- 1Gastrointestinal Surgery, The First Affiliated Hospital of Fujian Medical University, Fuzhou, China
- 2Department of Radiotherapy, The First Affiliated Hospital of Fujian Medical University, Fuzhou, China
Growing evidence suggests that immune-related genes (IRGs) and long non-coding RNAs (lncRNAs) can serve as prognostic markers of overall survival (OS) in patients with colon cancer. This study aimed to identify an immune-related lncRNA signature for the prospective assessment of prognosis in these patients. Gene expression and clinical data of colon cancer patients were downloaded from The Cancer Genome Atlas (TCGA). Immune-related lncRNAs were identified by a correlation analysis between IRGs and lncRNAs. In total, 447 samples were divided into a training cohort (224 samples) and a testing cohort (223 samples). Univariate, lasso and multivariate Cox regression analyses identified an immune-related nine-lncRNA signature closely related to OS in colon cancer patients in the training dataset. A risk score formula involving nine immune-related lncRNAs was developed to evaluate the prognostic value of the lncRNA signature in the training dataset. Colon cancer patients with a high risk score had poorer OS than those with a low risk score. A multivariate Cox regression analysis confirmed that the immune-related nine-lncRNA signature could be an independent prognostic factor in colon cancer patients. The results were further confirmed in the testing cohort and the entire TCGA cohort. Furthermore, a gene set enrichment analysis revealed several pathways with significant enrichment in the high- and low-risk groups that may be helpful in formulating clinical strategies and understanding the underlying mechanisms. Finally, a quantitative real-time polymerase chain reaction assay found that the nine lncRNAs were significantly differentially expressed in colon cancer cell lines. The results of this study indicate that this signature has important clinical implications for improving predictive outcomes and guiding individualized treatment in colon cancer patients. These lncRNAs could be potential biomarkers affecting the prognosis of colon cancer.
Introduction
Colon cancer is a common malignant tumor of the digestive tract. A study found that the incidence and mortality of colon cancer have gradually increased in recent years (Bray et al., 2018). The current diagnostic strategies for colon cancer mainly include biopsy, laboratory examination, and colonoscopy (Cross et al., 2019; Kawamura et al., 2019). Therefore, establishing a reliable risk assessment model is urgently needed to assess the survival prognosis of patients with colon cancer and optimize clinical treatment decisions. In recent years, genomic research has greatly enhanced our understanding of the mechanism underlying colon cancer development (Coebergh van den Braak et al., 2018) and revealed the potential value of molecular biomarkers in the diagnosis and evaluation of survival prognosis in patients with colon cancer (Galamb et al., 2019; Xu et al., 2019). Therefore, individualized treatment plans can be tailored to different patients with colon cancer to improve their survival prognosis.
Long non-coding RNAs (lncRNAs) constitute a class of non-coding RNAs that are more than 200 nucleotides in length and play an important role in the development of cancer (Geisler and Coller, 2013). Studies have shown that the expression or dysfunction of lncRNAs is closely related to the occurrence of human diseases, including cancers (Bermudez et al., 2019; Chi et al., 2019). A previous study found that the lncRNA HOTAIR promotes colon cancer development by downregulating miRNA-34a (Peng C. L. et al., 2019). The lncRNA FENDRR was found to inhibit colon cancer development by inhibiting the SOX4 protein (Liu and Du, 2019). In addition, a study found that the lncRNA CYTOR promotes metastasis in colon cancer through Wnt/β-catenin signaling (Yue et al., 2018). These studies indicate that the expression levels of lncRNAs affect colon cancer development and survival prognosis.
In recent decades, studies have shown that the immune system plays a key role in the development of tumors (Marshall and Djamgoz, 2018; Popovic et al., 2018). The expression and dysregulation of immune-related genes (IRGs) are involved in the regulation of the immune system. The activation of the JAK/STAT3 signaling pathway restores natural killer cell function and improves immune defense after cerebral ischemia (Jin et al., 2018). A related study found that galectin-1 restores immune tolerance to liver transplant patients by activating hepatic stellate cells (Jiang et al., 2018). Interferon regulatory factor 8 binds the Spp1 promoter to inhibit osteopontin expression in colonic epithelial cells, thereby increasing survival in colon cancer patients (Klement et al., 2018). These studies show that IRGs can be used to characterize the infiltration of immune cells in tumors. Therefore, advanced immunotherapy is expected to provide an alternative treatment for colon cancer patients.
In this study, we identified the expression of immune-related lncRNAs in 447 patients with colon adenocarcinoma. Cox regression models and lasso regression models were used to identify an immune-related lncRNA signature that was used to construct prognostic models. We identified an immune-related nine-lncRNA signature associated with the prognosis of colon cancer patients in the training cohort that performed well in the testing cohort and the entire The Cancer Genome Atlas (TCGA) cohort. Finally, we further verified that these nine lncRNAs were significantly differentially expressed between colon cancer cell lines and colon cell lines by a quantitative real-time polymerase chain reaction (qRT-PCR) test. This immune-related nine-lncRNA signature not only can improve the ability to predict prognosis in patients with colon cancer but also can promote better clinical strategies and elucidate the underlying mechanisms.
Materials and Methods
Data Acquisition and Processing
The RNA sequencing data and clinical data of colon adenocarcinoma samples were downloaded from TCGA1 based on the Illumina (San Diego, CA, United States) HiSeq 2000 RNA sequencing platform. The workflow type is counts. This dataset contains 488 colon adenocarcinoma tissues and 42 adjacent normal tissues. Samples with incomplete survival data were removed, and finally, 447 samples with complete clinical information were included in the subsequent analysis. The Ensemble IDs of the lncRNAs in TCGA database were extracted from the GENCODE project2 (Frankish et al., 2019). The R package (Auckland City, New Zealand) “limma” and “voom” function were used to normalize the data (Ritchie et al., 2015). The IRGs were obtained from the Molecular Signatures Database v4.0 (Immune system process M13664, Immune response M19817)3 (Barbie et al., 2009; Wei et al., 2019).
Identification of Immune-Related lncRNAs
The immune-related lncRNAs were identified by a Pearson correlation analysis between the IRGs and lncRNA expression levels in the colon cancer samples (Pearson correlation coefficient >0.4, p < 0.001). The R package “caret” was used to randomly divide the samples into the training cohort (224 samples) and the testing cohort (223 samples) (Kuhn, 2019). The expression levels of the immune-related lncRNAs were obtained in the training cohort, testing cohort, and entire TCGA cohort.
Construction of an Immune-Related lncRNA Signature Associated With Prognosis
The R packages “survival,” “survminer,” and “forestplot” were used to perform a univariate Cox regression analysis and multivariate Cox regression analysis (Alboukadel and Kosinski, 2019; Max and Lumley, 2019). The R package “glmnet” was used to perform a lasso regression analysis (Jerome et al., 2010). Immune-related lncRNAs with a significant prognostic value (p < 0.01) were screened. In the multivariate Cox regression analysis, the Akaike information criteria had a minimum value of 344.96 as the best cutoff point. The immune-related lncRNA signature is expressed as follows: risk score = (coefficient lncRNA1 × lncRNA1 expression) + (coefficient lncRNA2 × expression of lncRNA2) + … + (coefficient lncRNAn × expression lncRNAn). The median risk score served as a cutoff value to classify the patients in the training cohort into the high- and low-risk groups. The testing cohort was also divided into high- and low-risk groups using the same cutoff value. The R package “survival” was used to plot Kaplan–Meier survival curves. The R package “survivalROC” was used to investigate the prognostic value of the immune-related lncRNA signature over time (Patrick and Saha-Chaudhuri, 2013). A two-sided log-rank p < 0.05 was considered significant in the survival analysis.
Independence of Prognostic Factors From Other Clinical Parameters in TCGA
The complete information of the 447 patients included relevant clinical data for the univariate and multivariate Cox regression analyses. p < 0.05 was considered statistically significant.
Construction of a Predictive Nomogram
A prognostic model was constructed by obtaining the independent prognostic factors, and a time-dependent receiver operating characteristic (ROC) curve was used to compare the models. The most appropriate independent prognostic factors were chosen for the prognostic model to construct a nomogram in the entire TCGA cohort. The calibration plot and concordance index (C-index) were used to investigate the calibration and the discrimination of the nomogram (by a bootstrap method with 1,000 resamples) (Iasonos et al., 2008).
Gene Set Enrichment Analysis
To understand the Kyoto Encyclopedia of Genes and Genomes (KEGG) pathways of the immune-related lncRNA signature, a gene set enrichment analysis (GSEA) was used to analyze the enrichment terms in the entire TCGA cohort. GSEA software version 4.1.1 (Cambridge, MA, United States) was used to perform the GSEA, and p < 0.05 was considered statistically significant.
Gene Expression Profiling Interactive Analysis Dataset
Gene Expression Profiling Interactive Analysis4 is a cancer data website. The content that can be analyzed also covers many aspects, including single genes, multiple genes, cancer types, and so on (Tang et al., 2017). Using this database, the expression of each lncRNA in normal tissues and cancer tissues can be obtained.
Comparison of the Immune-Related lncRNA Signature With Other Colon Cancer Prognostic Models
To determine whether this immune-related lncRNA signature is better than other models, we compared (Zhou et al., 2018) six-lncRNA signature, (Xue et al., 2017) two-lncRNA signature, (Zhang et al., 2019) five-lncRNA signature, and (Zeng et al., 2017) four-lncRNA signature. We obtained the lncRNAs in these models from the literature and constructed 1-, 3-, and 5-year ROC curves and survival curves for the entire TCGA cohort. These lncRNA signatures were compared with the immune-related lncRNA signature identified in this study to evaluate their pros and cons.
Cell Culture
A colon cell line (CCD-841CoN) and colon cancer cell lines (HCT116 and SW480) were purchased from the Cell Bank of the Chinese Academy of Sciences (Shanghai, China). The CCD-841CoN cells were grown in High Glucose Dulbecco’s Modified Eagle Medium, the HCT116 cells were grown in McCoy’s 5A medium, and the SW480 cells were grown in RPMI-1640 medium (Gibco, Carlsbad, CA, United States) containing 10% fetal bovine serum (Gibco). The cells were grown at 37°C with 5% CO2.
Detection of lncRNA Expression in Cell Lines
The RNA was extracted with TRIzol reagent (TransGen Biotech, Beijing, China), and then, the expression levels of nine lncRNAs were detected by qRT-PCR. The qRT-PCR assay was performed as follows: RNA was detected using a reverse transcription kit (TaKaRa, Dalian, China) and an amplification kit (TaKaRa) following the manufacturer’s instructions. GAPDH was used as an internal control. The reaction mixture contained 10 μL of SYBR Premix Ex Taq 2, 1 μL of each primer, 2 μL of the cDNA template, and 6 μL of ddH2O for a final volume of 20 μL. The thermal cycling parameters used for amplification were as follows: a denaturation step at 95°C for 30 s, followed by 40 cycles at 95°C for 5 s, and a final holding step at 60°C for 34 s. The relative gene expression levels were evaluated with Data Assist software version 3.0 (Applied Biosystems, Foster City, CA, United States). The relative expression levels were determined according to the 2–ΔΔCT method. The assays were performed in triplicate. The primer sequences for each gene are shown in Supplementary Table S1.
Statistical Analysis
The statistical analyses were performed using SPSS software version 17.0 (Armonk, New York, United States) and R software version 3.6.0 (Auckland City, New Zealand). Pearson χ2 test or Fisher exact test were used to explore the qualitative variables as appropriate.
Results
Identification of Immune-Related lncRNAs
In total, 761 immune-related lncRNAs were identified in the 447 colon adenocarcinoma samples. The R package “caret” was used to randomly divide the samples into the training cohort and the testing cohort. The clinical features of the colon cancer patients are shown in Table 1. The expression profiles of the 761 immune-related lncRNAs in the training cohort were used to construct a prognostic model.
Construction and Validation of the Immune-Related lncRNA Signature in TCGA
The univariate Cox regression models identified 20 immune-related lncRNAs associated with overall survival (OS) (Figure 1A). Fourteen immune-related lncRNAs were identified by a lasso regression analysis (Figures 1B,C). Nine immune-related lncRNAs were identified for the construction of the prognostic model by a multivariate Cox regression analysis (Figure 1D). The results of the univariate and multivariate Cox regression analyses of the nine immune-related lncRNAs in the training cohort are shown in Table 2. The nine immune-related lncRNAs include AC008760.1, AC083809.1, AL445645.1, AC009237.14, AL391422.4, LINC01234, LINC02381, LINC01063, and AC016027.1. The model is expressed as follows: risk score = 0.409 × expression of AC008760.1 + 0.074 × expression of AC083809.1 + 0.906 × expression of AL445645.1 + 0.214 × expression of AC009237.14 + 0.737 × expression of AL391422.4 + 0.245 × expression of LINC01234 + 0.378 × expression of LINC02381 + 0.482 × expression of LINC01063-2.506 × expression of AC016027.1. The patients were divided into high- and low-risk groups by the median risk score (0.909), which served as a cutoff value. The model constructed with the nine-lncRNA immune signature showed that the area under the curve (AUC) values of the time–ROC curve of the 1-, 3-, and 5-year OS were 0.832, 0.906, and 0.891, respectively (Figure 2A). As the risk score increased, the patient mortality rate gradually increased (Figure 2B). The OS in the high-risk group was significantly worse than that in the low-risk group (Figure 2C, p < 0.0001). Then, the prognostic model was validated in the testing cohort, and this model showed that the AUC values of the 1-, 3-, and 5-year OS were 0.758, 0.794, and 0.784, respectively (Figure 2D). As the risk score increased, the patient mortality rate gradually increased (Figure 2E). According to the median risk score obtained from the training cohort (0.909), the patients in the testing cohort were also divided into high- and low-risk groups. The OS in the high-risk group was significantly worse than that in the low-risk group (Figure 2F, p < 0.001). Similarly, in the entire TCGA cohort, the results showed that the AUC values of the 1-, 3-, and 5-year OS were 0.758, 0.854, and 0.838, respectively (Figure 2G). As the risk score increased, the patient mortality rate gradually increased (Figure 2H). The OS in the high-risk group was significantly worse than that in the low-risk group (Figure 2I, p < 0.0001). These results indicate that the prognostic model is moderately sensitive and specific.
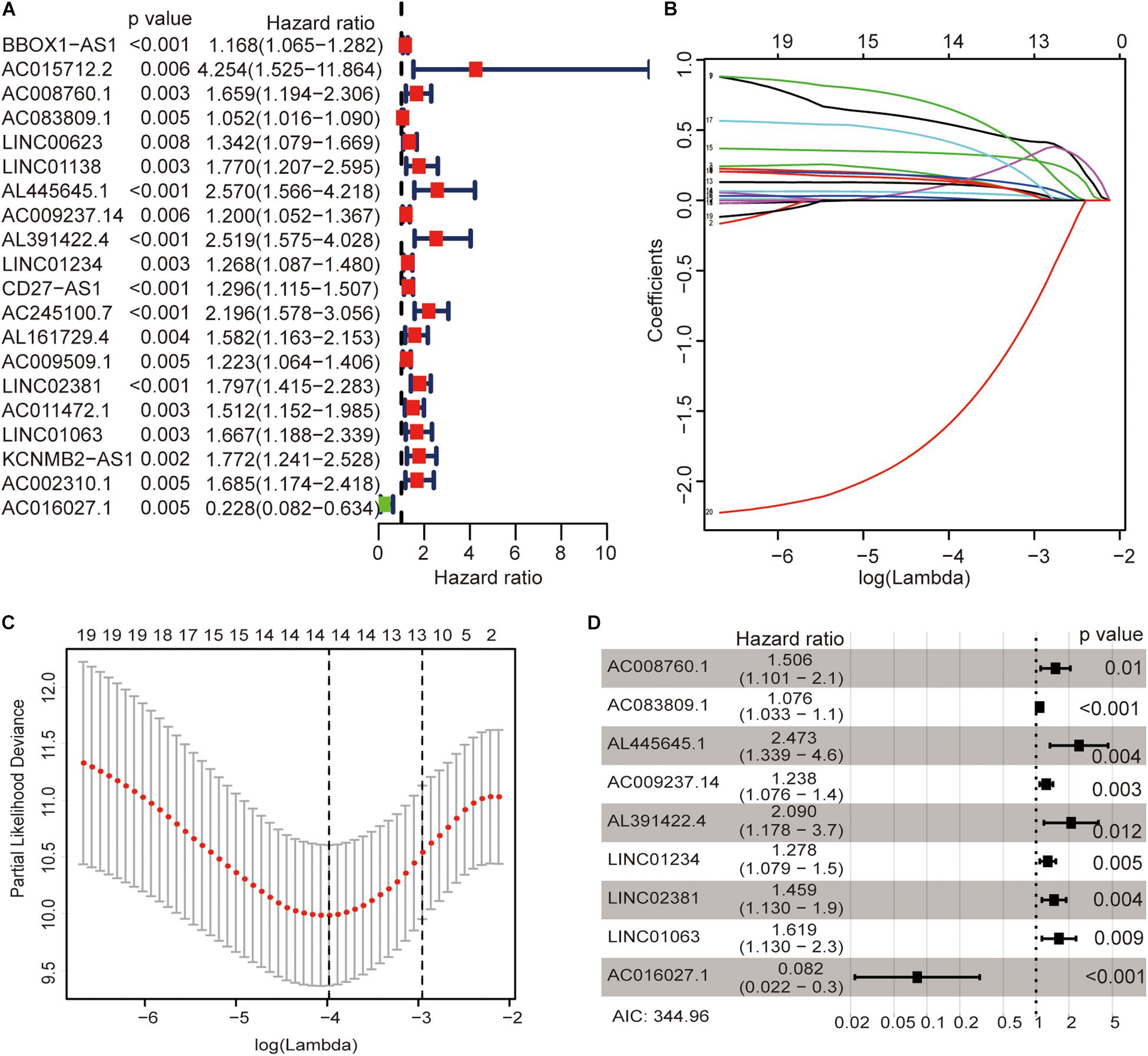
Figure 1. Identification of immune-related lncRNAs associated with colon cancer prognosis. Univariate Cox regression models identified 20 immune-related lncRNAs associated with OS (A). Fourteen immune-related lncRNAs were identified by a lasso regression analysis (B,C). Nine immune-related lncRNAs were identified for the construction of a prognostic model by a multivariate Cox regression analysis (D).
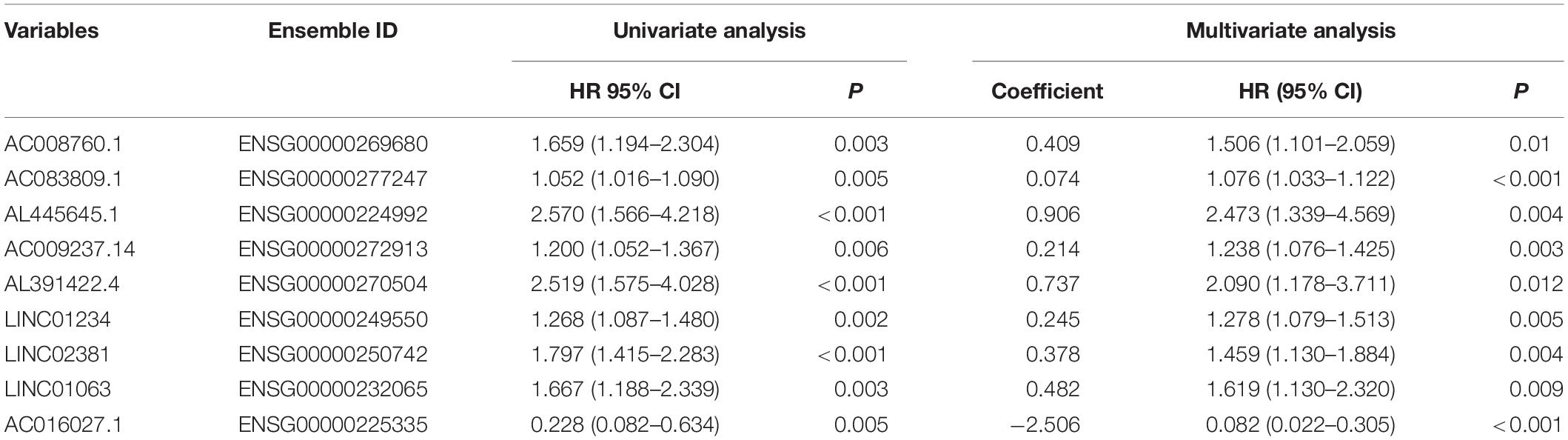
Table 2. Univariate and multivariate Cox regression analyses of nine immune-related lncRNAs in the training cohort.
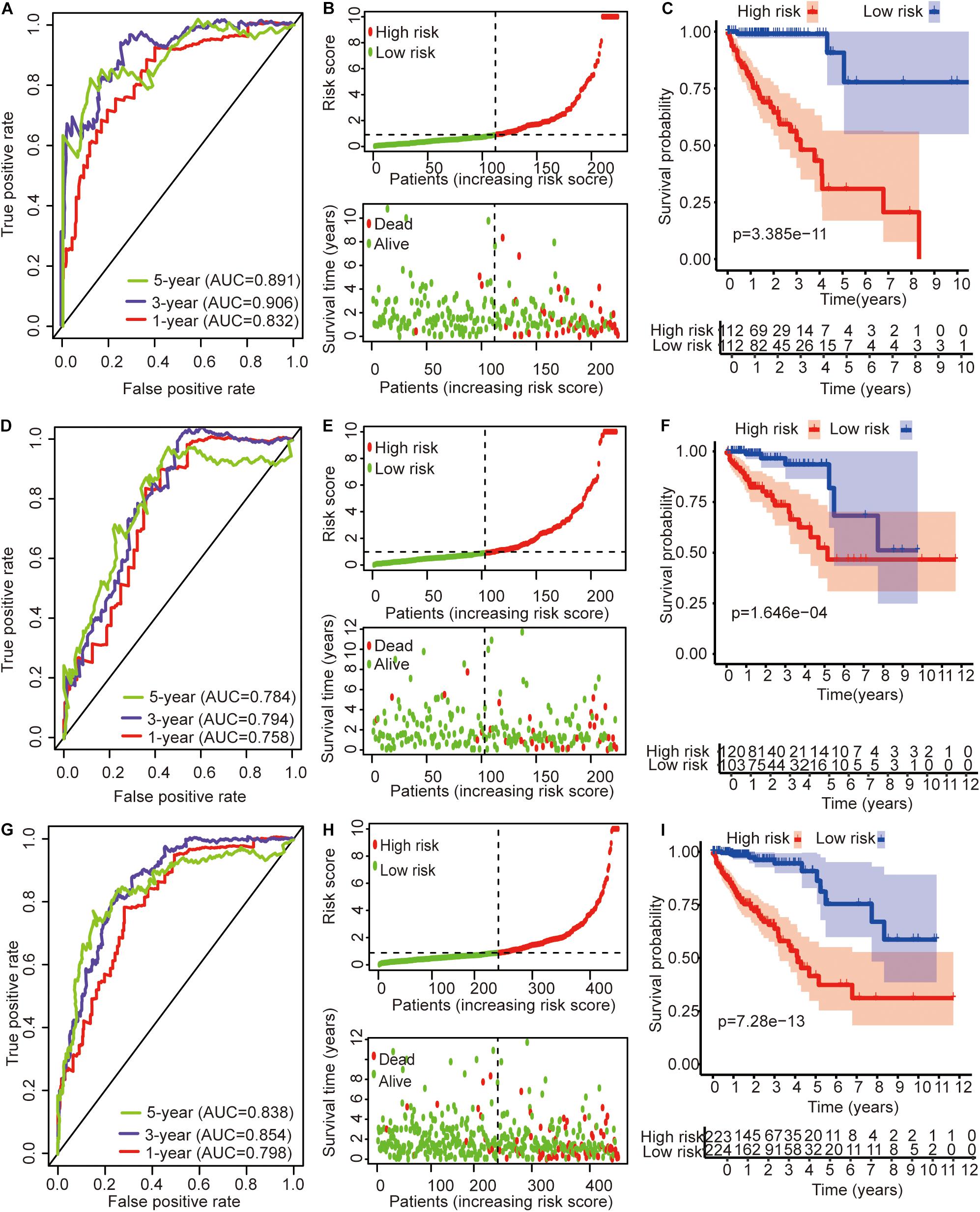
Figure 2. Time–ROC curve analysis, risk score analysis, and Kaplan–Meier curve survival analysis of the immune-related lncRNA signature in colon cancer. (A) Time–ROC curve analysis, (B) risk score, and (C) Kaplan–Meier survival curve of the immune-related lncRNA signature in the training cohort. (D) Time–ROC curve analysis, (E) risk score, and (F) Kaplan–Meier survival curve of the immune-related lncRNA signature in the testing cohort. (G) Time–ROC curve analysis, (H) risk score, and (I) Kaplan–Meier survival curve of the immune-related lncRNA signature in the entire TCGA cohort.
Correlation Between the Clinicopathologic Characteristics and the Immune-Related lncRNA Signature in Colon Cancer
The colon cancer patients were divided into two groups (high- and low-risk groups) according to the risk score of the model in the training cohort, testing cohort, and entire TCGA cohort (Figure 3 and Table 3). The colon cancer patient information included age, gender, tumor stage, tumor invasion, lymph node, and distant metastasis. The risk scores were found to be significantly correlated with gender, the tumor stage, and lymphatic metastasis in the training cohort. In the testing cohort, the risk scores were significantly correlated with the tumor stage and lymphatic metastasis. Similarly, the risk scores were significantly associated with gender, the tumor stage, tumor invasion, lymphatic metastasis, and distant metastasis in the entire TCGA cohort. Combining the results of the above three cohorts showed a significant association between the immune-related lncRNA signature model and the tumor stage and lymphatic metastasis.
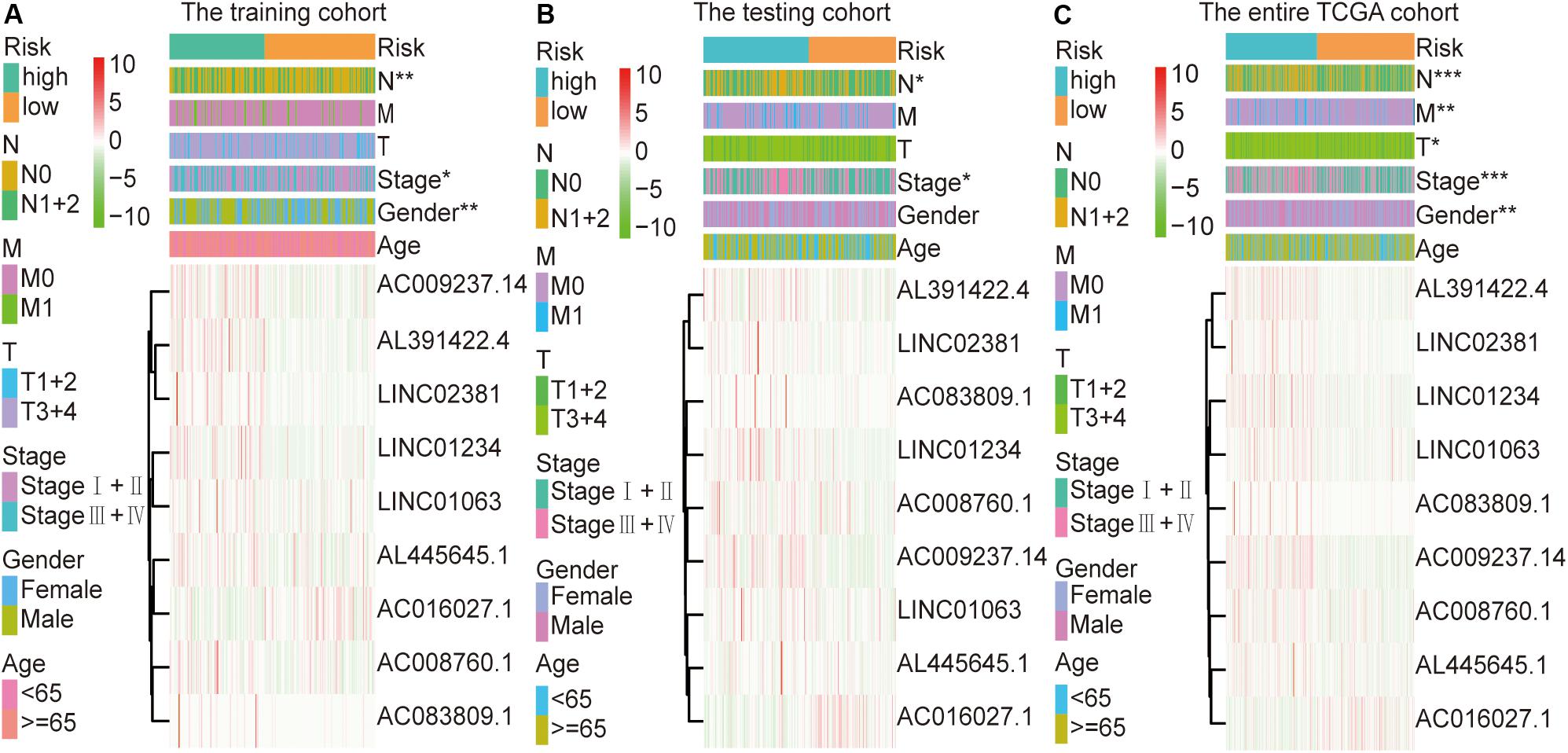
Figure 3. Relationship between the risk score and clinical characteristics of colon cancer in three cohorts. (A) Risk scores were significantly associated with gender, lymph nodes, and clinical stage in the training cohort. (B) Risk scores were significantly associated with lymph nodes and clinical stage in the testing cohort. (C) Risk scores were significantly associated with gender, lymph nodes, metastases, tumor depth, and clinical stage in the entire TCGA cohort. *p < 0.05, **p < 0.01, ***p < 0.001.
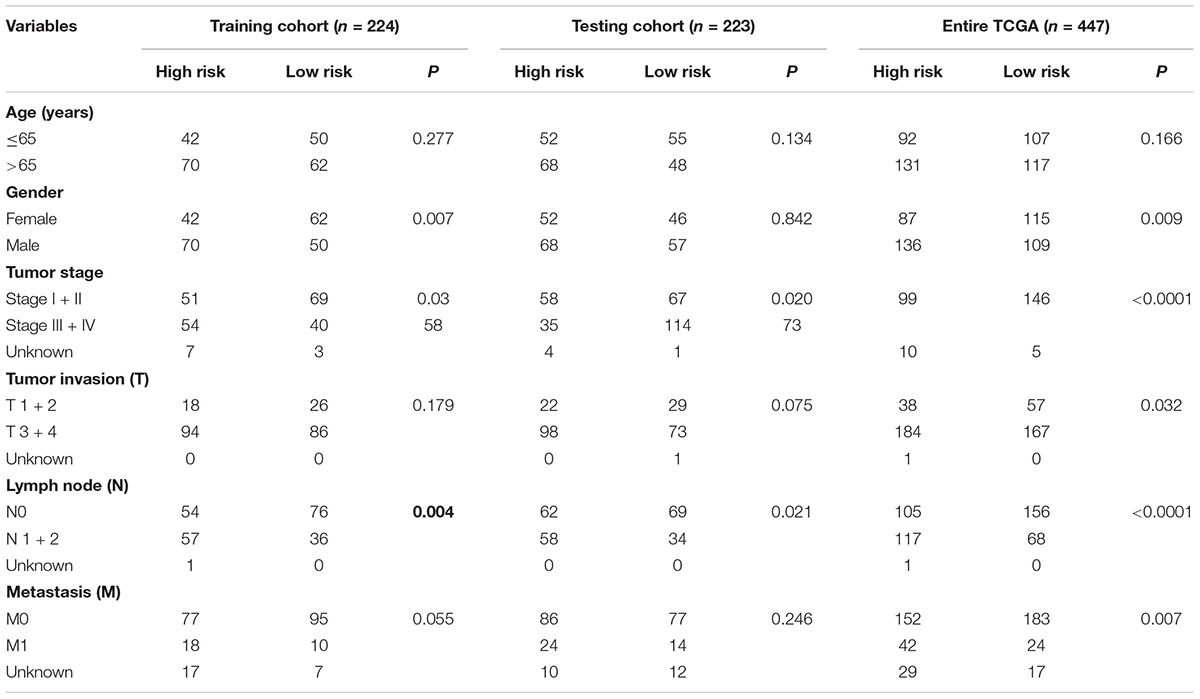
Table 3. Correlation between clinicopathologic characteristics and the immune-related lncRNA signature in colon cancer.
Identification of Independent Prognostic Factors
In the training cohort, the univariate and multivariate Cox regression analyses showed that the tumor stage and risk score were independent prognostic factors for OS in colon cancer patients (Table 4). In the testing cohort, the univariate and multivariate Cox regression analyses showed that age, the tumor stage, and the risk score were independent prognostic factors for OS (Table 4). Similarly, in the entire TCGA cohort, age, the tumor stage, and the risk score were also independent prognostic factors for OS (Table 4). Based on the above results, the p values of only the tumor stage and risk score were less than 0.05 in the three cohorts. This result suggests that the tumor stage and risk score may be independent prognostic factors for OS in colon cancer patients.
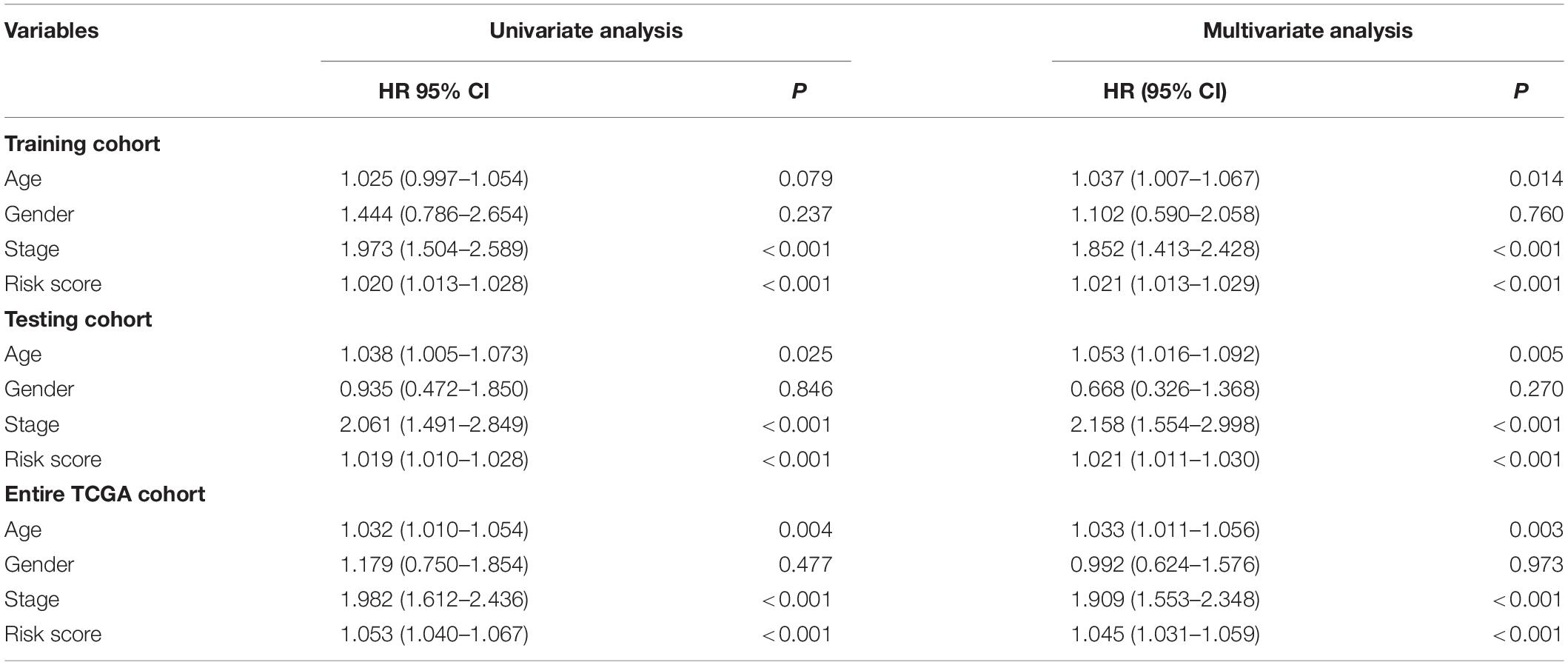
Table 4. Univariate and multivariate Cox regression analyses of the clinical features in each cohort.
Construction of the Prognostic Nomogram
Subsequently, we constructed a prognostic model including the tumor stage, and the AUC values of the time–ROC curve were used to assess the accuracy of the model (Figure 4A). In the training cohort, the AUC values of the 1-, 3-, and 5-year OS were 0.636, 0.814, and 0.809, respectively. In the testing cohort, the AUC values of the 1-, 3-, and 5-year OS were 0.756, 0.695, and 0.728, respectively. In the entire TCGA cohort, the AUC values of the 1-, 3-, and 5-year OS were 0.686, 0.741, and 0.756, respectively. This result indicates that the AUC values of the tumor staging model are lower than those of the prognostic model constructed with the immune-related lncRNA signature of 1-, 3-, and 5-year OS. Prognostic models constructed with the immune-related lncRNA signature may be better predictors of OS in colon cancer patients. Therefore, we constructed a nomogram to predict OS in patients with colon cancer based on the risk scores (Figure 4B). The calibration plots showed that the performance of the nomogram was the best in predicting the 1-, 3-, and 5-year OS (Figure 4C).
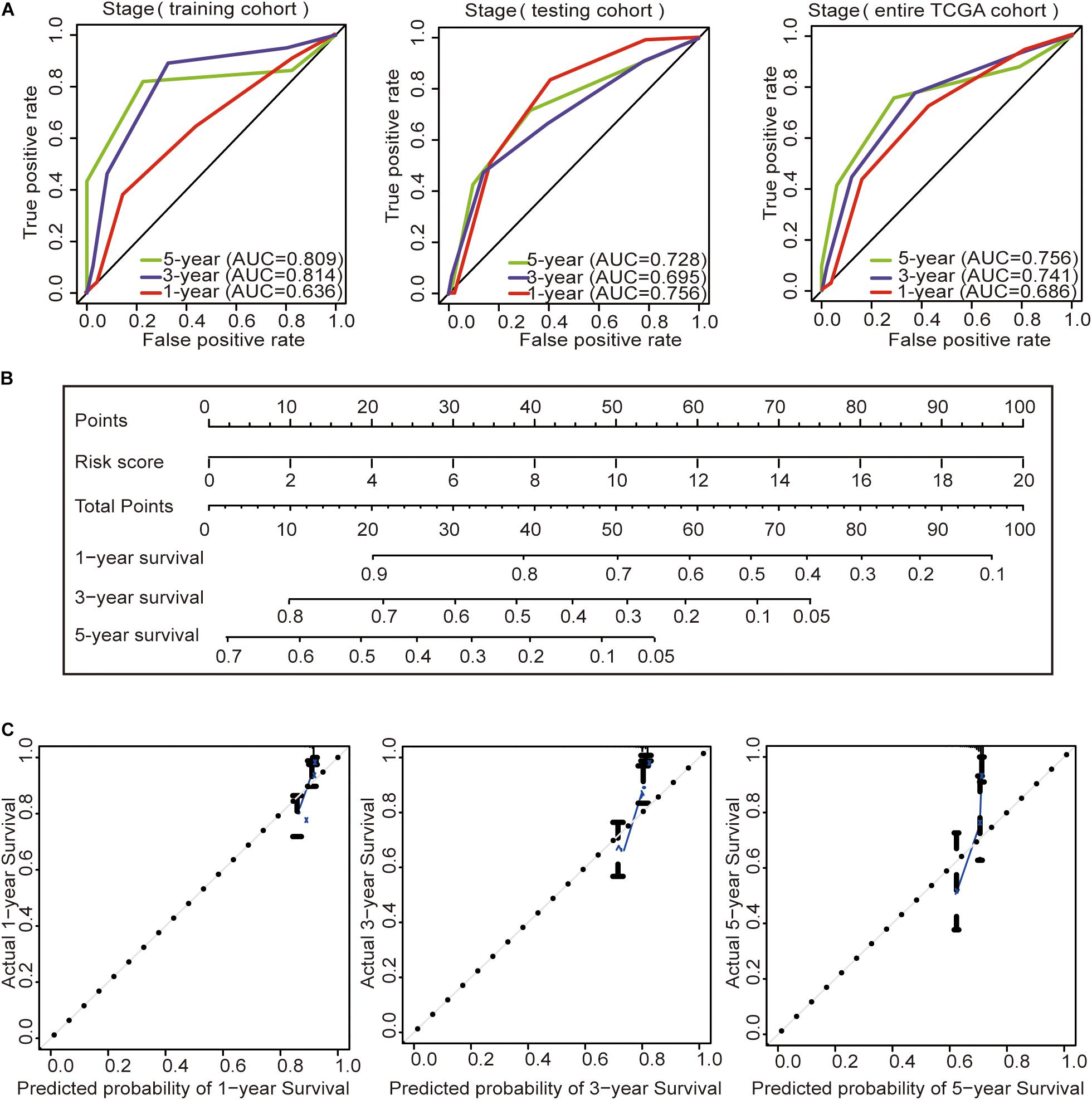
Figure 4. Construction of the prognostic model and GSEA. (A) Time–ROC curves of the tumor stage prognostic model were compared in terms of the 1-, 3-, and 5-year OS in each cohort. (B) Construction of the nomogram was based on the risk score in the entire TCGA cohort. (C) Calibration plot for the internal validation of the nomogram.
Gene Set Enrichment Analysis
The GSEA indicated that the immune-related lncRNA signature was mainly enriched in the complement and coagulation cascades, extracellular matrix (ECM) receptor interaction, focal adhesion, hedgehog signaling pathway, mitogen-activated protein kinase (MAPK) signaling pathway, notch signaling pathway, pathways in cancer and Wnt signaling pathway in the high-risk patients (Figure 5A) and the main citrate cycle, tricarboxylic acid cycle, porphyrin and chlorophyll metabolism, steroid biosynthesis, and terpenoid backbone biosynthesis in the low-risk patients (Figure 5B).
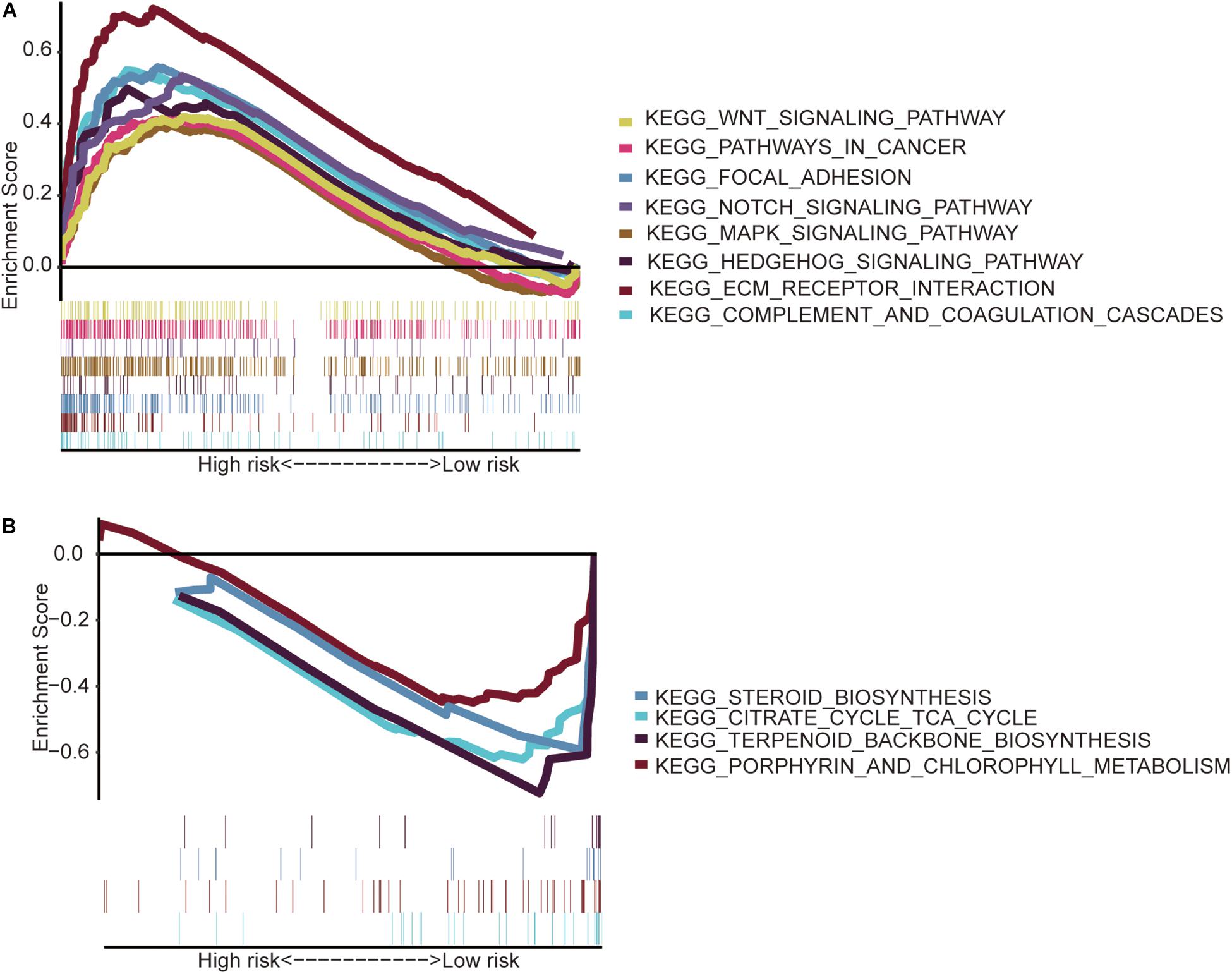
Figure 5. Gene set enrichment analysis. (A) High-risk patients with eight significantly enriched KEGG pathways in the entire TCGA cohort by GSEA. (B) Four significantly enriched KEGG pathways in the low-risk patients were analyzed by GSEA in the entire TCGA cohort.
Comparison of the Immune-Related lncRNA Signature With Other Colon Cancer Prognostic Models
To determine whether this immune-related lncRNA signature is better than other models, we compared (Zhou et al., 2018) six-lncRNA signature, (Xue et al., 2017) two-lncRNA signature, (Zhang et al., 2019) five-lncRNA signature, and (Zeng et al., 2017) four-lncRNA signature. The prognosis of the high-risk group and the low-risk group significantly differed across these four models (Figure 6). Zhou et al. (2018) six-lncRNA signature shows that the AUC values of the 1-, 3-, and 5-year OS are 0.578, 0.611, and 0.682, respectively (Figure 6A). Zhang et al. (2019) five-lncRNA signature shows that the AUC values of the 1-, 3-, and 5-year OS are 0.661, 0.641, and 0.688, respectively (Figure 6C). Zeng et al. (2017)four-lncRNA signature shows that the AUC values of the 1-, 3-, and 5-year OS are 0.690, 0.673, and 0.640, respectively (Figure 6D). This result shows that all three models have a certain predictive power. However, in Xue et al. (2017) two-lncRNA signature, the AUC values of the 1-, 3-, and 5-year OS are 0.527, 0.510, and 0.520, respectively (Figure 6B). This result indicates that the model has poor predictive ability. By comparing with immune-related lncRNA signatures, we find that the accuracy of the immune-related lncRNA signature prediction is higher than that of these four models (Table 5).
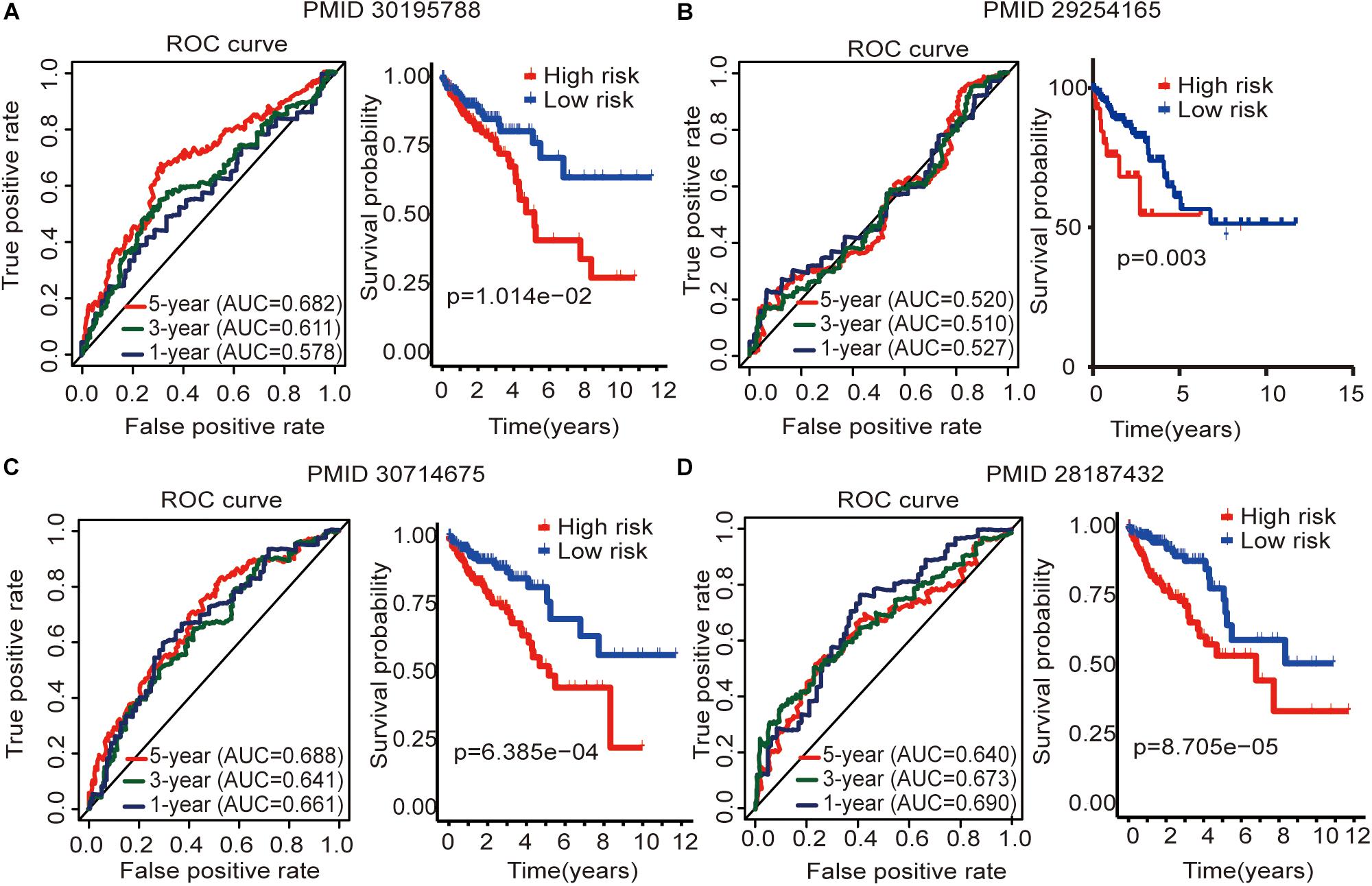
Figure 6. Four lncRNA signatures predict prognosis of colon cancer in the entire TCGA cohort. (A) Zhou et al. (2018) (PMID30195788) six-lncRNA signature in the entire TCGA cohort. (B) Xue et al. (2017) (PMID29254165) two-lncRNA signature in the entire TCGA cohort. (C) Zhang et al. (2019) (PMID30714675) five-lncRNA signature in the entire TCGA cohort. (D) Zeng et al. (2017) (PMID28187434) four-lncRNA signature in the entire TCGA cohort. The AUC value of the ROC curve is used to evaluate the ability to predict, and the survival curve is used to analyze the difference in prognosis between the high and low expression groups.
Relationship Between the Expression Levels of Nine lncRNAs and the Clinicopathological Characteristics and Survival Prognosis of Colon Cancer Patients
We compared the lncRNA expression levels in colon cancer tissues and normal colon tissues in the GEPIA database, and the results showed that AC008760.1, AC009237.14, AC083809.1, AL391422.4, AL445645.1, LINC01063, and LINC01234 were highly expressed in colon cancer, whereas AC016027.1 and LINC02381 exhibited low expression in colon cancer (Figure 7A). The expression of AC008760.1 was significantly correlated with the depth of colon cancer invasion (Figure 7B), and the expression of AC008760.1, AL445645.1, AC009237.14, AL391422.4, and LINC02381 was significantly associated with lymph node metastasis (Figure 7C). In addition, the expression of AC008760.1, AC009237.14, AL391422.4, and LINC01234 was significantly correlated with tumor cell metastasis (Figure 7D), and the expression of AC008760.1, AL445645.1, AC009237.14, and AL391422.4 was significantly related to the clinical stage of colon cancer (Figure 7E). The survival analysis showed that a high expression of AC008760.1 (Figure 8A), AC009237.14 (Figure 8B), AC083809.1 (Figure 8D), AL391422.4 (Figure 8E), AL445645.1 (Figure 8F), LINC01063 (Figure 8G), LINC01234 (Figure 8H), and LINC02381 (Figure 8I) was associated with a poor prognosis in colon cancer. The survival analysis results show that a low expression of AC016027.1 (Figure 8C) is associated with a good prognosis.
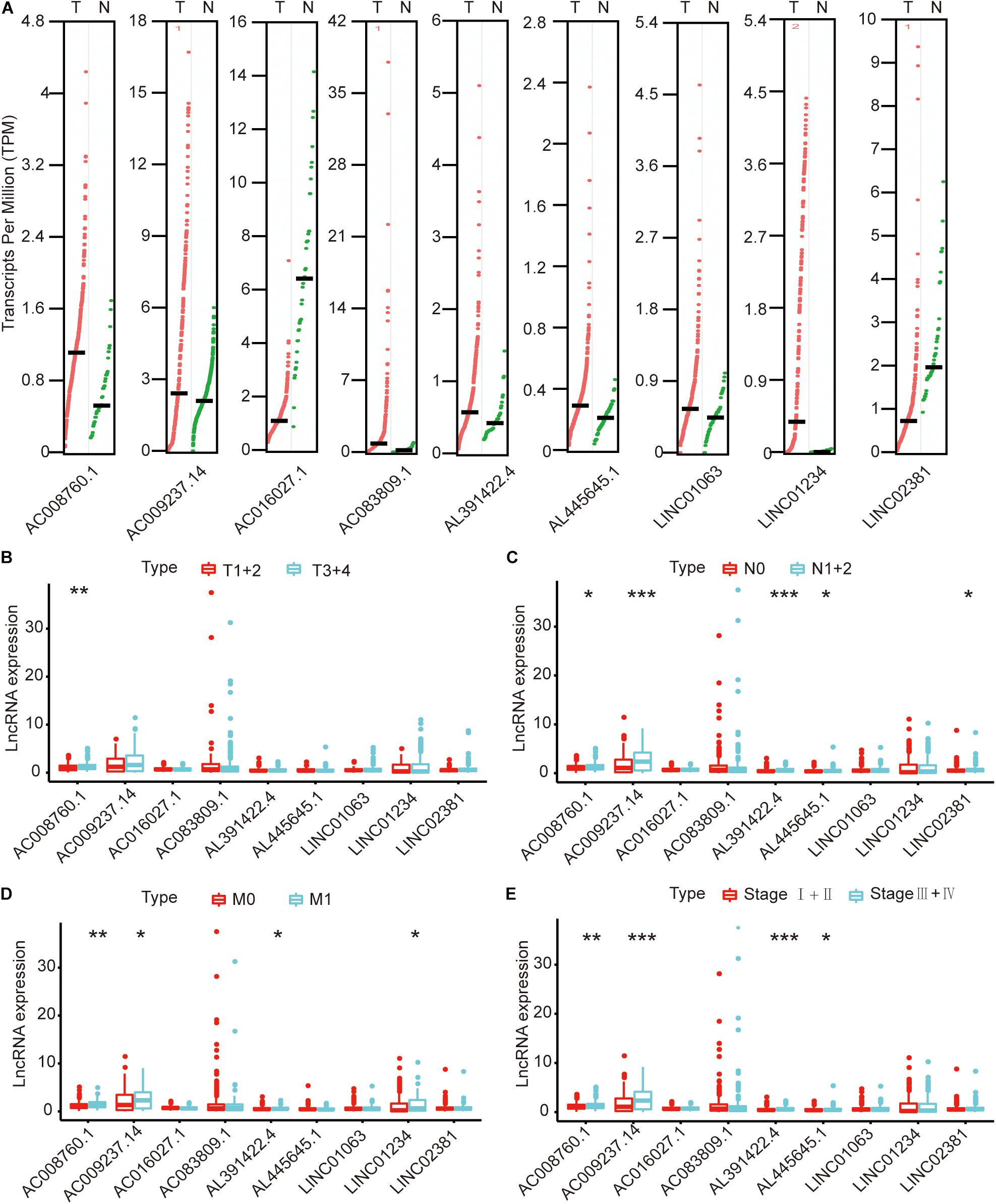
Figure 7. Relationship between nine lncRNAs and clinical characteristics of colon cancer in the entire TCGA cohort. (A) Expression levels of nine lncRNAs in the GEPIA database. (B) Relationship between the expression of nine lncRNAs and the depth of colon cancer tumors. (C) Relationship between the expression of nine lncRNAs and the number of lymphoma in colon cancer. (D) Relationship between the expression of nine lncRNAs and metastasis of colon cancer cells. (E) Relationship between the expression of nine lncRNAs and clinical stage of colon cancer. *p < 0.05, **p < 0.01, ***p < 0.001.
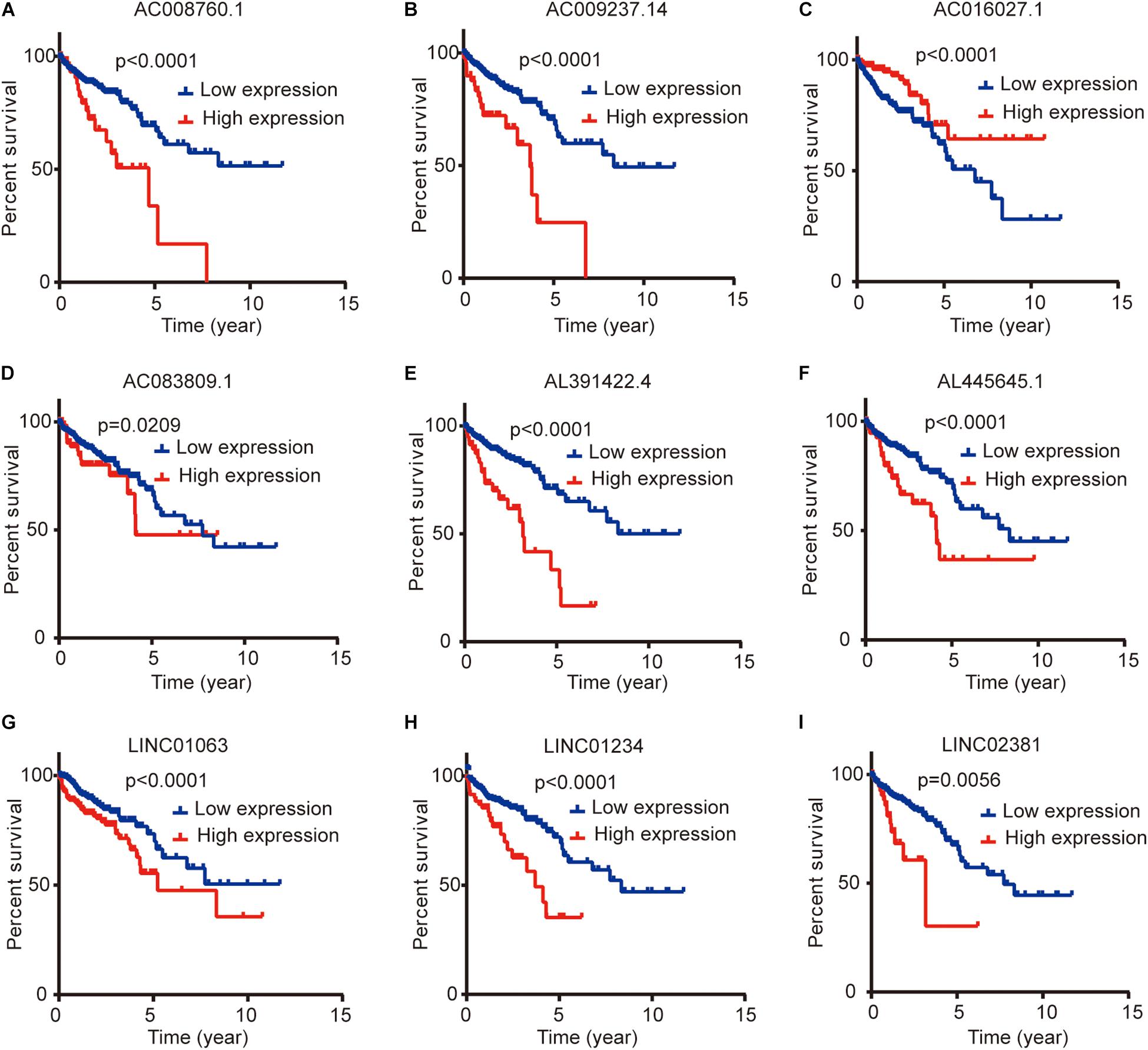
Figure 8. Relationship between the expression levels of nine lncRNAs and survival prognosis of colon cancer. (A,B,D–I) Low and high expression levels of AC008760.1, AC009237.14, AC083809.1, AL391422.4, AL445645.1, LINC01063, LINC01234, and LINC02381 are associated with significantly different prognosis, and the high expression group has a worse prognosis than the low expression group in colon cancer. (C) Prognosis significantly differs between groups with high expression and low expression of AC016027.1, and the low expression group has a worse prognosis than the high expression group.
Expression Level of Nine lncRNAs in Cell Lines as Detected by a qRT-PCR Assay
Finally, we detected the expression levels of nine lncRNAs in CCD-841CoN, HCT116, and SW480 by a qRT-PCR assay. The results showed that AC008760.1, AC009237.14, AC083809.1, AL391422.4, AL445645.1, LINC01063, LINC01234, and LINC02381 were highly expressed in HCT116 and SW480 and lowly expressed in CCD-841CoN. There were significant differences (Figure 9). However, the expression level of AC016027.1 was low in HCT116 and SW480 and high in CCD-841CoN. There were significant differences (Figure 9).
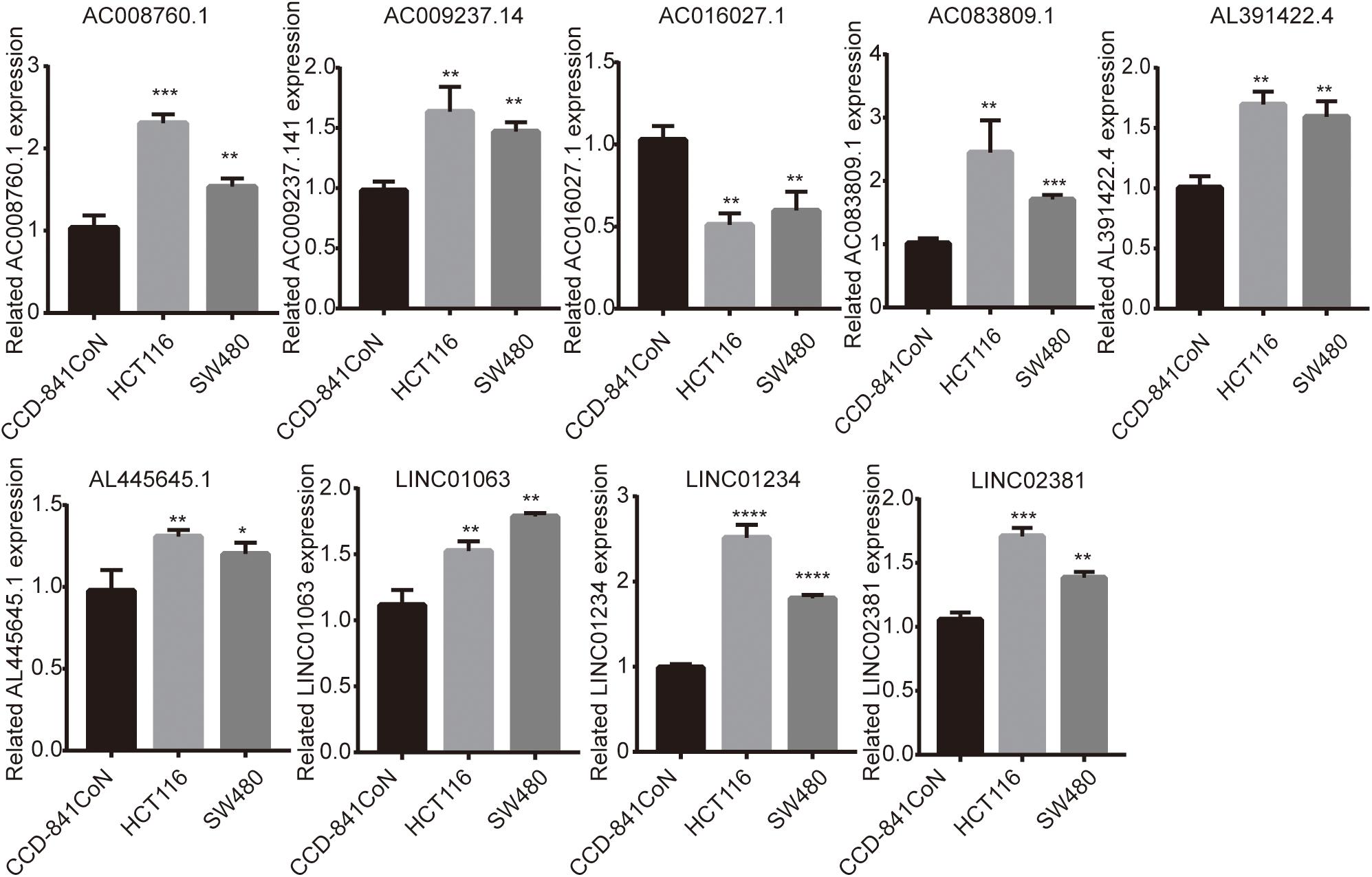
Figure 9. Expression of nine lncRNAs in colon cancer cell lines and colon cell lines. Expression levels of nine lncRNAs in colon cancer cell lines (HCT116 and SW480) significantly differed from those in colon cell lines (CCD-841CoN). AC008760.1, AC009237.14, AC083809.1, AL391422.4, AL445645.1, LINC01063, LINC01234, and LINC02381 were highly expressed in HCT116 and SW480. AC016027.1 was lowly expressed in HCT116 and SW480. *p < 0.05, **p < 0.01, ***p < 0.001, ****p < 0.0001.
Discussion
Colon cancer is a common malignant tumor of the digestive tract (Bray et al., 2018). The mechanisms underlying colon cancer development and progression remain unclear. Recent studies have found that cellular immune responses are involved in the development of cancer and may be among the factors influencing poor prognosis in cancer (Li et al., 2019; Thompson et al., 2019). Studies have found that lncRNAs are important regulators of the immune response in cancer. A related study reported that tumor cells use SNHG12 to evade immune-mediated attacks and enhance the immune response (Tamang et al., 2019). The lncRNA SNHG14 is involved in the development of diffuse large B-cell lymphoma and immune escape (Zhao et al., 2019). MIR155HG is involved in immune infiltration and immune checkpoint molecular expression in various cancers and may be a biomarker of tumor prognosis (Peng L. et al., 2019). These studies indicate that immune-related lncRNAs can serve as potential markers for cancer therapy and have an important impact on the survival prognosis of cancer patients. However, biomarkers that can effectively predict the survival prognosis of colon cancer patients are still lacking. Therefore, identifying effective colon cancer biomarkers is important.
The expression profile of 761 immune-related lncRNAs in colon cancer was identified by a correlation analysis of lncRNAs and IRGs. Nine immune-related lncRNAs were associated with OS in the training cohort by univariate Cox regression, lasso regression, and multivariate Cox regression analyses. The nine immune-related lncRNAs were used to construct a signature for the prediction of OS in colon cancer patients in the training cohort (Figure 2). The risk scores generated from the expression levels of these nine immune-related lncRNAs could accurately predict the OS of the patients in the training cohort at 1 year (AUC = 0.832), 3 years (AUC = 0.906), and 5 years (AUC = 0.891) (Figure 2A). The patients were divided into high- and low-risk groups based on their risk score (Figure 2B). There was a significant difference in prognosis between the high- and low-risk groups (Figure 2C). The immune-related lncRNA signature also performed well in the testing cohort and the entire TCGA cohort (Figures 2D–I). This result further shows that the immune-related lncRNA signature we constructed has a higher accuracy than the previously reported lncRNA signature.
To further explore the relationship between this immune-related lncRNA signature and the clinical characteristics of colon cancer, we analyzed the number of samples in the high- and low-risk groups according to age, gender, the tumor stage, tumor invasion (T), lymph node (N), and metastasis (M) in the training, testing, and entire TCGA cohorts. Combining the results of the above three cohorts showed a close association between the immune-related lncRNA signature model and the tumor stage and lymphatic metastasis (Figure 3 and Table 3). By analyzing the relationship between the expression of each lncRNA and the clinical characteristics, we also found that the expression of AC008760.1, AC009237.14, and AL391422.4 is related to lymphatic metastasis in colorectal cancer, distant metastasis of cancer cells, and clinicopathological staging, with a significant correlation. This finding suggests that the expression of these three lncRNAs may be related to the development of colon cancer and may promote metastasis in tumor cells, providing a potential target for further research investigating the mechanism underlying colon cancer development and metastasis.
Then, we analyzed the relationship between age, gender, tumor stage, and risk score in patients with colon cancer by univariate Cox regression analysis and multivariate Cox analysis in the three cohorts. The results showed that both the tumor stage and risk score had p < 0.05 in the univariate Cox regression analysis and multivariate Cox regression analysis in the three cohorts (Table 4), suggesting that the tumor stage and risk score may be independent prognostic factors in colon cancer patients. To determine which of the two independent prognostic factors can better predict OS in colon cancer patients, we constructed a tumor stage model in the three cohorts (Figure 4A). The AUC values of the time–ROC curve were used to assess the accuracy of the model. The results show that the 1-, 3-, and 5-year prediction accuracies of this model are lower than those of the immune-related lncRNA signature in the three cohorts. Then, we constructed a nomogram with the risk score for the OS prediction of colon cancer patients in the TCGA cohort (Figure 4B). We can initially assess the 1-, 3-, and 5-year OS of each patient based on their risk score. The survival prognosis of patients can be evaluated before surgery. Therefore, patients can have a certain understanding of their condition and may be more accurately treated.
Then, the GSEA revealed several significantly enriched pathway signatures. Patients with high-risk scores are mainly enriched in the complement and coagulation cascades, ECM receptor interaction, focal adhesion, hedgehog signaling pathway, MAPK signaling pathway, notch signaling pathway, pathways in cancer, and Wnt signaling pathway (Figure 5A). Studies have reported that these pathways are closely related to the immune process (Gao et al., 2019; Hanna et al., 2019; Ramesh et al., 2019; Yang et al., 2019). Patients with low-risk scores are mainly enriched in terpenoid backbone biosynthesis, steroid biosynthesis, porphyrin and chlorophyll metabolism, and the citrate cycle (Figure 5B). From this perspective, low-risk patients may benefit more from metabolic therapy, whereas high-risk patients may benefit more from immunotherapy. However, more effort is needed to investigate this relationship. Moreover, the results shed light on the potential molecular mechanisms of signatures that provide promising directions in immunotherapy. Previous studies have developed prognostic models of lncRNA signatures for colon cancer (Xue et al., 2017; Zeng et al., 2017; Zhou et al., 2018: Zhang et al., 2019). By comparing these models, we found that the immune-related lncRNA signature based on IRGs predicts 1-, 3-, and 5-year (Figure 2) prognostic capabilities that are higher than those in previous studies of lncRNA signatures (Figure 6). It is suggested that immune-related lncRNA signatures based on IRGs can better assess the prognosis of patients with colon cancer.
Finally, we analyzed the expression level of each lncRNA. The GEPIA database showed that AC008760.1, AC009237.14, AC083809.1, AL391422.4, AL445645.1, LINC01063, and LINC01234 were highly expressed in colon cancer and that AC016027.1 has low expression in colon cancer (Figure 7A). Moreover, the survival analysis showed that AC008760.1, AC009237.14, AC083809.1, AL391422.4, AL445645.1, LINC01063, and LINC01234 overexpression is associated with a poor prognosis, whereas AC016027.1 overexpression is associated with a good prognosis (Figure 8). This finding is consistent with the results of our previous Cox regression analysis (Figure 1). In the GEPIA database, LINC02381 was lowly expressed in colon cancer (Figure 7A), but both the Cox regression analysis and survival analysis showed that LINC02381 may be a procancer factor in colon cancer (Figure 1). In addition, we finally tested the expression of LINC02381 in cell lines and found that LINC02381 was highly expressed in colon cancer cell lines (Figure 9). Therefore, we speculate that this result may be due to too few normal colon samples in the GEPIA database, and more samples may need to be collected for verification in the future. Although our results may have potentially significant clinical implications, several issues need to be noted. First, some important immune genes may have been excluded before establishing the prognostic model, which may ultimately reduce the performance of the model. In addition, although immune regulation contributes to the development of colon cancer, more complex mechanisms may affect prognosis in colon cancer and require further exploration.
In summary, the immune-related lncRNA signature showed independent prognostic significance in colon cancer. The results of this study provide a method for predicting the prognosis and survival of colon cancer patients and may provide potential lncRNA targets for immunotherapy.
Data Availability Statement
Publicly available datasets were analyzed in this study. This data can be found here: https://cancergenome.nih.gov/, http://www.roadinstitute.org/gsea/msigdb/index.jsp.
Ethics Statement
Ethical review and approval was not required for the study on human participants in accordance with the local legislation and institutional requirements. Written informed consent for participation was not required for this study in accordance with the national legislation and the institutional requirements.
Author Contributions
SC sponsored the fund. YL, XP, and ZC designed research. YL and XP participated in the writing of the manuscript. SL participated in the research of data statistics and manuscript layout.
Funding
This work was supported by the Natural Science Foundation of Fujian Province of China [grant number 2019J01448].
Conflict of Interest
The authors declare that the research was conducted in the absence of any commercial or financial relationships that could be construed as a potential conflict of interest.
Acknowledgments
We thank the reviewers for their constructive comments.
Supplementary Material
The Supplementary Material for this article can be found online at: https://www.frontiersin.org/articles/10.3389/fgene.2020.00318/full#supplementary-material
Footnotes
- ^ https://cancergenome.nih.gov/
- ^ http://www.gencodegenes.org
- ^ http://www.broadinstitute.org/gsea/msigdb/index.jsp
- ^ http://gepia.cancer-pku.cn/index.html
References
Alboukadel, K., and Kosinski, M. (2019). survminer: Drawing Survival Curves using ’ggplot2’. R package version 0.4.4. Available online at: https://CRAN.R-project.org/package=survminer (accessed October 20, 2019).
Barbie, D. A., Tamayo, P., Boehm, J. S., Kim, S. Y., Moody, S. E., Dunn, I. F., et al. (2009). Systematic RNA interference reveals that oncogenic KRAS-driven cancers require TBK1. Nature 462, 108–112. doi: 10.1038/nature08460
Bermudez, M., Aguilar-Medina, M., Lizarraga-Verdugo, E., Avendano-Felix, M., Silva-Benitez, E., Lopez-Camarillo, C., et al. (2019). LncRNAs as regulators of autophagy and drug resistance in colorectal cancer. Front. Oncol. 9:1008. doi: 10.3389/fonc.2019.01008
Bray, F., Ferlay, J., Soerjomataram, I., Siegel, R. L., Torre, L. A., and Jemal, A. (2018). Global cancer statistics 2018: GLOBOCAN estimates of incidence and mortality worldwide for 36 cancers in 185 countries. CA Cancer J. Clin. 68, 394–424. doi: 10.3322/caac.21492
Chi, Y., Wang, D., Wang, J., Yu, W., and Yang, J. (2019). Long Non-Coding RNA in the pathogenesis of cancers. Cells 8:E1015,
Coebergh van den Braak, R. R. J., Sieuwerts, A. M., Lalmahomed, Z. S., Smid, M., Wilting, S. M., Bril, S. I., et al. (2018). Confirmation of a metastasis-specific microRNA signature in primary colon cancer. Sci. Rep. 8:5242. doi: 10.1038/s41598-018-22532-1
Cross, A. J., Wooldrage, K., Robbins, E. C., Kralj-Hans, I., MacRae, E., Piggott, C., et al. (2019). Faecal immunochemical tests (FIT) versus colonoscopy for surveillance after screening and polypectomy: a diagnostic accuracy and cost-effectiveness study. Gut 68, 1642–1652. doi: 10.1136/gutjnl-2018-317297
Frankish, A., Diekhans, M., Ferreira, A. M., Johnson, R., Jungreis, I., Loveland, J., et al. (2019). GENCODE reference annotation for the human and mouse genomes. Nucleic Acids Res. 47, D766–D773. doi: 10.1093/nar/gky955
Galamb, O., Bartak, B. K., Kalmar, A., Nagy, Z. B., Szigeti, K. A., Tulassay, Z., et al. (2019). Diagnostic and prognostic potential of tissue and circulating long non-coding RNAs in colorectal tumors. World J. Gastroenterol. 25, 5026–5048. doi: 10.3748/wjg.v25.i34.5026
Gao, J., Van Meter, M., Hernandez Lopez, S., Chen, G., Huang, Y., Ren, S., et al. (2019). Therapeutic targeting of Notch signaling and immune checkpoint blockade in a spontaneous, genetically heterogeneous mouse model of T-cell acute lymphoblastic leukemia. Dis. Models Mech. 12:dmm.040931. doi: 10.1242/dmm.040931
Geisler, S., and Coller, J. (2013). RNA in unexpected places: long non-coding RNA functions in diverse cellular contexts. Nat. Rev. Mol. Cell Biol. 14, 699–712. doi: 10.1038/nrm3679
Hanna, A., Metge, B. J., Bailey, S. K., Chen, D., Chandrashekar, D. S., Varambally, S., et al. (2019). Inhibition of Hedgehog signaling reprograms the dysfunctional immune microenvironment in breast cancer. Oncoimmunology 8:1548241. doi: 10.1080/2162402X.2018.1548241
Iasonos, A., Schrag, D., Raj, G. V., and Panageas, K. S. (2008). How to build and interpret a nomogram for cancer prognosis. J. Clin. Oncol. 26, 1364–1370. doi: 10.1200/JCO.2007.12.9791
Jerome, F., Hastie, T., and Tibshirani, R. (2010). Regularization paths for generalized linear models via coordinate descent. J. Stat. Softw. 33, 1–22.
Jiang, Z. J., Shen, Q. H., Chen, H. Y., Yang, Z., Shuai, M. Q., and Zheng, S. (2018). Galectin-1 Restores Immune Tolerance to Liver Transplantation Through Activation of Hepatic Stellate Cells. Cell Physiol. Biochem. 48, 863–879. doi: 10.1159/000491955
Jin, W. N., Ducruet, A. F., Liu, Q., Shi, S. X., Waters, M., Zou, M., et al. (2018). Activation of JAK/STAT3 restores NK-cell function and improves immune defense after brain ischemia. FASEB J. 32, 2757–2767. doi: 10.1096/fj.201700962R
Kawamura, T., Nakamura, S., Sone, D., Sakai, H., Amamiya, K., Inoue, N., et al. (2019). Risk of colorectal cancer for fecal immunochemistry test-positive, average-risk patients after a colonoscopy. J. Gastroenterol. Hepatol. 34, 532–536. doi: 10.1111/jgh.14517
Klement, J. D., Paschall, A. V., Redd, P. S., Ibrahim, M. L., Lu, C., Yang, D., et al. (2018). An osteopontin/CD44 immune checkpoint controls CD8+ T cell activation and tumor immune evasion. J. Clin. Invest. 128, 5549–5560. doi: 10.1172/JCI123360
Kuhn, M. (2019). caret: Classification and Regression Training. R package version 6.0-84. Available online at: https://CRAN.R-project.org/package=caret (accessed October 20, 2019).
Li, W., Wang, H., Ma, Z., Zhang, J., Ou-Yang, W., Qi, Y., et al. (2019). Multi-omics analysis of microenvironment characteristics and immune escape mechanisms of hepatocellular carcinoma. Front. Oncol. 9:1019. doi: 10.3389/fonc.2019.01019
Liu, J., and Du, W. (2019). LncRNA FENDRR attenuates colon cancer progression by repression of SOX4 protein. Oncol. Targets Ther. 12, 4287–4295. doi: 10.2147/OTT.S195853
Marshall, H. T., and Djamgoz, M. B. A. (2018). Immuno-oncology: emerging targets and combination therapies. Front. Oncol. 8:315. doi: 10.3389/fonc.2018.00315
Max, G., and Lumley, T. (2019). forestplot: Advanced Forest Plot Using ’grid’ Graphics. R package version 1.9. Available online at: https://CRAN.R-project.org/package=forestplot (accessed October 20, 2019).
Patrick, J. H., and Saha-Chaudhuri, P. (2013). survivalROC: Time-dependent ROC curve estimation from censored survival data. R package version 1.0.3. Available online at: https://CRAN.R-project.org/package=survivalROC (accessed October 20, 2019).
Peng, C. L., Zhao, X. J., Wei, C. C., and Wu, J. W. (2019). LncRNA HOTAIR promotes colon cancer development by down-regulating miRNA-34a. Eur. Rev. Med. Pharmacol. Sci. 23, 5752–5761. doi: 10.26355/eurrev_201907_18312
Peng, L., Chen, Z., Chen, Y., Wang, X., and Tang, N. (2019). MIR155HG is a prognostic biomarker and associated with immune infiltration and immune checkpoint molecules expression in multiple cancers. Cancer Med. 8, 7161–7173. doi: 10.1002/cam4.2583
Popovic, A., Jaffee, E. M., and Zaidi, N. (2018). Emerging strategies for combination checkpoint modulators in cancer immunotherapy. J. Clin. Invest. 128, 3209–3218. doi: 10.1172/JCI120775
Ramesh, A., Brouillard, A., Kumar, S., Nandi, D., and Kulkarni, A. (2019). Dual inhibition of CSF1R and MAPK pathways using supramolecular nanoparticles enhances macrophage immunotherapy. Biomaterials 227, 119559. doi: 10.1016/j.biomaterials.2019.119559
Ritchie, M. E., Phipson, B., Wu, D., Hu, Y., Law, C. W., Shi, W., et al. (2015). limma powers differential expression analyses for RNA-sequencing and microarray studies. Nucleic Acids Res. 43, e47. doi: 10.1093/nar/gkv007
Tamang, S., Acharya, V., Roy, D., Sharma, R., Aryaa, A., Sharma, U., et al. (2019). SNHG12: an LncRNA as a potential therapeutic target and biomarker for human cancer. Front. Oncol. 9:901. doi: 10.3389/fonc.2019.00901
Tang, Z., Li, C., Kang, B., Gao, G., Li, C., and Zhang, Z. (2017). GEPIA: a web server for cancer and normal gene expression profiling and interactive analyses. Nucleic Acids Res. 45, W98–W102. doi: 10.1093/nar/gkx247
Thompson, J. C., Hwang, W. T., Davis, C., Deshpande, C., Jeffries, S., Rajpurohit, Y., et al. (2019). Gene signatures of tumor inflammation and epithelial-to-mesenchymal transition (EMT) predict responses to immune checkpoint blockade in lung cancer with high accuracy. Lung Cancer 139, 1–8. doi: 10.1016/j.lungcan.2019.10.012
Wei, C., Liang, Q., Li, X., Li, H., Liu, Y., Huang, X., et al. (2019). Bioinformatics profiling utilized a nine immune-related long noncoding RNA signature as a prognostic target for pancreatic cancer. J. Cell. Biochem. 120, 14916–14927. doi: 10.1002/jcb.28754
Xu, W., Zhou, G., Wang, H., Liu, Y., Chen, B., Chen, W., et al. (2019). Circulating lncRNA SNHG11 as a novel biomarker for early diagnosis and prognosis of colorectal cancer. Int. J. Cancer 146, 2901–2912 doi: 10.1002/ijc.32747
Xue, W., Li, J., Wang, F., Han, P., Liu, Y., and Cui, B. (2017). A long non-coding RNA expression signature to predict survival of patients with colon adenocarcinoma. Oncotarget 8, 101298–101308. doi: 10.18632/oncotarget.21064
Yang, W., Li, Y., Gao, R., Xiu, Z., and Sun, T. (2019). MHC class I dysfunction of glioma stem cells escapes from CTL-mediated immune response via activation of Wnt/beta-catenin signaling pathway. Oncogene 39, 1098–1111. doi: 10.1038/s41388-019-1045-6
Yue, B., Liu, C., Sun, H., Liu, M., Song, C., Cui, R., et al. (2018). A Positive Feed-Forward Loop between LncRNA-CYTOR and Wnt/beta-catenin signaling promotes metastasis of colon cancer. Mol. Ther. 26, 1287–1298. doi: 10.1016/j.ymthe.2018.02.024
Zeng, J. H., Liang, L., He, R. Q., Tang, R. X., Cai, X. Y., Chen, J. Q., et al. (2017). Comprehensive investigation of a novel differentially expressed lncRNA expression profile signature to assess the survival of patients with colorectal adenocarcinoma. Oncotarget 8, 16811–16828. doi: 10.18632/oncotarget.15161
Zhang, H., Wang, Z., Wu, J., Ma, R., and Feng, J. (2019). Long noncoding RNAs predict the survival of patients with colorectal cancer as revealed by constructing an endogenous RNA network using bioinformation analysis. Cancer Med. 8, 863–873. doi: 10.1002/cam4.1813
Zhao, L., Liu, Y., Zhang, J., Liu, Y., and Qi, Q. (2019). LncRNA SNHG14/miR-5590-3p/ZEB1 positive feedback loop promoted diffuse large B cell lymphoma progression and immune evasion through regulating PD-1/PD-L1 checkpoint. Cell Death Dis. 10:731. doi: 10.1038/s41419-019-1886-5
Keywords: colon cancer, immune, LncRNA, signature, prognosis
Citation: Lin Y, Pan X, Chen Z, Lin S and Chen S (2020) Identification of an Immune-Related Nine-lncRNA Signature Predictive of Overall Survival in Colon Cancer. Front. Genet. 11:318. doi: 10.3389/fgene.2020.00318
Received: 26 November 2019; Accepted: 17 March 2020;
Published: 30 April 2020.
Edited by:
Juan Caballero, Universidad Autónoma de Querétaro, MexicoReviewed by:
Mohammad Taheri, Shahid Beheshti University of Medical Sciences, IranMeng Zhou, Wenzhou Medical University, China
Copyright © 2020 Lin, Pan, Chen, Lin and Chen. This is an open-access article distributed under the terms of the Creative Commons Attribution License (CC BY). The use, distribution or reproduction in other forums is permitted, provided the original author(s) and the copyright owner(s) are credited and that the original publication in this journal is cited, in accordance with accepted academic practice. No use, distribution or reproduction is permitted which does not comply with these terms.
*Correspondence: Shaoqin Chen, Y2hlbnNoYW9xbG4xNjEzQDE2My5jb20=
†These authors have contributed equally to this work