- 1Department of Genetics, Stellenbosch University, Stellenbosch, South Africa
- 2Department of Psychiatry, Stellenbosch University, Tygerberg, South Africa
Despite advances in pharmacogenetics, the majority of heritability for treatment response cannot be explained by common variation, suggesting that factors such as epigenetics may play a key role. Regulatory genes, such as those involved in DNA methylation and transcriptional repression, are therefore excellent candidates for investigating antipsychotic treatment response. This study explored the differential expression of regulatory genes between patients with schizophrenia (chronic and antipsychotic-naïve first-episode patients) and healthy controls in order to identify candidate genes for association with antipsychotic treatment response. Seven candidate differentially expressed genes were identified, and four variants within these genes were found to be significantly associated with treatment response (DNMT3A rs2304429, HDAC5 rs11079983, and HDAC9 rs1178119 and rs11764843). Further analyses revealed that two of these variants (rs2304429 and rs11079983) are predicted to alter the expression of specific genes (DNMT3A, ASB16, and ASB16-AS1) in brain regions previously implicated in schizophrenia and treatment response. These results may aid in the development of biomarkers for antipsychotic treatment response, as well as novel drug targets.
Introduction
The onset of schizophrenia is marked by a first psychotic episode, typically followed by subsequent relapse episodes, separated by intervals of remission (Lieberman et al., 2001). Diagnosis remains difficult due to a heterogeneity of symptoms as well as symptom overlap with other disorders (Tandon et al., 2009). Furthermore, treatment strategies are not optimal (Brandl et al., 2014), and it is estimated that approximately half of all patients with schizophrenia will not respond satisfactorily to antipsychotics (Lohoff and Ferraro, 2010), which are the mainstay of treatment and, as such, widely used. They are effective for positive symptoms (such as delusions and hallucinations); however, their efficacy for negative symptoms (such as apathy, anhedonia, and social withdrawal) is limited (Leucht and Davis, 2017). Moreover, antipsychotics are known to result in a number of adverse drug reactions (ADRs), including motor abnormalities (Chowdhury et al., 2011) and metabolic deficits (Tandon et al., 2010). These ADRs are often severe and long lasting, resulting in reduced compliance and diminished positive outcomes (Brandl et al., 2014). Considering the high rate of non-responders to treatment and the potential severe side effects of treatment, there is a clear need to improve our understanding of antipsychotic treatment response.
Pharmacogenetics, the study of the effects of genetic variation on treatment outcomes, has been moderately successful in explaining variability in inter-individual treatment response. Variation within the dopaminergic pathway has been extensively investigated, and several variants within dopamine receptor genes are associated with treatment response (Lencz et al., 2010) and ADRs (Bakker et al., 2008). In addition, variation within genes encoding drug-metabolizing enzymes has yielded similar findings of association (Lohoff and Ferraro, 2010; Brandl et al., 2014). Larger hypothesis-free-driven genome-wide association studies (GWAS) have also associated common variation with antipsychotic treatment response (Liou et al., 2012; Zhang and Malhotra, 2013); however, there has been little validation or replication of these associations, and their biological relevance remains to be determined (Liou et al., 2012; Zhang and Malhotra, 2013). In addition to these challenges, the majority of treatment response heritability is not explained by common variation, suggesting that other factors must also play a role (Manolio et al., 2009). This underscores the complexity and multi-factorial nature of treatment response since common and rare genetic factors, environmental factors, and gene-environment interactions need to be considered (Manolio et al., 2009; Majchrzak-Celińska and Baer-Dubowska, 2017).
Epigenetics refers to molecular mechanisms that determine inherited cellular phenotypes without alteration of the genotype (Wu et al., 2012). These mechanisms include various molecular processes, such as histone modification, nucleosome remodeling, non-coding RNAs, and DNA methylation (Wu et al., 2012). Both unique and overlapping altered epigenetic modifications are associated with schizophrenia etiology and pathogenesis as well as antipsychotic treatment (Swathy and Banerjee, 2017). As such, an extremely complex, multi-directional relationship needs to be considered in studying the role of epigenetics in treatment response (pharmacoepigenetics), since genes that are implicated may be regulated by epigenetic modification independent of disease etiology, treatment outcomes, and/or ADRs (Kurita et al., 2012; Melas et al., 2012; Tang et al., 2014; Majchrzak-Celińska and Baer-Dubowska, 2017). For example, alterations of DNA methylation profiles (Melas et al., 2012; Tang et al., 2014) and altered chromatin structure (Kurita et al., 2012) may influence treatment response.
Although specific gene–environment interactions are required for epigenetic modifications to occur, it is important to note that specific genes directly or indirectly produce proteins and other products necessary for these modifications. For example, the DNMT1 gene encodes for Dnmt1, which is responsible for the maintenance of existing methylation patterns during cell division (Bostick et al., 2007), while DNMT3A and DNMT3B encode for the enzymes responsible for establishing de novo methylation patterns (Okano et al., 1999). Regulatory genes, such as those involved in DNA methylation and transcriptional repression, are therefore excellent candidates for investigation with regards to antipsychotic treatment response.
The aims of this study were, therefore, to identify candidate regulatory genes that may be involved in antipsychotic treatment response, and then to determine if variation within these genes is associated with treatment outcome. In order to address these aims, we first sought to identify regulatory genes that were differentially expressed between patients with schizophrenia (including chronically medicated patients and drug-naïve first-episode patients) and healthy controls. By identifying genes differentially expressed between the chronically medicated patients and both the drug-naïve first-episode patients and healthy controls, we identified a subset of candidate genes that may be implicated in treatment response independent of schizophrenia pathogenesis. Variation within these candidate genes was then investigated in an independent cohort for association with treatment response over time.
Materials and Methods
An outline of the approach used to perform this study is provided in Figure 1.
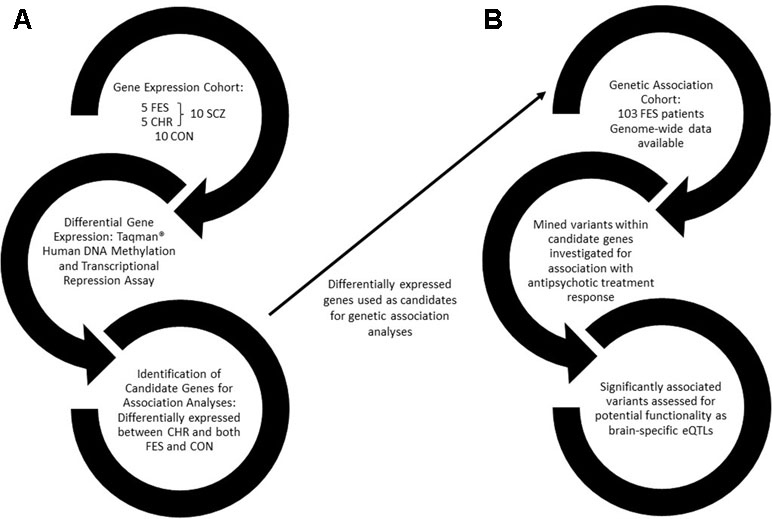
Figure 1 A flow diagram outlining the approach used in this study. (A) The first arm of the study involves identifying differentially expressed genes between healthy controls and schizophrenia patients, further divided into drug-naïve first-episode patients and chronic patients. (B) Differentially expressed genes were then used as candidates for genetic association with antipsychotic treatment response in a drug-naïve FES cohort. FES, first episode schizophrenia; CHR, chronic schizophrenia; SCZ, schizophrenia; CON, healthy control; eQTLs, expression quantitative trait loci.
Participants for Gene Expression Analyses
The cohort consisted of 20 unrelated, age-matched, and male South African participants of South African colored (SAC) descent [10 patients with schizophrenia (SCZ), 26.4 ± 7.9 years old, and 10 healthy controls (CON), 26.5 ± 7.6 years old]. Patients with SCZ were further divided into two equal groups consisting of five first-episode patients (FES) (24.8 ± 10.9 years old) and five chronic patients (CHR) (28.0 ± 4.1 years old). The FES patients were recruited and sampled within 1 week of their first episode of psychosis and were subsequently administered flupenthixol decanoate (Fluanxol, Lundbeck, Copenhagen, Denmark). The CHR patients were recruited 6.2 ± 0.4 years after their first episode, and all were treated with flupenthixol decanoate (Fluanxol, Lundbeck, Copenhagen, Denmark) in addition to other psychotropic medications (Supplementary Table 1). All patients were diagnosed using the Diagnostic and Statistical Manual of Mental Diseases, Fourth Edition, Text Revision (DSM-IV TR) (American Psychiatric Association, 2000) diagnostic criteria for SCZ, schizophreniform disorder, or schizo-affective disorder. Written informed consent was obtained from all patients, or their caregivers, prior to the study, and ethical approval was granted by the Human Research and Ethics Committee (HREC), Faculty of Medicine and Health Sciences, Stellenbosch University (N13/08/115).
Total RNA Isolation and cDNA Synthesis
Whole blood was collected from all participants by venipuncture of a forearm vein into PAXgene Blood RNA tubes (Qiagen, California, USA), which were stored at −20°C until processed. Total RNA was extracted using the PAXgene Blood RNA Kit IVD according to the manufacturer’s instructions (Qiagen, California, USA). All samples were eluted in 80 µl of elution buffer and stored at −80°C until further analysis. RNA yield and quality were assessed using an Agilent Model 2100 Bioanalyzer (Agilent Technologies, California, USA) and a DropSense 16 spectrophotometer (TRINEAN, Belgium). All samples had 260/280 > 2.0 and RIN > 7.0.
Reverse transcription was performed using the High-Capacity cDNA Reverse Transcription Kit with RNase Inhibitor (Applied Biosystems, California, USA), according to the manufacturer’s specifications. Briefly, for each sample, 100 ng of RNA was added to 2 μl of random and oligo (dT) primers in a final reaction of 20 μl. These reaction tubes were then placed in the GeneAmp® PCR Systems 2700 (Applied Biosystems, California, USA) thermocycler at 25°C for 10 min, 37°C for 120 min, and 85°C for 5 min to inactivate the reverse transcriptase enzyme. The cDNA samples were then stored at −20°C until analyzed.
Quantitative Real-Time PCR
Quantitative real-time PCR (qRT-PCR), to determine the relative mRNA abundance, was performed using the StepOnePlus Real-Time PCR System and SDS Software version 2.3 (Applied Biosystems, California, USA). The commercially available fluorescence-based TaqMan® Human DNA Methylation and Transcriptional Repression microarray plates (Applied Biosystems, California, USA) were used to assess the relative mRNA content of 27 regulatory genes (Table 1). Each sample was analyzed in two independent experiments to control for experimental bias using the following PCR conditions: a 10-min heat activation step (95°C) followed by 40 cycles of 15 s at 94°C and 1 min at 63°C. Fluorescence data, indicative of the amount of PCR product, was captured at each cycle. The relative mRNA concentrations were then calculated based on the cycle number that the threshold quantity of PCR product is obtained (Ct). The RefFinder online tool was used to assess the stability of potential housekeeping genes (https://www.heartcure.com.au/reffinder) (Xie et al., 2012), and GAPDH was identified as the most stable. Ct values were therefore normalized to values of GAPDH and expressed relative to this control (Livak and Schmittgen, 2001).
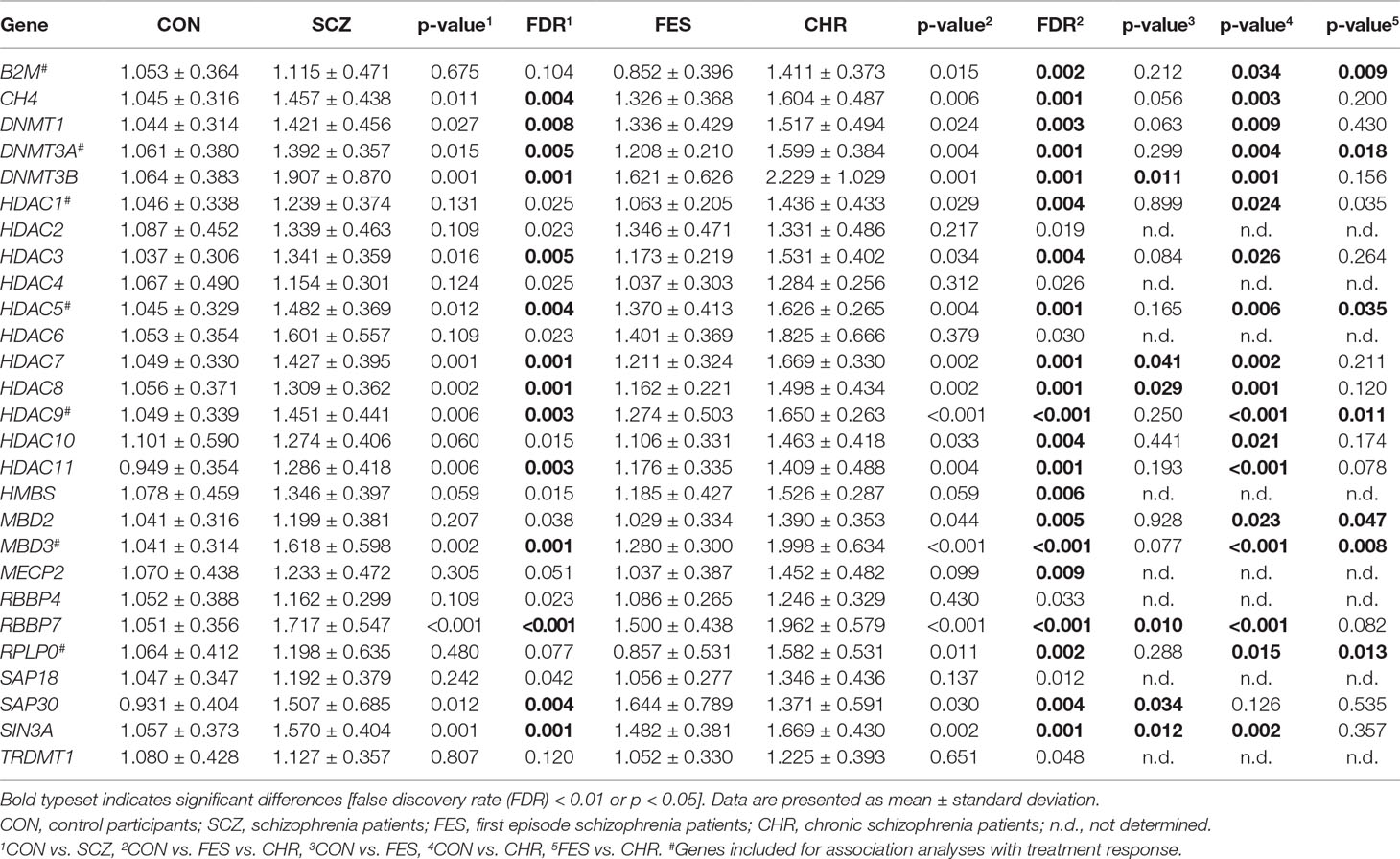
Table 1 Relative expression levels for DNA methylation and transcriptional repression genes between control participants and schizophrenia patients.
Gene Expression Analyses
Differential gene expression between the CON and SCZ groups was determined using unpaired t-tests or Mann-Whitney U tests where appropriate. Additionally, one-way analysis of variance (ANOVA) and Tukey’s post hoc tests were used to determine differences between the CON, FES, and CHR groups. The false discovery rate (FDR) correction (Benjamini and Hochberg, 1995) was used to correct for multiple testing in the CON vs. SCZ and CON vs. FES vs. CHR analyses (27 genes; FDR < 0.01). A significance threshold of p < 0.05 was used for the Tukey’s post hoc tests since these were only performed in the case of a significant (FDR < 0.01) ANOVA result. Candidate genes for genetic association analyses with treatment response were selected if they met the following three criteria: i) significant differences in gene expression between the CHR and CON groups, ii) no significant differences in gene expression between the FES and CON groups, and iii) significant differences in gene expression between the CHR and FES groups.
Participants for Genetic Association Analyses
The patient cohort included 103 unrelated South African FES patients meeting DSM-IV TR (American Psychiatric Association, 2000) diagnostic criteria for SCZ, schizophreniform disorder, or schizo-affective disorder (80% SAC, 12% Xhosa, and 8% European descent) and have been described previously (Drogemöller et al., 2014; Chiliza et al., 2015; Ovenden et al., 2017; O’Connell et al., 2018). All patients received treatment with flupenthixol decanoate (Fluanxol, Lundbeck, Copenhagen, Denmark), a long-acting injectable antipsychotic, according to a fixed protocol. Treatment response was assessed using the Positive and Negative Syndrome Scale (PANSS) (Kay et al., 1987) over a period of 12 months, with measurements taken biweekly for the first 6 weeks, and every 3 months thereafter. Written informed consent was obtained from all patients, or their caregivers, prior to the study, and ethical approval was granted by HREC, Faculty of Medicine and Health Sciences, Stellenbosch University (N06/08/148).
Genetic Association Analyses
Variants within these candidate genes, obtained from each National Center for Biotechnology Information gene page (https://www.ncbi.nlm.nih.gov/gene/), were mined from available genome-wide genotype data. All 103 FES patients described above were previously genotyped with the Infinium OmniExpressExome-8 Kit (Illumina, California, USA) in accordance with the standard Illumina protocol. Variants were excluded from downstream analysis if they exhibited high rates of missing genotype data (> 5%), if their minor allele frequency (MAF) was < 1% or if they showed departure from the Hardy Weinberg equilibrium (p < 1×10-4). For this study, only variants with a minor allele frequency greater than 5% and a call rate greater than 98% were included for the association analyses. Furthermore, only one representative variant was included when two or more variants were shown to be in linkage disequilibrium (LD, r2 > 0.6) with one another. In total, 274 variants were included for analyses. All processing of genetic data was performed using Plink v1.9 (Purcell et al., 2007; Purcell and Chang).
Association analyses were conducted in R (R Core Team, 2017) using R packages lme4 (Bates et al., 2014) and lmerTest (Kuznetsova et al., 2016). Linear mixed-effects models were used to investigate the effect of genetic variants on change in PANSS scores for each subscale (positive, negative, and general) and total over the 12-month period, adjusting for age, gender, proportion ancestry, and baseline PANSS scores. Multiple modes of inheritance were investigated and the Bonferroni correction method was used to correct for multiple testing (274 variants, four modes of inheritance, four PANSS domains; threshold p = 1.14 × 10-5).
Bioinformatic Analyses
Significantly associated variants were identified for intronic variants only, which were assessed for potential functionality as brain-specific eQTLs by interrogating the BRAINEAC (www.braineac.org) (Ramasamy et al., 2014) and GTex (www.gtexportal.org) (GTEx Consortium, 2013, GTEx Consortium, 2015) online databases.
Results
Relative Gene Expression Results
After the initial analysis, comparing gene expression between the CON and SCZ groups, 14 genes were shown to have significantly different expression between these groups. Specifically, the CH4, DNMT1, DNMT3A, DNMT3B, HDAC3, HDAC5, HDAC6, HDAC7, HDAC9, HDAC11, MBD3, RBBP7, SAP30, and SIN3A genes showed increased expression in the SCZ group when compared to the CON group (Table 1). When comparing the CON group to the FES and CHR groups, 19 genes were shown to be differentially expressed (Table 1). Post hoc analyses identified seven genes that were significantly over-expressed in the CHR group when compared to both the CON and FES groups.
For follow-up investigation as candidates for association with antipsychotic treatment response, seven genes were selected. Specifically, the B2M, DNMT3A, HDAC5, HDAC9, MBD2, MBD3, and RPLP0 genes were selected since the expression levels of these genes were significantly different between the CHR and CON groups and CHR and FES groups, respectively (Table 1, Figure 2). Furthermore, no significant differences in gene expression were identified for these genes when comparing the FES and CON groups (Table 1, Figure 2).
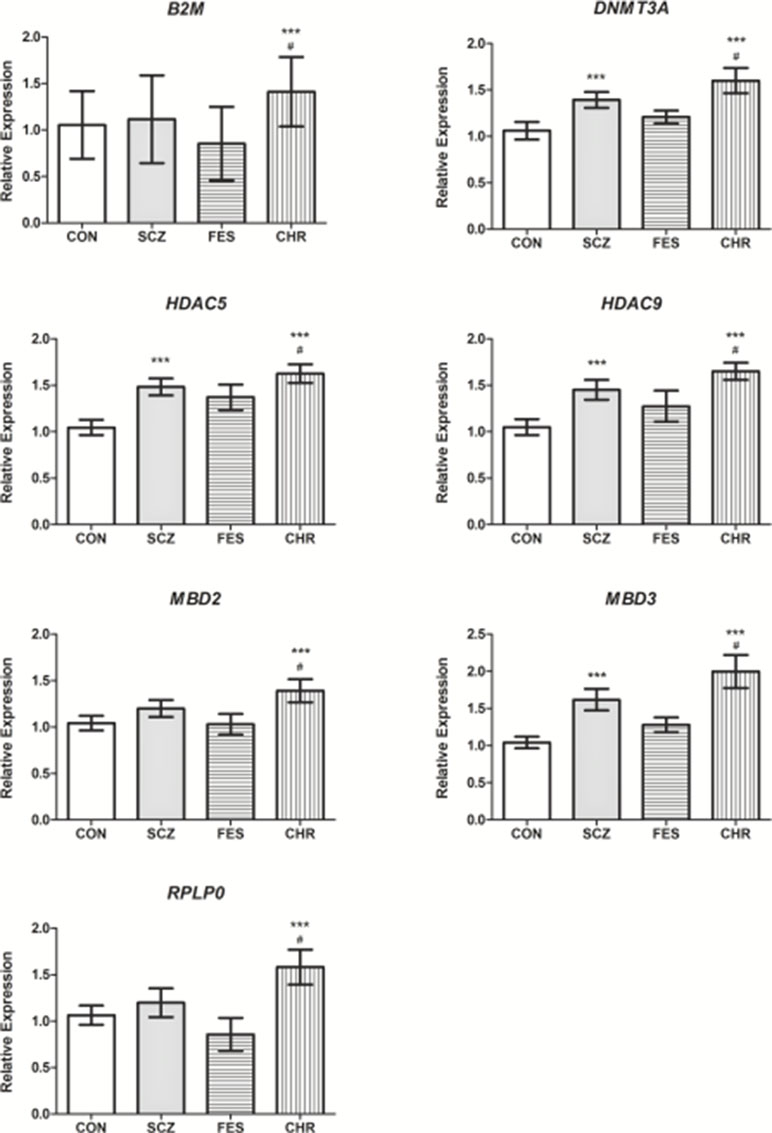
Figure 2 The relative expression levels of B2M, DNMT3A, HDAC5, HDAC9, MBD2, MBD3, and RPLP0 in the SCZ, FES, and CHR groups when compared to the CON group. ***Significant after correction, versus CON group. #Significant after correction, versus FES group. Data is presented as mean ± standard error. Additional details available in Table 1.
Genetic Association Results
Of the 274 variants investigated within the seven candidate genes, four were significantly associated with treatment response, as described by a change in PANSS scores over time, when considering correction for multiple testing (p < 1 × 10-5) (Table 2). All significant associations were identified with the PANSS-negative domain. Specifically, the DNMT3A rs2304429 CC genotype was significantly associated with an improved treatment response (greater reduction in PANSS-negative scores per month) when compared to the TC genotype. The rate of PANSS-N score reduction was significantly faster over time (an additional 1.92% per month) in patients with the DNMT3A rs2304429 CC genotype when compared to patients with the TC genotype. The HDAC5 rs11079983 TT genotype was significantly associated with a poorer treatment response (less reduction in PANSS-negative scores per month) when compared to the CC genotype. Patients with the HDAC5 rs11079983 TT genotype had significantly slower rate of PANSS-N score reduction over time (less by 2.36% per month) when compared to patients with the CC genotype. Two variants within HDAC9 (rs1178119 and rs11764843) were also significantly associated with poorer treatment response (less improved PANSS-negative treatment trajectory scores) as shown in Table 2. For HDAC9 rs1178119, patients with the GA genotype had a significantly slower rate of reduction in PANSS-N scores over time (less by 2.04% per month) than patients with the AA genotype. Presence of the HDAC9 rs1178119 G allele was also significantly associated with a slower rate of reduction in PANSS-N scores over time (less by 1.52% per month per G allele). Similarly, a significantly slower rate of reduction in PANSS-N scores over time (less by 1.92% per month) was also identified for patients with the HDAC9 rs11764843 CA genotype when compared to those with the AA genotype.
Bioinformatics
The variants significantly associated with change in PANSS scores were assessed using the BRAINEAC (Ramasamy et al., 2014) and GTex (GTEx Consortium, 2013, GTEx Consortium, 2015) databases. Two variants (rs2304429 and rs11079983) were identified as potential brain-specific eQTLs. The rs2304429 variant was suggested by BRAINEAC to alter the expression of DNMT3A in particular brain regions—namely, the putamen (PUTM), the cerebellar cortex (CRBL), the temporal cortex (TCTX), and the medulla (MEDU). Specifically, the TT genotype was associated with reduced expression of DNMT3A in these brain regions. In addition, the HDAC5 rs11079983 variant was shown to alter the expression of ASB16 and ASB16-AS1 in the cerebellum when considering the GTex database. Specifically, the TT genotype is associated with reduced expression of ASB16 and increased expression of ASB16-AS1 when compared to the CC genotype, respectively. None of the other variants were identified as brain-specific eQTLs in the BRAINEAC or GTex databases.
Discussion
We identified a number of DNA methylation and transcriptional repression genes to be significantly over-expressed in patients with SCZ, in first-episode and CHR, when compared to healthy controls (Table 1). Specifically, seven candidate regulatory genes were identified, including B2M, DNMT3A, HDAC5, HDAC9, MBD2, MBD3, and RPLP0, and variation within these genes was assessed for association with antipsychotic treatment response. Four variants were found to be significantly associated with poorer treatment trajectory in the PANSS-negative domain.
In this study, significant increases in the expression of DNMT1 and DNMT3A were identified between all patients with SCZ and controls; however, further analyses revealed that this increase was only present when considering the CHR patients and not in the FES patients. Increased DNMT1 and DNMT3A gene expression in the GABAergic neurons of SCZ patients has been previously identified (Zhubi et al., 2009), while the increased expression of DNMT1 was also established in the peripheral blood lymphocytes of patients with SCZ (Auta et al., 2013). When considering these previous studies, it is interesting to note that the mean ages of their study cohorts were 57 ± 11 and 56 ± 18 (Zhubi et al., 2009) and 43.6 ± 10.3 (Auta et al., 2013), respectively. Given the young age of onset of SCZ, it is likely that the patients in these cohorts are more indicative of chronic SCZ and that the results of this study therefore replicate these previous findings.
The expression of a number of HDAC genes (1–4, 6, and 9) was previously investigated in the prefrontal cortex of patients with SCZ (Sharma et al., 2008). Only HDAC1 was shown to have increased expression in patients with SCZ when compared to controls, while no significant differences in expression were identified for the other HDAC genes (Sharma et al., 2008). One possible explanation for the differences between these results and those of our study is that these patients were subject to a range of different medications, including typical and atypical antipsychotics, mood stabilizers (including valproic acid), antidepressants, stimulants, and sedatives which all may have an effect on the gene expression observed (Majchrzak-Celińska and Baer-Dubowska, 2017). Of the other differentially expressed genes in this study, SAP30 expression was previously investigated and no significant changes were identified (Vawter et al., 2006). These results highlight the need for well-defined and deep phenotyping when investigating the molecular etiologies of neuropsychiatric disorders since their molecular architecture is malleable to disorder progression as well as treatment and other environmental factors (Gurwitz and Pirmohamed, 2010).
Due to the nature of the genes investigated in this study, changes in gene expression that were identified are indicative of altered regulatory mechanisms in chronically medicated patients with SCZ (Table 1). The alteration of these regulatory mechanisms is likely the result of a combination of disease progression, antipsychotic medication (Swathy and Banerjee, 2017), and the influence of other environmental factors through the process of epigenetics (Feil and Fraga, 2012). To further elucidate these complex interactions, gene expression differences between first-episode and CHR, as well as healthy controls, were assessed. Significantly increased expression of seven genes (B2M, DNMT3A, HDAC5, HDAC9, MBD2, MBD3, and RPLP0) was observed in patients with chronic SCZ, when compared to FES and healthy controls (Figure 2). These seven genes were selected as candidates for association with antipsychotic treatment response.
Novel associations were identified between variants within DNMT3A (rs2304429), HDAC5 (rs11079983), and HDAC9 (rs1178119, rs11764843) and antipsychotic treatment response as defined by a change in PANSS scores over time (Table 2). Specifically, all four of these variants were associated with a significantly worse treatment trajectory in the negative PANSS symptom domain. Bioinformatics analyses of these variants revealed that two variants are predicted to exert functional changes as eQTLs. The DNMT3A rs2304429 and HDAC5 rs11079983 variants were predicted to alter expression of specific genes in particular brain regions. Specifically, individuals with the rs2304429 CC genotype have increased DNMT3A gene expression in the PUTM, CRBL, TCTX, and MEDU when compared to individuals with the TC or TT genotypes. In addition, individuals with the HDAC5 rs11079983 TT genotype have reduced expression of ASB16 and increased expression of ASB16-AS1 in the cerebellum when compared to the CC genotype. In this study, the rs2304429 CC and rs11079983 CC genotypes infer improved treatment response indicating that the brain region-specific gene expression changes associated with these variants may play a role. From these results, it may be hypothesized that increased expression of DNMT3A, as result of the rs2304429 CC genotype, in the abovementioned brain regions may result in de novo methylation patterns (Okano et al., 1999) that result in increased efficacy of antipsychotic medication. Similarly, reduced expression of ASB16 and ASB16-AS1 in the cerebellum, in the presence of the rs11079983 CC genotype, may result in altered ubiquitin-mediated pathways (Kohroki et al., 2005) and cytokine signaling (Babon et al., 2009) with beneficial effects when considering antipsychotic response. These brain regions have previously been implicated in SCZ (Hokama et al., 1995; Turetsky et al., 1995; Harrison, 2004; Yeganeh-Doost et al., 2011; Williams et al., 2014; Tohid et al., 2015) and suggested as targets for treatment (Buchsbaum et al., 2003; Hugdahl et al., 2009; Mitelman et al., 2009; Parker et al., 2014). These brain region-specific eQTLs should therefore be investigated as biomarkers and potential targets for antipsychotic treatment response.
The potential underlying mechanisms of action associated with the remaining two significant findings are not clear. Further studies investigating the exact role that these variants may have in antipsychotic treatment response is warranted. Furthermore, the novel associations identified in this study should be replicated and validated in additional independent cohorts. Moreover, the eQTL data presented were not generated from Sub-Saharan African-ancestry-related individuals and further studies are required to confirm whether the variants presented here have similar eQTL effects in populations of African-ancestry. Due to the small sample sizes used for the gene expression analyses in this study, these results should be interpreted with caution and require replication. Functional studies incorporating in situ and in vivo assays should also be considered for validation of these results. Moreover, the gene expression results presented in this study should be replicated in female patients with SCZ and controls.
In conclusion, this study identified significant differential expression of DNA methylation and transcriptional repression genes between FES with SCZ, chronically medicated patients with SCZ and healthy controls. Variants within specific differentially expressed genes were significantly associated with antipsychotic treatment response, and highlighted particular brain regions in which altered expression of specific genes may play a role in treatment outcome. These results may aid in the development of biomarkers for antipsychotic treatment response, as well as, novel drug targets, and treatment strategies.
Ethics Statement
Written and informed consent was obtained from all patients, or their caregivers, prior to the study and ethical approval was granted by the Human Research and Ethics Committee (HREC), Faculty of Medicine and Health Sciences, Stellenbosch University (N13/08/115 and N06/08/148).
Author Contributions
KO’C NM, and LW conceived the study. KO’C performed the laboratory work and statistical analyses. RE and SS were involved in participant recruitment. KO’C wrote the initial draft for the manuscript. NM, LW, RE, and SS provided detailed critique of the manuscript. All authors approved the final manuscript for submission.
Funding
This work was supported by the South African Medical Research Council “SHARED ROOTS” Flagship Project Grant no. MRC-RFA-IFSP-01-2013/SHARED ROOTS (Prof Soraya Seedat, Department of Psychiatry, Stellenbosch University) and the National Research Foundation (NRF): KO was funded by the Scarce Skills Post-Doctoral Fellowship (grant no. 96833). LW was funded by the Competitive Program for Rated Researchers (grant no. 93498) and the Bioinformatics and Functional Genomics Program (grant no. 93681). The opinions expressed and conclusions arrived at are those of the authors and are not necessarily attributed to these funding sources.
Conflict of Interest Statement
The authors declare that the research was conducted in the absence of any commercial or financial relationships that could be construed as a potential conflict of interest.
Supplementary Material
The Supplementary Material for this article can be found online at: https://www.frontiersin.org/articles/10.3389/fgene.2019.00641/full#supplementary-material
References
American Psychiatric Association (2000) Diagnostic and Statistical Manual of Mental Disorders. 4th Edition. (DSM-IV-TR).
Auta, J., Smith, R. C., Dong, E., Tueting, P., Sershen, H., Boules, S., et al. (2013). DNA-methylation gene network dysregulation in peripheral blood lymphocytes of schizophrenia patients. Schizophr. Res. 150, 312–318. doi: 10.1016/j.schres.2013.07.030
Babon, J. J., Sabo, J. K., Zhang, J.-G., Nicola, N. A., Norton, R. S. (2009). The SOCS box encodes a hierarchy of affinities for Cullin5: implications for ubiquitin ligase formation and cytokine signalling suppression. J. Mol. Biol. 387, 162–174. doi: 10.1016/j.jmb.2009.01.024
Bakker, P. R., van Harten, P. N., van Os, J. (2008). Antipsychotic-induced tardive dyskinesia and polymorphic variations in COMT, DRD2, CYP1A2 and MnSOD genes: a meta-analysis of pharmacogenetic interactions. Mol. Psychiatry 13, 544–556. doi: 10.1038/sj.mp.4002142
Bates, D., Mächler, M., Bolker, B., Walker, S. (2015). Fitting linear mixed-effects models using lme4. J. Stat. Softw. 67, 1–48. doi: 10.18637/jss.v067.i01
Benjamini, Y., Hochberg, Y. (1995). Controlling the false discovery rate: a practical and powerful approach to multiple testing. J. R. Stat. Soc. Ser. B Methodol. 57, 289–300. doi: 10.1111/j.2517-6161.1995.tb02031.x
Bostick, M., Kim, J. K., Estève, P.-O., Clark, A., Pradhan, S., Jacobsen, S. E. (2007). UHRF1 plays a role in maintaining DNA methylation in mammalian cells. Science 317, 1760–1764. doi: 10.1126/science.1147939
Brandl, E. J., Kennedy, J. L., Müller, D. J. (2014). Pharmacogenetics of antipsychotics. Can. J. Psychiatry Rev. Can. Psychiatr. 59, 76–88. doi: 10.1177/070674371405900203
Buchsbaum, M. S., Shihabuddin, L., Brickman, A. M., Miozzo, R., Prikryl, R., Shaw, R., et al. (2003). Caudate and putamen volumes in good and poor outcome patients with schizophrenia. Schizophr. Res. 64, 53–62. doi: 10.1016/S0920-9964(02)00526-1
Chiliza, B., Asmal, L., Kilian, S., Phahladira, L., Emsley, R. (2015). Rate and predictors of non-response to first-line antipsychotic treatment in first-episode schizophrenia. Hum. Psychopharmacol. 30, 173–182. doi: 10.1002/hup.2469
Chowdhury, N. I., Remington, G., Kennedy, J. L. (2011). Genetics of antipsychotic-induced side effects and agranulocytosis. Curr. Psychiatry Rep. 13, 156–165. doi: 10.1007/s11920-011-0185-3
Drogemöller, B. I., Niehaus, D. J. H., Chiliza, B., van der Merwe, L., Asmal, L., Malhotra, A. K., et al. (2014). Patterns of variation influencing antipsychotic treatment outcomes in South African first-episode schizophrenia patients. Pharmacogenomics 15, 189–199. doi: 10.2217/pgs.13.218
Feil, R., Fraga, M. F. (2012). Epigenetics and the environment: emerging patterns and implications. Nat. Rev. Genet. 13, 97–109. doi: 10.1038/nrg3142
GTEx Consortium (2013). The Genotype-Tissue Expression (GTEx) project. Nat. Genet. 45, 580–585. doi: 10.1038/ng.2653
Gurwitz, D., Pirmohamed, M. (2010). Pharmacogenomics: the importance of accurate phenotypes. Pharmacogenomics 11, 469–470. doi: 10.2217/pgs.10.41
Harrison, P. J. (2004). The hippocampus in schizophrenia: a review of the neuropathological evidence and its pathophysiological implications. Psychopharmacology (Berl). 174, 151–162. doi: 10.1007/s00213-003-1761-y
Hokama, H., Shenton, M. E., Nestor, P. G., Kikinis, R., Levitt, J. J., Metcalf, D., et al. (1995). Caudate, putamen, and globus pallidus volume in schizophrenia: a quantitative MRI study. Psychiatry Res. 61, 209–229. doi: 10.1016/0925-4927(95)02729-H
Hugdahl, K., Løberg, E.-M., Nygård, M. (2009). Left Temporal Lobe Structural and Functional Abnormality Underlying Auditory Hallucinations in Schizophrenia. Front. Neurosci. 3, 34–45. doi: 10.3389/neuro.01.001.2009
Kay, S. R., Fiszbein, A., Opler, L. A. (1987). The positive and negative syndrome scale (PANSS) for schizophrenia. Schizophr. Bull. 13, 261–276. doi: 10.1093/schbul/13.2.261
Kohroki, J., Nishiyama, T., Nakamura, T., Masuho, Y. (2005). ASB proteins interact with Cullin5 and Rbx2 to form E3 ubiquitin ligase complexes. FEBS Lett. 579, 6796–6802. doi: 10.1016/j.febslet.2005.11.016
Kurita, M., Holloway, T., García-Bea, A., Kozlenkov, A., Friedman, A. K., Moreno, J. L., et al. (2012). HDAC2 regulates atypical antipsychotic responses through the modulation of mGlu2 promoter activity. Nat. Neurosci. 15, 1245–1254. doi: 10.1038/nn.3181
Kuznetsova, A., Brockhoff, P., Bojesen Christensen, R. (2016). lmerTest: Tests in Linear Mixed Effects Models. R package version 2.0–33, Available at: https://CRAN.R-project.org/package=lmerTest.
Lencz, T., Robinson, D. G., Napolitano, B., Sevy, S., Kane, J. M., Goldman, D., et al. (2010). DRD2 promoter region variation predicts antipsychotic-induced weight gain in first episode schizophrenia. Pharmacogenet. Genomics 20, 569–572. doi: 10.1097/FPC.0b013e32833ca24b
Leucht, S., Davis, J. M. (2017). Schizophrenia, primary negative symptoms, and soft outcomes in psychiatry. Lancet Lond. Engl. 389, 1077–1078. doi: 10.1016/S0140-6736(17)30181-2
Lieberman, J. A., Perkins, D., Belger, A., Chakos, M., Jarskog, F., Boteva, K., et al. (2001). The early stages of schizophrenia: speculations on pathogenesis, pathophysiology, and therapeutic approaches. Biol. Psychiatry 50, 884–897. doi: 10.1016/S0006-3223(01)01303-8
Liou, Y.-J., Wang, H.-H., Lee, M.-T. M., Wang, S.-C., Chiang, H.-L., Chen, C.-C., et al. (2012). Genome-wide association study of treatment refractory schizophrenia in Han Chinese. PloS One 7, e33598. doi: 10.1371/journal.pone.0033598
Livak, K. J., Schmittgen, T. D. (2001). Analysis of relative gene expression data using real-time quantitative PCR and the 2(-Delta Delta C(T)) Method. Methods San Diego Calif. 25, 402–408. doi: 10.1006/meth.2001.1262
Lohoff, F. W., Ferraro, T. N. (2010). Pharmacogenetic considerations in the treatment of psychiatric disorders. Expert Opin. Pharmacother. 11, 423–439. doi: 10.1517/14656560903508762
Majchrzak-Celińska, A., Baer-Dubowska, W. (2017). Pharmacoepigenetics: an element of personalized therapy? Expert Opin. Drug Metab. Toxicol. 13, 387–398. doi: 10.1080/17425255.2017.1260546
Manolio, T. A., Collins, F. S., Cox, N. J., Goldstein, D. B., Hindorff, L. A., Hunter, D. J., et al. (2009). Finding the missing heritability of complex diseases. Nature 461, 747–753. doi: 10.1038/nature08494
Melas, P. A., Rogdaki, M., Ösby, U., Schalling, M., Lavebratt, C.Ekström, T. J. (2012). Epigenetic aberrations in leukocytes of patients with schizophrenia: association of global DNA methylation with antipsychotic drug treatment and disease onset. FASEB J. Off. Publ. Fed. Am. Soc. Exp. Biol. 26, 2712–2718. doi: 10.1096/fj.11-202069
Mitelman, S. A., Canfield, E. L., Chu, K.-W., Brickman, A. M., Shihabuddin, L., Hazlett, E. A., et al. (2009). Poor outcome in chronic schizophrenia is associated with progressive loss of volume of the putamen. Schizophr. Res. 113, 241–245. doi: 10.1016/j.schres.2009.06.022
O’Connell, K. S., McGregor, N. W., Malhotra, A., Lencz, T., Emsley, R., Warnich, L. (2018). Variation within voltage-gated calcium channel genes and antipsychotic treatment response in a South African first episode schizophrenia cohort. Pharmacogenomics J. 19, 109–114. doi: 10.1038/s41397-018-0033-5
Okano, M., Bell, D. W., Haber, D. A., Li, E. (1999). DNA methyltransferases Dnmt3a and Dnmt3b are essential for de novo methylation and mammalian development. Cell 99, 247–257. doi: 10.1016/S0092-8674(00)81656-6
Ovenden, E. S., Drögemöller, B. I., van der Merwe, L., Chiliza, B., Asmal, L., Emsley, R. A., et al. (2017). Fine-mapping of antipsychotic response genome-wide association studies reveals novel regulatory mechanisms. Pharmacogenomics 18, 105–120. doi: 10.2217/pgs-2016-0108
Parker, K. L., Narayanan, N. S., Andreasen, N. C. (2014). The therapeutic potential of the cerebellum in schizophrenia. Front. Syst. Neurosci. 8, 163. doi: 10.3389/fnsys.2014.00163
Purcell, S., Chang, C. Plink v1.9, Available at: https://www.cog-genomics.org/plink2.
Purcell, S., Neale, B., Todd-Brown, K., Thomas, L., Ferreira, M. A. R., Bender, D., et al. (2007). PLINK: a tool set for whole-genome association and population-based linkage analyses. Am. J. Hum. Genet. 81, 559–575. doi: 10.1086/519795
R Core Team (2017). R: A language and environment for statistical computing. Vienna, Austria: R Foundation for Statistical Computing . Available at: http://www.R-project.org/.
Ramasamy, A., Trabzuni, D., Guelfi, S., Varghese, V., Smith, C., Walker, R., et al. (2014). Genetic variability in the regulation of gene expression in ten regions of the human brain. Nat. Neurosci. 17, 1418–1428. doi: 10.1038/nn.3801
Sharma, R., Grayson, D. R., Gavin, D. P. (2008). Histone Deactylase 1 expression is increased in the prefrontal cortex of Schizophrenia subjects; analysis of the National Brain Databank microarray collection. Schizophr. Res. 98, 111–117. doi: 10.1016/j.schres.2007.09.020
Swathy, B., Banerjee, M. (2017). Understanding epigenetics of schizophrenia in the backdrop of its antipsychotic drug therapy. Epigenomics 9, 721–736. doi: 10.2217/epi-2016-0106
Tandon, R., Nasrallah, H. A., Keshavan, M. S. (2009). Schizophrenia, “just the facts” 4. Schizophr. Res. 110, 1–23. doi: 10.1016/j.schres.2009.03.005
Tandon, R., Nasrallah, H. A., Keshavan, M. S. (2010). Schizophrenia, “just the facts” 5. Schizophr. Res. 122, 1–23. doi: 10.1016/j.schres.2010.05.025
Tang, H., Dalton, C. F., Srisawat, U., Zhang, Z. J., Reynolds, G. P. (2014). Methylation at a transcription factor-binding site on the 5-HT1A receptor gene correlates with negative symptom treatment response in first episode schizophrenia. Int. J. Neuropsychopharmacol. Off. Sci. J. Coll. Int. Neuropsychopharmacol. CINP 17, 645–649. doi: 10.1017/S1461145713001442
Tohid, H., Faizan, M., Faizan, U. (2015). Alterations of the occipital lobe in schizophrenia. Neurosciences 20, 213–224. doi: 10.17712/nsj.2015.3.20140757
Turetsky, B., Cowell, P. E., Gur, R. C., Grossman, R. I., Shtasel, D., Gur, R. E. (1995). Frontal and temporal lobe brain volumes in schizophrenia. Arch. Gen. Psychiatry 52, 1061–1070. doi: 10.1001/archpsyc.1995.03950240079013
Vawter, M. P., Atz, M. E., Rollins, B. L., Cooper-Casey, K. M., Shao, L.Byerley, W. F. (2006). Genome scans and gene expression microarrays converge to identify gene regulatory loci relevant in schizophrenia. Hum. Genet. 119, 558–570. doi: 10.1007/s00439-006-0172-7
Williams, M. R., Galvin, K., O’Sullivan, B., MacDonald, C. D., Ching, E. W. K., Turkheimer, F., et al. (2014). Neuropathological changes in the substantia nigra in schizophrenia but not depression. Eur. Arch. Psychiatry Clin. Neurosci. 264, 285–296. doi: 10.1007/s00406-013-0479-z
Wu, H., Tao, J., Sun, Y. E. (2012). Regulation and function of mammalian DNA methylation patterns: a genomic perspective. Brief. Funct. Genomics 11, 240–250. doi: 10.1093/bfgp/els011
Xie, F., Xiao, P., Chen, D., Xu, L., Zhang, B. (2012). miRDeepFinder: a miRNA analysis tool for deep sequencing of plant small RNAs. Plant Mol. Biol. 80, 75–84. doi: 10.1007/s11103-012-9885-2
Yeganeh-Doost, P., Gruber, O., Falkai, P., Schmitt, A. (2011). The role of the cerebellum in schizophrenia: from cognition to molecular pathways. Clinics 66, 71–77. doi: 10.1590/S1807-59322011001300009
Zhang, J.-P., Malhotra, A. K. (2013). Pharmacogenetics of antipsychotics: recent progress and methodological issues. Expert Opin. Drug Metab. Toxicol. 9, 183–191. doi: 10.1517/17425255.2013.736964
Zhubi, A., Veldic, M., Puri, N. V., Kadriu, B., Caruncho, H., Loza, I., et al. (2009). An upregulation of DNA-methyltransferase 1 and 3a expressed in telencephalic GABAergic neurons of schizophrenia patients is also detected in peripheral blood lymphocytes. Schizophr. Res. 111, 115–122. doi: 10.1016/j.schres.2009.03.020
Keywords: schizophrenia, epigenetics, neuropsychiatric genetics, gene expression, treatment response
Citation: O’Connell KS, McGregor NW, Emsley R, Seedat S and Warnich L (2019) The Potential Role of Regulatory Genes (DNMT3A, HDAC5, and HDAC9) in Antipsychotic Treatment Response in South African Schizophrenia Patients. Front. Genet. 10:641. doi: 10.3389/fgene.2019.00641
Received: 31 October 2018; Accepted: 18 June 2019;
Published: 10 July 2019.
Edited by:
Zané Lombard, University of the Witwatersrand, South AfricaReviewed by:
Monica Uddin, University of South Florida, United StatesBoer Xie, St. Jude Children’s Research Hospital, United States
Copyright © 2019 O’Connell, McGregor, Emsley, Seedat and Warnich. This is an open-access article distributed under the terms of the Creative Commons Attribution License (CC BY). The use, distribution or reproduction in other forums is permitted, provided the original author(s) and the copyright owner(s) are credited and that the original publication in this journal is cited, in accordance with accepted academic practice. No use, distribution or reproduction is permitted which does not comply with these terms.
*Correspondence: Dr. Nathaniel Wade McGregor, nwm@sun.ac.za