- 1Department Sciences du vivant et de la sante, Université de Bordeaux, UR Œnologie EA 4577, Bordeaux, France
- 2Biolaffort, Bordeaux, France
- 3Department Micro-organismes, Génomes, Environnement, Université de Strasbourg, CNRS, GMGM UMR 7156, Strasbourg, France
The budding yeast Saccharomyces cerevisiae is certainly the prime industrial microorganism and is related to many biotechnological applications including food fermentations, biofuel production, green chemistry, and drug production. A noteworthy characteristic of this species is the existence of subgroups well adapted to specific processes with some individuals showing optimal technological traits. In the last 20 years, many studies have established a link between quantitative traits and single-nucleotide polymorphisms found in hundreds of genes. These natural variations constitute a pool of QTNs (quantitative trait nucleotides) that modulate yeast traits of economic interest for industry. By selecting a subset of genes functionally validated, a total of 284 QTNs were inventoried. Their distribution across pan and core genome and their frequency within the 1,011 Saccharomyces cerevisiae genomes were analyzed. We found that 150 of the 284 QTNs have a frequency lower than 5%, meaning that these variants would be undetectable by genome-wide association studies (GWAS). This analysis also suggests that most of the functional variants are private to a subpopulation, possibly due to their adaptive role to specific industrial environment. In this review, we provide a literature survey of their phenotypic impact and discuss the opportunities and the limits of their use for industrial strain selection.
Introduction
Between individuals of the same species, a broad palette of genetic variants is found, including large chromosomal rearrangements (deletions, duplications, inversions, translocations, and introgressions) and punctual mutations (Griffiths et al., 2000). This latter type includes small insertions/deletions (InDels) as well as single-nucleotide polymorphisms (SNPs) that are by far the most frequent polymorphic event found at the intraspecific level in fungi (Doniger et al., 2008), human (Sachidanandam et al., 2001), and plants (Ching et al., 2002). Depending on the organism and the genomic position, the SNP/InDel frequency ranges from 1×10−2 to 1×10−3 per base and constitutes a vast pool of genetic variants (Stucki et al., 2012; Almeida et al., 2014; Scozzari et al., 2014; Peter et al., 2018).
With the relative ease of obtaining genome-wide SNP-data, their impact on complex trait can be tracked by either genome-wide association studies (GWAS) or quantitative trait loci mapping (QTL mapping) in medicine (Beck et al., 2014) or agronomy (Brachi et al., 2011; Sharma et al., 2015). When they are statistically linked to a phenotype, these SNPs become QTNs (quantitative trait nucleotides) and could be listed in large databases for research communities (Grant et al., 2010; Youens-Clark et al., 2011). In contrast to multicellular eukaryotes, large SNP-database regrouping several studies for fungi and yeasts are not really developed. For Saccharomyces cerevisiae species, the first attempts to set up SNP databases have been made 10 years ago (Doniger et al., 2008; Schacherer et al., 2009) but without establishing a link between genotypic data and phenotypes.
With the emergence of high-throughput sequencing in the last 10 years, the number of available complete genomes rose impressively providing a quite complete landscape of genetic polymorphism for this species (Peter et al., 2018). A particular focus was done on strains belonging to food fermentation including wine (Borneman et al., 2016), beer (Gallone et al., 2016), distillery (Barbosa et al., 2018), and cheese/flor/distillery (Legras et al., 2018). As previously demonstrated, the S. cerevisiae population appears to be clearly structured according to the geography, the environmental niche, and the relation to human environment (Peter and Schacherer, 2016; Marsit et al., 2017; Peter et al., 2018). Among the food-related strains, the beer and bakery strains are polyphyletic and characterized by a high ploidy level (Gallone et al., 2016), whereas wine or sake strains are mostly diploid and derived from the genetic drift of a limited number of founders (Ohnuki et al., 2017). Since each industrial application is characterized by distinct populations, the strains of each group have been faced with specific selective pressures. These conditions have likely promoted the emergence of adaptive alleles conferring a phenotypic advantage to each particular industrial process. The identification of those adaptive mutations in the wide pool of natural variations that discriminate the different subpopulations remains a challenging task. It has been recently shown by GWAS that CNVs (copy number variants) and gross chromosomal reorganization exert a sound effect on phenotypic variation (Peter et al., 2018). However, the yeast strains of each subpopulation also exhibit a wide set of SNPs shaping their technological properties. The growing number of QTNs found in the last decade suggests that numerous functional variants will be found in the future.
In this review, we established an extensive catalog of S. cerevisiae QTNs experimentally validated that impact traits of biotechnological interest. First, we analyzed their allelic frequencies and their dispersion within a large population. Second, we reported their physiological effect. Third, we discussed how these QTNs can be used for significantly improving the technological properties of industrial yeast strains.
Genes and Polymorphisms Impacting Quantitative Traits of Industrial Interest
To establish an exhaustive catalog of functional variants, we focus our literature survey on QTNs impacting yeast traits relevant for biotechnological applications. Traits were sorted in three main phenotypic classes: traits linked to metabolism (e.g., nitrogen, carbon, vitamin, and fermentation activity), traits linked to stress resistance (e.g., acidic and basic, temperature, osmotic, and ethanol), and traits impacting the organoleptic properties of the products (Figure 1A). Most of QTNs were identified by linkage analysis, demonstrating the efficiency of this strategy in yeast (Liti and Louis, 2012; Fay, 2013). Other functional variants were identified by mutagenesis, comparative genomics, and adaptive laboratory evolution (ALE) approaches (Gresham and Hong, 2014). More recently, genome-wide associations were performed on an extensive set of fully sequenced natural isolates, providing a large list of SNPs statistically associated with the measured phenotypic diversity (Peter et al., 2018; Sardi et al., 2018).
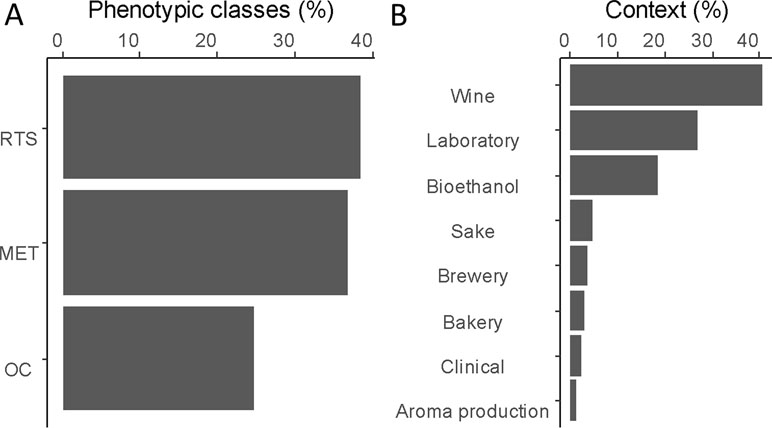
Figure 1 Overview of identified quantitative trait genes (QTGs). (A) Proportion of genetic variants according to three main categories. Resistance to stress (RTS), central metabolism (MET), and organoleptic compound (OC). (B) Proportion of QTGs according to the context of the study in which they were identified.
To provide a functional analysis, we included only genes that have been experimentally validated by reciprocal hemizygosity assay (RHA) (Steinmetz et al., 2002) or allele swapping (Storici and Resnick, 2006). In this context, a total of 147 QTGs (quantitative trait genes) were reported and described across a set of 85 articles (Table S1). In total, 71% of these genes were identified in an industrial context since they concern media and/or strains related to aroma production (1%), bioethanol (18%), or traditional fermented goods including wine (41%), sake (5%), bakery (3%), or beer (3%). The remaining 30% were identified by using laboratory or clinical strains cultivated in non-industrial conditions (Figure 1B). However, these genes were included since they possibly affect industrial properties [e.g., growth fitness, stress resistance, and flocculation). We tested the overall distribution of such genes across yeast genome, and no hotspot was found (hypergeometric distribution, with sliding windows of 100 kb and a 10-kb step, 1,000 permutation tests, False Discovery Rate (FDR) = 5%](Figure 2).
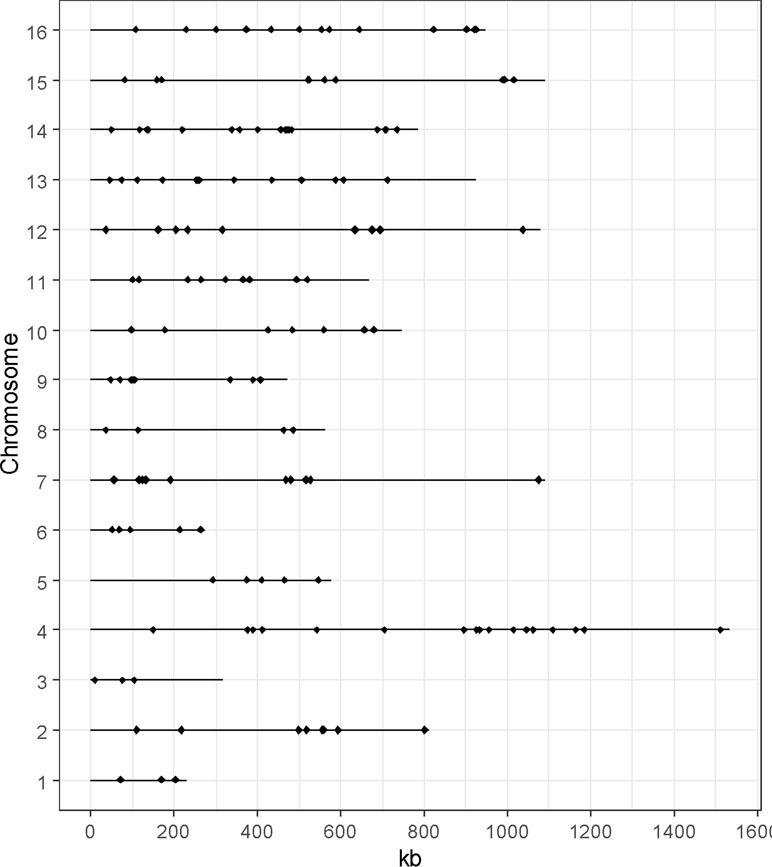
Figure 2 Distribution of the QTGs along the genome. Each dot represents the position of one QTG. No QTG hotspot was found (hypergeometric distribution, 1,000 permutations test, FDR = 5%).
Among these 147 QTGs, a significant enrichment was obtained for Gene Ontology terms (goTermFinder, https://www.yeastgenome.org) (Figure 3). This is the case for function and process terms related to transcription (nucleic acid-binding transcription factor activity, DNA binding, protein-binding transcription factor activity, and transcription factor binding) (p-value < 0.05) and transport (plasma membrane, transmembrane transporter activity, regulation of transport, amino acids transport, and carbohydrate transport) (p-value < 0.05). The strong enrichment of such categories confirms that these genes are important levers for generating phenotypic variability.
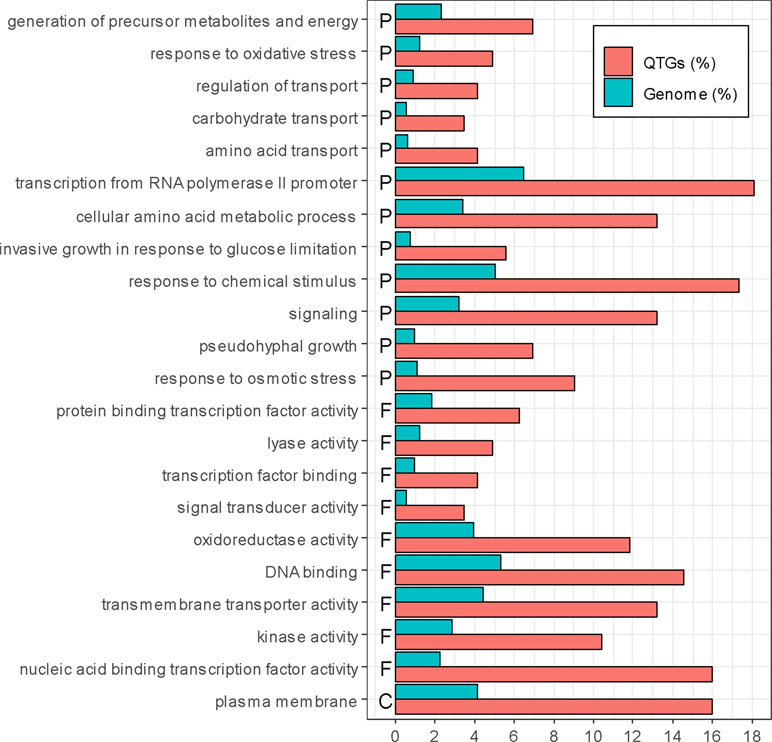
Figure 3 Enrichment of QTGs. Only significantly over-represented or under-represented categories are represented (chi-squared test, p-value < 0.05, with Bonferroni adjustment for multiple tests). The percentage of genes in the genome and in the QTGs is indicated in blue and red, respectively. F, P, and C represent molecular function, biological process, and cellular component, respectively.
In S. cerevisiae, the pangenome was recently defined using a population of 1,011 natural isolates. Overall, 4,940 core genes and 2,856 accessory genes were determined within the population (Peter et al., 2018). Interestingly, a strong enrichment has been shown for genes whose function is related to adaptation to the environment in the subset of accessory genes (Peter et al., 2018). Across the 147 identified QTGs, 117 and 30 are part of the core and accessory genomes, respectively (Figure 4A). This proportion clearly shows that a large fraction of the QTGs are part of the conserved core genome. Moreover, there is no bias toward the subset of accessory genes, although they are more prone to be involved to adaptation processes.
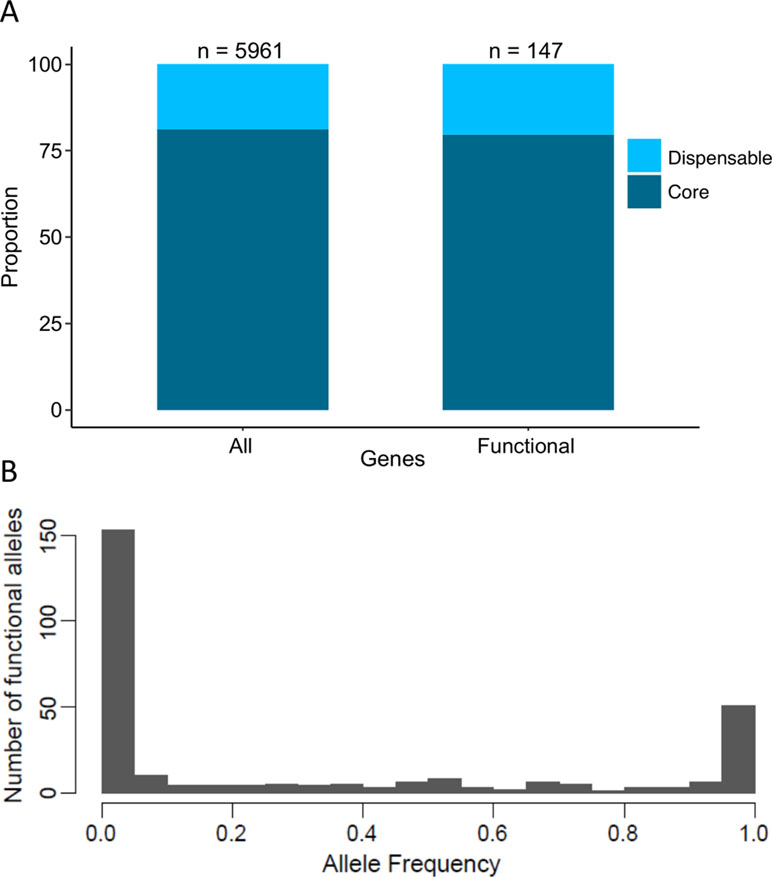
Figure 4 Proportion of dispensable/core gene and frequency of functional alleles. (A) Dispersion of QTGs allele frequency among 1,011 isolates. For each QTG, the frequency of the favorable alleles is used. (B) Proportion of dispensable and core genes among the pangenome (all) and among QTGs (functional).
In order to focus our review at the SNP level, we listed all the possible genetic polymorphisms found in these QTGs. Since most of the articles validated genes but not at the SNP level, we took into account for each QTG all the genetic polymorphisms described by the authors. These 284 QTNs can be sorted in several categories according to the type of genetic polymorphisms that discriminate their allelic variations. Most of the identified allelic variations correspond to one or a combination of missense mutations, also called non-synonymous substitutions (nsSNPs) (81%). Other minor cases correspond to SNPs or InDels in 5′UTR or 3′UTR regions (6%), InDels in the coding sequence (6%), synonymous SNPs (sSNPs) (4%), translocation (2%), and short tandem repeats.
The functional impact of a subset of 251 QTNs, corresponding to missense mutations, was estimated by using the predictive program SIFT (sorting tolerant from intolerant) (Ng and Henikoff, 2003). It turns out that 168 mutations are predicted to be tolerated, whereas only 83 are predicted to be deleterious, suggesting that most of the QTNs do not lead to a loss of function (Table S2). By contrast, 22 QTNs (approximately 8%) correspond to nonsense mutations, meaning that genetic variant results in a premature stop codon generating in most of the cases to a loss of function. In addition, one and two QTNs lead to the loss of start and stop codons, respectively.
One main feature of the S. cerevisiae species is the bias toward low-frequency variant. Among the 1,011 recently sequenced genomes, much of the detected genetic polymorphisms are very low-frequency variants with 93% of them having a minor allele frequency lower than 0.1 (Peter et al., 2018). This observation raises the question regarding the impact of these variants on the phenotypic diversity. Among the 284 QTNs, more than 150 have a frequency lower than 5%, meaning that these variants would be undetectable by GWAS (Figure 4B).
Population genomic studies in S. cerevisiae also allowed to define precisely the different subpopulations, which are related to either the ecological or geographical origins. Based on the 1,011 isolates, a total of 26 subpopulations were defined (Peter et al., 2018). Interestingly, the identified QTNs are not evenly distributed across these subpopulations, and biases toward some specific of them are observed (Table S3). For example, 26 QTNs are private to the wine subpopulation and are only found in 9 to 50 wine isolates. Two SNPs located the MSN2 and MSN4 genes are exclusively found in the sake subpopulation. And finally, a mutation in the MAL33 gene is private to the African beer population. This observation clearly suggests that most of the functional variants are private to a subpopulation. This could result to the adaptation to a specific industrial environment. However, since wine and sake subpopulations are derived from a limited number of founders, their presence could be also explained by simple genetic drift.
Overall, this set of 284 QTNs is very insightful but does not reflect the genetic architecture in its entirety. The genotypic landscape is not limited to SNPs located in protein coding regions. Indeed, SNPs as well as InDels located in promoter and 3′ untranslated regions were identified for some complex traits. These genetic variants do not affect the protein sequence but can impact the transcription level, mRNA processing, translation, export, and decay. Mutations altering a functional motif in the promoter region have been identified many times (Shahsavarani et al., 2012; Zimmer et al., 2014; Cubillos, 2016; Salinas et al., 2016; Tapia et al., 2018). They constitute allele-specific expression (ASE) changes that are certainly a source of phenotypic variation (see Cubillos, 2016, for a review). In addition, structural variants such as CNVs and translocations were identified as involved in the variation of some specific industrial traits. Recently, genome-wide association analyses, performed on a large collection of S. cerevisiae isolates, highlighted the importance of the CNVs, which explain a more considerable proportion of the phenotypic variance and have greater effects on phenotype compared with the SNPs (Peter et al., 2018). However, the detection of the structural variants at a population scale is yet technically limited, and consequently, their global impact on the complex traits is still to be explored.
Industrial Traits Impacted by Natural Genetic Variants
In this section, we shortly described the phenotypic impact of most of QTGs/QTNs reviewed. The phenotypes surveyed were arranged in three main subsections concerning central metabolism, resistance to toxins and stresses, and organoleptic contribution.
Central Metabolism
In total, 71 natural alleles have been identified, and they impact 70 traits related to the central metabolism (Table 1 and Table S1).
Nitrogen and Vitamin Metabolism
Nitrogen sources as well as the vitamin composition can vary in a wide range according to the raw vegetal material, the fertilization method, and the harvest date. Their composition may drastically affect the yeast fermentation performances in beer (Gibson et al., 2007), wine (Bell and Henschke, 2005), or bio-ethanol productions (Hahn-Hägerdal et al., 2005). Depending on the genetic background, the strain’s ability to use various nitrogen sources differs between subpopulations (Ibstedt et al., 2014) and within strains of the same industrial process (Jiranek et al., 1995; Manginot et al., 1998; Gutiérrez et al., 2013; Brice et al., 2014).
The identification of genetic factors controlling nitrogen consumption has been achieved by many QTL mapping studies (Ambroset et al., 2011; Brice et al., 2014; Ibstedt et al., 2014; Jara et al., 2014), one large-scale hemizygosity analysis (Gutiérrez et al., 2013), and one ALE experiment (Gresham and Hong, 2014). These studies revealed relevant genetic variants that could be used for improving the performance of fermenting yeast. In such studies, the diverse media employed consist of either a mixture of different nitrogen sources, mimicking a natural medium (Ambroset et al., 2011; Brice et al., 2014; Jara et al., 2014), or several distinct media containing each a single nitrogen source (Gutiérrez et al., 2013; Gresham and Hong, 2014; Ibstedt et al., 2014). When a single nitrogen source was used, the identified QTLs were chiefly due to deleterious mutations in genes involved in the pathway of the amino acid concerned (Ibstedt et al., 2014). In contrast, in mixed nitrogen media, the effects of the QTLs identified are more pleiotropic and impact a group of amino acids sharing the same biochemical structure (Jara et al., 2014).
Deleterious alleles, impairing the use of a particular nitrogen source, were identified for proline (PUT4), allantoin (DAL1 and DAL4) (Ibstedt et al., 2014), and methionine (ARO8, VBA3, and ADE5,7) (Gutiérrez et al., 2013). Similar deleterious mutations were also found for asparagine (ASP1) (Marullo et al., 2007a) or for folic acid metabolism (ABZ1), having an impact on wine fermentation kinetics (Ambroset et al., 2011). These recessive mutations are rare and generally of poor interest because industrial practices require prototrophic strains. However, these alleles can be used as auxotrophic markers for achieving breeding programs in a non-GMO context (Steensels et al., 2014) as the ura3 and lys2 markers (Timberlake et al., 2011; Dufour et al., 2013).
More interestingly, three pleiotropic genes (GLT1, ASI1, and AGP1) impacting consumption of several amino acids were identified by measuring the consumption profile of amino acids (Jara et al., 2014). By implementing a multi-parental design (SGRP-X4), these authors identified four additional genes (ASI2, CPS1, LYP1, and ALP1) involved in the consumption of aromatic and basic amino acids. In the same study, the comparative RNA-seq profiling of extreme progeny clones allowed the identification of two additional genes (PDC1 and ARO1) that influence the amino acid consumption in the wine fermentation (Cubillos et al., 2017). Following a similar strategy, the progenies of two enological strains were phenotyped for their fermentation capacity in a synthetic grape must containing a low assimilable nitrogen level (Brice et al., 2014). Four genes directly or indirectly linked with the nitrogen metabolism were identified (BIO3, GCN1, ARG81, and MDS3).
The expression level and the stability of proteins involved in nitrogen catabolism may also contribute to nitrogen assimilation. For example, the ASE of ASN1, the asparagine synthetase, modulates the consumption of aspartic and glutamic acid in wine-related fermentations (Salinas et al., 2016). Amino acid assimilation, in particular for proline, can be also induced by increasing the half-life of the membrane transporter Put4p by changing some N-terminal arginine residues involved in its ubiquitination (Omura et al., 2005). Although the optimal consumption of nitrogen source is generally considered as a suitable technological trait, the rapid and complete consumption of amino acids may negatively affect fermentation capacities (Martí-Raga et al., 2015) and reduces the chronological life span (Kwan et al., 2011) in specific conditions. All together, these studies support that multiple molecular mechanisms impact the nitrogen assimilation including the nitrogen signaling pathways, metabolic enzymes, and protein degradation as summarized in Figure 5.
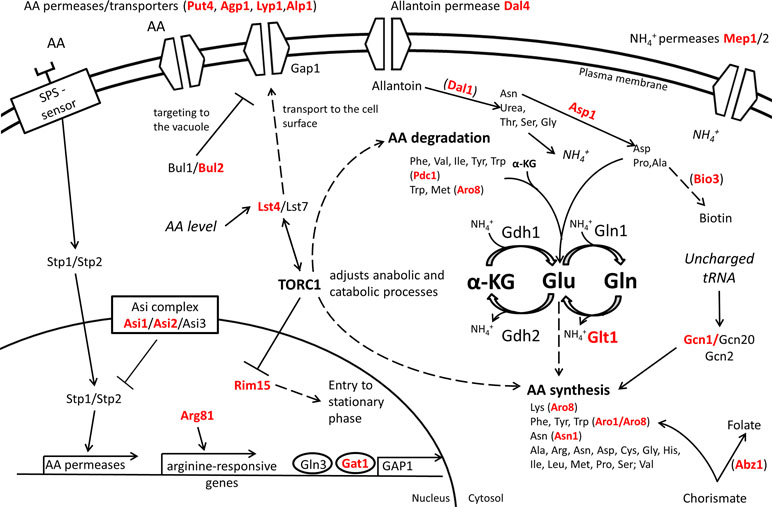
Figure 5 Overview of QTG involved in nitrogen metabolism. Proteins highlighted in red are coded by allelic variants that were experimentally validated for their contribution to variation in nitrogen source consumption in S. cerevisiae. Indirect relationships are shown in dashed lines. Proteins are between brackets when only the protein of interest of a pathway is shown.
Sugar Catabolism
Control of Fermentative and Respiratory Switch
The expanded use of S. cerevisiae in biotechnology is likely due to its strong efficiency to dissimilate small sugars by both respiratory and fermentative routes (Pronk et al., 1996; Zampar et al., 2013). In the past, deleterious mutants unable to switch between fermentative and respiratory metabolism have been identified for CAT8 (Zimmermann et al., 1977) and ADR1 (Denis and Young, 1983). Besides these drastic mutations, the fermentation/respiration balance is controlled by many other genes, and considerable variations have been measured within strains and species (Quirós et al., 2014). Some alleles impacting this metabolic control have been identified in HAP4 and MBR1 (Salinas et al., 2012). Both genes are involved in mitochondrial function, and HAP4 is a key transcription factor activating respiratory genes (Zampar et al., 2013). These particular alleles may partially activate the Krebs cycle, reducing the fermentation efficiency of yeast in winemaking conditions.
A similar alteration in fermentation/respiration balance was found in sake strains by a comparative transcriptomic approach (Watanabe et al., 2013). Two distinct loss-of-function alleles have been identified for ADR1 in the sake strains K7 and K701. This transcription factor is activated when glucose becomes limiting (diauxic shift) and promotes ethanol catabolism by activating the transcription of ADH2. Therefore, strains lacking a functional ADR1 might have an accelerated alcoholic fermentation in a sake-brewing context.
Sugar Uptake and Assimilation
Although S. cerevisiae is able to ferment many sugars, their uptake and catabolism obey priority rules. This regulation, called glucose catabolite repression, limits the speed and efficiency of the fermentation of many sugars (Gancedo, 1998). Industrial conditions offer selective constraints that have promoted the constitutive activation of non-preferred carbon sources uptake and catabolism.
An example is the fermentation of maltose and maltotriose, the two most abundant sugars in brewing wort (Stewart, 2006), which are also present in bakery doughs. The fermentation performance of beer strains is therefore defined by their capacity to transport those sugars. Depending on the species, i.e., S. cerevisiae (ale group) or S. pastorianus (lager group), different α-glucoside transporters have been described (ScAGT1, ScMALx1, SeMALx1, SeAGT1, MTT1, and ScMPHx). Most of the ale beer strains have a full-length AGT1 gene, which ensures complete wort fermentation. By contrast, the lager strains have a premature stop codon at the position 1183 that reduces their maltotriose uptake (Vidgren et al., 2009). Moreover, the MAL loci are present in several subtelomeric regions, resulting in CNVs that affect the transport capacity of the strains (Brown et al., 2010). The impact of sugar assimilation on fermentation kinetics has been also demonstrated for the SUC2 gene, encoding for the invertase, that can be present in numerous subtelomeric regions especially in bakery strains (Carlson and Botstein, 1983; Ness and Aigle, 1995). Besides their integrity and their copy number, α-glucoside transporters may be also differentially regulated. A documented example was provided in bakery strains having an nsSNP in the MALx3 gene, which encodes for the transcriptional activator of the maltose permease and maltase (Higgins et al., 1999). This leu243phe substitution abolishes the glucose catabolite repression conferring constitutive maltose consumption. In a similar way, galactose is a carbon source also present in many industrial media (cheese whey, molasses, and lignocelluloses) (Bro et al., 2005). Mutations in GAL80, the repressor of the galactose utilization pathway, have been generated by adaptive laboratory evolution and lead to a constitutive activation of galactose consumption (Segrè et al., 2006).
Hexoses (fructose and glucose) transport is also modulated by CNVs and punctual mutations. ALE studies demonstrated that hexose transporter genes alike HXT6/7 are found in numerous copies (Kvitek and Sherlock, 2011). The uptake of fructose, which is less assimilated than glucose (Berthels et al., 2004), is also affected by allelic variations. For instance, allelic variations in the major hexose transporter Hxt3p or in the uncharacterized protein Rbh1p have been reported (Guillaume et al., 2007; Salinas et al., 2012).
Glycerol Production/Consumption and the Modulation of Sugar-to-Ethanol Yield
Since glycerol represents the main metabolic by-product of the alcoholic fermentation, its consumption by microbial conversions has been evaluated in order to valorize the dramatic surplus of crude glycerol produced in the biofuel industry (Clomburg and Gonzalez, 2013). Favorable alleles enhancing the S. cerevisiae growth rate on glycerol as unique carbon source was identified by QTL mapping. Two genes were identified: GUT1, which encodes for a glycerol kinase (Swinnen et al., 2013), and TAO3, a scaffolding protein involved in cellular morphogenesis (Wilkening et al., 2014).
Alternatively, the genetic bases of the glycerol production were explored. In bioethanol industry, a reduction of glycerol production would enhance the ethanol yield, which is, therefore, a valuable trait. In this context, the glycerol/ethanol ratio variability was assessed in 50 strains, and this genetic variability was used for achieving QTL mapping programs (Hubmann et al., 2013a; Hubmann et al., 2013b). The SSK1 E330N … K356N allele (Hubmann et al., 2013a) was identified; this recessive allele found in the parental strain CBS6412 leads to a half-truncated protein. Its integration in the genome of the industrial background “Ethanol Red” has a substantial impact, decreasing by 23% its glycerol yield. Further investigations in the same background allowed the identification of three other alleles affecting the glycerol yield. Genes involved are related to the glycerol pathway (SMP1, HOT1, and GPD1) (Hubmann et al., 2013b). The GPD1 L164P allele produces the largest effect and shows epistatic relations with other two loci.
In contrast, a growing demand of strains showing a lower ethanol production is observed in the alcoholic beverage industry and especially in winemaking. This demand moves in the direction of public health policy and could be in part solved by the development of new strains (Kutyna et al., 2010; Dequin et al., 2017). The most explored route consists in redirecting a part of the glycolytic flux toward the production of glycerol since this compound is organoleptically neutral. Allelic variations in GAT1, YFL040W, GPD1, and ADH3 impacting glycerol yield (Salinas et al., 2012; Tapia et al., 2018) were identified by the same laboratory in a wine context. However, the highest production observed did not modify the sugar-to-ethanol conversion in a significant manner.
Acetic Acid Production
Acetic acid can be produced by spoilage microorganisms but is also produced by yeast during the alcoholic fermentation (Vilela-Moura et al., 2010). Acetic acid production level is a quantitative trait that varies across isolates (Giudici and Zambonelli, 1992; Marullo et al., 2004). In Lambic beers and some other sour beer styles, acetic acid can be a desirable component that contributes to the complexity of the flavor and aroma profile. However, in beverage industries, acetic acid generally has a negative impact on the organoleptic quality especially for wine (Ribéreau-Gayon et al., 2006). Therefore, the allelic variants controlling acid acetic production during fermentation were mostly identified in a winemaking context.
The major gene involved in acetic acid production in wine fermentation is ALD6, which encodes an aldehyde dehydrogenase (Remize et al., 2000; Saint-Prix et al., 2004). Depending on the yeast isolate, this gene is differentially expressed leading to various acetic acid production levels. Two SNPs in the promoter region of the ALD6 gene were linked to this variation in an enological context (Salinas et al., 2012). Due to its role in the redox balance homeostasis, the acetic acid production level could also correlate with cell growth. A deleterious mutation in the catalytic core of the asparaginase ASP1 gene was linked to the slow assimilation of asparagine in synthetic must. This affects the cell growth, causing overproduction of acetic acid in asparagine-rich media (Marullo et al., 2007a). More relevant are the premature stop codons found in the YAP1 gene (YAP1Q541X and YAP1Q573X). Those mutations were isolated by screening cerulenin-resistant mutants of the wine starter PDM. They dramatically decreased (−30%) acetic acid production during the fermentation of different grape juices (Cordente et al., 2013). Yap1p is a transcriptional factor involved in oxidative stress, and the metabolic link between these mutations and the phenotype was not perfectly understood. Interestingly, these mutants showed a high alcohol dehydrogenase activity with an increased production of acetate esters that could reduce the acetic acid production (see below).
Fermentation Efficiency
Several allelic variants affecting the overall fermentation rate in diverse industrial contexts have been identified. In the bakery context, a noteworthy trait is the FIL phenotype that consists in losing stress resistance when the alcoholic fermentation is initiated. Some strains show a recessive fil phenotype (fermentation-induced loss of stress resistance) and conserve a suitable resistance to heating and freezing even if they are collected in exponential growth phase during alcoholic fermentation (Thevelein et al., 1999). This particular feature can be obtained by using specific mutations in the gene CYR1 (adenylate cyclase) at the position G1682L or in the gene GPR1 (G-protein coupled receptor). The fil phenotype conferred is particularly relevant for obtaining full active strains for the fermentation from frozen doughs or active dry yeast.
Other allelic variants affecting the fermentation rate itself were identified in high-gravity matrices such as sake and wine. Genes impacted may encode for unexpected functions like the glycogen debranching protein (Gdp1p) (Cubillos, 2016). Interestingly, the positive allele of the GPD1 gene is located in both the promoter and coding regions. Although the physiological mechanisms of these natural variants are not particularly linked to alcoholic fermentation, the allele of a wine-related strain (WE) confers a faster fermentation. Other mechanisms of adaptation were found by investigating the performance of wine yeast in the second fermentation that took place in locked bottles (méthode champenoise). Fermentation kinetics were measured by following the CO2 pressure rise inside the bottle. Two genes encoding for components of the plasma (PMA1) and vacuolar (VMA13) membrane ATPases were identified. Positive alleles provide a faster fermentation kinetics in low pH conditions (2.8), which is a particular feature of sparkling wines. The same authors identified two other genes related to osmotic regulation (MSB2) and multidrug resistance (PDR1) (Martí-Raga et al., 2017). Interestingly, transcriptional regulators of this multidrug resistance family (PDR network) have been also linked to the fermentation resistance in a sake-brewing context. By applying a drug resistance screening, different alleles of the transcriptional factors PDR1 (M308I) and PDR3 (L950S, G948D, and G957D) were isolated. These alleles drastically improved the fermentation efficiency of sake strain, allowing the production of more than 21% (v/v) of ethanol in industrial trials (Mizoguchi et al., 2002).
Another relevant industrial property of fermenting yeast is the length of the lag phase that could be particularly critical for achieving the inoculation of non-sterile musts. The genetic determinism of the lag phase has been partially elucidated in wine fermentations by performing a QTL mapping between two wine-related strains (Zimmer et al., 2014). In this work, a major QTL explaining relevant differences in the lag phase duration (more than 24 h) was identified. In this specific case, the molecular cause of phenotypic discrepancy is due to a reciprocal translocation event (XV-t-XVI) involving the gene SSU1, which encodes for a sulfite pump. This gross chromosomal rearrangement increased the expression level of SSU1 in the parental strain GN that achieves a rapid fermentation start in synthetic grape juice containing SO2. This work illustrates an interesting case of phenotypic convergence since two other independent chromosomal rearrangements, VIII-t-XVI (Pérez-Ortín et al., 2002) and inv-XVI (García-Ríos et al., 2019), targeting SSU1 and conferring SO2 resistance were identified. Recently, the pleiotropic effect of these translocations has been demonstrated by seeking for QTLs that interact with environmental conditions (Peltier et al., 2018). Depending on the nature of the grape juice and the amount of free SO2 in the medium, the translocations associated with SSU1 may impact the production of SO2, lag phase, and fermentation rate.
Resistance to Toxins and Stresses
Industrial applications are characterized by a broad set of stresses such as osmotic, temperature, ethanol, pH, nutrient limitation, and presence of various toxins that affect the yeast cell growth and viability (Bauer and Pretorius, 2000; Gibson et al., 2007; Zhao and Bai, 2009; Sicard and Legras, 2011). Each particular stress activates specific and general stress responses, ensuring a better physiological adaptation (see Gasch, 2003). Although most of these stresses are common, each biotechnological process recreates particular conditions explaining the emergence of yeast strains adapted to each specific process (Sicard and Legras, 2011; Albertin et al., 2011). In this section, we point out natural genetic polymorphisms in 75 genes that impact the yeast resistance to several types of stress commonly found in biotechnological applications (Table 1 and Table S1).
Ethanol Tolerance
Ethanol accumulated during the fermentation impacts negatively the more sensitive strains, impairing the fermentation completion. Stuck fermentations affect the ethanol production yield and also the microbiological stability of beverages due to the presence of residual sugars. The selection of ethanol tolerant strains constitutes a real challenge in particular for sake and wine production where high concentrations are reached (respectively, 20% and 17%). Several hundred genes associated with ethanol tolerance were identified by functional genetics (see Ma and Liu, 2010; Snoek et al., 2016, for a review); however, identifications of causative SNPs are more rare.
Among the S. cerevisiae species, the sake strains demonstrate the highest capacity of ethanol accumulation with concentration reaching approximately 20% (v/v). Several genetic causes explaining this characteristic have been identified by comparing the transcriptome between sake and laboratory strains during sake fermentation (Wu et al., 2006; Shobayashi et al., 2007). Sake strains carry deleterious alleles in genes involved in the stress response and quiescent phase entry (RIM15 and MSN2/MSN4) (Watanabe et al., 2011, Watanabe et al., 2012). In addition, they lack the PPT1 genes involved in the heat shock stress response (Noguchi et al., 2012). These mutations private to the sake group (Table S3) may explain their high ethanol accumulation capacity.
Global transcription machinery engineering (gTME) was used to generate strains more tolerant to ethanol. One of the targeted genes is SPT15 encoding for a TATA-binding protein associated with ethanol tolerance (Alper et al., 2006). The random mutations generated globally modify the yeast transcriptional response, providing mutants with a higher ethanol tolerance (Yang et al., 2011). This strategy allowed the isolation of two SPT5 haplotypes that enhanced the ethanol production from 8% to 10% for the yeast strain L3262. Those alleles also confer a better tolerance to hyperosmotic stress (Kim et al., 2013), another character highly desirable for high-gravity brewing (HBV) fermentation.
Besides the differential activation of these transcriptional pathways, numerous QTNs impacting ethanol tolerance have been successfully identified by reverse genetics. Since ethanol exerts a toxic effect, resistant strains can be readily screened by applying selective media where only a part of the population overcomes a desired threshold. Historically, adaptive laboratory evolution has been successfully used (Chen et al., 2010; Stanley et al., 2010; Avrahami-Moyal et al., 2012; Voordeckers et al., 2015). By increasing ethanol concentration in turbidostatic cultures, causative SNPs in the SSD1 and UTH1 genes were identified (Avrahami-Moyal et al., 2012). The selective pressure imposed (up to 8% ethanol) and the laboratory background used in this study are, however, a bit far from the conditions met in industrial fermentations. In similar conditions, a long-term evolution experiment was applied for 200 generations in six independent bioreactors (Voordeckers et al., 2015). Ploidy level changes and CNVs were mainly observed. Some QTNs were also identified: alleles VPS70C590A, PRT1A1384G, IAI11G479T, and MEX67G456A conferred an adaptive advantage by impacting remarkably diverse molecular functions such as mRNA export (Mex67p), vacuolar protein sorting (Vps70p), and protein synthesis (Prt1p).
QTL mapping approaches were also used for exploring the tolerance to ethanol with concentrations closer to industrial conditions (up to 20%) (Swinnen et al., 2012a; Pais et al., 2013; Duitama et al., 2014). In these studies, segregants were screened on YPD plates with different ethanol concentrations and genotyped using a bulk-segregant analysis (BSA). RHA validated the effect of five genes involved in the ethanol tolerance: MKT1, SWS2, APJ1, ADE1, and KIN3. The negative impact of the S288c allele of MKT1 was found in both studies and is due to a rare deleterious allele (minor allele frequency (MAF) = 0.1%) that has a strong impact on many characters of the laboratory stain (Lee et al., 2009). This type of defective allele is not present in industrial strains and cannot be used for their improvement. However, pairs of positive alleles (KIN3 and ADE1) (Pais et al., 2013) and (APJ1 and SWS2) (Swinnen et al., 2012a) were brought by parental strains isolated from sake and biofuel, respectively. For APJ1, a clear ASE effect was observed demonstrating that the expression of APJ1 seems to be deleterious for high ethanol tolerance (Swinnen et al., 2012a).
Cold and High Temperature Tolerance
Each industrial process is characterized by the application of temperatures that often meet yeast physiological limits. In biofuel industry, distillery yeast must tolerate a high temperature (up to 40°C) in order to ferment in association with enzymatic cocktails used for the saccharification (Olofsson et al., 2008). The wine or sake industry imposes milder conditions with temperature that does not exceed 35°C (Marullo et al., 2009). Furthermore, cold temperature tolerance (6–15°C) can be required especially in brewery and white wine context. Finally, the bakery process imposes an extremely broad range of temperature by applying freeze/thaw cycles.
The first quantitative genetics study in yeast focused on high-temperature growth (HTG) phenotype, which consists in measuring the colony size after a 48-h culture at 41°C (Steinmetz et al., 2002). The dissection of a major QTL by RHA allowed identification of three genes (MKT1, END3, and RHO2) explaining the quantitative phenotypic variation between the laboratory strain S288c and a clinical isolate YJM145. The identification of causative SNPs was achieved few years later (Sinha et al., 2006) by using site-directed mutagenesis. RHO2 causative SNP is located in the 3′UTR region, which constitutes a rare example of polymorphism outside the coding sequence. A supplemental gene impacting this phenotype, NCS2, was finally identified by using a backcross strategy in order to eliminate the effect of the main segregating QTL (Sinha et al., 2008). Using a bulk sequencing analysis strategy (Yang et al., 2013) confirmed the deleterious inheritance of S288c alleles for NCS2 and MKT1. It also identified two other causative genes (PRP42 and SMD2) encoding for proteins belonging to the same spliceosome complex, suggesting complex epistatic relations. Indeed, the authors demonstrated that the thermotolerant alleles were PRP42 S288c and SMD2 MUCL2817. However, when the PRP42 S288c was introduced in the MUCL2817 genetic background, no additional effect was observed.
By implementing panmictic crosses (advanced intercross lines) between two natural isolates (West African and North American strains), Parts et al. (2011) underlined the role of RAS/cAMP signaling pathway for high-temperature growth by demonstrating the impact of the two paralogues (IRA1 and IRA2) encoding for the RAS inhibitor proteins. Similar phenotypes were also elucidated at the gene level and concern the pyruvate kinase protein Cdc19p (Benjaphokee et al., 2012) or the E3 ubiquitin ligase Rsp5p (Shahsavarani et al., 2012).
All these studies investigated the temperature tolerance of S. cerevisiae strains by evaluating their growing capacities. However, in industrial fermentation, the deleterious effect of high temperature is generally coupled with many other stressful conditions including high ethanol content (Marullo et al., 2009; Mitsumasu et al., 2014), presence of toxins (Taherzadeh et al., 2000; Hasunuma et al., 2011), and sterol or nitrogen depletion (Bely et al., 1990; Marullo et al., 2009).
In a recent study, two genes, OYE2 and VHS1, impacting the fermentation rate at high temperature were identified in a winemaking context (Marullo et al., 2019). In both cases, an SNP was generating a codon-stop insertion impairing the completion of the fermentation above 30°C. Interestingly, for the VHS1 gene, the truncated protein of 371 amino acids confers a more efficient fermentation above 30°C. The recently documented function of Vhs1p (Simpson-Lavy et al., 2017) allows establishing a link between respire–fermentative switch and the fermentation efficiency at high temperature.
Alternatively, some industrial processes (especially food-related fermentations) impose cold and negative temperatures that may have drastic consequences on yeast fitness. If the physiological and molecular mechanism of cold tolerance has been understood (Gunde-Cimerman et al., 2014), few studies have investigated the natural genetic variation impacting cold adaptation.
In a recent work, genes related to lipid remodeling in the plasma membrane or mitochondrial metabolism were identified (García-Ríos et al., 2017). The phenotype investigated was the specific ability to growth at low temperature (15°C) in a synthetic grape juice. The selective genotyping of a pool of progenies derived from two wine-related strains (P24 and P5) was used. The impact of the FPK1 gene encoding for a protein kinase that regulates phospholipid translocation and membrane asymmetry was clearly demonstrated. A substitution R520K in the P24 strain seems to be deleterious for growth at 15°C but not at 28°C. Interestingly, most of the QTLs mapped are located in subtelomeric regions of chromosomes XIII, XV, and XVI. Many of tested genes affect the time to achieve the fermentation, underlining the role of mitochondrial proteins (QCR2 and PET494), oligopeptide transporter (OPT2), and aquaporin (AQY1), which seem particularly important for maintaining a fermentation activity at low temperature. The same team carried out an evolution experiment using the P5 strain and identified an nsSNP in the gene GAA1, which encodes for a protein belonging to the GPI–protein transamidase complex. The introduction of a threonine at the position 108 of this protein enhances the growth fitness at 12°C, suggesting the involvement of mannoproteins in cold adaptation (García-Ríos et al., 2014).
The impact of yeast aquaporins encoded by AQY1 and its paralogue AQY2 was also implicated in freezing and osmotic tolerance. Many loss-of-function mutations in these genes are present in the Saccharomyces cerevisiae wild population (Will et al., 2010). Moreover, differential expression was described for AQY2 and are due to polymorphisms in the promoter region (Fay et al., 2004). In a bakery context, it is well known that functional and overexpressed aquaporins enhance freezing tolerance, while non-functional ones promote osmotic tolerance (Tanghe et al., 2002; Will et al., 2010). This suggests that AQY genes are submitted to balancing selection (Will et al., 2010).
Other mutations enhancing the proline accumulation also improve freezing resistance. The intracellular storage of this amino acid confers resistance to many stresses including freezing, desiccation, oxidation, and ethanol and enhances the fermentation kinetics (see Takagi, 2008 for review; Kitagaki and Takagi, 2014). Mutations in the PRO1 were generated by either the selection of proline-analogue resistant mutants (Takagi et al., 1997) or by PCR random mutagenesis (Sekine et al., 2007). These mutations desensitize Pro1p against the feedback inhibition exerted by proline. Disruption of PUT1 involved in the proline degradation pathway also enhances freezing resistance (Takagi, 2008) and was successfully combined with Pro1p (D154N) or (I150T) mutations in a self-cloned diploid strain (Kaino et al., 2008). Interestingly, a similar combination of a Pro1p mutation with an Mpr1p variant (F65L) enhances both freezing and air-dry resistance (Sasano et al., 2012).
Osmotic Stress
Most of the industrial processes involving Saccharomyces species are characterized by a high sugar content that goes hand in hand with a severe osmotic stress. Improving the resistance to osmotic pressure is therefore essential for achieving high-gravity ethanol fermentations. The osmotic stress response in yeast has been widely investigated (Tamás and Hohmann, 2003), and several mutations affecting osmotolerance have been intensively identified (Hohmann, 2002). Natural or induced mutations have been reported in many distinct pathways, including Hog1p activation (Pbs2pK389M (Reiser et al., 2000) and Sln1p P1148S/P1196L (Fassler et al., 1997)), proline accumulation (PRO1), and water efflux (AQY1-2) (see above). More recently, a QTL mapping study identified genetic causes of osmotic shock associated with very-high-gravity ethanol fermentations using the SGRP-X4 design (Greetham et al., 2014). The alleles RCK2 Q113/S456 of the wine strain DBVPG6765 enhance osmotolerance. This kinase performs a regulatory role in the Hog1p pathway and is involved in osmotic stress response since its overexpression improved growth in high osmotic conditions (Teige et al., 2001).
Resistance to Toxins
In the last decade, the biofuel industry has moved from the first- to second-generation production. This technological progress consists in transforming pentose sugars present in the plant cell walls in ethanol, in addition to hexoses. This additional step required lignocellulose pre-treatments that release aromatic and acidic compounds that are detrimental to the growth of S. cerevisiae (Palmqvist and Hahn-Hägerdal, 2000; Klinke et al., 2004). First of all, yeast has to face acetic acid and other weak acids that decrease the cytosolic pH, inhibit growth, and remodel gene expression (see Palma et al., 2018, for a review).
In a QTL mapping study, the COX20 Q9R allele of the cytochrome c oxidase assembly factor has been identified (Greetham et al., 2014). This allele confers sensitivity to mild concentrations of acetic acid and other weak acids (formic and levulinic) likely linked with the programmed cell death response. In a similar way, the fermentation kinetics in a culture medium spiked with acetic acid was measured for a progeny population derived from a cross between a biofuel strain (ethanol red) and an acetic acid-resistant strain JT22689. A major QTL was mapped on chromosome XVI and was associated with an nsSNP impacting the coding sequence of the gene HAA1. The resistant strain carries a unique polymorphism at the nucleic position 1571 that generates a single amino acid change S505N (Haa1*) (Meijnen et al., 2016). This transcription factor plays a central role in the S. cerevisiae adaptation and tolerance to weak acids (Palma et al., 2018). The Haa1* allele activates the expression of plasma membrane acetate exporters Tpo2p and Tpo3p. Interestingly, another punctual mutation (S135F) promoting acetic acid resistance has been identified by a gTEM approach (Swinnen et al., 2017).
In a second round of QTL mapping performed in a panmictic population (inbreeded lines), four other causative genes have been detected (CUP2, VMA7, GLO1, and DOT5) with the positive contribution of the acetic acid-resistant strain JT22689 (Meijnen et al., 2016). Interestingly, CUP2 is a paralogue of HAA1, suggesting that the expression of acetate transporter is a preferential target of acetic acid resistance. The VMA7 gene is involved in the vacuolar-pH homeostasis and was previously linked to acetic acid resistance. The last two genes, GLO1 and DOT5, were never linked to weak acid resistance. They are related to osmotic and oxidative stresses, respectively.
Adaptive laboratory evolution experiments were also implemented for obtaining mutations enhancing the acetic acid resistance. After ∼50 transfers of alternative microaerobic batch cultivations (with and without acetic acid), five independent evolved cultures showing a strong resistance to acetic acid were obtained. Four causal mutations in the genes ASG1, ADH3, SKS1, and GIS4 were identified by genome sequencing and validated by allele replacement (González-Ramos et al., 2016). Three of them (ADH3, SKS1, and GIS4) were not previously associated with acetic acid tolerance, providing new clues for understanding this complex trait. Interestingly, other genes related to weak acid resistance were found in a winemaking context. Although acetic acid does not impact cell growth in winemaking, a relevant allele impacting the weak acid resistance has been identified by eQTL mapping (Brion et al., 2013). By analyzing the whole-genome expression profile of 44 progeny clones (BY × 59A cross), these authors identified five nsSNPs in the coding sequence of WAR1 gene. This gene encodes for a transcription factor that controls the expression of a plasma membrane ABC transporter responsible (Pdr12p) for organic acid efflux. Allele swapping experiment demonstrated that the WAR1 59A wine yeast allele increases the expression level of PDR12 and enhances the sorbic acid resistance.
Cellulose and hemicellulose hydrolysis released furfural and 5-hydroxy-methyl-furfural (HMF) that have a strong toxic effect on yeast growth and fermentation (Palmqvist and Hahn-Hägerdal, 2000). To date, only one allele of the main alcohol dehydrogenase (ADH1) favoring resistance to HMF was identified in an industrial isolate. The protein sequence reveals multiple amino acid polymorphisms close to the substrate binding pocket (S109P, L116S, and Y294C). This allele has a NADH-dependent HMF reductase activity, which is not present in any other strains and allows reduction of HMF in a nontoxic form (Boaz et al., 2008).
Toxin resistance of lignocellulosic raw material has been recently investigated by two other original approaches. First, a sophisticated strategy combining mutagenesis, genome shuffling, and phenotypic selection was implemented in order to isolate mutations, enhancing resistance to hardwood spent sulfite liquor (Pinel et al., 2015). Among a dozen of putative SNPs identified by whole-genome sequencing, these authors demonstrated that a single mutation in the coding sequence of the gene UBP7 (2466 T > A) conferred a better tolerance to this medium. Second, a GWAS linked 76 SNPs with growth traits measured in complete hydrolysates spiked with a toxin cocktail (Sardi et al., 2018). The association was performed by keeping SNPs from having a minor allele frequency greater than 2% in a collection of 165 fully sequenced S. cerevisiae strains. The effect of allelic variants in the LEU3, MNE1, and SAP190 genes was validated by RHA in different genetic backgrounds (Sardi et al., 2018).
Organoleptic Properties
When they are used in food-related fermentation processes, the fermentation efficiency is not the only technological property desired. Yeast strains are also selected depending on their impact on the composition of several organoleptic compounds. In this last subsection, we reviewed the role of 48 genes and their relative QTNs that influence the organoleptic quality of beverages (Figure 6).
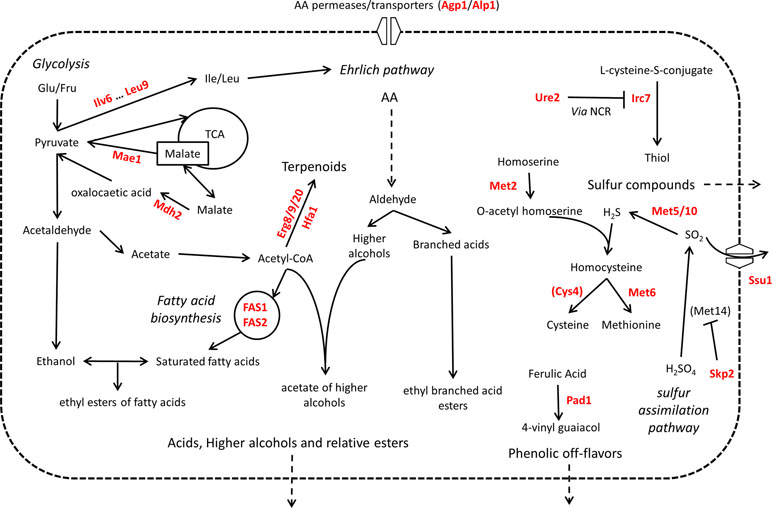
Figure 6 Overview of QTG involved in organoleptic compound production. Proteins highlighted in red are coded by allelic variants that were experimentally validated for their contribution to variation in organoleptic compound production in S. cerevisiae. Indirect relationships are shown in dashed lines. Proteins are between brackets when only the protein of interest of a pathway is shown. TCA = tricarboxylic acid cycle. NCR = nitrogen catabolite repression of transcription.
Higher Alcohols and Esters de Novo Synthesis
Higher alcohols and esters constitute groups of volatile compounds that are de novo produced during the alcoholic fermentation. Their organoleptic impact in fermentation beverage has been widely reviewed (Mason et al., 2000; Sumby et al., 2010), and the main genes and enzymatic activities controlling their biosynthesis have been identified (Malcorps et al., 1991; Verstrepen et al., 2003a; Saerens et al., 2006). Some nsSNPs in the sequence of these enzymes modulate the biosynthesis of these compounds. For example, the brewing yeast strains expressing a long form of the ATF1 genes (LgAFT1) produce more acetate esters of higher alcohols (Verstrepen et al., 2003a). ATF1 is also responsible for the majority of acetate ester production, an undesirable compound with a solvent-like off-flavor (Verstrepen et al., 2003b). To identify genetic factors responsible for the remaining acetate ester production, a QTL mapping study was carried out with or without ATF1 deletion in parental strains (Holt et al., 2018). QTNs in EAT1 and SNF8 were identified, with rare alleles that prevent acetate ester production.
Moreover, many genetic variations affecting proteins in connection with esterification reactions were described. They concern the Ehrlich pathway, the acetyl-CoA production, and the lipid biosynthesis. For example, allelic variation in ILV6 gene stimulates the production level of 2-methyl-propyl acetate by enhancing the biosynthesis of α-ketoisovalerate, its related precursor (Eder et al., 2018). The activity of the FAS complex (fatty acid synthase) has a direct effect on the biosynthesis of many esters. This complex of two proteins (Fas1p and Fas2p) synthesizes fatty acid by the repeated condensation of malonyl-CoA and acetyl-CoA. Since medium fatty acids (C6–C12) are the precursors of ethyl esters, their intracellular concentrations are directly linked to those of ethyl esters. Moreover, the activity FAS complex regulates the cytoplasmic acetyl-CoA pool; this metabolite is the substrate alcohol acetyl-CoA transferase (Atf1p and Atf2p) that produces acetic esters of higher alcohols. Therefore, mutations in the FAS complex affect both acetic and ethyl ester biosynthesis. For instance, ethyl esters biosynthesis in sake (i.e., ethyl-caproate) is modulated by the fas2 G1250C defective allele that could be easily obtained due to their resistance to cerulenin (Aritomi et al., 2004). Interestingly, natural allelic variants in the FAS1 gene explain a similar phenotypic discrepancy in a winemaking context (Eder et al., 2018). Other allelic versions of the FAS2 gene alter the acetylation of higher alcohols like phenyl-ethanol acetate and isoamyl-acetate (Trindade de Carvalho et al., 2017).
The production level of esters and higher alcohols is also impacted by amino acid uptake. Indeed, several nsSNPs in the amino acid transporters encoded by the ALP1, AGP1, and AGP2 genes control the phenotypic variance of acetate esters of higher alcohols observed among a large progeny (Eder et al., 2018). The same authors identified more distant enzymatic activities impacting the intracellular pool of acetyl-CoA (SIR2) or pyruvate (MAE1) that are building blocks of ester precursors. The framework between these precursor pathways and the esterification reactions shed light on the complex determinism of these aromas. The perturbation of folic acid biosynthesis has been also reported to modulate the production of phenyl-ethanol by identifying the deleterious allele ABZ1 via linkage mapping (Steyer et al., 2012). Finally, the deleterious mutation TOR1E216* affecting the regulatory protein Tor1p has been also reported as reducing ester production by an unknown mechanism (Trindade de Carvalho et al., 2017).
Sulfur Compounds
Volatile sulfur compounds are important contributors to the flavor of many foods. These molecules are characterized by a low sensory detection threshold due to the high volatility of sulfur atoms. During alcoholic fermentation, Saccharomyces cerevisiae releases different classes of volatile sulfur compounds that could be de novo produced or bio-converted from precursor molecules. Although present at very low concentration levels (close to ppb), differences within strains strongly contribute to the quality of the final product. A key node of sulfur compound production is centered on the sulfite reductase enzymatic activity involved in the reduction of sulfate into sulfide ions, which ensure the incorporation of a sulfur atom in both methionine and cysteine. A leak of sulfide ions during alcoholic fermentation results in the overproduction of hydrogen sulfide (H2S) and high undesirable off-flavors in beverage industry (Swiegers and Pretorius, 2007).
Several approaches were used to identify genetic variants that might decrease H2S production. By screening an EMS-mutagenized population, several alleles reducing H2S production were found in the CYS4, MET6, MET5, and MET10 genes (Linderholm et al., 2006; Cordente et al., 2009; Linderholm et al., 2010). A linkage analysis revealed natural genetic variations in this pathway affecting the MET1 ( A458T , T511I , G687D , E805K ), MET2 R301G, and MET5 V288X genes that strongly modulate the H2S production during alcoholic fermentation (Huang et al., 2014). Interestingly, the MET2 R301G allele was independently detected by another QTL mapping study as an enhancer of SO2 production (Noble et al., 2015) and is quite frequent in wine European group (Table S2). This finding highlights the fact that a reduced H2S production is often coupled with a high SO2 production as reported for MET2 (Noble et al., 2015), MET5 (Cordente et al., 2009), and MET10 (Cordente et al., 2009; Huang et al., 2014). More interestingly, Noble et al. identified beneficial alleles in the SKP2 gene that reduce both the SO2 and H2S productions. This protein encodes an F-box factor that regulates the abundance of Met14p involved in the first steps of the sulfate reduction pathway as previously demonstrated in a brewery context (Yoshida et al., 2010). By introducing an appropriate allelic variant (T357I) using a backcross strategy, the authors demonstrated that wine strains producing low SO2 amount can be readily selected (Blondin et al., 2014).
Another route for reducing the SO2 production consists in selecting strains devoid of a translocated SSU1 allele (either XV-t-XVI or VIII-t-XVI; see Fermentation Efficiency. Since the Ssu1p transporter pumps the SO2 outside the cell, the less active allelic forms (non-translocated) reduce the concentration of this toxic compound in the fermented matrices. Although non-translocated alleles are not suitable in white grape juice fermentations due to the high level of free SO2, this feature can be used for red-grape juice fermentation (Peltier et al., 2018).
Sulfur volatile compounds may also contribute positively to the aroma of fermented beverages. One of the most achieved examples is given by the bioconversion of volatile thiols (4MMP, 3MH, and 3MHA) that contribute to the typicity of Sauvignon blanc wines (Tominaga and Dubourdieu, 2000). These powerful odorant molecules are derived from cysteinylated and glutathionylated precursors present in grape juice that are converted in volatile thiols by yeast β-lyases (Marullo and Dubourdieu, 2010; Coetzee and du Toit, 2012). The molecular dissection of this bioconversion led to the identification of different β-lyases. A relevant allele of IRC7 explains most of variations in terms of 4MMP production (Roncoroni et al., 2011). The positive allele of IRC7 was carried by a clinical S. cerevisiae isolate having a full-length protein of 400 amino acids able to convert the cysteinylated precursor in its relative aroma, whereas the 60-bp truncated form is not functional. The expression level of this gene is controlled by the nitrogen catabolite repression. Indeed, the use of ure2 mutations is useful for enhancing the bioconversion rate of volatile thiols by enhancing the enzymatic activity of β-lyases (Thibon et al., 2008). Since non-GMO UV-mutations of ure2 can be readily obtained (Marullo et al., 2008), appropriate ure2 and IRC7 400 alleles were implemented for enhancing the volatile thiols bioconversion in order to select yeast strains expressing more intense notes of exotic fruits (Dufour et al., 2013).
Enhancement of Terpenoid Biosynthesis
Terpenoids constitute a wide class of natural molecules that can be produced by metabolic engineering for the production of antibiotics, anticancer, and other medicinal products and also for their aromas and fragrances (Ajikumar et al., 2008). These molecules can be synthetized by rerouting the sterol pathway that was first characterized in Saccharomyces cerevisiae. Natural genetic variations in the ergosterol pathway were identified for increasing the production of geraniol and linalool using a leaky FDP synthase (Erg20p) mutant (Chambon et al., 1991). This allele was introgressed into a wine yeast genetic background by repeated backcrosses (Javelot et al., 1991). The resulting strain has a satisfactory terpene production and aroma profile. Nevertheless, the alteration of the sterol synthesis pathway affected the ethanol tolerance, limiting its use in winemaking. A unique QTL mapping on terpene biosynthesis was carried out and allows the identification of one PDR8 allele that increases nerolidol release in a synthetic media spiked with geraniol (Steyer et al., 2013).
Off-Flavor Reduction
Phenolic off-flavors (POFs), 4-vinyl phenol and 4-vinyl guaiacol, are unwanted compounds produced by yeast during beer fermentation and also in white wine production from phenolic acids present in the must. Therefore, a desirable characteristic of fermenting yeast is the phenotype POF- that is determined by the combined action of two genes localized in the subtelomeric region of chromosome IV. The PAD1 gene encodes for a flavin prenyl-transferase, which catalyzes the formation of a prenylated cofactor required for the ferulic acid decarboxylase encoded by FDC1 (Clausen et al., 1994; Mukai et al., 2010). A recent whole-genome sequencing project (Gallone et al., 2016) reveals that most of brewery strains and also some wine yeast strains have loss-of-function mutations in both PAD1(Q86*, Y98*, and W102*) and FDC1(K54*, Q154*, c.495_496insA, c.864delA, R309*, and W497*). These loss-of-function mutations are strongly correlated with the POF- phenotype (Mertens et al., 2017). Another PAD1 allele (PAD1 D213G) has been identified by QTL mapping (Marullo et al., 2007b) and was used for the selection of various white wine strains to avoid the production of these undesirable compounds (Marullo, 2009).
Flocculation Properties
Flocculation is the yeast cells’ capacity to co-aggregate and form flocs that can provoke cell sedimentation or the creation of a floating biofilm at the broth surface (Vidgren and Londesborough, 2011). These characteristics are important in the brewing industry where flocculation is necessary for yeast sedimentation at the end of the fermentation (Vidgren and Londesborough, 2011) or in winemaking where flor yeast development is essential to sherry wine production (Ossa et al., 1987; Fidalgo et al., 2006). Natural genetic variants that affect the flocculation capacity have been identified especially in the well-studied FLO genes that mostly encode for surface proteins.
The ability to form a floating biofilm is driven by an allelic version of FLO11 in which a rearrangement within the central repeat domain leads to a more hydrophobic protein, and a 111-bp deletion in the promoter increases its expression (Fidalgo et al., 2006). FLO11 not only concerns floating biofilm as the number of repetitions in the Flo11p central domain is also positively correlated to the flocculation strength (Wilkening et al., 2014). The same pattern is also found for Flo1p (Verstrepen et al., 2005). In the S288C genetic background, a premature stop codon in FLO8 impairs flocculation. This gene encodes a transcription factor promoting the FLO1 expression (Li et al., 2013). Conversely, a premature stop codon in the gene SFL1 encoding a repressor of flocculation-related genes increases flocculation (Wilkening et al., 2014). Besides flocculation, the clumpiness of strains may be due to several allelic variants in the AMN1, GPA1, RGA1, CDC28, FLO8, END3, IRA2, MSS11, and TRR1 genes, which have been identified in laboratory conditions. These non-sexual adhesion properties could play a critical role in industry (Pretorius, 2000).
Moving from QTN Detection to Industrial Applications
In the previous section, we listed a catalogue of 147 genes with natural or induced genetic variants that impact quantitative traits of industrial relevance at least in one specific genetic background. Most of them correspond to alleles that could be considered as a promising reservoir of functional levers to modulate metabolic pathways and biological activity of the prime industrial microorganism, Saccharomyces cerevisiae. Since their number is steadily increasing, QTNs should have a profound impact for improving the selection methods of industrial strains in the future. These polymorphisms can be introduced in any desired yeast “chassis” by using GM (genetically modified) organisms as it was perfectly illustrated in the context of bioethanol production (Maurer et al., 2017). Depending on the industrial field and the local legal regulation, these QTNs may also be exploited by implementing more classical breeding strategies (Marullo et al., 2007b; Blondin et al., 2013; Dufour et al., 2013). Although the identification of causative SNPs is now a routine task, their efficiency for improving technological properties by marker-assisted selection (no GM) or allele replacement (GM) is still unpredictable. The two most critical issues that yeast researchers are facing are the incomplete penetrance/expressivity level of identified SNPs and the gene–environment interaction modulating their effect. These issues are well recognized in agronomical science and explain why numerous markers identified in academic studies failed to be translated in the domain of application (Xu and Crouch, 2008). In this last section, we documented examples in yeast and evaluate possible solutions to overcome them.
The Low Penetrance/Expressivity Issue
The penetrance is defined as the proportion of individuals in a population that express a phenotype associated with a specific genetic variation. Indeed, a genetic variation may affect the phenotype of some individuals but remains silent in other backgrounds. A practical illustration of this phenomenon is given by MET10, EAT1, and SNF8 alleles, which do not have the same effect according to the genetic backgrounds (Linderholm et al., 2010; Holt et al., 2018). Therefore, deleterious modifications of a key enzyme in a well-defined pathway do not ensure a predictable phenotype. Low penetrance examples have been particularly well documented in the four parents cross designed by Ed Louis’s group, suggesting that most QTLs are largely cross dependent (Cubillos et al., 2013).
The simplest explanation is given by the fact that most of the strains carry loss-of-function alleles that are preferably detected by QTL analysis. Among the 284 nsSNPs reported, 8% confer a nonsense mutation. Moreover, 33% of the referenced missense mutations have a possible deleterious effect (see Genes and Polymorphisms Impacting Quantitative Traits of Industrial Interest. Therefore, up to 60% of QTNs reviewed have a MAF lower than 5%. This is confirmed by two recent studies showing that rare variants explain a disproportionately large part of the variation of quantitative trait in yeast (Bloom et al., 2019; Fournier et al., 2019). This high proportion is explained by the yeast life history with clearly defined subpopulations that have been evolved mostly by genetic drift in separated habitats (Liti et al., 2009; Peter et al., 2018). Although easy to identify, these loss-of-function alleles are scarcely relevant since they negatively impact the phenotype of interest. This is, for instance, the case of the OYE2Ser77sf, ASP1D142H, and ABZ1S288c alleles that drastically reduce fermentation performances in an enological context (Marullo et al., 2007a; Ambroset et al., 2011; Marullo et al., 2019). As a consequence, the opposite alleles that were defined as favorable have a very low impact since they are present in most of the other genetic backgrounds. To overcome this problem, different approaches were used for identifying minor QTLs (Brem et al., 2005; Sinha et al., 2008; Yang et al., 2013; Holt et al., 2018; Marullo et al., 2019). The segregation of the most impacting loci is eliminated from the segregants by its deletion in the hybrid, by selecting only a part of the segregants for linkage analysis or by generating new segregants with a targeted backcross. These strategies can help to identify QTLs with lower effects that better reflect the true differences between industrial strains.
In some cases, recessive, deleterious mutations have an industrial relevance when loss-of-function is associated with the desired trait as for the POF character (Marullo et al., 2007b; Mertens et al., 2017), and the fil phenotype (Thevelein et al., 1999). In natural isolates, most of these deleterious SNPs are masked in diploid progenitors since they are recessive. Therefore, before starting any QTL detection programs, it is relevant to discard meiotic progenies showing extremely “bad” phenotypes because they would transmit such deleterious SNPs.
Besides that, incomplete penetrance mostly results from genetic interactions between the causative allele identified and many other loci that impair its complete expressivity. Indeed, the Mendelian inheritance of a trait may turn out to be a quantitative in other genetic backgrounds (Hou et al., 2016). This is caused by the presence of modifier (epistatic) loci that modulate the expressivity of a major locus. Such epistatic loci have been identified in different species and in particular in yeast (Yadav and Sinha, 2018). First of all, epistasis concerns genes belonging to the same pathways and in particular between upstream regulator(s) and downstream effector(s). For instance, the favorable GPD1L164P allele had a reducing effect on glycerol yield for biofuel industry. This positive effect is only observed when two of its transcriptional factors (HOT1 and SMP1) have the laboratory strain genotype (Hubmann et al., 2013b). Another example is given by the positive allelic combination of the fully active β-lyase Irc7pLT and the loss-of-function allele of the regulator Ure2pG181E that enhances the bioconversion of volatile thiols from cysteine conjugates precursors of grape juice (Dufour et al., 2013). In the same way, a strong positive epistatic interaction was found between FLX1 and MDH2 genes. Both genes play a role in the Krebs cycle, and the combination of FLX1SA and MDH2WE results in high levels of succinic acid during wine fermentation (Salinas et al., 2012). Besides these “obvious” metabolic connections, other interactions have been identified between functionally unrelated couples of genes. This is the case of NCS2–MKT1 (Sinha et al., 2008) and END3–RHO2 (Sinha et al., 2006), which strongly impacted HTG phenotype in the BY–RM11 cross. Once identified and understood, these epistatic relationships would be useful for dramatically enhancing a phenotype of interest by introducing suitable allelic combinations. Interestingly, a larger genetic modification such as aneuploidies (Sirr et al., 2015) may also play a modifier role and would be more difficult to control.
Genetic per Environment (GxE) Interactions
Environmental conditions represent the second major factor that drastically modifies the expressivity of genetic loci. It is noteworthy that among individuals of the same species, phenotypic plasticity is frequently observed (Veerkamp et al., 1994; Pigliucci and Kolodynska, 2002). These different non-parallel norms of reaction are due to GxE interactions. In yeast, the systematic research of genetic loci interacting with the environment has been achieved in several fundamental studies focusing on whole-genome expression level (Smith and Kruglyak, 2008; Gagneur et al., 2009), or growth traits (Gagneur et al., 2009; Bhatia et al., 2014; Wei and Zhang, 2017; Yadav et al., 2016) measured in divergent laboratory conditions. GxE interactions observed can be divided into two broad classes (Yadav and Sinha, 2018).
First, the effect of a locus may be environment-specific, reflecting the presence of a gene/allele adapted to a particular composition of the medium. This is often observed for traits related to a specific sugar transport (MAL13) (Bhatia et al., 2014) or for the adaptation to a specific toxin (SSU1) (Pérez-Ortín et al., 2002; Zimmer et al., 2014). Second, GxE interactions may explain phenotypic trade-offs illustrated by individuals showing a contrasted fitness across a pair of environments (Yadav et al., 2015; Wei and Zhang, 2017). These antagonistic effects are mostly due to the presence of one allele that has been positively selected in one environment but shows a negative effect in another one. Such contrasted responses are frequently observed when phenotyping is done in drastically different conditions such as respiratory versus fermentative conditions. In such cases, allelic variations of key regulatory genes have been detected in IRA2 (Smith and Kruglyak, 2008) or HAP1 (Wei and Zhang, 2017), which are both involved in metabolic pathway switches. Since most of these studies had a fundamental focus, the reaction norms were investigated in very divergent laboratory media in order to enhance the phenotypic plasticity. Therefore, it is likely that the importance of antagonistic effects claimed is certainly biased by the drastic physiological conditions imposed.
Pleiotropic QTLs showing large effects with both beneficial and negative impacts on species adaptation constitute an interesting case of GxE interactions. The description of pleiotropic QTLs/genes/SNPs has been achieved in several studies for IRA2 (Yadav et al., 2015), MKT1 (Deutschbauer and Davis, 2005; Fay, 2013), CYS4 (Kim et al., 2009), AQY1 (Will et al., 2010), and SSU1 (Peltier et al., 2018). In some cases, these loci showed antagonistic effects and could reflect balancing selection since the alleles involved are found in similar frequency in natural populations. The aquaporin genes (AQY1 and AQY2) are a notorious case of alleles under balancing selection with a possible effect in a bakery context. Indeed, loss of aquaporin reduces freeze–thaw tolerance but increases fitness in high-sugar environments, two conditions encountered in the bread industry (Will et al., 2010). Another case is given by the translocation XV-t-XVI that influences the expression level of the sulfite pump SSU1 (Zimmer et al., 2014). Although very beneficial for initiating the alcoholic fermentation in the presence of SO2, yeast strains presenting this translocation have a reduced fermentation rate in the late steps of wine fermentation (Peltier et al., 2018).
In agronomy, QTL programs take into account the environmental effect by achieving phenotyping in various conditions. The first benefit of this strategy is the gain of power detection of minor QTLs. The second one allows the detection of robust loci having an effect whatever the environment is, which is an obvious asset for achieving marker-assisted selection. In a recent work, QTL mapping was achieved in three natural grape juices using two independent F1-cross derived from wine starters. The conditions applied represent extreme environmental conditions from an enological point of view (different grape juice colors, various amounts of sugar and nitrogen, and different micro-oxygenation conditions). In such conditions, up to 72% of the mapped QTLs have the same effect regardless of the environment. This observation suggests that when QTL mapping is carried out with genetic rootstock adapted to the specific industrial context, the GxE interactions are quite moderate. Therefore, most of the mapped QTLs would be robust in various enological environments (Peltier et al., 2018).
Selecting an Appropriate Genetic Background
Until now, most QTNs were identified by using less than 20 yeast backgrounds (Figure 7). Usually, an elite strain with relevant features has been crossed with the laboratory background (S288c) or with one (or few) strains derived from another subpopulation. However, for achieving relevant research of biotechnological interest, the use of an “outgroup” partner may represent an important risk. This is particularly true for lab strains that have been cultivated for numerous generations in a controlled environment (growth on rich media at steady temperature). The use of various parental strains originated from distinct subpopulations (SGRP4X design) may have the same effect. Indeed, the NA, SA, WE, and WA strains belong to distinct clean lineages that have been evolved mostly by genetic drift in separated habitat. Although locally neutral in one environment, these alleles would have a deleterious effect on each other (Zörgö et al., 2013). Therefore, the selection of distant parental strains may enhance the chance to find QTNs with low expressivity by introducing a pool of not adapted alleles that in fine cause a drastic loss of fitness.
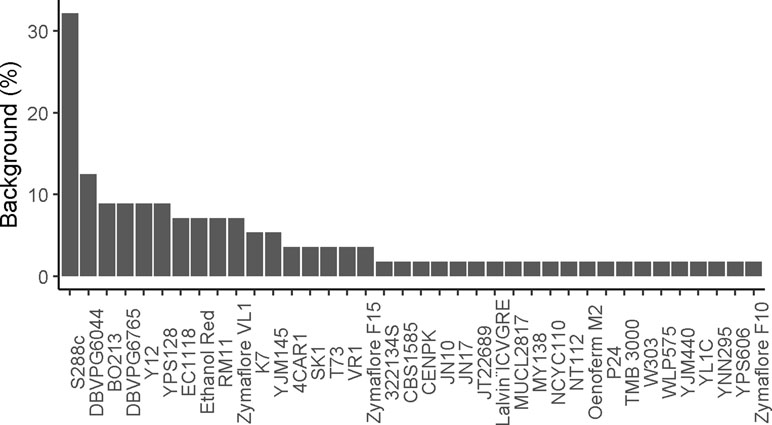
Figure 7 Proportion of genetic background used for QTL mapping studies. The percentage of time a genetic background was used in reported studies.
The cross of unrelated lineages also regroups a large pool of alleles that had never co-evolved together. This likely enhances epistatic interactions that have a negative impact on QTL expressivity. Then, we believed that the introduction of deleterious alleles must be prevented as far as possible. A rational way consists in using a set of strains adapted to the environment of interest. Indeed, by using such adapted strains, the pool of alleles submitted to positive selection would increase, while those having deleterious impact would be reduced. In most of the studies, parental strains showed very opposite trait values, which is not an obligation. In theory, two conditions are required for achieving linkage analyses. The first is to capture enough genetic polymorphisms for building a fine grain genetic map. This even can be obtained within strains of the same subpopulations. The second is a wide phenotypic segregation that is not necessary depending on parental traits. Recently, we demonstrated that the phenotypic and genetic distance within pairs of parental strains does not affect neither the phenotypic segregation nor the efficiency of QTL detection (Peltier et al., 2018). By extrapolation, the cross of two optimal strains with a sufficient phenotypic diversity would have the benefit to fix many positive alleles and allow the segregation of other QTLs that would also contribute positively to the phenotype.
Concluding Remarks
This review provides a first compendium of QTNs of biotechnological interest belonging to the Saccharomyces cerevisiae species. This emphasizes the success of quantitative yeast genetics for identifying relevant natural (or induced) genetic variations that confers technological properties. The SNPs reported here will constitute a rich reservoir of genetic variations useful for improving the technological properties of industrial strains by using breeding or genome editing strategies. However, for bridging the gap between the identification of causative SNP and their routine exploitation, the complex architecture of quantitative traits needs to be better understood.
Data Availability
The datasets analyzed for this study can be found in Peter et al. (2018).
Author Contributions
EP and PM wrote the first draft of the manuscript. AF and JS wrote sections of the manuscript and reviewed and edited the original draft.
Conflict of Interest Statement
EP and PM are employed by LAFFORT. The remaining authors declare that the research was conducted in the absence of any commercial or financial relationships that could be construed as a potential conflict of interest.
Abbreviations
3′UTR, 3′ untranslated transcribed region; ALE, adaptive laboratory evolution; CNVs, copy number variants; eQTL, expression QTL; gTME, global transcription machinery engineering; GM, genetically modified; GWAS, genome-wide association studies; GxE, genetic per environment; HMF, 5-hydroxy-methyl-furfural; InDels, insertions/deletions; MAF, minor allele frequency; MAS, marker-assisted selection; nsSNP, non-synonymous SNP; sSNP, synonymous SNP; QTL, quantitative trait loci; QTG, quantitative trait genes; QTN, quantitative trait nucleotides; RHA, reciprocal hemizygosity assay; SNP, single-nucleotide polymorphisms.
Supplementary Materials
The Supplementary Material for this article can be found online at: https://www.frontiersin.org/articles/10.3389/fgene.2019.00683/full#supplementary-material.
Table S1 | Description of the QTGs reported.
Table S2 | Allelic frequencies of the QTNs reported.
Table S3 | Distribution of the allelic frequencies among isolate origins.
References
Ajikumar, P. K., Tyo, K., Carlsen, S., Mucha, O., Phon, T. H., Stephanopoulos, G. (2008). Terpenoids: opportunities for biosynthesis of natural product drugs using engineered microorganisms. Mol. Pharm. 5, 167–190. doi: 10.1021/mp700151b
Akada, R., Matsuo, K., Aritomi, K., Nishizawa, Y. (1999). Construction of recombinant sake yeast containing a dominant FAS2 mutation without extraneous sequences by a two-step gene replacement protocol. J. Biosci. Bioeng. 87, 43–48. doi: 10.1016/S1389-1723(99)80006-1
Albertin, W., Marullo, P., Aigle, M., Dillmann, C., de Vienne, D., Bely, M., et al. (2011). Population size drives industrial Saccharomyces cerevisiae alcoholic fermentation and is under genetic control. Appl. Environ. Microbiol. 77, 2772–2784. doi: 10.1128/AEM.02547-10
Almeida, P., Gonçalves, C., Teixeira, S., Libkind, D., Bontrager, M., Masneuf-Pomarède, I., et al. (2014). A Gondwanan imprint on global diversity and domestication of wine and cider yeast Saccharomyces uvarum. Nat. Commun. 5, 4044. doi: 10.1038/ncomms5044
Alper, H., Moxley, J., Nevoigt, E., Fink, G. R., Stephanopoulos, G. (2006). Engineering yeast transcription machinery for improved ethanol tolerance and production. Science 314, 1565–1568. doi: 10.1126/science.1131969
Ambroset, C., Petit, M., Brion, C., Sanchez, I., Delobel, P., Guerin, C., et al., (2011). Deciphering the molecular basis of wine yeast fermentation traits using a combined genetic and genomic approach. G3 (Bethesda) 1, 263–281. doi: 10.1534/g3.111.000422
Aritomi, K., Hirosawa, I., Hoshida, H., Shigii, M., Nishizawa, Y., Susumu, K., et al. (2004). Self-cloning yeast strains containing novel FAS2 mutations produce a higher amount of ethyl caproate in Japanese sake. Biosci. Biotechnol. Biochem. 68, 206–214. doi: 10.1271/bbb.68.206
Avrahami-Moyal, L., Engelberg, D., Wenger, J. W., Sherlock, G., Braun, S. (2012). Turbidostat culture of Saccharomyces cerevisiae W303-1A under selective pressure elicited by ethanol selects for mutations in SSD1 and UTH1. FEMS Yeast Res. 12, 521–533. doi: 10.1111/j.1567-1364.2012.00803.x
Barbosa, R., Pontes, A., Santos, R. O., Montandon, G. G., de Ponzzes-Gomes, C. M., Morais, P. B., et al. (2018). Multiple rounds of artificial selection promote microbe secondary domestication—the case of Cachaça yeasts. Genome Biol. Evol. 10, 1939–1955. doi: 10.1093/gbe/evy132
Bauer, F. F., Pretorius, I. S. (2000). Yeast stress response and fermentation efficiency: how to survive the making of wine—a review. S Afr. J. Enol. Vitic. 21, 27–51. doi: 10.21548/21-1-3557
Beck, T., Hastings, R. K., Gollapudi, S., Free, R. C., Brookes, A. J. (2014). GWAS Central: a comprehensive resource for the comparison and interrogation of genome-wide association studies. Eur. J. Hum. Genet. 22, 949–952. doi: 10.1038/ejhg.2013.274
Bell, S.-J., Henschke, P. A. (2005). Implications of nitrogen nutrition for grapes, fermentation and wine. Aust. J. Grape Wine Res. 11, 242–295. doi: 10.1111/j.1755-0238.2005.tb00028.x
Bely, M., Sablayrolles, J. M., Barre, P. (1990). Description of alcoholic fermentation kinetics: its variability and significance. Am. J. Enol. Vitic. 41, 319–324.
Benjaphokee, S., Koedrith, P., Auesukaree, C., Asvarak, T., Sugiyama, M., Kaneko, Y., et al. (2012). CDC19 encoding pyruvate kinase is important for high-temperature tolerance in Saccharomyces cerevisiae. New Biotechnol. 29, 166–176. doi: 10.1016/j.nbt.2011.03.007
Berthels, N. J., Cordero Otero, R. R., Bauer, F. F., Thevelein, J. M., Pretorius, I. S. (2004). Discrepancy in glucose and fructose utilisation during fermentation by Saccharomyces cerevisiae wine yeast strains. FEMS Yeast Res. 4, 683–689. doi: 10.1016/j.femsyr.2004.02.005
Bhatia, A., Yadav, A., Zhu, C., Gagneur, J., Radhakrishnan, A., Steinmetz, L. M., et al. (2014). Yeast growth plasticity is regulated by environment-specific multi-QTL interactions. G3 (Bethesda) 4, 769–777. doi: 10.1534/g3.113.009142
Blondin, B., NOBLE, J., Sanchez, I., Agronomique, I.N.D.L.R., Supagro, C.I.D.S.E.S.A. (Montpellier (2013). Méthode de contrôle de la production de sulfites, d’hydrogène sulfureux et d’acétaldéhyde par des levures.
Blondin, B., NOBLE, J., Sanchez, I. (2014). Méthode de contrôle de la production de sulfites, d’hydrogène sulfureux et d’acétaldéhyde par des levures (Google Patents).
Bloom, J. S., Boocock, J., Treusch, S., Sadhu, M. J., Day, L., Oates-Barker, H., et al. (2019). Rare variants contribute disproportionately to quantitative trait variation in yeast. BioRxiv, 607291. doi: 10.1101/607291
Boaz, L., M., A. J. R., Peter, R., Bärbel, H.-H., Marie, G.-G. (2008). Identification of an NADH-dependent 5-hydroxymethylfurfural-reducing alcohol dehydrogenase in Saccharomyces cerevisiae. Yeast 25, 191–198. doi: 10.1002/yea.1578
Borneman, A. R., Forgan, A. H., Kolouchova, R., Fraser, J. A., Schmidt, S. A. (2016). Whole genome comparison reveals high levels of inbreeding and strain redundancy across the spectrum of commercial wine strains of Saccharomyces cerevisiae. G3 (Bethesda) 6, 957–971. doi: 10.1534/g3.115.025692
Brachi, B., Morris, G. P., Borevitz, J. O. (2011). Genome-wide association studies in plants: the missing heritability is in the field. Genome Biol. 12, 232. doi: 10.1186/gb-2011-12-10-232
Brem, R. B., Storey, J. D., Whittle, J., Kruglyak, L. (2005). Genetic interactions between polymorphisms that affect gene expression in yeast. Nature 436, 701–703. doi: 10.1038/nature03865
Brice, C., Sanchez, I., Bigey, F., Legras, J.-L., Blondin, B. (2014). A genetic approach of wine yeast fermentation capacity in nitrogen-starvation reveals the key role of nitrogen signaling. BMC Genomics 15, 495. doi: 10.1186/1471-2164-15-495
Brion, C., Ambroset, C., Sanchez, I., Legras, J.-L., Blondin, B. (2013). Differential adaptation to multi-stressed conditions of wine fermentation revealed by variations in yeast regulatory networks. BMC Genomics 14, 681. doi: 10.1186/1471-2164-14-681
Bro, C., Knudsen, S., Regenberg, B., Olsson, L., Nielsen, J. (2005). Improvement of galactose uptake in Saccharomyces cerevisiae through overexpression of phosphoglucomutase: example of transcript analysis as a tool in inverse metabolic engineering. Appl. Environ. Microbiol. 71, 6465–6472. doi: 10.1128/AEM.71.11.6465-6472.2005
Brown, C. A., Murray, A. W., Verstrepen, K. J. (2010). Rapid expansion and functional divergence of subtelomeric gene families in yeasts. Curr. Biol. 20, 895–903. doi: 10.1016/j.cub.2010.04.027
Carlson, M., Botstein, D. (1983). Organization of the SUC gene family in Saccharomyces. Mol. Cell. Biol. 3, 351–359. doi: 10.1128/MCB.3.3.351
Chambon, C., Ladeveze, V., Servouse, M., Blanchard, L., Javelot, C., Vladescu, B., et al. (1991). Sterol pathway in yeast. Identification and properties of mutant strains defective in mevalonate diphosphate decarboxylase and farnesyl diphosphate synthetase. Lipids 26, 633–636. doi: 10.1007/BF02536428
Chen, X., Zhao, F., Xu, S. (2010). Mapping environment-specific quantitative trait loci. Genetics 186, 1053–1066. doi: 10.1534/genetics.110.120311
Ching, A., Caldwell, K. S., Jung, M., Dolan, M., Smith, O. S., Tingey, S., et al. (2002). SNP frequency, haplotype structure and linkage disequilibrium in elite maize inbred lines. BMC Genet. 3, 19. doi: 10.1186/1471-2156-3-19
Clausen, M., Lamb, C. J., Megnet, R., Doerner, P. W. (1994). PAD1 encodes phenylacrylic acid decarboxylase which confers resistance to cinnamic acid in Saccharomyces cerevisiae. Gene 142, 107–112. doi: 10.1016/0378-1119(94)90363-8
Clomburg, J. M., Gonzalez, R. (2013). Anaerobic fermentation of glycerol: a platform for renewable fuels and chemicals. Trends Biotechnol. 31, 20–28. doi: 10.1016/j.tibtech.2012.10.006
Coetzee, C., du Toit, W. J. (2012). A comprehensive review on Sauvignon blanc aroma with a focus on certain positive volatile thiols. Food Res. Int. 45, 287–298. doi: 10.1016/j.foodres.2011.09.017
Cordente, A. G., Heinrich, A., Pretorius, I. S., Swiegers, J. H. (2009). Isolation of sulfite reductase variants of a commercial wine yeast with significantly reduced hydrogen sulfide production. FEMS Yeast Res. 9, 446–459. doi: 10.1111/j.1567-1364.2009.00489.x
Cordente, A. G., Cordero-Bueso, G., Pretorius, I. S., Curtin, C. D. (2013). Novel wine yeast with mutations in YAP1 that produce less acetic acid during fermentation. FEMS Yeast Res. 13, 62–73. doi: 10.1111/1567-1364.12010
Cubillos, F. A. (2016). Exploiting budding yeast natural variation for industrial processes. Curr. Genet. 62, 745–751. doi: 10.1007/s00294-016-0602-6
Cubillos, F. A., Parts, L., Salinas, F., Bergström, A., Scovacricchi, E., Zia, A., et al. (2013). High-resolution mapping of complex traits with a four-parent advanced intercross yeast population. Genetics 195, 1141–1155. doi: 10.1534/genetics.113.155515
Cubillos, F. A., Brice, C., Molinet, J., Tisné, S., Abarca, V., Tapia, S. M., et al. (2017). Identification of nitrogen consumption genetic variants in yeast through QTL mapping and bulk segregant RNA-Seq analyses. G3 (Bethesda) 7, 1693–1705. doi: 10.1534/g3.117.042127
Demogines, A., Smith, E., Kruglyak, L., Alani, E. (2008). Identification and dissection of a complex DNA repair sensitivity phenotype in baker’s yeast. PLoS Genet. 4, e1000123. doi: 10.1371/journal.pgen.1000123
Denis, C. L., Young, E. T. (1983). Isolation and characterization of the positive regulatory gene ADR1 from Saccharomyces cerevisiae. Mol. Cell. Biol. 3, 360–370. doi: 10.1128/MCB.3.3.360
Dequin, S., Escudier, J.-L., Bely, M., Noble, J., Albertin, W., Masneuf-Pomarède, I., et al. (2017). How to adapt winemaking practices to modified grape composition under climate change conditions. OENO One 51, 205. doi: 10.20870/oeno-one.2017.51.2.1584
Deutschbauer, A. M., Davis, R. W. (2005). Quantitative trait loci mapped to single-nucleotide resolution in yeast. Nat. Genet. 37, 1333–1340. doi: 10.1038/ng1674
Dhar, R., Sägesser, R., Weikert, C., Yuan, J., Wagner, A. (2011). Adaptation of Saccharomyces cerevisiae to saline stress through laboratory evolution. J. Evol. Biol. 24, 1135–1153. doi: 10.1111/j.1420-9101.2011.02249.x
Diezmann, S., Dietrich, F. S. (2011). Oxidative stress survival in a clinical Saccharomyces cerevisiae isolate is influenced by a major quantitative trait nucleotide. Genetics 188, 709–722. doi: 10.1534/genetics.111.128256
Doniger, S. W., Kim, H. S., Swain, D., Corcuera, D., Williams, M., Yang, S. P., et al. (2008). A catalog of neutral and deleterious polymorphism in yeast. PLoS Genet. 4, e1000183. doi: 10.1371/journal.pgen.1000183
Dufour, M., Zimmer, A., Thibon, C., Marullo, P. (2013). Enhancement of volatile thiol release of Saccharomyces cerevisiae strains using molecular breeding. Appl. Microbiol. Biotechnol. 97, 5893–5905. doi: 10.1007/s00253-013-4739-7
Duitama, J., Sánchez-Rodríguez, A., Goovaerts, A., Pulido-Tamayo, S., Hubmann, G., Foulquié-Moreno, M. R., et al. (2014). Improved linkage analysis of quantitative trait loci using bulk segregants unveils a novel determinant of high ethanol tolerance in yeast. BMC Genomics 15, 207. doi: 10.1186/1471-2164-15-207
Eder, M., Sanchez, I., Brice, C., Camarasa, C., Legras, J.-L., Dequin, S. (2018). QTL mapping of volatile compound production in Saccharomyces cerevisiae during alcoholic fermentation. BMC Genomics 19, 166. doi: 10.1186/s12864-018-4562-8
Fassler, J. S., Gray, W. M., Malone, C. L., Tao, W., Lin, H., Deschenes, R. J. (1997). Activated alleles of yeast SLN1 increase Mcm1-dependent reporter gene expression and diminish signaling through the Hog1 osmosensing pathway. J. Biol. Chem. 272, 13365–13371. doi: 10.1074/jbc.272.20.13365
Fay, J. C. (2013). The molecular basis of phenotypic variation in yeast. Curr. Opin. Genet. Dev. 23, 672–677. doi: 10.1016/j.gde.2013.10.005
Fay, J. C., McCullough, H. L., Sniegowski, P. D., Eisen, M. B. (2004). Population genetic variation in gene expression is associated with phenotypic variation in Saccharomyces cerevisiae. Genome Biol. 5, R26. doi: 10.1186/gb-2004-5-4-r26
Fidalgo, M., Barrales, R. R., Ibeas, J. I., Jimenez, J. (2006). Adaptive evolution by mutations in the FLO11 gene. Proc. Natl. Acad. Sci. U.S.A 103, 11228–11233. doi: 10.1073/pnas.0601713103
Fournier, T., Saada, O. A., Hou, J., Peter, J., Caudal, E., Schacherer, J. (2019). Extensive impact of low-frequency variants on the phenotypic landscape at population-scale. BioRxiv, 609917. doi: 10.1101/609917
Gagneur, J., Sinha, H., Perocchi, F., Bourgon, R., Huber, W., Steinmetz, L. M. (2009). Genome-wide allele- and strand-specific expression profiling. Mol. Syst. Biol. 5, 274. doi: 10.1038/msb.2009.31
Gallone, B., Steensels, J., Prahl, T., Soriaga, L., Saels, V., Herrera-Malaver, B., et al. (2016). Domestication and divergence of Saccharomyces cerevisiae beer yeasts. Cell 166, 1397–1410.e16. doi: 10.1016/j.cell.2016.08.020
García-Ríos, E., López-Malo, M., Guillamón, J. (2014). Global phenotypic and genomic comparison of two Saccharomyces cerevisiae wine strains reveals a novel role of the sulfur assimilation pathway in adaptation at low temperature fermentations. BMC Genomics 15, 1059. doi: 10.1186/1471-2164-15-1059
García-Ríos, E., Morard, M., Parts, L., Liti, G., Guillamón, J. M. (2017). The genetic architecture of low-temperature adaptation in the wine yeast Saccharomyces cerevisiae. BMC Genomics 18, 159. doi: 10.1186/s12864-017-3572-2
García-Ríos, E., Nuévalos, M., Barrio, E., Puig, S., Guillamón, J. M. (2019). A new chromosomal rearrangement improves the adaptation of wine yeasts to sulfite. Environ. Microbiol. 21, 1771–1781. doi: 10.1111/1462-2920.14586
Gasch, A. P. (2003). “The environmental stress response: a common yeast response to diverse environmental stresses,” in Yeast stress responses. Eds. Hohmann, S., Mager, W. H. Berlin, Heidelberg: Springer, 11–57. doi: 10.1007/3-540-45611-2_2
Gibson, B. R., Lawrence, S. J., Leclaire, J. P. R., Powell, C. D., Smart, K. A. (2007). Yeast responses to stresses associated with industrial brewery handling. FEMS Microbiol. Rev. 31, 535–569. doi: 10.1111/j.1574-6976.2007.00076.x
Giudici, P., Zambonelli, C. (1992). Biometric and genetic study on acetic acid production for breeding of wine yeast. Am. J. Enol. Vitic. 43, 370–374.
González-Ramos, D., Gorter de Vries, A. R., Grijseels, S. S., van Berkum, M. C., Swinnen, S., van den Broek, M., et al. (2016). A new laboratory evolution approach to select for constitutive acetic acid tolerance in Saccharomyces cerevisiae and identification of causal mutations. Biotechnol. Biofuels 9, 173. doi: 10.1186/s13068-016-0583-1
Grant, D., Nelson, R. T., Cannon, S. B., Shoemaker, R. C. (2010). SoyBase, the USDA-ARS soybean genetics and genomics database. Nucleic Acids Res. 38, D843–D846. doi: 10.1093/nar/gkp798
Greetham, D., Wimalasena, T. T., Leung, K., Marvin, M. E., Chandelia, Y., Hart, A. J., et al. (2014). The genetic basis of variation in clean lineages of Saccharomyces cerevisiae in response to stresses encountered during bioethanol fermentations. PLoS One 9, e103233. doi: 10.1371/journal.pone.0103233
Gresham, D., Hong, J. (2014). The functional basis of adaptive evolution in chemostats. FEMS Microbiol. Rev., 39, 1–19. doi: 10.1111/1574-6976.12082
Griffiths, A. J., Miller, J. H., Suzuki, D. T., Lewontin, R. C., Gelbart, W. M., Griffiths, A. J., et al. (2000). An introduction to genetic analysis. (San Francisco, CA:W. H. Freeman).
Guillaume, C., Delobel, P., Sablayrolles, J. M., Blondin, B. (2007). Molecular basis of fructose utilization by the wine yeast Saccharomyces cerevisiae: a mutated HXT3 allele enhances fructose fermentation. Appl. Environ. Microbiol. 73, 2432–2439. doi: 10.1128/AEM.02269-06
Gunde-Cimerman, N., Plementitas, A., Buzzini, P. (2014). “Cold-adapted yeasts,” in Cold-adapted yeasts. Eds. Buzzini, P., Margesin, R. (Berlin, Heidelberg: Springer Berlin Heidelberg), 225–242. doi: 10.1007/978-3-642-39681-6_10
Gutiérrez, A., Beltran, G., Warringer, J., Guillamón, J. M. (2013). Genetic basis of variations in nitrogen source utilization in four wine commercial yeast strains. PLoS One 8, e67166. doi: 10.1371/journal.pone.0067166
Hahn-Hägerdal, B., Karhumaa, K., Larsson, C. U., Gorwa-Grauslund, M., Görgens, J., van Zyl, W. H. (2005). Role of cultivation media in the development of yeast strains for large scale industrial use. Microb. Cell Fact. 4, 31. doi: 10.1186/1475-2859-4-31
Hasunuma, T., Sanda, T., Yamada, R., Yoshimura, K., Ishii, J., Kondo, A. (2011). Metabolic pathway engineering based on metabolomics confers acetic and formic acid tolerance to a recombinant xylose-fermenting strain of Saccharomyces cerevisiae. Microb. Cell Fact. 10, 2. doi: 10.1186/1475-2859-10-2
Higgins, V. J., Braidwood, M., Bissinger, P., Dawes, I. W., Attfield, P. V. (1999). Leu343Phe substitution in the Malx3 protein of Saccharomyces cerevisiae increases the constitutivity and glucose insensitivity of MAL gene expression. Curr. Genet. 35, 491–498. doi: 10.1007/s002940050444
Hohmann, S. (2002). Osmotic stress signaling and osmoadaptation in yeasts osmotic. Microbiol. Mol. Biol. Rev. 66, 300–372. doi: 10.1128/MMBR.66.2.300-372.2002
Holt, S., Carvalho, B. T., Foulquié-Moreno, M. R., Thevelein, J. M. (2018). Polygenic analysis in absence of major effector ATF1 unveils novel components in yeast flavor ester biosynthesis. MBio 9, e01279–18. doi: 10.1128/mBio.01279-18
Hong, J., Gresham, D. (2014). Molecular specificity, convergence and constraint shape adaptive evolution in nutrient-poor environments. PLoS Genet. 10, e1004041. doi: 10.1371/journal.pgen.1004041
Hou, J., Sigwalt, A., Fournier, T., Pflieger, D., Peter, J., de Montigny, J., et al. (2016). The hidden complexity of Mendelian traits across natural yeast populations. Cell Rep. 16, 1106–1114. doi: 10.1016/j.celrep.2016.06.048
Huang, C., Roncoroni, M., Gardner, R. C. (2014). MET2 affects production of hydrogen sulfide during wine fermentation. Appl. Microbiol. Biotechnol. 98, 7125–7135. doi: 10.1007/s00253-014-5789-1
Hubmann, G., Mathé, L., Foulquié-Moreno, M. R., Duitama, J., Nevoigt, E., Thevelein, J. M. (2013a). Identification of multiple interacting alleles conferring low glycerol and high ethanol yield in Saccharomyces cerevisiae ethanolic fermentation. Biotechnol. Biofuels 6, 87. doi: 10.1186/1754-6834-6-87
Hubmann, G., Foulquié-Moreno, M. R., Nevoigt, E., Duitama, J., Meurens, N., Pais, T. M., et al. (2013b). Quantitative trait analysis of yeast biodiversity yields novel gene tools for metabolic engineering. Metab. Eng. 17, 68–81. doi: 10.1016/j.ymben.2013.02.006
Ibstedt, S., Stenberg, S., Bages, S., Gjuvsland, A. B., Salinas, F., Kourtchenko, O., et al. (2014). Concerted evolution of life stage performances signals recent selection on yeast nitrogen use. Mol. Biol. Evol. 32, 153–161. doi: 10.1093/molbev/msu285
Inokoshi, J., Tomoda, H., Hashimoto, H., Watanabe, A., Takeshima, H., Omura, S. (1994). Cerulenin-resistant mutants of Saccharomyces cerevisiae with an altered fatty acid synthase gene. Mol. Gen. Genet. 244, 90–96. doi: 10.1007/BF00280191
Jara, M., Cubillos, F., García, V., Salinas, F., Aguilera, O., Liti, G., et al. (2014). Mapping genetic variants underlying differences in the central nitrogen metabolism in fermenter yeasts. PloS One 9, e86533. doi: 10.1371/journal.pone.0086533
Javelot, C., Girard, P., Colonna-Ceccaldi, B., Vladescu, B. (1991). Introduction of terpene-producing ability in a wine strain of Saccharomyces cerevisiae. J. Biotechnol. 21, 239–251. doi: 10.1016/0168-1656(91)90045-W
Jiranek, V., Langridge, P., Henschke, P. A. (1995). Amino acid and ammonium utilization by Saccharomyces cerevisiae wine yeasts from a chemically defined medium. Am. J. Enol. Vitic. 46, 75–83.
Kaino, T., Tateiwa, T., Mizukami-Murata, S., Shima, J., Takagi, H. (2008). Self-cloning baker’s yeasts that accumulate proline enhance freeze tolerance in doughs. Appl. Environ. Microbiol. 74, 5845–5849. doi: 10.1128/AEM.00998-08
Kim, H. S., Huh, J., Fay, J. C. (2009). Dissecting the pleiotropic consequences of a quantitative trait nucleotide. FEMS Yeast Res. 9, 713–722. doi: 10.1111/j.1567-1364.2009.00516.x
Kim, N.-R., Yang, J., Kwon, H., An, J., Choi, W., Kim, W. (2013). Mutations of the TATA-binding protein confer enhanced tolerance to hyperosmotic stress in Saccharomyces cerevisiae. Appl. Microbiol. Biotechnol. 97, 8227–8238. doi: 10.1007/s00253-013-4985-8
Kitagaki, H., Takagi, H. (2014). Mitochondrial metabolism and stress response of yeast: applications in fermentation technologies. J. Biosci. Bioeng. 117 (4), 383–393. doi: 10.1016/j.jbiosc.2013.09.011
Klinke, H. B., Thomsen, A. B., Ahring, B. K. (2004). Inhibition of ethanol-producing yeast and bacteria by degradation products produced during pre-treatment of biomass. Appl. Microbiol. Biotechnol. 66, 10–26. doi: 10.1007/s00253-004-1642-2
Kutyna, D. R., Varela, C., Henschke, P., Chambers, P. J., Stanley, G. A. (2010). Microbiological approaches to lowering ethanol concentration in wine. Trends Food Sci. Technol 21, 293–302. doi: 10.1016/j.tifs.2010.03.004
Kvitek, D. J., Sherlock, G. (2011). Reciprocal sign epistasis between frequently experimentally evolved adaptive mutations causes a rugged fitness landscape. PLoS Genet. 7, e1002056. doi: 10.1371/journal.pgen.1002056
Kwan, E. X., Foss, E., Kruglyak, L., Bedalov, A. (2011). Natural polymorphism in BUL2 links cellular amino acid availability with chronological aging and telomere maintenance in yeast. PLoS Genet. 7, e1002250. doi: 10.1371/journal.pgen.1002250
Lee, S.-I., Dudley, A. M., Drubin, D., Silver, P. A., Krogan, N. J., Pe’er, D., et al. (2009). Learning a prior on regulatory potential from eQTL data. PLoS Genet. 5, e1000358. doi: 10.1371/journal.pgen.1000358
Legras, J.-L., Galeote, V., Bigey, F., Camarasa, C., Marsit, S., Nidelet, T., et al. (2018). Adaptation of S. cerevisiae to fermented food environments reveals remarkable genome plasticity and the footprints of domestication. Mol. Biol. Evol. 35, 1712–1727. doi: 10.1093/molbev/msy066
Li, J., Wang, L., Wu, X., Fang, O., Wang, L., Lu, C., et al. (2013). Polygenic molecular architecture underlying non-sexual cell aggregation in budding yeast. DNA Res. 20, 55–66. doi: 10.1093/dnares/dss033
Linderholm, A., Dietzel, K., Hirst, M., Bisson, L. F. (2010). Identification of MET10-932 and characterization as an allele reducing hydrogen sulfide formation in wine strains of Saccharomyces cerevisiae. Appl. Environ. Microbiol. 76, 7699–7707. doi: 10.1128/AEM.01666-10
Linderholm, A. L., Olineka, T. L., Hong, Y., Bisson, L. F. (2006). Allele diversity among genes of the sulfate reduction pathway in wine strains of Saccharomyces cerevisiae. Am. J. Enol. Vitic. 57, 431–440.
Liti, G., Louis, E. J. (2012). Advances in quantitative trait analysis in yeast. PLoS Genet. 8, e1002912. doi: 10.1371/journal.pgen.1002912
Liti, G., Carter, D. M., Moses, A. M., Warringer, J., Parts, L., James, S. A., et al. (2009). Population genomics of domestic and wild yeasts. Nature 458, 337–341. doi: 10.1038/nature07743
Ma, M., Liu, Z. L. (2010). Mechanisms of ethanol tolerance in Saccharomyces cerevisiae. Appl. Microbiol. Biotechnol. 87, 829–845. doi: 10.1007/s00253-010-2594-3
Malcorps, P., Cheval, J. M., Jamil, S., Dufour, J.-P. (1991). A new model for the regulation of ester synthesis by alcohol acetyltransferases in Saccharomyces cerevisiae. J. Am. Soc. Brew. Chem. 49, 47–53. doi: 10.1094/ASBCJ-49-0047
Manginot, C., Roustan, J., Sablayrolles, J. (1998). Nitrogen demand of different yeast strains during alcoholic fermentation. Importance of the stationary phase. Enzyme Microb. Technol. 23, 511–517. doi: 10.1016/S0141-0229(98)00080-5
Marsit, S., Leducq, J.-B., Durand, É., Marchant, A., Filteau, M., Landry, C. R. (2017). Evolutionary biology through the lens of budding yeast comparative genomics. Nat. Rev. Genet. 18, 581–598. doi: 10.1038/nrg.2017.49
Martí-Raga, M., Sancho, M., Guillamón, J. M., Mas, A., Beltran, G. (2015). The effect of nitrogen addition on the fermentative performance during sparkling wine production. Food Res. Int. 67, 126–135. doi: 10.1016/j.foodres.2014.10.033
Martí-Raga, M., Peltier, E., Mas, A., Beltran, G., Marullo, P. (2017). Genetic causes of phenotypic adaptation to the second fermentation of sparkling wines in Saccharomyces cerevisiae. G3 (Bethesda). g3.116.037283. 7, 399–412. doi: 10.1534/g3.116.037283
Marullo, P., Dubourdieu, D. (2010). “Yeast selection for wine flavour modulation,” in Managing wine quality, vol. 2 . Ed. Reynolds, A. G. (Cambridge: Woodhead Publishing Limited), 296–320. doi: 10.1533/9781845699987.2.293
Marullo, P., Bely, M., Masneuf-Pomarede, I., Aigle, M., Dubourdieu, D. (2004). Inheritable nature of enological quantitative traits is demonstrated by meiotic segregation of industrial wine yeast strains. FEMS Yeast Res. 4, 711–719. doi: 10.1016/j.femsyr.2004.01.006
Marullo, P., Aigle, M., Bely, M., Masneuf-Pomarède, I., Durrens, P., Dubourdieu, D., et al. (2007a). Single QTL mapping and nucleotide-level resolution of a physiologic trait in wine Saccharomyces cerevisiae strains. FEMS Yeast Res. 7, 941–952. doi: 10.1111/j.1567-1364.2007.00252.x
Marullo, P., Yvert, G., Bely, M., Aigle, M., Dubourdieu, D. (2007b). Efficient use of DNA molecular markers to construct industrial yeast strains. FEMS Yeast Res. 7, 1295–1306. doi: 10.1111/j.1567-1364.2007.00281.x
Marullo, P., Thibon, C., Tominaga, T., Dubourdieu, D. (2008). Utilisation de levures mutantes ure2 pour augmenter la libération de thiols volatils aromatiques par la levure pendant la fermentation (Google Patents).
Marullo, P., Mansour, C., Dufour, M., Albertin, W., Sicard, D., Bely, M., et al. (2009). Genetic improvement of thermo-tolerance in wine Saccharomyces cerevisiae strains by a backcross approach. FEMS Yeast Res. 9, 1148–1160. doi: 10.1111/j.1567-1364.2009.00550.x
Marullo, P., Durrens, P., Peltier, E., Bernard, M., Mansour, C., Dubourdieu^, D., et al. (2019). Natural allelic variations of Saccharomyces cerevisiae impact stuck fermentation due to the combined effect of ethanol and temperature; a QTL-mapping study. BioRxiv. doi: 10.1101/576835
Mason, A. B., Dufour, J., Dufour, J. (2000). Alcohol acetyltransferases and the significance of ester synthesis in yeast. Yeast, 16, 1287–1298. doi: 10.1002/1097-0061(200010)16:14<1287::AID-YEA613>3.0.CO;2-I
Maurer, M. J., Sutardja, L., Pinel, D., Bauer, S., Muehlbauer, A. L., Ames, T. D., et al. (2017). Quantitative trait loci (QTL)-guided metabolic engineering of a complex trait. ACS Synth. Biol. 6, 566–581. doi: 10.1021/acssynbio.6b00264
Meijnen, J.-P., Randazzo, P., Foulquié-Moreno, M. R., van den Brink, J., Vandecruys, P., Stojiljkovic, M., et al. (2016). Polygenic analysis and targeted improvement of the complex trait of high acetic acid tolerance in the yeast Saccharomyces cerevisiae. Biotechnol. Biofuels 9, 5. doi: 10.1186/s13068-015-0421-x
Mertens, S., Steensels, J., Gallone, B., Souffriau, B., Malcorps, P., Verstrepen, K. J. (2017). Rapid screening method for phenolic off-flavor (POF) production in yeast. J. Am. Soc. Brew. Chem. 75, 318–323. doi: 10.1094/ASBCJ-2017-4142-01
Mitsumasu, K., Liu, Z.-S., Tang, Y.-Q., Akamatsu, T., Taguchi, H., Kida, K. (2014). Development of industrial yeast strain with improved acid- and thermo-tolerance through evolution under continuous fermentation conditions followed by haploidization and mating. J. Biosci. Bioeng. 118, 689–695. doi: 10.1016/j.jbiosc.2014.05.012
Mizoguchi, H., Yamauchi, T., Watanabe, M., Yamanaka, H., Nishimura, A., Hanamoto, H. (2002). Different missense mutations in PDR1 and PDR3 genes from clotrimazole-resistant sake yeast are responsible for pleiotropic drug resistance and improved fermentative activity. J. Biosci. Bioeng. 93, 221–227. doi: 10.1016/S1389-1723(02)80018-4
Mukai, N., Masaki, K., Fujii, T., Kawamukai, M., Iefuji, H. (2010). PAD1 and FDC1 are essential for the decarboxylation of phenylacrylic acids in Saccharomyces cerevisiae. J. Biosci. Bioeng. 109, 564–569. doi: 10.1016/j.jbiosc.2009.11.011
Ness, F., Aigle, M. (1995). RTM1: a member of a new family of telomeric repeated genes in yeast. Genetics 140, 945–956.
Ng, P. C., Henikoff, S. (2003). SIFT: predicting amino acid changes that affect protein function. Nucleic Acids Res. 31, 3812–3814. doi: 10.1093/nar/gkg509
Noble, J., Sanchez, I., Blondin, B. (2015). Identification of new Saccharomyces cerevisiae variants of the MET2 and SKP2 genes controlling the sulfur assimilation pathway and the production of undesirable sulfur compounds during alcoholic fermentation. Microb. Cell Fact. 14, 68. doi: 10.1186/s12934-015-0245-1
Nogami, S., Ohya, Y., Yvert, G. (2007). Genetic complexity and quantitative trait loci mapping of yeast morphological traits. PLoS Genet. 3, e31. doi: 10.1371/journal.pgen.0030031
Noguchi, C., Watanabe, D., Zhou, Y., Akao, T., Shimoi, H. (2012). Association of constitutive hyperphosphorylation of Hsf1p with a defective ethanol stress response in Saccharomyces cerevisiae sake yeast strains. Appl. Environ. Microbiol. 78, 385–392. doi: 10.1128/AEM.06341-11
Ohnuki, S., Okada, H., Friedrich, A., Kanno, Y., Goshima, T., Hasuda, H., et al. (2017). Phenotypic diagnosis of lineage and differentiation during sake yeast breeding. G3 (Bethesda) 7, 2807–2820. doi: 10.1534/g3.117.044099
Olofsson, K., Bertilsson, M., Lidén, G., Saidi, B., Braun, R., Jönsson, L., et al. (2008). A short review on SSF? An interesting process option for ethanol production from lignocellulosic feedstocks. Biotechnol. Biofuels 1, 7. doi: 10.1186/1754-6834-1-7
Omura, F., Fujita, A., Miyajima, K., Fukui, N. (2005). Engineering of yeast Put4 permease and its application to lager yeast for efficient proline assimilation. Biosci. Biotechnol. Biochem. 69, 1162–1171. doi: 10.1271/bbb.69.1162
Ossa, E. M. D., Pérez, L., Caro, I. (1987). Variations of the major volatiles through aging of sherry. Am. J. Enol. Vitic. 38, 293–297.
Pais, T. M., Foulquié-Moreno, M. R., Hubmann, G., Duitama, J., Swinnen, S., Goovaerts, A., et al. (2013). Comparative polygenic analysis of maximal ethanol accumulation capacity and tolerance to high ethanol levels of cell proliferation in yeast. PLoS Genet. 9, e1003548. doi: 10.1371/journal.pgen.1003548
Palma, M., Guerreiro, J. F., Sá-Correia, I. (2018). Adaptive response and tolerance to acetic acid in Saccharomyces cerevisiae and Zygosaccharomyces bailii: a physiological genomics perspective. Front. Microbiol. 9, 274. doi: 10.3389/fmicb.2018.00274
Palmqvist, E., Hahn-Hägerdal, B. (2000). Fermentation of lignocellulosic hydrolysates. II: inhibitors and mechanisms of inhibition. Bioresour. Technol. 74, 25–33. doi: 10.1016/S0960-8524(99)00161-3
Parts, L., Cubillos, F. A., Warringer, J., Jain, K., Salinas, F., Bumpstead, S. J., et al. (2011). Revealing the genetic structure of a trait by sequencing a population under selection. Genome Res. 21, 1131–1138. doi: 10.1101/gr.116731.110
Peltier, E., Sharma, V., Raga, M. M., Roncoroni, M., Bernard, M., Gibon, Yves, et al. (2018). Dissection of the molecular bases of genotype x environment interactions: a study of phenotypic plasticity of Saccharomyces cerevisiae in grape juices. BMC Genomics 19, 772. doi: 10.1186/s12864-018-5145-4
Pérez-Ortín, J. E., Querol, A., Puig, S., Barrio, E. (2002). Molecular characterization of a chromosomal rearrangement involved in the adaptive evolution of yeast strains. Genome Res. 12, 1533–1539. doi: 10.1101/gr.436602
Peter, J., Schacherer, J. (2016). Population genomics of yeasts: towards a comprehensive view across a broad evolutionary scale. Yeast 33, 73–81. doi: 10.1002/yea.3142
Peter, J., De Chiara, M., Friedrich, A., Yue, J.-X., Pflieger, D., Bergström, A., et al. (2018). Genome evolution across 1,011 Saccharomyces cerevisiae isolates. Nature 556, 339–344. doi: 10.1038/s41586-018-0030-5
Pigliucci, M., Kolodynska, A. (2002). Phenotypic plasticity to light intensity in Arabidopsis thaliana: invariance of reaction norms and phenotypic integration. Evol. Ecol. 16, 27–47. doi: 10.1023/A:1016073525567
Pinel, D., Colatriano, D., Jiang, H., Lee, H., Martin, V. J. J. (2015). Deconstructing the genetic basis of spent sulphite liquor tolerance using deep sequencing of genome-shuffled yeast. Biotechnol. Biofuels 8, 53. doi: 10.1186/s13068-015-0241-z
Pretorius, I. S. (2000). Tailoring wine yeast for the new millennium: novel approaches to the ancient art of winemaking. Yeast 16, 675–729. doi: 10.1002/1097-0061(20000615)16:8<675::AID-YEA585>3.0.CO;2-B
Pronk, J. T., Steensma, H. Y., van Dijken, J. P. (1996). Pyruvate metabolism in Saccharomyces cerevisiae.Yeast 12, 1607–1633. doi: 10.1002/(SICI)1097-0061(199612)12:16<1607::AID-YEA70>3.0.CO;2-4
Quirós, M., Rojas, V., Gonzalez, R., Morales, P. (2014). Selection of non-Saccharomyces yeast strains for reducing alcohol levels in wine by sugar respiration. Int. J. Food Microbiol. 181, 85–91. doi: 10.1016/j.ijfoodmicro.2014.04.024
Reiser, V., Salah, S. M., Ammerer, G. (2000). Polarized localization of yeast Pbs2 depends on osmostress, the membrane protein Sho1 and Cdc42. Nat. Cell Biol. 2, 620–627. doi: 10.1038/35023568
Remize, F., Andrieu, E., Dequin, S. (2000). Engineering of the pyruvate dehydrogenase bypass in Saccharomyces cerevisiae: role of the cytosolic Mg2+ and mitochondrial K+ acetaldehyde dehydrogenases Ald6p and Ald4p in acetate formation during alcoholic fermentation. Appl. Environ. Microbiol. 66, 3151–3159. doi: 10.1128/AEM.66.8.3151-3159.2000
Ribéreau-Gayon, P., Glories, Y., Maujean, A., Dubourdieu, D. (2006). “Alcohols and other volatile compounds,” in Handbook of enology (New York, USA: John Wiley & Sons, Ltd), 51–64. doi: 10.1002/0470010398.ch2
Romano, G. H., Gurvich, Y., Lavi, O., Ulitsky, I., Shamir, R., Kupiec, M. (2010). Different sets of QTLs influence fitness variation in yeast. Mol. Syst. Biol. 6, 346. doi: 10.1038/msb.2010.1
Roncoroni, M., Santiago, M., Hooks, D. O., Moroney, S., Harsch, M. J., Lee, S., et al. (2011). The yeast IRC7 gene encodes a b-lyase responsible for production of the varietal thiol 4-mercapto-4-methylpentan-2-one in wine. Food Microbiol. 28, 926–935. doi: 10.1016/j.fm.2011.01.002
Sachidanandam, R., Weissman, D., Schmidt, S. C., Kakol, J. M., Stein, L. D., Marth, G., et al. (2001). A map of human genome sequence variation containing 1.42 million single nucleotide polymorphisms. Nature 409, 928–933. doi: 10.1038/35057149
Saerens, S. M. G., Verstrepen, K. J., Van Laere, S. D. M., Voet, A. R. D., Van Dijck, P., Delvaux, F. R., et al. (2006). The Saccharomyces cerevisiae EHT1 and EEB1 genes encode novel enzymes with medium-chain fatty acid ethyl ester synthesis and hydrolysis capacity. J. Biol. Chem. 281, 4446–4456. doi: 10.1074/jbc.M512028200
Saint-Prix, F., Bönquist, L., Dequin, S. (2004). Functional analysis of the ALD gene family of Saccharomyces cerevisiae during anaerobic growth on glucose: the NADP+-dependent Ald6p and Ald5p isoforms play a major role in acetate formation. Microbiology 150, 2209–2220. doi: 10.1099/mic.0.26999-0
Salinas, F., Cubillos, F. A., Soto, D., Garcia, V., Bergström, A., Warringer, J., et al. (2012). The genetic basis of natural variation in oenological traits in Saccharomyces cerevisiae. PloS One 7, e49640. doi: 10.1371/journal.pone.0049640
Salinas, F., de Boer, C. G., Abarca, V., García, V., Cuevas, M., Araos, S., et al. (2016). Natural variation in non-coding regions underlying phenotypic diversity in budding yeast. Sci. Rep. 6, 21849. doi: 10.1038/srep21849
Sardi, M., Paithane, V., Place, M., Robinson, D. E., Hose, J., Wohlbach, D. J., et al. (2018). Genome-wide association across Saccharomyces cerevisiae strains reveals substantial variation in underlying gene requirements for toxin tolerance. PLoS Genet. 14, e1007217. doi: 10.1371/journal.pgen.1007217
Sasano, Y., Haitani, Y., Hashida, K., Ohtsu, I., Shima, J., Takagi, H. (2012). Enhancement of the proline and nitric oxide synthetic pathway improves fermentation ability under multiple baking-associated stress conditions in industrial baker’s yeast. Microb. Cell Fact. 11, 40. doi: 10.1186/1475-2859-11-40
Schacherer, J., Shapiro, J., Ruderfer, D. M., Kruglyak, L. (2009). Comprehensive polymorphism survey elucidates population structure of Saccharomyces cerevisiae. Nature 458, 342–345. doi: 10.1038/nature07670
Scozzari, R., Massaia, A., Trombetta, B., Bellusci, G., Myres, N. M., Novelletto, A., et al. (2014). An unbiased resource of novel SNP markers provides a new chronology for the human Y chromosome and reveals a deep phylogenetic structure in Africa. Genome Res. 24, 535–544. doi: 10.1101/gr.160788.113
Segrè, A. V., Murray, A. W., Leu, J.-Y. (2006). High-resolution mutation mapping reveals parallel experimental evolution in yeast. PLoS Biol. 4, e256. doi: 10.1371/journal.pbio.0040256
Sekine, T., Kawaguchi, A., Hamano, Y., Takagi, H. (2007). Desensitization of feedback inhibition of the Saccharomyces cerevisiaeγ-glutamyl kinase enhances proline accumulation and freezing tolerance. Appl. Environ. Microbiol. 73, 4011–4019. doi: 10.1128/AEM.00730-07
Shahsavarani, H., Sugiyama, M., Kaneko, Y., Chuenchit, B., Harashima, S. (2012). Superior thermotolerance of Saccharomyces cerevisiae for efficient bioethanol fermentation can be achieved by overexpression of RSP5 ubiquitin ligase. Biotechnol. Adv. 30, 1289–1300. doi: 10.1016/j.biotechadv.2011.09.002
Sharma, A., Lee, J. S., Dang, C. G., Sudrajad, P., Kim, H. C., Yeon, S. H., et al. (2015). Stories and challenges of genome wide association studies in livestock—a review. Asian-Australas. J. Anim. Sci. 28, 1371–1379. doi: 10.5713/ajas.14.0715
Shobayashi, M., Ukena, E., Fujii, T., IIefuji, H. (2007). Genome-wide expression profile of sake brewing yeast under shaking and static conditions. Biosci. Biotechnol. Biochem. 71, 323–335. doi: 10.1271/bbb.60190
Sicard, D., Legras, J. L. (2011). Bread, beer and wine: yeast domestication in the Saccharomyces sensu stricto complex. C. R. Biol. 334, 229–236. doi: 10.1016/j.crvi.2010.12.016
Simpson-Lavy, K., Xu, T., Johnston, M., Kupiec, M. (2017). The Std1 activator of the Snf1/AMPK kinase controls glucose response in yeast by a regulated protein aggregation. Mol. Cell 68, 1120–1133.e3. doi: 10.1016/j.molcel.2017.11.016
Sinha, H., Nicholson, B. P., Steinmetz, L. M., McCusker, J. H. (2006). Complex genetic interactions in a quantitative trait locus. PLoS Genet. 2, 140–147. doi: 10.1371/journal.pgen.0020013
Sinha, H., David, L., Pascon, R. C., Clauder-Münster, S., Krishnakumar, S., Nguyen, M., et al. (2008). Sequential elimination of major-effect contributors identifies additional quantitative trait loci conditioning high-temperature growth in yeast. Genetics 180, 1661–1670. doi: 10.1534/genetics.108.092932
Sirr, A., Cromie, G. A., Jeffery, E. W., Gilbert, T. L., Ludlow, C. L., Scott, A. C., et al. (2015). Allelic variation, aneuploidy, and nongenetic mechanisms suppress a monogenic trait in yeast. Genetics 199, 247–262. doi: 10.1534/genetics.114.170563
Smith, E. N., Kruglyak, L. (2008). Gene–environment interaction in yeast gene expression. PLoS Biol. 6, e83. doi: 10.1371/journal.pbio.0060083
Snoek, T., Verstrepen, K. J., Voordeckers, K. (2016). Curr. Genet 62, 475-480. doi: 10.1007/s00294-015-0561-3
Stanley, D., Fraser, S., Chambers, P. J., Rogers, P., Stanley, G. A. (2010). Generation and characterisation of stable ethanol-tolerant mutants of Saccharomyces cerevisiae. J. Ind. Microbiol. Biotechnol. 37, 139–149. doi: 10.1007/s10295-009-0655-3
Steensels, J., Snoek, T., Meersman, E., Nicolino, M. P., Voordeckers, K., Verstrepen, K. J. (2014). Improving industrial yeast strains: exploiting natural and artificial diversity. FEMS Microbiol. Rev., 38, 1–49. doi: 10.1111/1574-6976.12073
Steinmetz, L. M., Sinha, H., Richards, D. R., Spiegelman, J. I., Oefner, P. J., McCusker, J. H., et al. (2002). Dissecting the architecture of a quantitative trait locus in yeast. Nature 416, 326–330. doi: 10.1038/416326a
Stewart, G. G. (2006). Studies on the uptake and metabolism of wort sugars during brewing fermentations. MBAA Tech. Quart 43, 265–269. doi: 10.1094/TQ-43-0265
Steyer, D., Ambroset, C., Brion, C., Claudel, P., Delobel, P., Sanchez, I., et al. (2012). QTL mapping of the production of wine aroma compounds by yeast. BMC Genomics 13, 573. doi: 10.1186/1471-2164-13-573
Steyer, D., Erny, C., Claudel, P., Riveill, G., Karst, F., Legras, J.-L. (2013). Genetic analysis of geraniol metabolism during fermentation. Food Microbiol. 33, 228–234. doi: 10.1016/j.fm.2012.09.021
Storici, F., Resnick, M. A. (2006). The delitto perfetto approach to in vivo site-directed mutagenesis and chromosome rearrangements with synthetic oligonucleotides in yeast. Meth. Enzymol. 409, 329–345. doi: 10.1016/S0076-6879(05)09019-1
Stucki, D., Malla, B., Hostettler, S., Huna, T., Feldmann, J., Yeboah-Manu, D., et al. (2012). Two new rapid SNP-typing methods for classifying Mycobacterium tuberculosis complex into the main phylogenetic lineages. PLoS One 7, e41253. doi: 10.1371/journal.pone.0041253
Sumby, K. M., Grbin, P. R., Jiranek, V. (2010). Microbial modulation of aromatic esters in wine: current knowledge and future prospects. Food Chem. 121, 1–16. doi: 10.1016/j.foodchem.2009.12.004
Swiegers, J. H., Pretorius, I. S. (2007). Modulation of volatile sulfur compounds by wine yeast. Appl. Microbiol. Biotechnol. 74, 954–960. doi: 10.1007/s00253-006-0828-1
Swinnen, S., Schaerlaekens, K., Pais, T., Claesen, J., Hubmann, G., Yang, Y., et al. (2012a). Identification of novel causative genes determining the complex trait of high ethanol tolerance in yeast using pooled-segregant whole-genome sequence analysis. Genome Res. 22, 975–984. doi: 10.1101/gr.131698.111
Swinnen, S., Thevelein, J. M., Nevoigt, E. (2012b). Genetic mapping of quantitative phenotypic traits in Saccharomyces cerevisiae. FEMS Yeast Res. 12, 215–227. doi: 10.1111/j.1567-1364.2011.00777.x
Swinnen, S., Klein, M., Carrillo, M., McInnes, J., Nguyen, H. T. T., Nevoigt, E. (2013). Re-evaluation of glycerol utilization in Saccharomyces cerevisiae: characterization of an isolate that grows on glycerol without supporting supplements. Biotechnol. Biofuels 6, 157. doi: 10.1186/1754-6834-6-157
Swinnen, S., Henriques, S. F., Shrestha, R., Ho, P.-W., Sá-Correia, I., Nevoigt, E. (2017). Improvement of yeast tolerance to acetic acid through Haa1 transcription factor engineering: towards the underlying mechanisms. Microb. Cell Fact. 16, 7. doi: 10.1186/s12934-016-0621-5
Taherzadeh, M., Gustafsson, L., Niklasson, C. (2000). Physiological effects of 5-hydroxymethylfurfural on Saccharomyces cerevisiae. Appl. Microbiol. Biotechnol. 53 (6), 701–708. doi: 10.1007/s002530000328
Takagi, H. (2008). Proline as a stress protectant in yeast: physiological functions, metabolic regulations, and biotechnological applications. Appl. Microbiol. Biotechnol. 81, 211–223. doi: 10.1007/s00253-008-1698-5
Takagi, H., Iwamoto, F., Nakamori, S. (1997). Isolation of freeze-tolerant laboratory strains of Saccharomyces cerevisiae from proline-analogue-resistant mutants. Appl. Microbiol. Biotechnol. 47, 405–411. doi: 10.1007/s002530050948
Tamás, M. J., Hohmann, S. (2003). “The osmotic stress response of Saccharomyces cerevisiae,” in Yeast stress responses. Eds. Hohmann, S., Mager, W. H. (Berlin, Heidelberg: Springer Berlin Heidelberg), 121–200. doi: 10.1007/3-540-45611-2_4
Tanghe, A., Dijck, P. V., Dumortier, F., Teunissen, A., Hohmann, S., Thevelein, J. M. (2002). Aquaporin expression correlates with freeze tolerance in baker’s yeast, and overexpression improves freeze tolerance in industrial strains. Appl. Environ. Microbiol 68, 5981–5989. doi: 10.1128/AEM.68.12.5981-5989.2002
Tapia, S. M., Cuevas, M., Abarca, V., Delgado, V., Rojas, V., García, V., et al. (2018). GPD1 and ADH3 natural variants underlie glycerol yield differences in wine fermentation. Front. Microbiol 91460. doi: 10.3389/fmicb.2018.01460
Taylor, M. B., Ehrenreich, I. M. (2014). Genetic interactions involving five or more genes contribute to a complex trait in yeast. PLoS Genet. 10, e1004324. doi: 10.1371/journal.pgen.1004324
Teige, M., Scheikl, E., Reiser, V., Ruis, H., Ammerer, G. (2001). Rck2, a member of the calmodulin-protein kinase family, links protein synthesis to high osmolarity MAP kinase signaling in budding yeast. Proc. Natl. Acad. Sci. U.S.A 98, 5625–5630. doi: 10.1073/pnas.091610798
Thevelein, J. M., Gorwa-Grauslund, M. F., Dumortier, F., Van Dijck, P., Teunissen, A., Lemaire, K., et al., (1999). Yeast strains with fil phenotype, stress resistant under conditions of growth and/or fermentation.
Thibon, C., Marullo, P., Claisse, O., Cullin, C., Dubourdieu, D., Tominaga, T. (2008). Nitrogen catabolic repression controls the release of volatile thiols by Saccharomyces cerevisiae during wine fermentation. FEMS Yeast Res. 8, 1076–1086. doi: 10.1111/j.1567-1364.2008.00381.x
Timberlake, W. E., Frizzell, M. A., Richards, K. D., Gardner, R. C. (2011). A new yeast genetic resource for analysis and breeding. Yeast 28, 63–80. doi: 10.1002/yea.1821
Tominaga, T., Dubourdieu, D. (2000). Identification of cysteinylated aroma precursors of certain volatile thiols in passion fruit juice. J. Agric. Food Chem 48, 2874–2876. doi: 10.1021/jf990980a
Trindade de Carvalho, B., Holt, S., Souffriau, B., Lopes Brandão, R., Foulquié-Moreno, M. R., Thevelein, J. M. (2017). Identification of novel alleles conferring superior production of rose flavor phenylethyl acetate using polygenic analysis in yeast. MBio 8, e01173–17. doi: 10.1128/mBio.01173-17
Veerkamp, R. F., Simm, G., Oldham, J. D. (1994). Effects of interaction between genotype and feeding system on milk production, feed intake, efficiency and body tissue mobilization in dairy cows. Livest. Prod. Sci 39, 229–241. doi: 10.1016/0301-6226(94)90202-X
Verstrepen, K. J., Laere, S. D. M., Vanderhaegen, B. M. P., Derdelinckx, G., Dufour, J., Pretorius, I. S., et al. (2003a). Expression levels of the yeast alcohol acetyltransferase genes. Society 69, 5228–5237. doi: 10.1128/AEM.69.9.5228-5237.2003
Verstrepen, K. J., Derdelinckx, G., Dufour, J.-P., Winderickx, J., Thevelein, J. M., Pretorius, I. S., et al. (2003b). Flavor-active esters: adding fruitiness to beer. J. Biosci. Bioeng. 96, 110–118. doi: 10.1263/jbb.96.110
Verstrepen, K. J., Jansen, A., Lewitter, F., Fink, G. R. (2005). Intragenic tandem repeats generate functional variability. Nat. Genet. 37, 986–990. doi: 10.1038/ng1618
Vidgren, V., Londesborough, J. (2011). 125th anniversary review: yeast flocculation and sedimentation in brewing. J. Inst. Brew. 117, 475–487. doi: 10.1002/j.2050-0416.2011.tb00495.x
Vidgren, V., Huuskonen, A., Virtanen, H., Ruohonen, L., Londesborough, J. (2009). Improved fermentation performance of a lager yeast after repair of its AGT1 maltose and maltotriose transporter genes. Appl. Environ. Microbiol 75, 2333–2345. doi: 10.1128/AEM.01558-08
Vilela-Moura, A., Schuller, D., Mendes-Faia, A., Côrte-Real, M. (2010). Effects of acetic acid, ethanol, and SO2 on the removal of volatile acidity from acidic wines by two Saccharomyces cerevisiae commercial strains. Appl. Microbiol. Biotechnol. 87, 1317–1326. doi: 10.1007/s00253-010-2558-7
Voordeckers, K., Kominek, J., Das, A., Espinosa-Cantú, A., De Maeyer, D., Arslan, A., et al. (2015). Adaptation to high ethanol reveals complex evolutionary pathways. PLoS Genet. 11, e1005635. doi: 10.1371/journal.pgen.1005635
Watanabe, D., Wu, H., Noguchi, C., Zhou, Y., Akao, T., Shimoi, H. (2011). Enhancement of the initial rate of ethanol fermentation due to dysfunction of yeast stress response components Msn2p and/or Msn4p. Appl. Environ. Microbiol 77, 934–941. doi: 10.1128/AEM.01869-10
Watanabe, D., Araki, Y., Zhou, Y., Maeya, N., Akao, T., Shimoi, H. (2012). A loss-of-function mutation in the PAS kinase Rim15p is related to defective quiescence entry and high fermentation rates of Saccharomyces cerevisiae sake yeast strains. Appl. Environ. Microbiol 78, 4008–4016. doi: 10.1128/AEM.00165-12
Watanabe, D., Hashimoto, N., Mizuno, M., Zhou, Y., Akao, T., Shimoi, H., (2013). Accelerated alcoholic fermentation caused by defective gene expression related to glucose derepression in Saccharomyces cerevisiae. Biosci. Biotechnol. Biochem. 77, 2255–2262. doi: 10.1271/bbb.130519
Wei, X., Zhang, J. (2017). The genomic architecture of interactions between natural genetic polymorphisms and environments in yeast growth. Genetics 205, 925–937. doi: 10.1534/genetics.116.195487
Wilkening, S., Lin, G., Fritsch, E. S., Tekkedil, M. M., Anders, S., Kuehn, R., et al. (2014). An evaluation of high-throughput approaches to QTL mapping in Saccharomyces cerevisiae. Genetics 196, 853–865. doi: 10.1534/genetics.113.160291
Will, J. L., Kim, H. S., Clarke, J., Painter, J. C., Fay, J. C., Gasch, A. P. (2010). Incipient balancing selection through adaptive loss of aquaporins in natural Saccharomyces cerevisiae population. PLoS Genet 6, e1000893. doi: 10.1371/journal.pgen.1000893
Wu, H., Zheng, X., Araki, Y., Sahara, H., Takagi, H., Shimoi, H. (2006). Global gene expression analysis of yeast cells during sake brewing. Appl. Environ. Microbiol 72, 7353–7358. doi: 10.1128/AEM.01097-06
Xu, Y., Crouch, J. H. (2008). Marker-assisted selection in plant breeding: from publications to practice. Crop Sci 48, 391–407. doi: 10.2135/cropsci2007.04.0191
Yadav, A., Sinha, H. (2018). Gene–gene and gene–environment interactions in complex traits in yeast. Yeast 35, 403–416. doi: 10.1002/yea.3304
Yadav, A., Radhakrishnan, A., Bhanot, G., Sinha, H. (2015). Differential regulation of antagonistic pleiotropy in synthetic and natural populations suggests its role in adaptation. G3 (Bethesda) 5, 699–709. doi: 10.1534/g3.115.017020
Yadav, A., Dhole, K., Sinha, H. (2016). Genetic regulation of phenotypic plasticity and canalisation in yeast growth. PLoS One 11, e0162326. doi: 10.1371/journal.pone.0162326
Yang, J., Bae, J. Y., Lee, Y. M., Kwon, H., Moon, H.-Y., Kang, H. A., et al. (2011). Construction of Saccharomyces cerevisiae strains with enhanced ethanol tolerance by mutagenesis of the TATA-binding protein gene and identification of novel genes associated with ethanol tolerance. Biotechnol. Bioeng. 108, 1776–1787. doi: 10.1002/bit.23141
Yang, Y., Foulquié-Moreno, M. R., Clement, L., Erdei, E., Tanghe, A., Schaerlaekens, K., et al. (2013). QTL analysis of high thermotolerance with superior and downgraded parental yeast strains reveals new minor QTLs and converges on novel causative alleles involved in RNA processing. PLoS Genet. 9, e1003693. doi: 10.1371/journal.pgen.1003693
Yoshida, S., Imoto, J., Minato, T., Oouchi, R., Kamada, Y., Tomita, M., et al. (2010). A novel mechanism regulates H2S and SO2 production in Saccharomyces cerevisiae. Yeast 28, 109–121. doi: 10.1002/yea.1823
Youens-Clark, K., Buckler, E., Casstevens, T., Chen, C., DeClerck, G., Derwent, P., et al. (2011). Gramene database in 2010: updates and extensions. Nucleic Acids Res. 39, D1085–D1094. doi: 10.1093/nar/gkq1148
Yvert, G., Brem, R. B., Whittle, J., Akey, J. M., Foss, E., Smith, E. N., et al. (2003). Trans-acting regulatory variation in Saccharomyces cerevisiae and the role of transcription factors. Nat. Genet. 35, 57–64. doi: 10.1038/ng1222
Zampar, G. G., Kümmel, A., Ewald, J., Jol, S., Niebel, B., Picotti, P., et al. (2013). Temporal system-level organization of the switch from glycolytic to gluconeogenic operation in yeast. Mol. Syst. Biol. 9, 651. doi: 10.1038/msb.2013.11
Zhao, X. Q., Bai, F. W. (2009). Mechanisms of yeast stress tolerance and its manipulation for efficient fuel ethanol production. J. Biotechnol. 144, 23–30. doi: 10.1016/j.jbiotec.2009.05.001
Zimmer, A., Durand, C., Loira, N., Durrens, P., Sherman, D. J., Marullo, P. (2014). QTL dissection of lag phase in wine fermentation reveals a new translocation responsible for Saccharomyces cerevisiae adaptation to sulfite. PLoS One 9, 37–39. doi: 10.1371/journal.pone.0086298
Zimmermann, F. K., Kaufmann, I., Rasenberger, H., Haußmann, P. (1977). Genetics of carbon catabolite repression in Saccharomyces cerevisiae: genes involved in the derepression process. Mol. Gen. Genet. 151, 95–103. doi: 10.1007/BF00446918
Keywords: biotechnology, fermentation, QTL, QTN, QTG, yeast, variant, aroma
Citation: Peltier E, Friedrich A, Schacherer J and Marullo P (2019) Quantitative Trait Nucleotides Impacting the Technological Performances of Industrial Saccharomyces cerevisiae Strains. Front. Genet. 10:683. doi: 10.3389/fgene.2019.00683
Received: 15 April 2019; Accepted: 01 July 2019;
Published: 23 July 2019.
Edited by:
Isabel Sá-Correia, University of Lisbon, PortugalReviewed by:
Johan M. Thevelein, KU Leuven, BelgiumHimanshu Sinha, Indian Institute of Technology Madras, India
Copyright © 2019 Peltier, Friedrich, Schacherer and Marullo. This is an open-access article distributed under the terms of the Creative Commons Attribution License (CC BY). The use, distribution or reproduction in other forums is permitted, provided the original author(s) and the copyright owner(s) are credited and that the original publication in this journal is cited, in accordance with accepted academic practice. No use, distribution or reproduction is permitted which does not comply with these terms.
*Correspondence: Philippe Marullo, cGhpbGlwcGUubWFydWxsb0B1LWJvcmRlYXV4LmZy