- 1Department of Pathology and Biomedical Science, University of Otago, Christchurch, New Zealand
- 2Department of Psychological Medicine, University of Otago, Christchurch, New Zealand
- 3Department of Psychology, University of Canterbury, Christchurch, New Zealand
Many patients prescribed an antidepressant stop taking it because of side effects. Genetic factors and psychological factors including state or trait anxiety, may explain variation in side effect outcomes. Our aim was to examine the relative contribution of genetic and psychological factors in people with self-reported antidepressant side effects. We undertook a case control study (n = 194) of people who took a selective serotonin reuptake inhibitor (SSRI) or serotonin/noradrenaline reuptake inhibitor (SNRI) in the past 2 years, recruited via social media advertising. Cases had previously not tolerated at least one trial of an SSRI or SNRI, evidenced by stopping the drug or reducing the dose by at least 50% because of a side effect. Control participants had taken an SSRI or SNRI but did not meet case criteria. Variation in the genes CYP2D6, CYP2C19, and CYP2C9 was analyzed by Sanger sequencing on DNA extracted from blood or saliva. Participants completed the Short Health Anxiety Inventory—18, K10, and NEO-FFI-3 personality questionnaire. Participants were 87.1% female. 70.8% had a current K10 score of 22 or more. There was no consistent evidence that cases had higher psychological distress, health anxiety, or neuroticism. There was low correspondence between participants’ CYP2D6, CYP2C19, and CYP2C9 phenotypes and their history of antidepressant tolerability. For this cohort of patients a history of not tolerating SSRI or SNRI therapy was not associated with variation in the pharmacogenes we tested, nor was it associated with health anxiety or neuroticism.
Introduction
Antidepressants are used to treat many mental health conditions, but they have only modest efficacy in most situations (Hidalgo et al., 2007; Fournier et al., 2010; Mitchell et al., 2013) and their use is limited by side effects (Papakostas, 2008). One way to improve tolerability and therefore effectiveness may be through personalized drug prescribing. This involves using individual genetic data to inform drug and dose selection (Singh, 2015; Bousman and Hopwood, 2016 Wishart, 2016). Over time, this technology has become much cheaper and more widely available. Genetic testing products are now increasingly being marketed direct to consumers, despite some ethical concerns about this practice (European Society of Human Genetics, 2010).
Most of the advancement in antidepressant drug therapy in the past 50 years has been in tolerability, not efficacy. However, the drug classes now in most common use, the selective serotonin reuptake inhibitors (SSRIs), and serotonin and noradrenaline reuptake inhibitors (SNRIs), are still not free of tolerability problems (Carvalho et al., 2016). Some produce unpleasant withdrawal syndromes (Fava et al., 2015; Fava et al., 2018) while most are associated with side effects at higher doses, including sweating, sexual dysfunction, and in some cases serotonin toxicity (Bet et al., 2013). Cardiovascular, hepatotoxic, and metabolic effects are further potential issues (Carvalho et al., 2016).
The SSRIs and SNRIs are all metabolized by cytochrome P450 (CYP) enzymes in the liver, particularly CYP2D6, CYP2C19, and CYP2C9. The relative contribution of each enzyme varies between drugs. Genetic variation in these CYP enzymes produces individual variation in drug clearance (Tracy et al., 2016). In some ethnic groups, reduced activity in one or more CYP enzymes is common (Hicks et al., 2015). For example, about 5–7% of Caucasians are CYP2D6 poor metabolizers (PMs) (Tamminga et al., 2001), suggesting they may be less likely to tolerate drugs metabolized by CYP2D6 such as the SSRI fluoxetine.
Genetically informed prescribing guidelines are now available, such as those from the Clinical Pharmacogenetics Implementation Consortium (CPIC; www.cpicpgx.org). These guidelines help prescribers choose the “optimal” antidepressant and its starting dose for patients with known CYP phenotypes (Hicks et al., 2015). However, while pharmacogenetic testing is becoming more common, uptake has been low even among psychiatrists, suggesting clinicians either have not yet seen the value of this testing, or cannot easily access it (Foulds et al., 2016).
Patients who have already had problems tolerating an antidepressant are an important group because they are at risk of poorer overall clinical outcomes (Papakostas, 2008; Maggo et al., 2019). While it seems logical that CYP variants involving reduced drug clearance would be over-represented in this group, it is not well established that this is actually the case. Furthermore, other factors such as patient expectations, prior conditioning, anxiety, and somatization also influence drug tolerability (Barsky et al., 2002), potentially masking any effect of CYP status.
On this background, we tested two hypotheses regarding patients with a history of difficulty tolerating treatment with an SSRI or SNRI:
1. That compared to people who have tolerated antidepressant therapy, those with side effects are more likely to have genetic variants that adversely affect function of the drug metabolizing enzymes CYP2D6, CYP2C19, or CYP2C9.
2. That markers of state or trait anxiety (high scores on measures of neuroticism, health anxiety or psychological distress) are over-represented among people who have difficulty tolerating drug treatment.
Methods
Design
A case-control design was used. The New Zealand Northern B Health and Disability Ethics Committee (18/NTB/21) approved the study. Reporting was intended to conform to STROBE guidelines (von Elm et al., 2007).
Cases were those participants who reported any lifetime history of “not tolerating” any SSRI or SNRI, which was defined as having taken at least 1 dose and subsequently having discontinued the drug or reduced its dose by at least 50% (while taking a dose less than or equal to the published maximum dose) because of a drug side effect.
Control participants were those who had a history of SSRI or SNRI exposure but had no such history of tolerability problems. This definition of “caseness” was chosen because SSRIs and SNRIs have a broad range of possible side effects, and it would have been difficult to define case inclusion criteria and severity thresholds based on all possible side effects.
Setting and Participants
A “convenient” sample was obtained via social media advertising on Facebook using two strategies:
1. Targeted information about the study was made available to a group of primarily New Zealand-based social media users with an existing connection to a Facebook group with an interest in mental health.
2. Social media advertising on Facebook using keywords related to depression and antidepressant therapy.
To be included in the study, participants needed to fulfill the following criteria:
1. Capacity to consent to providing a saliva or blood sample for pharmacogenetic testing
2. Age 16 or over
3. Resident in New Zealand
4. Taken at least one dose of a selective serotonin reuptake inhibitor (SSRI) and/or selective serotonin and noradrenaline reuptake inhibitor (SNRI) within the past 2 years
5. Able to complete personal health and psychometric assessment questionnaires online or on a computer.
Drug Response Information
Participants were asked about their current and lifetime history of antidepressant use, including the names and doses of all antidepressant drugs previously taken, the duration of each treatment trial, and why any drugs were stopped or reduced in dose. For those who had previously stopped a drug or reduced the dose, the reason for this was recorded as being either primarily because of a drug side effect or for other reasons (including lack of efficacy). To minimize respondent burden, participants were asked to describe the side effect(s) leading to discontinuation in their own words. Where information provided by participants was unclear or incomplete, participants’ electronic medical records were consulted.
Genotyping and Phenotyping
Genomic DNA was extracted from peripheral blood using a method modified from Miller et al. (1988). This protocol consists of a salting-out method followed by a phenol-chloroform purification step. DNA extraction from saliva was carried out according to the manufacturer’s instructions (Oragene OG-250 kit; DNA Genotek, ON, Canada). Protocol: (http://www.dnagenotek.com/US/pdf/PD-PR-006.pdf)
Genetic analysis was conducted by Sanger sequencing for common variants in CYP2D6, CYP2C9, and CYP2C19 genes. CYP2D6 genotyping was conducted using a two-stage PCR. The first step used a previously described method (Wright et al., 2010) to isolate an amplicon approximately 6.6 kb in length. This step isolates a CYP2D6 product from a neighboring CYP2D7 pseudogene. This step also allows the identification of CYP2D6 duplication or deletion alleles using specific primers. In brief, the initial PCR consisted of a 10 µl reaction which was set-up as follows: 1X KAPA LR reaction buffer (Kapa Biosystems, Wilmington, USA), 1.75 mM Mg2+, 0.3 mM of each deoxynucleoside triphosphate (dNTP), 0.4 µM of each 6.6 kb primer, 0.3 µM of duplication or deletion primers, 1 M betaine, 0.25 U of KAPA LR DNA (Kapa Biosystems, Wilmington, USA) polymerase, and 50 ng of DNA. Cycling conditions included initial heating to 94°C for 3 min, followed by 35 cycles of 94°C for 25 s, 68°C for 10 s, and 68°C for 7 min, and a final elongation step of 72°C for 7 min. Four µl of the initial PCR product was run on a 1% agarose gel to confirm the successful amplification of a 6.6 kb product and identification of any 3.5 kb duplication or deletion bands. Duplication or deletion alleles were confirmed by comparison with in-house controls (known samples with CYP2D6 duplication or deletion). The second stage of the PCR utilized a 1,000 fold diluted PCR product (6.6 kb PCR product from first stage) to conduct a nested PCR to cover the CYP2D6 gene. For the second stage, a standard 20 µl reaction was set-up as follows: 1X Fisher Biotec reaction buffer, 2.5 ng/µl template, 0.2 mM of each dNTP, 0.2 µM of each forward and reverse primer, 1.5 mM Mg2+, and 0.025 u/µl of TAQ-TI DNA polymerase (Fisher Biotec, Wembley, WA, Australia). This method allows for the identification of common alleles referred to as *2, *3, *4, *6, *7, *8, *9, *10, and *41, as well as other variants not often assessed in commonly available assay kits.
CYP2C19 (*2–*8 and *17) and CYP2C9 (*2, *3, *8) genotyping was conducted to assess common variants known to affect drug pharmacokinetics. A 20 µl PCR reaction was set-up as described above with CYP2C19 and CYP2C9 specific primers. Cycling conditions were as follows: initial heating to 94°C for 2 min, followed by 15 cycles of 94°C for 15 s, 65°C for 15 s (reducing by 1 degree per cycle), 72°C for 1 min. Followed by 20 cycles of 94°C for 15 s, 50°C for 15 s, and 72°C for 1 min.
After confirmation of PCR products on a 1% agarose gel, PCR products were prepared for bi-directional Sanger sequencing which was carried out on an Applied Biosystems 3130xl genetic analyzer for capillary electrophoresis. Bioinformatic analysis of generated sequences was done with the software Geneious V8.1.9 (Biomatters, Ltd., Auckland, New Zealand). In brief, generated sequencing files were aligned against the reference gene sequences from the National Center for Biotechnology Information (NCBI) (https://www.ncbi.nlm.nih.gov/gene) of CYP2D6 (ID: 1565), CYP2C19 (ID: 1557) and CYP2C9 (ID: 1559). These reference gene sequences were annotated with variants from the Pharmacogene Variation (PharmVar) consortium (www.pharmvar.org) (Gaedigk et al., 2018). Participant samples were assigned “star” genotypes using translation tables available on PharmGKB. For example the CYP2D6 translation table is available here (https://www.pharmgkb.org/gene/PA128/haplotype) (Whirl-Carrillo et al., 2012).
CYP2D6, CYP2C9, and CYP2C19 metabolizer status was assigned using CPIC (Clinical Pharmacogenetics Implementation Consortium) guidelines (Caudle et al., 2017).
Psychometric Measures
The following measures were obtained using online or pen-and-paper self-report instruments:
K10: 10-item measure of past 1-month psychological distress (Kessler et al., 2003). Each item is scored from 1 to 5 giving a possible range of 10–50 on the whole instrument. Scores of 22 or more denote “high psychological distress” (Oakley-Browne et al., 2010)
Short Health Anxiety Inventory 18-item version (SHAI-18) (Salkovskis et al., 2002)
Personality traits: NEO-FFI 60-item version (McCrae and Costa, 2004)
Bias
Sampling via social media is likely to identify potential participants who are active on social media platforms and interested in mental health topics. This convenient sampling strategy was chosen because we wanted to obtain a community sample of people who had taken an antidepressant and this was the most efficient way of doing so. Identifying patients via clinicians in primary or secondary care would have identified patients with more severe or unusual patterns of drug response, and possibly higher levels of distress, which was not the intended target population.
Study Size
A power analysis was performed a priori and was used to guide recruitment. Assuming typical frequencies of uncommon CYP phenotypic variants being 5% in the control population, a total sample of 200 (125 cases and 75 controls) would give 80% power (with 5% type I error rate) to detect uncommon phenotypic variants which are present in at least 10% of cases. We considered this difference would be clinically significant if we were to detect it.
Quantitative Variables and Statistical Methods
All analyses were conducted using IBM SPSS 25. Chi-square tests or independent sample t-tests were used for statistical comparisons between cases and controls. Fisher’s exact test was used to compare the frequency distribution of CYP2D6, CYP2C19, and CYP2C9 phenotypes between cases and controls.
Results
The final sample size fell slightly short of the a priori target (n = 200) because of dropout between the initial enrolment phase and genetic analysis. Table 1 shows the characteristics of participants included in the case (n = 89) and control (n = 105) groups. Participants were predominantly female (87.1%) and they were aged 17 to 67 (mean 32.7). With respect to ethnicity, 70% of study participants identified as either European or New Zealand-European, 11% as Maori or Pacifica and the remainder consisted of Asian (4%) or unspecified ethnic groups. Current antidepressant use was reported by 96/105 (93.1%) controls and 68/89 (76.1%) cases.
Among the cases, 28/89 (31.4%) had not tolerated fluoxetine at some time in the past (including three CYP2D6 PMs and one CYP2C19 PM); 42/89 (47.2%) had not tolerated citalopram or escitalopram (including six CYP2D6 PMs and one CYP2C19 PM); and 14/89 (15.7%) had not tolerated sertraline (one CYP2D6 PM, one CYP2C19 PM, and one participant who was a PM for both genes). Among those who did not tolerate paroxetine (9/89; 10.1%) or venlafaxine (9/89; 10.1%) there were no CYP2D6 or CYP2C19 PMs. No participants reported exposure to fluvoxamine, desvenlafaxine, levomilnacipran, or duloxetine as these drugs are not available in New Zealand.
Table 2 shows the distribution of CYP2D6, CYP2C19, and CYP2C9 phenotypes [PM; IM; NM; RM (CYP2C19 only) and UM] for cases and controls. There were no statistically significant differences in phenotype distribution between cases and controls for any of the CYP genes.
Cases and controls also did not differ significantly on NEO-FFI-3 personality dimensions or SHAI scores (Table 3). High levels of psychological distress were present across the whole sample, with 70.3% scoring 22 or more (denoting high distress) and 39.5% scoring 30 or more (denoting very high distress) (Oakley-Browne et al., 2010).
Table 4 presents case series data from participants identified as PMs for CYP2D6 (n = 15), CYP2C19 (n = 6), or CYP2C9 (n = 7) with one participant being a PM for both CYP2D6 and CYP2C19. Notably, 7/15 CYP2D6 PMs reported tolerability problems with drugs for which CYP2D6 is not the major pathway for metabolism (escitalopram, citalopram, or sertraline) while three reported tolerating moderate to high doses of paroxetine or fluoxetine, which are affected by CYP2D6. Similarly, while CYP2C19 PMs were uncommon (n = 6), one CYP2C19 PM tolerated escitalopram (metabolized to a large extent by CYP2C19) but not fluoxetine (minimally affected by CYP2C19). Two CYP2C19 PMs were currently taking a drug metabolized by CYP2C19, but one of these participants had reduced the dose of sertraline from 150 to 100 mg in response to serotonergic side effects.
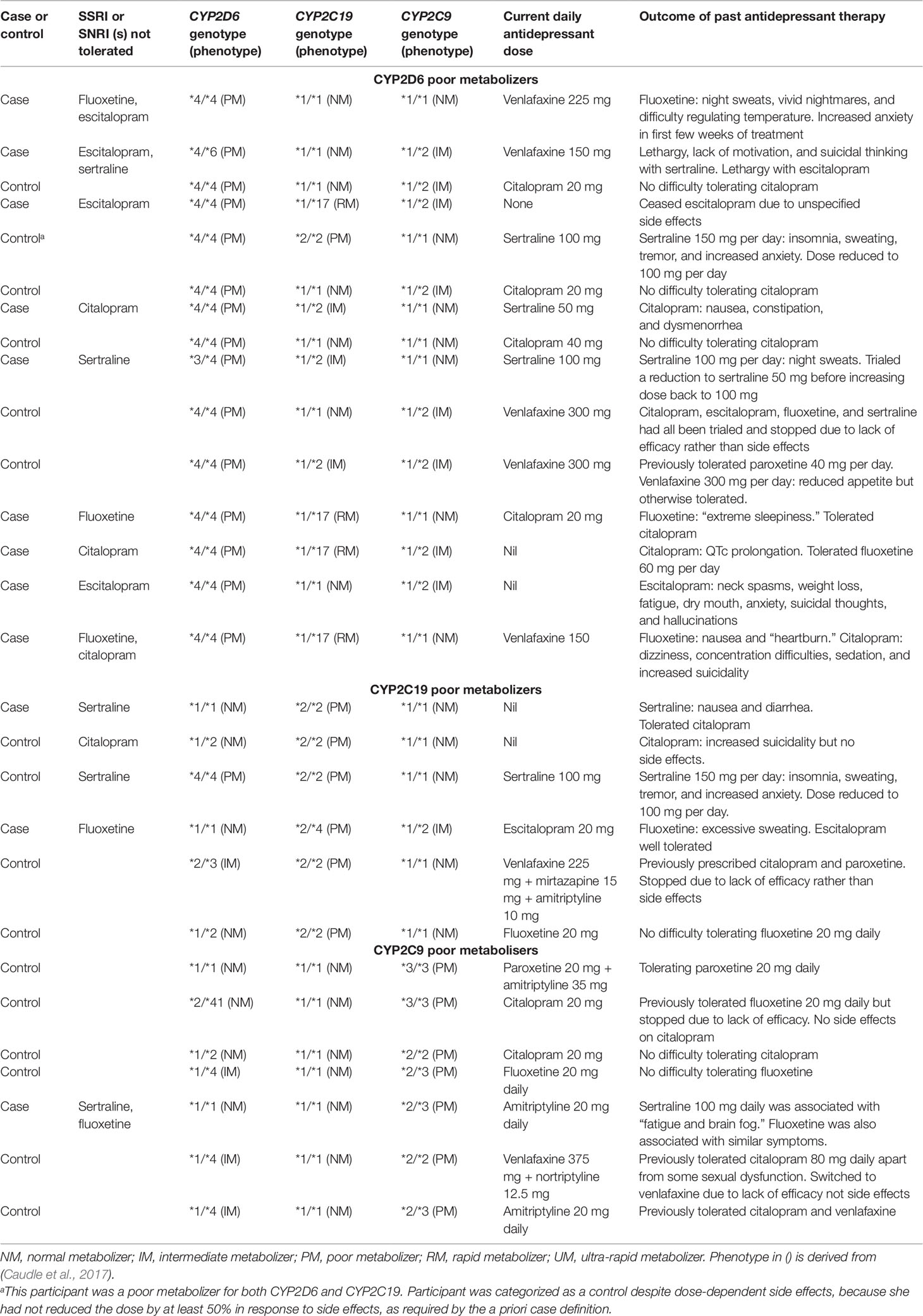
Table 4 Clinical histories of participants identified as CYP2D6, CYP2C9, or CYP2C19 poor metabolizers.
No CYP2D6 PMs reported currently taking a drug whose metabolism was substantially affected by CYP2D6: their current antidepressant therapy was citalopram (n = 4), sertraline (n = 3), venlafaxine (n = 5), no antidepressant (n = 2), and in one participant, it was unclear.
Discussion
The main premise of personalized antidepressant prescribing is that it helps to improve drug tolerability and avoid toxicity. While improved remission rates have been reported using genetic decision support algorithms (Singh, 2015), this finding has not yet been reliably replicated and the area remains somewhat controversial (Bousman and Hopwood, 2016; Singh and Bousman, 2017; Bousman and Muller, 2018). The clinical utility of such decision support tools is predicated on the belief that most antidepressant intolerability is linked to known genetic variants in pharmacogenes, which can be easily and cheaply detected. However, if antidepressant tolerability is not strongly related to genetic variability, such decision support tools may do little to improve antidepressant tolerability in a pre-emptive testing scenario. They may therefore be more valuable as an ad hoc tool in selected high-risk clinical samples (Nassan et al., 2016). Furthermore, while the premise of the technology is that it improves clinical outcomes and therefore lowers costs, it increases the complexity of clinical care. The technology might also increase inequality if it is only available in high-income countries or to more privileged or educated patient groups. Direct-to-consumer genetic testing raises even more complex ethical issues, and it has been argued it should be accompanied by genetic counseling (Bousman and Hopwood, 2016; Bousman et al., 2019).
We observed a low correlation between phenotypes and self-reported adverse outcomes among CYP2D6 and CYP2C19 PMs identified in our cohort. For example 8 of the 15 CYP2D6 PMs had experienced tolerability problems with an antidepressant not metabolized CYP2D6, i.e., citalopram or sertraline (Hicks et al., 2015). Conversely, several CYP2D6 PMs had no apparent trouble tolerating moderate to high doses of antidepressants for which CYP2D6 PM status is thought to induce side-effects, and for which CPIC guidelines recommend an alternative antidepressant (Hicks et al., 2015). The findings for CYP2C19 PMs were similarly inconsistent, albeit that their smaller numbers makes them harder to interpret. With respect to CYP2C19 and citalopram/escitalopram, a recent meta-analysis of over 4,000 patients by Fabbri et al. (2018) reported that compared to normal metabolizers, CYP2C19 PMs had a higher risk of gastrointestinal, neurological, and sexual side effects. However, CYP2C19 PMs had significantly increased rates of remission as well as marked improvement in symptoms of depression when compared with normal metabolizers (Fabbri et al., 2018).
Prior research indicates that the SNPs we investigated in CYP2D6 and CYP2C19 genes are associated with significant changes in plasma levels of SSRIs and SNRIs (Preskorn, 2003a; Preskorn, 2003; Gardiner and Begg, 2006; Shams et al., 2006). However, consistent with our findings, some previous studies found these SNPs are not predictive of side effects or antidepressant efficacy (Hodgson et al., 2015; Taranu et al., 2017). In addition, Serretti et al. (2009) showed no association between pharmacogenes (2D6, 2C19, 2C9, and 1A2) and response or remission to antidepressants (Serretti et al., 2009). The lack of an apparent signal in our study could also be explained by a “nocebo effect”(18) from antidepressants which overshadowed more specific dose-dependent side effects. Nocebo effects involve non-specific side effects which patients report after a treatment starts. They are analogous to the non-specific benefits which are often labeled as placebo effects. Although nocebo effects have been less well studied than placebo effects, the phenomenon appears more common in women and those with psychological symptoms (Wells and Kaptchuk, 2012), who made up the majority of our sample.
Despite most participants in this study currently being prescribed an antidepressant or having recently taken one, over two thirds were still reporting high psychological distress. While it is possible that people with a poor response to antidepressant treatment were more likely to take part in the study, it does speak to the ineffectiveness of these drugs for many people. Contrary to our a priori hypothesis, psychological distress, health anxiety, and trait anxiety (neuroticism) were not higher in cases compared to controls suggesting these psychological factors did not explain why some participants had not tolerated antidepressants. This is surprising since psychological factors are thought to affect drug tolerability in general (Barsky et al., 2002). Furthermore, the mean level of health anxiety among cases was well below the levels previously reported in patients with hypochondriasis (Alberts et al., 2013). It is possible the high current distress levels across the whole sample created a “ceiling effect” which obscured between-group differences in anxiety. Alternatively, prescribers are often skilled at detecting anxiety-prone patients: the lack of difference in state and trait anxiety measures between cases and controls might therefore simply reflect prescribing practices to avoid drug intolerability in patients whose expectations made them at risk of side effects.
The limitations of this study include the use of a non-random sampling strategy, which resulted in a low proportion of male participants in particular. Caution is therefore needed in generalizing the findings to a wider population of people who have taken antidepressants. The heterogeneity of the drug exposure history in the sample was a further limitation: since patients had failed to tolerate a range of SSRIs and SNRIs, each with different CYP metabolism, it is possible this heterogeneity overshadowed any signal for individual drugs. Due to budgetary constraints, our study did not assess antidepressant or metabolite levels in participants. Future studies assessing antidepressant adverse drug reactions (ADRs) or efficacy should consider this during the design phase.
An initial power analysis conducted assuming typical frequencies of uncommon CYP gene variants being 5% in the control population suggested that a sample of 125 cases would give 80% power (with 5% type I error rate) to detect a gene variant which is present in 10% of cases, a difference we considered would be clinically significant. However, our recruitment of cases was not focused on a specific drug-CYP-ADR, which needs to be considered in the future. Alternatively, a much larger sample might have allowed more robust subgroup analyses according to the drug(s) that were not tolerated and also allowed for analysis of phenoconversion by analysis of concomitant medications. Information about drug exposure was taken from self-report, and while most participants’ reports were likely to have been accurate, this was not verified against medical records except in cases where data were unclear. We have previously reported (Maggo et al., 2019) a high burden of pharmacogenetic variation in common CYP450 genes in patients who reported ADRs to commonly prescribed psychiatric medication. The major difference being, this small cohort of patients was selected by a specialist mental health pharmacist, and not self-referred as in the current study. Finally, we elected to use a clear behavioral phenotype (antidepressant reduction or discontinuation) rather than a structured adverse effect reporting tool, but this might have led to under-recognition of a subset of adverse effects which were more specific indicators of drug toxicity, for example serotonin toxicity symptoms.
Several clinical trials on genetically informed antidepressant prescribing have been either recently completed or are still recruiting (see www.clinicaltrials.gov). As the findings from these studies become available, the role of pharmacogenetic testing to guide antidepressant therapy will become clearer. Our findings suggest that most patients who self-report difficulty tolerating SSRI or SNRI therapy recruited through social media do not have a clear pharmacogenetic explanation for this. However, as already discussed, sample-size and methodological limitations do not allow strong conclusions to be made from the present study.
Data Availability Statement
The datasets for this manuscript are not publicly available because they may contain identifying participant information. Anonymised data such as phenotype/genotype and case/control classification can be requested. Requests to access the datasets should include a letter indicating the intended use and appropriate approval by your institution. This should be directed to the corresponding author.
Ethics Statement
The studies involving human participants were reviewed and approved by The New Zealand Northern B Health and Disability Ethics Committee. The patients/participants provided their written informed consent to participate in this study. Written informed consent was obtained from the individual(s) for the publication of any potentially identifiable images or data included in this article.
Author Contributions
SM: Recruited and consented the 194 study participants, collated psychometric and personal information, conducted genetic testing, assigned phenotypes and assisted in the write-up of the manuscript. MK: Provided advice on pharmacogenetic aspects of this manuscript. ZB: Assisted in the collation of the NEO-FFI assessment. AM: Assisted in the extraction of DNA and genetic testing of samples. JR: Assisted with social media advertisement and recruitment of study participants. RM: Provided professional unbiased input on assignment of participants as cases or control. JF: Principal investigator and holder of the research grant produced the first draft of this manuscript, conducted all statistical testing and collation of results into tables.
Funding
This study was funded by a University of Otago Research Grant. ZB’s salary was funded by a Summer Studentship jointly funded through University of Canterbury Foundation and Canterbury Medical Research Foundation. SM’s salary was partly funded by the Jim and Mary Carney Charitable Trust (Whangarei, New Zealand).
Conflict of Interest
The authors declare that the research was conducted in the absence of any commercial or financial relationships that could be construed as a potential conflict of interest.
Acknowledgments
We acknowledge the participants in the study, without whom this research could not have been done. We also acknowledge the Nicholls Centre at the University of Otago, Christchurch for providing assistance with phlebotomy services as required.
References
Alberts, N. M., Hadjistavropoulos, H. D., Jones, S. L., Sharpe, D. (2013). The short health anxiety inventory: a systematic review and meta-analysis. J. Anxiety Disord. 27 (1), 68–78. doi: 10.1016/j.janxdis.2012.10.009
Barsky, A. J., Saintfort, R., Rogers, M. P., Borus, J. F. (2002). Nonspecific medication side effects and the Nocebo phenomenon. JAMA 287 (5), 622–627. doi: 10.1001/jama.287.5.622
Bet, P. M., Hugtenburg, J. G., Penninx, B. W. J. H., Hoogendijk, W. J. G. (2013). Side effects of antidepressants during long-term use in a naturalistic setting. Eur. Neuropsychopharmacol. 23 (11), 1443–1451. doi: 10.1016/j.euroneuro.2013.05.001
Bousman, C. A., Hopwood, M. (2016). Commercial pharmacogenetic-based decision-support tools in psychiatry. Lancet Psychiatry 3 (6), 585–590. doi: 10.1016/S2215-0366(16)00017-1
Bousman, C. A., Muller, D. J. (2018). Pharmacogenetics in psychiatry: a companion, rather than competitor, to protocol-based care. JAMA Psychiatry 75 (10), 1090. doi: 10.1001/jamapsychiatry.2018.2344
Bousman, C., Maruf, A. A., Muller, D. J. (2019). Towards the integration of pharmacogenetics in psychiatry: a minimum, evidence-based genetic testing panel. Curr. Opin. Psychiatry 32 (1), 7–15. doi: 10.1097/YCO.0000000000000465
Carvalho, A. F., Sharma, M. S., Brunoni, A. R., Vieta, E., Fava, G. A. (2016). The safety, tolerability and risks associated with the use of newer generation antidepressant drugs: a critical review of the literature. Psychother. Psychosomatics 85 (5), 270–288. doi: 10.1159/000447034
Caudle, K. E., Dunnenberger, H. M., Freimuth, R. R., Peterson, J. F., Burlison, J. D., Whirl-Carrillo, M. (2017). Standardizing terms for clinical pharmacogenetic test results: consensus terms from the Clinical Pharmacogenetics Implementation Consortium (CPIC). Genet. Med. 19 (2), 215–223. doi: 10.1038/gim.2016.87
European Society of Human Genetics (2010). Statement of the ESHG on direct-to-consumer genetic testing for health-related purposes. Eur. J. Hum. Genet. 18 (12), 1271–1273. doi: 10.1038/ejhg.2010.129
Fabbri, C., Tansey, K. E., Perlis, R. H., Hauser, J., Henigsberg, N., Maier, W. (2018). Effect of cytochrome CYP2C19 metabolizing activity on antidepressant response and side effects: meta-analysis of data from genome-wide association studies. Eur. Neuropsychopharmacol. 28 (8), 945–954. doi: 10.1016/j.euroneuro.2018.05.009
Fava, G. A., Gatti, A., Belaise, C., Guidi, J., Offidani, E. (2015). Withdrawal symptoms after selective serotonin reuptake inhibitor discontinuation: a systematic review. Psychother. Psychosomatics 84 (2), 72–81. doi: 10.1159/000370338
Fava, G. A., Benasi, G., Lucente, M., Offidani, E., Cosci, F., Guidi, J. (2018). Withdrawal symptoms after serotonin-noradrenaline reuptake inhibitor discontinuation: systematic review. Psychother. Psychosomatics 87 (4), 195–203. doi: 10.1159/000491524
Foulds, J. A., Maggo, S. D., Kennedy, M. A. (2016). Personalised prescribing in psychiatry: has pharmacogenomics delivered on its promise? Aust. New Z. J. Psychiatry. 50 (6), 509–510. doi: 10.1177/0004867416640099
Fournier, J. C., DeRubeis, R. J., Hollon, S. D., Dimijian, S., Amsterdam, J, D., Shelton, R. C., (2010). Antidepressant drug effects and depression severity: a patient-level meta-analysis. JAMA 303 (1), 47–53. doi: 10.1001/jama.2009.1943
Gaedigk, A., Ingelman-Sundberg, M., Miller, N. A., Leeder, J. S., Whirl-Carrillo, M., Klein, T. E. (2018). The pharmacogene variation (PharmVar) consortium: incorporation of the human cytochrome P450 (CYP) allele nomenclature database. Clin. Pharmacol. Ther. 103 (3), 399–401. doi: 10.1002/cpt.910
Gardiner, S. J., Begg, E. J. (2006). Pharmacogenetics, drug-metabolizing enzymes, and clinical practice. Pharmacol. Rev. 58 (3), 521–590. doi: 10.1124/pr.58.3.6
Hicks, J. K., Bishop, J. R., Sangkuhl, K., Müller, D. J., Ji, Y., Leckband, S. G. (2015). Clinical Pharmacogenetics Implementation Consortium (CPIC) guideline for CYP2D6 and CYP2C19 genotypes and dosing of selective serotonin reuptake inhibitors. Clin. Pharmacol. Ther. 98 (2), 127–134. doi: 10.1002/cpt.147
Hidalgo, R. B., Tupler, L. A., Davidson, J. R. T. (2007). An effect-size analysis of pharmacologic treatments for generalized anxiety disorder. J. Psychopharmacol. 21 (8), 864–872. doi: 10.1177/0269881107076996
Hodgson, K., Tansey, K. E., Uher, R., Dernovsek, M. Z., Mors, O., Hauser, J. (2015). Exploring the role of drug-metabolising enzymes in antidepressant side effects. Psychopharmacol. (Berl.) 232 (14), 2609–2617. doi: 10.1007/s00213-015-3898-x
Kessler, R. C., Barker, P. R., Colpe, L. J., Epstein, J. F., Gfroerer, J. C., Hiripi, E. (2003). Screening for serious mental illness in the general population. Arch. Gen. Psychiatry 60 (2), 184–189. doi: 10.1001/archpsyc.60.2.184
Maggo, S. D. S., Sycamore, K. L. V., Miller, A. L., Kennedy, M. A. (2019). The Three Ps: Psychiatry, Pharmacy, and Pharmacogenomics, a brief report from New Zealand. Front. In Psychiatry 10 (690), 690. doi: 10.3389/fpsyt.2019.00690
McCrae, R. R., Costa, P. T. (2004). A contemplated revision of the NEO five-factor inventory. Pers. Individ. Dif. 36 (3), 587–596. doi: 10.1016/S0191-8869(03)00118-1
Miller, S. A., Dykes, D. D., Polesky, H. F. (1988). A simple salting out procedure for extracting DNA from human nucleated cells. Nucleic Acids Res. 16 (3), 1215. doi: 10.1093/nar/16.3.1215
Mitchell, J. E., Roerig, J., Steffen, K. (2013). Biological therapies for eating disorders. Int. J. Eating Disord. 46 (5), 470–477. doi: 10.1002/eat.22104
Nassan, M., Nicholson, W. T., Elliott, M. A., Rohrer Vitek, C. R., Black, J. L., Frye, M. A. (2016). Pharmacokinetic pharmacogenetic prescribing guidelines for antidepressants: a template for psychiatric precision medicine. Mayo Clin. Proc. 91 (7), 897–907. doi: 10.1016/j.mayocp.2016.02.023
Oakley-Browne, M. A., Wells, J. E., Scott, K. M., McGee, M. A. (2010). The kessler psychological distress scale in Te Rau Hinengaro: the New Zealand mental health survey. Aust. New Z. J. Psychiatry 44 (4), 314–322. doi: 10.3109/00048670903279820
Papakostas, G. I. (2008). Tolerability of modern antidepressants. J. Clin. Psychiatry 69Suppl E1, 8–13.
Preskorn, S. H. (2003a). Reproducibility of the in vivo effect of the selective serotonin reuptake inhibitors on the in vivo function of cytochrome P450 2D6: an update (part I). J. Psychiatr. Pract. 9 (2), 150–158. doi: 10.1097/00131746-200303000-00006
Preskorn, S. H. (2003b). Reproducibility of the in vivo effect of the selective serotonin reuptake inhibitors on the in vivo function of cytochrome P450 2D6: an update (part II). J. Psychiatr. Pract. 9 (3), 228–236. doi: 10.1097/00131746-200305000-00006
Salkovskis, P. M., Rimes, K. A., Warwick, H. M. C., Clark, D. M. (2002). The health anxiety inventory: development and validation of scales for the measurement of health anxiety and hypochondriasis. Psychol. Med. 32 (5), 843–853. doi: 10.1017/S0033291702005822
Serretti, A., Calati, R., Massat, I., Linotte, S., Kasper, S., Lecrubier, Y. (2009). Cytochrome P450 CYP1A2, CYP2C9, CYP2C19 and CYP2D6 genes are not associated with response and remission in a sample of depressive patients. Int. Clin. Psychopharmacol. 24 (5), 250–256. doi: 10.1097/YIC.0b013e32832e5b0d
Shams, M. E., Arneth, B., Hiemke, C., Dragicevic, A., Muller, M. J., Kaiser, R. (2006). CYP2D6 polymorphism and clinical effect of the antidepressant venlafaxine. J. Clin. Pharm. Ther. 31 (5), 493–502. doi: 10.1111/j.1365-2710.2006.00763.x
Singh, A. B., Bousman, C. A. (2017). Antidepressant Pharmacogenetics. Am. J. Psychiatry 174 (5), 417–418. doi: 10.1176/appi.ajp.2017.17020173
Singh, A. B. (2015). improved antidepressant remission in major depression via a pharmacokinetic pathway polygene pharmacogenetic report. Clin. Psychopharmacol. Neurosci. 13 (2), 150–156. doi: 10.9758/cpn.2015.13.2.150
Tamminga, W. W., Wemer, J. J., Oosterhuis, B. B., de Zeeuw, R. R., de Leij, L. L., Jonkman, J. J. (2001). The prevalence of CYP2D6 and CYP2C19 genotypes in a population of healthy Dutch volunteers. Eur. J. Clin. Pharmacol. 57 (10), 717–722. doi: 10.1007/s002280100359
Taranu, A., Colle, R., Gressier, F., El Asmar, K., Becquemont, L., Corruble, E. (2017). Should a routine genotyping of CYP2D6 and CYP2C19 genetic polymorphisms be recommended to predict venlafaxine efficacy in depressed patients treated in psychiatric settings? Pharmacogenomics 18 (7), 639–650. doi: 10.2217/pgs-2017-0003
Tracy, T. S., Chaudhry, A. S., Prasad, B., Thummel, K. E., Schuetz, E. G., Zhong, X.-b. (2016). Interindividual variability in cytochrome p450-mediated drug metabolism. Drug Metab. Dispos. 44 (3), 343–351. doi: 10.1124/dmd.115.067900
von Elm, E., Altman, D. G., Egger, M., Pocock, S. J., Gotzsche, P. C., Vandenbroucke, J. P. (2007). Strengthening the Reporting of Observational Studies in Epidemiology (STROBE) statement: guidelines for reporting observational studies. BMJ 335 (7624), 806–808. doi: 10.1136/bmj.39335.541782.AD
Wells, R. E., Kaptchuk, T. J. (2012). To tell the truth, the whole truth, may do patients harm: the problem of the nocebo effect for informed consent. Am. J. Bioethics 12 (3), 22–29. doi: 10.1080/15265161.2011.652798
Whirl-Carrillo, M., McDonagh, E. M., Hebert, J. M., Gong, L., Sangkuhl, K., Thorn, C. F. (2012). Pharmacogenomics knowledge for personalized medicine. Clin. Pharmacol. Ther. 92 (4), 414–417. doi: 10.1038/clpt.2012.96
Wishart, D. S. (2016). Emerging applications of metabolomics in drug discovery and precision medicine. Nat. Rev. Drug Discovery 15, 473. doi: 10.1038/nrd.2016.32
Wright, G. E., Niehaus, D. J., Drogemoller, B. I., Koen, L., Gaedigk, A., Warnich, L. (2010). Elucidation of CYP2D6 genetic diversity in a unique African population: implications for the future application of pharmacogenetics in the Xhosa population. Ann. Hum. Genet. 74 (4), 340–350. doi: 10.1111/j.1469-1809.2010.00585.x
Keywords: pharmacogenetics, adverse reactions, social media advertising, personalized medicine, Psychiatry
Citation: Maggo S, Kennedy MA, Barczyk ZA, Miller AL, Rucklidge JJ, Mulder RT and Foulds JA (2019) Common CYP2D6, CYP2C9, and CYP2C19 Gene Variants, Health Anxiety, and Neuroticism Are Not Associated With Self-Reported Antidepressant Side Effects. Front. Genet. 10:1199. doi: 10.3389/fgene.2019.01199
Received: 02 September 2019; Accepted: 29 October 2019;
Published: 26 November 2019.
Edited by:
Edoardo Spina, University of Messina, ItalyReviewed by:
Alessandro Serretti, University of Bologna, ItalyAlessio Squassina, University of Cagliari, Italy
Copyright © 2019 Maggo, Kennedy, Barczyk, Miller, Rucklidge, Mulder and Foulds. This is an open-access article distributed under the terms of the Creative Commons Attribution License (CC BY). The use, distribution or reproduction in other forums is permitted, provided the original author(s) and the copyright owner(s) are credited and that the original publication in this journal is cited, in accordance with accepted academic practice. No use, distribution or reproduction is permitted which does not comply with these terms.
*Correspondence: Simran Maggo, simran.maggo@otago.ac.nz