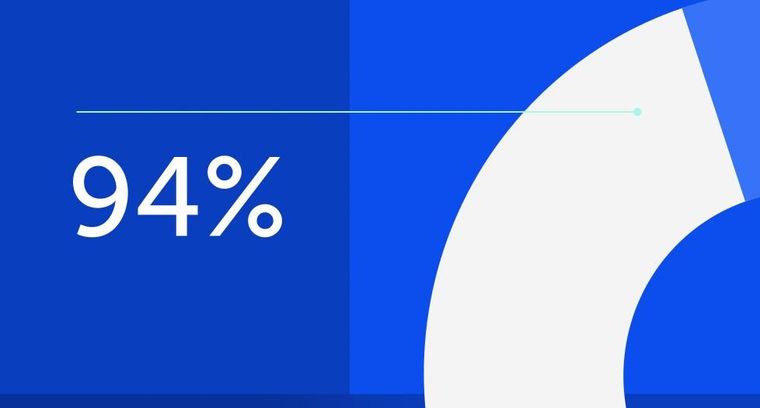
94% of researchers rate our articles as excellent or good
Learn more about the work of our research integrity team to safeguard the quality of each article we publish.
Find out more
POLICY AND PRACTICE REVIEWS article
Front. Genet., 29 July 2019
Sec. ELSI in Science and Genetics
Volume 10 - 2019 | https://doi.org/10.3389/fgene.2019.00611
This article is part of the Research TopicBig Data EthicsView all 4 articles
The clinical utility of computational phenotyping for both genetic and rare diseases is increasingly appreciated; however, its true potential is yet to be fully realized. Alongside the growing clinical and research availability of sequencing technologies, precise deep and scalable phenotyping is required to serve unmet need in genetic and rare diseases. To improve the lives of individuals affected with rare diseases through deep phenotyping, global big data interrogation is necessary to aid our understanding of disease biology, assist diagnosis, and develop targeted treatment strategies. This includes the application of cutting-edge machine learning methods to image data. As with most digital tools employed in health care, there are ethical and data governance challenges associated with using identifiable personal image data. There are also risks with failing to deliver on the patient benefits of these new technologies, the biggest of which is posed by data siloing. The Minerva Initiative has been designed to enable the public good of deep phenotyping while mitigating these ethical risks. Its open structure, enabling collaboration and data sharing between individuals, clinicians, researchers and private enterprise, is key for delivering precision public health.
All areas of health care stand to benefit from data sharing, but no group perhaps more so than patients with rare disorders affecting facial morphology and their families (Boycott et al., 2017). This paper presents the Minerva Initiative, a global initiative to enable integration of facial photographs and medical information across health care systems and for research. The purpose of this initiative is to empower global research into rare diseases through computational phenotyping from personally identifiable data.
New machine learning approaches hold great promise for transforming health care. By interrogating vast amounts of rich data, they can develop tools that empower clinical care. Making personal data available to researchers across the world raises a number of ethical, legal, data security, and societal challenges. Among the issues one must consider are the following: What is the scope for anonymization of data? How can the rights of individuals be protected in a rapidly changing digital world? How does one enable the potential positive benefits of data sharing? These require the development of new ways of working with, and securely sharing, identifiable data in a scalable and rigorous manner.
Below, we outline the Minerva Initiative—a research data resource (Minerva Image Resource—MIR) and an open research consortium (Minerva Consortium—MC), which has been set up to allow the sharing of identifiable patient data, such as facial photographs and collaborative research projects on rare diseases.
With the advances in clinical and research availability of next-generation sequencing technologies in settings exemplified by initiatives such as the 100,000 genomes project (Caulfield et al., 2017), the All of Us initiatives (“National Institutes of Health (NIH)—All of Us”, n.d.) and the Undiagnosed Diseases Network International (Taruscio et al., 2015; “UDNI”, n.d.), one could be forgiven for thinking that the problem of rare diseases has been largely solved. However, sequencing in clinical settings only aids diagnosis of about 50–60% of rare diseases (Boycott et al., 2017) (selection of patient population influences what this number means in practice), leaving a large group of undiagnosed patients.
We all carry de novo and rare variants predicted to have gene-damaging effects that could lead to the variant being interpreted as putatively pathogenic (MacArthur and Tyler-Smith, 2010). Coming to the conclusion that a particular variant is disease contributing for a set of phenotypes is not trivial. There are a number of initiatives to collect information on patient phenotypes, gene, and variants in databases, such as DECIPHER (Wright et al., 2018), PhenomeCentral (Buske et al., 2015a), PhenoDB (Hamosh et al., 2013), GeneMatcher (Sobreira et al., 2015), IRUD (Adachi et al., 2017), KCCG Patient Archive (“Patient Archive”, n.d.), MyGene2 (“MyGene2—Home” 2, n.d.), Human Disease Gene Website series (“Human Disease Genes”, n.d.), ClinVar (Landrum et al., 2016), and the Centers for Mendelian Genomics (Chong et al., 2015). Many of these resources are connected as the Matchmaker Exchange (Philippakis et al., 2015), via a common Application Program Interface (Buske et al., 2015b), allowing researchers and clinicians to identify additional patients for novel disorders, and in some cases collect additional cases for known ones. However, as these resources grow, it is no longer safe to infer pathogenic causality just because a putative variant has been observed in another patient previously. As the number of variants of uncertain significance grows, it is expected that the rate of false-positive mutation matches will also increase (Dorschner et al., 2013; Akle et al., 2015; Krawitz et al., 2015). Consequently, the current approach to search disease databases for a mutation match might be insufficient to identify new pathogenic mutations. Patients with very different rare diseases are still likely to have rare mutation matches by random chance. Employing phenotype metrics could add additional power to DNA variant pathogenicity classifications and should feature in efforts to understand genomic variants in clinical settings, such as ClinGen (Rehm et al., 2015). Deep and objective phenotyping delivers an independent source of information that can contribute to inferences about disease-contributing associations (Bone et al., 2016; Pena et al., 2018; Köhler et al., 2017; Robinson et al., 2015). If two patients share a variant of uncertain significance (VUS) and also have similar rare phenotypic manifestations, then the likelihood of the variant being disease contributing is much higher. Certainly, if the putative variants and the combination of clinical metrics are both rare, then the chance of randomly encountering the same combination in an unrelated individual is even less likely (Sifrim et al., 2013; Javed et al., 2014; Singleton et al., 2014; Robinson et al., 2014; Pengelly et al., 2017). Even so, such hypothetical associations should be contextualized in terms of null expectations given variant mutation and population allele frequencies, and additionally verified with functional genetic studies (Akle et al., 2015; Krawitz et al., 2015).
Precise deep phenotyping of patient traits, including facial characteristics, is one such way of aiding the mapping and matching of disease-associated traits. This has the potential to aid the clinical pathways to diagnosis and to empower inference of disease-contributing associations to genetic variants. This promise is contingent on having enough high-quality and accurate data to build methods for extracting disease relevant phenotypes, but also the numbers to link rare and ultra-rare disorders. Translating deep phenotyping approaches to clinical utility is a big data challenge.
Developments in computer vision and deep learning are being applied to patient datasets with the aim of aiding diagnosis, prediction of outcomes, and monitoring of clinical phenotypes. One of the first clinical settings where this is being applied is clinical dysmorphology for rare diseases.
It should be noted that the idea to objectively assess body form (anthropometrics) in Western medicine was pioneered by Francis Galton well over a century ago (Galton, 1879) and applied to images by Sheldon et al., in 1940 (Sheldon et al., 1945). However, the formalized discipline of clinical dysmorphology, as the study of birth defects, was not conceptualized until 1966 (Smith, 1966). The challenge of bringing to bear machine learning and data analytic approaches to clinical dysmorphology, and anthropometrics from image data, has been the target of many threads of research. Various research endeavors for extracting objective phenotype metrics have been applied to both 2D (Herpers et al., 1993; Loos et al., 2003; Vollmar et al., 2008; Balliu et al., 2014) and 3D craniofacial imaging (Hammond et al., 2004; Hammond et al., 2005; Hennessy et al., 2010; Hammond et al., 2012; Baynam G. et al., 2013; Baynam G.S. et al., 2013; Kung et al., 2015; Baynam et al., 2016).
Despite the precision and objectivity of these methods, their practical application has been relatively limited. This can be largely attributed to a combination of expensive instrumentation, a requirement that patients are able to pose for imaging, and the need for expertise for the manual steps in subsequent data analyses. Recent developments in imaging platforms and machine learning capabilities provide the foundation for applications with greater clinical utility. High-quality 2D digital imaging cameras have become ubiquitously available, and 3D capabilities are beginning to enter the consumer market. Also, cutting-edge deep convolutional neural networks approaches are transforming the way these images can be analyzed. It has been shown that they can be trained to be highly robust for imaging variation, reducing the need for highly controlled subject poses (Xiangyu Zhu et al., 2015). There are a number of current research and commercial efforts to create fully automated analysis pipelines for clinical interpretation of dysmorphologies (Ansari et al., 2014; Ferry et al., 2014; Manousaki et al., 2015; Basel-Vanagaite et al., 2016; Gripp et al., 2016; Baynam et al., 2017; Bengani et al., 2017; Dudding-Byth et al., 2017; Deciphering Developmental Disorders Study, 2017; Gardner et al., 2017; Hadj-Rabia et al., 2017; Kruszka et al., 2017a; Kruszka et al., 2017b; Kruszka et al., 2017c; Lumaka et al., 2017; Shukla et al., 2017; Valentine et al., 2017; Reijnders et al., 2018b; Gurovich et al., 2018; Knaus et al., 2018; Kruszka et al., 2018; Liehr et al., 2018; Pantel et al., 2018; Reijnders et al., 2018a; Reijnders et al., 2018b; Zarate et al., 2018). However, all these efforts are meeting the same barriers to progression of the methods and prospects for clinical impact, challenges to do with data access, ethics, governance, and security. Without addressing these issues on a common basis, these efforts will struggle to deliver on their potential patient benefits.
There is a long history of the use of medical algorithms. The clinical process of establishing a diagnosis and suitable treatment is essentially a step-wise application of decisions facilitated by objective tools and metrics and augmented by cumulative knowledge and experience. Artificial intelligence, or machine learning, in health care employs the same principles to improve and accelerate clinical pathways. New machine learning approaches could add value in health care sectors challenged by extreme data volumes or complexity of inferences needed.
While there is a noticeable rush of “big data” applications in health care, the delivered clinical utility has so far been very limited. This lack of clinical utility has been attributed to the poor model interpretability or performance on real world data. All deep learning approaches are reliant on large datasets to learn from in order to perform accurately. Identifiable medical datasets are typically relatively small in the context of deep learning, and data are usually sourced from single health care settings or specific populations. This makes models over fit, that is to say, become overly specific to the particular datasets they have been shown, and consequently, they perform poorly on new datasets. To efficiently use big data approaches, we need to address the challenges of working with data outside of the isolated silos of single projects or health care systems.
Ethical, legal, data security, and intellectual property issues are key barriers preventing the ascertainment, interrogation of big datasets, and the implementation of big data solutions in health care. Traditional research consent structures may constrain or prevent data sharing and the integration of datasets essential for these new approaches. In the context of the strict regulation of and accountability in health care data, if there are any doubts about the rules around sharing, the default position is to not share. Consequently, data sharing is unlikely to happen without specific consent for integration of personally identifiable information. Consequently, most data-sharing efforts bringing together global data rely heavily on de-identification or the minimization of sharing more sensitive data (Dyke et al., 2017).
The reality of big data is that anonymization might not be possible (Gymrek et al., 2013; de Montjoye et al., 2015). Furthermore, data security concerns for data access rights and the prospects of malicious digital activity (hacking) imply often unacceptable risk where organizations are liable for data loss. Finally, data sharing is inhibited by concerns about intellectual property rights: who owns the data and to whom do any findings or inventions derived from the data belong? In practice, these factors all tend to result in data siloing, since the easiest way to control the use of data is to keep it hidden. However, such practices hamper the prospects of new public good, increased clinical utility, and impact.
Moreover, data silos inevitably build in data biases, which create implicitly biased models, which in turn limit generalization to new data. For big medical data to deliver on its potential clinical utility, generally and equitably, approaches must be able to generalize across health care systems and different populations. Such an approach will deliver solutions from a basis of “n = 1” approach prevalent in precision medicine approaches to an “n = many” impact under a precision public health paradigm (Collins and Varmus, 2015; Weeramanthri et al., 2018; “Precision Public Health: What Is It? | | Blogs | CDC”, n.d.).
The Minerva Initiative has been developed to enable research into the use of image analysis for the diagnosis of diseases, prevent data siloing, and foster further healthy competition between various image phenotyping approaches. The Minerva Initiative is an effort to construct a precompetitive space for enabling research on clinical phenotyping tool development. It has been constructed in the spirit of open science (Woelfle et al., 2011; Nielsen, 2012) and within the bounds of ethics and data governance constraints.
The Minerva Initiative has the following objectives: to build a community of researchers and clinicians; to continue to develop ethical structures and provisions for working on identifiable clinical images; and to deliver secure data sharing between consortium members. It has been constructed to align with the goals and objectives of the Global Alliance for Genomics & Health (“Global Alliance for Genomics and Health”, n.d.) and the International Rare Diseases Research Consortium (IRDiRC) (“IRDiRC”, n.d.).
The Minerva Consortium (MC) is an international network of clinicians and researchers, from both public and private organizations, involved in the Minerva Initiative. Within the MC, there are many purposes and interests, but all MC members seek to establish a commonly agreed set of conduct and praxis for the benefit of all stakeholders: patients, participants, researchers, clinicians, and social systems.
The MC consists of a management group, clinical collaborators, working groups, and phenotyping groups. The management group is tasked with guiding directions of joint MC efforts, MC policy and strategy, acceptance of new MC Computational Phenotyping Groups, and dispute resolution. For prospective data collected under the Minerva Initiative consent, the Management Group also acts as a Data Access Committee overseeing Phenotyping Groups’ access to the Minerva Image Resource. The goal of the MC is not to consolidate all phenotyping projects to one unified approach but rather to foster an environment to enable a multiplicity of methods to develop.
To improve the models for phenotype descriptions through deep learning, data need to be brought together in a unified compute system. Distributed systems are not feasible because either they sacrifice security, by virtue of exchange of semi-identifiable data, or because data transfer time and volumes are increased to such a level they are not workable. While fixed algorithms can be deployed in distributed frameworks, this would also prevent iterative learning regimens, which are required for continuing improvements. Consequently, the Minerva Consortium has focused on legal, ethical, and data governance structures to allow the global pooling of data in a unified system.
The Minerva Image Resource (MIR) is a centralized repository of personally identifiable data, which covers both images and linked medical data. While the facial images are inherently identifiable, other directly identifiable data, such as names, addresses, social security numbers, or any direct hospital identifiers, are not stored in the MIR. Coded identifiers, supporting privacy preserving record linkage, are stored in the MIR, but the linkage to any other data remains with the original data controllers—but are expected to be available through Matchmaker Exchange linked initiatives (Philippakis et al., 2015).
Patient datasets that comprise collected data and images from previous projects can be joined into the MIR if the existing consent allows for data use for general research purposes. The threshold that should be met for data to be contributed to the MIR is consented for use in research, not necessarily consented for publication. No identifiable information held in the MIR is shared outside the scope of legal agreements covering agreed research purposes.
The MC has a defined standardized Material Transfer Agreement and collaborator letter that is recommended for use within the consortium, but ultimately it is up to each clinical collaborator and phenotyping group to sign any given agreement. It is up to MC Clinical Collaborators to vouch that this consent is in place and that the wording of the patient consent encompasses image data. This is also specified in the Terms and Conditions for the Material Transfer Agreements. The expectation is that data should be shared as openly as possible within the consortium within the permissible scope of consent and data governance restrictions.
Clinicians can recruit patients into the Minerva Initiative if they obtain consent for the use of patient’s data and images collected through routine clinical practice. The Minerva Consortium have drafted patient information and consent forms, but locally valid research ethics approvals need to be sought for the inclusion of patient data and images in Minerva Consortium research. The patient consent forms cover a broad remit of health-related research using images and associated clinical data.
Once research ethics committee-approved based consent has been given and a Data Submission Agreement is in place, data can be entered into the MIR and become part of the Minerva Initiative. The data flow pathway for this is not prescriptive. The data could, in future, be deposited in the MIR directly or through affiliated third-party apps or other health care record platforms.
Data collected prospectively through clinical collaborators using the Minerva Initiative consent will be shared between all Phenotyping Groups in good standing with the Minerva Consortium (having been approved by the MC Management Group and having a legal agreement for data access with the MIR). To be clear, in line with these structures, the original photographs will thereby be shared with these phenotyping groups.
A public website has been constructed, Minerva&Me (https:///www.minervaandme.com) (“Minerva and Me—Help Rare Disease Research”, n.d.), which allows anyone around the world to participate directly in the Minerva Initiative. Through Minerva&Me participants are able to enter basic information about themselves and any medical diagnoses they might have and to upload images. Photographs of themselves can be “selfies” or scanned images from photographs in existing family albums. Participants retain control of the use of their image and associated personal data. Minerva&Me employs a dynamic consent model (Kaye et al., 2015; “Platform for Engaging Everyone Responsibly | GeneticAlliance.Org”, n.d.), whereby participants are able to amend their consent or delete their data from the MIR directly.
Minerva&Me has been reviewed and approved by a research ethics committee (Oxford Tropical Research Ethics Committee at the University of Oxford), and also has a governing Advisory Board. This Advisory Board has representatives from clinicians, lawyers, data security experts, and patient advocacy groups including Unique, NORD, Rare Voices Australia, and Rare Disease UK. This Advisory Board has oversight of any future developments and directions for Minerva&Me.
In future versions of Minerva&Me, we intend to allow participants the option to enable their doctor to have limited access to their data analyses through coded linkers. The purpose of such links would be to allow further clinical information to be integrated by the MC Clinical Collaborator and, in future, allow a potential feedback route through the clinician to patient (if and when returnable findings are generated). Consent sought between patient and clinician must then cover the use of clinical data through the Minerva Consortium and the linkage to Minerva&Me through coded identifiers.
Identifiable data within the MIR is only available to the original data submitters or MC Computational Phenotyping Groups. MC Computational Phenotyping Group researchers could be from all over the world and may be working in academia or commercial companies. Access to the Minerva Image Resource is only granted for purposes that align with the research goals of the Minerva Initiative (to improve diagnosis, clinical treatment, and understanding of a wide range of illnesses). Data access conditions are further explained below.
The MIR, as a precompetitive space, does not enforce a single approach or initiative but rather seeks to encourage multiple efforts. There is the expectation that results from analyses on the images and identifiable data will be returned and shared within the MC. This is to enable comparisons between different approaches using commonly agreed upon testing sets but also to keep the science as open and collaborative as possible. A schematic overview of the flow of data in the Minerva Initiative is shown in Figure 1.
Figure 1 Schematic overview of the Minerva Initiative structure and data flow into the Minerva Image Resource.
One of the purposes of the MC is to encourage and enable collaborative work on identifiable patient data. These collaborative initiatives are not centrally coordinated but are expected to be conducted through normal scientific practice. In addition, there is a framework for collective attribution through a publication policy.
Any data submitted that contribute to a publication using the pooled dataset will be acknowledged in accordance with the guidelines of the International Committee of Medical Journal Editors (“ICMJE | Recommendations | Defining the Role of Authors and Contributors”, n.d.). We envisage two broad groups of publications to arise from project data, each with distinct guidelines for recognizing contributions:
I. “Core” MC papers have a broad focus and use large amounts of data from multiple aspects of the project. Named authors (details below) plus “Minerva Consortium” with all project participants listed in the end matter [cf. Mells et al. WTCCC3 paper (Mells et al., 2011)]
II. “Affiliated” MC papers arising from more specific collaborations focused on a particular phenotype, methodology, policy question, etc. Named authors based on contribution and the Minerva Consortium as a “corporate” author [project participants not listed, cf. Firth, Wright & DDD Study paper (Firth et al., 2011)].
Manuscripts derived from research conducted on data acquired through the Minerva Initiative are reviewed by a Publication Review Group to affirm that author attribution follows the publication policy. The publication review group also ensures that all statements, and images used in Minerva Initiative publications comply with participant consents, image usage rights, data privacy, and data governance considerations.
While analyses produced by models are shared within the Minerva Initiative, the initiative does not require that the models themselves are shared. This is to ensure data protection and intellectual property rights. For many models being trained, there is a large gap in understanding of interpretability—to the degree that there is no guarantee that personally identifiable information from individuals in the training data might not be recoverable. Thus, to assure future compliance with the protection of individuals’ data rights within the MIR, the models must not be shared without corresponding data governance assurances. Secondly, as the Minerva Initiative allows the coexistence of both academic and commercial enterprise initiatives, the fair and equitable basis of IP domains must be clear.
In other words, in line with personality rights, ownership of the data and photographs remains with the original creators (the person in the image). The means to compare data between people, the models, is where we expect new intellectual property to be created.
It should also be noted that for most anticipated uses, models, in themselves, will contribute very little without access to the datasets. To successfully identify patients with rare disease and match them with others around the world, broad and unfettered interrogation of data is key. No model will have the accuracy or confidence to make assertions about rare diseases without reference back to the original patients and data. Consequently, it is in every stakeholder’s interest that the data are shared as openly and as widely as possible.
As a clinician or research academic seeking to collaborate on de-identified data or wishing to submit consented patient data to the MIR for analysis, the Minerva Initiative is open to join. The criteria are a formal affiliation and good standing with professional organizations in either a clinical or academic capacity. The Minerva Consortium relies on a peer system of oversight on membership, with the Management Group having power to rule in disputes.
In the case of a group seeking to become a Phenotyping Group, who can access identifiable data held in the MIR, this status should only be granted to groups who align with the research goals of the Minerva Initiative (to improve diagnosis, clinical treatment, and understanding of a wide range of illnesses). In addition, the Management Group will assess the stated purpose, ethics approvals, data management plans, and legal status of the applicant organization and proposed legal agreement for access to the MIR. Legal agreements will specify the parties’ obligations to adhere to the allowed uses and purposes for the data and the rights to change, revoke, or enforce these conditions (i.e., how access can be revoked and who is liable for possible misuses).
The Minerva Initiative is a framework for global collaboration on identifiable patient data including photographs. It is designed to complement current initiatives for global data sharing in rare diseases by specifically addressing the analysis of data where anonymization is not possible. The Minerva Initiative directly addresses the ethical, legal, and data security challenges associated with inherently identifiable data, thereby enabling big data research. We envisage that the frameworks established within the Minerva Initiative will provide a useful community model to ensure that the amount or variety of identifiable data that any one group, institution, or country can assemble is no longer the limiting factor for advancements in clinical translation of new machine learning methods. Through these approaches, we aim to improve diagnostic rate and classification of DNA variants with a focus on rare diseases. Accordingly, we envisage that this will assist in achieving the International Rare Diseases Consortium Vision, specifically to enable all people living with a rare disease to receive an accurate diagnosis, care, and available therapy within 1 year of coming to medical attention.
All authors contributed to the conception and design of the Minerva Initiative. CN drafted the manuscript. All authors contributed to manuscript revision and read and approved the submitted version.
This work was supported by MRC Fellowship MR/M014568/1 to CN and MRC Grant MR/M01326X/1 for MF. RFK and AVD are supported by grants from the ERA-NET NEURON through the Research Foundation – Flanders (FWO). EEE is supported by NIH 5R01MH101221 and as an investigator of Howard Hughes Medical Institute. CR acknowledges funding by the Italian Ministry of Health, Project RC2019 No. 2751604.
The authors declare that the research was conducted in the absence of any commercial or financial relationships that could be construed as a potential conflict of interest.
We would like to thank the patients and families who have participated on Minerva&Me and with all Minerva Initiative-associated studies. This work was supported by MRC Fellowship MR/M014568/1 to CN, MRC Grant MR/M01326X/1 for MF, and VRS supported by NIH 5UM1HG006542.
Adachi, T., Kawamura, K., Furusawa, Y., Nishizaki, Y., Imanishi, N., Umehara, S., et al. (2017). Japan’s Initiative on Rare and Undiagnosed Diseases (IRUD): towards an end to the diagnostic odyssey. Eur. J. Hum. Genet. 25, 1025. doi: 10.1038/ejhg.2017.106
Akle, S., Chun, S., Jordan, D. M., Cassa, C. A. (2015). Mitigating false-positive associations in rare disease gene discovery. Hum. Mutat. 36 (10), 998–1003. doi: 10.1002/humu.22847
Ansari, M., Poke, G., Ferry, Q., Williamson, K., Aldridge, R., Meynert, A. M., et al. (2014). Genetic heterogeneity in Cornelia de Lange syndrome (CdLS) and CdLS-like phenotypes with observed and predicted levels of mosaicism. J. Med. Genet. 51 (10), 659–668. doi: 10.1136/jmedgenet-2014-102573
Balliu, B., Würtz, R. P., Horsthemke, B., Wieczorek, D., Böhringer, S. (2014). Classification and visualization based on derived image features: application to genetic syndromes. PLoS One 9 (11), e109033. doi: 10.1371/journal.pone.0109033
Basel-Vanagaite, L., Wolf, L., Orin, M., Larizza, L., Gervasini, C., Krantz, I. D., et al. (2016). Recognition of the Cornelia de Lange syndrome phenotype with facial dysmorphology novel analysis. Clin. Genet. 89 (5), 557–563. doi: 10.1111/cge.12716
Baynam, G., Bauskis, A., Pachter, N., Schofield, L., Verhoef, H., Palmer, R. L., et al. (2017). 3-Dimensional facial analysis—facing precision public health. Front. Public Health 5, 31. doi: 10.3389/fpubh.2017.00031
Baynam, G., Pachter, N., McKenzie, F., Townshend, S., Slee, J., Kiraly-Borri, C., et al. (2016). The rare and undiagnosed diseases diagnostic service—application of massively parallel sequencing in a state-wide clinical service. Orphanet J. Rare Dis. 11 (1),1–7. doi: 10.1186/s13023-016-0462-7
Baynam, G. S., Walters, M., Dawkins, H., Bellgard, M., Halber, A. R., Claes, P. (2013). Objective monitoring of MTOR inhibitor therapy by three-dimensional facial analysis. Twin Res. Hum. Genet. 16 (04), 840–44. doi: 10.1017/thg.2013.49
Baynam, G., Walters, M., Claes, P., Kung, S., LeSouef, P., Dawkins, H., et al. (2013). The facial evolution: looking backward and moving forward. Hum. Mutat. 34 (1), 14–22. doi: 10.1002/humu.22219
Bengani, H., Handley, M., Alvi, M., Ibitoye, R., Lees, M., Lynch, S. A., et al. (2017). Clinical and molecular consequences of disease-associated de novo mutations in SATB2. Genet. Med. 19 (8), 900–908. doi: 10.1038/gim.2016.211
Bone, W. P., Washington, N. L., Buske, O. J., Adams, D. R., Davis, J., Draper, D., et al. (2016). Computational evaluation of exome sequence data using human and model organism phenotypes improves diagnostic efficiency. Genet. Med. 18 (6), 608–617. doi: 10.1038/gim.2015.137
Boycott, K. M., Rath, A., Chong, J. X., Hartley, T., Alkuraya, F. S., Baynam, G., et al. (2017). International cooperation to enable the diagnosis of all rare geneticd iseases. Am. J. Hum. Genet. 100 (5), 695–705. doi: 10.1016/j.ajhg.2017.04.003
Buske, O. J., Girdea, M., Dumitriu, S., Gallinger, B., Hartley, T., Trang, H., et al. (2015a). PhenomeCentral: a portal for phenotypic and genotypic matchmaking of patients with rare genetic diseases. Hum. Mutat. 36 (10), 931–940. doi: 10.1002/humu.22851
Buske, O. J., Schiettecatte, F., Hutton, B., Dumitriu, S., Misyura, A., Huang, L., et al. (2015b). The Matchmaker Exchange API: automating patient matching through the exchange of structured phenotypic and genotypic profiles. Hum. Mutat. 36 (10), 922–927. doi: 10.1002/humu.22850
Caulfield, M., Davies, J., Dennys, M., Elbahy, L., Fowler, T., Hill, S., et al. 2017. “The 100,000 Genomes Project Protocol.” https://doi.org/10.6084/m9.figshare.4530893.v2.
Chong, J. X., Buckingham, K. J., Jhangiani, S. N., Boehm, C., Sobreira, N., Smith, J. D., et al. (2015). The genetic basis of Mendelian phenotypes: discoveries, challenges, and opportunities. Am. J. Hum. Genet. 97 (2), 199–215. doi: 10.1016/j.ajhg.2015.06.009
Collins, F. S., Varmus, H. (2015). A new initiative on precision medicine. N. Engl. J. Med. 372 (9), 793–795. doi: 10.1056/NEJMp1500523
de Montjoye, Y.-A., Radaelli, L., Singh, V. K., Pentland, A. S. (2015). Unique in the shopping mall: on the reidentifiability of credit card metadata. Science 347 (6221), 536–539. doi: 10.1126/science.1256297
Deciphering Developmental Disorders Study (2017). Prevalence and architecture of de novo mutations in developmental disorders. Nature 542 (7642), 433–438. doi: 10.1038/nature21062
Dorschner, M. O., Amendola, L. M., Turner, E. H., Robertson, P. D., Shirts, B. H., Gallego, C. J., et al. (2013). Actionable, pathogenic incidental findings in 1,000 participants’ exomes. Am. J. Hum. Genet. 93 (4), 631–640. doi: 10.1016/j.ajhg.2013.08.006
Dudding-Byth, T., Baxter, A., Holliday, E. G., Hackett, A., O’Donnell, S., White, S. M., et al. (2017). Computer face-matching technology using two-dimensional photographs accurately matches the facial gestalt of unrelated individuals with the same syndromic form of intellectual disability. BMC Biotechnol. 17 (1), 90. doi: 10.1186/s12896-017-0410-1
Dyke, S. O. M., Knoppers, B. M., Hamosh, A., Firth, H. V., Hurles, M., Brudno, M., et al. (2017). Matching’ consent to purpose: the example of the matchmaker exchange. Hum. Mutat. 38 (10), 1281–1285. doi: 10.1002/humu.23278
Ferry, Q., Steinberg, J., Webber, C., FitzPatrick, D. R., Ponting, C. P., Zisserman, A., et al. (2014). Diagnostically relevant facial gestalt information from ordinary photos. ELife 3, e02020. doi: 10.7554/eLife.02020
Firth, H. V., Wright, C. F., and DDD Study (2011). The Deciphering Developmental Disorders (DDD) Study. Dev. Med. Child Neurol. 53 (8), 702–703. doi: 10.1111/j.1469-8749.2011.04032.x
Galton, F. (1879). Composite portraits, made by combining those of many different persons into a single resultant figure. J. Anthropol. Inst. G. B. Irel 8, 132. doi: 10.2307/2841021
Gardner, O. K., Haynes, K., Schweitzer, D., Johns, A., Magee, W. P., Urata, M. M., et al. (2017). Familial recurrence of 3MC syndrome in consanguineous families: a clinical and molecular diagnostic approach with review of the literature. Cleft Palate Craniofac. J. 54 (6), 739–748. doi: 10.1597/15-151
“Global Alliance for Genomics and Health” (n.d.) Accessed September 6, 2018. https://www.ga4gh.org/.
Gripp, K. W., Baker, L., Telegrafi, A., Monaghan, K. G. (2016). The role of objective facial analysis using FDNA in making diagnoses following whole exome analysis. Report of two patients with mutations in the BAF complex genes. Am. J. Med. Genet. A 170 (7), 1754–1762. doi: 10.1002/ajmg.a.37672
Gurovich, Y., Hanani, Y., Bar, O., Fleischer, N., Gelbman, D., Basel-Salmon, L., et al. (2018). DeepGestalt—identifying rare genetic syndromes using deep learning. Nat. Med. doi: 10.1038/s41591-018-0279-0
Gymrek, M., McGuire, A. L., Golan, D., Halperin, E., Erlich, Y. (2013). Identifying personal genomes by surname inference. Science (New York, N.Y). 339 (6117), 321–24. doi: 10.1126/science.1229566
Hadj-Rabia, S., Schneider, H., Navarro, E., Klein, O., Kirby, N., Huttner, K., et al. (2017). Automatic recognition of the XLHED phenotype from facial images. Am. J. Med. Genet. A 173 (9), 2408–2414. doi: 10.1002/ajmg.a.38343
Hammond, P., Hutton, T. J., Allanson, J. E., Buxton, B., Campbell, L. E., Clayton-Smith, J., et al. (2005). Discriminating power of localized three-dimensional facial morphology. Am. J. Hum. Genet. 77 (6), 999–1010. doi: 10.1086/498396
Hammond, P., Hutton, T. J., Allanson, J. E., Campbell, L. E., Hennekam, R. C. M., Holden, S., et al. (2004). 3D analysis of facial morphology. Am. J. Med. Genet. A 126A (4), 339–348. doi: 10.1002/ajmg.a.20665
Hammond, P., Suttie, M., Hennekam, R. C., Allanson, J., Shore, E. M., Kaplan, F. S. (2012). The face signature of fibrodysplasia ossificans progressiva. Am. J. Med. Genet. A 158A (6), 1368–1380. doi: 10.1002/ajmg.a.35346
Hamosh, A., Sobreira, N., Hoover-Fong, J., Sutton, V. R., Boehm, C., Schiettecatte, F., et al. (2013). PhenoDB: a new web-based tool for the collection, storage, and analysis of phenotypic features. Hum. Mutat. 34 (4), 566–571. doi: 10.1002/humu.22283
Hennessy, R. J., Baldwin, P. A., Browne, D. J., Kinsella, A., Waddington, J. L. (2010). Frontonasal dysmorphology in bipolar disorder by 3D laser surface imaging and geometric morphometrics: comparisons with schizophrenia. Schizophr. Res. 122 (1–3), 63–71. doi: 10.1016/j.schres.2010.05.001
Herpers, R., Rodax, H., Sommer, G. (1993). A neural network identifies faces with morphological syndromes. Stud. Health Technol. Inform., 481–485.
“Human Disease Genes” (n.d.) Human disease genes. Accessed September 5, 2018. http://humandiseasegenes.nl/.
“ICMJE | Recommendations | Defining the Role of Authors and Contributors” (n.d.) Accessed July 24, 2018. http://www.icmje.org/recommendations/browse/roles-and-responsibilities/defining-the-role-of-authors-and-contributors.html.
“IRDiRC” (n.d.) IRDiRC. Accessed September 6, 2018. http://www.irdirc.org/.
Javed, A., Agrawal, S., Ng, P. C. (2014). Phen-Gen: combining phenotype and genotype to analyze rare disorders. Nat. Methods 11 (9), 935–937. doi: 10.1038/nmeth.3046
Kaye, J., Whitley, E. A., Lund, D., Morrison, M., Teare, H., Melham, K. (2015). Dynamic consent: a patient interface for twenty-first century research networks. Eur. J. Hum. Genet. 23 (2), 141–146. doi: 10.1038/ejhg.2014.71
Knaus, A., Pantel, J. T., Pendziwiat, M., Hajjir, N., Zhao, M., Hsieh, T.-C., et al. (2018). Characterization of glycosylphosphatidylinositol biosynthesis defects by clinical features, flow cytometry, and automated image analysis. Genome Med. 10 (1), 3. doi: 10.1186/s13073-017-0510-5
Köhler, S., Vasilevsky, N. A., Engelstad, M., Foster, E., McMurry, J., Aymé, S., et al. (2017). The human phenotype ontology in 2017. Nucleic Acids Res. 45 (D1), D865–D876. doi: 10.1093/nar/gkw1039
Krawitz, P., Buske, O., Zhu, N., Brudno, M., Robinson, P. N. (2015). The genomic birthday paradox: how much is enough? Hum. Mutat. 36 (10), 989–997. doi: 10.1002/humu.22848
Kruszka, P., Addissie, Y. A., McGinn, D. E., Porras, A. R., Biggs, E., Share, M., et al. (2017a). 22q11.2 deletion syndrome in diverse populations. Am. J. Med. Genet. A 173 (4), 879–888. doi: 10.1002/ajmg.a.38199
Kruszka, P., Porras, A. R., Addissie, Y. A., Moresco, A., Medrano, S., Mok, G. T. K., et al. (2017b). Noonan syndrome in diverse populations. Am. J. Med. Genet. A 173 (9), 2323–2334. doi: 10.1002/ajmg.a.38362
Kruszka, P., Porras, A. R., Sobering, A. K., Ikolo, F. A., Qua, S. L., Shotelersuk, V., et al. (2017c). Down syndrome in diverse populations. Am. J. Med. Genet. A 173 (1), 42–53. doi: 10.1002/ajmg.a.38043
Kruszka, P., Porras, A. R., de Souza, D. H., Moresco, A., Huckstadt, V., Gill, A. D., et al. (2018). Williams–Beuren syndrome in diverse populations. Am. J. Med. Genet. A 176 (5), 1128–1136. doi: 10.1002/ajmg.a.38672
Kung, S., Walters, M., Claes, P., LeSouef, P., Goldblatt, J., Martin, A., et al., (2015). “Monitoring of therapy for mucopolysaccharidosis type I using dysmorphometric facial phenotypic signatures.” In JIMD Reports, vol. 22. Eds. Zschocke, J., Baumgartner, M., Morava, E., Patterson, M., Rahman, S., Peters, V. (Berlin, Heidelberg: Springer Berlin Heidelberg), 99–106. doi: 10.1007/8904_2015_417
Landrum, M. J., Lee, J. M., Benson, M., Brown, G., Chao, C., Chitipiralla, S., et al. (2016). ClinVar: public archive of interpretations of clinically relevant variants. Nucleic Acids Res. 44 (D1), D862–D868. doi: 10.1093/nar/gkv1222
Liehr, T., Acquarola, N., Pyle, K., St-Pierre, S., Rinholm, M., Bar, O., et al. (2018). Next generation phenotyping in Emanuel and Pallister–Killian syndrome using computer-aided facial dysmorphology analysis of 2D photos. Clin. Genet. 93 (2), 378–381. doi: 10.1111/cge.13087
Loos, H. S., Wieczorek, D., Würtz, R. P., von der Malsburg, C., Horsthemke, B. (2003). Computer-based recognition of dysmorphic faces. Eur. J. Hum. Genet. 11 (8), 555–560. doi: 10.1038/sj.ejhg.5200997
Lumaka, A., Cosemans, N., Lulebo Mampasi, A., Mubungu, G., Mvuama, N., Lubala, T., et al. (2017). Facial dysmorphism is influenced by ethnic background of the patient and of the evaluator. Clin. Genet. 92 (2), 166–171. doi: 10.1111/cge.12948
MacArthur, D. G., Tyler-Smith, C. (2010). Loss-of-function variants in the genomes of healthy humans. Hum. Mol. Genet. 19 (R2), R125–R130. doi: 10.1093/hmg/ddq365
Manousaki, D., Allanson, J., Wolf, L., Deal, C. (2015). Characterization of facial phenotypes of children with congenital hypopituitarism and their parents: a matched case–control study. Am. J. Med. Genet. A 167 (7), 1525–1533. doi: 10.1002/ajmg.a.37069
Mells, G. F., Floyd, J. A. B., Morley, K. I., Cordell, H. J., Franklin, C. S., Shin, S.-Y., et al. (2011). Genome-wide association study identifies 12 new susceptibility loci for primary biliary cirrhosis. Nat. Genet. 43 (4), 329–332. doi: 10.1038/ng.789
“Minerva and Me—Help Rare Disease Research” (n.d.) Accessed July 31, 2018. https://www.minervaandme.com/.
“MyGene2—Home” (n.d.) Accessed August 17, 2018. https://mygene2.org/MyGene2/.
“National Institutes of Health (NIH)—All of us” (n.d.) Accessed July 24, 2018. https://allofus.nih.gov/.
Nielsen, M. A. (2012). Reinventing discovery: The new era of networked science. Princeton, N.J: Princeton University Press. doi: 10.2307/j.ctt7s4vx
Pantel, J. T., Zhao, M., Mensah, M. A., Hajjir, N., Hsieh, T.-C., Hanani, Y., et al. (2018). Advances in computer-assisted syndrome recognition by the example of inborn errors of metabolism. J. Inherit. Metab. Dis. 41 (3), 533–539. doi: 10.1007/s10545-018-0174-3
“Patient Archive” (n.d.) Accessed August 17, 2018. http://patientarchive.org/#/home.
Pena, L. D. M., Jiang, Y.-H., Schoch, K., Spillmann, R. C., Walley, N., Stong, N., et al. (2018). Looking beyond the exome: a phenotype-first approach to molecular diagnostic resolution in rare and undiagnosed diseases. Genet. Med. 20 (4), 464–469. doi: 10.1038/gim.2017.128
Pengelly, R. J., Alom, T., Zhang, Z., Hunt, D., Ennis, S., Collins, A. (2017). Evaluating phenotype-driven approaches for genetic diagnoses from exomes in a clinical setting. Sci. Rep. 7 (1), 13509. doi: 10.1038/s41598-017-13841-y
Philippakis, A. A., Azzariti, D. R., Beltran, S., Brookes, A. J., Brownstein, C. A., Brudno, M., et al. (2015). The matchmaker exchange: a platform for rare disease gene discovery. Hum. Mutat. 36 (10), 915–921. doi: 10.1002/humu.22858
“Platform for Engaging Everyone Responsibly | GeneticAlliance.Org” (n.d.) Accessed July 24, 2018. http://www.geneticalliance.org/programs/biotrust/peer/.
“Precision Public Health: What Is It? | | Blogs | CDC” (n.d.) Accessed August 20, 2018. https://blogs.cdc.gov/genomics/2018/05/15/precision-public-health-2/.
Rehm, H. L., Berg, J. S., Brooks, L. D., Bustamante, C. D., Evans, J. P., Landrum, M. J., et al. (2015). ClinGen—the clinical genome resource. N. Engl. J. Med. 372 (23), 2235–2242. doi: 10.1056/NEJMsr1406261
Reijnders, M. R. F., Janowski, R., Alvi, M., Self, J. E., van Essen, T. J., Vreeburg, M., et al. (2018a). PURA syndrome: clinical delineation and genotype–phenotype study in 32 individuals with review of published literature. J. Med. Genet. 55 (2), 104–113. doi: 10.1136/jmedgenet-2017-104946
Reijnders, M. R. F., Miller, K. A., Alvi, M., Goos, J. A. C., Lees, M. M., de Burca, A., et al. (2018b). De novo and inherited loss-of-function variants in TLK2: clinical and genotype–phenotype evaluation of a distinct neurodevelopmental disorder. Am. J. Hum. Genet. 102 (6), 1195–1203. doi: 10.1016/j.ajhg.2018.04.014
Robinson, P. N., Köhler, S., Oellrich, A., Sanger Mouse Genetics Project, Wang, K., Mungall, C. J., et al. (2014). Improved exome prioritization of disease genes through cross-species phenotype comparison. Genome Res. 24 (2), 340–348. doi: 10.1101/gr.160325.113
Robinson, P. N., Mungall, C. J., Haendel, M. (2015). Capturing phenotypes for precision medicine. Cold Spring Harb. Mol. Case Stud. 1 (1), a000372. doi: 10.1101/mcs.a000372
Sheldon, W. H., Tucker, W. B., Stevens, S. S. (1945). The varieties of human physique; an introduction to constitutional psychology. New York London: Harper & brothers. https://catalog.hathitrust.org/Record/002001289
Shukla, P., Gupta, T., Saini, A., Singh, P., Balasubramanian, R. (2017). A deep learning frame-work for recognizing developmental disorders, in 2017 IEEE Winter Conference on Applications of Computer Vision (WACV) (Santa Rosa, CA, USA: IEEE), 705–714. doi: 10.1109/WACV.2017.84
Sifrim, A., Popovic, D., Tranchevent, L.-C., Ardeshirdavani, A., Sakai, R., Konings, P., Vermeesch, J. R., et al. (2013). EXtasy: variant prioritization by genomic data fusion. Nat. Methods 10 (11), 1083–1084. doi: 10.1038/nmeth.2656
Singleton, M. V., Guthery, S. L., Voelkerding, K. V., Chen, K., Kennedy, B., Margraf, R. L., et al. (2014). Phevor combines multiple biomedical ontologies for accurate identification of disease-causing alleles in single individuals and small nuclear families. Am. J. Hum. Genet. 94 (4), 599–610. doi: 10.1016/j.ajhg.2014.03.010
Smith, D. W. (1966). Dysmorphology (teratology). J. Pediatr. 69 (6), 1150–1169. doi: 10.1016/S0022-3476(66)80311-6
Sobreira, N., Schiettecatte, F., Valle, D., Hamosh, A. (2015). GeneMatcher: a matching tool for connecting investigators with an interest in the same gene. Hum. Mutat. 36 (10), 928–930. doi: 10.1002/humu.22844
Taruscio, D., Groft, S. C., Cederroth, H., Melegh, B., Lasko, P., Kosaki, K., et al. (2015). Undiagnosed Diseases Network International (UDNI): white paper for global actions to meet patient needs. Mol. Genet. Metab. 116 (4), 223–225. doi: 10.1016/j.ymgme.2015.11.003
“UDNI” (n.d.) Accessed August 16, 2018. http://www.udninternational.org/.
Valentine, M., Bihm, D. C. J., Wolf, L., Eugene Hoyme, H., May, P. A., Buckley, D., et al. (2017). Computer-aided recognition of facial attributes for fetal alcohol spectrum disorders. Pediatrics 140 (6), 1–8. doi: 10.1542/peds.2016-2028
Vollmar, T., Maus, B., Wurtz, R. P., Gillessen-Kaesbach, G., Horsthemke, B., Wieczorek, D., et al. (2008). Impact of geometry and viewing angle on classification accuracy of 2D based analysis of dysmorphic faces. Eur. J. Med. Genet. 51 (1), 44–53. doi: 10.1016/j.ejmg.2007.10.002
Weeramanthri, T. S., Dawkins, H. J. S., Baynam, G., Bellgard, M., Gudes, O., Semmens, J. B. (2018). Editorial: precision public health. Front. Public Health 6, 1–3. doi: 10.3389/fpubh.2018.00121
Woelfle, M., Olliaro, P., Todd, M. H. (2011). Open science is a research accelerator. Nat. Chem. 3 (10), 745–748. doi: 10.1038/nchem.1149
Wright, C. F., FitzPatrick, D. R., Firth, H. V. (2018). Paediatric genomics: diagnosing rare disease in children. Nat. Rev. Genet 19, 325. doi: 10.1038/nrg.2018.12
Xiangyu Zhu, Z. L., Yan, J., Yi, D., Li, S. Z. (2015). High-fidelity pose and expression normalization for face recognition in the wild. IEEE, Piscataway, New Jersey, US, 787–796. doi: 10.1109/CVPR.2015.7298679
Keywords: data sharing, phenotyping, patient information, data protection, rare disease, Faces
Citation: Nellåker C, Alkuraya FS, Baynam G, Bernier RA, Bernier FPJ, Boulanger V, Brudno M, Brunner HG, Clayton-Smith J, Cogné B, Dawkins HJS, deVries BBA, Douzgou S, Dudding-Byth T, Eichler EE, Ferlaino M, Fieggen K, Firth HV, FitzPatrick DR, Gration D, Groza T, Haendel M, Hallowell N, Hamosh A, Hehir-Kwa J, Hitz M-P, Hughes M, Kini U, Kleefstra T, Kooy RF, Krawitz P, Küry S, Lees M, Lyon GJ, Lyonnet S, Marcadier JL, Meyn S, Moslerová V, Politei JM, Poulton CC, Raymond FL, Reijnders MRF, Robinson PN, Romano C, Rose CM, Sainsbury DCG, Schofield L, Sutton VR, Turnovec M, Van Dijck A, Van Esch H, Wilkie AOM and The Minerva Consortium (2019) Enabling Global Clinical Collaborations on Identifiable Patient Data: The Minerva Initiative. Front. Genet. 10:611. doi: 10.3389/fgene.2019.00611
Received: 21 September 2018; Accepted: 12 June 2019;
Published: 29 July 2019.
Edited by:
Dov Greenbaum, Yale University, United StatesReviewed by:
Chih-hsing Ho, Academia Sinica, TaiwanCopyright © 2019 Nellåker, Alkuraya, Baynam, Bernier, Bernier, Boulanger, Brudno, Brunner, Clayton-Smith, Cogné, Dawkins, deVries, Douzgou, Dudding-Byth, Eichler, Ferlaino, Fieggen, Firth, FitzPatrick, Gration, Groza, Haendel, Hallowell, Hamosh, Hehir-Kwa, Hitz, Hughes, Kini, Kleefstra, Kooy, Krawitz, Küry, Lees, Lyon, Lyonnet, Marcadier, Meyn, Moslerová, Politei, Poulton, Raymond, Reijnders, Robinson, Romano, Rose, Sainsbury, Schofield, Sutton, Turnovec, Van Dijck, Van Esch,Wilkie and The Minerva Consortium. This is an open-access article distributed under the terms of the Creative Commons Attribution License (CC BY). The use, distribution or reproduction in other forums is permitted, provided the original author(s) and the copyright owner(s) are credited and that the original publication in this journal is cited, in accordance with accepted academic practice. No use, distribution or reproduction is permitted which does not comply with these terms.
*Correspondence: Christoffer Nellåker, Y2hyaXN0b2ZmZXIubmVsbGFrZXJAd3JoLm94LmFjLnVr
†Minerva Consortium Management Group
‡Consortium Lead
Disclaimer: All claims expressed in this article are solely those of the authors and do not necessarily represent those of their affiliated organizations, or those of the publisher, the editors and the reviewers. Any product that may be evaluated in this article or claim that may be made by its manufacturer is not guaranteed or endorsed by the publisher.
Research integrity at Frontiers
Learn more about the work of our research integrity team to safeguard the quality of each article we publish.