for in silico discovery of SNPs, SSRs and candidate genes. BMC Genomics 13:571. doi: 10.1186/1471-2164-13-571
Astle, W., and Balding, D. J. (2009). Population structure and cryptic relatedness in genetic association studies. Stat. Sci. 24, 451–471. doi: 10.1214/09-STS307
Begum, H., Spindel, J. E., Lalusin, A., Borromeo, T., Gregorio, G., Hernandez, J., et al. (2015). Genome-wide association mapping for yield and other agronomic traits in an elite breeding population of tropical rice (Oryza sativa). PLoS One 10:e0119873. doi: 10.1371/journal.pone.0119873
Bianco, L., Cestaro, A., Linsmith, G., Muranty, H., Denancé, C., Théron, A., et al. (2016). Development and validation of the Axiom® Apple480K SNP genotyping array. Plant J. 86, 62–74. doi: 10.1111/tpj.13145
Bianco, L., Cestaro, A., Sargent, D. J., Banchi, E., Derdak, S., Di Guardo, M., et al. (2014). Development and validation of a 20K Single nucleotide polymorphism (SNP) whole genome genotyping array for apple (Malus × domestica Borkh). PLoS One 9:e0110377. doi: 10.1371/journal.pone.0110377
Bourke, P. M., Voorrips, R. E., Visser, R. G. F., and Maliepaard, C. (2018). Tools for genetic studies in experimental populations of polyploids. Front. Plant Sci. 9:513. doi: 10.3389/fpls.2018.00513
Boyles, R. E., Cooper, E. A., Myers, M. T., Brenton, Z., Rauh, B. L., Morris, G. P., et al. (2016). Genome-wide association studies of grain yield components in diverse sorghum germplasm. Plant Genome 9, 1–17. doi: 10.3835/plantgenome2015.09.0091
Bradbury, P. J., Zhang, Z., Kroon, D. E., Casstevens, T. M., Ramdoss, Y., and Buckler, E. S. (2007). TASSEL: software for association mapping of complex traits in diverse samples. Bioinformatics 23, 2633–2635. doi: 10.1093/bioinformatics/btm308
Campoy, J. A., Lerigoleur-Balsemin, E., Christmann, H., Beauvieux, R., Girollet, N., Quero-García, J., et al. (2016). Genetic diversity, linkage disequilibrium, population structure and construction of a core collection of Prunus avium L. landraces and bred cultivars. BMC Plant Biol. 16:49. doi: 10.1186/s12870-016-0712-9
Cao, K., Li, Y., Deng, C. H., Gardiner, S. E., Zhu, G., Fang, W., et al. (2019). Comparative population genomics identified genomic regions and candidate genes associated with fruit domestication traits in peach. Plant Biotechnol. J. 17, 1954–1970. doi: 10.1111/pbi.13112
Cao, K., Zhou, Z., Wang, Q., Guo, J., Zhao, P., Zhu, P., et al. (2016). Genome-wide association study of 12 agronomic traits in peach. Nat. Commun. 7:13246. doi: 10.1038/ncomms13246
Cavanagh, C. R., Chao, S., Wang, S., Huang, B. E., Stephen, S., Kiani, S., et al. (2013). Genome-wide comparative diversity uncovers multiple targets of selection for improvement in hexaploid wheat landraces and cultivars. Proc. Natl. Acad. Sci. U.S.A. 110, 8057–8062. doi: 10.1073/pnas.1217133110
Chagné, D., Crowhurst, R. N., Troggio, M., Davey, M. W., Gilmore, B., Lawley, C., et al. (2012). Genome-wide SNP detection, validation, and development of an 8K SNP array for apple. PLoS One 7:e31745. doi: 10.1371/journal.pone.0031745
Chalhoub, B., Denoeud, F., Liu, S., Parkin, I. A. P., Tang, H., Wang, X., et al. (2014). Early allopolyploid evolution in the Post-neolithic brassica napus oilseed. Genome Sci. 345, 950–953. doi: 10.1126/science.1253435
Chen, H., Xie, W., He, H., Yu, H., Chen, W., Li, J., et al. (2014). A high-density snp genotyping array for rice biology and molecular breeding. Mol. Plant 7, 541–553. doi: 10.1093/mp/sst135
Clarke, W. E., Higgins, E. E., Plieske, J., Wieseke, R., Sidebottom, C., Khedikar, Y., et al. (2016). A high-density SNP genotyping array for brassica napus and its ancestral diploid species based on optimised selection of single-locus markers in the allotetraploid genome. Theor. Appl. Genet. 129, 1887–1899. doi: 10.1007/s00122-016-2746-7
Colonna, V., D’Agostino, N., Garrison, E., Albrechtsen, A., Meisner, J., Facchiano, A., et al. (2019). Genomic diversity and novel genome-wide association with fruit morphology in Capsicum, from 746k polymorphic sites. Sci. Rep. 9:10067. doi: 10.1038/s41598-019-46136-5
D’Agostino, N., Taranto, F., Camposeo, S., Mangini, G., Fanelli, V., Gadaleta, S., et al. (2018). GBS-derived SNP catalogue unveiled wide genetic variability and geographical relationships of Italian olive cultivars. Sci. Rep. 8:15877. doi: 10.1038/s41598-018-34207-y
D’Agostino, N., and Tripodi, P. (2017). NGS-based genotyping, high-throughput phenotyping and genome-wide association studies laid the foundations for next-generation breeding in horticultural crops. Diversity 9:38. doi: 10.3390/d9030038
Danecek, P., Auton, A., Abecasis, G., Albers, C. A., Banks, E., DePristo, M. A., et al. (2011). The variant call format and VCFtools. Bioinformatics 27, 2156–2158. doi: 10.1093/bioinformatics/btr330
Darrier, B., Russell, J., Milner, S. G., Hedley, P. E., Shaw, P. D., Macaulay, M., et al. (2019). A comparison of mainstream genotyping platforms for the evaluation and use of barley genetic resources. Front. Plant Sci. 10:554. doi: 10.3389/fpls.2019.00544
Davey, J. W., and Blaxter, M. L. (2011). RADSeq: next-generation population genetics. Brief. Funct. Genomics 9, 416–423. doi: 10.1093/bfgp/elr007
Di Guardo, M., Micheletti, D., Bianco, L., Koehorst-Van Putten, H. J. J., Longhi, S., Costa, F., et al. (2015). ASSIsT: an automatic SNP scoring tool for in- and outbreeding species. Bioinformatics 31, 3873–3874. doi: 10.1093/bioinformatics/btv446
Dinesh, A., Patil, A., Zaidi, P. H., Kuchanur, P. H., Vinayan, M. T., and Seetharam, K. (2016). Genetic diversity, linkage disequilibrium and population structure among CIMMYT maize inbred lines, selected for heat tolerance study. Maydica 61, 1–7.
Diniz, A. L., Giordani, W., Costa, Z. P., Margarido, G. R. A., Perseguini, J. M. K. C., Benchimol-Reis, L. L., et al. (2019). Evidence for strong kinship influence on the extent of linkage disequilibrium in cultivated common beans. Genes 10:5. doi: 10.3390/genes10010005
Du, X., Huang, G., He, S., Yang, Z., Sun, G., Xiongfeng, M., et al. (2018). Resequencing of 243 diploid cotton accessions based on an updated A genome identifies the genetic basis of key agronomic traits. Nat. Genet. 50, 796–802. doi: 10.1038/s41588-018-0116-x
Ellison, S. L., Luby, C. H., Corak, K. E., Coe, K. M., Senalik, D., Iorizzo, M., et al. (2018). Carotenoid presence is associated with the or gene in domesticated carrot. Genetics 210, 1497–1508. doi: 10.1534/genetics.118.301299
Elshire, R. J., Glaubitz, J. C., Sun, Q., Poland, J. A., Kawamoto, K., Buckler, E. S., et al. (2011). A robust, simple genotyping-by-sequencing (GBS) approach for high diversity species. PLoS One 6:e19379. doi: 10.1371/journal.pone.0019379
Ganal, M. W., Durstewitz, G., Polley, A., Bérard, A., Buckler, E. S., Charcosset, A., et al. (2011). A large maize (zea mays L.) SNP genotyping array: development and germplasm genotyping, and genetic mapping to compare with the B73 reference genome. PLoS One 6:e28334. doi: 10.1371/journal.pone.0028334
Garcia-Mas, J., Benjak, A., Sanseverino, W., Bourgeois, M., Mir, G., Gonźalez, V. M., et al. (2012). The genome of melon (Cucumis melo L.). Proc. Natl. Acad. Sci. U.S.A. 109, 11872–11877. doi: 10.1073/pnas.1205415109
Glaubitz, J. C., Casstevens, T. M., Lu, F., Harriman, J., Elshire, R. J., Sun, Q., et al. (2014). TASSEL-GBS: a high capacity genotyping by sequencing analysis pipeline. PLoS One 9:e90346. doi: 10.1371/journal.pone.0090346
Gondro, C., Porto-Neto, L. R., and Lee, S. H. (2014). SNPQC-an R pipeline for quality control of Illumina SNP genotyping array data. Anim. Genet. 45, 758–761. doi: 10.1111/age.12198
Gosselin, T. (2017). Radiator: RADseq Data Exploration, Manipulation and Visualization Using R. R Package Version 0.0.5. Available at: https://github.com/thierrygosselin/radiator (accessed May 15, 2018).
Guo, S., Zhao, S., Sun, H., Wang, X., Wu, S., Lin, T., et al. (2019). Resequencing of 414 cultivated and wild watermelon accessions identifies selection for fruit quality traits. Nat. Genet. 51, 1616–1623. doi: 10.1038/s41588-019-0518-4
Gur, A., Tzuri, G., Meir, A., Sa’Ar, U., Portnoy, V., Katzir, N., et al. (2017). Genome-wide linkage-disequilibrium mapping to the candidate gene level in melon (Cucumis melo). Sci. Rep. 7:9770. doi: 10.1038/s41598-017-09987-4
Hamilton, J. P., Hansey, C. N., Whitty, B. R., Stoffel, K., Massa, A. N., Van Deynze, A., et al. (2011). Single nucleotide polymorphism discovery in elite north american potato germplasm. BMC Genomics 12:302. doi: 10.1186/1471-2164-12-302
Happ, M. M., Wang, H., Graef, G. L., and Hyten, D. L. (2019). Generating high density, low cost genotype data in soybean [Glycine max (L.) Merr.]. G3 Genes Genom Genet. 9, 2153–2160. doi: 10.1534/g3.119.400093
He, Y., Yan, L., Ge, C., Yao, X., Han, X., Wang, R., et al. (2019). Pinoid is required for formation of the stigma and style in rice. Plant Physiol. 180, 926–936. doi: 10.1104/pp.18.01389
Hirsch, C. D., Evans, J., Buell, C. R., and Hirsch, C. N. (2014). Reduced representation approaches to interrogate genome diversity in large repetitive plant genomes. Brief. Funct. Genom 13, 257–267. doi: 10.1093/bfgp/elt051
Hou, S., Zhu, G., Li, Y., Li, W., Fu, J., Niu, E., et al. (2018). Genome-wide association studies reveal genetic variation and candidate genes of drought stress related traits in cotton (Gossypium hirsutum l.). Front. Plant. Sci. 9:1276. doi: 10.3389/fpls.2018.01276
Huang, S., Li, R., Zhang, Z., Li, L., Gu, X., Fan, W., et al. (2009). The genome of the cucumber. Cucumis sativus L. Nat. Genet. 41, 1275–1281. doi: 10.1038/ng.475
Hulse-Kemp, A. M., Lemm, J., Plieske, J., Ashrafi, H., Buyyarapu, R., Fang, D. D., et al. (2015). Development of a 63K SNP array for cotton and high-density mapping of intraspecific and interspecific populations of Gossypium spp. G3-Genes Genom. Genet. 5, 1187–1209. doi: 10.1534/g3.115.018416
International Wheat Genome Sequencing and Consortium. (2014). A chromosome-based draft sequence of the hexaploid bread wheat (Triticum Aestivum) Genome. Science 345:1251788. doi: 10.1126/science.1251788
Iorizzo, M., Ellison, S., Senalik, D., Zeng, P., Satapoomin, P., Huang, J., et al. (2016). A high-quality carrot genome assembly provides new insights into carotenoid accumulation and asterid genome evolution. Nat. Genet. 48, 657–666. doi: 10.1038/ng.3565
Jaillon, O., Aury, J.-M., Noel, B., Policriti, A., Clepet, C., Casagrande, A., et al. (2007). The grapevine genome sequence suggests ancestral hexaploidization in major angiosperm phyla. Nature 449, 463–467. doi: 10.1038/nature06148
Joiret, M., Mahachie John, J. M., Gusareva, E. S., and Van Steen, K. (2019). Confounding of linkage disequilibrium patterns in large scale DNA based gene-gene interaction studies. BioData Min. 12:11. doi: 10.1186/s13040-019-0199-7
Kang, H. M., Sul, J. H., Service, S. K., Zaitlen, N. A., Kong, S.-Y., Freimer, N. B., et al. (2010). Variance component model to account for sample structure in genome-wide association studies. Nat. Genet. 42, 348–354. doi: 10.1038/ng.548
Kang, H. M., Zaitlen, N. A., Wade, C. M., Kirby, A., Heckerman, D., Daly, M. J., et al. (2008). Efficient control of population structure in model organism association mapping. Genetics 178, 1709–1723. doi: 10.1534/genetics.107.080101
Kim, S., Park, M., Yeom, S.-I., Kim, Y.-M., Lee, J. M., Lee, H.-A., et al. (2014). Genome sequence of the hot pepper provides insights into the evolution of pungency in Capsicum species. Nat. Genet. 46, 270–278. doi: 10.1038/ng.2877
Kishikawa, T., Momozawa, Y., Ozeki, T., Mushiroda, T., Inohara, H., Kamatani, Y., et al. (2019). Empirical evaluation of variant calling accuracy using ultra-deep whole-genome sequencing data. Sci. Rep. 9:1784. doi: 10.1038/s41598-018-38346-0
Korte, A., and Farlow, A. (2013). The advantages and limitations of trait analysis with GWAS: a review. Plant Methods 9:29. doi: 10.1186/1746-4811-9-29
Lachance, J., and Tishkoff, S. A. (2013). SNP ascertainment bias in population genetic analyses: why it is important, and how to correct it. BioEssays 35, 780–786. doi: 10.1002/bies.201300014
LaFramboise, T. (2009). Single nucleotide polymorphism arrays: a decade of biological, computational and technological advances. Nucleic. Acids. Res. 37, 4181–4193. doi: 10.1093/nar/gkp552
Larsen, B., Migicovsky, Z., Jeppesen, A. A., Gardner, K. M., Toldam-Andersen, T. B., Myles, S. D., et al. (2019). Genome-wide association studies in apple reveal loci for aroma volatiles, sugar composition, and harvest date. Plant Genome 12:180104. doi: 10.3835/plantgenome2018.12.0104
Le Paslier, M.-C., Choisne, N., Bacilieri, R., Bounon, R., Boursiquot, J.-M., Brunel, D., et al. (2013). “The GrapeReSeq 18k Vitis genotyping chip,” in Proceeding of the Ninth International Symposium on Grapevine Physiologyand Biotechnology, La Serena.
Lee, Y.-G., Jeong, N., Kim, J. H., Lee, K., Kim, K. H., Pirani, A., et al. (2015). Development, validation and genetic analysis of a large soybean SNP genotyping array. Plant J. 81, 625–636. doi: 10.1111/tpj.12755
Li, F., Fan, G., Lu, C., Xiao, G., Zou, C., Kohel, R. J., et al. (2015). Genome sequence of cultivated Upland cotton (Gossypium hirsutum TM-1) provides insights into genome evolution. Nat. Biotechnol. 33, 524–530. doi: 10.1038/nbt.3208
Li, X.-W., Meng, X.-Q., Jia, H.-J., Yu, M.-L., Ma, R.-J., Wang, L.-R., et al. (2013). Peach genetic resources: diversity, population structure and linkage disequilibrium. BMC Genet. 14:84. doi: 10.1186/1471-2156-14-84
Liang, Z., Duan, S., Sheng, J., Zhu, S., Ni, X., Shao, J., et al. (2019). Whole-genome resequencing of 472 Vitis accessions for grapevine diversity and demographic history analyses. Nat. Commun. 10:1190. doi: 10.1038/s41467-019-09135-8
Lin, T., Zhu, G., Zhang, J., Xu, X., Yu, Q., Zheng, Z., et al. (2014). Genomic analyses provide insights into the history of tomato breeding. Nat. Genet. 46, 1220–1226. doi: 10.1038/ng.3117
Lischer, H. E. L., and Excoffier, L. (2012). PGDSpider: an automated data conversion tool for connecting population genetics and genomics programs. Bioinformatics 28, 298–299. doi: 10.1093/bioinformatics/btr642
Liu, H., and Yan, J. (2019). Crop genome-wide association study: a harvest of biological relevance. Plant J. 97, 8–18. doi: 10.1111/tpj.14139
Liu, H., Zhan, J., Li, J., Lu, X., Liu, J., Wang, Y., et al. (2020). Genome-wide association study (GWAS) for mesocotyl elongation in rice (Oryza sativa L.) under multiple culture conditions. Genes 11:49. doi: 10.3390/genes11010049
Liu, J., He, Z., Rasheed, A., Wen, W., Yan, J., Zhang, P., et al. (2017). Genome-wide association mapping of black point reaction in common wheat (Triticum aestivum L.). BMC Plant Biol. 17:220. doi: 10.1186/s12870-017-1167-3
Liu, L., Zhang, D., Liu, H., and Arendt, C. (2013). Robust methods for population stratification in genome wide association studies. BMC Bioinformatics 14:132. doi: 10.1186/1471-2105-14-132
Liu, R., Gong, J., Xiao, X., Zhang, Z., Li, J., Liu, A., et al. (2018). Gwas analysis and qtl identification of fiber quality traits and yield components in upland cotton using enriched high-density snp markers. Front. Plant. Sci. 9:1067. doi: 10.3389/fpls.2018.01067
Liu, Z., Li, H., Wen, Z., Fan, X., Li, Y., Guan, R., et al. (2017). Comparison of genetic diversity between Chinese and American soybean (Glycine max (L.)) accessions revealed by high-density SNPs. Front. Plant. Sci. 8:2014. doi: 10.3389/fpls.2017.02014
Mamidi, S., Chikara, S., Goos, R. J., Hyten, D. L., Annam, D., Moghaddam, S. M., et al. (2011). Genome-wide association analysis identifies candidate genes associated with iron deficiency chlorosis in soybean. Plant Genome 4, 154–164. doi: 10.3835/plantgenome2011.04.0011
Manichaikul, A., Mychaleckyj, J. C., Rich, S. S., Daly, K., Sale, M., and Chen, W. (2010). Robust relationship inference in genome-wide association studies. Bioinformatics 26, 2867–2873. doi: 10.1093/bioinformatics/btq559
Marees, A. T., de Kluiver, H., Stringer, S., Vorspan, F., Curis, E., Marie-Claire, C., et al. (2018). A tutorial on conducting genome-wide association studies: quality control and statistical analysis. Int. J. Methods Psychiatr. Res. 27:e1608. doi: 10.1002/mpr.1608
Morgan, A. P. (2016). argyle: an R package for analysis of illumina genotyping arrays. G3 Genes Genom.Genet. 6, 281–286. doi: 10.1534/g3.115.023739
Motazedi, E., Finkers, R., Maliepaard, C., and de Ridder, D. (2018). Exploiting next-generation sequencing to solve the haplotyping puzzle in polyploids: a simulation study. Brief. Bioinform 19, 387–403. doi: 10.1093/bib/bbw126
Myles, S., Boyko, A. R., Owens, C. L., Brown, P. J., Grassi, F., Aradhya, M. K., et al. (2011). Genetic structure and domestication history of the grape. Proc. Natl. Acad. Sci. U.S.A. 108, 3530–3535. doi: 10.1073/pnas.1009363108
Nicolas, S. D., Péros, J.-P., Lacombe, T., Launay, A., Le Paslier, M.-C., Bérard, A., et al. (2016). Genetic diversity, linkage disequilibrium and power of a large grapevine (Vitis vinifera L.) diversity panel newly designed for association studies. BMC Plant Biol. 16:74. doi: 10.1186/s12870-016-0754-z
Nicolazzi, E. L., Iamartino, D., and Williams, J. L. (2014). AffyPipe: an open-source pipeline for Affymetrix Axiom genotyping workflow. Bioinformatics 30, 3118–3119. doi: 10.1093/bioinformatics/btu486
Nielsen, R., Paul, J. S., Albrechtsen, A., and Song, Y. S. (2011). Genotype and SNP calling from next-generation sequencing data. Nat. Rev. Genet. 12, 443–451. doi: 10.1038/nrg2986
Nimmakayala, P., Levi, A., Abburi, L., Abburi, V. L., Tomason, Y. R., Saminathan, T., et al. (2014). Single nucleotide polymorphisms generated by genotyping by sequencing to characterize genome-wide diversity, linkage disequilibrium, and selective sweeps in cultivated watermelon. BMC Genomics 15:767. doi: 10.1186/1471-2164-15-767
Pavan, S., Bardaro, N., Fanelli, V., Marcotrigiano, A. R., Mangini, G., Taranto, F., et al. (2019). Genotyping by sequencing of cultivated lentil (lens culinaris medik.) highlights population structure in the mediterranean gene pool associated with geographic patterns and phenotypic variables. Front. Genet. 10:872. doi: 10.3389/fgene.2019.00872
Pavan, S., Curci, P. L., Zuluaga, D. L., Blanco, E., and Sonnante, G. (2018). Genotyping-by-sequencing highlights patterns of genetic structure and domestication in artichoke and cardoon. PLoS One 13:e0205988. doi: 10.1371/journal.pone.0205988
Pavan, S., Lotti, C., Marcotrigiano, A. R., Mazzeo, R., Bardaro, N., Bracuto, V., et al. (2017). A distinct genetic cluster in cultivated chickpea as revealed by genome-wide marker discovery and genotyping. Plant Genome 10, 1–9. doi: 10.3835/plantgenome2016.11.0115
Pavan, S., Schiavulli, A., Marcotrigiano, A. R., Bardaro, N., Bracuto, V., Ricciardi, F., et al. (2016). Characterization of low-strigolactone germplasm in pea (pisum sativum L.) resistant to crenate broomrape (orobanche crenata forsk.). Mol. Plant Microbe Interact. 29, 743–749. doi: 10.1094/MPMI-07-16-0134-R
Price, A. L., Patterson, N. J., Plenge, R. M., Weinblatt, M. E., Shadick, N. A., and Reich, D. (2006). Principal components analysis corrects for stratification in genome-wide association studies. Nat. Genet. 38, 904–909. doi: 10.1038/ng1847
Price, A. L., Zaitlen, N. A., Reich, D., and Patterson, N. (2010). New approaches to population stratification in genome-wide association studies. Nat. Rev. Genet. 11, 459–463. doi: 10.1038/nrg2813
Pritchard, J. K., Stephens, M., and Donnelly, P. (2000). Inference of population structure using multilocus genotype data. Genetics 155, 945–959. doi: 10.1111/j.1471-8286.2007.01758.x
Purcell, S., Neale, B., Todd-Brown, K., Thomas, L., Ferreira, M. A. R., Bender, D., et al. (2007). PLINK: a tool set for whole-genome association and population-based linkage analyses. Am. J. Hum. Genet. 81, 559–575. doi: 10.1086/519795
Qi, J., Liu, X., Shen, D., Miao, H., Xie, B., Li, X., et al. (2013). A genomic variation map provides insights into the genetic basis of cucumber domestication and diversity. Nat. Genet. 45, 1510–1515. doi: 10.1038/ng.2801
Qin, C., Yu, C., Shen, Y., Fang, X., Chen, L., Min, J., et al. (2014). Whole-genome sequencing of cultivated and wild peppers provides insights into Capsicum domestication and specialization. Proc. Natl. Acad. Sci. U.S.A. 111, 5135–5140. doi: 10.1073/pnas.1400975111
Rasheed, A., Hao, Y., Xia, X., Khan, A., Xu, Y., Varshney, R. K., et al. (2017). Crop breeding chips and genotyping platforms: progress, challenges, and perspectives. Mol. Plant. 10, 1047–1064. doi: 10.1016/j.molp.2017.06.008
Remington, D. L., Thornsberry, J. M., Matsuoka, Y., Wilson, L. M., Whitt, S. R., Doebley, J., et al. (2001). Structure of linkage disequilibrium and phenotypic associations in the maize genome. Proc. Natl. Acad. Sci. U.S.A. 98, 11479–11484. doi: 10.1073/pnas.201394398
Rosyara, U. R., de Jong, W. S., Douches, D. S., and Endelman, J. B. (2016). Software for genome-wide association studies in autopolyploids and its application to potato. Plant Genome. 9, 1–10. doi: 10.3835/plantgenome2015.08.0073
Rousselle, Y., Jones, E., Charcosset, A., Moreau, P., Robbins, K., Stich, B., et al. (2015). Study on essential derivation in maize: III. selection and evaluation of a panel of single nucleotide polymorphism loci for use in European and North American germplasm. Crop Sci 55, 1170–1180. doi: 10.2135/cropsci2014.09.0627
Rubinstein, M., Katzenellenbogen, M., Eshed, R., Rozen, A., Katzir, N., Colle, M., et al. (2015). Ultrahigh-density linkage map for cultivated cucumber (Cucumis sativus L.) using a single-nucleotide polymorphism genotyping array. PLoS One. 10:e0124101. doi: 10.1371/journal.pone.0124101
Ruggieri, V., Francese, G., Sacco, A., D’Alessandro, A., Rigano, M. M., Parisi, M., et al. (2014). An association mapping approach to identify favourable alleles for tomato fruit quality breeding. BMC Plant Biol. 14:337. doi: 10.1186/s12870-014-0337-9
Sasaki, T. (2005). The map-based sequence of the rice genome. Nature 436, 793–800. doi: 10.1038/nature03895
Sato, S., Tabata, S., Hirakawa, H., Asamizu, E., Shirasawa, K., Isobe, S., et al. (2012). The tomato genome sequence provides insights into fleshy fruit evolution. Nature 485, 635–641. doi: 10.1038/nature11119
Scheben, A., Batley, J., and Edwards, D. (2017). Genotyping-by-sequencing approaches to characterize crop genomes: choosing the right tool for the right application. Plant Biotecnol. J. 15, 149–161. doi: 10.1111/pbi.12645
Schmutz, J., Cannon, S. B., Schlueter, J., Ma, J., Mitros, T., Nelson, W., et al. (2010). Genome sequence of the palaeopolyploid soybean. Nature 463, 178–183. doi: 10.1038/nature08670
Schmutz, J., McClean, P. E., Mamidi, S., Wu, G. A., Cannon, S. B., Grimwood, J., et al. (2014). A reference genome for common bean and genome-wide analysis of dual domestications. Nat. Genet. 46, 707–713. doi: 10.1038/ng.3008
Schnable, P. S., Ware, D., Fulton, R. S., Stein, J. C., Wei, F., Pasternak, S., et al. (2009). The B73 maize genome: complexity, diversity, and dynamics. Science 326, 1112–1115. doi: 10.1126/science.1178534
Shang, Y., Ma, Y., Zhou, Y., Zhang, H., Duan, L., Chen, H., et al. (2014). Biosynthesis, regulation, and domestication of bitterness in cucumber. Science 346, 1084–1088. doi: 10.1126/science.1259215
Sim, S.-C., Durstewitz, G., Plieske, J., Wieseke, R., Ganal, M. W., van Deynze, A., et al. (2012). Development of a large snp genotyping array and generation of high-density genetic maps in tomato. PLoS One 7:e40563. doi: 10.1371/journal.pone.0040563
Singh, N., Jayaswal, P. K., Panda, K., Mandal, P., Kumar, V., Singh, B., et al. (2015). Single-copy gene based 50 K SNP chip for genetic studies and molecular breeding in rice. Sci. Rep. 5:11600. doi: 10.1038/srep11600
Siol, M., Jacquin, F., Chabert-Martinello, M., Smıkal, P., Le Paslier, M., Aubert, G., et al. (2017). Patterns of genetic structure and linkage disequilibrium in a large collection of pea germplasm. G3-Genes Genom Genet. 7, 2461–2471. doi: 10.1534/g3.117.043471
Song, Q., Hyten, D. L., Jia, G., Quigley, C. V., Fickus, E. W., Nelson, R. L., et al. (2013). Development and evaluation of SoySNP50K, a high-density genotyping array for soybean. PLoS One 8:e54985. doi: 10.1371/journal.pone.0054985
Song, Q., Jia, G., Hyten, D. L., Jenkins, J., Hwang, E.-Y., Schroeder, S. G., et al. (2015). SNP assay development for linkage map construction, anchoring whole-genome sequence, and other genetic and genomic applications in common bean. G3-Genes Genom Genet. 5, 2285–2290. doi: 10.1534/g3.115.020594
Taranto, F., D’Agostino, N., Greco, B., Cardi, T., and Tripodi, P. (2016). Genome-wide SNP discovery and population structure analysis in pepper (Capsicum annuum) using genotyping by sequencing. BMC Genomics 17:943. doi: 10.1186/s12864-016-3297-7
Taranto, F., D’Agostino, N., Rodriguez, M., Pavan, S., Minervini, A. P., Pecchioni, N., et al. (2020). Whole genome scan reveals molecular signatures of divergence and selection related to important traits in durum wheat germplasm. Front. Genet. 11:217. doi: 10.3389/fgene.2020.00217
Taranto, F., Nicolia, A., Pavan, S., De Vita, P., and D’Agostino, N. (2018). Biotechnological and digital revolution for climate-smart plant breeding. Agronomy 8:277. doi: 10.3390/agronomy8120277
Thomson, M. J., Singh, N., Dwiyanti, M. S., Wang, D. R., Wright, M. H., Agosto Perez, F., et al. (2017). Large-scale deployment of a rice 6 K SNP array for genetics and breeding applications. Rice 10:40. doi: 10.1186/s12284-017-0181-2
Truong, H. T., Ramos, A. M., Yalcin, F., de Ruiter, M., van der Poel, H. J. A., Huvenaars, K. H. J., et al. (2012). Sequence-based genotyping for marker discovery and co-dominant scoring in germplasm and populations. PLoS One 7:e37565. doi: 10.1371/journal.pone.0037565
Turner, S., Armstrong, L. L., Bradford, Y., Carlsony, C. S., Crawford, D. C., Crenshaw, A. T., et al. (2011). Quality control procedures for genome-wide association studies. Curr. Protoc. Hum. Genet. 68, 1.19.1–1.19.18. doi: 10.1002/0471142905.hg0119s68
Unterseer, S., Bauer, E., Haberer, G., Seidel, M., Knaak, C., Ouzunova, M., et al. (2014). A powerful tool for genome analysis in maize: development and evaluation of the high density 600 k SNP genotyping array. BMC Genomics 15:823. doi: 10.1186/1471-2164-15-823
Unver, T., Wu, Z., Sterck, L., Turktas, M., Lohaus, R., Li, Z., et al. (2017). Genome of wild olive and the evolution of oil biosynthesis. Proc. Natl. Acad. Sci. U.S.A. 114, E9413–E9422. doi: 10.1073/pnas.1708621114
Velasco, R., Zharkikh, A., Affourtit, J., Dhingra, A., Cestaro, A., Kalyanaraman, A., et al. (2010). The genome of the domesticated apple (Malus × domestica Borkh.). Nat. Genet. 42, 833–839. doi: 10.1038/ng.654
Verde, I., Abbott, A. G., Scalabrin, S., Jung, S., Shu, S., Marroni, F., et al. (2013). The high-quality draft genome of peach (Prunus persica) identifies unique patterns of genetic diversity, domestication and genome evolution. Nat. Genet. 45, 487–494. doi: 10.1038/ng.2586
Verde, I., Bassil, N., Scalabrin, S., Gilmore, B., Lawley, C. T., Gasic, K., et al. (2012). Development and evaluation of a 9k snp array for peach by internationally coordinated snp detection and validation in breeding germplasm. PLoS One 7:e35668. doi: 10.1371/journal.pone.0035668
Vos, P. G., Paulo, M. J., Voorrips, R. E., Visser, R. G. F., van Eck, H. J., and van Eeuwijk, F. A. (2017). Evaluation of LD decay and various LD-decay estimators in simulated and SNP-array data of tetraploid potato. Theor. Appl. Genet. 130, 123–135. doi: 10.1007/s00122-016-2798-8
Vos, P. G., Uitdewilligen, J. G. A. M. L., Voorrips, R. E., Visser, R. G. F., and van Eck, H. J. (2015). Development and analysis of a 20K SNP array for potato (Solanum tuberosum): an insight into the breeding history. Theor. Appl. Genet. 128, 2387–2401. doi: 10.1007/s00122-015-2593-y
Wang, D., Sun, Y., Stang, P., Berlin, J. A., Wilcox, M. A., and Li, Q. (2009). Comparison of methods for correcting population stratification in a genome-wide association study of rheumatoid arthritis: principal-component analysis versus multidimensional scaling. BMC Proc. 3(Suppl. 7):S109. doi: 10.1186/1753-6561-3-s7-s109
Wang, H., Xu, X., Vieira, F. G., Xiao, Y., Li, Z., Wang, J., et al. (2016). The power of inbreeding: NGS-based GWAS of rice reveals convergent evolution during rice domestication. Mol. Plant. 9, 975–985. doi: 10.1016/j.molp.2016.04.018
Wang, S., Wong, D., Forrest, K., Allen, A., Chao, S., Huang, B. E., et al. (2014). Characterization of polyploid wheat genomic diversity using a high-density 90 000 single nucleotide polymorphism array. Plant Biotechnol. J. 12, 787–796. doi: 10.1111/pbi.12183
Wang, X., Bao, K., Reddy, U. K., Bai, Y., Hammar, S. A., Jiao, C., et al. (2018). The USDA cucumber (Cucumis sativus L.) collection: genetic diversity, population structure, genome-wide association studies, and core collection development. Hortic. Res. 5:64. doi: 10.1038/s41438-018-0080-8
Wen, T., Dai, B., Wang, T., Liu, X., You, C., and Lin, Z. (2019). Genetic variations in plant architecture traits in cotton (Gossypium hirsutum) revealed by a genome-wide association study. Crop J. 7, 209–216. doi: 10.1016/j.cj.2018.12.004
Winfield, M. O., Allen, A. M., Burridge, A. J., Barker, G. L. A., Benbow, H. R., Wilkinson, P. A., et al. (2016). High-density SNP genotyping array for hexaploid wheat and its secondary and tertiary gene pool. Plant Biotechnol. J. 14, 1195–1206. doi: 10.1111/pbi.12485
Xu, C., Ren, Y., Jian, Y., Guo, Z., Zhang, Y., Xie, C., et al. (2017). Development of a maize 55 K SNP array with improved genome coverage for molecular breeding. Mol. Breed. 37:20. doi: 10.1007/s11032-017-0622-z
Xu, X., Pan, S., Cheng, S., Zhang, B., Mu, D., Ni, P., et al. (2011). Genome sequence and analysis of the tuber crop potato. Nature 475, 189–195. doi: 10.1038/nature10158
Yan, J., Shah, T., Warburton, M. L., Buckler, E. S., McMullen, M. D., and Crouch, J. (2009). Genetic characterization and linkage disequilibrium estimation of a global maize collection using SNP markers. PLoS One 4:e8451. doi: 10.1371/journal.pone.0008451
Yano, K., Yamamoto, E., Aya, K., Takeuchi, H., Lo, P.-C., Hu, L., et al. (2016). Genome-wide association study using whole-genome sequencing rapidly identifies new genes influencing agronomic traits in rice. Nat. Genet. 48, 927–934. doi: 10.1038/ng.3596
You, Q., Yang, X., Peng, Z., Xu, L., and Wang, J. (2018). Development and applications of a high throughput genotyping tool for polyploid crops: single nucleotide polymorphism (SNP) array. Front. Plant Sci. 9:104. doi: 10.3389/fpls.2018.00104
Yu, H., Xie, W., Li, J., Zhou, F., and Zhang, Q. (2014). A whole-genome SNP array (RICE6K) for genomic breeding in rice. Plant Biotechnol. J. 12, 28–37. doi: 10.1111/pbi.12113
Yu, J., Pressoir, G., Briggs, W. H., Bi, I. V., Yamasaki, M., Doebley, J. F., et al. (2006). A unified mixed-model method for association mapping that accounts for multiple levels of relatedness. Nat. Genet. 38, 203–208. doi: 10.1038/ng1702
Yu, Y., Fu, J., Xu, Y., Zhang, J., Ren, F., Zhao, H., et al. (2018). Genome re-sequencing reveals the evolutionary history of peach fruit edibility. Nat. Commun. 9:5404. doi: 10.1038/s41467-018-07744-3
Yuan, Y., Wang, X., Wang, L., Xing, H., Wang, Q., Saeed, M., et al. (2018). Genome-wide association study identifies candidate genes related to seed oil composition and protein content in Gmposition and protein content in Gossypium hirsutum L. Front. Plant Sci. 9:1359. doi: 10.3389/fpls.2018.01359
Zhang, S., Chen, X., Lu, C., Ye, J., Zou, M., Lu, K., et al. (2018). Genome-wide association studies of 11 agronomic traits in cassava (Manihot esculenta crantz). Front. Plant Sci. 9, 503. doi: 10.3389/fpls.2018.00503
Zhao, K., Aranzana, M. J., Kim, S., Lister, C., Shindo, C., Tang, C., et al. (2007). An Arabidopsis example of association mapping in structured sample. PLoS Genet. 3:e4. doi: 10.1371/journal.pgen.0030004
Zhao, X., Li, B., Zhang, K., Hu, K., Yi, B., Wen, J., et al. (2016). Breeding signature of combining ability improvement revealed by a genomic variation map from recurrent selection population in Brassica napus. Sci. Rep. 6:29553. doi: 10.1038/srep29553
Keywords: crops, GWAS, genotyping, quality control, bioinformatics tools
Citation: Pavan S, Delvento C, Ricciardi L, Lotti C, Ciani E and D’Agostino N (2020) Recommendations for Choosing the Genotyping Method and Best Practices for Quality Control in Crop Genome-Wide Association Studies. Front. Genet. 11:447. doi: 10.3389/fgene.2020.00447
Received: 19 October 2019; Accepted: 14 April 2020;
Published: 05 June 2020.
Edited by:
Hans D. Daetwyler, La Trobe University, AustraliaReviewed by:
Christian Werner, The University of Edinburgh, United KingdomKai P. Voss-Fels, The University of Queensland, Australia
Copyright © 2020 Pavan, Delvento, Ricciardi, Lotti, Ciani and D’Agostino. This is an open-access article distributed under the terms of the Creative Commons Attribution License (CC BY). The use, distribution or reproduction in other forums is permitted, provided the original author(s) and the copyright owner(s) are credited and that the original publication in this journal is cited, in accordance with accepted academic practice. No use, distribution or reproduction is permitted which does not comply with these terms.
*Correspondence: Stefano Pavan, stefano.pavan@uniba.it; Nunzio D’Agostino, nunzio.dagostino@unina.it