- 1State Key Laboratory of Marine Environmental Science, Xiamen University, Xiamen, China
- 2College of Fisheries, Henan Normal University, Xinxiang, China
- 3Henan Academy of Fishery Sciences, Zhengzhou, China
- 4State Key Laboratory of Large Yellow Croaker Breeding, Ningde Fufa Fisheries Company Limited, Ningde, China
- 5Laboratory for Marine Biology and Biotechnology, Qingdao National Laboratory for Marine Science and Technology, Qingdao, China
Head size is important economic trait for many aquaculture fish which is directly linked to their carcass yield. The genetic basis of head size trait remains unclear in many widely cultured fish species. Common carp (Cyprinus carpio) is one of the most widely studied fish due to its importance on both economic and environmental aspects. In this study, we performed genome-wide association study using 433 Yellow River carp individuals from multiple families to identify loci and genes potentially associated with head size related traits including head length (HL), head length/body length ratio (HBR), eye diameter (ED), and eye cross (EC). QTL mapping was utilized to filter the effects of population stratification and improve power for the candidates identification in the largest surveyed family with a published genetic linkage map. Twelve SNPs showed significant for head size traits in GWAS and 18 QTLs were identified in QTL mapping. Our study combining both GWAS and QTL mapping could compensate the deficiency from each other and advance our understanding of head size traits in common carp. To acquire a better understanding of the correlation between head size and body growth, we also performed comparisons between QTLs of head size traits and growth-related traits. Candidate genes underlying head size traits were identified surrounding the significant SNPs, including parvalbumin, srpk2, fsrp5, igf1, igf3, grb10, igf1r, notch2, sfrp2. Many of these genes have been identified with potential functions on bone formation and growth. Igf1 was a putative gene associated with both head size and body growth in Yellow River carp. The teleost-specific igf3 was a candidate head size related gene, related to both HL and HBR. Our study also indicated the importance of Igf signaling pathway for both growth and head size determination in common carp, which could be potentially used in future selective breeding in common carp as well as other species.
Introduction
Head size related traits such as head length (HL), head length/body length ratio (HBR), eye diameter (ED) and eye cross (EC) are not only important morphological indicators for fish taxonomy, but also play important roles in fish behaviors and environmental adaptation like locomotion. Head size is also important economic trait in fish because of the direct effect on carcass yield. Dog is another representative species with various strains distinguished by head size. HL was regarded as growth related trait (Fu B. et al., 2016). ED and EC are responsible for vision, and may have impact on feeding, predator avoidance and so on. ED and EC has also been shown a positive correlation with body weight (BW) and body length, hence can be used for indirect selection for other commercial traits (Jin et al., 2012). Because of the great value of head size in biology and economics, researchers have attempted to unravel the genetic basis of head size determination for many years, with the final goal of the development of molecular markers suitable for phenotype prediction and eventually used in molecular marker-assisted breeding (MAS). However, understanding the associations between effect loci or genes and head size related traits is still at the early stage. As commercial traits, it is of great value to investigate the genetic architecture responsible for head size traits with the final goal to produce fish with extreme big or small head for ornamental or economic reasons.
Genome-wide association study (GWAS) and quantitative trait loci (QTL) mapping have been proved powerful in the identification and characterization of genes influencing complex traits (Fu Z. et al., 2016; Zhu et al., 2016). A huge effort has been invested using QTL and GWAS strategies with growing number of molecular genetic markers in aquatic animals. Most of them were on the topics of growth (Gutierrez et al., 2015; Peng et al., 2016), immunity (Vallejo et al., 2014; Xin et al., 2015), environmental adaptability (Jin et al., 2016; Wang et al., 2017), and also some focused on the genetic basis of body shape (Carmelo et al., 2016; Jing et al., 2016). In fish, several reports have already identified genomic loci or regions putatively involved in head size. QTLs associated with HL, ED, and EC were reported in a common carp gynogenetic line (Liu et al., 2009). HL, HBR, ED and EC related QTLs were also identified in German mirror carp (Wang et al., 2010; Jin et al., 2012). Multiple regions associated with HL, head width and head depth were reported in catfish (Geng et al., 2016). Many kind of molecular markers were used in previous studies, including amplified fragment length polymorphism (AFLP), simple sequence repeats (SSRs). With the development of sequencing technology, single-nucleotide polymorphisms (SNPs) are increasingly used in genetic studies. Most of the previous studies only reflect the genetic content of a limited number of markers, result in a failure to cover the whole genome, especially for the species with complex genomes, for instance, common carp, leading to difficulties in achieving accurate mapping of the related traits. In addition, lack of genomic and genetic maps makes it even more difficult for some species to conduct genetic analysis and localize gene precisely. In consideration of all these problems, it’s particularly important to improve marker density and the genome resources.
Common carp (Cyprinus carpio) is one of the most important aquaculture species with a long domestication history and is widely distributed from Asia to Europe. There are abundant populations and domesticated strains in China, including Yellow River carp, Hebao red carp, Oujiang color carp, Songpu mirror carp, as well as many hybrid strains. The genetic diversity and allotetraploidized genome of common carp lead an increasing interest in the studies of this special species (Xu P. et al., 2014). Great efforts have been made on genetic and genomic studies of common carp over the past decade. Abundant omics data have become available, including the comparative exomes study of Cyprinus carpio and Danio rerio (Henkel et al., 2012), the complete genome assembling and annotation (Xu P. et al., 2014) and the full-body transcriptome and proteome resource (Kolder et al., 2016). Besides, a large number of genetic markers have been developed, especially the SNP markers, which were used for the production of common carp 250 K SNP array with markers evenly distributed across the reference genome with an average spacing of 6.6 kb (Xu J. et al., 2014). Furthermore, a large number of genetic maps have been conducted over the past few years, including many important aquaculture species, such as catfish (Li et al., 2014), tilapia (Liu et al., 2013), Atlantic salmon (Gonen et al., 2014), as well as common carp. The recently published high density linkage map contains 28,194 SNP markers with an average locus interval of 0.75 cM (Peng et al., 2016). All these resources provide convenience and efficiency for the subsequent studies on evolutionary genomics, ecology, physiology, immunology, as well as the QTLs localization of significant traits.
In the current study, we were interested to unravel the genetic architecture of head size traits in Yellow River carp. For this purpose, we conducted GWAS using 433 Yellow River carp individuals from multiple families to identify loci potentially associated with head size related traits including HL, HBR, ED, and EC. QTL mapping was utilized in a full-sib family of Yellow River carp to improve power and make the result robust against population stratification and admixture. To get a view of candidate genes, we searched the reference genome to identify possible candidate genes surrounding the significant SNPs and QTL regions. Furthermore, KEGG pathway analysis was performed, which could increase our understanding of the potential molecular mechanisms underlying fish head size differentiation. Considering these results can provide some references for the phenotype prediction in common carp populations and can be of great value to future MAS. However, further analyses are needed in order to confirm these results.
Materials and Methods
Ethics Statement
This study was approved by the Animal Care and Use committee at College of Ocean and Earth Sciences, Xiamen University. The methods were carried out in accordance with approved guidelines.
Samples and Phenotypic Data
The Yellow River carp families were constructed at Breeding Station of Henan Academy of Fishery Research, Zhengzhou, China, using parents from different ancestors. The offspring were cultured in 2000 m2 pond and fed routinely under standard feeding regime. A total of 474 individuals were randomly collected 18 month post hatch from multiple families.
Traits including BW, BL, HL, EC and ED were measured, HBR was calculated accordingly. A Pearson’s correlation was tested to reflect the relationship between the phenotypic pairs, growth traits were included for comparison with head size traits.
Genotyping and Quality Control
Genomic DNA was extracted from blood samples using DNeasy 96 Blood and Tissue Kit (Qiagen, Shanghai, China), quantified by Nanodrop-1000 spectrophotometer (Thermo Scientific, Wilmington, DE, United States). After integrity examination with agarose gel electrophoresis, genomic DNA samples were diluted to the final concentration of 50 ng/μl for genotyping hybridization. The genotyping process had been described in previous study (Peng et al., 2016). Briefly, DNA samples were genotyped with common carp 250K SNP array at GeneSeek (Lincoln, NE, United States). Custom perl scripts were used for data cleaning and assessment. Affymetrix Genotyping Console software (version 4.0) was used for quality control and Affymetrix Axiom GT1 algorithm was used for SNP calling. SNPs with call rates greater than 95% were collected for further analysis. All individuals with missing genotypes > 5% and SNPs with missing genotypes > 5%, minor allele frequency < 5% were removed using specific parameters in PLINK, leaving 433 individuals (Supplementary Table S3) and 106,959 SNPs for the association analysis. Only the SNPs that are heterozygous in at least one parent and are conformed to Mendelian inheritance were used for QTL mapping. The genetic linkage map contains 28,194 SNP markers with a total length of 14,146 cM and an average marker interval of 0.75 cM (Peng et al., 2016).
Genome-Wide Association Study
Because the individuals were from several families (Supplementary Table S3), sample structure based on a centered relatedness matrix in GEMMA was drawn using SNPs remained after quality control. The first and second dimensions were plotted using R package (Supplementary Figure S2).
PLINK was initially used to perform genome-wide association analyses. Genome-wide empirical p-values were corrected by 100,000 max(T) permutations. The genomic inflation factor (λ, based on median chisq) ranged from 1.50 to 8.73 for the different phenotypes (Table 1), suggesting the population stratification. To adjust for population stratification and relatedness, GWAS was additionally performed using the GEMMA software, in which a centered relatedness matrix estimated from genotypes for each phenotype could be used for association studies. GEMMA implements the Genome-wide Efficient Mixed Model Association algorithm and the GWAS was performed with the relatedness matrix, phenotype and genotype fitted into a univariate linear mixed model as follows:
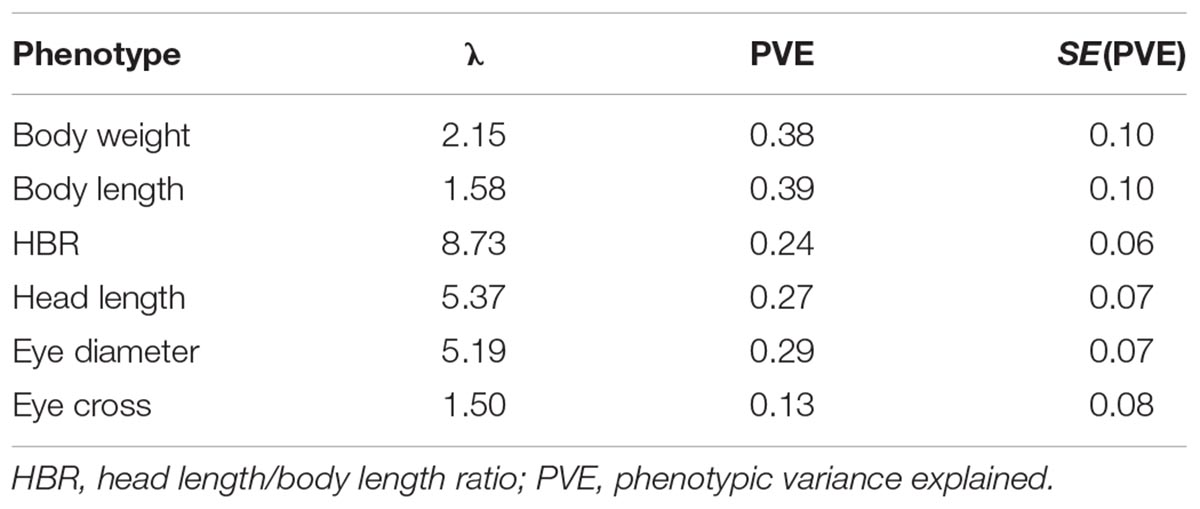
TABLE 1. Genomic inflation factor (λ) from the initial GWAS in PLINK, PVE or “chip heritability” in GEMMA.
where y is a vector of phenotypes, W is a covariate matrix with the vector α of the fixed effects including the intercept, x is a vector of marker genotypes and the effect size of the marker is β, u is a vector of random effects and 𝜀 is a vector of residual errors. Wald frequentist test was chosen to test for significance and Bonferroni threshold for P < 0.05 was determined as genome-wide significance. Furthermore, GEMMA estimates the proportion of variance in the phenotypes explained (PVE or “chip heritability”). Manhattan plot representing the −log10(P-value) and QQ plot expressing the expected and observed p-value were produced using R software.
Given that none of the SNPs from GEMMA reached the Bonferroni threshold for P < 0.05, we further tried TASSEL software and performed GWAS with the same batch of data. The Kinship matrix generated from TASSEL was regarded as a covariate to improve statistical power. The mixed liner model (MLM) comprising the Q + K model was used to perform GWAS between phenotypes and genotypes.
In this study, −log10(1/n) was used as a threshold because the Bonferroni test (0.05/numbers of SNPs) criterion is extremely strict to be a threshold, considering GWAS is hypothesis generating (Yang et al., 2011; Wang et al., 2012). The threshold of suggestive association was arbitrarily set as −log10(P) > 4.5.
A within-family test of association might have less power but could control better for possible population stratification (Menzel et al., 2007), therefore we conduct GWAS of G1 family using several models including Multi-locus mixed model GWAS (MLMM) in Mixed Linear Model Analysis in SVS 8.8.1 (Golden Helix)1, Genome-wide Efficient Mixed Model in GEMMA and QFAM in PLINK (Purcell et al., 2007) as comparisons to the QTL results.
QTL Mapping
QTL mapping with head size phenotypes from 103 individuals was performed with the high density genetic linkage map recently published (Peng et al., 2016). QTL mapping was performed using MapQTL 6.0 software with “multiple QTL model (MQM) mapping” method using “Mixture Model” algorithms. LOD significance threshold levels were determined by Permutation Test on the basis of 1,000 permutations for the traits. QTL with LOD scores exceeding the chromosome-wide LOD threshold at P < 0.01 or the genome-wide LOD threshold at P < 0.05 were considered as significant.
Candidate Genes
We mapped candidate SNPs and QTL regions onto the common carp genome to identify candidate genes associated with head size traits. Briefly, we screened the ± 50 K bp genome regions surrounding the significant SNPs based on common carp reference genome that released at GeneBank (Accession number: GCF_000951615.1)2, and annotated the candidate genes accurately by BLAST against the non-redundant protein database.
For better understand the genetic mechanism, pathway analysis was performed using online tools KEGG3 and Omicshare4.
Results and Discussion
Traits Measurement and Analysis
Phenotypic data used in GWAS were shown in Supplementary Table S1. BW of the samples ranged from 815 to 1,895 g. Body length ranged from 30.70 to 46.90 cm. HL was 7.87 ± 0.72 cm (Mean ± SD); HBR was 0.23 ± 0.01; ED was 1.12 ± 0.14 cm; EC was 3.88 ± 0.34 cm. Phenotypic data used in QTL mapping were also listed in Supplementary Table S1.
There was a certain degree of phenotypic correlation between phenotypic traits (Supplementary Figure S1), and most of them reached a significant level of 0.05, among which, more than half of the correlation coefficients reached a very significant level of 0.01. There was a very strong, positive correlation between BW and body length (r = 0.859, p < 0.01). HL was moderately correlated with BW (r = 0.381, p < 0.01) and weakly correlated with body length (r = 0.256, p < 0.01). There was a weak, negative correlation between HBR and BW (r = −0.225, p < 0.05), a weak and negative correlation between HBR and body length (r = −0.354, p < 0.01). A moderate, positive correlation was observed between EC and BW (r = 0.515, p < 0.01), EC and body length (r = 0.518, p < 0.01). No strong phenotypic correlations were observed within head size traits, including a moderate correlation between HL and ED (r = 0.498, p < 0.01), a weak correlation between HL and EC (r = 0.312, p < 0.01) and a weak correlation between ED and EC (r = 0.247, p < 0.05). No significant correlation was found between HBR and EC, ED and BW, ED and BW. The results of pairwise comparisons indicated the phenotypic correlations among the related traits, which might suggest the potential relevance underlying the genetic basis, and provide valuable information for the following genetic localization of the related traits.
Head size had been previously studied in catfish, which focused on head length, head width and head depth (Geng et al., 2016). Relevant studied were performed mostly in Songpu mirror carp and the related hybrids, including HL, EC, ED, HBR with few molecular markers (Liu et al., 2009; Wang et al., 2010; Xing et al., 2011; Shan et al., 2014). Related research was rarely reported in the Yellow River carp, except for the recent publication focusing on growth-related traits (Peng et al., 2016). HL was reported to be important for determining the weight of rounded fish (Reis Neto et al., 2012). The direct effect of HL on BW in Large Yellow Croaker was greater than that of body length (Liu et al., 2008). Given that HBR has similarity with HL and could better reflect the meat yield, it is of great interest to investigate the genetic basis of HBR to provide insights into head size determination.
GWAS and QTL Mapping of Head Size Related Traits
Twelve SNPs reached the genome-wide significance (−log10P-value > 5.02) in GWAS distributed on 6 linkage groups (LGs), including 4 SNPs for HL, one single for HBR, 4 for ED and 4 for EC (Table 2 and Figure 1). Interestingly, HL and EC shared the same snp166316, with p-value 6.06E-6 and 9.26E-7, respectively. There were 23 SNPs suggestively associated with head size with −log10(P-value) > 4.5. The phenotypic variance explained (PVE) by genetic factors was no more than 27% for HL, 24% for head/body length ratio, 29% for ED and 13% for EC (Table 1).
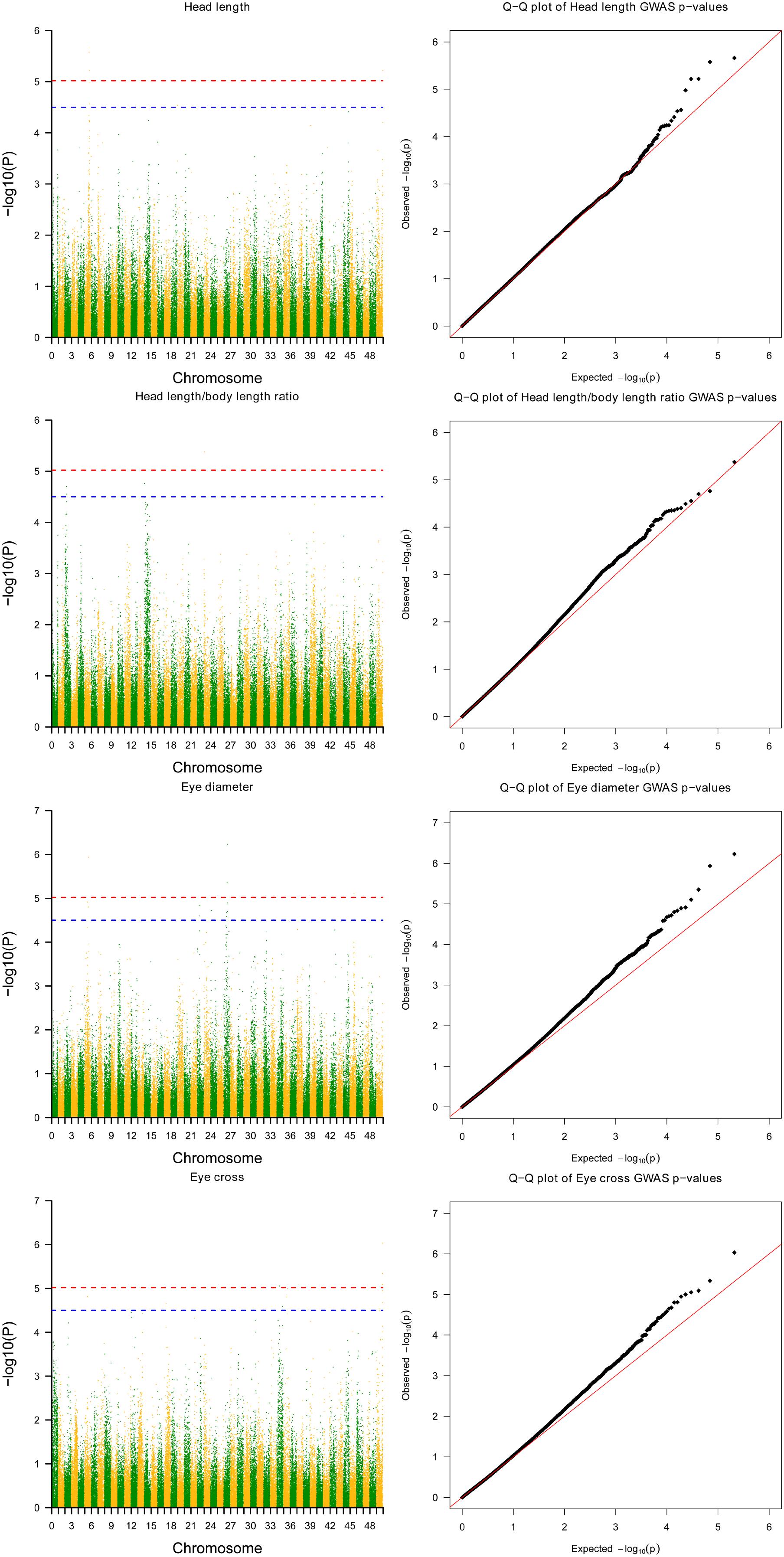
FIGURE 1. Results from genome-wide association study on head size related traits in Yellow River carp. Significance levels are shown by the red line [–log10(1/n) = 5.02] and suggestive the blue line (4.5). HL, head length; HBR, head length/body length ratio; ED, eye diameter; EC, eye cross.
In QTL mapping, a total of 18 QTL regions associated with head size (Table 3) were identified based on chromosome-wide LOD significance with P < 0.01. The large distribution of the QTLs suggested the complex genetic control of head size. The 18 QTL regions harbored 151 significant SNPs, including 47 SNPs for HL, 61 SNPs for head/body length ratio, 23 SNPs for ED and 20 for EC. Three QTLs were identified for HL (Figure 2 and Table 3), explaining between 17.4 and 51% of the phenotypic variance. The most significant region was located on LG15 at 29.95–37.31 cM, presenting the highest LOD value of 6.25, explaining the highest total phenotypic variation of 51%. Eight QTLs for HBR were distributed on seven LGs (Figure 3 and Table 3). Surprisingly, HBR15 shared almost the same interval with HL15, demonstrating the similarity between the both traits, which agreed with the result of phenotypic comparison. Another QTL sharing the same LOD score as HBR15 was HBR8, explaining 20.7% of the total PVE. The interval of HBR8, was also the smallest one with a range from 110.97 to 111.27 cM and included 7 SNP markers. Interestingly, the HBR8 shared almost all the SNPs with qBW8a, a QTL responsible for BW detected in our previous study (Peng et al., 2016) (Supplementary Figure S3), which strongly proved the correlation between BW and HBR, suggesting that HBR could be an indirect indicator of BW in common carp. While, QTLs for BW shared less with that of HL and HBR. For ED, three QTLs were identified, distributed on two LGs as listed below (Figure 4 and Table 3), explained between 13.4 and 24.2% of the total PVE. Four QTLs were discovered for EC and explained 13.5–21.4% of the total PVE (Figure 5 and Table 3). QTL mapping for the four head size traits showed significant differences among QTL profiles with only a few overlaps, implying different but interactional genetic basis underlying these traits.
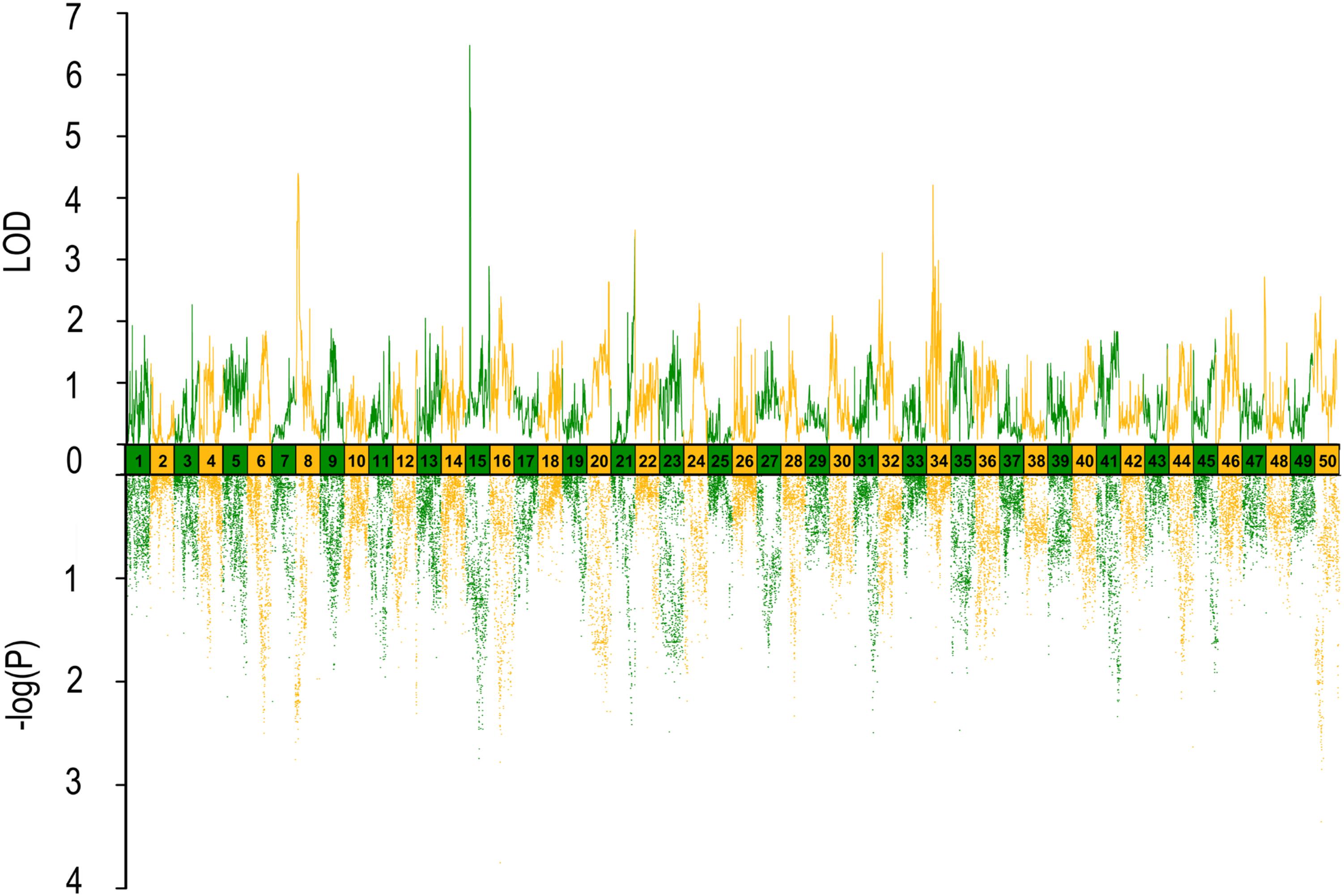
FIGURE 2. QTL mapping and GWAS of head length in Yellow River carp. Results are based on mapQTL 6 and SVS using a single full-sib family.
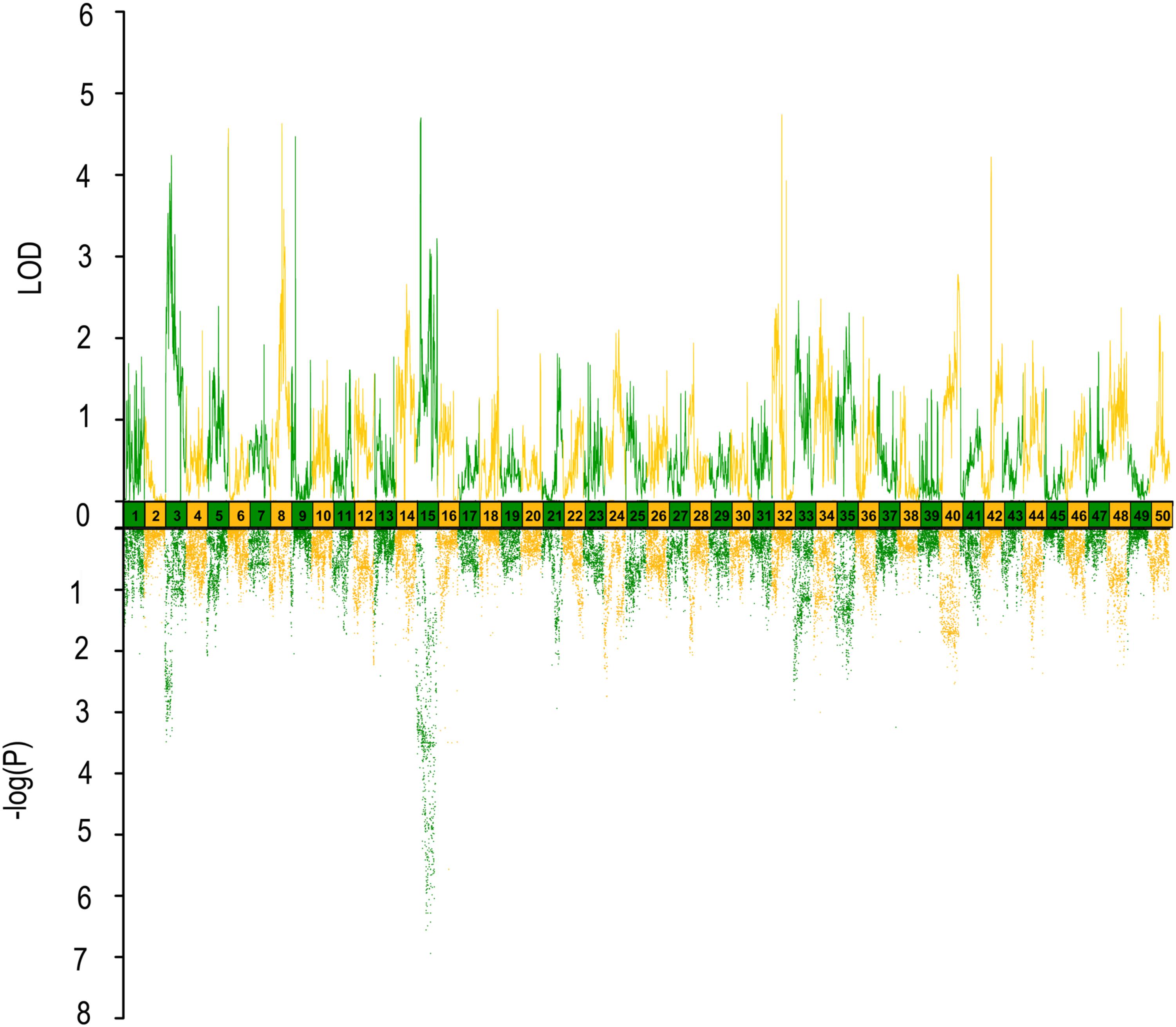
FIGURE 3. QTL mapping and GWAS of head length/body length ratio in Yellow River carp. Results are based on mapQTL 6 and SVS using a single full-sib family.
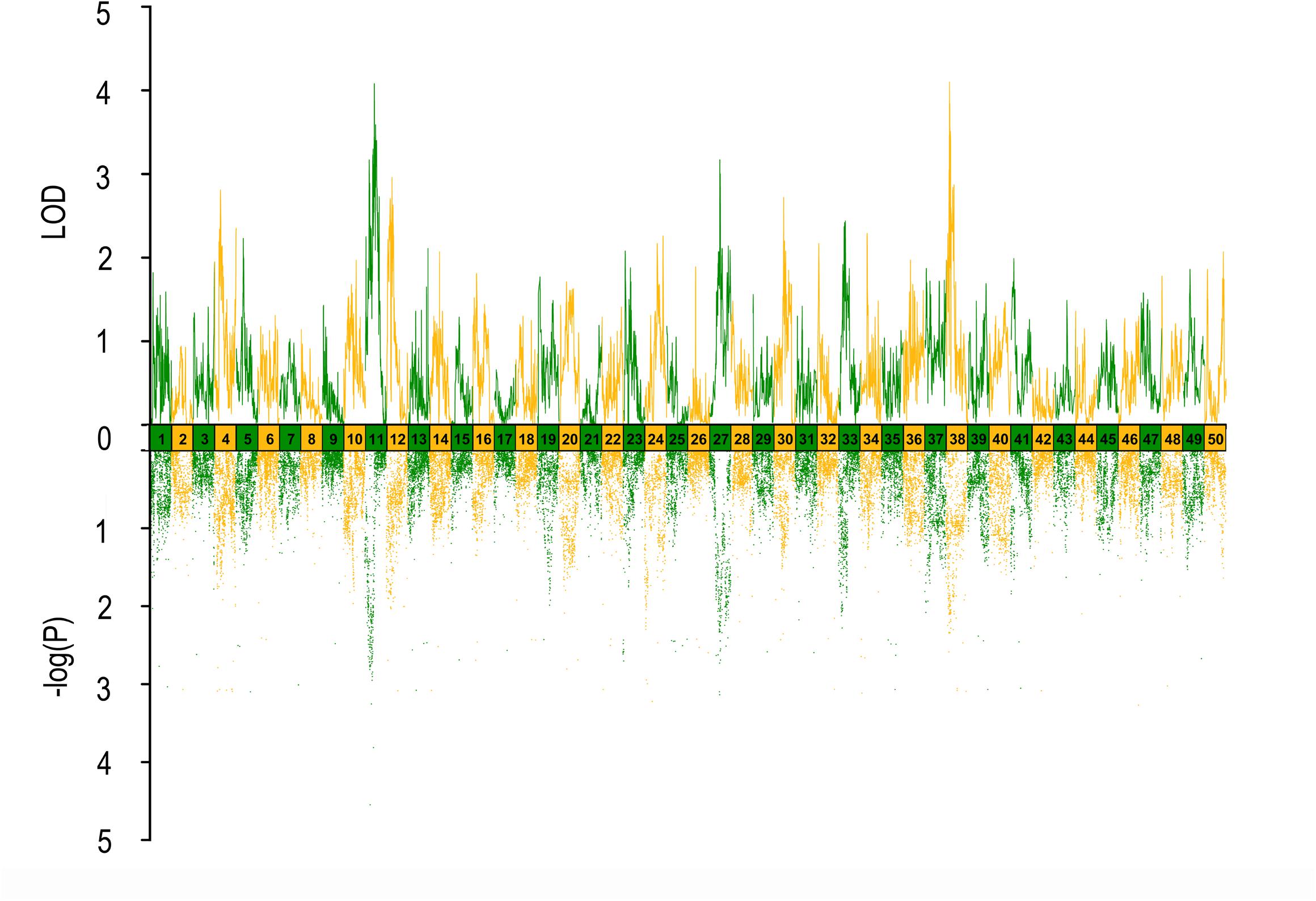
FIGURE 4. QTL mapping and GWAS of eye diameter in Yellow River carp. Results are based on mapQTL 6 and SVS using a single full-sib family.
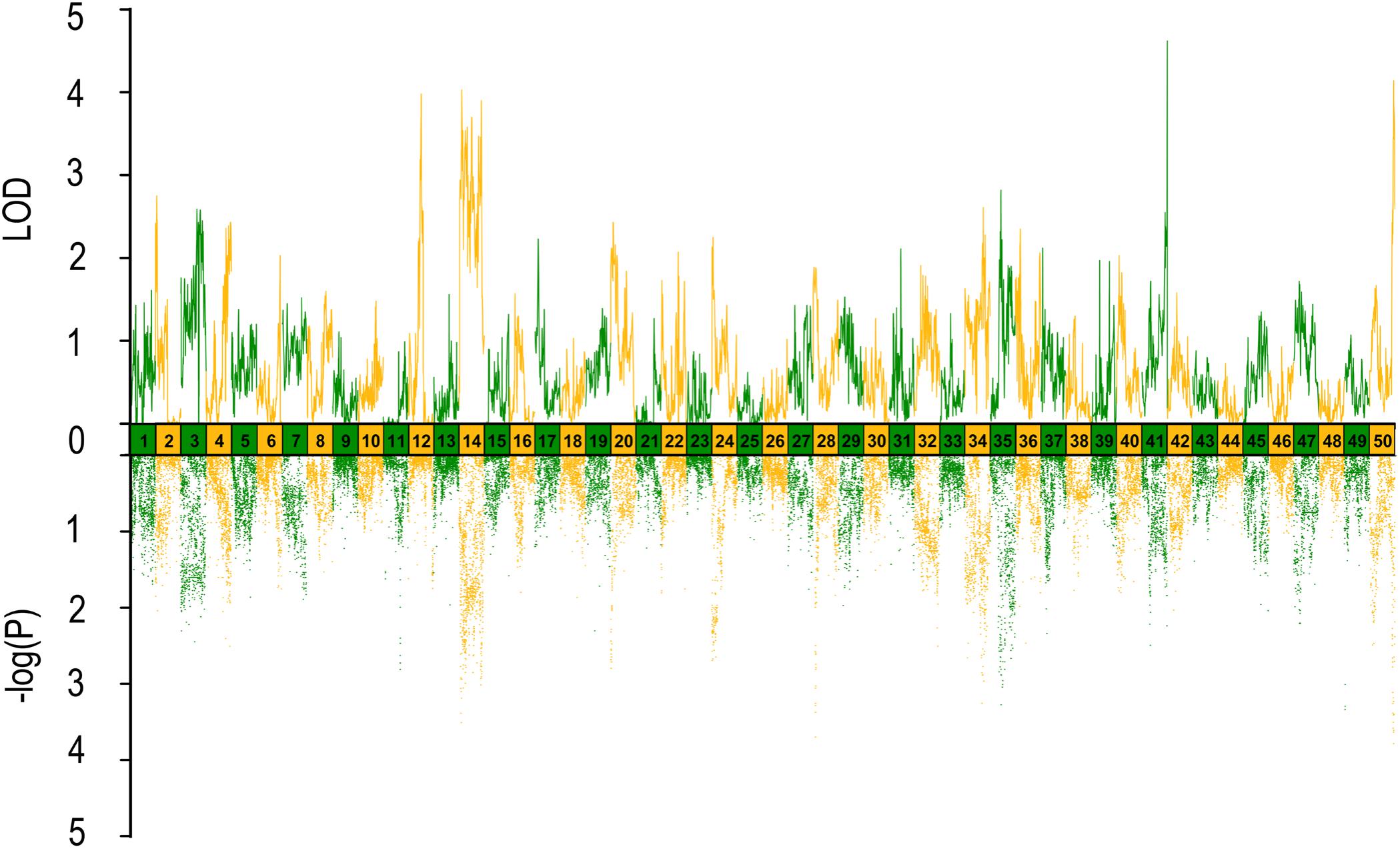
FIGURE 5. QTL mapping and GWAS of eye cross in Yellow River carp. Results are based on mapQTL 6 and SVS using a single full-sib family.
Genome-wide association methodology has recently identified amount of loci for several traits in fish (Geng et al., 2016, 2017). GWAS with dense markers could get closer to the important gene with large enough sample size, but it has a relative high per-sample cost and requires large samples to detect the modest risk effects. QTL mapping in common carp has identified various QTLs associated with head size or other traits (Wang et al., 2010; Shan et al., 2014), some of their results seemed to show some similarity to ours. While, previous studies focusing on head size traits were limited to marker density and could hardly identify traits-related loci accurately. With the popularity of SNP genotyping array, fast, high density and accurate genotyping becomes available in aquaculture specie (Li et al., 2014; Xu J. et al., 2014), which with no doubt facilitate the fine mapping of important traits. The genetic map of common carp provides a dense chromosome framework to correctly order the genome scaffolds and locate QTLs on chromosomes and hence localize more promising candidate genes.
Strategies to increase power of genetic localization include increasing the sample size and marker density or using a comparatively small number of study subjects taken from the extremes of a quantitative distribution (Menzel et al., 2007). Looking back to the GWAS results, none of the markers achieved the Bonferroni threshold of 0.05, which might suggest the less power of our GWAS caused by environment or some other uncertain factors. Then single family based GWAS or QTL analysis could compensate for the deficiency to some extent, making the result robust against population stratification and admixture, both of which can cause potential false-positive associations (Marchini et al., 2004). In fact, the single full-sib family based GWAS using three models all showed high similarity with the result of QTL because of the filtering of population structure and uncertain variables, and MLMM in SVS was remained at last. From this point of view, QTL mapping might be more powerful and allows identification of rare alleles. Combining both the GWAS and QTL would provide more comprehensive understanding of the genetic architecture of head size related traits. It is reasonable that further studies are required to elucidate and confirm the effects of associated loci.
Candidate Genes Related to Head Size Traits
Fifteen genes were extracted significantly and 32 genes were suggestively associated with head size traits based on GWAS results (Table 4 and Supplementary Table S2). For HL, parvalbumin (parv) was identified on LG6. Parvalbumin is a muscle protein that aids in relaxation from contraction, patterns of parvalbumin expression determine relaxation rate along the length of the fish (Wilwert et al., 2006). Increased Parvalbumin protein concentration improved both sprint and sustained locomotion in zebrafish (Danio rerio), which is linked to fitness and health of animals (Seebacher and Walter, 2012). Furthermore, parvalbumin is involved in Insulin-like growth factors (Igf) signaling (Latres et al., 2005). One of the parvs was significantly associated with BW and body length in Asian seabass (Xu et al., 2006). Both GWAS and QTL identified the SRSF protein kinases (SRPKs), with srpk2 on LG50 and srpk1 on LG15, respectively, suggesting the important effects on HL. Interleukin-3 receptor class 2 subunit beta (il3rb2) was another gene identified for HL, the elevated expression of which improved hematopoietic recovery and facilitated bone marrow recovery (Chen et al., 2016). Only one gene associated with HBR was identified, named PH and SEC7 domain-containing protein 1 (psd), but the effect on head size remained unclear.
For ED, follistatin-related protein 5 was identified on LG27. Follistatin-related genes are major negative regulators of myostatin in vivo and could block some bone morphogenetic proteins (Amthor et al., 2002; Hill et al., 2002). Overexpression of follistatin in skeletal muscle of mice could result in double-muscle phenotype (Lee and Mcpherron, 2001). Suggestively associated genes including fibroblast growth factor receptor-like 1 (fgfrl1), which is a member of the fibroblast growth factor receptor family that controls the formation of musculoskeletal tissues and skull (Iseki et al., 1999; Beyeler and Trueb, 2006). Srpk2 was identified both for HL and EC in GWAS, revealing the overlapping genetic control of the both traits.
A total of 193, 223, 56, and 73 genes were identified for HL, HBR, ED and EC, respectively in QTL mapping (Table 5 and Figure 6). Pathway analysis showed that 176 of these genes were enriched in several related pathways, including FOXO signaling pathway, TGF-beta signaling pathway, WNT signaling pathway, and so on (Supplementary Figures S4–S10). HL15 and HBR15 shared almost all the candidates. Interestingly, we identified igf3 in HL15 and HBR15, explaining relatively high total phenotypic variations. Significant attention has been paid to the functions of the fish specific gene, igf3 in gonad development and its interaction with growth hormone pathways (Wang et al., 2008; Nelson and Van Der Kraak, 2010; Wan and Chan, 2010; Li et al., 2015). The candidate gene nras identified in HL15 belongs to small GTPases, which were important for head formation in early Xenopus development (Wunnenberg-Stapleton et al., 1999). We also identified igf1 in the region of HBR8, which was previously reported to be possibly associated with BW in common carp (Peng et al., 2016), revealing the genetic overlap between growth related traits and head size traits. Igf1 is also an excellent candidate gene closely associated with body size, birth weight, head formation and head size (Richard-Parpaillon et al., 2002; Sutter et al., 2007; Reynaud et al., 2010; Forman, 2013; Geng et al., 2016). Thereafter, we could speculate that Igf signaling pathway might show more potential for body growth and head size in common carp by cross talk with other related signaling, for example FOXO signaling pathway (Supplementary Figure S5).
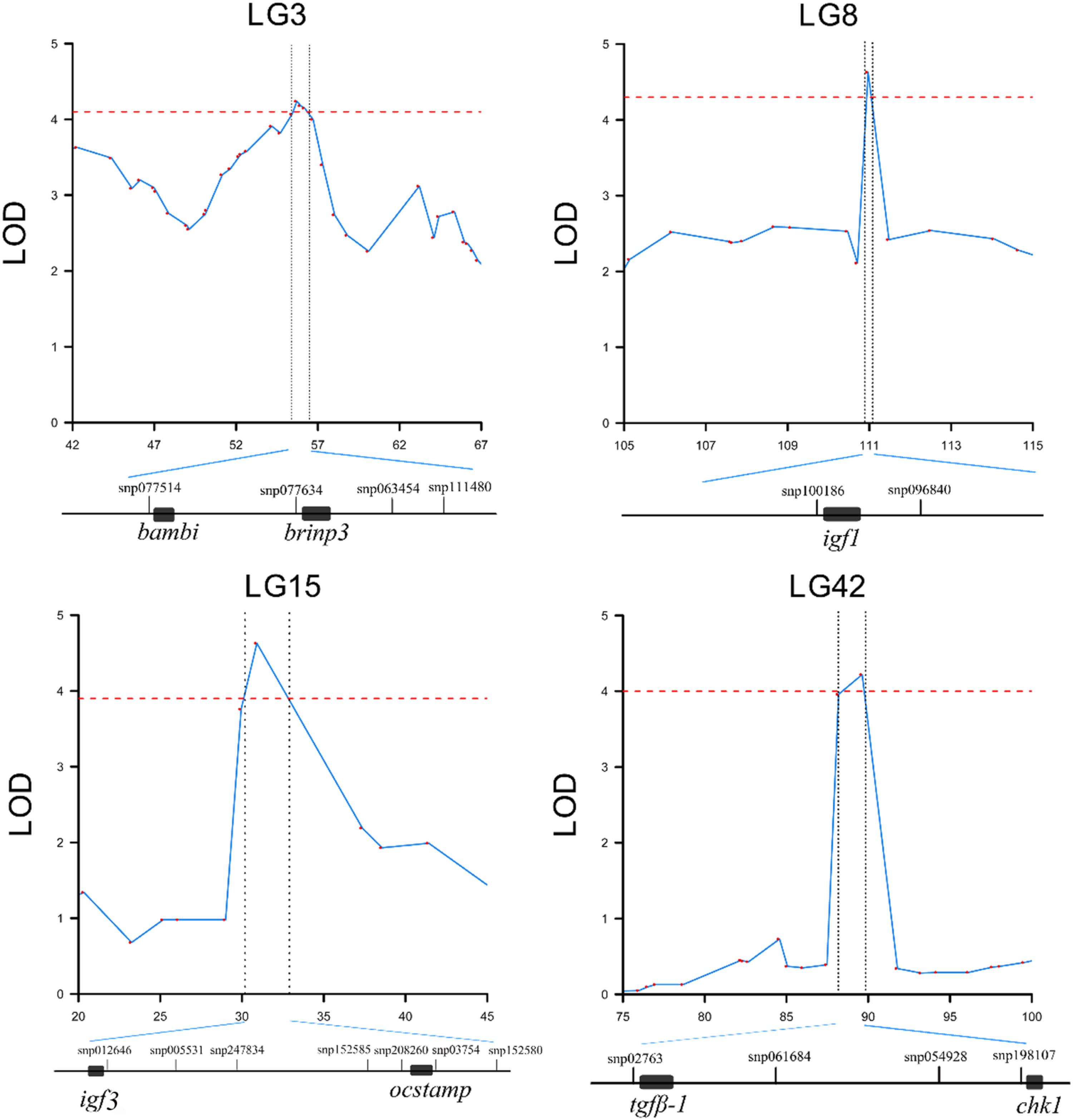
FIGURE 6. QTL region and candidate genes for head length/body length ratio in Yellow River carp. Genes are extracted in the ± 50 K bp genome regions around the significant SNPs. Black rectangles represent the candidate genes.
BMP and activin membrane-bound inhibitor homolog (bambi) was identified as a candidate gene in the genome region of HBR3. Bambi is a BMP signaling transmembrane glycoprotein and functions as a negative regulator of TGF-β signaling during development (Sekiya et al., 2004) (Supplementary Figure S6). Thus, bambi is seemingly to have role in shaping the HBR in Yellow River carp through bone or growth regulation. On LG15, we also identified neurogenic locus notch homolog protein 2 (notch2) in the QTL region of HBR15, which was in the Grk/Egfr pathway (Supplementary Figure S8) and played critical roles in regulation of bone mass (Simpson et al., 2011), hence may leading to some effects on HBR. As for HBR32, growth factor receptor-bound protein 10 (grb10, also known as insulin receptor-binding protein) was identified, which was an interacting partner of the igf1 receptor (igf1r) and insulin receptor (ir) (Dey et al., 1996; Vecchione et al., 2003). It could be speculated that the binding of grb10 to igf1r inhibits the binding of igf1 to the ligand igf1r and then affects the Igf signaling, leading an impact on head size.
For ED, we identified separin (esp1), a gene proposed to be required for embryonic anterior-posterior axis formation together in Caenorhabditis elegans (Rappleye et al., 2002). In ED38, glycogen synthase kinase-3 beta (gsk3b) was identified (Supplementary Figure S9), which functions as a negative regulator of IL-17-mediated inflammatory responses, and was depended by IL-17-induced chemokine expression enhanced by igf1, thus involved in Obesity (Ge et al., 2013). For EC, we identified igf1r in EC14. A non-synonymous mutation in igf1r contributed to reduce size in dogs (Hoopes et al., 2012). In EC50, secreted frizzled-related protein 2 (sfrp2) was annotated. Sfrp2 was a Wnt signaling antagonist with known function during eye lens development (Lin et al., 2007; Sugiyama et al., 2013) and potential functions on body conformation in catfish (Geng et al., 2017). Certainly, some of these genes within QTL regions might have unknown but related functions.
Overall, both the literature data and our present study show that Igf signaling pathways and some other signaling pathways related to bone and muscle development may play crucial roles in head formation and head size determination together with body growth. Genetic modification and selection of the related loci or genes may subsequently affect the phenotypes and provide more insights in the future molecular marker assisted breeding.
Conclusion
Here we performed GWAS and QTL mapping strategies to identify loci and genes associated with head size. GWAS identified 12 SNPs significantly associated with head size. A total of 18 QTLs were identified in QTL mapping, revealing the polygenic nature of these complex traits. Candidate genes were identified around the significant SNPs, including parv, fsrp5, igf1 and igf3, grb10, igf1r, sfrp2, indicating the importance of Igf signaling as well as some other bone and muscle development related signaling pathways in head size determination. The combination of both GWAS and QTL provides more understanding for fish head formation and could be considered in genetic breeding program on common carp for economical purpose on both carcass yield and ornamental value.
Data Availability
The common carp reference genome used in this study is accessible either at CarpBase (http://www.carpbase.org) in the Help/Download section or in the GeneBank with Accession number of GCF_000951615.1 (https://www.ncbi.nlm.nih.gov/assembly/GCF_000951615.1/). All raw sequencing data have been deposited in the NCBI Sequence Read Archive (SRA: SRP026407).
Author Contributions
PX conceived and supervised the study. LC analyzed the data and drafted the manuscript. WP and BC helped on data analysis. SK, FP, and ZZ helped on manuscript preparation. JF helped on carp family construction and sample collection. PX and XL revised the manuscript. All the authors have read and approved the manuscript.
Funding
We acknowledge grant support from the National Natural Science Foundation of China (No. 31422057), the Local Science and Technology Development Project Guide by The Central Government (2017L3019), Fundamental Research Funds for the Central Universities, Xiamen University (20720160110), the Fundamental Research Funds for the Central Universities (20720180123), and State Key Laboratory of Large Yellow Croaker Breeding (Fujian Fuding Seagull Fishing Food Co., Ltd.) (LYC2017RS05).
Conflict of Interest Statement
The authors declare that the research was conducted in the absence of any commercial or financial relationships that could be construed as a potential conflict of interest.
Supplementary Material
The Supplementary Material for this article can be found online at: https://www.frontiersin.org/articles/10.3389/fgene.2018.00448/full#supplementary-material
FIGURE S1 | Phenotypic correlation of the related traits in Yellow River carp. Circle size was distinguished by correlation coefficient; x indicate no correlation.
FIGURE S2 | Sample structure for GWAS identified by a centered relatedness matrix in GEMMA. The first dimension (mds 1) was assigned to X axis, and the second dimension (mds 2) was assigned to Y axis. Individuals from G1 (the QTL family) were circled in pink.
FIGURE S3 | Comparison of growth traits and head size traits in Yellow River carp. HL, head length; HBR, head length/body length ratio; BW, body weight; BL, body length.
FIGURE S4 | KEGG pathway enrichment of the candidates from QTL. Gene number was assigned to X axis, pathways were assigned to Y axis.
FIGURE S5 | QTL candidates in FOXO signaling pathway. The candidates were marked in red. The plots were drawn using KEGG online tools.
FIGURE S6 | QTL candidates in TGF-BETA signaling pathway. The candidates were marked in red. The plots were drawn using KEGG online tools.
FIGURE S7 | QTL candidates in WNT signaling pathway. The candidates were marked in red. The plots were drawn using KEGG online tools.
FIGURE S8 | QTL candidates in Grk/Egfr pathway. The candidates were marked in red. The plots were drawn using KEGG online tools.
FIGURE S9 | QTL candidates in INSULIN signaling pathway. The candidates were marked in red. The plots were drawn using KEGG online tools.
FIGURE S10 | QTL candidates in CALCIUM signaling pathway. The candidates were marked in red. The plots were drawn using KEGG online tools.
TABLE S1 | Phenotypic data of Yellow River carp in this study. Min, minimum; Max, maximum; SD, standard deviation.
TABLE S2 | Genes suggestively associated with head size of Yellow River carp. HL, head length; HBR, head length/body length ratio; ED, eye diameter; EC, eye cross.
TABLE S3 | Individuals and family information of common carp. NA: information lost.
Footnotes
- ^ http://www.goldenhelix.com
- ^ https://www.ncbi.nlm.nih.gov/assembly/GCF_000951615.1/
- ^ http://www.genome.jp/kegg/pathway.html
- ^ http://www.omicshare.com/tools/Home/Soft/pathwaygsea
References
Amthor, H., Christ, B., Rashid-Doubell, F., Kemp, C. F., Lang, E., and Patel, K. (2002). Follistatin regulates bone morphogenetic protein-7 (BMP-7) activity to stimulate embryonic muscle growth. Dev. Biol. 243, 115–127. doi: 10.1006/dbio.2001.0555
Beyeler, M., and Trueb, B. (2006). Fgfrl1, a fibroblast growth factor receptor-like gene, is found in the cephalochordate Branchiostoma floridae but not in the urochordate Ciona intestinalis. Comp. Biochem. Physiol. Part B 145, 43–49. doi: 10.1016/j.cbpb.2006.06.002
Carmelo, F., Paolo, F., Viera, K., Elmer, K. R., Frederico, H., and Axel, M. (2016). Genetic linkage of distinct adaptive traits in sympatrically speciating crater lake cichlid fish. Nat. Commun. 7:12736. doi: 10.1038/ncomms12736
Chen, J.-J., Gao, X.-T., Yang, L., Fu, W., Liang, L., Li, J.-C., et al. (2016). Disruption of Notch signaling aggravates irradiation-induced bone marrow injury, which is ameliorated by a soluble Dll1 ligand through Csf2rb2 upregulation. Sci. Rep. 6:26003. doi: 10.1038/srep26003
Dey, B. R., Frick, K., Lopaczynski, W., Nissley, S. P., and Furlanetto, R. W. (1996). Evidence for the direct interaction of the insulin-like growth factor I receptor with IRS-1. Shc, and Grb10. Mol. Endocrinol. 10, 631–641.
Forman, M. R. (2013). Maternal BMI, IGF-I Levels, and birth weight in African American and white infants. Int. J. Pediatr. 2013:191472. doi: 10.1155/2013/191472
Fu, B., Liu, H., Yu, X., and Tong, J. (2016). A high-density genetic map and growth related QTL mapping in bighead carp (Hypophthalmichthys nobilis). Sci. Rep. 6:28679. doi: 10.1038/srep28679
Fu, Z., Li, W., Xing, X., Xu, M., Liu, X., Li, H., et al. (2016). Genetic analysis of arsenic accumulation in maize using QTL mapping. Sci. Rep. 6:21292. doi: 10.1038/srep21292
Ge, D., Dauchy, R. T., Liu, S., Zhang, Q., Mao, L., Dauchy, E. M., et al. (2013). Insulin and IGF1 enhance IL-17-induced chemokine expression through a GSK3B-dependent mechanism: a new target for melatonin’s anti-inflammatory action. J. Pineal Res. 55, 377–387. doi: 10.1111/jpi.12084
Geng, X., Liu, S., Yao, J., Bao, L., Zhang, J., Li, C., et al. (2016). A genome-wide association study identifies multiple regions associated with head size in catfish. G3 6, 3389–3398. doi: 10.1534/g3.116.032201
Geng, X., Liu, S., Yuan, Z., Jiang, Y., Zhi, D., and Liu, Z. (2017). A genome-wide association study reveals that genes with functions for bone development are associated with body conformation in catfish. Mar. Biotechnol. 19, 570–578. doi: 10.1007/s10126-017-9775-3
Gonen, S., Lowe, N. R., Cezard, T., Gharbi, K., Bishop, S. C., and Houston, R. D. (2014). Linkage maps of the Atlantic salmon (Salmo salar) genome derived from RAD sequencing. BMC Genomics 15:166. doi: 10.1186/1471-2164-15-166
Gutierrez, A. P., Yáñez, J. M., Fukui, S., Swift, B., and Davidson, W. S. (2015). Genome-Wide association study (GWAS) for growth rate and age at sexual maturation in atlantic salmon (Salmo salar). PLoS One 10:e0119730. doi: 10.1371/journal.pone.0119730
Henkel, C. V., Dirks, R. P., Jansen, H. J., Forlenza, M., Wiegertjes, G. F., Howe, K., et al. (2012). Comparison of the exomes of common carp (Cyprinus carpio) and zebrafish (Danio rerio). Zebrafish 9, 59–67. doi: 10.1089/zeb.2012.0773
Hill, J. J., Davies, M. V., Pearson, A. A., Wang, J. H., Hewick, R. M., Wolfman, N. M., et al. (2002). The myostatin propeptide and the follistatin-related gene are inhibitory binding proteins of myostatin in normal serum. J. Biol. Chem. 277, 40735–40741. doi: 10.1074/jbc.M206379200
Hoopes, B. C., Rimbault, M., Liebers, D., Ostrander, E. A., and Sutter, N. B. (2012). The insulin-like growth factor 1 receptor (IGF1R) contributes to reduced size in dogs. Mamm. Genome 23, 780–790. doi: 10.1007/s00335-012-9417-z
Iseki, S., Wilkie, A. O., and Morriss-Kay, G. M. (1999). Fgfr1 and Fgfr2 have distinct differentiation- and proliferation-related roles in the developing mouse skull vault. Development 126, 5611–5620.
Jin, S., Zhang, X., Jia, Z., Fu, H., Zheng, X., and Sun, X. (2012). Genetic linkage mapping and genetic analysis of QTL related to eye cross and eye diameter in common carp (Cyprinus carpio L.) using microsatellites and SNPs. Aquaculture 358–359, 176–182. doi: 10.1016/j.aquaculture.2012.06.014
Jin, Y., Zhou, T., Geng, X., Liu, S., Chen, A., Yao, J., et al. (2016). A genome-wide association study of heat stress-associated SNPs in catfish. Anim. Genet. 48, 233–236. doi: 10.1111/age.12482
Jing, Y., Guo, B., Shikano, T., Liu, X., and Merilä, J. (2016). Quantitative trait locus analysis of body shape divergence in nine-spined sticklebacks based on high-density SNP-panel. Sci. Rep. 6:26632. doi: 10.1038/srep26632
Kolder, I. C. R. M., van der Plas-Duivesteijn, S. J., Tan, G., Wiegertjes, G. F., Forlenza, M., Guler, A. T., et al. (2016). A full-body transcriptome and proteome resource for the European common carp. BMC Genomics 17:701. doi: 10.1186/s12864-016-3038-y
Latres, E., Amini, A. R., Amini, A. A., Griffiths, J., Martin, F. J., Wei, Y., et al. (2005). Insulin-like growth factor-1 (IGF-1) inversely regulates atrophy-induced genes via the phosphatidylinositol 3-kinase/Akt/mammalian target of rapamycin (PI3K/Akt/mTOR) pathway. J. Biol. Chem. 280, 2737–2744. doi: 10.1074/jbc.M407517200
Lee, S. J., and Mcpherron, A. C. (2001). Regulation of myostatin activity and muscle growth. Proc. Natl. Acad. Sci. U.S.A. 98, 9306–9311. doi: 10.1073/pnas.151270098
Li, J., Chu, L., Sun, X., Liu, Y., and Cheng, C. H. (2015). IGFs mediate the action of LH on Oocyte maturation in zebrafish. Mol. Endocrinol. 29, 373–383. doi: 10.1210/me.2014-1218
Li, Y., Liu, S., Qin, Z., Waldbieser, G., Wang, R., Sun, L., et al. (2014). Construction of a high-density, high-resolution genetic map and its integration with BAC-based physical map in channel catfish. DNA Res. 22, 39–52. doi: 10.1093/dnares/dsu038
Lin, C. T., Lin, Y. T., and Kuo, T. F. (2007). Investigation of mRNA expression for secreted frizzled-related protein 2 (sFRP2) in chick embryos. J. Reprod. Dev. 53, 801–810. doi: 10.1262/jrd.18081
Liu, F., Sun, F., Li, J., Xia, J. H., Lin, G., Tu, R. J., et al. (2013). A microsatellite-based linkage map of salt tolerant tilapia (Oreochromis mossambicus x Oreochromis spp.) and mapping of sex-determining loci. BMC Genomics 14:58. doi: 10.1186/1471-2164-14-58
Liu, J. H., Zhang, Y., Chang, Y. M., Liang, L. Q., Lu, C. Y., Zhang, X. F., et al. (2009). Mapping QTLs related to head length, eye diameter and eye cross of common carp (Cyprinus carpio L.). Hereditas 31, 508–514. doi: 10.3724/SP.J.1005.2009.00508
Liu, X. D., Cai, M. Y., Wang, Z. Y., and You, Z. Y. (2008). The correlation and path analysis for growth-related traits of large yellow croaker Pseudosciaena crocea from min-yuedong tribe. Period. Ocean Univ. China 916–920.
Marchini, J., Cardon, L. R., Phillips, M. S., and Donnelly, P. (2004). The effects of human population structure on large genetic association studies. Nat. Genet. 36, 512–517. doi: 10.1038/ng1337
Menzel, S., Garner, C., Gut, I., Matsuda, F., Yamaguchi, M., Heath, S., et al. (2007). A QTL influencing F cell production maps to a gene encoding a zinc-finger protein on chromosome 2p15. Nat. Genet. 39, 1197–1199. doi: 10.1038/ng2108
Nelson, S. N., and Van Der Kraak, G. (2010). Characterization and regulation of the insulin-like growth factor (IGF) system in the zebrafish (Danio rerio) ovary. Gen. Comp. Endocrinol. 168, 111–120. doi: 10.1016/j.ygcen.2010.04.020
Peng, W., Xu, J., Zhang, Y., Feng, J., Dong, C., Jiang, L., et al. (2016). An ultra-high density linkage map and QTL mapping for sex and growth-related traits of common carp (Cyprinus carpio). Sci. Rep. 6:26693. doi: 10.1038/srep26693
Purcell, S., Neale, B., Todd-Brown, K., Thomas, L., Ferreira, M. A. R., Bender, D., et al. (2007). PLINK: a tool set for whole-genome association and population-based linkage analyses. Am. J. Hum. Genet. 81, 559–575. doi: 10.1086/519795
Rappleye, C. A., Tagawa, A., Lyczak, R., Bowerman, B., and Aroian, R. V. (2002). The anaphase-promoting complex and separin are required for embryonic anterior-posterior axis formation. Dev. Cell 2, 195–206. doi: 10.1016/S1534-5807(02)00114-4
Reis Neto, R. V., de Freitas, R. T. F., Serafini, M. A., Costa, A. C., Freato, T. A., Rosa, P. V., et al. (2012). Interrelationships between morphometric variables and rounded fish body yields evaluated by path analysis. Revista Brasileira de Zootecnia 41, 1576–1582. doi: 10.1590/S1516-35982012000700004
Reynaud, K., Chastant-Maillard, S., Batard, S., Thoumire, S., and Monget, P. (2010). IGF system and ovarian folliculogenesis in dog breeds of various sizes: is there a link? J. Endocrinol. 206, 85–92. doi: 10.1677/joe-09-0450
Richard-Parpaillon, L., Héligon, C., Chesnel, F., Boujard, D., and Philpott, A. (2002). The IGF pathway regulates head formation by inhibiting Wnt signaling in Xenopus. Dev. Biol. 244, 407–417. doi: 10.1006/dbio.2002.0605
Seebacher, F., and Walter, I. (2012). Differences in locomotor performance between individuals: importance of parvalbumin, calcium handling and metabolism. J. Exp. Biol. 215, 663–670. doi: 10.1242/jeb.066712
Sekiya, T., Oda, T. K., and Akiyama, T. (2004). Transcriptional regulation of the TGF-beta pseudoreceptor BAMBI by TGF-beta signaling. Biochem. Biophys. Res. Commun. 320, 680–684. doi: 10.1016/j.bbrc.2004.06.017
Shan, Y., Cuiyun, L. U., Zhang, X., Chao, L. I., Cheng, L., and Sun, X. (2014). Excavating the main effect of quantitative trait loci related to head length, head length and body length ratio in mirror carp(Cyprinus carpio). J. Fish. China 38, 625–631.
Simpson, M. A., Irving, M. D., Asilmaz, E., Gray, M. J., Dafou, D., Elmslie, F. V., et al. (2011). Mutations in NOTCH2 cause Hajdu-Cheney syndrome, a disorder of severe and progressive bone loss. Nat. Genet. 43, 303–305. doi: 10.1038/ng.779
Sugiyama, Y., Shelley, E. J., Wen, L., Stump, R. J. W., Shimono, A., Lovicu, F. J., et al. (2013). Sfrp1 and Sfrp2 are not involved in Wnt/β-catenin signal silencing during lens induction but are required for maintenance of Wnt/β-catenin signaling in lens epithelial cells. Dev. Biol. 384, 181–193. doi: 10.1016/j.ydbio.2013.10.008
Sutter, N. B., Bustamante, C. D., Chase, K., Gray, M. M., Zhao, K., Zhu, L., et al. (2007). A single IGF1 allele is a major determinant of small size in dogs. Science 316, 112–115. doi: 10.1126/science.1137045
Vallejo, R. L., Palti, Y., Liu, S., Evenhuis, J. P., Gao, G., Rexroad, C. E., et al. (2014). Detection of QTL in rainbow trout affecting survival when challenged with Flavobacterium psychrophilum. Mar. Biotechnol. 16, 349–360. doi: 10.1007/s10126-013-9553-9
Vecchione, A., Marchese, A., Henry, P., Rotin, D., and Morrione, A. (2003). The Grb10/Nedd4 complex regulates ligand-induced ubiquitination and stability of the insulin-like growth factor I receptor. Mol. Cell. Biol. 23, 3363–3372. doi: 10.1128/MCB.23.9.3363-3372.2003
Wan, G., and Chan, K. M. (2010). A study of somatolactin actions by ectopic expression in transgenic zebrafish larvae. J. Mol. Endocrinol. 45, 301–315. doi: 10.1677/JME-09-0173
Wang, D.-S., Jiao, B., Hu, C., Huang, X., Liu, Z., and Cheng, C. H. (2008). Discovery of a gonad-specific IGF subtype in teleost. Biochem. Biophys. Res. Commun. 367, 336–341. doi: 10.1016/j.bbrc.2007.12.136
Wang, M., Yan, J., Zhao, J., Song, W., Zhang, X., Xiao, Y., et al. (2012). Genome-wide association study (GWAS) of resistance to head smut in maize. Plant Sci. 196, 125–131. doi: 10.1016/j.plantsci.2012.08.004
Wang, X., Liu, S., Jiang, C., Geng, X., Zhou, T., Li, N., et al. (2017). Multiple across-strain and within-strain QTLs suggest highly complex genetic architecture for hypoxia tolerance in channel catfish. Mol. Genet. Genomics 292, 63–76. doi: 10.1007/s00438-016-1256-2
Wang, X. P., Zhang, X. F., Zhang, T. Q., Li, W. S., and Sun, X. W. (2010). Mapping and genetic effect analysis of quantitative trait loci related to head length, body height, and body thickness of common carp(Cyprinus carpio L.). J. Fish. China 34, 1645–1655.
Wilwert, J. L., Madhoun, N. M., and Coughlin, D. J. (2006). Parvalbumin correlates with relaxation rate in the swimming muscle of sheepshead and kingfish. J. Exp. Biol. 209(Pt 2), 227–237. doi: 10.1242/jeb.01987
Wunnenberg-Stapleton, K., Blitz, I. L., Hashimoto, C., and Cho, K. W. (1999). Involvement of the small GTPases XRhoA and XRnd1 in cell adhesion and head formation in early Xenopus development. Development 126, 5339–5351.
Xin, G., Jin, S., Liu, S., Bao, L., Zhang, J., Wang, R., et al. (2015). A genome-wide association study in catfish reveals the presence of functional hubs of related genes within QTLs for columnaris disease resistance. BMC Genomics 16:196. doi: 10.1186/s12864-015-1409-4
Xing, X., Yan, Z., Peng, X. U., Pan, Z., Wei, L., Sun, T., et al. (2011). Correlation analysis between microsatellite markers and body-shape traits in Cyprinus carpio L. J. Fish. Sci. China 18, 965–982.
Xu, J., Zhao, Z., Zhang, X., Zheng, X., and Li, J. (2014). Development and evaluation of the first high-throughput SNP array for common carp (Cyprinus carpio). BMC Genomics 15:307. doi: 10.1186/1471-2164-15-307
Xu, P., Zhang, X., Wang, X., Li, J., Liu, G., Kuang, Y., et al. (2014). Genome sequence and genetic diversity of the common carp, Cyprinus carpio. Nat. Genet. 46, 1212–1219. doi: 10.1038/ng.3098
Xu, Y. X., Zhu, Z. Y., Lo, L. C., Wang, C. M., Lin, G., Feng, F., et al. (2006). Characterization of two parvalbumin genes and their association with growth traits in Asian seabass (Lates calcarifer). Anim. Genet. 37, 266–268. doi: 10.1111/j.1365-2052.2006.01423.x
Yang, J., Manolio, T. A., Pasquale, L. R., Boerwinkle, E., Caporaso, N., Cunningham, J. M., et al. (2011). Genome partitioning of genetic variation for complex traits using common SNPs. Nat. Genet. 43, 519–525. doi: 10.1038/ng.823
Keywords: common carp, QTL, GWAS, head size, head length, linkage mapping
Citation: Chen L, Peng W, Kong S, Pu F, Chen B, Zhou Z, Feng J, Li X and Xu P (2018) Genetic Mapping of Head Size Related Traits in Common Carp (Cyprinus carpio). Front. Genet. 9:448. doi: 10.3389/fgene.2018.00448
Received: 28 August 2017; Accepted: 18 September 2018;
Published: 09 October 2018.
Edited by:
Noelle E. Cockett, Utah State University, United StatesReviewed by:
Christopher Bidwell, Purdue University, United StatesShaolin Wang, China Agricultural University, China
Copyright © 2018 Chen, Peng, Kong, Pu, Chen, Zhou, Feng, Li and Xu. This is an open-access article distributed under the terms of the Creative Commons Attribution License (CC BY). The use, distribution or reproduction in other forums is permitted, provided the original author(s) and the copyright owner(s) are credited and that the original publication in this journal is cited, in accordance with accepted academic practice. No use, distribution or reproduction is permitted which does not comply with these terms.
*Correspondence: Peng Xu, eHVwZW5nNzdAeG11LmVkdS5jbg==; eHVwZW5nNzdAZ21haWwuY29t