- 1Department of Cell Biology, 2011 Collaborative Innovation Center of Tianjin for Medical Epigenetics, Tianjin Key Laboratory of Medical Epigenetics, Tianjin Medical University, Tianjin, China
- 2Center for Applied Genomics, Children’s Hospital of Philadelphia, Philadelphia, PA, United States
- 3Division of Human Genetics, Children’s Hospital of Philadelphia, Philadelphia, PA, United States
- 4Department of Pediatrics, Perelman School of Medicine, University of Pennsylvania, Philadelphia, PA, United States
Juvenile idiopathic arthritis (JIA) is the most common chronic rheumatic disease among children which could cause severe disability. Genomic studies have discovered substantial number of risk loci for JIA, however, the mechanism of how these loci affect JIA development is not fully understood. Neutrophil is an important cell type involved in autoimmune diseases. To better understand the biological function of genetic loci in neutrophils during JIA development, we took an integrated multi-omics approach to identify target genes at JIA risk loci in neutrophils and constructed a protein-protein interaction network via a machine learning approach. We identified genes likely to be JIA risk loci targeted genes in neutrophils which could contribute to JIA development.
Introduction
Juvenile idiopathic arthritis (JIA) is the most common chronic rheumatic disease in childhood at a prevalence rate of 1 in 1000, and JIA is a common cause of disability among children (Oen and Cheang, 1996). The typical clinical manifestation of JIA is joint enlargement of unknown origin for more than 6 weeks in children under 16 years old (Petty et al., 2004). JIA has long been considered as a type of autoimmune disease, however, its etiology is still not fully understood. Similar to other complex diseases, genetic and environmental factors both contribute to its pathogenesis (Glass and Giannini, 1999). Substantial evidence suggests the large contribution of genetic components. Previous studies showed that monozygotic twin concordance rates for JIA are between 25 and 40%, much higher than the population prevalence rate (Savolainen et al., 2000). Affected sibling studies showed that siblings of JIA probands had an over 10-fold increased risk of developing the disease (Frisell et al., 2016). Our recent heritability study based on SNP-h2 estimated that the heritability of JIA is 0.73 among the most highly heritable pediatric autoimmune diseases (Li et al., 2015b). Several genome-wide association studies (GWAS) have been carried out and discovered a number of JIA susceptibility loci, but how these loci affect the pathogenesis and development of JIA remains to be explored (Behrens et al., 2008; Hinks et al., 2009, 2013; Thompson et al., 2012; Cobb et al., 2014; Aydin-Son et al., 2015; Li et al., 2015a; Finkel et al., 2016; Ombrello et al., 2017; Haasnoot et al., 2018).
Neutrophils are one of the most important innate immune cells in human bodies. When infection or inflammation occurs, they are recruited to the disease site under the attraction of chemokines. In recent years, studies have found that neutrophils can secrete a variety of cytokines to play a key role in immunomodulation. The clinical manifestations of JIA are highly similar to those of classical autoinflammatory diseases. The large accumulation of white blood cells is one of the causes for local tissue damage and loss of joint function due to the inflammatory response at the joint (Fattori et al., 2016). Neutrophils likely play an important role in the effector phase of autoimmune diseases including JIA, and their action can cause or exacerbate articular inflammation (Németh and Mócsai, 2012). Neutrophil extracellular traps (NETs) are the newly discovered mechanism by which neutrophils fight infection, and has been demonstrated to play a role in pathogenesis of systemic immune diseases such as systemic lupus erythematosus (SLE) (Hakkim et al., 2010), antineutrophil cytoplasmic antibodies (ANCA)-associated systemic vasculitis (Kessenbrock et al., 2009) and multiple sclerosis (Naegele et al., 2012). However, little is known about the genes involved in JIA development in neutrophils.
A number of JIA loci have been identified in GWAS (Behrens et al., 2008; Hinks et al., 2009, 2013; Thompson et al., 2012; Cobb et al., 2014; Aydin-Son et al., 2015; Li et al., 2015a; Finkel et al., 2016; Ombrello et al., 2017; Haasnoot et al., 2018), but few have been functionally characterized as most of the GWAS SNPs are located at the intronic or intergenic regions, without directly affecting the sequence of any protein product. We hypothesize that they may function as cis-regulatory elements, regulating target gene expression. Therefore, we focused on understanding the target genes of JIA GWAS loci in neutrophils.
JIA is a heterogeneous group of diseases including several different subtypes. In recent years, due to the progress in disease management, their prognosis has been greatly improved, but there are still few effective treatments. Our study took an in silico analysis approach, utilizing genomics, transcriptome, epigenome, and methylome data to find genes targeted by JIA risk loci in neutrophils, facilitating the design of precision strategy of JIA prevention and treatment.
Materials and Methods
Extraction of JIA GWAS Loci
Juvenile idiopathic arthritis loci identified in previous GWAS were found in GWAS catalog (MacArthur et al., 2017) by conducting search using keyword “Juvenile idiopathic arthritis.” All loci found were downloaded without further imposing any significance threshold.
eQTL Analysis
eQTL analysis was performed via Genotype-Tissue Expression (GTEx) Project website (Lonsdale et al., 2013), from which the correlation between each SNP genotype and gene expression level in whole blood was extracted. We set the significance threshold as P-value < 0.05. The boxplots for the SNP-gene pairs were reviewed via GTEx Portal.
Analysis of Microarray Data
Series matrix files of microarray datasets GSE11083 (Frank et al., 2009a,b) and GSE67596 (Jiang et al., 2015) containing transcriptome data from neutrophils of 36 JIA patients and 26 healthy controls were downloaded from NCBI Gene Expression Omnibus (GEO) (Edgar et al., 2002; Barrett et al., 2013). Gene expression levels were compared between JIA patient group and control group. Expression values across studies was summarized through median polish and normalization was performed using Robust Multi-array Average (RMA) algorithm which minimizes variance across arrays and log transformation was conducted for variance stabilization (Irizarry et al., 2003). Meta-analysis were performed using RankProd package (Hong et al., 2006) in R 3.5.1 (R Core Team, 2018). The threshold used to select for differentially expressed genes was defined as possibility of false positives (PFP) < 0.05 and absolute value of fold change (FC) > 1.2.
Histone Modification Analysis
The SNPs of interest were input into web portal Haploreg1 (Ward and Kellis, 2012) and their overlap with histone modification regions in neutrophil cell line E030 BLD.CD15.PC (primary neutrophils from peripheral blood) was evaluated using epigenome data from ROADMAP epigenomics database (Kundaje et al., 2015).
Methylation Data Analysis
The methylation data were extracted from the genome-wide methylation profiles of 843 subjects processed on the Infinium HumanMethylation450 BeadChip at the Center for Applied Genomics, the Children’s Hospital of Philadelphia, which has been described in previous publication (Van Ingen et al., 2016). The log2 ratio between the methylated and unmethylated intensities of each probe on the chip was represented by the M-values. The association between JIA SNP genotype and methylation probes in each of the 11 genes was assessed in a linear regression model conditioned on gender, age and 10 genotype-derived principle components.
Construction of Protein-Protein Interaction (PPI) Network
Protein-Protein Interaction network on the 11 target genes was constructed via NetworkAnalyst2 (Xia et al., 2014) which was based on integration of machine learning and Walktrap algorithms (Pons and Latapy, 2005). The resource of protein-protein interaction data was IMEx Interactome database (Orchard et al., 2012). Hypergeometric test for gene set enrichment analysis was implemented in NetworkAnalyst and the Kyoto Encyclopedia of Genes and Genomes (KEGG) database (Kanehisa et al., 2017) was used as the pathway database resource. In addition to FDR P-value calculated based on hypergeometric test and multiple-testing adjustment, empirical P-value of pathways was derived from permutation analysis. A list of 11 genes was randomly generated from the human genome and such resampling was performed 100 times. For each 11-gene list randomly drawn, the steps of network construction, pathway analysis were similarly performed as for JIA target genes in neutrophils, and a list of significantly enriched pathways with FDR < 0.05 was resulted from each resampling. For each enriched pathway in PPI network of JIA target genes, its empirical P-value was derived based on the number of times it appears as significantly enriched pathway from 100 permutations.
Hi-C Data Visualization
Hi-C data visualization for the JIA loci and target genes were carried out via the 3D Genome browser3 (Wang et al., 2018) and FUMA GWAS4 (Watanabe et al., 2017). Hi-C data from cell line K562 (Rao et al., 2014; Schmitt et al., 2016) were used.
Results
A large number of GWAS loci have been identified for human complex diseases, including JIA. We extracted all 127 genomic regions that have been reported to be associated with JIA from GWAS catalog (Behrens et al., 2008; Hinks et al., 2009, 2013; Thompson et al., 2012; Cobb et al., 2014; Aydin-Son et al., 2015; Li et al., 2015a; Finkel et al., 2016; MacArthur et al., 2017; Ombrello et al., 2017; Haasnoot et al., 2018). All these SNPs are located outside of gene exons, which may contribute to disease etiology by affecting gene expression. We then input these SNPs into GTEx database (Lonsdale et al., 2013) to identify genes that are regulated by these SNPs. Because GWAS SNPs and their target genes may not always exhibit highly significant correlation in eQTL analysis, exemplified by obesity SNP rs9930506 and IRX3 gene (Smemo et al., 2014), we set the significance threshold as nominal P-value < 0.05. We found that the expression level of 238 genes correlates with JIA SNP genotype in whole blood.
As we are particularly interested in identifying genes regulated by JIA GWAS loci in neutrophils, we examined which of these 238 genes showed differential expression in neutrophils between JIA cases and controls. We extracted two microarray datasets from gene expression omnibus (GEO) database, GSE11083 (Frank et al., 2009a,b) and GSE67596 (Jiang et al., 2015). Gene expression data from a total of 36 JIA cases and 26 controls were meta-analyzed. Among the 264 eQTL genes for JIA SNPs, only 11 genes showed significant differential expression, including 5 up-regulated and 6 down-regulated (Table 1). Our in silico analysis suggested that these genes may function as JIA loci targeted genes in neutrophils. Among the 13 pairs of JIA SNPs and target genes, only SNP rs79893749 is located in the intron of its target gene CCR3; all the other SNPs are located outside of the transcript region of their target genes. Hi-C data provide additional supporting evidence for plausible chromatin interactions between some JIA SNPs and their target genes (Supplementary Figures 1, 2), with the caveat that these data came from a chronic myelogenous leukemia cell line K562 (Rao et al., 2014; Schmitt et al., 2016). Experiments using neutrophils would be necessary to further explore their possible interactions.
To understand how these genes coordinately contribute to JIA development, we constructed PPI network among proteins encoded by these genes and their direct interactors (Figure 1) using NetworkAnalyst which integrates statistical analyses and machine learning for interactive PPI network visualization. We further conducted pathway analysis and found several signaling pathways significantly enriched among proteins in this network, including neurotrophin signaling pathway, cardiac muscle contraction, cell cycle and hypertrophic cardiomyopathy (HCM) (Table 2). To test the cell type specificity of 11 target genes and enriched pathways, we repeated the whole process using microarray gene expression data from PBMC samples of the same GEO datasets. We found that among the 11 target genes in neutrophils, one gene (TRIM58) was shared with PBMC (Table 1 and Supplementary Table 1). No enriched pathway was shared between neutrophils and PBMC (Figure 1, Table 2, Supplementary Figure 3, and Supplementary Table 2). To further determine the specificity of the 4 enriched pathways among JIA target genes in neutrophils, we checked the distribution of significantly enriched pathways from 100 randomly generated 11-gene lists and derived the empirical P-values for each of the 4 pathways of interest (Table 2). The pathways of cardiac muscle contraction and hypertrophic cardiomyopathy were of empirical P-value < 0.01. Based on these two control gene set analyses, we demonstrated the specificity of these target genes and pathways in neutrophils, serving the initial screening purpose for further functional validation.
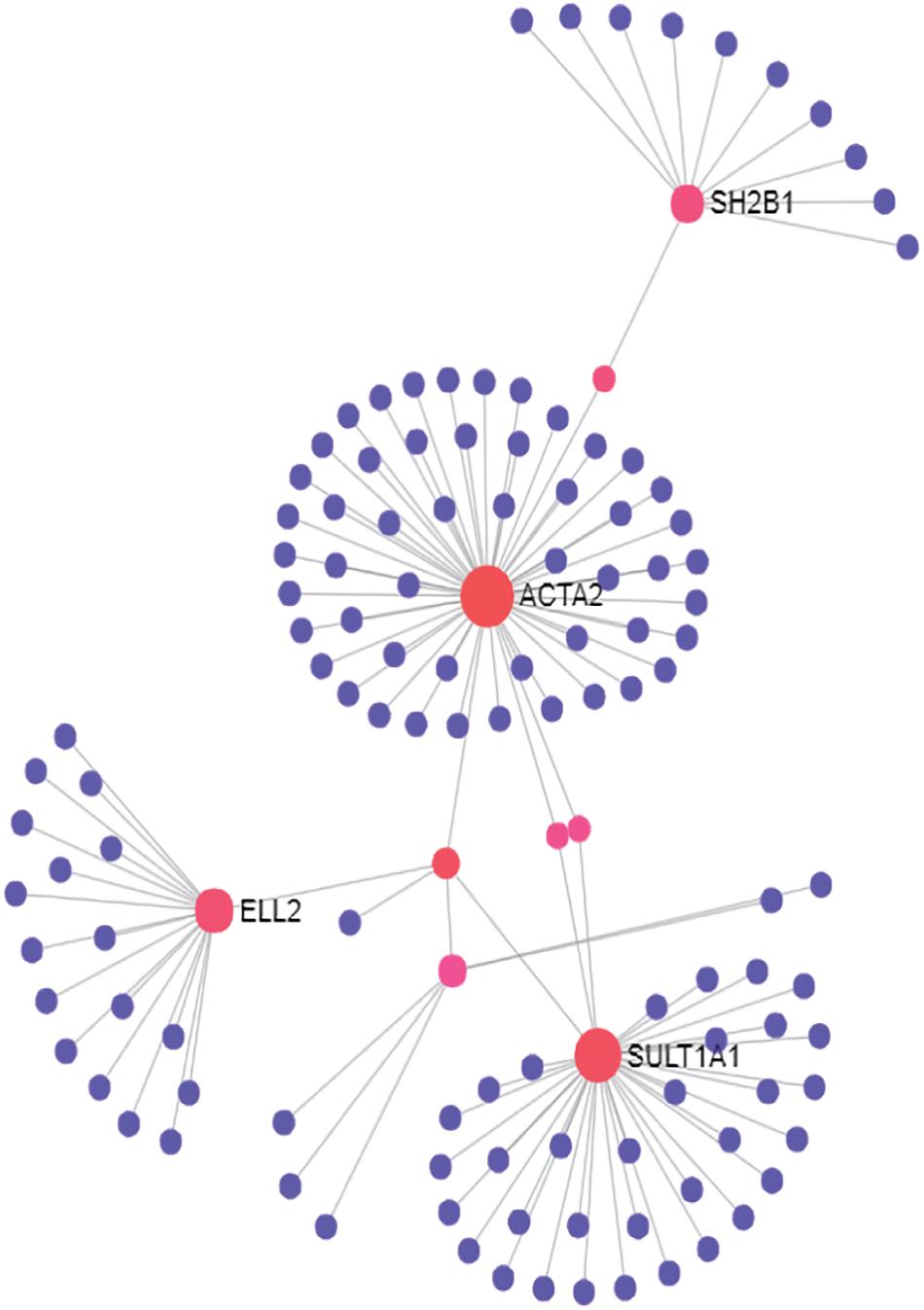
Figure 1. PPI network of first-order interactions constructed for JIA loci target genes in neutrophils.

Table 2. KEGG pathways enriched among the PPI network formed by JIA loci target gene and their interactors (FDR < 0.05).
Next, we investigated how JIA loci may regulate the expression of their targeted genes. To address this question, we examined the ROADMAP database (Kundaje et al., 2015) through HaploReg (Ward and Kellis, 2012). We found rs79893749 and rs149850873 overlap with histone marks in a neutrophil cell line E030 BLD.CD15.PC, suggesting that these loci may regulate their targeted gene expression through histone modifications in the promoter or enhancer region. We also looked into the potential mechanism of DNA methylation. In our methylation analysis, we tested the 10 JIA SNPs against their corresponding one or two genes which each contains ∼11 methylation probes on average. A total of 144 SNP-methylation-probe pairs were tested, thus the multiple-testing adjusted P-value cutoff is set at 3.5 × 10-4. The correlation between four SNP-methylation-probe pairs reached this experiment-wide significance threshold, suggesting that these JIA SNPs may regulate the expression of their target genes through DNA methylation (Table 1).
Discussion
In this study, we conducted data mining in existing datasets to gain a better understanding of the molecular mechanism of JIA GWAS loci. By eQTL and transcriptome analyses, we identified 11 genes may be JIA loci target genes in neutrophils. We further built PPI network and found pathways enriched among target genes and their interactors. We also found multiple JIA GWAS SNPs overlap with histone marks and/or correlate with methylation level in their target genes.
We did not observe extensive overlap between JIA eQTL genes in whole blood and genes of differential expression in neutrophils. It possibly resulted from the small sample size in our microarray datasets which did not have enough power to detect certain differentially expressed genes. In addition, JIA eQTL genes may be expressed in cell types other than neutrophils which we are particularly interested in.
Several of the target genes we identified are highly related to the immune system, such as CCR3, ELL2, and HLA-DPA1. Others play a role in cell proliferation, carcinogenesis and/or other biological functions. The Human leukocyte antigen (HLA) gene complex encodes human major histocompatibility complex (MHC), a group of cell-surface proteins playing important roles in the regulation of human immune system. HLA genes have been reported to be associated with autoimmune diseases (Sollid, 2017; Kawabata et al., 2018), including rheumatoid arthritis (Onuora, 2015; Smolen et al., 2018) and JIA (Smerdel et al., 2002). The HLA-DPA1 locus has been particularly linked to ankylosing spondylitis, a type of chronic inflammatory rheumatic disease (Diaz-Pena et al., 2011). As expected, HLA genes were also found as target genes in PBMC, suggesting they contribute to pathogenesis of JIA in diverse immune cell types. CCR3 gene encodes a protein as a member of the G protein-coupled receptor family, responding to the C-C type chemokines. SNP in CCR3-CCR5 region has been linked to family history of autoimmune disease among children with type I diabetes (Parkkola et al., 2017). It has been reported that CCR3 expression was increased under rheumatoid arthritis conditions, and it mediated eotaxin-1 induced matrix metalloproteinase (MMP)-9 upregulation in fibroblast-like synoviocyte which may further result in articular damage (Liu et al., 2017). Previous studies have also demonstrated that CCR3 is highly expressed in infiltrated synovial neutrophils of rheumatoid arthritis patients (Hartl et al., 2008). ELL2 gene encodes Elongation Factor for RNA Polymerase II 2, a component of the super-elongation complex. It functions in immune regulation by affecting IgH alternative processing, Ig secretion and plasma cell differentiation. Missense mutation in ELL2 gene affects IgA and IgG level associated with multiple myeloma (Swaminathan et al., 2015). Study has shown that ELL2 is expressed in mature neutrophils and its expression is elevated in responses to inflammatory stimuli (Zhang et al., 2004). Our results suggest that these genes may also play a role in neutrophils mediating the effect of JIA risk loci during JIA pathogenesis which should be further investigated by experimental approaches.
The pathways of cardiac muscle contraction and hypertrophic cardiomyopathy are significantly and specifically enriched in PPI network of JIA target genes and their interactors in neutrophils. Multiple studies have reported that patients with rheumatoid arthritis have a higher incidence and mortality of cardiovascular disease (Maradit-Kremers et al., 2005; Voskuyl, 2006; Avina-Zubieta et al., 2008; Georgiadis et al., 2008). Cardiac involvement has similarly been found in JIA patients (Svantesson et al., 1983; Hull, 1988). However, whether JIA increases the long-term risk of cardiovascular disease is still uncertain (Coulson et al., 2013). Our results suggest that JIA and cardiovascular disease may share common underlying molecular mechanism.
High-throughput omics technology provides a wealth of experimental data for disease gene discovery. The multi-omics studies on the interplay between genes, RNA, proteins and small molecules reveal new directions for the research of complex diseases (Bersanelli et al., 2016; Bock et al., 2016). Integration of data from different dimensions of multi-omics data via different analytical approaches facilitates prioritizing genes for efficient functional studies and contributes to the understanding of disease etiology.
Data Availability
All datasets generated for this study are included in the manuscript and/or the Supplementary Files.
Author Contributions
JL, XM, and QX conceived and designed the study. JYL, XCY, XMY, YS, and QX performed the analysis. MM, XC, and HH provided the methylation analysis data. JYL wrote the manuscript. JL and XM reviewed and edited the manuscript critically. All authors read and approved the manuscript.
Funding
This study was supported by National Natural Science Foundation of China (81771769); Tianjin Natural Science Foundation (18JCYBJC42700); Startup Funding from Tianjin Medical University and the Thousand Youth Talents Plan of Tianjin.
Conflict of Interest Statement
The authors declare that the research was conducted in the absence of any commercial or financial relationships that could be construed as a potential conflict of interest.
Supplementary Material
The Supplementary Material for this article can be found online at: https://www.frontiersin.org/articles/10.3389/fgene.2019.00181/full#supplementary-material
Footnotes
- ^https://pubs.broadinstitute.org/mammals/haploreg/haploreg.php
- ^https://www.networkanalyst.ca
- ^promoter.bx.psu.edu/hi-c/
- ^http://fuma.ctglab.nl/
References
Avina-Zubieta, J. A., Choi, H. K., Sadatsafavi, M., Etminan, M., Esdaile, J. M., and Lacaille, D. (2008). Risk of cardiovascular mortality in patients with rheumatoid arthritis: a meta-analysis of observational studies. Arthritis Rheum 59, 1690–1697. doi: 10.1002/art.24092
Aydin-Son, Y., Batu, E. D., Demirkaya, E., Bilginer, Y., Kasapcopur, O., Unsal, E., et al. (2015). Systems-level analysis of genome wide association study results for a pilot juvenile idiopathic arthritis family study. Turk J. Pediatr. 57,324–333.
Barrett, T., Wilhite, S. E., Ledoux, P., Evangelista, C., Kim, I. F., Tomashevsky, M., et al. (2013). NCBI GEO: archive for functional genomics data sets–update. Nucleic Acids Res. 41, D991–D995. doi: 10.1093/nar/gks1193
Behrens, E. M., Finkel, T. H., Bradfield, J. P., Kim, C. E., Linton, L., Casalunovo, T., et al. (2008). Association of the TRAF1-C5 locus on chromosome 9 with juvenile idiopathic arthritis. Arthritis Rheum 58, 2206–2207. doi: 10.1002/art.23603
Bersanelli, M., Mosca, E., Remondini, D., Giampieri, E., Sala, C., Castellani, G., et al. (2016). Methods for the integration of multi-omics data: mathematical aspects. BMC Bioinformatics 17(Suppl. 2):15. doi: 10.1186/s12859-015-0857-9
Bock, C., Farlik, M., and Sheffield, N. C. (2016). Multi-omics of single cells: strategies and applications. Trends Biotechnol. 34, 605–608. doi: 10.1016/j.tibtech.2016.04.004
Cobb, J., Cule, E., Moncrieffe, H., Hinks, A., Ursu, S., Patrick, F., et al. (2014). Genome-wide data reveal novel genes for methotrexate response in a large cohort of juvenile idiopathic arthritis cases. Pharmacogenomics J. 14:356. doi: 10.1038/tpj.2014.3
Coulson, E. J., Ng, W.-F., Goff, I., and Foster, H. E. (2013). Cardiovascular risk in juvenile idiopathic arthritis. Rheumatology 52, 1163–1171. doi: 10.1093/rheumatology/ket106
Diaz-Pena, R., Aransay, A. M., Bruges-Armas, J., Lopez-Vazquez, A., Rodriguez-Ezpeleta, N., Mendibil, I., et al. (2011). Fine mapping of a major histocompatibility complex in ankylosing spondylitis: association of the HLA-DPA1 and HLA-DPB1 regions. Arthritis Rheum 63, 3305–3312. doi: 10.1002/art.30555
Edgar, R., Domrachev, M., and Lash, A. E. (2002). Gene expression omnibus: NCBI gene expression and hybridization array data repository. Nucleic Acids Res. 30, 207–210. doi: 10.1093/nar/30.1.207
Fattori, V., Amaral, F. A., and Verri, W. A. Jr. (2016). Neutrophils and arthritis: role in disease and pharmacological perspectives. Pharmacol. Res. 112, 84–98. doi: 10.1016/j.phrs.2016.01.027
Finkel, T. H., Li, J., Wei, Z., Wang, W., Zhang, H., Behrens, E. M., et al. (2016). Variants in CXCR4 associate with juvenile idiopathic arthritis susceptibility. BMC Med. Genet. 17:24. doi: 10.1186/s12881-016-0285-3
Frank, M. B., Wang, S., Aggarwal, A., Knowlton, N., Jiang, K., Chen, Y., et al. (2009a). Disease-associated pathophysiologic structures in pediatric rheumatic diseases show characteristics of scale-free networks seen in physiologic systems: implications for pathogenesis and treatment. BMC Med. Genomics 2:9. doi: 10.1186/1755-8794-2-9
Frank, M. B., Wang, S., Aggarwal, A., Knowlton, N., Kaiyu, J., Chen, Y., et al. (2009b). Childhood Onset Rheumatic Disease Gene Expression Profile. GEO. Available at: https://www.ncbi.nlm.nih.gov/geo/query/acc.cgi?acc=GSE11083
Frisell, T., Hellgren, K., Alfredsson, L., Raychaudhuri, S., Klareskog, L., and Askling, J. (2016). Familial aggregation of arthritis-related diseases in seropositive and seronegative rheumatoid arthritis: a register-based case-control study in Sweden. Ann. Rheum Dis. 75, 183–189. doi: 10.1136/annrheumdis-2014-206133
Georgiadis, A. N., Voulgari, P. V., Argyropoulou, M. I., Alamanos, Y., Elisaf, M., Tselepis, A. D., et al. (2008). Early treatment reduces the cardiovascular risk factors in newly diagnosed rheumatoid arthritis patients. Semin. Arthritis Rheum 38, 13–19. doi: 10.1016/j.semarthrit.2007.09.008
Glass, D. N., and Giannini, E. H. (1999). Juvenile rheumatoid arthritis as a complex genetic trait. Arthritis Rheum 42, 2261–2268. doi: 10.1002/1529-0131(199911)42:11<2261::AID-ANR1>3.0.CO;2-P
Haasnoot, A. J. W., Schilham, M. W., Kamphuis, S., Hissink Muller, P. C. E., Heiligenhaus, A., Foell, D., et al. (2018). Identification of an amino acid motif in HLA-DRbeta1 that distinguishes uveitis in patients with juvenile idiopathic arthritis. Arthritis Rheumatol. 70, 1155–1165. doi: 10.1002/art.40484
Hakkim, A., Furnrohr, B. G., Amann, K., Laube, B., Abed, U. A., Brinkmann, V., et al. (2010). Impairment of neutrophil extracellular trap degradation is associated with lupus nephritis. Proc. Natl. Acad. Sci. U.S.A. 107, 9813–9818. doi: 10.1073/pnas.0909927107
Hartl, D., Krauss-Etschmann, S., Koller, B., Hordijk, P. L., Kuijpers, T. W., Hoffmann, F., et al. (2008). Infiltrated neutrophils acquire novel chemokine receptor expression and chemokine responsiveness in chronic inflammatory lung diseases. J. Immunol. 181, 8053–8067. doi: 10.4049/jimmunol.181.11.8053
Hinks, A., Barton, A., Shephard, N., Eyre, S., Bowes, J., Cargill, M., et al. (2009). Identification of a novel susceptibility locus for juvenile idiopathic arthritis by genome-wide association analysis. Arthritis Rheum 60, 258–263. doi: 10.1002/art.24179
Hinks, A., Cobb, J., Marion, M. C., Prahalad, S., Sudman, M., Bowes, J., et al. (2013). Dense genotyping of immune-related disease regions identifies 14 new susceptibility loci for juvenile idiopathic arthritis. Nat. Genet. 45, 664–669. doi: 10.1038/ng.2614
Hong, F., Breitling, R., Mcentee, C. W., Wittner, B. S., Nemhauser, J. L., and Chory, J. (2006). RankProd: a bioconductor package for detecting differentially expressed genes in meta-analysis. Bioinformatics 22, 2825–2827. doi: 10.1093/bioinformatics/btl476
Irizarry, R. A., Hobbs, B., Collin, F., Beazer-Barclay, Y. D., Antonellis, K. J., Scherf, U., et al. (2003). Exploration, normalization, and summaries of high density oligonucleotide array probe level data. Biostatistics 4, 249–264. doi: 10.1093/biostatistics/4.2.249
Jiang, K., Frank, M. B., Osban, J., Chen, Y., Hartman, C., and Jarvis, J. N. (2015). Common Immunologic Pathways Between Oligoarticular and Polyarticular Juvenile Idiopathic Arthritis. GEO. Available at: https://www.ncbi.nlm.nih.gov/geo/query/acc.cgi?acc=GSE67596
Kanehisa, M., Furumichi, M., Tanabe, M., Sato, Y., and Morishima, K. (2017). KEGG: new perspectives on genomes, pathways, diseases and drugs. Nucleic Acids Res. 45, D353–D361. doi: 10.1093/nar/gkw1092
Kawabata, Y., Nishida, N., Awata, T., Kawasaki, E., Imagawa, A., Shimada, A., et al. (2018). A genome-wide association study confirming a strong effect of HLA and identifying variants in CSAD/lnc-ITGB7-1 on chromosome 12q13.13 associated with susceptibility to fulminant type 1 diabetes. Diabetes 68, 665–675. doi: 10.2337/db18-0314
Kessenbrock, K., Krumbholz, M., Schonermarck, U., Back, W., Gross, W. L., Werb, Z., et al. (2009). Netting neutrophils in autoimmune small-vessel vasculitis. Nat. Med. 15, 623–625. doi: 10.1038/nm.1959
Kundaje, A., Meuleman, W., Ernst, J., Bilenky, M., Yen, A., Heravi-Moussavi, A., et al. (2015). Integrative analysis of 111 reference human epigenomes. Nature 518, 317–330. doi: 10.1038/nature14248
Li, Y. R., Li, J., Zhao, S. D., Bradfield, J. P., Mentch, F. D., Maggadottir, S. M., et al. (2015a). Meta-analysis of shared genetic architecture across ten pediatric autoimmune diseases. Nat. Med. 21, 1018–1027. doi: 10.1038/nm.3933
Li, Y. R., Zhao, S. D., Li, J., Bradfield, J. P., Mohebnasab, M., Steel, L., et al. (2015b). Genetic sharing and heritability of paediatric age of onset autoimmune diseases. Nat. Commun. 6:8442. doi: 10.1038/ncomms9442
Liu, X., Zhang, H., Chang, X., Shen, J., Zheng, W., Xu, Y., et al. (2017). Upregulated expression of CCR3 in rheumatoid arthritis and CCR3-dependent activation of fibroblast-like synoviocytes. Cell Biol. Toxicol. 33, 15–26. doi: 10.1007/s10565-016-9356-7
Lonsdale, J., Thomas, J., Salvatore, M., Phillips, R., Lo, E., Shad, S., et al. (2013). The genotype-tissue expression (GTEx) project. Nat. Genet. 45:580. doi: 10.1038/ng.2653
MacArthur, J., Bowler, E., Cerezo, M., Gil, L., Hall, P., Hastings, E., et al. (2017). The new NHGRI-EBI Catalog of published genome-wide association studies (GWAS Catalog). Nucleic Acids Res. 45, D896–D901. doi: 10.1093/nar/gkw1133
Maradit-Kremers, H., Nicola, P. J., Crowson, C. S., Ballman, K. V., and Gabriel, S. E. (2005). Cardiovascular death in rheumatoid arthritis: a population-based study. Arthritis Rheum 52, 722–732. doi: 10.1002/art.20878
Naegele, M., Tillack, K., Reinhardt, S., Schippling, S., Martin, R., and Sospedra, M. (2012). Neutrophils in multiple sclerosis are characterized by a primed phenotype. J. Neuroimmunol. 242, 60–71. doi: 10.1016/j.jneuroim.2011.11.009
Németh, T., and Mócsai, A. (2012). The role of neutrophils in autoimmune diseases. Immunol. Lett. 143, 9–19. doi: 10.1016/j.imlet.2012.01.013
Oen, K. G., and Cheang, M. (1996). Epidemiology of chronic arthritis in childhood. Semin. Arthritis Rheum 26, 575–591. doi: 10.1016/S0049-0172(96)80009-6
Ombrello, M. J., Arthur, V. L., Remmers, E. F., Hinks, A., Tachmazidou, I., Grom, A. A., et al. (2017). Genetic architecture distinguishes systemic juvenile idiopathic arthritis from other forms of juvenile idiopathic arthritis: clinical and therapeutic implications. Ann. Rheumatic Dis. 76, 906–913. doi: 10.1136/annrheumdis-2016-210324
Onuora, S. (2015). Rheumatoid arthritis: clues to the HLA-RA connection from T-cell crossreactivity to vinculin and microorganisms. Nat. Rev. Rheumatol. 11:384. doi: 10.1038/nrrheum.2015.73
Orchard, S., Kerrien, S., Abbani, S., Aranda, B., Bhate, J., Bidwell, S., et al. (2012). Protein interaction data curation: the International Molecular Exchange (IMEx) consortium. Nat. Methods 9, 345–350. doi: 10.1038/nmeth.1931
Parkkola, A., Laine, A. P., Karhunen, M., Harkonen, T., Ryhanen, S. J., Ilonen, J., et al. (2017). HLA and non-HLA genes and familial predisposition to autoimmune diseases in families with a child affected by type 1 diabetes. PLoS One 12:e0188402. doi: 10.1371/journal.pone.0188402
Petty, R. E., Southwood, T. R., Manners, P., Baum, J., Glass, D. N., Goldenberg, J., et al. (2004). International league of associations for rheumatology classification of juvenile idiopathic arthritis: second revision, Edmonton, 2001. J. Rheumatol. 31, 390–392.
Pons, P., and Latapy, M. (2005). “Computing communities in large networks using random walks,” in Computer and Information Sciences ISCIS 2005, eds P. Yolum, T. Güngör, F. Gürgen, and C. Özturan (Berlin: Springer), 284–293. doi: 10.1007/11569596_31
R Core Team (2018). R: A Language and Environment for Statistical Computing. Vienna: R Foundation for Statistical Computing.
Rao, S. S., Huntley, M. H., Durand, N. C., Stamenova, E. K., Bochkov, I. D., Robinson, J. T., et al. (2014). A 3D map of the human genome at kilobase resolution reveals principles of chromatin looping. Cell 159, 1665–1680. doi: 10.1016/j.cell.2014.11.021
Savolainen, A., Saila, H., Kotaniemi, K., Kaipianen-Seppanen, O., Leirisalo-Repo, M., and Aho, K. (2000). Magnitude of the genetic component in juvenile idiopathic arthritis. Ann. Rheum Dis. 59:1001. doi: 10.1136/ard.59.12.1001
Schmitt, A. D., Hu, M., Jung, I., Xu, Z., Qiu, Y., Tan, C. L., et al. (2016). A compendium of chromatin contact maps reveals spatially active regions in the human genome. Cell Rep. 17, 2042–2059. doi: 10.1016/j.celrep.2016.10.061
Smemo, S., Tena, J. J., Kim, K. H., Gamazon, E. R., Sakabe, N. J., Gomez-Marin, C., et al. (2014). Obesity-associated variants within FTO form long-range functional connections with IRX3. Nature 507, 371–375. doi: 10.1038/nature13138
Smerdel, A., Ploski, R., Flato, B., Musiej-Nowakowska, E., Thorsby, E., and Forre, O. (2002). Juvenile idiopathic arthritis (JIA) is primarily associated with HLA-DR8 but not DQ4 on the DR8-DQ4 haplotype. Ann. Rheum Dis. 61, 354–357. doi: 10.1136/ard.61.4.354
Smolen, J. S., Aletaha, D., Barton, A., Burmester, G. R., Emery, P., Firestein, G. S., et al. (2018). Rheumatoid arthritis. Nat. Rev. Dis. Primers 4:18001. doi: 10.1038/nrdp.2018.1
Sollid, L. M. (2017). The roles of MHC class II genes and post-translational modification in celiac disease. Immunogenetics 69, 605–616. doi: 10.1007/s00251-017-0985-7
Svantesson, H., Bjorkhem, G., and Elborgh, R. (1983). Cardiac involvement in juvenile rheumatoid arthritis. A follow-up study. Acta Paediatr. Scand. 72, 345–350. doi: 10.1111/j.1651-2227.1983.tb09726.x
Swaminathan, B., Thorleifsson, G., Joud, M., Ali, M., Johnsson, E., Ajore, R., et al. (2015). Variants in ELL2 influencing immunoglobulin levels associate with multiple myeloma. Nat. Commun. 6:7213. doi: 10.1038/ncomms8213
Thompson, S. D., Marion, M. C., Sudman, M., Ryan, M., Tsoras, M., Howard, T. D., et al. (2012). Genome-wide association analysis of juvenile idiopathic arthritis identifies a new susceptibility locus at chromosomal region 3q13. Arthritis Rheum 64, 2781–2791. doi: 10.1002/art.34429
Van Ingen, G., Li, J., Goedegebure, A., Pandey, R., Li, Y. R., March, M. E., et al. (2016). Genome-wide association study for acute otitis media in children identifies FNDC1 as disease contributing gene. Nat. Commun. 7:12792. doi: 10.1038/ncomms12792
Voskuyl, A. E. (2006). The heart and cardiovascular manifestations in rheumatoid arthritis. Rheumatology 45(Suppl. 4), iv4–iv7. doi: 10.1093/rheumatology/kel313
Wang, Y., Song, F., Zhang, B., Zhang, L., Xu, J., Kuang, D., et al. (2018). The 3D genome browser: a web-based browser for visualizing 3D genome organization and long-range chromatin interactions. Genome Biol. 19:151. doi: 10.1186/s13059-018-1519-9
Ward, L. D., and Kellis, M. (2012). HaploReg: a resource for exploring chromatin states, conservation, and regulatory motif alterations within sets of genetically linked variants. Nucleic Acids Res. 40, D930–D934. doi: 10.1093/nar/gkr917
Watanabe, K., Taskesen, E., Van Bochoven, A., and Posthuma, D. (2017). Functional mapping and annotation of genetic associations with FUMA. Nat. Commun. 8:1826. doi: 10.1038/s41467-017-01261-5
Xia, J., Benner, M. J., and Hancock, R. E. (2014). NetworkAnalyst–integrative approaches for protein-protein interaction network analysis and visual exploration. Nucleic Acids Res. 42, W167–W174. doi: 10.1093/nar/gku443
Keywords: juvenile idiopathic arthritis, target gene identification, epigenetic regulation, protein-protein interaction, pathway enrichment
Citation: Li J, Yuan X, March ME, Yao X, Sun Y, Chang X, Hakonarson H, Xia Q, Meng X and Li J (2019) Identification of Target Genes at Juvenile Idiopathic Arthritis GWAS Loci in Human Neutrophils. Front. Genet. 10:181. doi: 10.3389/fgene.2019.00181
Received: 15 October 2018; Accepted: 19 February 2019;
Published: 27 March 2019.
Edited by:
Tao Huang, Shanghai Institutes for Biological Sciences (CAS), ChinaReviewed by:
Abhay Sharma, Institute of Genomics and Integrative Biology (CSIR), IndiaHaitao Zhang, National Institutes of Health (NIH), United States
Jiangong Niu, University of Texas MD Anderson Cancer Center, United States
Copyright © 2019 Li, Yuan, March, Yao, Sun, Chang, Hakonarson, Xia, Meng and Li. This is an open-access article distributed under the terms of the Creative Commons Attribution License (CC BY). The use, distribution or reproduction in other forums is permitted, provided the original author(s) and the copyright owner(s) are credited and that the original publication in this journal is cited, in accordance with accepted academic practice. No use, distribution or reproduction is permitted which does not comply with these terms.
*Correspondence: Xinyi Meng, bWVuZ3h5QHRtdS5lZHUuY24= Jin Li, amxpMDFAdG11LmVkdS5jbg==