- 1Commonwealth Scientific and Industrial Research Organisation Agriculture and Food, Queensland Bioscience Precinct, St Lucia, QLD, Australia
- 2Zoology Department, Faculty of Science, Benha University, Benha, Egypt
- 3Commonwealth Scientific and Industrial Research Organisation Agriculture, Hobart, TAS, Australia
- 4Tassal Ltd., Hobart, TAS, Australia
The availability of a reference genome assembly for Atlantic salmon, Salmo salar, SNP genotyping platforms and low cost sequencing are enhancing the understanding of both life history and production-related traits in this important commercial species. We collected and analyzed transcriptomes from selected tissues of Atlantic salmon to inform future functional and comparative genomics studies. Messenger RNA (mRNA) was isolated from pituitary gland, brain, ovary, and liver before Illumina sequencing produced a total of 640 million 150-bp paired-end reads. Following read mapping, feature counting, and normalization, cluster analysis identified genes highly expressed in a tissue-specific manner. We identified a cluster of 508 tissue specific genes for pituitary gland, 3395 for brain, 2939 for ovary, and 539 for liver. Functional profiling identified gene clusters describing the unique functions of each tissue. Moreover, highly-expressed transcription factors (TFs) present in each tissue-specific gene cluster were identified. TFs belonging to homeobox and bhlh families were identified for pituitary gland, pou and zf-c2h2 families for brain, arid, and zf-c2h2 for ovary and rxr-like family for liver. The data and analysis presented are relevant to the emerging Functional Annotation of All Salmonid Genomes (FAASG) initiative that is seeking to develop a detailed understanding of both salmonid evolution and the genomic elements that drive gene expression and regulation.
Introduction
The Atlantic salmon (Salmo salar), a member of the family Salmonidae, is endemic to the northern Atlantic Ocean and cultivated worldwide including in Tasmania, Australia. The Australian salmon industry is founded on introduced North American wild stock originating from the River Philip in Nova Scotia (Jungalwalla, 1991). To enhance productivity using genetics, an applied breeding program has been in operation since 2004, and the Tasmanian salmon industry is now the highest valued commercial fishery-related industry in the state (Australian Bureau of Agricultural Resource Economics and Sciences, 2014). A number of production issues remain which are potentially tractable using genomic approaches, including unwanted early maturation, disease susceptibility, product quality traits, and imperfect DNA based diagnostics for sex determination.
The ability to elucidate the basis of complex salmonid traits has been dramatically improved by the availability of reference genomes for both rainbow trout (Oncorhynchus mykiss) (Berthelot et al., 2014) and Atlantic salmon (Lien et al., 2016). To date, their major impact has been through enabling the development and application of high density SNP genotyping platforms and GWAS. The availability of annotated protein coding gene models supports the interpretation of GWAS to identify putatively causal variants contributing to trait variation (Ayllon et al., 2015; Barson et al., 2015). However, these reference genomes currently lack annotation of the sequences that regulate gene expression, including proximal, and distal promotors and enhancer elements. Functional annotation requires significant time and cost, and the animal science community has self-assembled into consortia to tackle the task for livestock genomes (FAANG consortium, Andersson et al., 2015) and salmonids inside the “Functional Annotation of All Salmonid Genomes” (FAASG, Macqueen et al., 2017). The experimental component involves collection of multiple data types to support functional annotation, including histone modification marks, DNA methylation status and gene expression. RNA-Seq has revolutionized transcriptomic research, previously based on testing expression of candidate genes using RT-qPCR or even thousands of genes using cDNA microarrays. RNA-Seq provides an efficient and unbiased tool for large scale gene expression analyses in non-model organisms (for example Mohamed et al., 2016, 2018) as well as in salmonids (Salem et al., 2015; Kim et al., 2016; Robinson et al., 2017). Additional background information about available genomic tools for salmonid research, in the broader context of aquaculture genomics, is captured in a recent review (Abdelrahman et al., 2017).
The objective of this study was to begin contributions to FAASG by transcriptomic profiling selected tissues. We prioritized the brain-pituitary-gonad (BPG) axis, which plays a critical role in the development and regulation of the reproductive and immune systems in vertebrates including teleosts (Weltzien et al., 2004; Cowan et al., 2017). Fluctuations in this axis regulate hormone production, which exert local and systemic effects on the animal relating to sexual development, maturation, and other traits. The liver is an essential metabolic organ as it regulates most chemical levels in the blood, detoxifies various metabolites, synthesizes proteins, and produces energy. Hence, analyzing the complement of expressed genes within and across these four tissues will provide a basis for understanding key production and life history traits.
Materials and Methods
Ethics Statement
The animals used are from the SALTAS selective breeding program which has been described previously (Dominik et al., 2010; Eisbrenner et al., 2014; Kijas et al., 2017). The four animals used were sexually immature 3 year old female fish from the 2014 year class. The fish were reared and euthanized in compliance with the CSIRO Animal Ethics Committee, under approval number 2017–02.
Tissue Collection and Total RNA Isolation
Samples from mid-brain, pituitary gland, ovary and liver were collected and stored in RNA-later at −80°C until RNA isolation. Total RNA was isolated from each tissue using RNeasy Kit (QIAGEN), eluted in 40 μL RNase-Free Water and stored at −80°C. RNA quantity were assessed using a Nanodrop™ ND-1000 spectrophotometer (Thermo Scientific), and integrity checked by electrophoretic profiling with Agilent Bioanalyzer 2100 (Agilent, CA).
High-Throughput mRNA Sequencing (Illumina RNA-Seq)
Messenger RNA (mRNA) was isolated from 500 ng total RNA and a total of 16 RNA-Seq libraries (4 tissues × 4 biological replicates per tissue) were prepared using the Illumina TruSeq stranded library Preparation Kit. Libraries were sequenced on an Illumina NovaSeq 6,000 sequencing platform producing 640.3 million individual 150-bp paired-end reads.
Read Mapping, Differential Gene Expression, and Clustering Analyses
Illumina reads were checked for quality using FastQC software. High quality reads (Q>30) were mapped to Atlantic salmon genome ICSASG_v2 (Lien et al., 2016) using TopHat2 version 2.1.1 (Kim et al., 2009) with default parameters. Alignment BAM files were sorted by read name and converted into SAM files using SAMtools version 1.4 (Li et al., 2009). The Python package HTSeq version 0.7.2 (Anders et al., 2015) was applied to count unique reads mapped to exons, which is suitable for RNA-Seq analysis. Raw counts were analyzed using the edgeR package (Robinson et al., 2010) in the R statistical computing environment to infer differential gene expression among tissues. To identify differential expression between samples and tissues, we applied both fold-change and false discovery rate (FDR) thresholds. Specifically, we set FDR to ≤ 0.001 and log2 (fold change) ≥2 to declare significance in line with previous recommendations (Haas et al., 2013). It is important to note a log2 (fold change) >2 equates with a minimum 4-fold change. To compare multiple expression profiles, we used the value of log2 (FPKM+1) centered by subtracting the mean across profiles (Haas et al., 2013), i.e. the mean-centered log2 (FPKM+1) value in which the FPKM+1 circumvents the non-definable instances of log2(0) if FPKM = 0. Tissue-specific gene clusters were identified as those exhibiting high expression in one tissue versus all others.
Gene Ontology (GO) Enrichment Analysis of the Tissue-Specific Gene Clusters
To infer function for identified tissue-specific clusters, GO enrichment analyses were performed using the Database For Annotation, Visualization And Integrated Discovery (DAVID) (Huang et al., 2009). The ENTREZ_GENE_ID of the gene clusters was used as identifier and Salmo salar was selected as the background dataset for the enrichment analysis. DAVID uses Fisher's exact test to ascertain statistically significant enrichment of pathways amongst differentially expressed genes (DEGs) relative to the background transcriptome. For the purpose of the enrichment analysis, GO categories with a Benjamini-corrected P ≤ 0.05 were considered significant (Huang et al., 2009). The RNA-Seq reads used in this study have been submitted to the NCBI Gene Expression Omnibus (GEO) database under Accession no. GSE114247.
Identification of Highly Expressed Transcription Factors (TFs)
In order to determine transcription factors (TFs) in the tissue-specific gene clusters in Salmon, BLASTX analysis were performed (E-value < 10−3) against the Cod annotated transcription factors database downloaded from the AnimalTFDB database (http://bioinfo.life.hust.edu.cn/AnimalTFDB/#!/tf_summary?species=Gadus_morhua) for Gadus morhua. The retrieved transcription factors were ranked based on their expression in each of the tissue-specific clusters.
Results
Expression Within and Between Four Selected Tissues
Transcriptomic data was collected from 16 RNA-Seq libraries constructed in a 4 (tissues) × 4 (replicates) experimental design. Sequencing yielded a total of 640 M raw reads, which after mapping to the Atlantic salmon reference assembly ICSASG_v2 yielded an average of 28 M reads per library (Table S1). To begin assessment between libraries, multidimensional scaling (based on the top 500 genes that best differentiate the samples) was performed (Figure S1). This confirmed biological replicates within tissue clustered together, and libraries from different tissues took non-overlapping positions. We performed hierarchical clustering using Spearman correlations of pairwise normalized expression for all 16 samples to explore the relative amount of variation between replicates within a tissue compared to that between the four tissues. This revealed that brain displayed the highest variation between the four biological replicates (animals) while the lowest was observed for ovary tissue. Further, clustering grouped the brain and pituitary as the two tissues with the most similar patterns of gene expression (Figure S2). Together these assessments indicated gene expression variability was much lower between replicates of the same tissue as compared with variation between tissues, as expected for a high quality dataset.
Gene Clusters With Tissue Specific Expression
Genes exhibiting significant differential expression (DEG, ≥4-fold change with false discovery rate FDR ≤ 0.001) were identified and used to search for gene clusters exhibiting tissue specific expression. Hierarchical clustering using only significant DEGs revealed distinctive expression profiles for each tissue (Figure 1). Brain appeared to have the highest occurrence of tissue specific expression (3395 genes) followed by ovary (2939), liver (539), and the pituitary gland (508) (Figure 2). Apart from 17542 genes that were shared among the four tissues, the highest number of shared genes were 8819 between pituitary gland and brain followed by 5547 between brain and ovary, and interestingly 1839 among pituitary gland, brain and ovary (Figure 2). This can be expected given the important role of the brain-pituitary-gonad (BPG) axis in regulating sexual development in vertebrates including fish.
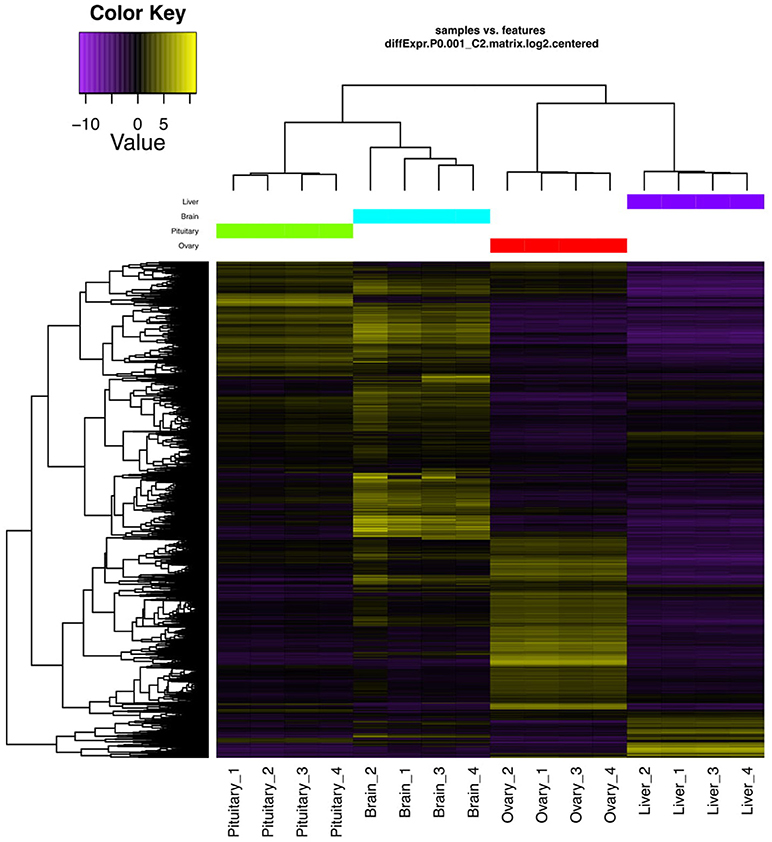
Figure 1. DEGs hierarchical clustering. Heat map showing genes (rows) with differential expression (fold ≥ 4, FDR ≤ 0.001) among the four replicates of the pituitary gland, brain, ovary, and liver samples. Expression values are log2-transformed and median-centered by gene.
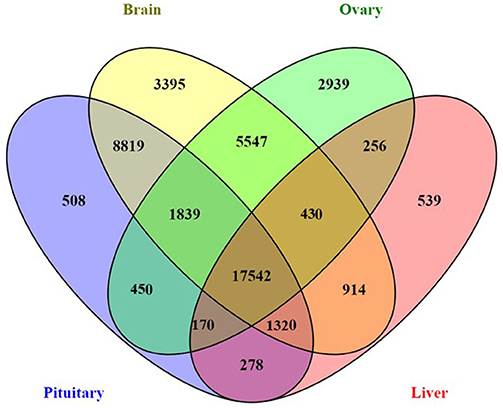
Figure 2. Four-way Venn diagram showing the number of shared and unique expressed genes across four salmon tissues (pituitary gland, brain, ovary, and liver).
The relative expression of the identified tissue specific clusters is compared across tissues in Figure 3. We sought to explore if tissue specific gene clusters were enriched for gene ontologies (GO) relating to molecular function (GO-MF), cellular component (GO-CC), or biological process (GO-BP). Pituitary gland-specific genes contained significant enrichment for all three gene ontology classes (GO-BP, GO-CC GO-MF terms at corrected P ≤ 0.05, Table 1). Despite being comprised of only three genes (growth hormone pre-peptide, prolactin, somatotropin-2), the most highly over-represented term (149 fold) was regulation of immunoglobulin secretion that includes genes coding for growth hormones, prolactin, and somatotropin. The GO-CC extracellular region was identified via pituitary gland hormones such as somatolactins, thyroid stimulating hormone, pro-opiomelanocortin, and gonadotropin which is consistent with the expected gene expression profile of the pituitary (Table 1, Table S3). The list of highly expressed genes in the pituitary gland included genes with function related to hormone activity such as somatolactin beta, pro-opiomelanocortin A1 and B (Figure 4; Table S2). The list of highly expressed transcription factors (TFs) identified in the pituitary gland (Table 2) included lhx3, six1b, other homeodomain proteins, ascl1a, bhlha15, zf-c2h2, and zbtb. Brain-specific genes were enriched for the GO-CC extracellular region term due to expression of neuropeptides including proenkephalin, myostatins, cholecystokinins, and thyrotropin-releasing hormone (Table 1, Table S4). Further, GO-MF term calcium ion binding was enriched based on expressed neuronal calcium-sensor/binding proteins such as visinin-like proteins, calretinin-like proteins and calmodulins (Table 1; Table S4). The list of highly expressed genes in the brain (Figure 4; Table S2) included Vesicular glutamate transporter 2.1 (gene ID = gene48716:106587477) and several genes coding for neuropeptides such as Glucagon family neuropeptides-like (gene ID = gene39762:106578701). The list of highly expressed TFs identified in the brain included zic2a, zic5, pou3f2a, pou3f3b, esrrb (Table 2).
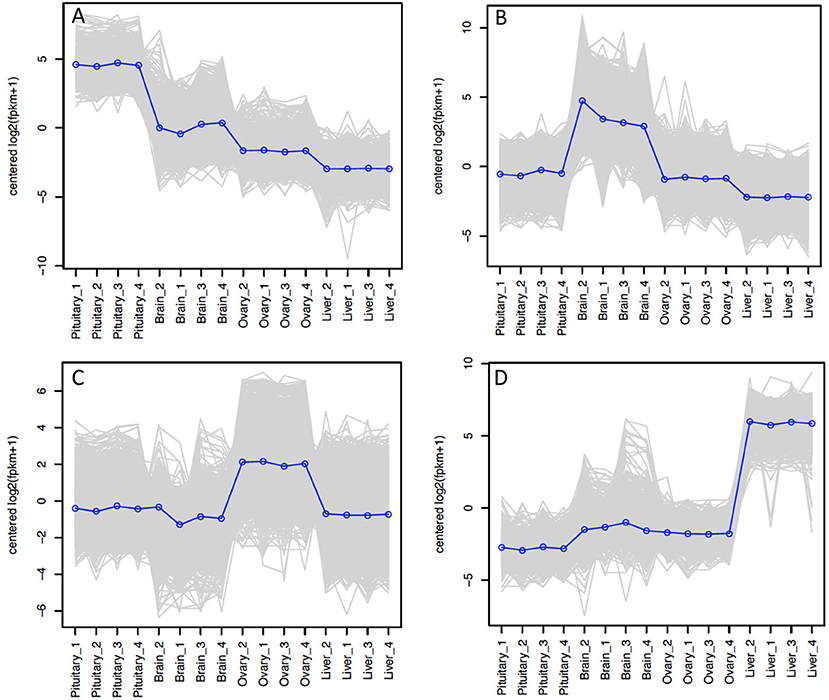
Figure 3. Tissue-specific clusters in Atlantic Salmon from Tasmania. Cluster of 508 genes was identified for pituitary gland (A), 3395 for brain (B), 2939 for ovary (C), and 539 for liver (D). The y-axis in each graph represents the mean-centered log2 (FPKM+1) value. Expression of single genes is plotted in gray, while the mean expression of the genes in each cluster is plotted in blue.
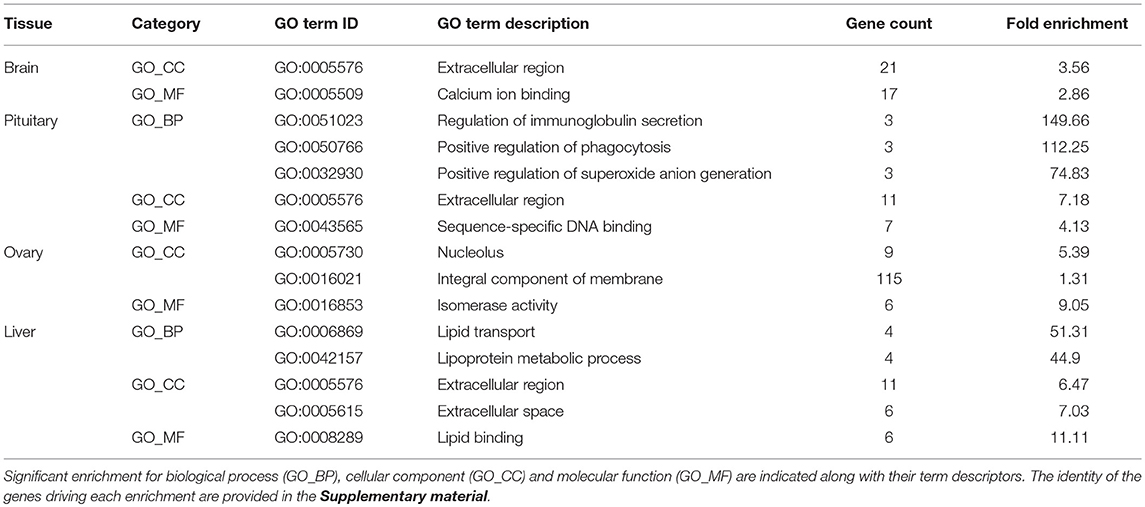
Table 1. Gene Ontology (GO) categories enriched (corrected P ≤ 0.05) among tissue-specific gene clusters.
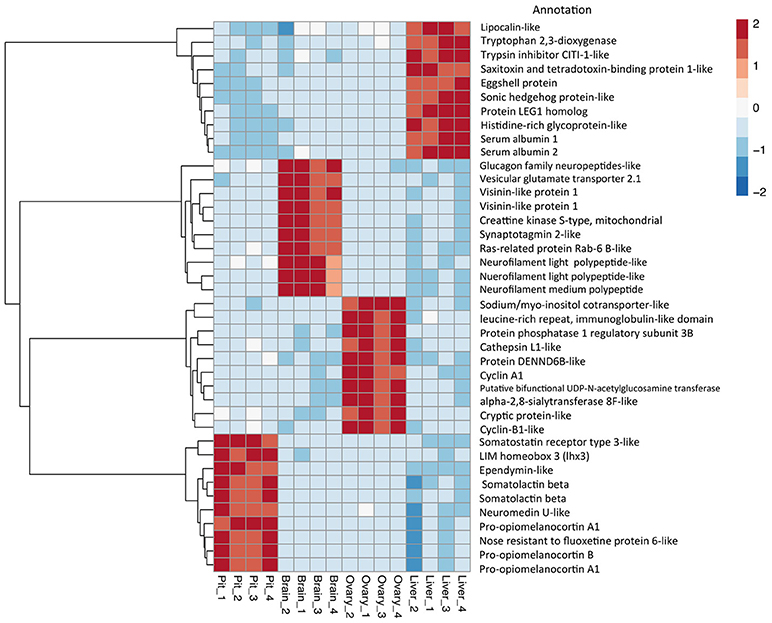
Figure 4. Top 10 differential expressed gene expression (DEGs). Heat map and dendrogram of the 10 most highly expressed genes in each tissue-specific cluster. The red-blue spectrum represents the scaled expression values.
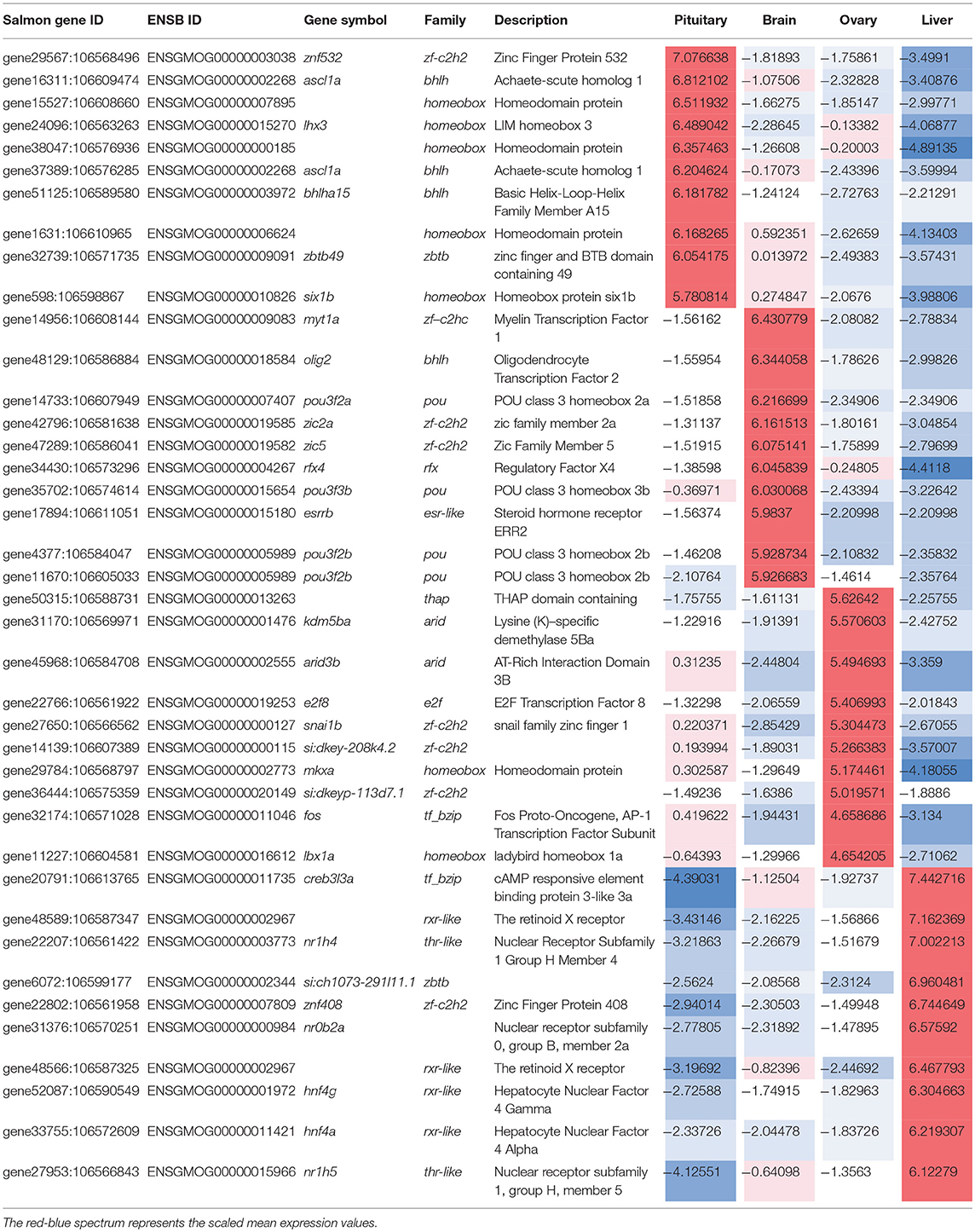
Table 2. Transcription factors highly expressed (top 10 ranked) in each tissue-specific gene clusters along with their expression.
Ovary-specific genes were enriched for two GO-CC terms nucleolus and integral component of membrane (Table S5) and one GO-MF term isomerase activity (Table 1). The drivers of enrichment included hormone receptors such as follicle stimulating hormone receptor, growth hormone receptor, trans-membrane proteins members of solute carrier family and 3-oxo-5-alpha-steroid 4-dehydrogenase. The list of highly expressed genes in the ovary is shown in Figure 4 and Table S2. The list of highly expressed TFs identified in the ovary (Table 2) included e2f8, fos, kdm5ba, arid3b, two Homeodomain proteins (lbx1a and mkxa) and two zinc finger proteins (snai1b and si:dkey-208k4.2).
The final tissue investigated was the liver, which had enriched GO terms in all three categories that included lipid transport, lipoprotein metabolic process, extracellular region, extracellular space, and lipid binding (Table 1; Table S6). The lipid transport term was the most highly over-represented (51-fold) based on genes coding for lipid transport-proteins (apolipoproteins). Other notable genes expressed primarily in the liver included coagulation factor, insulin-like growth factor-binding protein, peptide hormones, and genes with immune-related functions. The list of highly expressed genes in the liver (Figure 4; Table S2) included serum albumins (gene ID = gene1886:100136575, gene38403:100136922), eggshell protein (gene ID = gene22108:100136930), and saxitoxin and tetrodotoxin-binding protein 1-like (gene ID = gene18707:106611802). The list of highly expressed TFs identified in the liver (Table 2) included creb3l3a, nuclear receptors (nr1h4, nr0b2a, nr1h5), and hepatocyte nuclear factors (hnf4g, hnf4a).
Discussion
Identifying the genes expressed in cells of a given tissue represents important baseline information essential for understanding tissue function and physiology more broadly. This study cataloged the collection of expressed genes in four salmon tissues, and documented significant changes that characterized tissues from each other. We identified the most abundant transcripts and the collection of genes and transcription factors (TFs) exhibiting tissue specific expression, and our findings were in broad agreement with expectation about the specific role of each organ. It is worthwhile noting that tissue specificity was considered only within the context of the tissues tested, and that examination of a more diverse collection of tissues may alter these findings. Nonetheless, the profile of each transcriptome captured GO enrichment terms consistent with expectation, and provided confidence that the dataset is of both high quality and useful for subsequent analysis. For example the enrichment of the GO terms regulation of immunoglobulin secretion and extracellular region derived from the presence of genes coding for pituitary hormones. Similarly in the ovary the enrichment of the GO term integral component of membrane derived from the presence of many hormone receptors such as follicle stimulating hormone receptor and growth hormone receptor which aligns with functions of the ovary. The identified signals with high expression reflect the physiological function of each tissue. For example, the high expression of the pituitary hormone somatolactin beta and pro-opiomelanocortin (pomc) was observed in the pituitary gland. Similarly the pomc gene is expressed in both the anterior and intermediate lobes of the pituitary gland. The same is true for other tissues as expression of genes encoding neuropeptides in the brain and genes encoding liver proteins such as albumin and eggshell proteins in the liver.
The dataset adds to the increasing volume of RNA-Seq information being made available by the research community. The majority of existing Atlantic salmon RNA-Seq datasets have been generated to address specific biological questions, including host response to disease challenge (Dettleff et al., 2017; Valenzuela-Muñoz et al., 2017; Rozas-Serri et al., 2018), developmental progression (Wang et al., 2014) or nutritional requirements (Jin et al., 2018). The consequence is a paucity of transcriptomic profiling of animals not subject to an experimental regime designed to elicit specific responses or that characterize a biological extreme. The survey sequencing performed here can therefore be considered baseline data, making it applicable to general studies seeking to understand normal state expression levels across tissues. Importantly, when co-analyzed with the other datatypes identifying gene regulatory elements inside FAASG, we anticipate utility for at least three experimental applications. Firstly, for interpretation of GWAS to prioritize candidate genes on the basis of their tissue specific expression in relation to the biology of the trait under investigation. Secondly, it will assist assigning putative function to salmonid specific genes without annotation using the “guilt by association” principal. Specifically, the function of uncharacterized genes can be deduced from their tissue specific co-expression with genes of known function. Finally, we anticipate the data to contribute to characterization of transcriptomic complexity that includes expression of transcript isoforms and temporal variation in expression that can only be addressed by extensive tissue sampling across multiple life history time points.
Author Contributions
AM, HK, JK, and BE conceived the study and contributed to the experimental design. HK and BE performed animal management and AM and JK contributed to sample acquisition. AM generated and analyzed the RNA-Seq data. TR contributed to the analysis. AM and JK drafted the manuscript which all authors contributed to.
Funding
Funding for this work was provided by the CSIRO Office of the Cheif Executive (OCE) program, with kind in support provided by Tassal Operations, Tasmania.
Conflict of Interest Statement
BE was employed by company Tassal.
The remaining authors declare that the research was conducted in the absence of any commercial or financial relationships that could be construed as a potential conflict of interest.
Supplementary Material
The Supplementary Material for this article can be found online at: https://www.frontiersin.org/articles/10.3389/fgene.2018.00369/full#supplementary-material
References
Abdelrahman, H., ElHady, M., Alcivar-Warren, A., Allen, S., Al-Tobasei, R., and Bao, L. (2017). Aquaculture genomics, genetics and breeding in the United States: current status, challenges, and priorities for future research. BMC Genomics 18:191. doi: 10.1186/s12864-017-3557-1
Anders, S., Pyl, P. T., and Huber, W. (2015). HTseq - a Python framework to work with high-throughput sequencing data. Bioinformatics 31, 166–169. doi: 10.1093/bioinformatics/btu638
Andersson, L., Archibald, A. L., Bottema, C. D., Brauning, R., Burgess, S. C., Burt, D. W., et al. (2015). Coordinated international action to accelerate genome-to-phenome with FAANG, the Functional Annotation of Animal Genomes project. Genome Biol. 16:57. doi: 10.1186/s13059-015-0622-4
Australian Bureau of Agricultural Resource Economics and Sciences (2014). Australian Fisheries Statistics. Canberra: ABARES.
Ayllon, F., Kjærner-Semb, E., Furmanek, T., Wennevik, V., Solberg, M. F., Dahle, G., et al. (2015). The vgll3 locus controls age at maturity in wild and domesticated Atlantic salmon (Salmo salar L.) males. PLoS Genet. 11:e1005628. doi: 10.1371/journal.pgen.1005628
Barson, N. J., Aykanat, T., Hindar, K., Baranski, M., Bolstad, G. H., Fiske, P., et al. (2015). Sex dependent dominance at a single locus maintains variation in age at maturity in salmon. Nature 528, 405–408. doi: 10.1038/nature16062
Berthelot, C., Brunet, F., Chalopin, D., Juanchich, A., Bernard, M., Noël, B., et al. (2014). The rainbow trout genome provides novel insights into evolution after whole-genome duplication in vertebrates. Nat. Commun. 5:3657. doi: 10.1038/ncomms4657
Cowan, M., Azpeleta, C., and López-Olmeda, J. F. (2017). Rhythms in the endocrine system of fish: a review. J. Comp. Physiol. B Biochem. Syst. Environ. Physiol. 187, 1057–1089. doi: 10.1007/s00360-017-1094-5
Dettleff, P., Moen, T., Santi, N., and Martinez, V. (2017). Transcriptomic analysis of spleen infected with infectious salmon anemia virus reveals distinct pattern of viral replication on resistant and susceptible Atlantic salmon (Salmo salar). Fish Shellfish Immunol. 61, 187–193. doi: 10.1016/j.fsi.2017.01.005
Dominik, S., Henshall, J., Kube, P., King, H., Lien, S., Kent, M., et al. (2010). Evaluation of an Atlantic salmon SNP chip as a genomic tool for the application in a Tasmanian Atlantic salmon (Salmo salar) breeding population. Aquaculture 308, S56–S61. doi: 10.1016/j.aquaculture.2010.05.038
Eisbrenner, W. D., Botwright, N., Cook, M., Davidson, E. A., Dominik, S., Elliott, N. G., et al. (2014). Evidence for multiple sex-determining loci in Tasmanian Atlantic salmon (Salmo salar). Heredity 113, 86–92. doi: 10.1038/hdy.2013.55
Haas, B. J., Papanicolaou, A., Yassour, M., Grabherr, M., Blood, P. D., Bowden, J., et al. (2013). De novotranscript sequence reconstruction from RNA-seq using the Trinity platform for reference generation and analysis. Nat. Protoc. 8, 1494–1512. doi: 10.1038/nprot.2013.084
Huang, D. W., Sherman, B. T., and Lempicki, R. A. (2009). Systematic and integrative analysis of large gene lists using DAVID bioinformatics resources. Nat. Protoc. 4, 44–57. doi: 10.1038/nprot.2008.211
Jin, Y., Olsen, R. E., Østensen, M. A., Gillard, G. B., Korsvoll, S. A., Santi, N., et al. (2018). Transcriptional development of phospholipid and lipoprotein metabolism in different intestinal regions of Atlantic salmon (Salmo salar) fry. BMC Genomics 19:253. doi: 10.1186/s12864-018-4651-8
Jungalwalla, P. J. (1991). The development of an integrated saltwater salmonid farming industry in Tasmania, Australia. Can. Tech. Rep. Fish. Aquat. Sci. 1831, 65–73.
Kijas, J., Elliot, N., Kube, P., Evans, B., Botwright, N., and King, H. (2017). Diversity and linkage disequilibrium in farmed Tasmanian Atlantic salmon. Anim. Genet. 48, 237–241. doi: 10.1111/age.12513
Kim, D., Pertea, G., Trapnell, C., Pimentel, H., Kelley, R., Salzberg, S. L., et al. (2009).TopHat2: accurate alignment of transcriptomes in the presence of insertions, deletions and gene fusions. Genome Biol. 14:R36. doi: 10.1186/gb-2013-14-4-r36
Kim, J. H., Leong, J. S., Koop Ben, F., and Devlin, R. H. (2016). Multi-tissue transcriptome profiles for coho salmon (Oncorhynchus kisutch), a species undergoing rediploidization following whole-genome duplication. Mar. Genomics 25, 33–37. doi: 10.1016/j.margen.2015.11.008
Li, H., Handsaker, B., Wysoker, A., Fennell, T., Ruan, J., Homer, N., et al. (2009). The sequence alignment/map format and SAMtools. Bioinformatics 25, 2078–2079. doi: 10.1093/bioinformatics/btp352
Lien, S., Koop, B. F., Sandve, S. R., Miller, J. R., Kent, M. P., Nome, T., et al. (2016). The Atlantic salmon genome provides insights into rediploidization. Nature 533:200. doi: 10.1038/nature17164
Macqueen, D. J., Primmer, C. R., Houston, R. D., Nowak, B. F., Bernatchez, L., et al. (2017). Functional annotation of all salmonid genomes (FAASG): an international initiative supporting future salmonid research, conservation and aquaculture. BMC Genomics 18:484. doi: 10.1186/s12864-017-3862-8
Mohamed, A. R., Cumbo, V., Harii, S., Shinzato, C., Chan, C. X., Ragan, M. A., et al. (2018). Deciphering the nature of the coral-Chromera association. ISME J. 12, 776–790. doi: 10.1038/s41396-017-0005-9
Mohamed, A. R., Cumbo, V. R, Harii, S., Shinzato, C., Chan, C. X., Ragan, M. A., et al. (2016). The transcriptomic response of the coral Acropora digitifera to a competent Symbiodinium strain: the symbiosome as an arrested early phagosome. Mol Ecol. 25, 3127–3141. doi: 10.1111/mec.13659
Robinson, M. D., McCarthy, D. J., and Smyth, G. K. (2010). edgeR: a Bioconductor package for differential expression analysis of digital gene expression data. Bioinformatics 26, 139–140. doi: 10.1093/bioinformatics/btp616
Robinson, N. A., Timmerhaus, G., Baranski, M., Andersen, Ø., Takle, H., and Krasnov, A. (2017). Training the salmon's genes: influence of aerobic exercise, swimming performance and selection on gene expression in Atlantic salmon. BMC Genomics. 18:971. doi: 10.1186/s12864-017-4361-7
Rozas-Serri, M., Peña, A., and Maldonado, L. (2018). Transcriptomic profiles of post-smolt Atlantic salmon challenged with Piscirickettsia salmonis reveal a strategy to evade the adaptive immune response and modify cell-autonomous immunity. Dev. Comp. Immunol. 81, 348–362. doi: 10.1016/j.dci.2017.12.023
Salem, M., Paneru, B., Al-Tobasei, R., Abdouni, F., Thorgaard, G. H., Rexroad, C. E., et al. (2015). Transcriptome assembly, gene annotation and tissue gene expression atlas of the rainbow trout. PLoS ONE 10:e0121778. doi: 10.1371/journal.pone.0121778
Valenzuela-Muñoz, V., Novoa, B., Figueras, A., and Gallardo-Escárate, C. (2017). Modulation of Atlantic salmon miRNome response to sea louse infestation. Dev. Comp. Immunol. 76, 380–391. doi: 10.1016/j.dci.2017.07.009
Wang, S., Furmanek, T., Kryvi, H., Krossøy, C., Totland, G. K., Grotmol, S., et al. (2014). Transcriptome sequencing of Atlantic salmon (Salmo salar L.) notochord prior to development of the vertebrae provides clues to regulation of positional fate, chordoblast lineage and mineralisation. BMC Genomics. 15:141. doi: 10.1186/1471-2164-15-141
Keywords: Atlantic salmon, RNA-Seq, transcriptome, gene expression, transcription factors, next-gen sequencing
Citation: Mohamed AR, King H, Evans B, Reverter A and Kijas JW (2018) Multi-Tissue Transcriptome Profiling of North American Derived Atlantic Salmon. Front. Genet. 9:369. doi: 10.3389/fgene.2018.00369
Received: 14 May 2018; Accepted: 22 August 2018;
Published: 13 September 2018.
Edited by:
Nguyen Hong Nguyen, University of the Sunshine Coast, AustraliaReviewed by:
Daniel D. Heath, University of Windsor, CanadaMadeleine Carruthers, University of Glasgow, United Kingdom
Copyright © 2018 Mohamed, King, Evans, Reverter and Kijas. This is an open-access article distributed under the terms of the Creative Commons Attribution License (CC BY). The use, distribution or reproduction in other forums is permitted, provided the original author(s) and the copyright owner(s) are credited and that the original publication in this journal is cited, in accordance with accepted academic practice. No use, distribution or reproduction is permitted which does not comply with these terms.
*Correspondence: James W. Kijas, amFtZXMua2lqYXNAY3Npcm8uYXU=