- 1Teagasc, Food Research Centre, Ashtown, Ireland
- 2Leibniz Institute for Farm Animal Biology (FBN), Institute for Genome Biology, Dummerstorf, Germany
- 3Teagasc, Pig Production Department, AGRIC, Moorepark, Fermoy, Co. Cork, Ireland
- 4Faculty of Agricultural and Environmental Sciences, University Rostock, Rostock, Germany
Liver is a metabolically complex organ that influences nutrient partitioning and potentially modulates the efficiency of converting energy acquired from macronutrients ingestion into a muscle and/or adipose tissue (referred to as feed efficiency, FE). The objective of this study was to sequence the hepatic tissue transcriptome of closely related but differently feed efficient pigs (n = 16) and identify relevant biological processes that underpin the differences in liver phenotype between FE groups. Liver weight did not significantly differ between the FE groups, however, blood parameters showed that total protein, glucose, cholesterol and percentage of lymphocytes were significantly greater in high-FE pigs. Ontology analysis revealed carbohydrate, lipid and protein metabolism to be significantly enriched with differentially expressed genes. In particular, high-FE pigs exhibited gene expression patterns suggesting improved absorption of carbohydrates and cholesterol as well as enhanced reverse cholesterol transport. Furthermore, the inferred decrease in bile acid synthesis in high-FE pigs may contribute to the observed greater levels of serum glucose, which can be then delivered to cells and utilized for growth and maintenance. Gene ontology analysis also suggested that livers of more efficient pigs may be characterized by higher protein turnover and increased epithelial cell differentiation, whereby an enhanced quantity of invariant natural killer T-cells and viability of natural killer cells could induce a quicker and more effective hepatic response to inflammatory stimuli. Our findings suggest that this prompt hepatic response to inflammation in high-FE group may contribute to the more efficient utilization of nutrients for growth in these animals.
Introduction
Liver is a central organ for systemic metabolism (Rui, 2014; Shimizu et al., 2015) and plays an important role in modulating the efficiency of converting energy acquired from macronutrients into muscle and/or adipose tissue affecting feed efficiency (FE). Energy from ingested macronutrients can be stored by the liver in the form of glycogen, which during food deprivation is broken down into glucose and delivered to the bloodstream (Sherwin, 1980; Zhang et al., 2014). Liver can also convert energy from a dietary source to triacylglycerol and export it by very low density lipoproteins (VLDL) either to muscle for use there or to adipose tissue for storage (Gruffat et al., 1996). Moreover liver is a key organ for synthesis of cholesterol, a vital constituent of cell membrane, and lipoproteins that function as cholesterol transporting particles (Charlton-Menys and Durrington, 2008). Low density lipoproteins (LDL) deliver cholesterol to peripheral organs, whilst high density lipoproteins (HDL) transport excess cholesterol from these tissues back to the liver (Feingold and Grunfeld, 2000), which is then utilized, e.g., for synthesis of bile acids that enable intestinal absorption of dietary fats (Boyer, 2013).
Being continually subjected to antigens entering from the gut via blood supply, liver also exhibits immunological properties (Gao, 2016; Peng et al., 2016). The lymphocyte population of the liver is primarily represented by macrophages, natural killer and natural killer T cells that are involved in innate immune defense and regulation of liver regeneration (Racanelli and Rehermann, 2006; Gao, 2016). Fueling immune response is an energetically expensive process resulting in less nutrients available for growth (Patience et al., 2015). This alteration in prioritizing nutrients toward stimulating immune response would be suspected to negatively impact animal’s FE. Nevertheless, it has been postulated that high-FE animals have a more efficient immune response to fight off inflammation and therefore more energy available for growth and muscle deposition (Paradis et al., 2015).
Transcriptomic approach is a relevant tool in developing a deeper understanding of the physiological processes in liver which may be related to FE. To date only a few studies have analyzed the transcriptome of liver from FE-divergent pigs wherein shifts in biological processes that were observed to be differentially regulated in association with FE included cell proliferation, vitamin A metabolism, protein synthesis and catabolism, lipid and carbohydrate metabolism, reverse cholesterol transport, integrin signaling, as well as oxidative stress, inflammation and immune responses (Zhao et al., 2016; Gondret et al., 2017; Reyer et al., 2017; Ramayo-Caldas et al., 2018). Additionally, another study investigated mitochondrial proteomics of liver from pigs divergent in FE and as a result identified a shift in mitochondrial protein profile (Grubbs et al., 2013). Although these studies have offered insights on the regulation of FE via liver physiology, the biological processes governing the differences in FE are not fully elucidated and further research is needed. Hence the purpose of the present study was to characterize phenotypes relevant to liver physiology and to perform RNA sequencing of liver tissue in pigs divergent for FE to gain deeper insights into differences in hepatic transcriptome architecture and its relationship to liver function in more efficient pigs.
Materials and Methods
Ethics Statement
The care, slaughter and sample collection of the animals fulfilled the national regulations of animal research and commercial slaughtering and were approved by the Teagasc Animal Ethics Committee for the care and use of animals.
Animals and Experimental Design
A total of 138 Maxgro (Hermitage Genetics) × (Landrace × Large White) pigs were used in this study. Housing, diets, and selection on FE were as previously described (Horodyska et al., 2018a). Briefly, residual feed intake (RFI, a measure of FE defined as the difference between actual feed intake and predicted feed requirements) was calculated after day 120 of age as the residual from a least squares regression model of average daily feed intake on average daily gain, metabolic live weight, gender and also all relevant two-way interactions, and the effects of back-fat and muscle depth using the PROC REG procedure in SAS (version 9.4; SAS Inst. Inc., Cary, NC, United States). According to RFI values, pigs were assigned within litter and gender as high (H) and low (L) RFI. The minimum and maximum RFI values (g/day) for the 138 pigs were -329 and 494, respectively. A total of 40 pigs (20 extremes from LRFI (high-FE) group – 10 males and 10 females, and 20 extremes from HRFI (low-FE) group – 10 males and 10 females), with an average body weight of 99 kg, were sampled considering the relatedness of pigs. The mean RFI (g/day) of the LRFI and HRFI pigs was -100.2 (SD: 97.9) and 150.7 (SD: 163.3), respectively.
Phenotypic Measurements
For each animal (n = 40), liver weights were recorded and blood samples were collected in vacuette tubes (ROI: Labstock, Dublin, Ireland; AT: Sarstedt, Nürnbrecht, Germany) during slaughter. For biochemical analysis, upon allowing the blood to clot at room temperature, the samples were centrifuged at 1,500 × g for 10 min and the serum was collected and stored at -80°C until analyzed. Creatinine, creatine kinase, total protein, blood urea nitrogen, triglycerides, glucose and cholesterol were analyzed with a calibrated ABS Pentra 400 clinical chemistry analyzer (Horiba, ABX, North Hampton, United Kingdom). In order to determine analyzer accuracy, every fifth sample was run in duplicate. For hematological analysis, blood was treated with EDTA to prevent clotting. It was then subjected to analysis, within 4 h of sample collection, whereby white blood cells, lymphocytes, monocytes, granulocytes, red blood cells, red blood cell distribution width, hemoglobin, hematocrit, mean corpuscular volume, mean corpuscular hemoglobin, platelets and mean platelet volume were measured with a Beckman Coulter Ac T Diff analyzer (Beckman Coulter Ltd., High Wycombe, United Kingdom). The PROC MIXED procedure in the SAS was used to evaluate associations between FE and liver weight as well as biochemical and hematological parameters in the Maxgro × (Landrace × Large White) pigs (n = 40). The model included RFI group as a fixed effect, slaughter day as a random effect, and the absolute values of RFI as a weight statement. Additionally for liver weight, final live body weight was incorporated in the model as a covariate. Moreover, correlations between RFI and hematological and biochemical parameters were determined using the PROC CORR procedure in the SAS system (version 9.4; SAS Inst. Inc., Cary, NC, United States).
RNA Sequencing of Liver Samples, Data Processing, and Ontology Analysis
Samples of the right liver lobe (Lobus Spigelii) tissue were collected and snap frozen in liquid nitrogen within 10 min post-mortem followed by storage at -80°C until RNA isolation. Of these 40 liver tissues from RFI-divergent pigs, 16 samples from four sets of full siblings were selected and each set consisted of 2 males – 1 LRFI (high-FE) and 1 HRFI (low-FE) and 2 females 1 LRFI (high-FE) and 1 HRFI (low-FE) so that 8 LRFI (high-FE) pigs – 4 males and 4 females and 8 HRFI pigs (low-FE) – 4 males and 4 females were analyzed. Total RNA of these liver samples was isolated with Tri-Reagent (Sigma-Alrich, Taufkirchen, Germany), and subjected to DNase treatment and a column-based purification (Nucleospin RNA II kit, Macherey-Nagel, Düren, Germany). Total RNA was used as input for the library preparation according to the TruSeq Stranded mRNA protocol (Illumina, San Diego, CA, United States). Subsequently, sequencing was performed on an Illumina HiSeq2500 generating paired-end reads. Reads were mapped to the reference (Ensembl release 84) using TopHat (2.1.0) (Kim et al., 2013) and read counts were assigned to the gene features employing HTSeq 0.6.1 (Anders et al., 2015). The assessment of the differentially expressed genes included RFI groups and slaughter dates as fixed effects and was performed using the Wald test implemented in DESeq2 (3.4.0)1. To integrate gene expression data, the list of DE genes (P < 0.01) and corresponding fold changes were passed to Ingenuity Pathway Analysis (IPA; Ingenuity®Systems)2 and significantly enriched bio-functions and canonical pathways (P < 0.01) were extracted. They were considered significantly activated and inhibited at an absolute z-score greater than 2. In addition, potential important interaction networks enriched with DE genes were generated using the Ingenuity® Knowledge Base.
Validation of RNA-Seq Through Quantitative Real-Time PCR (qPCR)
Following cDNA synthesis from 1 μg of total RNA and in presence of random primers (Promega, Mannheim, Germany), oligo (dT) primer and Superscript®III reverse transcriptase (Invitrogen Corp., San Diego, CA, United States), qPCR were carried out on a LightCycler 96 system (Roche Mannheim, Germany). Gene-specific primers (Table 1) were designed using the Primer-BLAST software3 and the BLAST search tool database4. PCR reactions were carried out in a final volume of 12 μl consisting of 2 μl cDNA, 6 μl SYBR Green I Master (Roche), 0.6 μl (10 μM) of each forward and reverse primer, and 2.8 μl qPCR grade water (Roche). After an initial denaturation at 95°C for 5 min, 45 cycles of amplification followed (95°C for 10 s, 60°C for 15 s and 72°C for 25 s). Melting curve analysis was performed at the end of the amplification to verify the specificity of all amplification reactions. RPL32 expression values were used to normalize qPCR results. Subsequently, qPCR data was analyzed in a mixed model including RFI group as a fixed effect and slaughter date as a random effect (lme4; R). The correlation between RNA-seq and qPCR data were assessed in R considering a significance threshold of P < 0.05.
Results
Phenotypic Measurements
Liver weight did not significantly differ between the FE groups (high-FE = 1.62 kg ± 0.04 kg and low-FE = 1.67 kg ± 0.04 kg). The effect of divergence in FE on biochemical and hematological parameters are shown in Table 2. Biochemical analysis of serum revealed that total protein, glucose and cholesterol were significantly (P < 0.05) higher in high-FE pigs compared to low-FE pigs. Creatinine, creatine kinase, blood urea nitrogen and triglycerides did not differ significantly between the groups. Hematological analysis exposed significantly (P < 0.05) reduced number of white blood cells but increased percentage of lymphocytes in high-FE pigs. The number of platelets and mean platelet volume was significantly (P < 0.001 and P < 0.05, respectively) lower in high-FE pigs. Remaining hematological parameters were not significantly altered by FE group. Spearman correlations between phenotypic parameters of pigs divergent in FE are depicted in Table 3. A number of significant correlations at a P < 0.001 were observed between phenotypes. The strongest linear relationships were noted between total protein and cholesterol (r = 0.781), glucose and cholesterol (r = 0.737), red blood cells and hemoglobin (r = 0.726), creatinine and cholesterol (r = 0.723), creatinine and total protein (r = 0.704), total protein and triglycerides (r = 0.629), total protein and glucose (r = 0.608), as well as creatinine and glucose (r = 0.601). This was followed by moderate linear relationships between triglycerides and creatinine (r = 0.566), cholesterol (r = 0.563) and blood urea nitrogen (r = 0.560).
Differentially Expressed Genes and Ontological Interpretation
89.2% of the revealed sequences were successfully mapped to the reference resulting in an average of 105.6 million high quality paired-end reads per sample assigned to 14,910 genes expressed in liver. A total of 922 genes were differentially expressed with a P < 0.01 (Figure 1) corresponding to false discovery rate (q) ≤ 0.16, and of these 818 were annotated (Figure 2 and Supplementary Table S1). Twenty-one molecular and cellular functions and twenty-one physiological system development and function categories were significantly enriched (P < 0.01) amongst the DE genes in relation to FE, as inferred from functional enrichment analysis (Supplementary Tables S2, S3). The highest distribution of all DE genes entries were observed in “cell death and survival” (12%), “cellular development” (11%), “organismal development” (11%), and “organismal survival” (10%). Twenty canonical pathways were significantly associated with DE genes in relation to FE at P < 0.01 (Table 4 and Supplementary Table S4), wherein the highest distribution of all DE gene entries were observed in “protein ubiquitination pathway” (8%) and “role of NFAT in regulation of the immune response” (8%), followed by “EIF2 signaling” (7%), “ILK signaling” (7%), “B cell receptor signaling” (7%), “aldosterone signaling in epithelial cells” (7%), and “gap junction signaling” (7%). Twenty-five networks were obtained upon integration of all DE genes. The most significant network (network 1) contained functions related to gastrointestinal and hepatic system disease and liver cirrhosis. Carbohydrate and lipid metabolism, and small molecule biochemistry were represented by 25 DE molecules in network 12 (Figure 3).
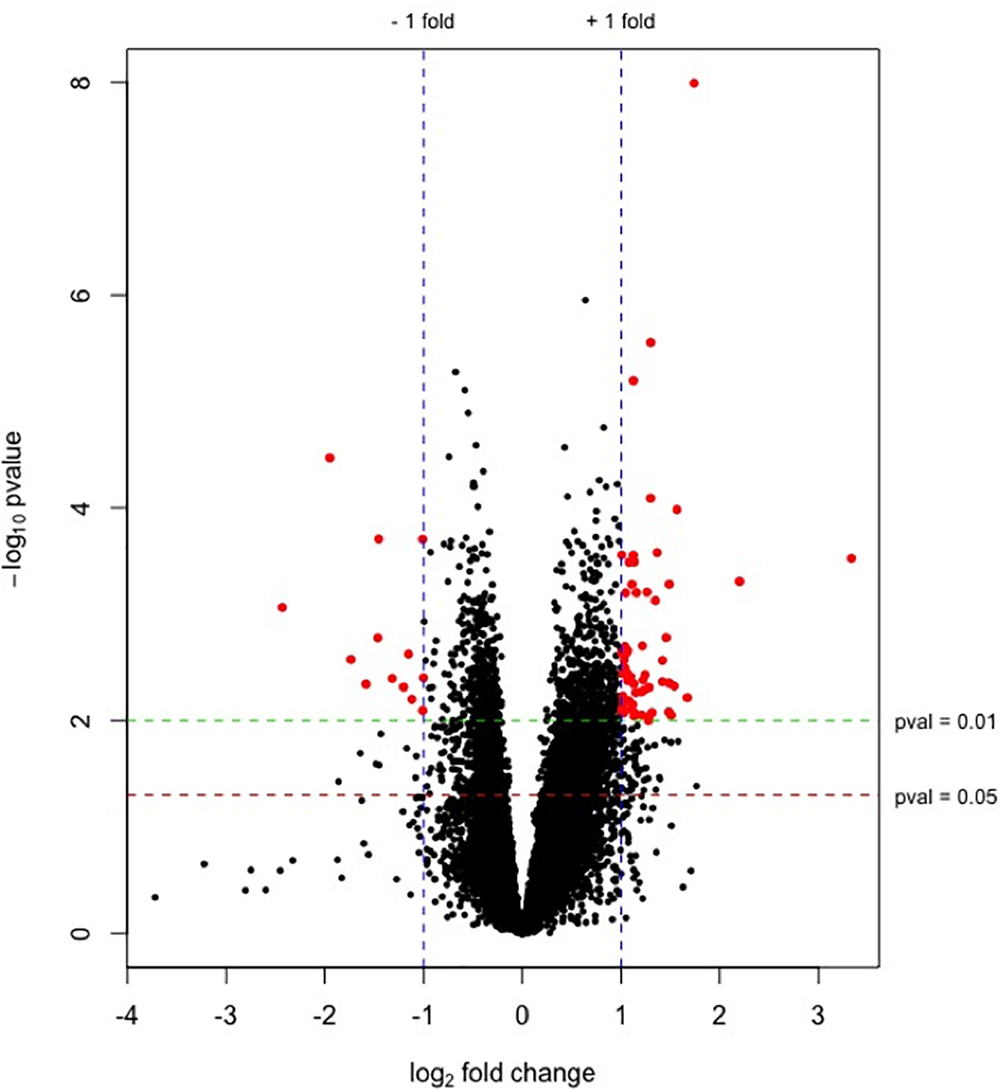
Figure 1. Plot of –log10(p-values) versus log2 fold changes for genes expressed in liver from high-FE pigs. The green and red lines indicate P = 0.01 and 0.05, respectively. Blue lines represent the threshold of genes with a log2 fold change ≥|1| (fold change ≥ |2|). Significantly differentially expressed genes (P < 0.01, log2 fold change ≥|1| [fold change ≥|2|]) are highlighted by red dots. Up-regulated genes in high-FE pigs are indicated by positive fold changes.
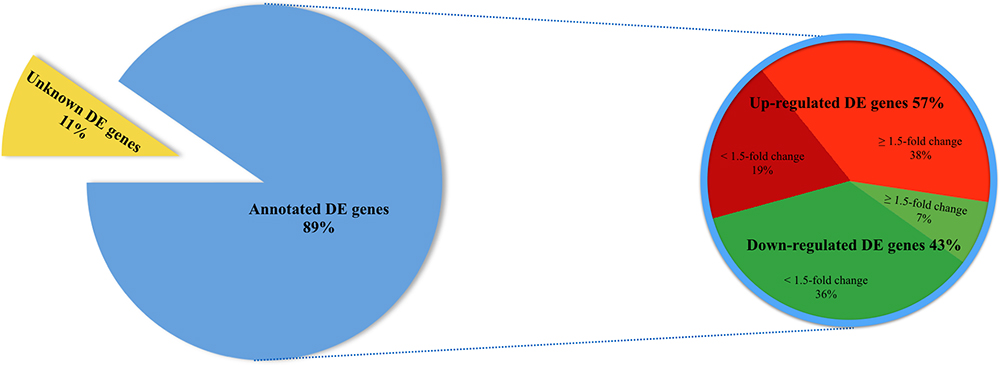
Figure 2. Pie chart depicting a total of 922 differentially expressed (DE) genes (P < 0.01) in liver of FE-divergent pigs and a percentage of annotated up- and down-regulated genes in high-FE pigs. The most up-regulated gene was PON3 (fold change = 10.08) and the most down-regulated gene was CCNT2 (fold change = -5.40) in high FE pigs.
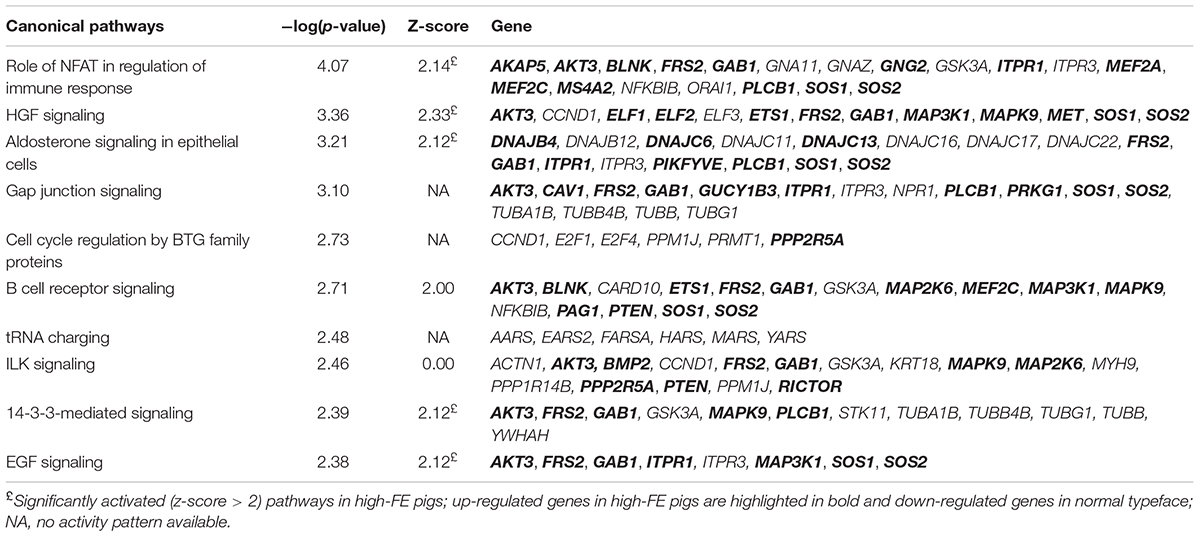
Table 4. Most significantly enriched canonical signaling pathways identified in liver samples of feed efficiency (FE)-divergent pigs.
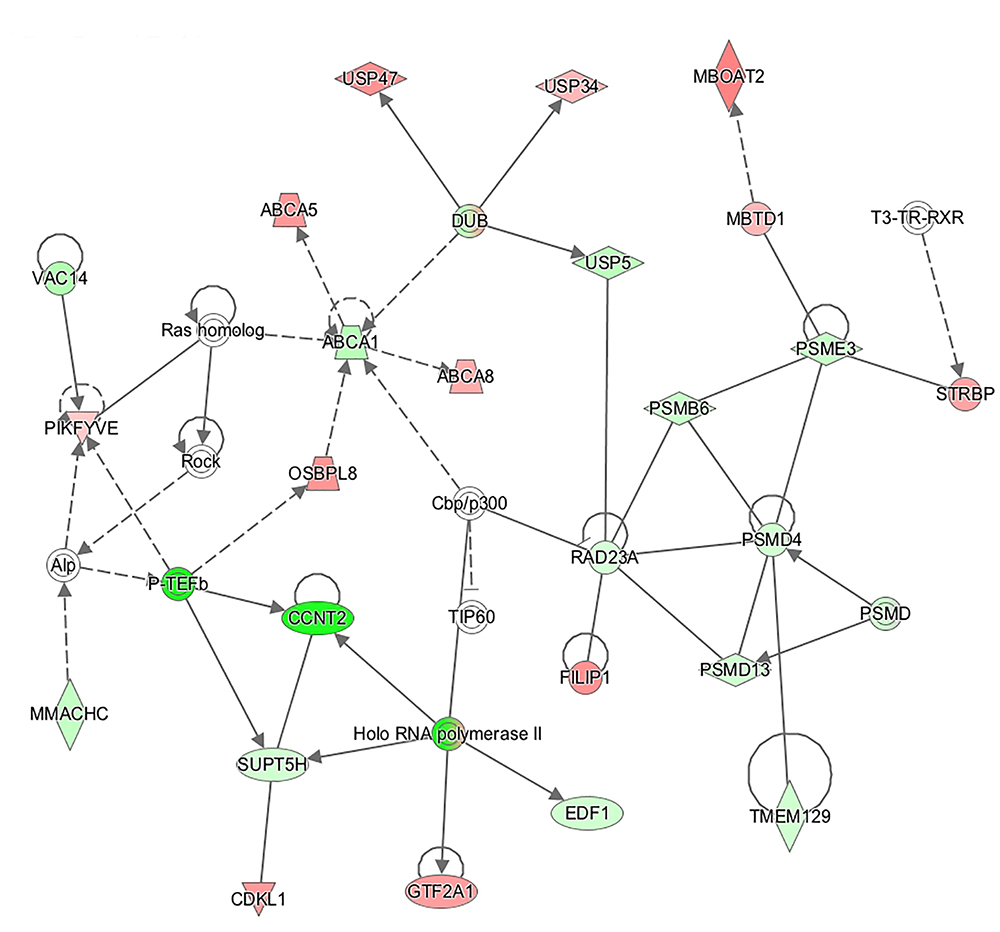
Figure 3. Connection of genes affecting functions related to “carbohydrate metabolism,” “lipid metabolism,” and “small molecule biochemistry” represented in a gene network (network 12). Biological relationship between genes is depicted as an edge/line (solid lines and dashed lines show direct and indirect interactions, respectively). Colors represent up- (red) and down- (green) regulated genes in high-feed efficient pigs.
Verification of RNA-Seq Results
KIT (KIT proto-oncogene receptor tyrosine kinase), and SAA3 (serum amyloid A3), selected randomly, as well as PON3 (paraoxonase 3), selected based on its abundance, were amplified through qPCR. Significant differences in the expression levels of all three measured transcripts between the FE groups were confirmed. Spearman correlations attained by comparing gene expression levels measured using RNA-seq and qPCR, were found to be significant for KIT (r = 0.604, P < 0.05), PON3 (r = 0.968, P < 0.001), and SAA3 (r = 0.946, P < 0.001) (Figure 4).
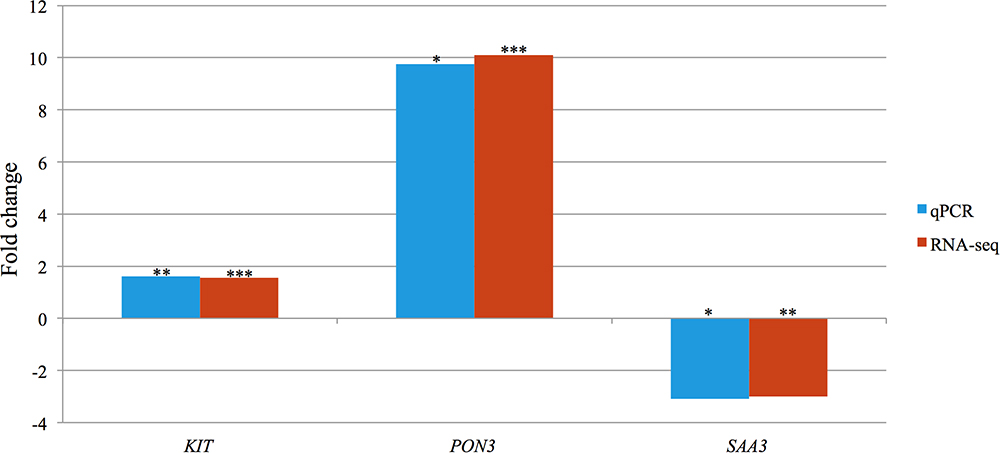
Figure 4. Bar chart portraying the RNA-seq and qPCR fold changes of three selected differentially expressed genes in high-FE pigs. Significance levels of differences affected by feed efficiency: ∗P < 0.05, ∗∗P < 0.01, ∗∗∗P < 0.001.
Discussion
In this study we investigated the hepatic transcriptome of pigs divergent in FE and identified a number of biological functions and pathways affiliated with lipid, protein and carbohydrate metabolism as well as hepatic growth and immune response. These differences help explain the physiological differences associated with divergence in FE and the recorded biochemical and hematological parameters.
Macronutrients Metabolism
Paraoxonase 3 (PON3) was the most up-regulated (fold change = 10.1) gene in high-FE pigs. PON3 codes for an enzyme that associates with HDL (Getz and Reardon, 2004) and prevents oxidation of LDL (Reddy et al., 2001) which otherwise would result in endothelial dysfunction (Leiva et al., 2015). Increased adipose deposition observed in PON3 knockout mice (Shih et al., 2015) is consistent with a potential role of PON3 in promoting lean growth and this is in keeping with decreased intramuscular fat content in the high-FE pigs found in our previous report (Horodyska et al., 2018a). Enrichment of DE genes in lipid metabolism networks was further portrayed by molecule connectivity analysis (Figure 3). Suppression of ATP binding cassette subfamily A member 1 (ABCA1) in mouse liver increased absorption of dietary cholesterol (Oram and Lawn, 2001). In the present study, the network illustrates ABCA1 being down-regulated (fold change = -1.37) in high-FE pigs. Other members of the ABCA family (ABCA5 and ABCA8) were over-expressed (fold change = 1.67 and 1.52, respectively) in high-FE pigs. In mice, abundance of ABCA5 expression was previously associated with increased macrophage cholesterol efflux to HDL (Ye et al., 2010), whilst hepatic abundance of ABCA8 led to significantly increased plasma HDL level and reverse cholesterol transport (Trigueros-Motos et al., 2017). Indeed, serum analysis pointed toward enhanced cholesterol level in high-FE pigs (Table 1). In addition, a positive correlation between serum cholesterol and total protein, which mainly constitutes of albumin, was observed. Interestingly, serum albumin mediates cholesterol efflux and may be a significant player in reverse cholesterol transport (Ha et al., 2003). Moreover, a previous study has also shown reverse cholesterol transport to be over-expressed in the livers of high-FE pigs (Gondret et al., 2017).
“Fibroblast growth factor (FGF) signaling,” which is involved in bile acid metabolism (Ornitz and Itoh, 2015), was predicted to be activated in high-FE livers (z-score = 3.00). In this pathway, fibroblast growth factor receptor substrate 2 (FRS2) was up-regulated (fold change = 1.31) in high-FE pigs. FRS2α deficiency led to increased bile acid synthesis in mouse liver (Wang et al., 2014) therefore it seems probable that high-FE pigs experience decreased bile acid production. In support of this, a previous study showed a lower abundance of genes involved in bile acid metabolism, nuclear receptor subfamily 1 group H member 4 (NR1H4) and squalene epoxidase (SQLE), in the liver of more feed efficient pigs (Reyer et al., 2017). Besides its well established functions, bile acids are also involved in lowering glucose levels (Staels and Fonseca, 2009) and hindering gluconeogenesis (Chai et al., 2015). Differential expression of cholesterol-related genes in the livers of pigs divergent for FE points toward increased absorption of dietary cholesterol and reverse cholesterol transport in high-FE pigs. Therefore, the inferred reduction in bile acid synthesis may be a measure to prevent drops in serum glucose level rather than explained by HDL cholesterol shortage. This presumption is in accordance with the higher glucose and cholesterol concentrations found in the serum of high-FE pigs. Gene ontology analysis also revealed “uptake and conversion of carbohydrates,” enclosed within a broader “carbohydrate metabolism” category, significantly enriched with DE genes and “aldosterone signaling in epithelial cells” significantly activated (z-score = 2.12) in high-FE pigs. Alongside its role in the regulation of sodium absorption (Briet and Schiffrin, 2010), aldosterone was shown to be involved in glucose transport through molecular regulation of SGLT1 (sodium-dependent glucose co-transporter) and GLUT2 (glucose transporter) in the chicken intestine (Garriga et al., 2001). The increased aldosterone signaling may indicate superior metabolic capacity in the liver of high-FE pigs compared to their counterparts (Grubbs et al., 2013).
The increased serum total protein concentration observed in high-FE pigs supports the suggested activation of “protein degradation and trafficking” sub-categories such as “protein catabolism and secretion” (z-score = 2.16 and 1.00, respectively) in the high-FE livers inferred from the functional enrichment analysis. Moreover, the greater serum protein concentration could have stimulated endogenous glucose synthesis (Promintzer and Krebs, 2006), which is consistent with the positive correlation between total protein and glucose concentrations in the serum of FE-divergent pigs. Over-expression of genes involved in protein synthesis and degradation have been reported in livers of high-FE pigs (Gondret et al., 2017). Lobley (2003) postulated that protein synthesis is much more energetically expensive in comparison to protein degradation. In our previous report (Horodyska et al., 2018b), we have suggested that high-FE muscle exhibits increased protein turnover and potentially reuses existing proteins, while directing the conserved energy toward more efficient growth. This phenomenon could also be occurring in the liver of high-FE pigs.
Hepatic Growth
“Hepatocyte growth factor (HGF) signaling,” “epidermal growth factor (EGF) signaling,” and “FGF signaling” were significantly activated pathways (z-score = 2.33, 2.12, and 3.00, respectively) in high-FE pigs. Previous studies revealed a role for growth factors, e.g., HGF, EGF and FGF, in stimulating proliferation and differentiation of hepatic oval cells (Hu et al., 1993; Jones et al., 2009; Sánchez and Fabregat, 2010) and also in liver regeneration (Jiang et al., 1993; Steiling et al., 2003; Zimmers et al., 2017). Over-expression of growth factor receptor bound protein 2-associated protein 1 (GAB1) was a common feature shared between the three pathways. A study in GAB1 knockout mice reported defects in liver regeneration (Bard-Chapeau et al., 2006) and also reduced embryonic liver size (Sachs et al., 2000). AKT serine/threonine kinase 3 (AKT3) was another over-expressed gene enriched in these pathways. Akt3 is a member of AKT kinase family playing a role in modulation of cell survival and proliferation (Xu et al., 2012). Accordingly, “differentiation of epithelial cells,” enclosed within a “cellular development” function, was activated (z-score = 2.13). Moreover a “cell cycle” sub-category, “senescence of cells,” which is characterized by cell cycle arrest leading to loss of its ability to divide (Hoare et al., 2010), was suppressed (z-score = -2.90) in high-FE pigs. In the present study liver weights did not significantly differ between the FE groups, although a previous report found significantly heavier liver weights in high-FE pigs (Reyer et al., 2017). Cyclin T2 (CCNT2), coding for a protein regulating cell differentiation through activation of cyclin-dependent kinase 9 (CDK9) (Simone et al., 2002; Garriga et al., 2003), was the most down-regulated (fold change = -5.40) gene in high-FE pigs. CDK9 also functions in the inflammatory response (Han et al., 2014). Here, CDK9 was down-regulated (fold change = -1.22) in high-FE pigs at a P < 0.05. It is possible that suppression of CCNT2 could influence CDK9 function in differentiation of monocytes (De Falco et al., 2005) rather than hepatic epithelial cells.
Immune Response
The “role of nuclear factor of activated T cells (NFAT) in regulation of the immune response” was significantly activated (z-score = 2.14) in high-FE pigs. NFAT proteins play a role in the first line of defense through regulating innate leukocyte response to inflammatory stimuli (Zanoni and Granucci, 2012). Myocyte enhancer factor 2C (MEF2C), which orchestrates immune cell activation and differentiation (Schuler et al., 2008), was enriched in this pathway and up-regulated in high-FE animals. Additionally, MEF2C belongs to a family of transcriptional factors that acts in conjunction with NFAT (Mancini and Toker, 2009). “Quantity of invariant natural killer T-cells” and “cell viability of natural killer cells,” falling under the broader “hematological system development and function” theme, were also significantly activated (z-score = 2.10 and 2.20, respectively) in high-FE pigs. In these sub-categories, ETS proto-oncogene 1 transcription factor (ETS1) and KIT proto-oncogene receptor tyrosine kinase (KIT) were up-regulated. ETS1 plays an essential role in the development and function of natural killer T cells, a group of cells exhibiting properties of both natural killer cells and T cells (Choi et al., 2011), whilst KIT is crucial for survival and maturation of natural killer cells (Colucci and Di Santo, 2000). Mutations within KIT gene are known to be pleiotropic, meaning that they affect several traits simultaneously and could cause expression bias in various tissues (Venhoranta et al., 2013; Gratten and Visscher, 2016). Therefore, although validation of KIT confirmed significant expression differences in FE-divergent liver, this gene needs to be considered with caution (Papakostas et al., 2014).
Consistent with the gene ontology, hematological analysis found an increased percentage of serum lymphocytes in the high-FE group. It is widely considered that during immune response dietary nutrients are shifted away from growth, which may lower animal’s FE, toward the immune-related processes (Patience et al., 2015). Nevertheless, a prompt response to hepatic pro-inflammatory stimuli may result in less energy consumed for combating systemic inflammation and hence more efficient utilization of nutrients for growth and protein accretion (Paradis et al., 2015). Several studies have reported a diverse hepatic inflammatory response in high- versus low-FE pigs (Gondret et al., 2017) and cattle (Alexandre et al., 2015; Paradis et al., 2015), thereby supporting this connection.
Conclusion
Hepatic nutrient partitioning has a direct influence on the efficiency of energy utilization and potentially plays an important role in FE. In this study, carbohydrate, lipid and protein metabolism were significantly over-represented within the DE genes, confirming the hepatic influence on divergent energy utilization in high- versus low-FE pigs. In particular, high-FE pigs exhibited gene expression patterns suggesting improved hepatic absorption of carbohydrates and cholesterol as well as enhanced reverse cholesterol transport. Furthermore, the inferred decrease in bile acid synthesis in high-FE pigs may contribute to the increased concentrations of serum glucose observed. This increased glucose can be delivered to cells and utilized for increased growth. Gene ontology analysis also suggests that the liver of more feed efficient pigs may be characterized by higher protein turnover and increased epithelial cell differentiation, whilst enhanced quantity of invariant natural killer T-cells and viability of natural killer cells could induce a faster and more effective hepatic response to inflammatory stimuli.
Availability of Data
RNA-seq data generated during the current study are available on ArrayExpress at EMBL-EBI (www.ebi.ac.uk/arrayexpress; accession number: E-MTAB-6256).
Author Contributions
JH collected samples, extracted RNA, prepared libraries, validated RNA-seq via qPCR, carried out data analysis, and wrote the manuscript. RH conceived the experiment and contributed to experimental design, collected samples, and edited the manuscript. HR participated in statistical analysis and edited the manuscript. NT assisted in library preparation, performed the RNA-seq and data analysis, and edited the manuscript. PL provided the animals screened on RFI, participated in data collection and analysis, and edited the manuscript. UM determined serum and blood parameters, and edited the manuscript. KW contributed to experimental design, established lab protocols, and edited the manuscript.
Funding
The ECO-FCE project was funded by the European Union Seventh Framework Programme (FP7 2007/2013) under Grant Agreement No. 311794.
Conflict of Interest Statement
The authors declare that the research was conducted in the absence of any commercial or financial relationships that could be construed as a potential conflict of interest.
Supplementary Material
The Supplementary Material for this article can be found online at: https://www.frontiersin.org/articles/10.3389/fgene.2019.00117/full#supplementary-material
Abbreviations
FE, feed efficiency; HDL, high density lipoprotein; HRFI, high RFI; LDL, low density lipoprotein; LRFI, low RFI; RFI, residual feed intake.
Footnotes
- ^www.R-project.org
- ^www.ingenuity.com
- ^https://www.ncbi.nlm.nih.gov/tools/primer-blast
- ^http://www.ncbi.nlm.nih.gov/BLAST
References
Alexandre, P. A., Kogelman, L. J. A., Santana, M. H. A., Passarelli, D., Pulz, L. H., Fantinato-Neto, P., et al. (2015). Liver transcriptomic networks reveal main biological processes associated with feed efficiency in beef cattle. BMC Genomics 16:1073. doi: 10.1186/s12864-015-2292-8
Anders, S., Pyl, P. T., and Huber, W. (2015). HTSeq—a Python framework to work with high-throughput sequencing data. Bioinformatics 31, 166–169. doi: 10.1093/bioinformatics/btu638
Bard-Chapeau, E. A., Yuan, J., Droin, N., Long, S. N., Zhang, E. E., Nguyen, T. V., et al. (2006). Concerted functions of Gab1 and Shp2 in liver regeneration and hepatoprotection. Mol. Cell. Biol. 26, 4664–4674. doi: 10.1128/MCB.02253-05
Boyer, J. L. (2013). Bile formation and secretion. Compr. Physiol. 3, 1035–1078. doi: 10.1002/cphy.c120027
Briet, M., and Schiffrin, E. L. (2010). Aldosterone: effects on the kidney and cardiovascular system. Nat. Rev. Nephrol. 6, 261–273. doi: 10.1038/nrneph.2010.30
Chai, J., Zou, L., Li, X., Han, D., Wang, S., Hu, S., et al. (2015). Mechanism of bile acid-regulated glucose and lipid metabolism in duodenal-jejunal bypass. Int. J. Clin. Exp. Pathol. 8, 15778–15785.
Charlton-Menys, V., and Durrington, P. N. (2008). Human cholesterol metabolism and therapeutic molecules. Exp. Physiol. 93, 27–42. doi: 10.1113/expphysiol.2007.035147
Choi, H. J., Geng, Y., Cho, H., Li, S., Giri, P. K., Felio, K., et al. (2011). Differential requirements for the Ets transcription factor Elf-1 in the development of NKT cells and NK cells. Blood 117, 1880–1887. doi: 10.1182/blood-2010-09-309468
Colucci, F., and Di Santo, J. P. (2000). The receptor tyrosine kinase c-kit provides a critical signal for survival, expansion, and maturation of mouse natural killer cells. Blood 95, 984–991.
De Falco, G., Bellan, C., D’Amuri, A., Angeloni, G., Leucci, E., Giordano, A., et al. (2005). Cdk9 regulates neural differentiation and its expression correlates with the differentiation grade of neuroblastoma and PNET tumors. Cancer Biol. Ther. 4, 277–281. doi: 10.4161/cbt.4.3.1497
Feingold, K. R., and Grunfeld, C. (2000). “Introduction to Lipids and Lipoproteins,” in Endotext, eds L. J. De Groot, G. Chrousos, K. Dungan, K. R. Feingold, A. Grossman, J. M. Hershman, et al. (South Dartmouth, MA: MDText.com, Inc).
Garriga, C., Planas, J. M., and Moretó, M. (2001). Aldosterone mediates the changes in hexose transport induced by low sodium intake in chicken distal intestine. J. Physiol. 535(Pt 1), 197–205. doi: 10.1111/j.1469-7793.2001.00197.x
Garriga, J., Bhattacharya, S., Calbo, J., Marshall, R. M., Truongcao, M., Haines, D. S., et al. (2003). CDK9 is constitutively expressed throughout the cell cycle, and its steady-state expression is independent of SKP2. Mol. Cell. Biol. 23, 5165–5173. doi: 10.1128/MCB.23.15.5165-5173.2003
Getz, G. S., and Reardon, C. A. (2004). Paraoxonase, a cardioprotective enzyme: continuing issues. Curr. Opin. Lipidol. 15, 261–267. doi: 10.1097/00041433-200406000-00005
Gondret, F., Vincent, A., Houée-Bigot, M., Siegel, A., Lagarrigue, S., Causeur, D., et al. (2017). A transcriptome multi-tissue analysis identifies biological pathways and genes associated with variations in feed efficiency of growing pigs. BMC Genomics 18:244. doi: 10.1186/s12864-017-3639-0
Gratten, J., and Visscher, P. M. (2016). Genetic pleiotropy in complex traits and diseases: implications for genomic medicine. Genome Med. 8:78. doi: 10.1186/s13073-016-0332-x
Grubbs, J. K., Fritchen, A. N., Huff-Lonergan, E., Gabler, N. K., and Lonergan, S. M. (2013). Selection for residual feed intake alters the mitochondria protein profile in pigs. J. Proteomics 80, 334–345. doi: 10.1016/j.jprot.2013.01.017
Gruffat, D., Durand, D., Graulet, B., and Bauchart, D. (1996). Regulation of VLDL synthesis and secretion in the liver. Reprod. Nutr. Dev. 36, 375–389. doi: 10.1051/rnd:19960404
Ha, J. S., Ha, C. E., Chao, J. T., Petersen, C. E., Theriault, A., and Bhagavan, N. V. (2003). Human serum albumin and its structural variants mediate cholesterol efflux from cultured endothelial cells. Biochim. Biophys. Acta 1640, 119–128. doi: 10.1016/S0167-4889(03)00027-2
Han, Y., Zhan, Y., Hou, G., and Li, L. I. (2014). Cyclin-dependent kinase 9 may as a novel target in downregulating the atherosclerosis inflammation (Review). Biomed. Rep. 2, 775–779. doi: 10.3892/br.2014.322
Hoare, M., Das, T., and Alexander, G. (2010). Ageing, telomeres, senescence, and liver injury. J. Hepatol. 53, 950–961. doi: 10.1016/j.jhep.2010.06.009
Horodyska, J., Oster, M., Reyer, H., Mullen, A. M., Lawlor, P. G., Wimmers, K., et al. (2018a). Analysis of meat quality traits and gene expression profiling of pigs divergent in residual feed intake. Meat Sci. 137, 265–274. doi: 10.1016/j.meatsci.2017.11.021
Horodyska, J., Wimmers, K., Reyer, H., Trakooljul, N., Mullen, A. M., Lawlor, P. G., et al. (2018b). RNA-seq of muscle from pigs divergent in feed efficiency and product quality identifies differences in immune response, growth, and macronutrient and connective tissue metabolism. BMC Genomics 19:791. doi: 10.1186/s12864-018-5175-y
Hu, Z., Evarts, R. P., Fujio, K., Marsden, E. R., and Thorgeirsson, S. S. (1993). Expression of hepatocyte growth factor and c-met genes during hepatic differentiation and liver development in the rat. Am. J. Pathol. 142, 1823–1830.
Jiang, W. G., Hallett, M. B., and Puntis, M. C. A. (1993). Hepatocyte growth factor/scatter factor, liver-regeneration and cancer metastasis. Br. J. Surg. 80, 1368–1373. doi: 10.1002/bjs.1800801104
Jones, C. N., Tuleuova, N., Lee, J. Y., Ramanculov, E., Reddi, A. H., Zern, M. A., et al. (2009). Cultivating liver cells on printed arrays of hepatocyte growth factor. Biomaterials 30, 3733–3741. doi: 10.1016/j.biomaterials.2009.03.039
Kim, D., Pertea, G., Trapnell, C., Pimentel, H., Kelley, R., and Salzberg, S. L. (2013). TopHat2: accurate alignment of transcriptomes in the presence of insertions, deletions and gene fusions. Genome Biol. 14:R36. doi: 10.1186/gb-2013-14-4-r36
Leiva, E., Wehinger, S., Guzmán, L., and Orrego, R. (2015). “Role of oxidized LDL in atherosclerosis,” in Hypercholesterolemia, ed. S. A. Kumar (Rijeka: InTech).
Lobley, G. E. (2003). Protein turnover—what does it mean for animal production? Canadian J. Anim. Sci. 83, 327–340. doi: 10.4141/A03-019
Mancini, M., and Toker, A. (2009). NFAT proteins: emerging roles in cancer progression. Nat. Rev. Cancer 9, 810–820. doi: 10.1038/nrc2735
Olsen, H. G., Skovgaard, K., Nielsen, O. L., Leifsson, P. S., Jensen, H. E., Iburg, T., et al. (2013). Organization and biology of the porcine serum amyloid A (SAA) gene cluster: isoform specific responses to bacterial infection. PLoS One 8:e76695. doi: 10.1371/journal.pone.0076695
Oram, J. F., and Lawn, R. M. (2001). ABCA1. The gatekeeper for eliminating excess tissue cholesterol. J. Lipid Res. 42, 1173–1179.
Ornitz, D. M., and Itoh, N. (2015). The fibroblast growth factor signaling pathway. Wiley Interdiscip. Rev. Dev. Biol. 4, 215–266. doi: 10.1002/wdev.176
Papakostas, S., Vøllestad, L. A., Bruneaux, M., Aykanat, T., Vanoverbeke, J., Ning, M., et al. (2014). Gene pleiotropy constrains gene expression changes in fish adapted to different thermal conditions. Nat. Commun. 5:4071. doi: 10.1038/ncomms5071
Paradis, F., Yue, S., Grant, J. R., Stothard, P., Basarab, J. A., and Fitzsimmons, C. (2015). Transcriptomic analysis by RNA sequencing reveals that hepatic interferon-induced genes may be associated with feed efficiency in beef heifers. J. Anim. Sci. 93, 3331–3341. doi: 10.2527/jas.2015-8975
Patience, J. F., Rossoni-Serão, M. C., and Gutiérrez, N. A. (2015). A review of feed efficiency in swine: biology and application. J. Anim. Sci. Biotechnol. 6:33. doi: 10.1186/s40104-015-0031-2
Peng, H., Wisse, E., and Tian, Z. (2016). Liver natural killer cells: subsets and roles in liver immunity. Cell. Mol. Immunol. 13, 328–336. doi: 10.1038/cmi.2015.96
Promintzer, M., and Krebs, M. (2006). Effects of dietary protein on glucose homeostasis. Curr. Opin. Clin. Nutr. Metab. Care 9, 463–468. doi: 10.1097/01.mco.0000232909.84483.a9
Racanelli, V., and Rehermann, B. (2006). The liver as an immunological organ. Hepatology 43(2 Suppl. 1), S54–S62. doi: 10.1002/hep.21060
Ramayo-Caldas, Y., Ballester, M., Sánchez, J. P., González-Rodríguez, O., Revilla, M., Reyer, H., et al. (2018). Integrative approach using liver and duodenum RNA-Seq data identifies candidate genes and pathways associated with feed efficiency in pigs. Sci. Rep. 8:558. doi: 10.1038/s41598-017-19072-5
Reddy, S. T., Wadleigh, D. J., Grijalva, V., Ng, C., Hama, S., Gangopadhyay, A., et al. (2001). Human paraoxonase-3 is an HDL-associated enzyme with biological activity similar to paraoxonase-1 protein but is not regulated by oxidized lipids. Arterioscler. Thromb. Vasc. Biol. 21, 542–547. doi: 10.1161/01.ATV.21.4.542
Reyer, H., Oster, M., Magowan, E., Dannenberger, D., Ponsuksili, S., and Wimmers, K. (2017). Strategies towards improved feed efficiency in pigs comprise molecular shifts in hepatic lipid and carbohydrate metabolism. Int. J. Mol. Sci. 18:E1674. doi: 10.3390/ijms18081674
Rui, L. (2014). Energy metabolism in the liver. Compr. Physiol. 4, 177–197. doi: 10.1002/cphy.c130024
Sachs, M., Brohmann, H., Zechner, D., Müller, T., Hülsken, J., Walther, I., et al. (2000). Essential role of Gab1 for signaling by the C-met receptor in vivo. J. Cell Biol. 150, 1375–1384. doi: 10.1083/jcb.150.6.1375
Sánchez, A., and Fabregat, I. (2010). Growth factor- and cytokine-driven pathways governing liver stemness and differentiation. World J. Gastroenterol. 16, 5148–5161. doi: 10.3748/wjg.v16.i41.5148
Schuler, A., Schwieger, M., Engelmann, A., Weber, K., Horn, S., Muller, U., et al. (2008). The MADS transcription factor Mef2c is a pivotal modulator of myeloid cell fate. Blood 111, 4532–4541. doi: 10.1182/blood-2007-10-116343
Sherwin, R. S. (1980). Role of liver in glucose homeostasis. Diabetes Care 3, 261–265. doi: 10.2337/diacare.3.2.261
Shih, D. M., Yu, J. M., Vergnes, L., Dali-Youcef, N., Champion, M. D., Devarajan, A., et al. (2015). PON3 knockout mice are susceptible to obesity, gallstone formation, and atherosclerosis. FASEB J. 29, 1185–1197. doi: 10.1096/fj.14-260570
Shimizu, N., Maruyama, T., Yoshikawa, N., Matsumiya, R., Ma, Y., Ito, N., et al. (2015). A muscle-liver-fat signalling axis is essential for central control of adaptive adipose remodelling. Nat. Commun. 6:6693. doi: 10.1038/ncomms7693
Simone, C., Stiegler, P., Bagella, L., Pucci, B., Bellan, C., De Falco, G., et al. (2002). Activation of MyoD-dependent transcription by cdk9/cyclin T2. Oncogene 21, 4137–4148. doi: 10.1038/sj.onc.1205493
Staels, B., and Fonseca, V. A. (2009). Bile acids and metabolic regulation: mechanisms and clinical responses to bile acid sequestration. Diabetes Care 32(Suppl. 2), S237–S245. doi: 10.2337/dc09-S355
Steiling, H., Wustefeld, T., Bugnon, P., Brauchle, M., Fassler, R., Teupser, D., et al. (2003). Fibroblast growth factor receptor signalling is crucial for liver homeostasis and regeneration. Oncogene 22, 4380–4388. doi: 10.1038/sj.onc.1206499
Trigueros-Motos, L., van Capelleveen, J. C., Torta, F., Castaño, D., Zhang, L.-H., Chai, C., et al. (2017). ABCA8 regulates cholesterol efflux and high-density lipoprotein cholesterol levels. Arterioscler. Thromb. Vasc. Biol. 37, 2147–2155. doi: 10.1161/ATVBAHA.117.309574
Venhoranta, H., Pausch, H., Wysocki, M., Szczerbal, I., Hänninen, R., Taponen, J., et al. (2013). Ectopic KIT copy number variation underlies impaired migration of primordial germ cells associated with gonadal hypoplasia in cattle (Bos taurus). PLoS One 8:e75659. doi: 10.1371/journal.pone.0075659
Wang, C., Yang, C., Chang, J. Y., You, P., Li, Y., Jin, C., et al. (2014). Hepatocyte FRS2alpha is essential for the endocrine fibroblast growth factor to limit the amplitude of bile acid production induced by prandial activity. Curr. Mol. Med. 14, 703–711. doi: 10.2174/1566524014666140724095112
Xu, N., Lao, Y., Zhang, Y., and Gillespie, D. A. (2012). Akt: a double-edged sword in cell proliferation and genome stability. J. Oncol. 2012:951724. doi: 10.1155/2012/951724
Ye, D., Meurs, I., Ohigashi, M., Calpe-Berdiel, L., Habets, K. L., Zhao, Y., et al. (2010). Macrophage ABCA5 deficiency influences cellular cholesterol efflux and increases susceptibility to atherosclerosis in female LDLr knockout mice. Biochem. Biophys. Res. Commun. 395, 387–394. doi: 10.1016/j.bbrc.2010.04.027
Zanoni, I., and Granucci, F. (2012). Regulation and dysregulation of innate immunity by NFAT signaling downstream of pattern recognition receptors (PRRs). Eur. J. Immunol. 42, 1924–1931. doi: 10.1002/eji.201242580
Zhang, Y., Xu, D., Huang, H., Chen, S., Wang, L., Zhu, L., et al. (2014). Regulation of glucose homeostasis and lipid metabolism by PPP1R3G-mediated hepatic glycogenesis. Mol. Endocrinol. 28, 116–126. doi: 10.1210/me.2013-1268
Zhao, Y., Hou, Y., Liu, F., Liu, A., Jing, L., Zhao, C., et al. (2016). Transcriptome analysis reveals that vitamin A metabolism in the liver affects feed efficiency in pigs. G 3, 3615–3624. doi: 10.1534/g3.116.032839
Keywords: FE, RFI, residual feed intake, gene expression, transcriptomics
Citation: Horodyska J, Hamill RM, Reyer H, Trakooljul N, Lawlor PG, McCormack UM and Wimmers K (2019) RNA-Seq of Liver From Pigs Divergent in Feed Efficiency Highlights Shifts in Macronutrient Metabolism, Hepatic Growth and Immune Response. Front. Genet. 10:117. doi: 10.3389/fgene.2019.00117
Received: 22 December 2017; Accepted: 04 February 2019;
Published: 19 February 2019.
Edited by:
Ino Curik, University of Zagreb, CroatiaReviewed by:
Luca Fontanesi, University of Bologna, ItalyJohann Sölkner, University of Natural Resources and Life Sciences Vienna, Austria
Copyright © 2019 Horodyska, Hamill, Reyer, Trakooljul, Lawlor, McCormack and Wimmers. This is an open-access article distributed under the terms of the Creative Commons Attribution License (CC BY). The use, distribution or reproduction in other forums is permitted, provided the original author(s) and the copyright owner(s) are credited and that the original publication in this journal is cited, in accordance with accepted academic practice. No use, distribution or reproduction is permitted which does not comply with these terms.
*Correspondence: Ruth M. Hamill, Ruth.Hamill@teagasc.ie