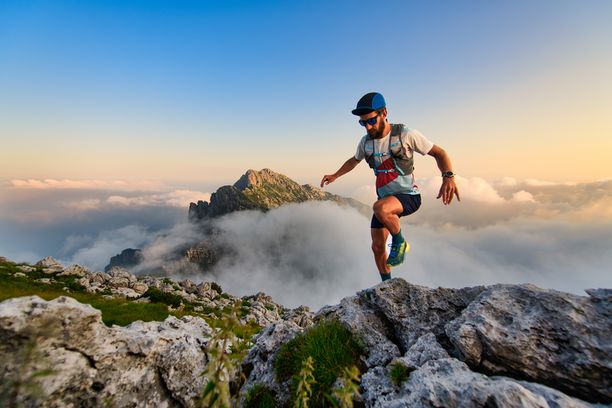
94% of researchers rate our articles as excellent or good
Learn more about the work of our research integrity team to safeguard the quality of each article we publish.
Find out more
ORIGINAL RESEARCH article
Front. Genet., 24 April 2020
Sec. Evolutionary and Genomic Microbiology
Volume 11 - 2020 | https://doi.org/10.3389/fgene.2020.00375
This article is part of the Research TopicGenomic Characterization of Emerging Human Fungal PathogensView all 11 articles
Candida albicans is a normal member of the human microbiome. It is also an opportunistic pathogen, which can cause life-threatening systemic infections in severely immunocompromized individuals. Despite the availability of antifungal drugs, mortality rates of systemic infections are high and new drugs are needed to overcome therapeutic challenges including the emergence of drug resistance. Targeting known disease pathways has been suggested as a promising avenue for the development of new antifungals. However, <30% of C. albicans genes are verified with experimental evidence of a gene product, and the full complement of genes involved in important disease processes is currently unknown. Tools to predict the function of partially or uncharacterized genes and generate testable hypotheses will, therefore, help to identify potential targets for new antifungal development. Here, we employ a network-extracted ontology to leverage publicly available transcriptomics data and identify potential candidate genes involved in disease processes. A subset of these genes has been phenotypically screened using available deletion strains and we present preliminary data that one candidate, PEP8, is involved in hyphal development and immune evasion. This work demonstrates the utility of network-extracted ontologies in predicting gene function to generate testable hypotheses that can be applied to pathogenic systems. This could represent a novel first step to identifying targets for new antifungal therapies.
Candida albicans is the most common cause of nosocomial fungal infections and is particularly important in vulnerable populations with suppressed immune function (Brown et al., 2012b). Mortality rates for systemic candidiasis have been estimated to be as high as 40% (Kullberg and Arendrup, 2015) and antifungal treatments for these invasive infections have only modest success in reducing these high rates (Brown et al., 2012a), despite the introduction of new antifungals, such as the echinocandins (Denning and Bromley, 2015). The discovery and development of new antifungal drugs to treat these life-threatening infections must overcome several challenges including the evolutionary similar fundamental cellular processes of humans and fungi, and the emergence of drug resistance (Denning and Hope, 2010). With high mortality rates, the emergence of drug resistance and the emergence of other pathogenic fungal species, such as Candida auris (Chowdhary et al., 2017), there is a drastic need for methods and targets to aid development of novel antifungal therapies.
The C. albicans community have employed elegant molecular and genetic approaches to identify genes involved in disease processes (Mayer et al., 2013). The key processes involved in disease are well-known and include; adhesion of yeast cells to host cell surfaces, morphological transition from yeast cells to hyphal cells, hyphal invasion by thigmotrophism, endocytosis, release of candidalysin, micronutrient scavenging, stress responses, and biofilm formation (Mayer et al., 2013; Brown A. J. et al., 2014; Brown A. J. P. et al., 2014; Moyes et al., 2016; Noble et al., 2016; Lohse et al., 2018). Indeed, targeting of these processes has been proposed as a promising strategy for antifungal development (Gauwerky et al., 2009; Vila et al., 2017). However, to identify promising targets we need a detailed understanding of these processes and, in many cases, the full complement of genes involved in these pathways is unknown. In fact, ~70% of C. albicans genes are uncharacterized (Candida Genome Database, Nov 2019) and have no experimental evidence for functional annotation (Skrzypek et al., 2016). The bioinformatic prediction of genes involved in known disease processes provides an alternative, complementary approach to potentially time-consuming experimental screens for such genes.
In the last decade, high-throughput RNA sequencing methods have empowered systems-wide studies of cellular organization and function. Methods to identify differential expression of genes (Robinson et al., 2010; Love et al., 2014) and infer gene co-expression networks (Langfelder and Horvath, 2008; Ames et al., 2013; Kramer et al., 2014) aim to predict the function of uncharacterized genes. One such method, Clique Extracted Ontology (CliXO) infers a network-extracted ontology (NeXO) from gene similarity data, such as correlated gene expression (Kramer et al., 2014). An ontology consists of entities, such as groupings or clusters of genes, that are connected by relationships. The structure is a hierarchy and provides several advantages over static network representations, as NeXOs are capable of representing the organization of biological systems on multiple scales such as pathways, complexes, and individual genes as well as capturing pleiotropy. In addition, NeXOs are data-driven and unbiased, enabling de novo modeling of biological processes that can generate predictions about the functions of uncharacterized genes for experimental validation. NeXOs have previously been applied to predict the functions of uncharacterized genes and identify novel functional links in yeast (Dutkowski et al., 2013). More recently, NeXOs have been used to generate hypotheses about autophagy processes implicated in disease (Kramer et al., 2017) and novel, disease-related genes in a prominent fungal phytopathogen (Ames, 2017).
In this study we have assembled publicly available transcriptomics data for C. albicans and use CliXO to infer a C. albicans NeXO that represents pathogenicity without relying on existing annotation. We demonstrate that this network is robust and can recapitulate known biological functions. By overlaying the C. albicans NeXO with data on characterized disease genes, we are able to make predictions about uncharacterized genes involved in various disease processes. These genes have putative roles in adhesion, hyphal development and stress responses. Furthermore, using available deletion strains we are able to phenotypically screen a subset of these candidates. Our data suggest that one such gene, PEP8, might play a role in hyphal development and immune evasion, which has also been suggested by other authors. Ultimately, this study demonstrates the utility of NeXOs to identify functional links between genes that can be used to generate testable hypotheses. This has allowed us to identify a potential role for a partially characterized gene in an important disease process. These tools therefore, may represent an attractive and cost effective approach to identifying virulence targets for new antifungal therapies.
RNA-Seq data for C. albicans grown in a number of conditions, stresses and environments were downloaded from the Gene Expression Omnibus (GEO) database. These data include C. albicans grown in YPD and YPS (GSE41749, Grumaz et al., 2013), in planktonic and biofilm states (GSE45141, Desai et al., 2013), with a range of weak organic acids (GSE49310, Cottier et al., 2015), and in contact with host cells (GSE56091, Liu et al., 2015). In all cases all replicates, treatments and control data for strain SC5314 were used. In total, 130 RNA-Seq samples were used, as maximizing the number of samples used allows us to create the most robust NeXO possible. We note that the majority of samples were taken from an infection-related environment composed of C. albicans cells exposed to endothelial and epithelial cells with RNA taken at 1.5, 5, and 8 h during infection (Liu et al., 2015). Therefore, the NeXO built in this study will predominantly represent C. albicans infection processes related to these cell types and timepoints and is suitable for the application in this study. The addition of more expression data, from varied environments or infection stages, would allow the NeXO to represent a broader range of functions and processes.
Raw data for all publicly available data sets was re-analyzed as experimental setups and conditions may vary between sources. The C. albicans (strain SC5314, assembly 22, haplotype A) reference genome was downloaded from the Candida Genome Database (CGD, Inglis et al., 2012). RNA-Seq reads were aligned to the reference genome using bowtie (Langmead et al., 2009) and bowtie2 (Langmead and Salzberg, 2012) for single-end and paired-end reads, respectively. Alignment was performed using default parameters on a single processor and alignment files were sorted and converted to binary format using samtools (Li et al., 2009). Reads aligned to known genes were counted using the union model with HT-Seq (Anders et al., 2014) and these counts were used to estimate gene expression as reads per kilobase per million mapped reads (RPKM) with edgeR (Robinson et al., 2010). By generating estimates of expression from raw data we ensure that the data is as comparable as possible. Finally, correlations were calculated for all pairs of genes across all samples using the Pearson's correlation coefficient (PCC).
CliXO was used to create a C. albicans NeXO from correlations in gene expression (Kramer et al., 2014). CliXO requires a user-defined noise parameter α and a parameter to infer missing edges β. CliXO has been benchmarked on several 'omics data sets for the yeast Saccharomyces cerevisiae, including gene expression profile correlations, and it has been shown that α = 0.01 and β = 0.5 produces ontologies that are very similar to that of the Gene Ontology (GO) (Kramer et al., 2014). Therefore, in this study α and β were set to 0.01 and 0.5, respectively. Varying these parameters produces similar NeXO structures. Altering the α parameter to α = 0.02 produces a NeXO structure where entities have an average similarity to the reference C. albicans NeXO of 0.66 ± 0.28 (max 1.0) using the alignment and similarity metrics of Kramer et al. (2014) with a the strict hierarchy model. Varying the β parameter to β = 0.4 and β = 0.6 also produced a similar NeXO structure with average entity similarity of 0.83 ± 0.22 and 0.75 ± 0.23, respectively.
The Gene Ontology (GO) structure (OBO file) and annotations for C. albicans were downloaded from geneontology.org. To prepare the GO for alignment to the C. albicans NeXO, the structure of the GO was extracted using “is_a” GO relations, terms with no annotations to known C. albicans genes were removed, and redundant terms were removed. Redundant terms were defined as terms that share the same gene content as their direct descendants. When redundant terms were identified the more general term, i.e., the parent, was removed. After processing of the GO there were 776, 1,919, and 3,804 terms in the Cellular Component, Molecular Function, and Biological Process ontologies, respectively. The method of Kramer et al. (2014) was used to align the C. albicans NeXO to the GO ontologies using the strict hierarchy model and to generate an alignment for all nodes.
The C. albicans NeXO is split into a number of entities with hierarchical relationships between them. As these entities will represent biological pathways and processes it was important to estimate the robustness of the entities with regard to the underlying experimental data used to construct the NeXO. To score the robustness of each term a measure of entity quality that considers the network support of a term and its robustness to perturbations of input data was taken from Dutkowski et al. (2013). In this measure, network support NS(e) for an entity e is defined as the enrichment for co-expression between genes within an entity where co-expression is defined as PCC > 0.2. Enrichment [-log(P-value)] is estimated using the hypergeometric distribution where the sample size is equal to all possible co-expressed genes in an entity, sample successes are the observed co-expressed genes in an entity, the population size is all possible co-expressed genes in the NeXO, and population successes are the observed co-expressed genes in the NeXO.
To quantify an entity's robustness to changes in the input data, a bootstrapping approach was employed. Samples were randomly selected with replacement to build an expression set of 130 samples. Correlations for all pairs of genes were calculated and a NeXO was inferred as described above. This process was repeated to produce 100 bootstrapped ontologies. Each bootstrapped NeXO was then aligned to the original NeXO using the method described in Kramer et al. (2014). Alignment was performed using the strict hierarchy model and all nodes were aligned. The bootstrap score B(e) for an entity (e) was then calculated as:
where n is the number of bootstrapped NeXOs and Si is the alignment score for entity e when the original NeXO is aligned to the i-th bootstrapped NeXO. Finally, the robustness score for each entity was calculated as a geometric mean of the entity's network support and bootstrap score:
Entities, clusters of genes identified from their correlated expression profiles, potentially important for disease, were identified as those containing more than two members and that show enrichment for genes implicated in disease. Two sources were used to annotate disease genes; (i) 167 disease genes identified from the pathogen-host interaction database (Winnenburg et al., 2006) and (ii) 255 genes that produce secreted and cell wall proteins thought to be associated with disease were taken from Butler et al. (2009). Fisher's exact test was used to identify enrichment by comparing the proportion of genes within each entity associated with disease to the proportion of genes in the whole NeXO associated with disease. The method of Benjamini and Hochberg (1995) was used to control for a false discovery rate of 0.05.
Entities within the NeXO were annotated by identifying enriched GO terms (Ashburner et al., 2000). The GO slim ontology and annotations were downloaded from the CGD and Fisher's exact test was used to identify enriched GO terms in each entity. Briefly, for each entity each annotated GO term was tested for enrichment where the population size was the number of genes in the C. albicans NeXO and the sample size was the size of the entity. The population successes are the number of genes in the C. albicans NeXO (i.e., whole network) annotated with the specific GO term and the sample successes are the number of genes within the entity (i.e., a single cluster) annotated with that term. The method of Benjamini and Hochberg (1995) was used to control for a false discovery rate of 0.05.
Homozygous deletion strains for genes identified in entities associated with disease were assembled from Rauceo et al. (2008) and Noble et al. (2010) in VB GKO plate 1 obtained from the Fungal Genetics Stock Center (McCluskey et al., 2010). A full list of strains used in this study can be found in Supplementary File 1. Strains were stored at −80°C in 96-well plates, replicated on agar plates and subjected to a variety of phenotypic assays including; (i) thermotolerance on YPD at 25, 30, 37, and 42°C, (ii) osmotic stress with YPD+NaCl and YPD+KCl at 30°C at both 0.5 and 1 M; (iii) YPD+H2O2 at 30°C with concentrations of 2.5, 5, 7.5, and 10 mM; (iv) acidic environments from pH 4.0 to pH 7.0 at both 30 and 37°C; and (v) YPD+Fluconazole and YPD+Caspofungin at 30°C with concentrations of 1, 2 μg/ml and 0.064 and 0.128 μg/ml, respectively. Strains were incubated under these conditions for 48 h.
Hyphal growth was assessed by first examining colony morphology on agar plates containing YPD+20% serum and in Spider medium incubated at 30 and 37°C for 48 h (Liu et al., 1994). Second, overnight cultures were diluted to OD600, incubated in a shaking incubator in liquid YPD containing 20% serum at 37°C and cells were fixed in 10% formalin after 90 m so that hyphal growth could be observed by microscopy.
Bone marrow was harvested from three eight-week old male C57BL/6 mice, from which bone marrow derived macrophages (BMDMs) were differentiated, as described previously (Davies and Gordon, 2005). BMDMs were transferred to a 96-well plate at 2.4 ×104 cells per well. C. albicans cells were grown overnight in YPD and then washed three times in phosphate-buffered saline (PBS) and resuspended in 1 ml PBS. Following counting, 7.2 ×104 of C. albicans cells were added to each well, ensuring a 3:1 ratio of yeast to macrophage. YOYO-1 dye (150 μl) was then added to each well. Fluorescence arising from lysed macrophages was measured at 30 m intervals for 12 h in a FLUOstar Optima plate reader (BMG Labtech) using excitation 485 nm/emission 520 nm at 37°C (Bain et al., 2014).
BMDMs were prepared from three 8-week old male C57BL/6 mice, which were selected randomly, bred in-house, and housed in stock cages under specific pathogen-free conditions. The mice did not undergo surgical procedures prior to culling by cervical dislocation. All animal experimentation was approved by the UK Home Office and by the University of Aberdeen Animal Welfare and Ethical Review Body.
Expression of C. albicans genes from different growth states, environments, stresses, and infection models representing 130 individual samples were used to characterize the C. albicans system. Correlated gene expression profiles for 5,698 C. albicans genes were used to infer a network-extracted ontology (NeXO) with the CliXO method (Kramer et al., 2014). The resulting NeXO consists of 1,366 entities connected by 1,801 relationships where larger entities split into smaller subsets representing functions on multiple scales. The C. albicans NeXO structure can be found in Supplementary File 2.
Aligning this C. albicans NeXO to the GO shows that 1,033/1,366 (75.6%) entities can be aligned to at least one ontology within the GO, with many terms mapping to more than one (Figure 1). Of the 496 entities that contain more than 4 genes, 336 can be aligned to GO terms. The C. albicans NeXO captures 70.6% of terms in the Cellular Component ontology but only captures 18.1 and 20.9% of terms in the Biological Process and Molecular Function ontologies, respectively (Supplementary File 3). This suggests that the C. albicans NeXO largely describes subcellular structures and macromolecular complexes.
Figure 1. Venn diagram showing the number of entities in the C. albicans NeXO (white) that align to Biological Process (BP, red), Molecular Function (MF, green) or Cellular Component (CC, blue) GO ontologies.
Aligning the C. albicans NeXO to the GO provides useful information about the similarity between the structure of the two ontologies. To gain more information about the function, or multiple functions, of entities within the C. albicans NeXO, enriched GO terms for each entity were identified using Fisher's Exact Test (adjusted P <0.05) and used as a functional annotation. There are 333/1,366 (24.3%) entities with significant GO term enrichment and 159/496 (32.0%) entities with significant enrichment when examining entities with greater than four genes. GO term enrichment covers terms from all three GO ontologies with 35, 20, and 23 unique GOslim terms enriched from the biological process, molecular function, and cellular component ontologies, respectively (Supplementary File 4). This suggests that our C. albicans NeXO focusses on a subset of functions across all GO ontologies with many entities showing no functional enrichment. These may represent noise in NeXO generation or the identification of currently unknown or unannotated pathways and functions.
To validate the C. albicans NeXO, robustness scores for each entity were calculated based on network support from co-expression and bootstrapping of the input expression data. We find a strong correlation between term size and robustness with larger terms being more robust (R2 = 0.87, P = <0.0001 on log transformed data, Figure S1). Therefore, the larger entities in the NeXO are more robust. Excluding entities with fewer than 10 members yields a mean robustness score of 4.47 compared to 0.82 when all entities are analyzed. There were two clear outliers: these were two entities immediately under the root, which both contain >5,400 genes and are far larger than any other entities in the NeXO (Figure S1), suggesting that these may be spurious entities. Overall, entities within the C. albicans NeXO, particularly larger entities, are robust to the underlying data suggesting that the addition or removal of data wouldn't change their composition and so they are likely to represent biological functions.
Although many entities in the NeXO were annotated by identifying enriched GO terms, many remain unannotated (Figure 1). The unannotated entities may represent understudied, functionally-related genes with roles in pathogenicity that are lacking GO annotation. To identify entities related to pathogenesis irrespective of GO annotation, we identified entities enriched for known disease genes in the pathogen-host interaction database (Winnenburg et al., 2006) (Figure 2). This is a major advantage of using a data-driven NeXO that can complement existing GO annotation but can also discover new entities with roles in specific biological processes.
Figure 2. (A) The C. albicans co-expression network represented as nodes (genes) connected by edges where genes show correlated expression profiles with Pearson's Correlation Coefficient > 0.4. Entities enriched for disease genes, described in Table 1, are highlighted by colored circles and the genes that are part of these entities are also highlighted. (B) A network-extracted ontology (NeXO) inferred from the co-expression data, organized into entities (nodes) of genes and connected by relationships (edges). Node sizes represent the number of genes associated with each entity and only entities associated with 10 or more genes are shown. Node colors represent the robustness of the entities calculated by bootstrap analysis, where darker colors indicate a higher robustness score. Entities enriched for disease genes, described in Table 1, are highlighted by colored circles.
Table 1. Co-expression entities enriched for known disease genes in the pathogen-host interaction database.
Eight entities within the C. albicans NeXO are enriched for known disease genes. Six of these eight entities are also enriched for the GO term “pathogenesis” (GO:0009405) indicating that the genes in these entities are related to disease. Additionally, several other terms related to C. albicans disease processes are also enriched in these entities. GO terms “interspecies interaction between organisms” (GO:0044419), “filamentous growth” (GO:0030447), and “growth of unicellular organism as a thread of attached cells” (GO:0070783) are all regularly enriched in putative disease-related entities. However, entities CLX:6939 and CLX:6889 show no GO enrichment and may represent areas of unstudied function associated with disease that have only been detected using this approach.
In those entities that are enriched for known disease genes, many other genes are uncharacterized and could potentially be involved in disease processes. In this way we can predict functional annotations for genes not present in literature-curated sources such as the GO. The entity CLX:6916 (Figure 3), contains 24 genes with 5 genes annotated as being part of the disease process in the pathogen-host interaction database (Winnenburg et al., 2006). Of the other 19 genes in this entity 6 genes have experimental annotation in the CGD and the remaining 11 genes are uncharacterized. CLX:6916 is a highly connected entity with 189 edges with a Pearson correlation coefficient >0.2, suggesting that the genes in this entity may be functionally related. The entity CLX:6916 contains genes involved in the disease process. These include RCN1 (C6_01160W), which encodes a calcineurin-dependent signaling protein that has a role in controlling the stress response and virulence (Reedy et al., 2010). The genes VPS24 (C2_00930C) and C6_02690C have been linked to the processes of adherence Marchais et al. (2005) and hyphal growth (Bensen et al., 2004), respectively. Also, the entity CLX:6857 (Figure S2) contains the gene TRY5 (C6_01500C), which acts as a regulator of adhesion genes (Finkel et al., 2012). These entities display tight clustering suggesting that the genes they contain are functionally related. Since these entities are enriched for known disease genes, we reasoned that the uncharacterized genes in these entities may also play roles in disease and C. albicans pathogenicity.
Figure 3. Entity CLX:6916 in the C. albicans NeXO is enriched for known disease genes in the pathogen-host interaction database (Winnenburg et al., 2006). The hierarchical structure shows CLX:6916 connected by edges to sub-entities (circles), and the genes (rounded rectangles) contained within the entity. Entities that are aligned to the Gene Ontology are shown in blue. Genes found in the pathogen-host interaction database are highlighted in green. Enriched Gene Ontology terms are also shown.
Entity CLX:6889 (Figure 4) shows enrichment for known disease genes but no significant enrichment for any GO terms. CLX:6889 may represent an understudied process, detectable directly from transcriptomic data using the NeXO approach, where incorrect or missing GO term annotation means we cannot detect any significant GO term enrichment or existing functional annotation. Interestingly, CLX:6939 (Figure S3) shows no GO term enrichment. However, its descendent entities, CLX:6819 and CLX:6036, are enriched for multiple GO terms. Possible interpretations again include the possibility of missing GO term annotation, or that CLX:6939 represents multiple disease-related processes with no coherent GO description while the descendent entities CLX:6819 and CLX:6036 represent singular functions with adequate GO descriptions. In these entities we find that the majority of genes annotated with GO terms have been annotated on the basis of sequence similarity or inferred from electronic annotation, with few terms annotated by mutant phenotypes or from direct assays. This suggests that the GO annotations for these genes may be inaccurate or incomplete. Indeed, ~70% of C. albicans genes are uncharacterized (Candida Genome Database, Nov 2019) and have no experimental evidence for functional annotation (Skrzypek et al., 2016). Together, the enrichment of known disease genes in this entity and the lack of experimental annotation of GO terms, may suggest that these entities, and their genes, represent coherent, but under-characterized, functional units related to disease.
Figure 4. Entity CLX:6889 in the C. albicans NeXO is enriched for known disease genes in the pathogen-host interaction database (Winnenburg et al., 2006). The hierarchical structure shows CLX:6889 connected by edges to sub-entities (circles), and the genes (rounded rectangles) contained within the entity. Entities that are aligned to the Gene Ontology are shown in blue. Genes found in the pathogen-host interaction database are highlighted in green. Enriched Gene Ontology terms are also shown.
The entity CLX:6889 (Figure 4) contains characterized genes involved in biofilm production (MRPL33—C3_01080W) (Nett et al., 2009) and response to mouse macrophages (RPS14B—C1_06450C) (Lorenz et al., 2004). Both of these genes have not been shown to contribute to the disease process, but have functions associated with disease. CLX:6889 also contains uncharacterized genes that are induced in response to stress (C5_04300C), involved in protein secretion (C5_01900C) and involved in the response to macrophages (THS1) (Nobile et al., 2012). These have inferred functions potentially related to disease. These entities suggest that some genes, that may have been partially characterized or annotated with GO terms, might yet have undiscovered functions involved in the disease process, which might be niche-specific, for example.
To test whether some partially characterized genes might have undiscovered functions involved in the disease process, we selected available, homozygous null mutants corresponding to genes associated with disease-related entities in the NeXO (AHR1, WOR2, CST20, GRR1, HGC1, HOG1, HST7, HWP1, INT1, PBS2, PEP8, RIM101, RSR1, SEF1, TRY5 HIT1, NVL6) and irrespective of GO annotation. The mutants were subjected to a range of phenotypic assays alongside their respective control strains (CAI4+Clp10, RM1000+Clp20, SN250). These virulence-related assays included yeast-hypha morphogenesis, macrophage phagocytosis, thermotolerance, resistance to osmotic, oxidative and acid stresses, and sensitivity to the antifungal drugs, fluconazole, and caspofungin (Figure S4).
There were detectable phenotypes for mutant strains in a range of conditions. Several mutants (HIT1, NVL6, HOG1, PBS2) showed differing levels of sensitivity to YPD+H2O2, particularly at concentrations of 7.5 and 10 mM. We also observed potential reduced growth of hit1Δ when exposed to fluconazole and rsr1Δ when treated with caspofungin. Finally, pep8Δ, showed an aberrant colony morphology on Spider medium (Figure 5). Whilst the control strain, SN250, displayed the distinctive wrinkly appearance associated with hyphal development, pep8Δ colonies were smooth, similar to the appearance of hwp1Δ colonies with known hyphal abnormalities, suggesting a potential defect in hyphal development (Figure S4).
Figure 5. (A) Images of colony formation for wild-type (SN250) and pep8 mutants grown on Spider media at 37°C for 48 h. (B) Evidence of reduced hyphal formation by pep8Δ cells compared to wild-type cells fixed in formalin at 90 m hyphal development.
To explore the potential defect of pep8Δ cells in hyphal development, we compared their morphology to control cells (SN250) after growth in YPD containing 20% serum at 37°C (Figure 5B). The pep8Δ cells formed stunted hyphae compared to the control. This confirmed that the loss of Pep8 either delays or stunts hyphal development in C. albicans, suggesting that Pep8 plays a role in hyphal development.
The formation of hyphae after phagocytosis is known to promote the escape of C. albicans cells from innate immune cells (Lo et al., 1997; McKenzie et al., 2010). Therefore, we reasoned that, given their attenuated hypha formation, pep8Δ cells might be less able to lyse and escape macrophages following phagocytosis. We tested this by comparing the ability of C. albicans strains to promote the release of the fluorescent dye, YOYO-1, from macrophages (Figure 6). Macrophages infected with wild type C. albicans control strains (SN250 or RM1000+CIp20) released significant amounts of the dye over the time course, whereas uninfected macrophages did not. Also, macrophages infected with the hypha-defective C. albicans cph1Δ efg1Δ mutant did not display significant levels of lysis (Figure 6), which was consistent with the role that hypha formation plays in fungal escape from macrophages (Lo et al., 1997; McKenzie et al., 2010). Significantly, the pep8Δ cells displayed a reduced ability to lyse the macrophages compared to the wild-type controls RM1000 (Mann–Whitney U, P = 5.875e-11) and SN250 (Mann–Whitney U, P = 0.002). However, the pep8Δ cells were able to escape these innate immune cells to some degree (Figure 6). This was entirely consistent with our observation that loss of Pep8 partially attenuates, but does not completely block, hypha formation (Figure 6). We conclude that Pep8 plays a role in hyphal development and defects in this gene's function affect the ability of C. albicans to evade the host immune system.
Figure 6. The lysis of macrophages was assayed by measuring release of the fluorescent dye, YOYO-1 (section Materials and Methods). The pep8Δ mutant (dark blue) displays a reduced ability to lyse and escape macrophages compared to the control wild-type strains RM1000 (green; Mann–Whitney U, ***P = 5.875e-11) and SN250 (brown; Mann–Whitney U, **P = 0.002). Uninfected macrophages (light blue, Control 1; purple, Control 2), and a hypha-deficient cph1Δ efg1Δ mutant (Lo et al., 1997) (red, Ca80) were also included as controls.
Ontologies extracted from network data have been used to predict novel, disease-related genes in an important phytopathogen (Ames, 2017) and identify the function of uncharacterized genes that can be experimentally validated (Dutkowski et al., 2013). In this study, we compiled RNA-Seq data for the human fungal pathogen C. albicans, inferred a NeXO from correlated expression profiles, and overlaid information on known disease genes to make functional predictions for poorly- and un-characterized genes. A subset of these predictions were then tested using phenotypic screens and infection assays to identify potentially novel disease-related genes.
NeXOs have been proposed as useful tools to predict a range of cellular phenotypes and answer important biological questions (Carvunis and Ideker, 2014). These network structures have proven to be robust (Dutkowski et al., 2013), as is the C. albicans NeXO (Figure S1). NeXOs also recapitulate known functions; a NeXO built from a range of interaction data for S. cerevisiae aligns to many GO categories and best represents the Cellular Component ontology (Dutkowski et al., 2013). The same pattern can be seen in this study with many entities aligning to GO terms and best representing the Cellular Component ontology (Figure 1). Previous work looking at functional modules identified from a yeast co-expression network shows that many (>80%) Biological Process and Cellular Component terms can be represented by co-expression data (Ames et al., 2013). These results therefore suggest that the structure of NeXOs may be well suited to representing Cellular Component terms. To better represent biological processes and molecular functions, methods of generating NeXOs may have to take into account functional links between genes whose expression could be dispersed over time. Indeed, sequential activation of functionally related genes has been demonstrated during differentiation and development (Queva et al., 1998), cell wall stress in S. cerevisiae (Bermejo et al., 2008) and C. albicans-host interactions (Wilson et al., 2009).
Based on location in a NeXO and functions of characterized genes in the same entity, it is possible to generate hypotheses about the functions of poorly- or un-characterized genes. Here, we used enrichment of disease-associated genes in entities as evidence that these entities may represent disease related pathways containing as yet unknown disease genes. CLX:6916 (Figure 3) is enriched for disease-associated genes with virulence related functions, RSR1 (CR_02140W) guides hyphal growth (Hausauer et al., 2005), IRS4 (C3_03660W) contributes to hyphal formation (Badrane et al., 2005), HGC1 (C1_00780C) regulates hyphal morphogenesis (Zheng et al., 2004), CDC11 (C5_00070W) is also involved in hyphal morphology (Warenda et al., 2003) and HWP1 (C4_03570W) encodes a major protein in the cell wall and functions in cell-surface adhesion (Staab and Sundstrom, 1998; Staab et al., 2004). This entity also contains several characterized genes that have implicated roles in disease but are not present in the pathogen-host interaction database. GRR1 (C5_04600C) is required for cell cycle progression and involved in the negative control of pseudohyphal growth (Butler et al., 2006) and RCN1 (C6_01160W) is a calcineurin-dependent signaling protein that controls stress response and virulence (Reedy et al., 2010). The identification of entities containing known disease genes not found in the pathogen-host interaction database highlights the reliability of these methods and also the usefulness of using pre-existing databases without the need to include expert or literature-curated knowledge. There are also several partially or uncharacterized genes, that have been linked to the disease process. For example PEP8, a partially characterized gene, has previously been associated with filamentation in a large-scale haploinsufficiency assay (Uhl et al., 2003).
A homozygous pep8Δ/pep8Δ deletion strain was included in the strains phenotypically assayed in this study. We find that pep8Δ cells produce delayed or stunted hyphae and show attenuated macrophage escape (Figures 5, 6), confirming a role for this gene in filamentation as previously reported (Uhl et al., 2003) and suggesting a role in infection. This finding also confirms recent work, which is entirely independent from this study, that has shown that pep8 mutants demonstrate severe filamentation defects in a range of conditions (Azadmanesh et al., 2017) and these mutants abrogate azole resistance (Mount et al., 2018). Loss of function of Pep8 abrogates azole resistance by overwhelming the functional capacity of calcineurin. Interestingly, PEP8 is clustered with RCN1, which encodes a calcineurin-dependent signaling protein, among other genes involved in filamentation and drug resistance. We note that not all the strains phenotypically tested appear to have roles in virulence-related processes with the recall and precision of these approaches estimated to be 0.43 and 0.31, respectively (Yu et al., 2019). However, given that NeXOs are data-driven and can be generated quickly with little expert knowledge or human intervention, they provide useful approaches for the identification of novel pathways, prediction of functions and generation of testable hypotheses, especially in those organisms that lack comprehensive annotation.
This work also demonstrates the utility of NeXOs to reveal functions at multiple levels. Entities CLX:6916 (Figure 3), CLX:6088 (Figure S2) and CLX:7006 all contain the hyphal cell wall protein Hwp1. This indicates that the C. albicans NeXO is capturing function at different levels and may indicate a role for Hwp1 in several disease related pathways. CLX:6088 and CLX:7006 both seem to be specifically associated with cell adhesion based on alignment to the GO and enrichment for GO terms, respectively (Supplementary Files 3, 4). These entities are children of the much larger entity CLX:7055 (2,764 members), that shows enrichment for multiple disease-related GO terms including “pathogenesis,” “cell adhesion,” and “biofilm formation,” and may represent a higher, or more general, description of pathogenicity. CLX:6916 appears to be associated with yeast-hypha morphogenesis and hypha function. The entity is enriched for terms relating to “filamentous growth” (GO:0030447), “site of polarized growth” (GO:0030427) and “pseudohyphal growth” (GO:0007124). The presence of Hwp1 in entities related to adhesion and hyphal growth tallies perfectly with the known presence of Hwp1 on the surface of hyphal cells and the protein's role in adhesion (Staab and Sundstrom, 1998; Staab et al., 1999). The ontological view allows us to identify the importance of Hwp1 in multiple-related processes that are important for pathogenesis. This is a major advantage of organizing these data into a NeXO to identify relations between entities, highlight genes present in multiple pathways or processes and find entities that represent function on different scales (Dutkowski et al., 2013).
NeXOs may also provide a tool for the functional annotation of poorly characterized genes (Dutkowski et al., 2013). The entity, CLX:6889 (Figure 4), shows an enrichment of disease-associated genes, but does not align to any term in the GO and has no enrichment for GO terms despite all genes having GO annotations. Although descendants of CLX:6889 do align to the GO and CLX:6514 is enriched for the GO term for pathogenesis (GO:0009405). The members of CLX:6889 include: HOG1 (C2_03330C) which encodes the stress activated protein kinase involved in the core stress response (Alonso-Monge et al., 2009), MLT1 (C1_08210C) a vacuolar membrane transporter that is needed for virulence (Theiss et al., 2002), MNT2 (C3_01830C) a transferase with a role in adherence and virulence (Munro et al., 2005), and MDS3 (C3_07320W) encoding a TOR signaling pathway component required for growth and hyphal formation (Davis et al., 2002; Richard et al., 2005). Other genes in this entity, both verified and uncharacterized, have roles that can be linked to disease processes. For example, a putative metallodipeptidase (C5_04300C) is a target gene of a small network of transcription regulators that control biofilm development (Nobile et al., 2012) and is targeted for sumoylation, where sumoylation targets have been shown to have roles in cell cycle progression and stress responses (Leach et al., 2011). The genes in this entity have varied roles and seem loosely to be related to growth and its regulation. Although this entity might be produced by a random clustering of genes, the enrichment for disease-associated genes and functions assigned to the entity's descendents suggests CLX:6889 may represent a true functional module related to disease not described by current GO annotations. Indeed, most of the GO annotations for genes in this entity are inferred from electronic annotation rather than direct experimental evidence and previous work using network extracted ontologies has shown that entities that do not align to the GO can represent true biological modules (Dutkowski et al., 2013). The genes in this entity therefore, may represent new targets for functional characterization related to pathogenesis.
In this study, we use a C. albicans NeXO to generate testable hypotheses about this important fungal pathogen. It is important to note that the underlying data used to infer NeXOs is crucial to the types of questions that can be answered. The C. albicans NeXO, leverages publicly available data and is largely constructed from infection-related data (see section Materials and Methods), making it well suited to generating hypotheses about infection-related processes. This may be why the NeXO is useful to identify groups of genes related to hyphal development and similar pathogenic processes. However, we note that other processes are clearly represented by the C. albicans NeXO, with 24% of entities enriched for GO terms across all 3 Gene Ontologies and only 2~% of entities enriched with the “pathogenesis” GO term. Previous studies have used NeXOs to describe broad functional categories for the yeast S. cerevisiae and generate hypotheses about the function of uncharacterized genes (Dutkowski et al., 2013). This was made possible by utilizing integrated data from protein-protein interactions, gene co-expression and genetic interactions, which represent varied areas of biological function (Ames et al., 2013). Therefore, the addition of further data, both for different infection scenarios and environments, would produce a C. albicans NeXO that represents more varied areas of biological function and may well increase alignment to the GO. Likewise, the addition of known disease genes from the literature to supplement the pathogen-host interaction database would increase our set of known disease genes and may well allow the identification of further disease processes in the NeXO. Nevertheless, the data used in this study have been able to recapitulate known functions (Figure 1) and generate hypotheses for the role of several uncharacterized genes in disease processes (Figures 3, 4, Figures S2, S3). Even though only a handful of genes predicted to be involved in disease were available from the FGSC, we were still able to identify PEP8 as having a potential role in hyphal development and immune system evasion.
Here, we have built a NeXO for C. albicans, leveraging publicly available data, to identify novel gene networks involved in pathogenicity. We have shown that the NeXO is robust and recapitulates known biology by aligning to the GO. By identifying enrichment of known disease genes in entities we make predictions about pathways and partially or uncharacterized genes potentially involved in pathogenicity. One such gene, PEP8, is shown to produce stunted or delayed hyphae and attenuated immune system evasion. This work, along with that of others, suggests further characterization of PEP8 would be important for a more detailed understanding of infection processes. This study, therefore, shows the utility of NeXOs for pathway identification, functional annotation, and hypothesis generation that can be applied to multiple systems and processes.
The publicly-available datasets analyzed for this study can be found in the Gene Expression Omnibus (IDs: GSE41749, GSE45141, GSE49310, GSE56091). The network-extracted ontology generated as part of this study is available in the Supplementary Information.
RA and AB contributed conception and design of the study. RA performed the computation analysis and wrote the first draft of the manuscript. RA, JB, and SB performed the experimental analysis. GT, JB, and AB wrote sections of the manuscript. All authors contributed to manuscript revision, read, and approved the submitted version.
RA was generously supported by a Wellcome Trust Institutional Strategic Support Award [WT105618MA], a Microbiology Research Visit Grant [RVG16/18], and a EPSRC/BBSRC Innovation Fellowship [EP/S001352/1]. AB was supported by a programme grant from the UK Medical Research Council [MR/M026663/1] and by the Medical Research Council Centre for Medical Mycology at the University of Aberdeen [MR/N006364/1]. The funders had no role in study design, data collection and analysis, decision to publish, or preparation of the manuscript.
The authors declare that the research was conducted in the absence of any commercial or financial relationships that could be construed as a potential conflict of interest.
This is a short text to acknowledge the contributions of specific colleagues, institutions, or agencies that aided the efforts of the authors.
The Supplementary Material for this article can be found online at: https://www.frontiersin.org/articles/10.3389/fgene.2020.00375/full#supplementary-material
Alonso-Monge, R., Carvaihlo, S., Nombela, C., Rial, E., and Pla, J. (2009). The Hog1 MAP kinase controls respiratory metabolism in the fungal pathogen Candida albicans. Microbiology 155, 413–423. doi: 10.1099/mic.0.023309-0
Ames, R. M. (2017). Using network extracted ontologies to identify novel genes with roles in appressorium development in the rice blast fungus Magnaporthe oryzae. Microorganisms 5:3. doi: 10.3390/microorganisms5010003
Ames, R. M., MacPherson, J. I., Pinney, J. W., Lovell, S. C., and Robertson, D. L. (2013). Modular biological function is most effectively captured by combining molecular interaction data types. PLoS ONE 8:e62670. doi: 10.1371/journal.pone.0062670
Anders, S., Pyl, P. T., and Huber, W. (2014). HTSeq–a python framework to work with high-throughput sequencing data. Bioinformatics 31, 166–169. doi: 10.1093/bioinformatics/btu638
Ashburner, M., Ball, C., Blake, J., Botstein, D., Butler, H., Cherry, J., et al. (2000). Gene ontology: tool for the unification of biology. The Gene Ontology Consortium. Nat. Genet. 25, 25–29. doi: 10.1038/75556
Azadmanesh, J., Gowen, A. M., Creger, P. E., Schafer, N. D., and Blankenship, J. R. (2017). Filamentation involves two overlapping, but distinct, programs of filamentation in the pathogenic fungus Candida albicans. G3 7, 3797–3808. doi: 10.1534/g3.117.300224
Badrane, H., Cheng, S., Nguyen, M. H., Jia, H. Y., Zhang, Z., Weisner, N., et al. (2005). Candida albicans IRS4 contributes to hyphal formation and virulence after the initial stages of disseminated candidiasis. Microbiology 151, 2923–2931. doi: 10.1099/mic.0.27998-0
Bain, J. M., Louw, J., Lewis, L. E., Okai, B., Walls, C. A., Ballou, E. R., et al. (2014). Candida albicans hypha formation and mannan masking of beta-glucan inhibit macrophage phagosome maturation. MBio 5:e01874. doi: 10.1128/mBio.01874-14
Benjamini, Y., and Hochberg, Y. (1995). Controlling the false discovery rate: a practical and powerful approach to multiple testing. J. R. Stat. Soc. Ser. B 57, 289–300. doi: 10.1111/j.2517-6161.1995.tb02031.x
Bensen, E. S., Martin, S. J., Li, M., Berman, J., and Davis, D. A. (2004). Transcriptional profiling in Candida albicans reveals new adaptive responses to extracellular pH and functions for Rim101p. Mol. Microbiol. 54, 1335–1351. doi: 10.1111/j.1365-2958.2004.04350.x
Bermejo, C., Rodríguez, E., García, R., Rodríguez-Peña, J. M., de la Concepción, M. L. R., Rivas, C., et al. (2008). The sequential activation of the yeast HOG and SLT2 pathways is required for cell survival to cell wall stress. Mol. Biol. Cell 19, 1113–1124. doi: 10.1091/mbc.e07-08-0742
Brown, A. J., Brown, G. D., Netea, M. G., and Gow, N. A. (2014). Metabolism impacts upon candida immunogenicity and pathogenicity at multiple levels. Trends Microbiol. 22, 614–622. doi: 10.1016/j.tim.2014.07.001
Brown, A. J. P., Budge, S., Kaloriti, D., Tillmann, A., Jacobsen, M. D., Yin, Z., et al. (2014). Stress adaptation in a pathogenic fungus. J. Exp. Biol. 217, 144–155. doi: 10.1242/jeb.088930
Brown, G. D., Denning, D. W., Gow, N. A., Levitz, S. M., Netea, M. G., and White, T. C. (2012a). Hidden killers: human fungal infections. Sci. Transl. Med. 4:165rv13. doi: 10.1126/scitranslmed.3004404
Brown, G. D., Denning, D. W., and Levitz, S. M. (2012b). Tackling human fungal infections. Science 336, 647–647. doi: 10.1126/science.1222236
Butler, D. K., All, O., Goffena, J., Loveless, T., Wilson, T., and Toenjes, K. A. (2006). The GRR1 gene of Candida albicans is involved in the negative control of pseudohyphal morphogenesis. Fungal Genet. Biol. 43, 573–582. doi: 10.1016/j.fgb.2006.03.004
Butler, G., Rasmussen, M. D., Lin, M. F., Santos, M. A., Sakthikumar, S., Munro, C. A., et al. (2009). Evolution of pathogenicity and sexual reproduction in eight candida genomes. Nature 459, 657–662. doi: 10.1038/nature08064
Carvunis, A.-R., and Ideker, T. (2014). Siri of the cell: what biology could learn from the iphone. Cell 157, 534–538. doi: 10.1016/j.cell.2014.03.009
Chowdhary, A., Sharma, C., and Meis, J. F. (2017). Candida auris: a rapidly emerging cause of hospital-acquired multidrug-resistant fungal infections globally. PLoS Pathog. 13:e1006290. doi: 10.1371/journal.ppat.1006290
Cottier, F., Tan, A. S. M., Chen, J., Lum, J., Zolezzi, F., Poidinger, M., et al. (2015). The transcriptional stress response of Candida albicans to weak organic acids. G3 5, 497–505. doi: 10.1534/g3.114.015941
Davies, J. Q., and Gordon, S. (2005). Isolation and culture of murine macrophages. Methods Mol. Biol. 290, 91–103. doi: 10.1385/1-59259-838-2:091
Davis, D. A., Bruno, V. M., Loza, L., Filler, S. G., and Mitchell, A. P. (2002). Candida albicans Mds3p, a conserved regulator of pH responses and virulence identified through insertional mutagenesis. Genetics 162, 1573–1581. Available online at: https://www.genetics.org/content/162/4/1573.long
Denning, D. W., and Bromley, M. J. (2015). How to bolster the antifungal pipeline. Science 347, 1414–1416. doi: 10.1126/science.aaa6097
Denning, D. W., and Hope, W. W. (2010). Therapy for fungal diseases: opportunities and priorities. Trends Microbiol. 18, 195–204. doi: 10.1016/j.tim.2010.02.004
Desai, J. V., Bruno, V. M., Ganguly, S., Stamper, R. J., Mitchell, K. F., Solis, N., et al. (2013). Regulatory role of glycerol in Candida albicans biofilm formation. MBio 4, e00637–12. doi: 10.1128/mBio.00637-12
Dutkowski, J., Kramer, M., Surma, M., Balakrishnan, R., Cherry, J., Krogan, N., et al. (2013). A gene ontology inferred from molecular networks. Nat. Biotechnol. 31, 38–45. doi: 10.1038/nbt.2463
Finkel, J. S., Xu, W., Huang, D., Hill, E. M., Desai, J. V., Woolford, C. A., et al. (2012). Portrait of Candida albicans adherence regulators. PLoS Pathog. 8:e1002525. doi: 10.1371/journal.ppat.1002525
Gauwerky, K., Borelli, C., and Korting, H. C. (2009). Targeting virulence: a new paradigm for antifungals. Drug Discov. Today 14, 214–222. doi: 10.1016/j.drudis.2008.11.013
Grumaz, C., Lorenz, S., Stevens, P., Lindemann, E., Schöck, U., Retey, J., et al. (2013). Species and condition specific adaptation of the transcriptional landscapes in Candida albicans and Candida dubliniensis. BMC Genomics 14:212. doi: 10.1186/1471-2164-14-212
Hausauer, D. L., Gerami-Nejad, M., Kistler-Anderson, C., and Gale, C. A. (2005). Hyphal guidance and invasive growth in Candida albicans require the Ras-like GTPase Rsr1p and its GTPase-activating protein Bud2p. Eukaryot. cell 4, 1273–1286. doi: 10.1128/EC.4.7.1273-1286.2005
Inglis, D. O., Arnaud, M. B., Binkley, J., Shah, P., Skrzypek, M. S., Wymore, F., et al. (2012). The candida genome database incorporates multiple candida species: multispecies search and analysis tools with curated gene and protein information for Candida albicans and Candida glabrata. Nucleic Acids Res. 40, D667–D674. doi: 10.1093/nar/gkr945
Kramer, M., Dutkowski, J., Yu, M., Bafna, V., and Ideker, T. (2014). Inferring gene ontologies from pairwise similarity data. Bioinformatics 30, i34–i42. doi: 10.1093/bioinformatics/btu282
Kramer, M. H., Farré, J.-C., Mitra, K., Yu, M. K., Ono, K., Demchak, B., et al. (2017). Active interaction mapping reveals the hierarchical organization of autophagy. Mol. Cell 65, 761–774. doi: 10.1016/j.molcel.2016.12.024
Kullberg, B. J., and Arendrup, M. C. (2015). Invasive candidiasis. N. Engl. J. Med. 373, 1445–1456. doi: 10.1056/NEJMra1315399
Langfelder, P., and Horvath, S. (2008). WGCNA: an R package for weighted correlation network analysis. BMC Bioinformatics 9:559. doi: 10.1186/1471-2105-9-559
Langmead, B., and Salzberg, S. L. (2012). Fast gapped-read alignment with Bowtie 2. Nat. Methods 9, 357–359. doi: 10.1038/nmeth.1923
Langmead, B., Trapnell, C., Pop, M., Salzberg, S. L., et al. (2009). Ultrafast and memory-efficient alignment of short DNA sequences to the human genome. Genome Biol. 10:R25. doi: 10.1186/gb-2009-10-3-r25
Leach, M. D., Stead, D. A., Argo, E., and Brown, A. J. (2011). Identification of sumoylation targets, combined with inactivation of SMT3, reveals the impact of sumoylation upon growth, morphology, and stress resistance in the pathogen Candida albicans. Mol. Biol. Cell 22, 687–702. doi: 10.1091/mbc.e10-07-0632
Li, H., Handsaker, B., Wysoker, A., Fennell, T., Ruan, J., Homer, N., et al. (2009). The sequence alignment/map format and samtools. Bioinformatics 25, 2078–2079. doi: 10.1093/bioinformatics/btp352
Liu, H., Kohler, J., and Fink, G. R. (1994). Suppression of hyphal formation in Candida albicans by mutation of a STE12 homolog. Science 266:1723. doi: 10.1126/science.7992058
Liu, Y., Shetty, A. C., Schwartz, J. A., Bradford, L. L., Xu, W., Phan, Q. T., et al. (2015). New signaling pathways govern the host response to C. albicans infection in various niches. Genome Res. 25, 679–689. doi: 10.1101/gr.187427.114
Lo, H.-J., Köhler, J. R., DiDomenico, B., Loebenberg, D., Cacciapuoti, A., and Fink, G. R. (1997). Nonfilamentous C. albicans mutants are avirulent. Cell 90, 939–949. doi: 10.1016/S0092-8674(00)80358-X
Lohse, M. B., Gulati, M., Johnson, A. D., and Nobile, C. J. (2018). Development and regulation of single- and multi-species Candida albicans biofilms. Nat. Rev. Microbiol. 16, 19–31. doi: 10.1038/nrmicro.2017.107
Lorenz, M. C., Bender, J. A., and Fink, G. R. (2004). Transcriptional response of Candida albicans upon internalization by macrophages. Eukaryot. Cell 3, 1076–1087. doi: 10.1128/EC.3.5.1076-1087.2004
Love, M. I., Huber, W., and Anders, S. (2014). Moderated estimation of fold change and dispersion for RNA-Seq data with DESeq2. Genome Biol. 15:550. doi: 10.1186/s13059-014-0550-8
Marchais, V., Kempf, M., Licznar, P., Lefrançois, C., Bouchara, J.-P., Robert, R., et al. (2005). DNA array analysis of Candida albicans gene expression in response to adherence to polystyrene. FEMS Microbiol. Lett. 245, 25–32. doi: 10.1016/j.femsle.2005.02.014
Mayer, F. L., Wilson, D., and Hube, B. (2013). Candida albicans pathogenicity mechanisms. Virulence 4, 119–128. doi: 10.4161/viru.22913
McCluskey, K., Wiest, A., and Plamann, M. (2010). The fungal genetics stock center: a repository for 50 years of fungal genetics research. J. Biosci. 35, 119–126. doi: 10.1007/s12038-010-0014-6
McKenzie, C. G. J., Koser, U., Lewis, L. E., Bain, J. M., Mora-Montes, H. M., Barker, R. N., et al. (2010). Contribution of Candida albicans cell wall components to recognition by and escape from murine macrophages. Infect. Immun. 78, 1650–1658. doi: 10.1128/IAI.00001-10
Mount, H. O., Revie, N. M., Todd, R. T., Anstett, K., Collins, C., Costanzo, M., et al. (2018). Global analysis of genetic circuitry and adaptive mechanisms enabling resistance to the azole antifungal drugs. PLoS Genet. 14:e1007319. doi: 10.1371/journal.pgen.1007319
Moyes, D. L., Wilson, D., Richardson, J. P., Mogavero, S., Tang, S. X., Wernecke, J., et al. (2016). Candidalysin is a fungal peptide toxin critical for mucosal infection. Nature 532, 64–68. doi: 10.1038/nature17625
Munro, C. A., Bates, S., Buurman, E. T., Hughes, H. B., MacCallum, D. M., Bertram, G., et al. (2005). Mnt1p and mnt2p of Candida albicans are partially redundant α-1, 2-mannosyltransferases that participate in o-linked mannosylation and are required for adhesion and virulence. J. Biol. Chem. 280, 1051–1060. doi: 10.1074/jbc.M411413200
Nett, J. E., Lepak, A. J., Marchillo, K., and Andes, D. R. (2009). Time course global gene expression analysis of an in vivo candida biofilm. J. Infect. Dis. 200, 307–313. doi: 10.1086/599838
Nobile, C. J., Fox, E. P., Nett, J. E., Sorrells, T. R., Mitrovich, Q. M., Hernday, A. D., et al. (2012). A recently evolved transcriptional network controls biofilm development in Candida albicans. Cell 148, 126–138. doi: 10.1016/j.cell.2011.10.048
Noble, S. M., French, S., Kohn, L. A., Chen, V., and Johnson, A. D. (2010). Systematic screens of a Candida albicans homozygous deletion library decouple morphogenetic switching and pathogenicity. Nat. Genet. 42, 590–598. doi: 10.1038/ng.605
Noble, S. M., Gianetti, B. A., and Witchley, J. N. (2016). Candida albicans cell-type switching and functional plasticity in the mammalian host. Nat. Rev. Microbiol. 15, 96–108. doi: 10.1038/nrmicro.2016.157
Queva, C., Hurlin, P. J., Foley, K. P., and Eisenman, R. N. (1998). Sequential expression of the mad family of transcriptional repressors during differentiation and development. Oncogene 16, 967–977. doi: 10.1038/sj.onc.1201611
Rauceo, J. M., Blankenship, J. R., Fanning, S., Hamaker, J. J., Deneault, J.-S., Smith, F. J., et al. (2008). Regulation of the Candida albicans cell wall damage response by transcription factor Sko1 and PAS kinase Psk1. Mol. Biol. Cell 19, 2741–2751. doi: 10.1091/mbc.e08-02-0191
Reedy, J. L., Filler, S. G., and Heitman, J. (2010). Elucidating the Candida albicans calcineurin signaling cascade controlling stress response and virulence. Fungal Genet. Biol. 47, 107–116. doi: 10.1016/j.fgb.2009.09.002
Richard, M. L., Nobile, C. J., Bruno, V. M., and Mitchell, A. P. (2005). Candida albicans biofilm-defective mutants. Eukaryot. Cell 4, 1493–1502. doi: 10.1128/EC.4.8.1493-1502.2005
Robinson, M. D., McCarthy, D. J., and Smyth, G. K. (2010). edgeR: a bioconductor package for differential expression analysis of digital gene expression data. Bioinformatics 26, 139–140. doi: 10.1093/bioinformatics/btp616
Skrzypek, M. S., Binkley, J., Binkley, G., Miyasato, S. R., Simison, M., and Sherlock, G. (2016). The candida genome database (CGD): incorporation of assembly 22, systematic identifiers and visualization of high throughput sequencing data. Nucleic Acids Res. 45, D592–D596. doi: 10.1093/nar/gkw924
Staab, J. F., Bahn, Y.-S., Tai, C.-H., Cook, P. F., and Sundstrom, P. (2004). Expression of transglutaminase substrate activity on Candida albicans germ tubes through a coiled, disulfide-bonded N-terminal domain of Hwp1 requires C-terminal glycosylphosphatidylinositol modification. J. Biol. Chem. 279, 40737–40747. doi: 10.1074/jbc.M406005200
Staab, J. F., Bradway, S. D., Fidel, P. L., and Sundstrom, P. (1999). Adhesive and mammalian transglutaminase substrate properties of Candida albicans Hwp1. Science 283, 1535–1538. doi: 10.1126/science.283.5407.1535
Staab, J. F., and Sundstrom, P. (1998). Genetic organization and sequence analysis of the hypha-specific cell wall protein gene Hwp1 of Candida albicans. Yeast 14, 681–686. doi: 10.1002/(SICI)1097-0061(199805)14:7 <681::AID-YEA256>3.0.CO;2-8
Theiss, S., Kretschmar, M., Nichterlein, T., Hof, H., Agabian, N., Hacker, J., et al. (2002). Functional analysis of a vacuolar ABC transporter in wild-type Candida albicans reveals its involvement in virulence. Mol. Microbiol. 43, 571–584. doi: 10.1046/j.1365-2958.2002.02769.x
Uhl, M. A., Biery, M., Craig, N., and Johnson, A. D. (2003). Haploinsufficiency-based large-scale forward genetic analysis of filamentous growth in the diploid human fungal pathogen C. albicans. EMBO J. 22, 2668–2678. doi: 10.1093/emboj/cdg256
Vila, T., Romo, J. A., Pierce, C. G., McHardy, S. F., Saville, S. P., and Lopez-Ribot, J. L. (2017). Targeting Candida albicans filamentation for antifungal drug development. Virulence 8, 150–158. doi: 10.1080/21505594.2016.1197444
Warenda, A. J., Kauffman, S., Sherrill, T. P., Becker, J. M., and Konopka, J. B. (2003). Candida albicans septin mutants are defective for invasive growth and virulence. Infect. Immun. 71, 4045–4051. doi: 10.1128/IAI.71.7.4045-4051.2003
Wilson, D., Thewes, S., Zakikhany, K., Fradin, C., Albrecht, A., Almeida, R., et al. (2009). Identifying infection-associated genes of Candida albicans in the postgenomic era. FEMS Yeast Res. 9, 688–700. doi: 10.1111/j.1567-1364.2009.00524.x
Winnenburg, R., Baldwin, T. K., Urban, M., Rawlings, C., Köhler, J., and Hammond-Kosack, K. E. (2006). PHI-base: a new database for pathogen host interactions. Nucleic Acids Res. 34(Suppl. 1), D459–D464. doi: 10.1093/nar/gkj047
Yu, M. K., Ma, J., Ono, K., Zheng, F., Fong, S. H., Gary, A., et al. (2019). DDOT: A swiss army knife for investigating data-driven biological ontologies. Cell Syst. 8, 267–273.e3. doi: 10.1016/j.cels.2019.02.003
Keywords: Candida albicans, co-expression network, network-extracted ontology, pathogen, pathogenicity genes, PEP8
Citation: Thomas G, Bain JM, Budge S, Brown AJP and Ames RM (2020) Identifying Candida albicans Gene Networks Involved in Pathogenicity. Front. Genet. 11:375. doi: 10.3389/fgene.2020.00375
Received: 29 January 2020; Accepted: 26 March 2020;
Published: 24 April 2020.
Edited by:
Christina A. Cuomo, Broad Institute, United StatesReviewed by:
David Kadosh, The University of Texas Health Science Center at San Antonio, United StatesCopyright © 2020 Thomas, Bain, Budge, Brown and Ames. This is an open-access article distributed under the terms of the Creative Commons Attribution License (CC BY). The use, distribution or reproduction in other forums is permitted, provided the original author(s) and the copyright owner(s) are credited and that the original publication in this journal is cited, in accordance with accepted academic practice. No use, distribution or reproduction is permitted which does not comply with these terms.
*Correspondence: Ryan M. Ames, ci5hbWVzQGV4ZXRlci5hYy51aw==
Disclaimer: All claims expressed in this article are solely those of the authors and do not necessarily represent those of their affiliated organizations, or those of the publisher, the editors and the reviewers. Any product that may be evaluated in this article or claim that may be made by its manufacturer is not guaranteed or endorsed by the publisher.
Research integrity at Frontiers
Learn more about the work of our research integrity team to safeguard the quality of each article we publish.