- 1Department of Genetic Medicine, Faculty of Medicine, King Abdulaziz University, Jeddah, Saudi Arabia
- 2Princess Al-Jawhara Center of Excellence in Research of Hereditary Disorders, King Abdulaziz University, Jeddah, Saudi Arabia
Rheumatoid arthritis (RA) is a chronic autoimmune disorder of bone joints caused by the complex interplay between several factors like body physiology, the environment with genetic background. The recent meta-analysis of GWAS has expanded the total number of RA-associated loci to more than 100, out of which approximately ∼97% (98 variants) loci are located in non-coding regions, and the other ∼3% (3 variants) are in three different non-HLA genes, i.e., TYK2 (Prp1104Ala), IL6R (Asp358Ala), and PTPN22 (Trp620Arg). However, whether these variants prompt changes in the protein phenotype with regards to its stability, structure, and interaction with other molecules, remains unknown. Thus, we selected the three clinically pathogenic variants described above, as positive controls and applied diverse computational methods to scrutinize if those mutations cause changes in the protein phenotype. Both wild type and mutant protein structures of PTPN22 (W620R), IL6R (D358A), and TYK2 (P1104A) were modeled and studied for structural deviations. Furthermore, we have also studied the secondary structure characteristics, solvent accessibility and stability, and the molecular interaction deformities caused by the amino acid substitutions. We observed that simple nucleotide predictions of SIFT, PolyPhen, CADD and FATHMM yields mixed findings in screening the RA-missense variants which showed a ≥P-value threshold of 5 × 10−8 in genome wide association studies. However, structure-based analysis confirms that mutant structures shows subtle but significant changes at their core regions, but their functional domains seems to lose wild type like functional interaction. Our findings suggest that the multidirectional computational analysis of clinically potential RA-mutations could act as a primary screening step before undertaking functional biology assays.
Introduction
Rheumatoid arthritis (RA) is a chronic, progressive and disabling autoimmune disease of bone joints (Fazal et al., 2018). Although the full etiology of RA remains unclear, it is widely seen as a complex disease caused by the combined influence of several factors like body physiology, environment and genetic background (Chaudhari et al., 2016; Angelotti et al., 2017). The genetic basis of RA is well demonstrated by epidemiological studies which reported variable disease prevalence’s among different ethnic groups, inheritance pattern in families, and shared susceptibility loci with other autoimmune diseases (Ek et al., 2014). The heritable nature of RA is assessed to be approximately 60%, while the contribution of the human leucocyte antigen region (HLA), which allows the immune system to differentiate between self and foreign bodies, to heritability is estimated to be between 11 and 37%. Out of all HLA genes, the HLA-DRB1 region is recognized as a major contributor of RA susceptibility, i.e., ∼30% (Knevel et al., 2017). In addition to the HLA region genes, some non-HLA candidate genes like PTPN22 and PAD14 have also been discovered to play a major role in RA susceptibility (Rodriguez-Elias et al., 2016).
As in other complex diseases, the advent of Genome Wide Association Studies (GWAS) have dramatically enhanced the resolution of RA genetics, by successfully identifying disease associated common genetic variants (Manolio, 2010). More notably, GWAS followed by subsequent meta-analysis of GWAS data derived from the single nucleotide polymorphisms (SNPs) based dense genotyping of 73,758 controls and 29,880 RA cases, belonging to Asian and European ethnicities, has expanded the total number of RA-associated loci to more than 100 (Okada et al., 2014). This finding has expanded the list of non-HLA loci genes (including PADI4, PTPN22, TNFAIP3, IRF5, STAT4, TRAF1/C5, REL, and CCR6 genes etc) proposed in the genetic etiology of RA. Together, these loci can share the heritability of RA among different ethnicities by ∼80%. Approximately 97% (98 lead SNPs) of these risk loci are located in non-coding regions, and the other 3% (3 SNPs) are found in three different genes, i.e., TYK2 (rs34536443), IL6R (rs2228145), and PTPN22 (rs2476601), which play a critical role in mediating autoinflammation. However, the specific structural and functional deformations caused by these non-synonymous (nsSNPs) is not yet fully explored.
The SNPs which occur in protein coding regions are often evolutionarily conserved and are significant to disease etiology, by changing the amino acid sequence and physicochemical properties of the polypeptide chains they encode. It is therefore possible that these coding SNPs may possess the largest impact in disease pathogenesis, compared to their counterparts, i.e., non-coding SNPs which are mostly neutral to disease pathogenesis (Ng and Henikoff, 2006). Although, both in vitro and in vivo experimental methodologies can improve our ability to explore the effects of nsSNPs, they often require vast technical resources and manpower in addition to incurring huge costs. In recent decades various computational methods have been launched for predicting the impacts of mutations on protein structure and function. These methods can successfully identify several structural and stability changes which occur in proteins due to mutations (Anwer et al., 2015; Sneha and Doss, 2016; Mohajer et al., 2017). The aim of this study was to assess the ability of diverse computational methods in predicting the missense variant induced alterations in amino acid sequence conservation, structural and functional features of RA candidate proteins. Therefore, in the conext of the current study’s objective, we selected PTPN22 (W620R,) IL6R (D358A), and TYK2 (P1104A) as “positive control variants” because we know their pathogenic potential in contributing to the development of RA, from GWAS findings (≥P-value significance threshold of 5 × 10−8).
Methodology
Selection of RA Susceptibility Loci
The 101 RA susceptibility loci, as confirmed by Okada, Jostins et al. (2012), were used as the core data set for this study. The relevant information (e.g., rsID, Genome wide significance with a ≥P-value threshold of 5 × 10−8, odd ratios) of these SNPs was retrieved from the article. Only missense mutations were studied in the present investigation. The full-length amino acid sequence of the candidate proteins was collected from UniProt. The description terms like variants, SNPs, and mutations are interchangeably used in this manuscript.
In silico Assessment of Deleterious Potential of nsSNPs of RA
The Variant Effect Predictor (VEP) toolset, hosted on the Ensembl webpage, was used for the analysis and annotation of coding region genomic variants. This powerful computational tool offers accessibility to an extensive range of genetic annotations and provides simple options for configuring and extending the data analysis. VCF (variant call format) is an accepted format of input data for VEP. However, other variant identification formats of dbSNP and HGVS are also recognized by this toolset. The output consists of comprehensive information about mutation (chromosomal location, variant effect, transcript ID) and its functional impact on the protein in form of prediction scores for different computational methods. In this study, the prediction scores of Sorting Tolerant from Intolerant (SIFT) (Vaser et al., 2016). Polymorphism Phenotyping v2 (PolyPhen-2); (Adzhubei et al., 2013), Combined Annotation Dependent Depletion (CADD) (Rentzsch et al., 2018); and Functional Analysis through Hidden Markov Models (FATHMM) (Shihab et al., 2013) for each variant query were generated. The consequences of variants are described in accordance to the standard annotation terms of Sequence Ontology (Cunningham et al., 2015).
Structural Analysis of RA Proteins
3-Dimensional Modeling of Wild Type and Mutant Protein Models
In the initial stage, the protein data bank (PDB) database was searched for x-ray crystallized structures of candidate proteins. Wherever full length protein structures were not available, we simulated them through ab initio procedures using I- TASSER (Iterative Threading ASSEmbly Refinement) web server (Yang et al., 2015), by providing an amino acid sequence of the corresponding candidate protein as an input. This computational tool utilizes basic protein structure templates available in PDB for constructing the full-length atomic models of three-dimensional proteins following the iterative template fragment assembly approach (Yang and Zhang, 2015). Out of the top five models generated for each candidate protein, the best models’ selection was based on the estimated template model (TM) score, confidence score, and root mean square deviation (RMSD) scores. In the eventual stages, these selected models were further processed for energy minimization using the gromacs-steepest descent energy minimization method in NOMAD-Ref web server (Lindahl et al., 2006). The energy minimized three-dimensional protein models were further used in constructing the mutant versions of proteins, by substituting the amino acid residues in place of the reference amino acid sequence of each candidate protein, through a homology modeling procedure using Modeller v9.21 (Webb and Sali, 2017). This software adopts an automated approach for comparative protein structure modeling, through the satisfaction of spatial restraints in the candidate protein of interest (Sanchez and Sali, 2000). The three-dimensional protein structure modeling procedure starts with the alignment of a target model against similar known protein structures. The energy minimization and stereochemical quality check of built mutant models was done using the Procheck tool (Laskowski et al., 1996). Finally, mutant models were visulized by PyMol (Janson et al., 2017) and Chimera computational programs (Banaganapalli et al., 2016; Shaik et al., 2018).
Secondary Structure Analysis
The correlation between mutant amino acid sequences and protein structures can be made unambiguous by obtaining information about the alterations of different secondary structural elements such as the orientation and numbers of helices (α), strands (β), bends and turns, which are basic structural elements of protein scaffolds. To obtain the knowledge of the structural context of the amino acid substitution variants of RA-candidate proteins, we used an online web server program called PDBsum. The input for this tool is a PDB structure four-letter code of queried proteins and the output is in the form of a ‘wiring diagram,’ which shows the important secondary structural elements and their orientation along the query protein’s sequence.
Solvent Accessibility Analysis
Solvent accessibility refers to the exposed surface area of amino acid residues within a three-dimensional structure and in an extended tripeptide conformation. The ratio of relative surface accessibility of mutated amino acids of RA candidate proteins towards solvents was predicted using the NetSurfP server (Petersen et al., 2009). The data input for this web server consists of a FASTA format of amino acid sequences of the queried protein. The relative solvent accessibility of each amino acid is predicted in the form of a Z-score (Klausen et al., 2019).
Protein Stability Analysis
DUET, an easy-to-use bioinformatics web server, which integrates two complementary methods such as, mCSM (mutation Cutoff Scanning Matrix) and SDM (Site Directed Mutator) into a consensus/optimized prediction, using Support Vector Machines (SVMs), was used to understand the effect of point mutations on the protein structure. Input options for this tool consists of a PDB structure of a wild-type protein, in addition to amino acid information (wild type or mutant) in one letter codes. The output is in form of individual and combined predictions, in addition to the interactive visualization of proteins. The prediction results are expressed as Gibbs Free Energy (ΔΔG) values, where negative values indicate that the given mutation is highly destabilizing (Pires et al., 2014).
Protein Structural Equivalency Analysis
We have superimposed the wild type proteins on to mutant protein structures, to examine structural equivalency of native versus mutant amino acid residues and their corresponding whole protein structures, using PyMOL software (Janson et al., 2017). The input options for superposition requires PDB files (txt format) or PDB accession numbers of both wild and mutant protein structures. The outputs of a structural alignment are a superposition of the atomic coordinate sets (Ca traces and backbone atoms) and a minimal RMSD between the structures. The difference in RMSD scores, of two aligned amino acid residues (<0.2 Å) or whole protein structures (<2.0 Å), indicates their divergence from one another.
Conserved Domains Identification
The functional domains in RA candidate proteins were searched in the Conserved Domain Database using query sequences (nucleotide or amino acids) to gain insights into the relationships between mutated amino acid sequence, with both protein structure and function. This tool relies on RPS-BLAST, which can quickly scan the pre-computed position-specific scoring matrices (PSSMs) in the query protein, to identify the different conservation features related to protein domains. The output is in the form of annotated protein domains against the input query sequence, along with visualization options. The high level associations between query protein sequences and annotated conserved domains are shown as specific hits (Marchler-Bauer et al., 2011).
Gene Functional Analysis
The STRING1 web interface, which generates data on gene functions, gene list analysis, and gene prioritization for functional biology assays, was used to identify direct and indirect genetic interaction networks of RA candidate proteins. The input options include either query genes or protein names, their sequences, or large data sets (Szklarczyk et al., 2017). The output format is in the form of network node predictions, which reveal the functional associations between query genes based on their neighborhood or evidence of co-expression in data generated from high-throughput biological experiments. The highest interacting gene or proteins were identified based on the strength of the confidence score generated in the network.
Protein–Protein Molecular Docking
The Hex protein docking server was employed to execute the molecular docking of RA proteins and interacting proteins, to examine their mutual structural plasticity and interaction potential, while forming molecular complexes. In this step, the protein partner showing the highest confidence score of the queried protein in the molecular network (derived from STRING results), was taken as a ligand molecule and the query protein as a receptor. The molecular models of the experimentally solved crystal structures of proteins retrieved from PDB or ab initio modeled protein structures, were used in this step. The major default setting used in the docking procedure is the “free energy calculation,” which is set at 180° to enable the total possible rotational increments for receptor and ligand sampling of their own centroids. The other parameters include a grid spacing set at 0.6 Å, positive and negative steps at 0.75 Å, 53 intermolecular separations calculated in 20 steric scan phases. In the final calculations the final 25 phase was applied to obtain the highest orientation score of 0.76.2 Å. At the end, to obtain 10,000 the lowest ordered docking energy score, 500 clusters were retained from the best 1000 orientations (Ritchie, 2003; Shaik et al., 2014).
Results
Coding SNPs of RA Risk Loci
The 101 RA risk loci included 98 (97%) non-coding SNPs and three (3%) coding region SNPs. The molecular details of these three coding SNPs, including chromosomal position, gene name, cDNA change, type and position of amino acid variant are provided in Table 1.
Deleterious Potential of Missense Mutations
The SIFT prediction algorithm uses the nucleotide sequence homology principle to classify the pathogenic amino acid substitutions, based on their deleterious potential on the function of the concerned protein. SIFT prediction values range from 0 to 1, where lower scores reflect the highly deleterious potential of non-synonymous mutation towards the structural and functional features of the corresponding protein. The missense mutation P1104A (SIFT score is ‘0.’) revealed its highly deleterious potential towards the function of TYK2 protein. The remaining two missense mutations, i.e., W620R of PTPN22 and D358A of IL6 are tolerant to protein’s function (tolerance score index is 0.06 to 1). The Polyphen algorithm uses a Naive Bayes probabilistic score to calculate the pathogenicity potential of amino acid substitution mutations. The P1104A missense mutation of the TYK2 gene showed a probable damaging potential (score is 0.97), whereas W620R of PTPN22 (score is 0) and D358A of IL6 (score is 0.05) are found to be benign in their effect. The CADD is an integrative annotation of multiple mutation prediction methods into one framework. It generates a combined annotation score (c-score) for each query variant and classifies them as non-functional variants (if C-score is ≥10%), damaging variants (if C-score is ≥20%), and lethal (if C-score is ≥30%). As per this criterion, only the P1104A variant of TYK2 is a damaging variant. The remaining two variants, i.e., W620R of PTPN22 and D358A of IL6R are non-functional variants with an unknown significance. The FATHMM is a species-independent method, with optional species-specific/evolutionary unit weighting, in order to predict the functional effects of coding variants in form of P-values (ranging from 0 to 1) based on ENCODE outcomes. If a given variant shows a p-score of ≥0.5 then it is considered as deleterious and if it shows a p-score of ≤0.5, then it suggests the neutral nature of the queried genetic variant. Similar to the above three predictions FATHAMM has also predicted the deleterious effect of the P1104A variant on the TYK2 protein (Score is 0.821) (Table 2).

Table 2. The SIFT, Polyphen-2, CADD, and FATHMM prediction scores for RA-GWAS amino acid substitution mutations.
Protein Structural Analysis
Protein Modeling and Structural Equivalency Analysis
3D modeling
The I-Tasser prediction server has produced five protein models for each queried amino acid sequence (Uniport ID: PTPN22-Q9Y2R2; TYK2-P29597; IL6R-P08887). From the output, the best protein models were selected based on their TM scores, confidence (c) scores and RMSD scores. The prediction scores of three studied proteins are as follows, PTPN22 (c score = −2.12, TM score is 0.46 ± 0.15 and RMSD score is 13.7 ± 4.0 Å), TYK2 (c score = −1.12, TM score is 0.26 ± 0.10 and RMSD score is 12.7 ± 2.8 Å) and IL6R (c score = −1.65, TM score is 0.35 ± 0.05 and RMSD score is 12.2 ± 3.0 Å). The C-score reflects the quality of the predicted protein models and its value ranges from −5 to 2. A higher C-score indicates the high-quality of the predicted protein model. A C-score of >−1.5 indicates the accurate folding of the polypeptide chain. The full-length models of IL6R, TYK2, and PTPN22 were subjected to energy minimization applying Gromacs 96 force field in Nomad-Ref server (Figure 1). The energy minimization step repairs distorted geometries by moving atoms and releasing the internal constraints in the protein structure. The stereochemical quality checking of full-length models with Procheck software showed that few amino acid residues have their phi/psi angles in the disallowed regions of the protein. Approximately 98.5% of all amino acid residues in three proteins were in the core (allowed region) and 1.5% were in the non-core (disallowed) region, respectively. We have created the mutant versions of proteins, by manually inserting the amino acid change in the protein sequence and template structures of IL6R, TYK2, and PTPN22 native proteins. Out of the 100 guess structures produced (based on randomized initial models), we have selected the best homologous structures of these mutant proteins for energy minimization, stereochemical quality checks and Ramachandran plot assessments.
Secondary structure and surrounding amino acid changes analysis
In order to understand the effect of amino acid substitutions on secondary structural features, we calculated the structural differences in elements such as sheets (β-sheets, β-hairpins, β-bulges, and β-strands), helices (helix, helix–helix interactions), and turns (β turns, γ turns, and disulfide bonds) in both native and mutant models of the PTPN22, IL10RA, and TYK proteins (Figure 2). In PTPN22, the substitution of arginine residue at the 620th position generated two β-hairpins, one β-bulge, four helixes, three helix–helix interactions, two γ-turns and one di-sulfide bond and lost one β-sheet and two strands, and 19 β-turns in the secondary structure of the protein. In IL6R (Asp358Ala) and TYK2 (Pro1104Ala) mutant proteins, the numbers of secondary structural elements are observed to be equal like in their native forms. Overall, the structural analysis results inferred that out of three deleterious mutations of RA (PTPN22-Trp620Arg; IL6R-Asp358Ala; and TYK2-Pro1104Ala), only the Trp620Arg (located in loop region) mutant brought drastic changes, disturbing the secondary structure of PTPN22.
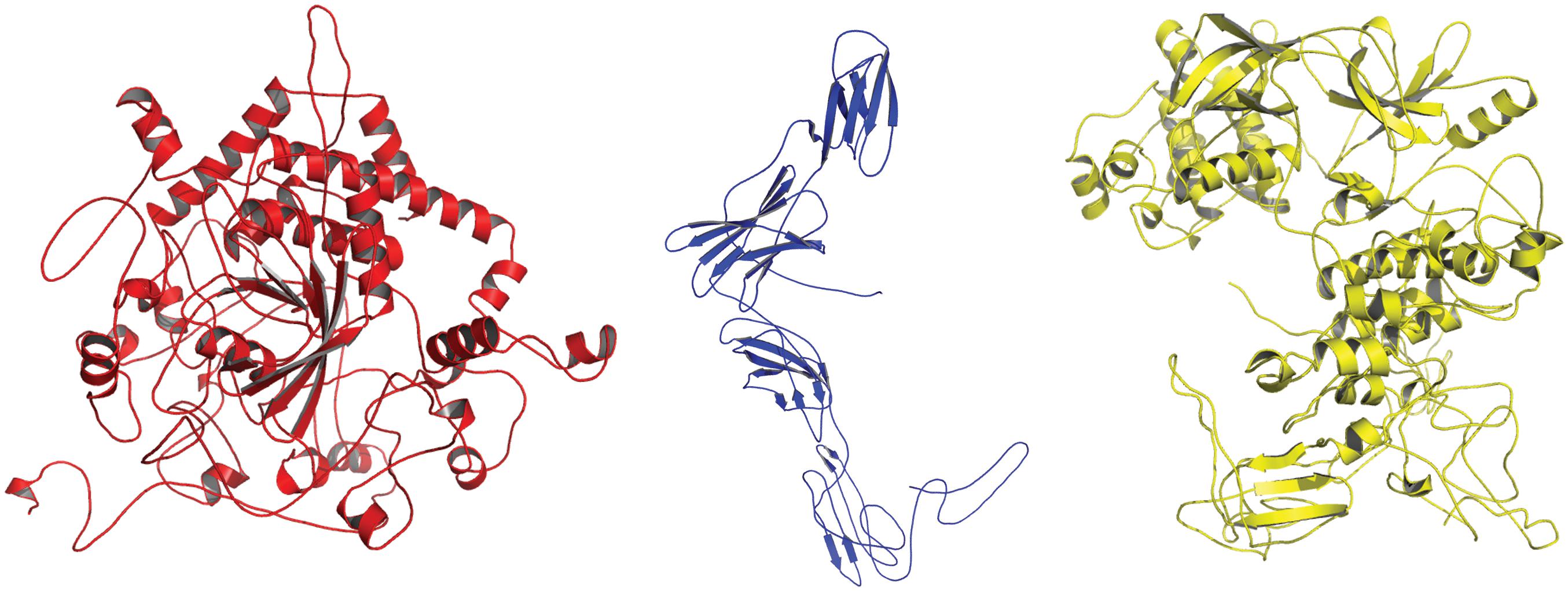
Figure 2. 3D-Structural representation of the refined protein models of (1) PTPN22 (red in color); (2) IL6R (blue in color) (3) TYK2 (yellow in color), generated from I-Tasser.
Root mean square deviation
The structural impact of amino acid substitution mutations can be estimated if there is a divergence at the amino acid or polypeptide chain level of the corresponding proteins. We have observed changes in the cavity volume and residue RMSD scores of Arg620 (2.85 Å) compared to Trp620 of PTPN22. For IL6R (D358V) and TYK2 (P1104A) mutations, the residue level changes were 1.89 and 1.24 Å, respectively (Figure 3).
C&D: solvent accessibility and stability (SAS) analysis
The Tryptophan to Arginine conversion at the 620th amino acid residue of the PTPN22 protein, increases its solvent accessibility property by 17.20%. In the IL6R protein, the mutated Valine amino acid residue at the 358th position is exposed to solvents by 51.90% compared to its native counterpart Aspartic acid, which exists in a buried state. In the TYK2 protein, both Proline (wild type) and Alanine (mutant) amino acid residues at the 1104th position, remain in the buried state and inaccessible to solvents (Table 3).

Table 3. The solvent accessibility (SA), stability and root mean square deviation (RMSD) prediction scores for three RA-GWAS amino acid substitution mutations.
Solvent accessibility and stability is one of the decisive factors in protein folding and to further evaluate the protein stability (net balance of forces that determine the native fold of protein structure) we used an integrated computational approach, the DUET method. This DUET analysis provides the consensus output of SDM and mCSM methods in a non-linear regression fashion, based on Radial Basis Function Kernel function. The Gibbs free energy (ΔΔG) change of the PTPN22, W620R (−0.453 Kcal/Mol), IL6R, D358V (−0.093 Kcal/Mol), and TYK2, P1104A (−1.493 Kcal/Mol) missense mutations were found to be in the range of negative values, further indicating their deleterious potential to the stability of the proteins concerned.
Conserved Domains Identification
The mapping of evolutionary conserved domains of disease-causing proteins, is an essential step in inferring the relationship between the nucleotide sequence and protein structure and its function. Functional annotation of 807 amino sequences of PTPN22 has revealed that the Trp620Arg variant is located outside (332 amino acids downstream) of the protein tyrosine phosphatase (PTP) domain which exists in between the 24th and 288th amino acids. The PTP domain catalyzes the dephosphorylation of phosphorylated tyrosine peptides and regulates its levels in the signal transduction pathways. The Asp358Val of human IL-6R alpha, is located outside of three domains [Ig superfamily, two fibronectin type III (FnIII) domains each ≈100 residues] of the extracellular region of the 468 amino acids long protein molecule. The domain mapping of Pro1104Ala mutation of the TYK2 protein, which is made up of 1187 amino acids, revealed that it was located in the c-terminus pseudo kinase catalytical domain (897–1169) of the TYK2 protein (Figure 4).
Gene Network Analysis
We analyzed the genetic association networks of three RA genes, i.e., PTPN22, IL-6RA, and TYK2, to identify their best physical interacting partners in a cellular context. The PTPN22 gene network analysis revealed its tight network with 10 genes including CSK, LCK, ZAP70, HLA-DRB1, CD3E, HLA-DQA2, HLA-DPB1, HLA-DRA, HLA-DRB5, and CD4, of which the highest interaction score (c-score = 0.995) was observed with ZAP70. The IL6R protein showed highest interaction with its ligand IL6 molecule (c-score = 0.998). It also showed diverse association levels with other networking genes such as IL6ST (0.990), STAT3 (0.989), JAK1 (0.971), SOCS3 (0.971), PTPN11 (0.962), JAK2 (0.957), CNTF (0.952), STAT1 (0.951), and TYK2 (0.942). For the TYK2, the best interacting partner was IL23R, which had a c-score of 0.98. Besides these protein molecules, it also interacts with STAT5B, STAT5A, STAT1, STAT2, IL23A, STAT6, STAT3, SOCS1, and PTPN1 proteins (c-scores ranging from 0.98 to 0.99). From the predicted interaction networks, it is evident that all three RA genes, in conjunction with their gene partners, activate different auto immune reactions central to the pathogenesis of RA (Figure 5). We also identified the relationship between PTN22, TYK2, and IL6R, using protein–protein network analysis, we identified that TYK2 and IL6R are significantly enriched in the Interleukin-6 signaling and Tyrosine phosphorylation pathways, the hallmark pathway in inflammation (Figure 5).
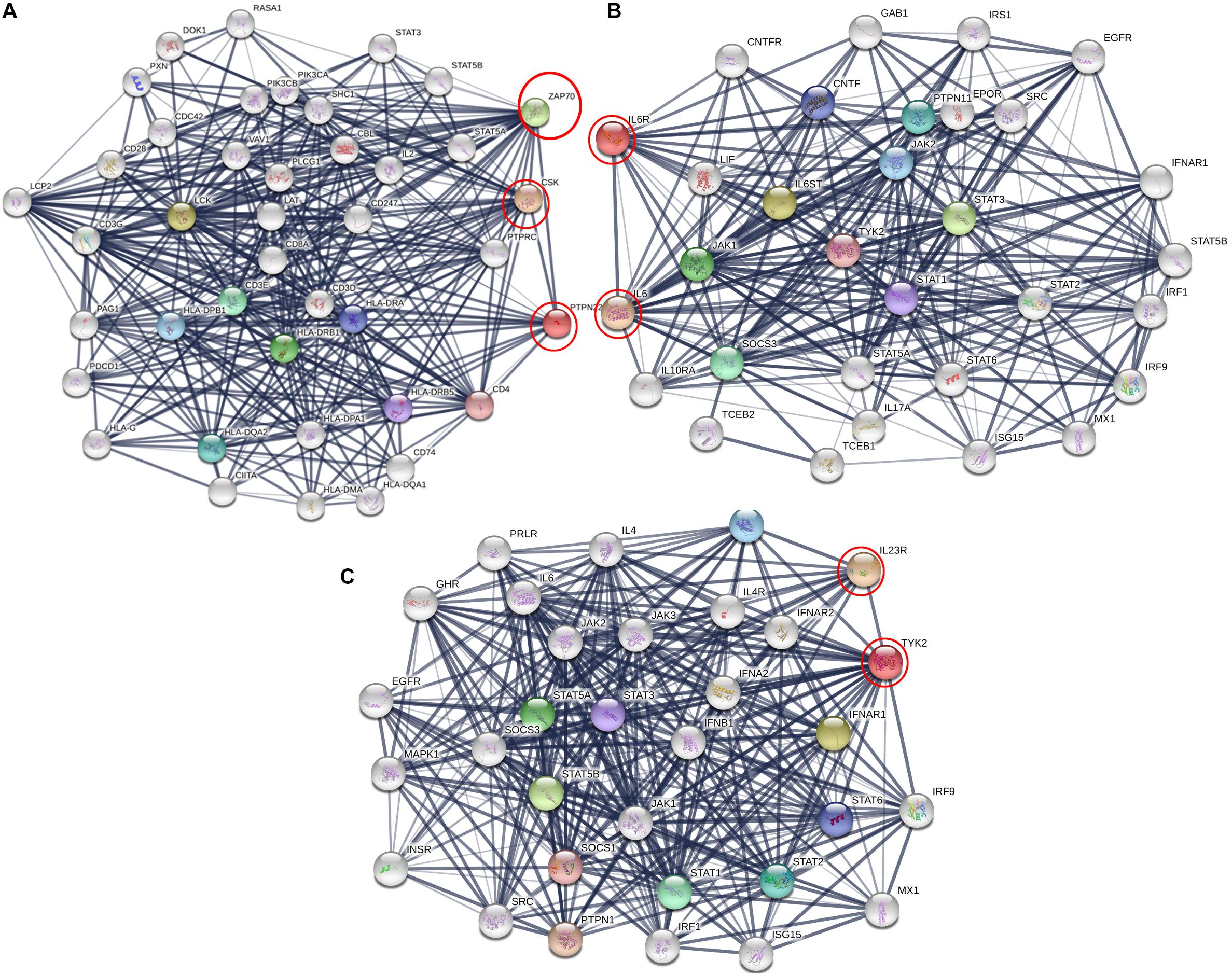
Figure 5. Protein interaction network of (A) PTPN22, (B) IL6R, and (C) TYK2 using STRING web server. Here genes are represented as nodes and edges indicating different types of interaction between genes. Black circles are the query genes and coloring on edges indicate different types of interaction which is defined in the network legend.
Molecular Docking
Based on the results obtained from the protein networking analysis, we identified the best interacting partners of the PTPN22, IL6R, and TYK2 proteins and studied the impact of mutations on their molecular function. Table 3 shows that RA missense mutations contribute to altered interactions with ligand molecules, primarily because of the shift in interacting amino acid residues. Among all the proteins analyzed, TYK2 was calculated to show the lowest binding energy value of −19.90 Kcal/mol with IL23R.
TYK2 (P1104A) showed a weaker interaction (−29.25 Kcal/mol) with IL23R. In native TYK2, Arg539, Thr1161, and Ser1157, amino acids form a hydrophobic interaction with IL23, whereas in the P1104A mutant stage, two new Asp949, Arg952 amino acids participate (in addition to Arg539, Thr1161, and Ser1157) in forming strong H-bonds with Asp349 and Arg351 of IL23R. The PTPN22 wild type protein interacts with six amino acid residues (Ala372, Lys683, Asn806, Gln457, Asp388, and Asn432) of the ZAP70 protein with a binding constant of −391.18 KJ/Mol. However, the W620R mutant PTPN22 binds at a different region of ZAP70 (Lys663, Meth729, Lys300, Ser643, Gln307, Thr545, Gly303, and Ser652) due to a change in the conformation of the mutant structure showing a reduced binding affinity (ΔG is −407.20 KJ/Mol) towards ZAP70. In case of the native IL10R molecule, the docking analysis revealed that five amino acid residues (Ala7, Ser, 171, Gln118, Arg137, and Pro30) interact with the IL10 ligand molecule, by forming strong Hydrogen, ionic interactions and a binding affinity of −554.7 Kcal/Mol. However, in the D358V mutant, Ala7, Leu12, Gln118, Arg137, Pro308, and Trp306 amino acids participate in forming the majority of the weak interactions with IL10, and positively shifts the interaction energy to +28.17 Kj/Mol (Table 4 and Figures 6–8).
Discussion
There have been numerous attempts to understand the mechanisms through which RA genetic variants could influence the activity of candidate proteins and subsequently affect the cell physiology and function. For PTPN22, animal model studies have shown that the tryptophan (W) amino acid at the 620th position positively increases the autoreactive B cell selection, the enlargement of the thymus and spleen, T cells, and dendritic cell activation (Stanford et al., 2010; Zhang et al., 2011). In mice, the expression levels of LYP/Pep were found to be reduced when Pep619W (equivalent of W620R variant) was expressed. It is suggested to be due to the calpain1-mediated rapid degradation of the Pep619W variant compared to that of wild types (Menard et al., 2011; Diaz-Gallo and Martin, 2012). The PTPN22/W620R expression enhances the production and function of neutrophils, calcium release and free radical oxygen concentration around joints. The hyperactivation of W620R carrying cells may directly cause damage to bone joints, in addition to the initiation and continuation of other inflammatory reactions that contribute to the disease development (Bayley et al., 2015). The cytokine interleukin-6 (IL-6) is known to regulate the complex mechanisms that underlie the inflammatory reactions of diverse chronic diseases (Ferreira et al., 2013). In RA patients, the IL-6 molecule is overexpressed in inflamed synovial tissues, where it effects the functions of lymphocytes (T and B), macrophages and osteoclasts by binding to IL-6R (Smolen et al., 2008). A genetic polymorphism of the IL-6 receptor locus (Asp358Ala) is known to cause changes in serum IL-6R levels and a modest change in levels of IL-6. Functional studies demonstrated that IL-6,358Ala allele increases the expression of soluble ILR isoform, but reduces the membrane bound (CD4+ T cells and monocytes) isoform, which further results in the impaired IL-6R response (Ferreira et al., 2013). The protein TYK2, which belongs to the Janus kinase superfamily (JAKs), interacts with the intracellular domain portion of the type 1 interferon receptor. Other receptors such as IL-6, IL-10, IL-12, and IFNλ are also known to interact with TYK2 (Ghoreschi et al., 2009). The rs34536443 variant that causes the Proline to Alanine substitution at the 1104th amino acid, occurs in the αFG helix of TYK2. Experimental studies observed that the TYK2, P1104A variant influences the enzyme activity of the protein and thereby also the associated cytokine pathways (Li et al., 2013). The modified TYK2 activity may exacerbate T helper cell polarization across bone joints in individuals that carry the RA associated AlaTYK2 allele.
Recent decades have seen a surge in the discovery of different variant filtering computational methods to analyze the GWAS data (Hou and Zhao, 2013). Most of these better performing methods align multiple protein sequences and judge the functional relevance of residues based on their evolutionary conservation across different species. Detailed comparisons of different variant prediction methods (SIFT, Polyphen, CADD, and FATHMM) and their prediction sensitivities are reviewed elsewhere (Cooper and Shendure, 2011; Miosge et al., 2015; Lu et al., 2016). Although, W620R of PTPN22, D358A of IL6R and P1104A of TYK2 variants are known to have very strong genome wide significant associations (≥P-value threshold of 5 × 10−8) with the RA risk, computational methods like SIFT, Polyphen-2, CADD, and FATHMM have predicted them to be non-deleterious. These findings underline that nucleotide variant prediction methods are not always sensitive in predicting the pathogenic potential of clinically significant variants. This could be due to the differences in datasets used in training these variant prediction programs (Al-Abbasi et al., 2018). We have therefore further investigated the impact of RA mutations on structural features of proteins.
It is noteworthy to mention that disease can arise if missense mutation leads to the loss or gain of critical functions, due to the altered conformation of secondary structures. Secondary structures are the most common energetically favorable polypeptide structures, which further folds to form super secondary structures, domains, motifs and tertiary structures (Khan and Vihinen, 2007). We have investigated the existence, position and distribution of three missense mutations in secondary structures of those corresponding proteins. Overall, the secondary structural analysis results inferred that out of three deleterious mutations of RA (PTPN22-Trp620Arg; IL6R-Asp358Ala; and TYK2-Pro1104Ala), only the Trp620Arg (located in loop region) mutant brought drastic changes that disturbed the secondary structure of PTPN22. The substitution of the arginine residue at the 620th position in PTPN22, resulted in the gain of extra sheets (two β-hairpins and one β-bulge), helices (four helixes and three helix–helix interactions) and turns (two γ-turns and one disulfide bond) and the loss of one β-sheet and two strands. Since changes in the secondary structural elements were observed; we can expect some promising changes in the pattern of hydrogen bonds between amino hydrogen and carboxyl oxygen atoms in the peptide backbone of PTPN22, which may result in the alteration of dihedral angles in the protein structure.
Our three-dimensional protein modeling and structural equivalency analysis observed huge changes in cavity volume and amino residue RMSD scores of Arg620 (2.85 Å) compared to the Trp620 of PTPN22. Following this trend, even IL6R (D358V) and TYK2 (P1104A) variants have also shown similar structural changes. The degree of protein structure difference caused by mutant amino acid residues, in general, corresponds to their biophysical and chemical properties such as charge, size, molecular weight, hydrophobic nature and chemical side chains (Miao and Cao, 2016). These structural aberrations may, in turn, affect H-bond, ionic, and Vander wall interactions required to uphold the secondary (α helix and β-sheets), tertiary (3D) and quaternary (biomolecular complexes) structural features of RA proteins. The specific function of a protein is related to the interaction of its exposed surface with a solvent. Amino acid residues that form the hydrophobic core of a protein are critical for its stability, therefore they are the site of deleterious mutations. Our predictions show that the Arginine residue at the 620th position of the PTPN22 protein, and the Valine residue at the 358th of IL6R, and the Proline residue at the 1,104th of TYK2 changes the physical orientation and solvent accessibility of the concerned protein molecules. A change, whether an increase or decrease in the surface accessible area to solvents, is determined by the physical orientation of the amino acid (exposed or buried), which could in turn affect the tertiary structure of the proteins (Ramsey et al., 2011). The consensus output of the mutation Cutoff Scanning Matrix and Site Directed Mutator methods revealed the negative Gibbs free energy (DDG) changes for W620R of PTPN22, D358V of IL6R and P1104A of the TYK2 mutations. Negative free energy changes (namely the ΔΔG sign) shifts the stability of proteins to destabilization.
Most disease associated mutations not only interfere with the structural conformation and stability of protein structures, but also induce changes in the molecular binding energies (Hijikata et al., 2017). We therefore further studied the impact of three RA missense mutations in the context of intermolecular interactions. Our protein networking analysis of three RA proteins, i.e., PTPN22, IL-6RA, and TYK2 highlighted their functional interlinking with several immune system molecules, reinforcing the centrality of immune dysfunction in the pathogenesis of RA. Our molecular docking results show that RA missense mutations contribute to altered interactions with ligand molecules, primarily because of the shift in interacting amino acid residues. For molecular docking, we used the Hex Sever, which conveniently performs high quality exhaustive rigid body docking predictions. It takes only ∼15 s to perform 6D docking calculations with this server (Macindoe et al., 2010). All three proteins analyzed, PTPN22 (W620R variant) IL6R (D358V variant) and TYK2 (P1104 variant) were predicted to manifest shifts in the interacting amino acid residues and ligand binding energy values with their corresponding ligand molecules. Furthermore, these three SNPs may also indirectly influence the RA development through the expression of the genes located in the regions of their under-linkage disequilibrium.
Conclusion
In the present study, the difference between variant prediction methods (pathogenicity predictions, 3D protein structure mapping and alterations in molecular interaction abilities) in classifying the three best known RA missense mutations was shown. We noticed that simple nucleotide predictions of SIFT, PolyPhen, CADD, and FATHMM yielded mixed findings when screening the clinically potential variants. The underlying reason for these diverse prediction outcomes could be due to the differences in datasets used in training these variant prediction programs. In fact, a comprehensive multidirectional screening ranging from secondary structure analysis, 3-D modeling, structure equivalency, surface accessibility and stability analysis, protein networking analysis, to molecular docking analysis will provide a more realistic prediction of variant effects. However, the variant prediction test outcomes require validation by functional biology assays.
Data Availability
Publicly available datasets were analyzed in this study. This data can be found here: https://www.ebi.ac.uk/gwas/.
Author Contributions
NS and BB: conceptualization, formal analysis, investigation, methodology, resources, supervision, validation, writing original draft, and writing review and editing. BB: data curation, software, and visualization. NS: funding acquisition and project administration.
Funding
This work was supported by the Deanship of Scientific Research (DSR) at King Abdulaziz University, Jeddah, under grant no. 140-972-D1435.
Conflict of Interest Statement
The authors declare that the research was conducted in the absence of any commercial or financial relationships that could be construed as a potential conflict of interest.
Acknowledgments
The authors gratefully acknowledge the DSRs technical and financial support.
Footnotes
References
Adzhubei, I., Jordan, D. M., and Sunyaev, S. R. (2013). Predicting functional effect of human missense mutations using PolyPhen-2. Curr. Protoc. Hum. Genet. 76, 7.20.1–7.20.41. doi: 10.1002/0471142905.hg0720s76
Al-Abbasi, F. A., Mohammed, K., Sadath, S., Banaganapalli, B., Nasser, K., and Shaik, N. A. (2018). Computational protein phenotype characterization of IL10RA mutations causative to early onset inflammatory bowel disease (IBD). Front. Genet. 9:146. doi: 10.3389/fgene.2018.00146
Angelotti, F., Parma, A., Cafaro, G., Capecchi, R., Alunno, A., and Puxeddu, I. (2017). One year in review 2017: pathogenesis of rheumatoid arthritis. Clin. Exp. Rheumatol. 35, 368–378.
Anwer, F., Yun, S., Nair, A., Ahmad, Y., Krishnadashan, R., and Deeg, H. J. (2015). Severe refractory immune thrombocytopenia successfully treated with high-dose pulse cyclophosphamide and eltrombopag. Case Rep. Hematol. 2015:583451. doi: 10.1155/2015/583451
Banaganapalli, B., Mohammed, K., Khan, I. A., Al-Aama, J. Y., Elango, R., and Shaik, N. A. (2016). A computational protein phenotype prediction approach to analyze the deleterious mutations of human MED12 gene. J. Cell. Biochem. 117, 2023–2035. doi: 10.1002/jcb.25499
Bayley, R., Kite, K. A., Mcgettrick, H. M., Smith, J. P., Kitas, G. D., Buckley, C. D., et al. (2015). The autoimmune-associated genetic variant PTPN22 R620W enhances neutrophil activation and function in patients with rheumatoid arthritis and healthy individuals. Ann. Rheum. Dis. 74, 1588–1595. doi: 10.1136/annrheumdis-2013-204796
Chaudhari, K., Rizvi, S., and Syed, B. A. (2016). Rheumatoid arthritis: current and future trends. Nat. Rev. Drug Discov. 15, 305–306. doi: 10.1038/nrd.2016.21
Cooper, G. M., and Shendure, J. (2011). Needles in stacks of needles: finding disease-causal variants in a wealth of genomic data. Nat. Rev. Genet. 12, 628–640. doi: 10.1038/nrg3046
Cunningham, F., Moore, B., Ruiz-Schultz, N., Ritchie, G. R., and Eilbeck, K. (2015). Improving the sequence ontology terminology for genomic variant annotation. J. Biomed. Semantics 6:32. doi: 10.1186/s13326-015-0030-4
Diaz-Gallo, L. M., and Martin, J. (2012). PTPN22 splice forms: a new role in rheumatoid arthritis. Genome Med. 4:13. doi: 10.1186/gm312
Ek, W. E., D’amato, M., and Halfvarson, J. (2014). The history of genetics in inflammatory bowel disease. Ann. Gastroenterol. 27, 294–303.
Fazal, S. A., Khan, M., Nishi, S. E., Alam, F., Zarin, N., Bari, M. T., et al. (2018). A clinical update and global economic burden of rheumatoid arthritis. Endocr. Metab. Immune Disord Drug Targets 18, 98–109. doi: 10.2174/1871530317666171114122417
Ferreira, R. C., Freitag, D. F., Cutler, A. J., Howson, J. M., Rainbow, D. B., Smyth, D. J., et al. (2013). Functional IL6R 358Ala allele impairs classical IL-6 receptor signaling and influences risk of diverse inflammatory diseases. PLoS Genet. 9:e1003444. doi: 10.1371/journal.pgen.1003444
Ghoreschi, K., Laurence, A., and O’shea, J. J. (2009). Janus kinases in immune cell signaling. Immunol. Rev. 228, 273–287. doi: 10.1111/j.1600-065X.2008.00754.x
Hijikata, A., Tsuji, T., Shionyu, M., and Shirai, T. (2017). Decoding disease-causing mechanisms of missense mutations from supramolecular structures. Sci. Rep. 7:8541. doi: 10.1038/s41598-017-08902-1
Hou, L., and Zhao, H. (2013). A review of post-GWAS prioritization approaches. Front. Genet. 4:280. doi: 10.3389/fgene.2013.00280
Janson, G., Zhang, C., Prado, M. G., and Paiardini, A. (2017). PyMod 2.0: improvements in protein sequence-structure analysis and homology modeling within PyMOL. Bioinformatics 33, 444–446. doi: 10.1093/bioinformatics/btw638
Jostins, L., Ripke, S., Weersma, R. K., Duerr, R. H., Mcgovern, D. P., Hui, K. Y., et al. (2012). Host-microbe interactions have shaped the genetic architecture of inflammatory bowel disease. Nature 491, 119–124. doi: 10.1038/nature11582
Khan, S., and Vihinen, M. (2007). Spectrum of disease-causing mutations in protein secondary structures. BMC Struct. Biol. 7:56. doi: 10.1186/1472-6807-7-56
Klausen, M. S., Jespersen, M. C., Nielsen, H., Jensen, K. K., Jurtz, V. I., Soenderby, C. K., et al. (2019). NetSurfP-2.0: Improved prediction of protein structural features by integrated deep learning. Proteins. doi: 10.1002/prot.25674
Knevel, R., Huizinga, T. W. J., and Kurreeman, F. (2017). Genomic influences on susceptibility and severity of rheumatoid arthritis. Rheum. Dis. Clin. North Am. 43, 347–361. doi: 10.1016/j.rdc.2017.04.002
Laskowski, R. A., Rullmannn, J. A., Macarthur, M. W., Kaptein, R., and Thornton, J. M. (1996). AQUA and PROCHECK-NMR: programs for checking the quality of protein structures solved by NMR. J. Biomol. NMR 8, 477–486. doi: 10.1007/BF00228148
Li, Z., Gakovic, M., Ragimbeau, J., Eloranta, M. L., Ronnblom, L., Michel, F., et al. (2013). Two rare disease-associated Tyk2 variants are catalytically impaired but signaling competent. J. Immunol. 190, 2335–2344. doi: 10.4049/jimmunol.1203118
Lindahl, E., Azuara, C., Koehl, P., and Delarue, M. (2006). NOMAD-Ref: visualization, deformation and refinement of macromolecular structures based on all-atom normal mode analysis. Nucleic Acids Res. 34, W52–W56. doi: 10.1093/nar/gkl082
Lu, Q., Yao, X., Hu, Y., and Zhao, H. (2016). GenoWAP: GWAS signal prioritization through integrated analysis of genomic functional annotation. Bioinformatics 32, 542–548. doi: 10.1093/bioinformatics/btv610
Macindoe, G., Mavridis, L., Venkatraman, V., Devignes, M. D., and Ritchie, D. W. (2010). HexServer: an FFT-based protein docking server powered by graphics processors. Nucleic Acids Res. 38, W445–W449. doi: 10.1093/nar/gkq311
Manolio, T. A. (2010). Genomewide association studies and assessment of the risk of disease. N. Engl. J. Med. 363, 166–176. doi: 10.1056/NEJMra0905980
Marchler-Bauer, A., Lu, S., Anderson, J. B., Chitsaz, F., Derbyshire, M. K., Deweese-Scott, C., et al. (2011). CDD: a conserved domain database for the functional annotation of proteins. Nucleic Acids Res. 39, D225–D229. doi: 10.1093/nar/gkq1189
Menard, L., Saadoun, D., Isnardi, I., Ng, Y. S., Meyers, G., Massad, C., et al. (2011). The PTPN22 allele encoding an R620W variant interferes with the removal of developing autoreactive B cells in humans. J. Clin. Invest. 121, 3635–3644. doi: 10.1172/JCI45790
Miao, Z., and Cao, Y. (2016). Quantifying side-chain conformational variations in protein structure. Sci. Rep. 6:37024. doi: 10.1038/srep37024
Miosge, L. A., Field, M. A., Sontani, Y., Cho, V., Johnson, S., Palkova, A., et al. (2015). Comparison of predicted and actual consequences of missense mutations. Proc. Natl. Acad. Sci. U S A. 112, E5189–E5198. doi: 10.1073/pnas.1511585112
Mohajer, F. S., Parvizpour, S., Razmara, J., Khoshkhooy Yazdi, M., and Shamsir, M. S. (2017). Structural, functional and molecular dynamics analysis of the native and mutated actin to study its effect on congenital myopathy. J. Biomol. Struct. Dyn. 35, 1608–1614. doi: 10.1080/07391102.2016.1190299
Ng, P. C., and Henikoff, S. (2006). Predicting the effects of amino acid substitutions on protein function. Ann. Rev. Genomics Hum. Genet. 7, 61–80. doi: 10.1146/annurev.genom.7.080505.115630
Okada, Y., Wu, D., Trynka, G., Raj, T., Terao, C., Ikari, K., et al. (2014). Genetics of rheumatoid arthritis contributes to biology and drug discovery. Nature 506, 376–381. doi: 10.1038/nature12873
Petersen, B., Petersen, T. N., Andersen, P., Nielsen, M., and Lundegaard, C. (2009). A generic method for assignment of reliability scores applied to solvent accessibility predictions. BMC Struct. Biol. 9:51. doi: 10.1186/1472-6807-9-51
Pires, D. E., Ascher, D. B., and Blundell, T. L. (2014). DUET: a server for predicting effects of mutations on protein stability using an integrated computational approach. Nucleic Acids Res. 42, W314–W319. doi: 10.1093/nar/gku411
Ramsey, D. C., Scherrer, M. P., Zhou, T., and Wilke, C. O. (2011). The relationship between relative solvent accessibility and evolutionary rate in protein evolution. Genetics 188, 479–488. doi: 10.1534/genetics.111.128025
Rentzsch, P., Witten, D., Cooper, G. M., Shendure, J., and Kircher, M. (2018). CADD: predicting the deleteriousness of variants throughout the human genome. Nucleic Acids Res. 47, D886–D894. doi: 10.1093/nar/gky1016
Ritchie, D. W. (2003). Evaluation of protein docking predictions using Hex 3.1 in CAPRI rounds 1 and 2. Proteins 52, 98–106. doi: 10.1002/prot.10379
Rodriguez-Elias, A. K., Maldonado-Murillo, K., Lopez-Mendoza, L. F., and Ramirez-Bello, J. (2016). [Genetics and genomics in rheumatoid arthritis (RA): an update]. Gac. Med. Mex. 152, 218–227.
Sanchez, R., and Sali, A. (2000). Comparative protein structure modeling. Introduction and practical examples with modeller. Methods Mol. Biol. 143, 97–129. doi: 10.1385/1-59259-368-2:97
Shaik, N. A., Awan, Z. A., Verma, P. K., Elango, R., and Banaganapalli, B. (2018). Protein phenotype diagnosis of autosomal dominant calmodulin mutations causing irregular heart rhythms. J. Cell. Biochem. 119, 8233–8248. doi: 10.1002/jcb.26834
Shaik, N. A., Kaleemuddin, M., Banaganapalli, B., Khan, F., Shaik, N. S., Ajabnoor, G., et al. (2014). Structural and functional characterization of pathogenic non- synonymous genetic mutations of human insulin-degrading enzyme by in silico methods. CNS Neurol. Disord. Drug Targets 13, 517–532. doi: 10.2174/18715273113126660161
Shihab, H. A., Gough, J., Cooper, D. N., Stenson, P. D., Barker, G. L., Edwards, K. J., et al. (2013). Predicting the functional, molecular, and phenotypic consequences of amino acid substitutions using hidden Markov models. Hum. Mutat. 34, 57–65. doi: 10.1002/humu.22225
Smolen, J. S., Beaulieu, A., Rubbert-Roth, A., Ramos-Remus, C., Rovensky, J., Alecock, E., et al. (2008). Effect of interleukin-6 receptor inhibition with tocilizumab in patients with rheumatoid arthritis (OPTION study): a double-blind, placebo-controlled, randomised trial. Lancet 371, 987–997. doi: 10.1016/S0140-6736(08)60453-5
Sneha, P., and Doss, C. G. (2016). Molecular dynamics: new frontier in personalized medicine. Adv. Protein Chem. Struct. Biol. 102, 181–224. doi: 10.1016/bs.apcsb.2015.09.004
Stanford, S. M., Mustelin, T. M., and Bottini, N. (2010). Lymphoid tyrosine phosphatase and autoimmunity: human genetics rediscovers tyrosine phosphatases. Semin. Immunopathol. 32, 127–136. doi: 10.1007/s00281-010-0201-4
Szklarczyk, D., Morris, J. H., Cook, H., Kuhn, M., Wyder, S., Simonovic, M., et al. (2017). The STRING database in 2017: quality-controlled protein-protein association networks, made broadly accessible. Nucleic Acids Res. 45, D362–D368. doi: 10.1093/nar/gkw937
Vaser, R., Adusumalli, S., Leng, S. N., Sikic, M., and Ng, P. C. (2016). SIFT missense predictions for genomes. Nat. Protoc. 11, 1–9. doi: 10.1038/nprot.2015.123
Webb, B., and Sali, A. (2017). Protein structure modeling with MODELLER. Methods Mol. Biol. 1654, 39–54. doi: 10.1007/978-1-4939-7231-9_4
Yang, J., Yan, R., Roy, A., Xu, D., Poisson, J., and Zhang, Y. (2015). The I-TASSER suite: protein structure and function prediction. Nat. Methods 12, 7–8. doi: 10.1038/nmeth.3213
Yang, J., and Zhang, Y. (2015). I-TASSER server: new development for protein structure and function predictions. Nucleic Acids Res. 43, W174–W181. doi: 10.1093/nar/gkv342
Keywords: deleterious mutations, rheumatoid arthritis, molecular analysis, protein modeling, biological network
Citation: Shaik NA and Banaganapalli B (2019) Computational Molecular Phenotypic Analysis of PTPN22 (W620R), IL6R (D358A), and TYK2 (P1104A) Gene Mutations of Rheumatoid Arthritis. Front. Genet. 10:168. doi: 10.3389/fgene.2019.00168
Received: 16 October 2018; Accepted: 15 February 2019;
Published: 07 March 2019.
Edited by:
Abjal Pasha Shaik, King Saud University, Saudi ArabiaReviewed by:
Jaffer Mohiddin, Universidad de las Fuerzas Armadas – ESPE, EcuadorNeeraja Reddy Matavalam, Sri Venkateswara Veterinary University, India
Copyright © 2019 Shaik and Banaganapalli. This is an open-access article distributed under the terms of the Creative Commons Attribution License (CC BY). The use, distribution or reproduction in other forums is permitted, provided the original author(s) and the copyright owner(s) are credited and that the original publication in this journal is cited, in accordance with accepted academic practice. No use, distribution or reproduction is permitted which does not comply with these terms.
*Correspondence: Babajan Banaganapalli, YmJhYmFqYW5Aa2F1LmVkdS5zYQ==; amFhbm5hd2F6QGdtYWlsLmNvbQ==