- 1Department of Environmental Health Sciences, Columbia University Mailman School of Public Health, New York, NY, United States
- 2Department of Epidemiology, Johns Hopkins University Bloomberg School of Public Health, Baltimore, MD, United States
- 3Clinical and Translational Research, Johns Hopkins School of Medicine, Baltimore, MD, United States
- 4Department of Nutrition, UNC Nutrition Research Institute, University of North Carolina at Chapel Hill, Kannapolis, NC, United States
- 5Department of Genetics, Texas Biomedical Research Institute, San Antonio, TX, United States
- 6Department of Epidemiology, University of North Carolina at Chapel Hill, Chapel Hill, NC, United States
- 7South Texas Diabetes and Obesity Institute, University of Texas Rio Grande Valley School of Medicine, Brownsville, TX, United States
- 8MedStar Health Research Institute, Hyattsville, MD, United States
- 9Georgetown and Howard Universities Center for Clinical and Translational Science, Washington, DC, United States
- 10Center for American Indian Health Research, College of Public Health, University of Oklahoma Health Sciences Center, Oklahoma City, OK, United States
- 11Missouri Breaks Industries Research, Inc., Eagle Butte, SD, United States
Background: Genetic research may inform underlying mechanisms for disparities in the burden of type 2 diabetes mellitus among American Indians. Our objective was to assess the association of genetic variants in cardiometabolic candidate genes with B cell dysfunction via HOMA-B, insulin resistance via HOMA-IR, and type 2 diabetes mellitus in the Strong Heart Family Study (SHFS).
Methods and Results: We examined the association of variants, previously associated with cardiometabolic traits (∼200,000 from Illumina Cardio MetaboChip), using mixed models of HOMA-B residuals corrected for HOMA-IR (cHOMA-B), log transformed HOMA-IR, and incident diabetes, adjusted for age, sex, population stratification, and familial relatedness. Center-specific estimates were combined using fixed effect meta-analyses. We used Bonferroni correction to account for multiple testing (P < 4.13 × 10−7). We also assessed the association between variants in candidate diabetes genes with these metabolic traits. We explored the top SNPs in an independent, replication sample from Southwestern Arizona. We identified significant associations with cHOMA-B for common variants at 26 loci of which 8 were novel (PRSS7, FCRL5, PEL1, LRP12, IGLL1, ARHGEF10, PARVA, FLJ16686). The most significant variant association with cHOMA-B was observed on chromosome 5 for an intergenic variant near PARP8 (rs2961831, P = 6.39 × 10−9). In the replication study, we found a signal at rs4607517 near GCK/YKT6 (P = 0.01). Variants near candidate diabetes genes (especially GCK and KCNQ1) were also nominally associated with HOMA-IR and cHOMA-B.
Conclusion: We identified variants at novel loci and confirmed those at known candidate diabetes loci associations for cHOMA-B. This study also provided evidence for association of variants at KCNQ2, CTNAA2, and KCNQ1with cHOMA-B among American Indians. Further studies are needed to account for the high heritability of diabetes among the American Indian participants of the SHFS cohort.
Introduction
The burden of type 2 diabetes mellitus among American Indians is disproportionately high with an estimated prevalence ranging between 34 and 68% compared to 9.3% in the general U.S. population (Acton et al., 2002; Lee et al., 2002). In addition to high prevalence, incidence is twice as high among American Indians compared to the U.S. general population (Welty et al., 2002; Narayan et al., 2006). While largely attributed to the obesity epidemic, other risk factors especially among American Indians are not well understood, particularly genetic susceptibility (North et al., 2003; Franceschini et al., 2008; Haiman et al., 2012; Yang et al., 2012).
Pathogenesis is increasingly being attributed to B cell dysfunction compared to insulin resistance in peripheral tissues (Patti, 2004). Heritability for HOMA-beta cell function (HOMA-B), HOMA-IR, type 2 diabetes mellitus are 0.28–0.78, 0.08–0.75, and 0.26–0.70, respectively (Jenkins et al., 2000; Mills et al., 2004; Poulsen et al., 2005; Almgren et al., 2011). Several of the diabetes-associated genes, including PPARG and SLC30A8, initially identified in individuals of European ancestry, have also been replicated in other populations (Lewis et al., 2008; Chauhan et al., 2010; Yang et al., 2010). Generalization to other ethnic groups has been limited, especially among American Indians (Franceschini et al., 2008; Fesinmeyer et al., 2013; Hanson et al., 2014). Genome-wide linkage analysis in Strong Heart Family Study (SHFS) has demonstrated segregation of diabetes and metabolic trait related variants among American Indian families (North et al., 2003; Franceschini et al., 2008). Yet, most genetic loci identified in other ancestries have not replicated in American Indians (Kovacs et al., 2003; Rong et al., 2009; Haiman et al., 2012; Fesinmeyer et al., 2013). The main goal of this study was to assess the associations between genetic variants previously associated with cardiometabolic traits and dysglycemia traits of HOMA-B, HOMA-IR, and incident diabetes in American Indians among SHFS participants who were free of diabetes at baseline and replicated significant associations in an independent sample from Southwestern Arizona.
Materials and Methods
Study Population
The SHFS is an extension of a large, population-based cohort of American Indians in the Strong Heart Study (SHS), recruited from thirteen tribes from three centers: Arizona, Oklahoma and North and South Dakota. Details on participant recruitment and information obtained in clinical visits have been published (Lee et al., 1990; North et al., 2002). Briefly, the SHFS included family members with a core sibship including at least 5 living members of whom at least 3 had to be original SHS participants. Recruitment of the SHFS participants was conducted in two phases; 533 participants attended the baseline visit in 1998–99 and 1,941 participants attended the baseline visit in 2001–03. Follow-up examinations were performed in 2001–03, 2005–06, and 2014–15. Demographic and clinical characteristics were collected at baseline and follow-up visits, including fasting plasma glucose (FPG) and HbA1c. For this study, we included participants without diabetes at baseline since our primary outcome was HOMA-B. We further excluded individuals without measured phenotypic and genotypic data (n = 1,923). During follow-up, 256 participants developed diabetes. The replication sample included 3,244 participants from a community in Southwestern Arizona. Detailed information on participant recruitment and data have been described elsewhere (Knowler et al., 1990; Hanson et al., 2013).
All participants in this study provided written informed consent and tribal consent. The study protocols were approved by the Indian Health Service Institutional Review Board, by the Institutional Review Boards of the participating Institutions, and by the participating American Indian tribes.
Outcome Definition
HOMA-IR (mmol/L) was calculated by fasting insulin in mU/L ∗ fasting glucose in mmol/L)/22.5 (Matthews et al., 1985). HOMA-B was calculated using baseline data of participants without diabetes, and the equation described by Matthews et al. (1985) (20 ∗ fasting insulin in mU/L)/(fasting glucose in mmol/L - 3.5). To incorporate the influence of insulin resistance, corrected HOMA-B (cHOMA-B) was created as the residuals when HOMA-B was regressed on HOMA-IR (HOMA-B = mean HOMA-B + beta coefficient ∗ HOMA-IR). Diabetes was defined as a fasting plasma glucose ≥ 6.99 mmol/L or use of insulin or oral hypoglycemic medications. Incident diabetes was defined as new cases during subsequent follow-up visits (mean follow-up 6.6 years, range 3.0–12.3 years).
Genotyping
Blood DNA from baseline was genotyped using the Illumina Cardio-Metabo DNA Analysis BeadChip (MetaboChip) (Voight et al., 2010). MetaboChip included 196,725 single nucleotide polymorphisms (SNPs) selected based on meta-analysis of cardiometabolic traits and includes replication targets and fine-mapping regions. Samples were excluded when sample call rate < 95%, mismatch between genotyped and reported gender, outlier in identity by descent (IBD) clustering, or outlier in principal components analysis (PCA). SNPs were excluded if: call rate < 98% or no data (n = 33,604); not autosomal (n = 250); monomorphic (n = 158); or violated Hardy-Weinberg equilibrium (HWE) P < 1 × 10−5 (n = 1,519). PCA was performed on a matrix of doses of copies of minor allele for SNPs selected among genotyped founders or unrelated individuals based on minimum spacing of 1 kb, minor allele frequency (MAF) ≥ 0.05, pairwise correlation of genotype scores < 0.1, and within a sliding window of 100 kb. The first four principal components (PCs) account for substantially more than the rest (cumulatively 8.8% of total variance) (Burdick et al., 2006). In SHFS, the first three PCs cluster by study center and some clustering with less clear separation is apparent for the fourth PC reported in the electronic Supplementary Material. Family-based imputation of genotyped SNPs was done with a PEDSYS-compatible version of Merlin using human genome build 18 (NCBI36/hg18); genotype information from relatives was used to impute missing values (Dyke, 1996; Pruitt et al., 2006). After imputation and quality control, 120,975 SNPs were available for analyses.
Statistical Analysis
All analyses were performed using mixed effects models, to account for family relatedness, for quantitative traits (i.e., HOMA-IR and HOMA-B) and qualitative outcomes (incident diabetes). Analyses was implemented using Sequential Oligogenic Linkage Analysis Routines (SOLAR) assuming additive effect (Blangero and Almasy, 1996). HOMA-B values cannot be evaluated without taking HOMA-IR into account (Pfützner et al., 2010). Based on previous literature, we regressed HOMA-B on HOMA-IR and added the mean HOMA-B to the model residual for interpretability (Willett and Stampfer, 1986; Balakrishnan et al., 2018). These scores were called cHOMA-B and were used as traits in genetic analyses (Supplementary Figure 1). Since HOMA-IR values were right-skewed, they were natural log transformed. For HOMA scores, models were adjusted for age at baseline, sex, and first four PCs to adjust for global population stratification. For diabetes, models were adjusted for age at follow-up (mean 42.4 years), sex, and first four PCs. Healthy controls were defined as participants who did had normal or impaired fasting glucose and who were not taking medications. Due to possible differences in allele frequencies among recruiting centers, all models were stratified by recruiting centers. The results from center-stratified association analyses were meta-analyzed using inverse-variance-weighting models implemented using METAL software (Willer et al., 2010).
The array wide significance threshold for multiple testing using Bonferroni correction was 4.13 × 10−7 and using the Moskvina and Schmidt method accounting for linkage disequilibrium (LD) was 7.77 × 10−7. We performed conditional analysis on the SNPs with lowest p-values to identify independent associations at each locus. Models were assessed for genomic inflation (Supplementary Figure 2). As sensitivity analyses, we excluded participants with diabetes during follow-up for the HOMA score models. For incident diabetes, we also modified the case definition to include HbA1c thresholds and control definition to exclude participants with impaired fasting glucose. Replication of significant SNPs was assessed for nominal significance (P < 0.05) in an independent sample from a community in Southwestern Arizona (n = 3,244) without diabetes for cHOMA-B. Models were adjusted for age, sex, and first five PCs to account for population stratification in the sample.
Results
Table 1 shows the baseline characteristics of the study population. Over a mean follow-up of 6.6 years (12,667.6 person-years), 256 participants or 13.5% of the study population developed incident diabetes. On average, participants who developed diabetes had nearly 1 year longer follow-up than participants who did not develop diabetes. Participants developing incident diabetes were older, more often obese, and had a higher fasting glucose, HOMA-B and HOMA-IR at baseline (P < 0.05). When corrected for HOMA-IR, cHOMA-B was lower among those who developed diabetes (median 158.1) compared to those who did not develop diabetes during follow-up (median 188.6) (P < 0.01).
The genomic inflation factor (λ) for cHOMA-B, HOMA-IR, and diabetes were 1.11, 1.02, and 1.24, respectively (Supplementary Figure 2). We identified several genes in the Cardio MetaboChip significantly associated with cHOMA-B (P < 4.13 × 10−7) (Supplementary Table 1). A total of 28 variants in 25 distinct loci were statistically significantly associated with cHOMA-B (Figure 1). The associations observed near PRSS7, FCRL5, PEL1, LRP12, IGLL1, ARHGEF10, PARVA, and FLJ16686 are novel for a biomarker of B cell dysfunction. GCK demonstrated the most consistent association with B-cell function phenotypes. In particular, rs4607517 (G > A) was associated with a decrease in cHOMA-B of 19.54 units in SHFS (P = 2.67 × 10−7) and 11.26 in the replication sample (P = 0.01). The most significant SNP associated with cHOMA-B was rs2961831 (A > C), an intergenic variant, located at 5q11/PARP8 (Table 2). Each copy of allele C was associated with increases of 23.11 units of cHOMA-B (P = 6.39 × 10−9). Of the variants, 3 regions at 5q11/PARP8, 12q24/CUX2, and 15q12/ATP10A had multiple variant associations that passed the significance threshold (Figure 2). Conditional analysis on index SNPs suggested the presence of a single association at each locus. Results for all significant SNP associations for log transformed HOMA-B and cHOMA-B are presented as Supplementary Material (Supplementary Table 1).
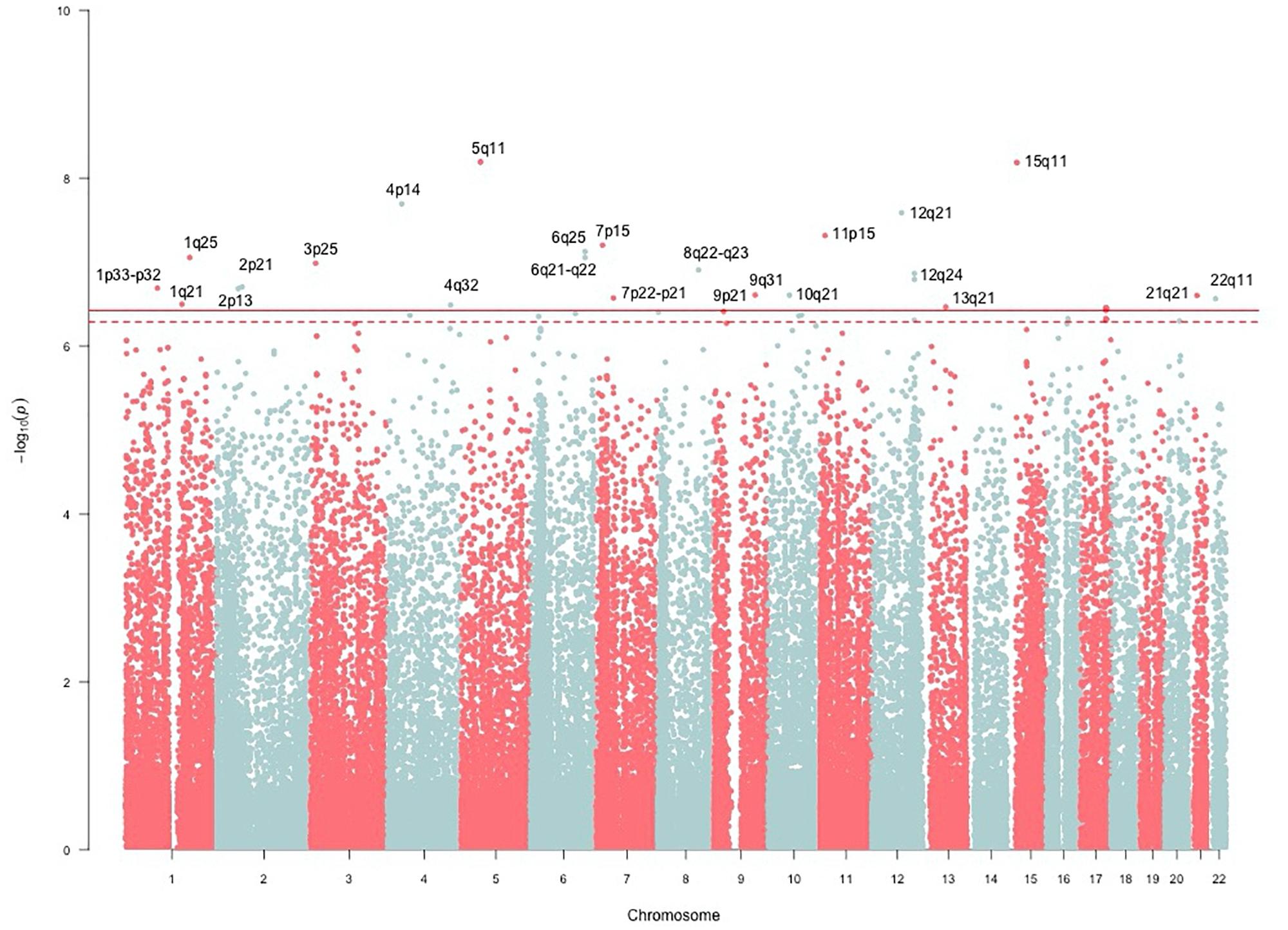
FIGURE 1. Manhattan plot of corrected HOMA-B. SNP associations mapped using NCBI36/hg18 build position. The solid line is the MetaboChip-wide Bonferroni significance threshold at −log(4.13 × 10−7) or 6.38. The dashed line is the MetaboChip-wide Moskvina-Schmidt LD significance threshold at −log(7.77 × 10−7) or 6.11.
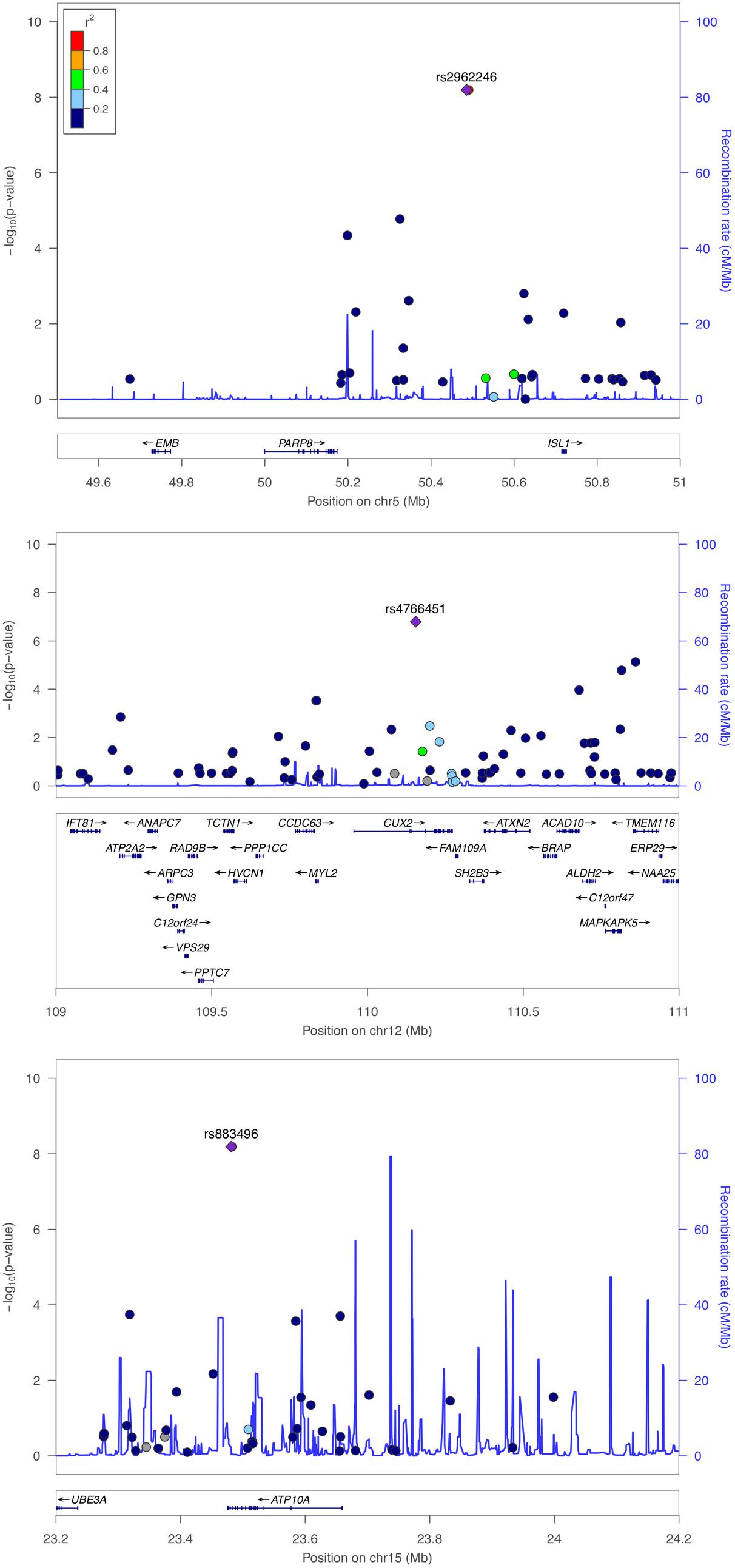
FIGURE 2. Regional association plot of PARP8, CUX2, and ATP10A for corrected HOMA-B. Multiple SNP associations for cHOMA-B are 5q11/PARP8 (top panel), 12q24/CUX2 (middle panel), and 15q12/ATP10A (bottom panel), using NCBI36/hg18 build and European American recombination rates.
We assessed the top 28 SNPs for cHOMA-B in an independent sample from Southwestern Arizona (Table 2). Variants had similar MAF in the replication sample compared to the SHFS, but we were not able to replicate many of these associations in the replication sample except rs4607517. One variant showed nominal significance; an intergenic SNP rs4607517 (G > A; MAF in replication sample 0.31) showed an effect estimate of –11.26 (P = 0.01) compared to an effect estimate of –19.54 (P = 2.67 × 10−7) in the SHFS (MAF 0.26).
The top association for log transformed HOMA-IR was with rs7609071 (G > C) near CTNAA2 (P = 1.34 × 10−5) (Supplementary Table 1). Each copy of the C allele was associated with an increase of 0.84 and 0.79 units of HOMA-IR in Oklahoma, and the Dakotas respectively, (effect estimate –0.18, –0.24) (Supplementary Table 1). Other SNPs at 2p11-12 were nominally associated with cHOMA-B. In addition to being associated with cHOMA-B, rs7609071 was associated with the index HOMA-IR (P = 2.51 × 10−3) but not with incident diabetes (P = 0.87). There was no significant evidence for association of SNPs with incident diabetes (Supplementary Table 1).
In the MetaboChip data, there were seven candidate diabetes loci (Supplementary Table 2) with 1,446 SNPs that passed quality control. Among the observed associations, an intronic SNP rs163170 (KCNQ1) was significantly associated with HOMA-IR and with cHOMA-B (Table 3). This variant is located near the previously reported SNP at this locus (rs8181588) although their correlation is low in our American Indian population (LD r2 = 0.29) (McCarthy and Zeggini, 2009; Hanson et al., 2014). None of the MetaboChip SNPs in the candidate genes were associated with incident diabetes.
Discussion
Our study validates diabetes-related loci in American Indians, a population with a large burden of diabetes. Among the SHFS participants, the diabetes prevalence was 15.6%, which is high compared to the 9.3% prevalence reported in the general U.S. population (Acton et al., 2002; Lee et al., 2002). The diabetes prevalence is especially disproportionate among younger age groups, where the crude prevalence in NHANES among 18–44 ages was 5.0% (Menke et al., 2015) compared to 12.4% in the SHFS. Our study demonstrated that known cardiometabolic loci are involved in B cell dysfunction and insulin resistance in American Indians. In particular, we identified GCK variant rs4607517, which was previously identified including MAGIC consortium and MESA cohort (Dupuis et al., 2010; Rasmussen-Torvik et al., 2012). We also identified some novel associations using the Cardio MetaboChip array, such as the association of cHOMA-B with PARP8. In the diabetes candidate gene analysis, we identified an association of an intronic SNP rs163170 in KCNQ1 with both cHOMA-B and HOMA-IR.
The variants associated with cHOMA-B are located near or in genes that show involvement in biological mechanisms such as metabolism (GCK, PRSS7), movement across extracellular and intracellular membranes (FCRL5, SEC16B, TGFBR3, PEL1, FSTL5, SLC22A1, LRP12, ABCA1, ATP10A, KCNJ2, NCAM2, IGLL1), activation and deactivation of hydrolase enzymes (ARHGEF10, NAV3), transcriptional and DNA-binding regulation (CAND2, ZFPM2, TUSC1, TEAD1, CUX2), cell movement and adhesion (SPTBN1, PCDH15, PARVA, DIAPH3), and cell cycle and apoptosis (FLJ16686, FRK, MEOX2) (Pruitt et al., 2006). The most significant variants for cHOMA-B were located at PARP8 [Poly(adenosine disphosphate/ADP-ribosyl)ation – member VIII], which catalyze the transfer of ADP-ribose from glutamic acid to aspartic acid, possibly involving zinc fingers (Amé et al., 2004). Both CUX2 (Cut like homeobox 2), a DNA binding motif, and ATP10A (ATPase phospholipid transporting 10A), a maternally expressed aminophospholipid translocase across the lipid bilayer, were previously reported in associations with diabetes related traits including metabolic syndrome (Shim et al., 2014) and insulin resistance (Irvin et al., 2011). In this study, we identified associations with variants at loci not encompassed in linkage peaks on chromosomes 3 and 4 as previously reported in the SHFS (North et al., 2005). Our replication yielded one nominal association for rs4607517 near GCK/YKT6 with consistent directions for the beta estimates. This was the only association that has been previously identified to be associated with HOMA-B (Dupuis et al., 2010). Overall, we did not find consistency between the findings in the two samples possibly due to heterogeneity between the cohorts and centers in SHFS. There may be heterogeneity in LD structure between the populations. A preliminary measure is the notable difference in MAF particularly in the variants of top candidate genes (GCK and KCNQ1). There may also be heterogeneity in measurement of variables including phenotypes that could partially account for the differences in effect sizes and statistical significance.
In analyses of diabetes candidate genes, we identified an association with several variants near KCNQ1, which encodes a voltage-gated potassium channel and has been associated with diabetes in individuals of European and East Asian ancestries but also in Southwest American Indians (Schroeder et al., 2000; McCarthy and Zeggini, 2009). KCNQ1 variants showed evidence of functional effects in knockout mice (Hanson et al., 2014). In vitro and murine studies have shown that the overactivity of the potassium channels from overexpression of KCNQ1 can create a current across the plasma membrane and impair insulin secretion, thereby resulting in hyperglycemia (McCarthy and Zeggini, 2009; Yamagata et al., 2011). In a study among American Indians from central Arizona, KCNQ1 variants were associated with incident diabetes (Franceschini et al., 2013; Hanson et al., 2014). In our study, KCNQ1 variants are related to both a decrease in HOMA-IR (effect -0.11) and with an increase in cHOMA-B (effect 0.04). ENCODE data using HaploReg (v4.0) of the diabetes candidate genes shows possible functional regulation including binding to DNA hypersensitivity site, histone promoter and enhancer sites to investigate in future studies.
Our study is one of the few that have investigated the association of genetic determinants of diabetes traits among American Indians. While the number of participants who developed diabetes was relatively small for a genetic analysis, we still identified several significant associations with cHOMA-B. The small sample size with incident diabetes could partly account for why our study was not able to replicate associations seen in other ethnic groups. The SHFS is a unique cohort especially for genetic studies because of the inclusion of related participants from complex pedigrees with high burden of diabetes. This allows for possible fine-mapping of association signals by exploiting differences in LD patterns between American Indians and European Americans among whom most association studies have been conducted. Moreover, it allows for the examination of generalizability of the detected associations in non-European American ethnic groups. We tested variants from the MetaboChip as it provides good coverage and also prioritizes cardiometabolic SNPs and thus minimizes multiple testing. Although it provides overall good coverage in different populations, the MetaboChip or any GWAS chip for that matter may miss SNPs in American Indian populations and measured SNPs may have lower allele frequencies in American Indian populations. Even so, our study was able to replicate some associations (Kovacs et al., 2003; Rong et al., 2009; Yang et al., 2010). While the top SNPs showed limited evidence of consistency in our replication sample, we believe this warrants further investigation of the heterogeneity of genetic susceptibility among American Indians. We observed inflation in the genomic control factor (λ) statistic. However, previous investigations in other cohorts have also reported this inflation when using the MetaboChip panel, most likely because of fine-mapping of several loci (Huertas-Vazquez et al., 2013). In addition, none of the significant findings reported for cHOMA-B were in the loci showing deviation from the diagonal.
Also, HOMA-B and HOMA-IR are surrogate measures of B cell dysfunction and insulin resistance, respectively, and therefore may not truly reflect mechanisms of developing diabetes (Wallace et al., 2004). There is limited assessment of the validity of HOMA scores, especially among various ethnic groups. Pfützner et al. (2010) conducted a randomized control trial that highlighted the inability of HOMA-B scores to replicate the findings of laboratory measures of B cell function. Yet compared to other measures, HOMA models are favorably used in diabetes epidemiologic due to ease of measure and accuracy (Song et al., 2007). Another caveat for HOMA-B is that it cannot be interpreted without accounting for HOMA-IR. We used methodology developed in nutritional epidemiology to account for correlated measures or as in the case with HOMA-B and HOMA-IR, measures built from similar underlying variables (Willett and Stampfer, 1986). Thus, we corrected for the influence of HOMA-IR on HOMA-B by using the HOMA-B residuals and adding a constant of mean HOMA-B. The cHOMA-B phenotype is also less well studied and therefore makes it difficult to compare with previously reported associations. Finally, we cannot discount the possibility that our incident diabetes participants include type 1 diabetes as is usual in large epidemiologic cohorts such as the SHFS. However, given that participants who developed diabetes during follow-up were middle-aged (mean 39.2 years), we believe the number of participants who may have had type 1 diabetes is low.
The novel and replicated associations for cHOMA-B provide new information of genetic association for diabetes traits in American Indians. We also validated associations of GCK and KCNQ1 variants with diabetes in our study population. Although our study has a small number of incident cases and did not validate diabetes-associated loci, we identified several variants in novel loci that are hypothesis-generating for understanding genetic susceptibility in American Indians. Further studies with larger sample size and dense markers are needed to validate our results and identify additional loci unique to American Indians. Further investigation is therefore warranted to better understand the genetic susceptibility to diabetes among American Indians.
Data Availability
The datasets for this manuscript are not publicly available because data is governed by the review of the Strong Heart Steering Committee to ensure that the researchers agree that tribes need to review and approve the manuscripts before submission for publication and to ensure that researchers are responsible and respect the ethical concerns and requirements of the American Indian communities. Requests to access the datasets should be directed to Dr. Shelley Cole, scole@txbiomed.org.
Ethics Statement
This study was carried out in accordance with the recommendations of Indian Health Service Institutional Review Board, by the Institutional Review Boards of the participating Institutions, and by the participating American Indian tribes with written informed consent from all subjects. All subjects gave written informed consent in accordance with the Declaration of Helsinki. The protocol was approved by the Indian Health Service Institutional Review Board, by the Institutional Review Boards of the participating Institutions, and by the participating American Indian tribes.
Author Contributions
All authors were involved in conception and design of study, drafting and revising of the manuscript, and final approval of paper.
Funding
This study was supported by the National Institutes of Health/National Institute of Health Sciences (Grants R01ES021367, R01ES025216, P42ES010349, and P30ES009089) and the National Heart, Lung, and Blood Institute (cooperative agreements grants U01-HL41642, U01-HL41652, U01-HL41654, U01-HL65520, and U01-HL65521and research grants R01-HL109315, R01-HL10 553 9301, R01-HL109284, R01-HL109282, R01-HL109319, and R01-HL090863).
Conflict of Interest Statement
LB was employed at Missouri Breaks Industries Research, Inc. The remaining authors declare that the research was conducted in the absence of any commercial or financial relationships that could be construed as a potential conflict of interest.
Acknowledgments
The authors would like to thank the participants and staff of the Strong Heart and Strong Heart Family Study for their important contributions. We would also like to thank Dr. Robert Hanson (National Institute of Diabetes and Digestive and Kidney Diseases) for his help with the replication study.
Supplementary Material
The Supplementary Material for this article can be found online at: https://www.frontiersin.org/articles/10.3389/fgene.2018.00466/full#supplementary-material
Abbreviations
cHOMA-B, HOMA-beta cell function residuals corrected for HOMA-IR; chr, chromosome; FPG, fasting plasma glucose; HOMA-B, HOMA-beta cell function; HWE, Hardy-Weinberg equilibrium; IBD, identity by descent; MetaboChip, Illumina Cardio-Metabo DNA Analysis BeadChip; MAF, minor allele frequency; PC, principal component; PCA, principal components analysis; SHFS, Strong Heart Family Study; SHS, Strong Heart Study; SNP, single nucleotide polymorphism.
References
Acton, K. J., Burrows, N. R., Moore, K., Querec, L., Geiss, L. S., and Engelgau, M. M. (2002). Trends in diabetes prevalence among American Indian and Alaska native children, adolescents, and young adults. Am. J. Public Health 92, 1485–1490. doi: 10.2105/AJPH.92.9.1485
Almgren, P., Lehtovirta, M., Isomaa, B., Sarelin, L., Taskinen, M. R., Lyssenko, V., et al. (2011). Heritability and familiality of type 2 diabetes and related quantitative traits in the Botnia Study. Diabetologia 54, 2811–2819. doi: 10.1007/s00125-011-2267-5
Amé, J. C., Spenlehauer, C., and de Murcia, G. (2004). The PARP superfamily. Bioessays 26, 882–893. doi: 10.1002/bies.20085
Balakrishnan, P., Navas-Acien, A., Haack, K., Vaidya, D., Umans, J. G., Best, L. G., et al. (2018). Arsenic-gene interactions and beta-cell function in the strong heart family study. Toxicol. Appl. Pharmacol. 348, 123–129. doi: 10.1016/j.taap.2018.03.034
Blangero, J., and Almasy, L. (1996). SOLAR: Sequential Oligogenic Linkage Analysis Routines. Population Genetics Laboratory Technical Report No. 6. San Antonio, TX: Southwest Foundation for Biomedical Research.
Burdick, J. T., Chen, W. M., Abecasis, G. R., and Cheung, V. G. (2006). In silico method for inferring genotypes in pedigrees. Nat. Genet. 38, 1002–1004. doi: 10.1038/ng1863
Chauhan, G., Spurgeon, C. J., Tabassum, R., Bhaskar, S., Kulkarni, S. R., Mahajan, A., et al. (2010). Impact of common variants of PPARG, KCNJ11, TCF7L2, SLC30A8, HHEX, CDKN2A, IGF2BP2, and CDKAL1 on the risk of type 2 diabetes in 5,164 Indians. Diabetes 59, 2068–2074. doi: 10.2337/db09-1386
Dupuis, J., Langenberg, C., Prokopenko, I., Saxena, R., Soranzo, N., Jackson, A. U., et al. (2010). New genetic loci implicated in fasting glucose homeostasis and their impact on type 2 diabetes risk. Nat. Genet. 42, 105–116. doi: 10.1038/ng.520
Dyke, B. (1996). PEDSYS: A Pedigree Data Management Software. San Antonio, TX: Southwest Foundation for Biomedical Research.
Fesinmeyer, M. D., Meigs, J. B., North, K. E., Schumacher, F. R., Buzkova, P., Franceschini, N., et al. (2013). Genetic variants associated with fasting glucose and insulin concentrations in an ethnically diverse population: results from the population architecture using genomics and epidemiology (PAGE) study. BMC Med. Genet. 14:98. doi: 10.1186/1471-2350-14-98
Franceschini, N., Almasy, L., MacCluer, J. W., Göring, H. H. H., Cole, S. A., Diego, V. P., et al. (2008). Diabetes-specific genetic effects on obesity traits in American Indian populations: the strong heart family study. BMC Med. Genet. 9:90. doi: 10.1186/1471-2350-9-90
Franceschini, N., Haack, K., Göring, H. H. H., Voruganti, V. S., Laston, S., Almasy, L., et al. (2013). Epidemiology and genetic determinants of progressive deterioration of glycaemia in American Indians: the strong heart family study. Diabetologia 56, 2194–2202. doi: 10.1007/s00125-013-2988-8
Haiman, C. A., Fesinmeyer, M. D., Spencer, K. L., Buzkova, P., Voruganti, V. S., Wan, P., et al. (2012). Consistent directions of effect for established type 2 diabetes risk variants across populations: the population architecture using genomics and epidemiology (PAGE) Consortium. Diabetes 61, 1642–1647. doi: 10.2337/db11-1296
Hanson, R. L., Guo, T., Muller, Y. L., Fleming, J., Knowler, W. C., Kobes, S., et al. (2013). Strong parent-of-origin effects in the association of KCNQ1 variants with type 2 diabetes in American Indians. Diabetes 62, 2984–2991. doi: 10.2337/db12-1767
Hanson, R. L., Muller, Y. L., Kobes, S., Guo, T., Bian, L., Ossowski, V., et al. (2014). A genome-wide association study in American Indians implicates DNER as a susceptibility locus for type 2 diabetes. Diabetes 63, 369–376. doi: 10.2337/db13-0416
Huertas-Vazquez, A., Nelson, C. P., Guo, X., Reinier, K., Uy-Evanado, A., Teodorescu, C., et al. (2013). Novel loci associated with increased risk of sudden cardiac death in the context of coronary artery disease. PLoS One 8:e59905. doi: 10.1371/journal.pone.0059905
Irvin, M. R., Wineinger, N. E., Rice, T. K., Pajewski, N. M., Kabagambe, E. K., Gu, C. C., et al. (2011). Genome-wide detection of allele specific copy number variation associated with insulin resistance in African Americans from the HyperGEN study. PLoS One 6:e24052. doi: 10.1371/journal.pone.0024052
Jenkins, A. B., Samaras, K., Carey, D. G. P., Kelly, P., and Campbell, L. V. (2000). Improved indices of insulin resistance and insulin secretion for use in genetic and population studies of type2 diabetes mellitus. Twin Res. 3, 148–151. doi: 10.1375/twin.3.3.148
Knowler, W. C., Pettitt, D. J., Saad, M. F., and Bennett, P. H. (1990). Diabetes mellitus in the Pima Indians: incidence, risk factors and pathogenesis. Diabetes Metab. Rev. 6, 1–27. doi: 10.1002/dmr.5610060101
Kovacs, P., Hanson, R. L., Lee, Y. H., Yang, X., Kobes, S., Permana, P. A., et al. (2003). The role of insulin receptor substrate-1 gene (IRS1) in type 2 diabetes in Pima Indians. Diabetes 52, 3005–3009. doi: 10.2337/diabetes.52.12.3005
Lee, E. T., Welty, T. K., Cowan, L. D., Wang, W., Rhoades, D. A., Devereux, R., et al. (2002). Incidence of diabetes in American Indians of three geographic areas: the strong heart study. Diabetes Care 25, 49–54. doi: 10.2337/diacare.25.1.49
Lee, E. T., Welty, T. K., Fabsitz, R., Cowan, L. D., Le, N.-A., Oopik, A. J., et al. (1990). The strong heart study. A study of cardiovascular disease in American Indians: design and methods. Am. J. Epidemiol. 132, 1141–1155. doi: 10.1093/oxfordjournals.aje.a115757
Lewis, J. P., Palmer, N. D., Hicks, P. J., Sale, M. M., Langefeld, C. D., Freedman, B. I., et al. (2008). Association analysis in african americans of European-derived type 2 diabetes single nucleotide polymorphisms from whole-genome association studies. Diabetes 57, 2220–2225. doi: 10.2337/db07-1319
Matthews, D. R., Hosker, J. P., Rudenski, A. S., Naylor, B. A., Treacher, D. F., and Turner, R. C. (1985). Homeostasis model assessment: insulin resistance and β-cell function from fasting plasma glucose and insulin concentrations in man. Diabetologia 28, 412–419. doi: 10.1007/BF00280883
McCarthy, M. I., and Zeggini, E. (2009). Genome-wide association studies in type 2 diabetes. Curr. Diab. Rep. 9, 164–171. doi: 10.1007/s11892-009-0027-4
Menke, A., Casagrande, S., Geiss, L., and Cowie, C. C. (2015). Prevalence of and trends in diabetes among adults in the United States, 1988-2012. JAMA 314, 1021–1029. doi: 10.1001/jama.2015.10029
Mills, G. W., Avery, P. J., McCarthy, M. I., Hattersley, A. T., Levy, J. C., Hitman, G. A., et al. (2004). Heritability estimates for beta cell function and features of the insulin resistance syndrome in UK families with an increased susceptibility to type 2 diabetes. Diabetologia 47, 732–738. doi: 10.1007/s00125-004-1338-2
Narayan, K. M., Boyle, J. P., Geiss, L. S., Saaddine, J. B., and Thompson, T. J. (2006). Impact of recent increase in incidence on future diabetes burden: U.S., 2005-2050. Diabetes Care 29, 2114–2116. doi: 10.2337/dc06-1136
North, K. E., Almasy, L., Goring, H. H., Cole, S. A., Diego, V. P., Laston, S., et al. (2005). Linkage analysis of factors underlying insulin resistance: strong heart family study. Obes. Res. 13, 1877–1884. doi: 10.1038/oby.2005.230
North, K. E., MacCluer, J. W., Devereux, R. B., Howard, B. V., Welty, T. K., Best, L. G., et al. (2002). Heritability of carotid artery structure and function: the strong heart family study. Arterioscler. Thromb. Vasc. Biol. 22, 1698–1703. doi: 10.1161/01.ATV.0000032656.91352.5E
North, K. E., Williams, J. T., Welty, T. K., Best, L. G., Lee, E. T., Fabsitz, R. R., et al. (2003). Evidence for joint action of genes on diabetes status and CVD risk factors in American Indians: the strong heart family study. Int. J. Obes. Metab. Disord. 27, 491–497. doi: 10.1038/sj.ijo.0802261
Patti, M. E. (2004). Gene expression in humans with diabetes and prediabetes: what have we learned about diabetes pathophysiology? Curr. Opin. Clin. Nutr. Metab. Care 7, 383–390. doi: 10.1097/01.mco.0000134359.23288.72
Pfützner, A., Derwahl, M., Jacob, S., Hohberg, C., Blümner, E., Lehmann, U., et al. (2010). Limitations of the HOMA-B Score for assessment of β-Cell functionality in interventional trials-results from the PIOglim study. Diabetes Technol. Ther. 12, 599–604. doi: 10.1089/dia.2010.0019
Poulsen, P., Levin, K., Petersen, I., Christensen, K., Beck-Nielsen, H., and Vaag, A. (2005). Heritability of insulin secretion, peripheral and hepatic insulin action, and intracellular glucose partitioning in young and old Danish twins. Diabetes 54, 275–283. doi: 10.2337/diabetes.54.1.275
Pruitt, K. D., Tatusova, T., and Maglott, D. R. (2006). NCBI reference sequences (RefSeq): a curated non-redundant sequence database of genomes, transcripts and proteins. Nucleic Acids Res. 35, D61–D65. doi: 10.1093/nar/gkl842
Rasmussen-Torvik, L. J., Guo, X., Bowden, D. W., Bertoni, A. G., Sale, M. M., Yao, J., et al. (2012). Fasting glucose GWAS candidate region analysis across ethnic groups in the multiethnic study of atherosclerosis (MESA). Genet. Epidemiol. 36, 384–391. doi: 10.1002/gepi.21632
Rong, R., Hanson, R. L., Ortiz, D., Wiedrich, C., Kobes, S., Knowler, W. C., et al. (2009). Association analysis of variation in/near FTO, CDKAL1, SLC30A8, HHEX, EXT2, IGF2BP2, LOC387761, and CDKN2B with type 2 diabetes and related quantitative traits in Pima Indians. Diabetes 58, 478–488. doi: 10.2337/db08-0877
Schroeder, B. C., Waldegger, S., Fehr, S., Bleich, M., Warth, R., Greger, R., et al. (2000). A constitutively open potassium channel formed by KCNQ1 and KCNE3. Nature 403, 196–199. doi: 10.1038/35003200
Shim, U., Kim, H. N., Sung, Y. A., and Kim, H. L. (2014). Pathway analysis of metabolic syndrome using a genome-wide association study of Korea associated resource (KARE) cohorts. Genomics Inform. 12, 195–202. doi: 10.5808/GI.2014.12.4.195
Song, Y., Manson, J. E., Tinker, L., Howard, B. V., Kuller, L. H., Nathan, L., et al. (2007). Insulin sensitivity and insulin secretion determined by homeostasis model assessment and risk of diabetes in a multiethnic cohort of women: the Women’s Health Initiative Observational Study. Diabetes Care 30, 1747–1752. doi: 10.2337/dc07-0358
Voight, B. F., Scott, L. J., Steinthorsdottir, V., Morris, A. P., Dina, C., Welch, R. P., et al. (2010). Twelve type 2 diabetes susceptibility loci identified through large-scale association analysis. Nat. Genet. 42, 579–589. doi: 10.1038/ng.609
Wallace, T. M., Levy, J. C., and Matthews, D. R. (2004). Use and abuse of HOMA modeling. Diabetes Care 27, 1487–1495. doi: 10.2337/diacare.27.6.1487
Welty, T. K., Rhoades, D. A., Yeh, F., Lee, E. T., Cowan, L. D., Fabsitz, R. R., et al. (2002). Changes in cardiovascular disease risk factors among American Indians: the strong heart study. Ann. Epidemiol. 12, 97–106. doi: 10.1016/S1047-2797(01)00270-8
Willer, C. J., Li, Y., and Abecasis, G. R. (2010). METAL: fast and efficient meta-analysis of genomewide association scans. Bioinformatics 26, 2190–2191. doi: 10.1093/bioinformatics/btq340
Willett, W., and Stampfer, M. J. (1986). Total energy intake: implications for epidemiologic analyses. Am. J. Epidemiol. 124, 17–27. doi: 10.1093/oxfordjournals.aje.a114366
Yamagata, K., Senokuchi, T., Lu, M., Takemoto, M., Karim, M. F., Go, C., et al. (2011). Voltage-gated K channel KCNQ1 regulates insulin secretion in MIN6 β-cell line. Biochem. Biophys. Res. Commun. 407, 620–625. doi: 10.1016/j.bbrc.2011.03.083
Yang, J., Zhu, Y., Cole, S. A., Haack, K., Zhang, Y., Beebe, L. A., et al. (2012). A gene-family analysis of 61 genetic variants in the nicotinic acetylcholine receptor genes for insulin resistance and type 2 diabetes in American Indians. Diabetes 61, 1888–1894. doi: 10.2337/db11-1393
Yang, Q., Liu, T., Shrader, P., Yesupriya, A., Chang, M. H., Dowling, N. F., et al. (2010). Racial/ethnic differences in association of fasting glucose-associated genomic loci with fasting glucose, HOMA-B, and impaired fasting glucose in the U.S. adult population. Diabetes Care 33, 2370–2377. doi: 10.2337/dc10-0898
Keywords: American Indian, insulin-secreting cells, insulin resistance, genome-wide association study, diabetes mellitus
Citation: Balakrishnan P, Vaidya D, Voruganti VS, Haack K, Kent JW Jr, North KE, Laston S, Howard BV, Umans JG, Lee ET, Best LG, MacCluer JW, Cole SA, Navas-Acien A and Franceschini N (2018) Genetic Variants Related to Cardiometabolic Traits Are Associated to B Cell Function, Insulin Resistance, and Diabetes Among AmeriCan Indians: The Strong Heart Family Study. Front. Genet. 9:466. doi: 10.3389/fgene.2018.00466
Received: 12 June 2018; Accepted: 24 September 2018;
Published: 12 October 2018.
Edited by:
Daniel Shriner, National Human Genome Research Institute (NHGRI), United StatesReviewed by:
Kenneth M. Weiss, Pennsylvania State University, United StatesIvan Tkac, University of Pavol Jozef Šafárik, Slovakia
Copyright © 2018 Balakrishnan, Vaidya, Voruganti, Haack, Kent, North, Laston, Howard, Umans, Lee, Best, MacCluer, Cole, Navas-Acien and Franceschini. This is an open-access article distributed under the terms of the Creative Commons Attribution License (CC BY). The use, distribution or reproduction in other forums is permitted, provided the original author(s) and the copyright owner(s) are credited and that the original publication in this journal is cited, in accordance with accepted academic practice. No use, distribution or reproduction is permitted which does not comply with these terms.
*Correspondence: Poojitha Balakrishnan, cGJhbGFrcjJAamh1LmVkdQ==