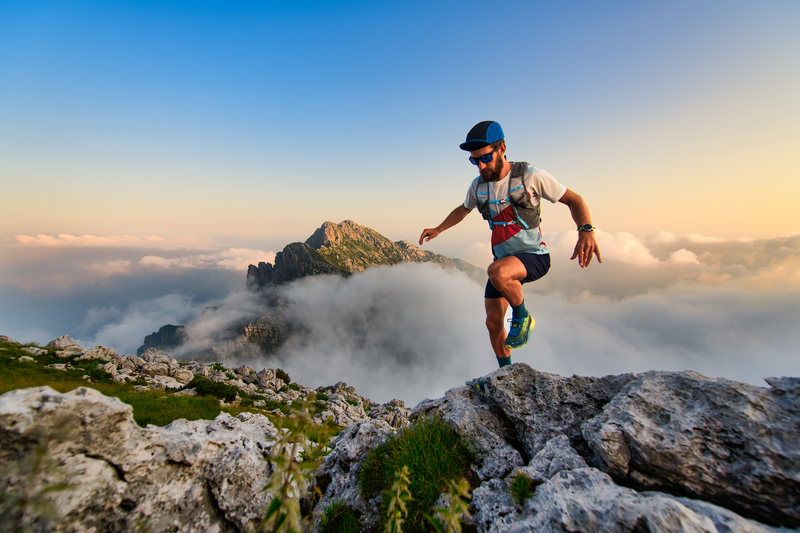
95% of researchers rate our articles as excellent or good
Learn more about the work of our research integrity team to safeguard the quality of each article we publish.
Find out more
REVIEW article
Front. Genet. , 14 June 2017
Sec. Computational Genomics
Volume 8 - 2017 | https://doi.org/10.3389/fgene.2017.00083
This article is part of the Research Topic New Trends on Genome and Transcriptome Characterizations View all 13 articles
Advances in DNA sequencing technologies have allowed the characterization of somatic mutations in a large number of cancer genomes at an unprecedented level of detail, revealing the extreme genetic heterogeneity of cancer at two different levels: inter-tumor, with different patients of the same cancer type presenting different collections of somatic mutations, and intra-tumor, with different clones coexisting within the same tumor. Both inter-tumor and intra-tumor heterogeneity have crucial implications for clinical practices. Here, we review computational methods that use somatic alterations measured through next-generation DNA sequencing technologies for characterizing tumor heterogeneity and its association with clinical variables. We first review computational methods for studying inter-tumor heterogeneity, focusing on methods that attempt to summarize cancer heterogeneity by discovering pathways that are commonly mutated across different patients of the same cancer type. We then review computational methods for characterizing intra-tumor heterogeneity using information from bulk sequencing data or from single cell sequencing data. Finally, we present some of the recent computational methodologies that have been proposed to identify and assess the association between inter- or intra-tumor heterogeneity with clinical variables.
Somatic mutations, alterations of the DNA which accumulate during the lifetime of an individual, are the most common cause of cancer. High-throughput sequencing technologies now allow to identify and catalog the entire complement of somatic mutations in a tumor (Mardis, 2008; Meyerson et al., 2010) and many studies, including the ones from TCGA1 and ICGC 2, have used these technologies to measure mutations in the whole exome or whole genome of hundreds or thousands of tumors (e.g., see The Cancer Genome Atlas Research Network, 2017a,b for recent studies). These studies provide a detailed characterization of the landscape of somatic mutations in cancer, describing the hundreds-thousands of somatic mutations appearing in each tumor. Such somatic mutations include single nucleotide variants (SNVs) as well as copy number aberrations (CNAs), larger scale events which modify (by amplifications or deletions) the number of copies of a DNA region. Only a handful of all somatic mutations, called driver mutations, confer selecting advantage to cancer cells, while most somatic mutations are passenger mutations not contributing to the disease (Garraway and Lander, 2013; Vogelstein et al., 2013).
One of the most striking features of cancer mutational landscape is its inter-tumor heterogeneity (Figure 1): no two cancer genomes bear the same collection of somatic mutations, with many pairs of tumors having no mutation in common (Stratton et al., 2009), and a limited number of mutations appear in a large fraction of tumors, with most genes being mutated (by SNVs or CNAs) in <5% of all patients with a given cancer type (Ciriello et al., 2013; Kandoth et al., 2013; Tamborero et al., 2013). Inter-tumor heterogeneity hinders efforts to identify driver genes, bearing driver mutations, by detecting frequently mutated genes, i.e., genes mutated in a significantly high fraction of patients (Dees et al., 2012; Lawrence et al., 2013). In addition, frequency-based methods may result in several false positives (D'Antonio and Ciccarelli, 2013) since genomic features not related to the disease, including (normal) gene expression levels and replication time (Lawrence et al., 2013), can nonetheless lead to a high mutation frequency for a gene and must therefore be taken into account to identify significantly mutated genes (Lawrence et al., 2014).
Figure 1. Inter-tumor heterogeneity and its causes. Driver mutations (in red) target genes which are members of different cancer pathways, sets of interacting genes which perform specific functions and are altered in cancer. Passenger mutations (in black) not related to the disease comprise the majority of mutations in a tumor. Different mutated genes in cancer pathways and different passenger mutations are observed in tumors of the same type, with two cancer genomes often having no mutation in common.
One of the causes of inter-tumor heterogeneity is the fact that driver mutations target signaling and molecular pathways (Vogelstein and Kinzler, 2004; Vogelstein et al., 2013), groups of interacting proteins and genes performing specific functions in a cell. Mutations in genes belonging to cancer pathways lead to the acquisition of the biological capabilities (e.g., resisting cell death and inducing angiogenesis) or hallmarks (Hanahan and Weinberg, 2000, 2011) featured by cancer cells. A cancer pathway may be altered by mutations in any of its genes, leading to a wide spectrum of mutation frequencies for genes in the same cancer pathway, with one or few genes mutated with relatively high frequency and many genes mutated at much smaller frequency, which may not be sufficient for detection by frequency-based methods. In addition, each cancer genome is exposed to different mutational processes characterized by different combinations of mutations or signatures (Alexandrov et al., 2013b; Petljak and Alexandrov, 2016), with different cancer types presenting different mixtures of such signatures (Nik-Zainal et al., 2012a, 2016; Alexandrov et al., 2013a, 2015, 2016). Studying and characterizing mutations at the level of pathways is therefore crucial to deal with heterogeneity for the identification of driver mutations and to identify common themes extending the “rulebook” of cancer (McGranahan and Swanton, 2015), with important implications in prognosis and therapy (Swanton, 2016).
In addition to uncover such inter-tumor heterogeneity, cancer genome sequencing has also uncovered intra-tumor heterogeneity (Figure 2): a tumor is often composed by different populations of cancer cells (Anderson et al., 2011; Gerlinger et al., 2012, 2014; Schuh et al., 2012; Newburger et al., 2013; Bolli et al., 2014; Brastianos et al., 2015; Gundem et al., 2015; Ling et al., 2015; Sottoriva et al., 2015), called clones, arising from the evolutionary process (Nowell, 1976) which starting from a normal cell leads, through somatic mutations, to a collection of related but different cancer cells (Greaves and Maley, 2012; Swanton, 2012). While only providing measurements at the level of the entire cell population, deep (e.g., >100-fold) bulk sequencing offers the opportunity to study intra-tumor heterogeneity: the variant allele frequency (VAF), or fraction of reads supporting a variant among all the reads mapped to the same genomic location, of a heterozygous variant in a diploid region is proportional to the fraction of cells with the variant among all cells in the sample. VAFs from a tumor can then be used to identify the various clones present in a tumor. In addition, since the VAFs in a cell are constrained by evolutionary relationships among the clones in a tumor, they can be used to infer the evolutionary trajectory followed by the observed tumor (Ding et al., 2012; Nik-Zainal et al., 2012b; Yates and Campbell, 2012; Burrell et al., 2013). Understanding the clonal composition of a tumor is crucial for prognosis and therapy (Greaves and Maley, 2012; Swanton, 2012), since different clones may present drug resistant mutations (Greaves and Maley, 2012; Swanton, 2012) and the reliable characterization of the evolutionary history of a tumor is needed to predict the development of the disease (Yachida et al., 2010; Lipinski et al., 2016).
Figure 2. Intra-tumor heterogeneity and its causes. Cancer evolves from a normal cell that accumulates mutations (in red, yellow, and blue), leading to different clones, populations of cells of different genotypes, coexisting in the same tumor. Bulk sequencing measures mutations from a sample of the resulting cell mixture, that also comprises normal cells. The fraction of reads supporting a mutation (VAF) is proportional to the number of cells with the mutation in the sample.
A more direct approach to study intra-tumor heterogeneity is single-cell sequencing (Hou et al., 2012; Xu et al., 2012; Wang et al., 2014; Navin, 2015a,b). Single-cell sequencing allows the direct observation of the cooccurrence of mutations within cells from different clones. However, single-cell data is currently noisy, with high false-positive and false-negative rates for mutation calls, and the number of cells that can be assessed is still limited compared to the billions of cells in a tumor.
In this review we describe bioinformatic and computational approaches to characterize cancer heterogeneity from next-generation sequencing data. We consider methods to deal with three aspects of cancer heterogeneity, after alterations such as SVNs and/or CNAs have been identified in a tumor or in multiple tumors (Raphael et al., 2014). First, we consider methods that tackle inter-tumor heterogeneity by characterizing tumor mutations at the pathway level. Second, we describe methods to characterize intra-tumor heterogeneity by using mutations from bulk sequencing or single-cell sequencing. Third, we describe some methods to relate cancer heterogeneity with clinical variables. The computational characterization of cancer heterogeneity is a topic which has spurred a lot of work in recent years and we only cover some of the tools that have been recently proposed. In particular, we only focus on methods assuming that somatic variants have already been called using one of the many methods currently available (e.g., Lawrence et al., 2013), and we refer the reader to other reviews discussing methods for variant calling in cancer (e.g., Raphael et al., 2014). The methods discussed in this review are mostly complementary, describing different characteristics of inter- or intra-tumor heterogeneity, which we believe constitute useful, multi-faceted information for cancer researchers and practitioners.
Several methods have been designed to characterize inter-tumor heterogeneity by identifying pathways and processes altered in a significant number of patients. These approaches can be categorized into 3 classes (Figure 3): methods based on predefined pathways; methods that extract pathways from a large interaction network of genes or proteins; de novo methods that do not use prior information of interactions among genes. Below we review some of the representative methods in each class. In general, the input to each method can be a list of genes mutated in the patients cohort or a score (e.g., frequency of mutation, a score reflecting the significance of the fraction of mutated genes in the cohort; Lawrence et al., 2013, etc.) for each gene in the cohort. As described below, while some of the methods require in input a list of putative driver mutations, identified for example by frequency-based approaches (e.g., Dees et al., 2012; Lawrence et al., 2013), other methods try to leverage the information regarding interactions among genes/proteins to identify novel driver genes which cannot be identified by frequency-based approaches. We highlight here the main methods that produce, in output, pathways, or sets genes summarizing inter-patient heterogeneity, while we do not consider methods which provide instead a ranking of genes (e.g., Vanunu et al., 2010; Shrestha et al., 2014), or which focus on patients stratification (e.g., Hofree et al., 2013), or which combine mutations with other data types (e.g., Vaske et al., 2010; McPherson et al., 2012; Paull et al., 2013). See Creixell et al. (2015) for a more comprehensive review of network approaches to analyze cancer genomes.
Figure 3. Computational analyses to characterize inter-tumor heterogeneity. Starting from somatic mutations measured in many patients, different types of analyses are possible: annotation and enrichment analysis for known pathways; network analyses to discover significantly mutated subnetworks of a large interaction networks; the de novo identification of pathways, based for example on the identification of mutual exclusivity patterns.
A common way to identify significantly mutated pathways is to use predefined pathways, obtained from databases such as KEGG (Kanehisa and Goto, 2000; Kanehisa et al., 2015, 2017) and MSigDB (Subramanian et al., 2005; Liberzon et al., 2015), an then asses whether the set of genes in a predefined pathway is significantly enriched for mutated genes or scores compared to the entire set of genes. The simplest approach is to assess whether a list of mutated genes is enriched for genes in predefined set of genes, for example by using an hypergeometric test on the overlap of the intersection among the list of genes and the gene set. There are several tools [e.g., DAVID (Huang et al., 2009), g:Profiler (Reimand et al., 2016)], some of which originally designed for gene expression data, that can be used for gene lists obtained from mutation data. A common feature of these approaches is that they require the definition of the list of mutated genes, commonly based on thresholds based on frequency or statistical significance of single genes. An alternative is to use Gene Set Enrichment Analysis (GSEA) (Subramanian et al., 2005), a general methodology to assess the association of a ranking of genes with a given gene set. The rank of the genes can be obtained from the various tools mentioned above; for example, Lin et al. (2007) used the Cancer Mutation Prevalence (CaMP) scores (Sjöblom et al., 2006), but other scores can be used. A different approach is taken by PathScan (Wendl et al., 2011), that computes a p-value for the enrichment of mutations in a given set separately for each patient, and then combines the p-values across all patients. Similarly, the method from Boca et al. (2010) defines, given a gene set, a score for each patient and then combines such scores across all patients.
The methods above are useful to characterize inter-tumor heterogeneity using known sets of genes and pathways, but have some major limitations. First, they require the a priori definition of the list of mutated genes, and therefore, while they are useful in organizing a list of mutated genes into pathways, they cannot be used to reliably identify novel driver genes. Second, some of the genes sets from datasets are extremely large (>300 genes). With such large gene sets it may not be possible to identify a small subset associated with the disease. Third, these methods ignore the interactions among genes in a network, considering all genes in a pathway equally, without including the topology of network in their analysis. Fourth, they consider each set of genes as a separate entity, while it is well-known that there is cross-talk among pathways, which interact into larger networks (McCormick, 1999).
A different approach to characterize cancer inter-tumor heterogeneity at the pathway level while not restricting to known sets of genes is to use a genome-scale protein-protein interaction network. Several computational methods that combine mutation data and networks to infer gene sets have been designed. A first class of methods (e.g., NetBox; Cerami et al., 2010) looks for significant network modules among a list of genes which is provided as input. Such approaches require to define a score threshold to include genes in the analysis, limiting the possibility of the method to identify novel driver genes. A different approach is to identify significant subnetworks (comprising connected genes) that are significantly mutated in the patients cohort. While allowing to expand from predefined sets of interacting genes to general interacting subnetworks, the identification of significantly mutated subnetwork presents computational and statistical challenges. There is a huge number of subnetworks which need to be screened and which need to be considered into a multiple hypothesis testing framework to identify the significantly mutated ones, therefore naïve approaches (e.g., the enumeration and testing of all subnetworks) cannot be employed and more sophisticated techniques are required.
HotNet (Vandin et al., 2011) and HotNet2 (Leiserson et al., 2015a) address the challenges above by using a diffusion process on a graph to combine gene scores with the network topology while capturing the local structure of the network. A novel statistical test is used by HotNet and HotNet2, allowing the identification of a set of subnetworks while bounding the false discovery rate (FDR). The combination of gene scores and network topology solves the issue of choosing a threshold for the inclusion of genes in the analysis and allows the identification of subnetworks whose significance is due to the mutation scores of the genes and the local topology of a subnetwork. In the analysis of >3,000 samples from 12 cancer types from TCGA (Leiserson et al., 2015a), HotNet2 identified 16 significantly mutated subnetworks that comprise well-known cancer pathways as well as subnetworks with less established contributions to cancer, including the cohesin complex.
MEMo (Ciriello et al., 2012) is an algorithm that uses a different approach to identify subnetworks: provided in input with a relative short of list of (frequently mutated) genes from which subnetworks (called modules) are to be found, it identifies groups of genes sharing several neighbors in the interaction network and showing significant mutual exclusivity of mutations in the patients cohort. MEMo therefore identifies modules summarize inter-patient heterogeneity through mutual exclusivity, but it is unlikely to include in its modules genes that are not significantly mutated on their own. MEMCover (Kim et al., 2015) is a different algorithm that combines network information and mutual exclusivity of mutations to identify modules of mutated genes. MEMCover employs a greedy strategy to identify high scoring subnetworks, where a subnetwork score is a combination of the number of patients with at a least a mutated subnetwork member and of the mutual exclusivity of mutations in the subnetwork genes. Babur et al. (2015) present a greedy approach to find gene sets sharing a common down-stream target in the network and showing high mutual exclusivity. They assess mutual exclusivity by comparing each gene in the set with the union of the other genes.
Network-based approaches are useful to characterize inter-tumor heterogeneity without restricting to know sets of genes and pathways, but they suffer from the limitations of currently available network. Such networks have only partial coverage of genes and interactions: some genes have no interactions in current networks, and interactions of different genes may have been assayed to different extents, with genes known to be associated to diseases that are likely to have been more thoroughly assayed for interactions (ascertainment bias). In addition, current networks include interactions that occur among proteins in different tissues or at different phases of the cell cycle. Improved methods are needed to integrate additional information (e.g., co-location of proteins in cells) with the interaction information provided by currently available networks.
Previous approaches are based on knowledge of the interactions among genes/proteins. A different class of methods characterize inter-tumor heterogeneity by finding groups of genes or pathways without restricting to predefined sets or to groups of interacting genes in a large network. The de novo extraction of pathways poses enormous computational and statistical challenges, since every subset of genes is a candidate which may need to be considered. However, some methods use combinatorial properties (Yeang et al., 2008) of important mutations in cancer to restrict the set of potential candidates. One such property is mutual exclusivity, with sets of genes displaying at most 1 mutation in many patients. Mutual exclusivity of mutations has been observed in various cancer types (Kandoth et al., 2013) and may be due to the relatively low number of driver mutations in each tumor and to the fact that driver mutations target different pathways (Hanahan and Weinberg, 2011; Garraway and Lander, 2013; Vogelstein et al., 2013).
Several methods have been recently designed to identify gene sets with high mutual exclusivity. Since most genes are mutated with low frequency in a cohort of patients, it is easy to find a set of unrelated genes with high mutual exclusivity. For this reason, one needs to assess the statistical significance of the gene set, assessing whether the observed mutual exclusivity is likely to be due to chance alone. RME (Miller et al., 2011) identifies mutually exclusive sets using a score derived from information theory, and starts from pairs of genes to build larger sets. It includes only frequently mutated genes (>10%), limiting its applicability to characterize inter-tumor heterogeneity. Dendrix (Vandin et al., 2012b) defines a gene set score that combines the number of patients with at least a mutation in the set and the mutual exclusivity of mutations in the set, and uses a Markov Chain Monte Carlo (MCMC) approach for identifying mutually exclusive gene sets altered in a large fraction of the patients. Multi-Dendrix (Leiserson et al., 2013) employs the same score as Dendrix and extends it to multiple sets, and uses an integer linear program (ILP) based algorithm to simultaneously find multiple sets of mutually exclusive genes. CoMET (Leiserson et al., 2015b) uses a generalization of Fisher exact test to higher dimensional contingency tables to define a score that better characterizes mutually exclusive gene sets altered in relatively low fraction of the samples, and uses an efficient MCMC approach to identify such sets. WExT (Leiserson et al., 2015b) generalizes the test from CoMET to incorporate individual gene weights (probabilities) for each mutation in each sample, and provides an efficient way to assess the statistical significance of the sets using a saddle-point approximation. Similarly, WeSME (Kim Y.A. et al., 2016) introduces a test which incorporates the mutation rates of patients and genes and uses a fast permutation approach to assess the statistical significance of the sets. TiMEx (Constantinescu et al., 2015) assumes a generative model for mutations and defines a test to assess the null hypothesis that mutual exclusivity of a gene set is due to the interplay between waiting times to alterations and the time at which the tumor is sequenced. The test is used to assess pairs of genes, and larger sets are built from significant pairs and then assessed using the same test. As mentioned above, MEMo and the method from Babur et al. (2015) employ mutual exclusivity to find gene sets, but use an interaction network to limit the candidate gene sets. The method by Raphael and Vandin (2015) and PathTIMEx (Cristea et al., 2016) introduce an additional dimension to the characterization of inter-tumor heterogeneity, by reconstructing the order in which mutually exclusive gene sets are mutated. Kim J.W. et al. (2016) recently developed REVEALER, a method to identify mutually exclusive genes sets associated with functional phenotypes (see Section 4).
While the approaches above allow the de novo discovery of cancer gene sets, there are challenges that remain to be solved. For example, larger sample sizes than currently available may be needed to discover low frequency cancer pathways by using mutual exclusivity (Vandin et al., 2012c, 2016). The methods above are in general computationally intensive, mainly due to the large search space that must be explored, and more effective exploration strategies may be needed for larger datasets.
In recent years, several methods have been proposed to characterize intra-tumor heterogeneity. Such methods can be classified into three classes (Figure 4). First, methods that use mutation data from bulk sequencing to reconstruct the clonal composition of a tumor, thus identifying the different clones, populations of cells, present in a tumor sample and quantifying the fraction that each clone contributes to the tumor. Second, methods that use mutation data from bulk sequencing to reconstruct the evolutionary relationships among different clones and mutations in the tumor. Third, more recent methods that use mutation data from single cell sequencing to infer the evolution of a tumor at the single cell level. Due to space constraints, below we describe some of the methods in the three classes; we point the reader to the recent reviews by Schwartz and Schäffer (2017) and by Kuipers et al. (2017) for more details on approaches to infer tumor evolution.
Figure 4. Computational analyses to characterize intra-tumor heterogeneity. Starting from measurements (e.g.,VAFs) obtained from bulk sequencing of one or more tumor samples, one can infer the clonal composition of the sample and also the evolutionary relationships among clones. Single-cell sequencing can be use to infer the evolutionary relationships among the individual cells for which mutations have been assayed.
Bulk sequencing data provides information regarding the fraction of cells containing a mutation, and, therefore, regarding the fraction of cells defining the clone with the given mutation. In fact, for a heterozygous mutation in a copy neutral region the expected number of reads supporting the mutation (VAF) equals half of the clone frequency in the sample, since the mutation appears in only one of the two copies of the DNA. However, there are many confounders that make the identification of the clones not straightforward. First, the relation above holds only in expectation or for infinite coverage, while with finite coverage the actual VAF can deviate substantially from the corresponding clone frequency. Second, there are experimental biases in sequencing technologies that can change the relation between VAF and clonal frequency. Third, CNAs are quite common in cancer and nullify the relation above, making the inference of clones much more complex. Andor et al. (2016) have recently shown that the number of clones in a tumor is associated with mortality risk, which increases when between 2 and 4 clones are present in a tumor, while it decreases when >4 clones are present. The accurate characterization of the clonal composition of a tumor is therefore extremely important for diagnosis and therapy.
Several methods have been developed to identify the different clones, or cell populations, in a tumor starting from mutation data obtained from bulk sequencing. PyClone (Roth et al., 2014) identifies clones and their abundances by considering VAFs and allele-specific copy number data. It uses a beta-binomial model for VAFs and identifies clusters of mutations and their frequencies in a tumor sample with Bayesian nonparametric clustering which simultaneously infers clusters and the number of clusters. SciClone (Miller et al., 2014) considers VAFs in copy number neutral, loss of heterozygosity (LOH) free regions of the genome, and uses a variational Bayesian mixture model to infer clones and their frequency in the sample. Zare et al. (2014) present an algorithm to infer groups of mutations and their frequency in a tumor using mutation data from multiple sections of a tumor at a given time point. Their method is based a generative binomial model to incorporate information from the multiple sections and employs an expectation-maximization (EM) algorithm to estimate clones and their relative frequencies. BayClone (Sengupta et al., 2015) defines a class of nonparametric models, the categorical Indian buffet process, and uses bayesian inference to obtain posterior probabilities for the number clones, their genotypes, and their proportions, in a tumor sample.
With the coverage (30x–40x) used in many large scale cancer studies, there is a high variance in the number of reads covering a given position in the genome, weakening the relation between VAF and clonal frequency. In contrast, each copy number aberration perturbs many reads, and can provide a more reliable signal for clonal inference for tumors in which clones present different copy number profiles. THetA (Oesper et al., 2013) uses CNAs profiles from whole genome sequencing to characterize clones and their frequencies in a tumor mixture. It defines and optimizes an explicit probabilistic model for the generation of the observed sequencing data from a mixture of normal cells and different clones, and uses a BIC criteria to choose among the many models that may explain the data while balancing the likelihood of the data and the model complexity. THetA2 (Oesper et al., 2014) extends THetA in various directions, including the possibility to consider whole exome sequencing data and the use of B-allele frequencies (which indicates the relative quantity of the one allele compared to the other) to distinguish among several clonal population models consistent with the data. A different approach is taken by TITAN (Ha et al., 2014), which employs a generative factorial hidden Markov model framework to simultaneously infer CNA and LOH segments from read depths and digital allele ratios at heterozygous variant loci in the genome from whole genome sequencing data. CloneHD (Fischer et al., 2014) provides a statistical framework using read depth, B-allele frequencies, and VAFs to infer the clonal population structure of a tumor, allowing the simultaneous analysis of multiple samples from different regions of the same tumor or from longitudinal sequencing of the same tumor.
While methods to infer clones, their mutations, and their abundance, provide important and clinically relevant insights into intra-tumor heterogeneity, they do not explicitly provide information about the evolutionary relations among mutations and clones in a tumor. In addition to expanding our understanding of how a tumor arises, such information can provide extremely important information for clinical intervention. For example, the order in which mutations arise can influence the prognosis of a patient (Ortmann et al., 2015). Moreover, the characterization of the evolutionary paths followed by tumors is crucial to be able to predict the development of the disease for future patients (Yachida et al., 2010; Lipinski et al., 2016).
The computational reconstruction of the evolutionary relations among clones in a tumor from bulk sequencing data is a challenging task, due to several reasons. First, we do not directly observe clones in a tumor, but bulk sequencing provide aggregate information, in the form of VAFs, from a mixture of clones. Second, a natural model to describe tumor evolution is provided by phylogenetic or evolutionary trees, but there are in general several evolutionary trees consistent with the data from a single tumor sample. In most cases this may be mitigated by sequencing several sections of the same tumor, but reconciling the information from the different sections is a complex problem. Third, VAFs in regions affected by CNAs and LOH can be significantly different from VAFs of other mutations in the same clone, complicating the reliable identification of clones and their relations.
Many methods have been designed to reconstruct the evolutionary history of a tumor from bulk sequencing of one or more sections of the tumor and address the challenges above. TrAp (Strino et al., 2013) is a method designed to infer clones, their abundance, and clones' evolutionary paths using VAFs for SNVs from a single tumor sample. It first groups together mutations with similar frequencies, and then uses an iterative procedure to build evolutionary paths for such groups, starting from simple (height 1) trees. PhyloSub (Jiao et al., 2014) considers VAFs from deep sequencing experiments to infer the evolutionary relationship of clones, and uses a Dirichlet process prior over phylogenetic trees to group SNVs into clones. It employs Bayesian inference, based on MCMC sampling, to infer a distribution over possible evolutionary trees. PhyloWGS (Deshwar et al., 2015) builds on PhyloSub and allows the reconstruction of tumor evolution from SNVs and CNAs obtained from whole genome sequencing data. CITUP (Malikic et al., 2015) proposes a combinatorial model for the problem of inferring clonal evolution from SNVs obtained from multiple tumor samples, and designs an exact algorithm based on a quadratic integer programming to solve the problem, which may require high computational resources when the tumor contains a large number of clones. LICHeE (Popic et al., 2015) is another method to reconstruct clones, abundances, and their evolutionary relationships starting from SNVs measured in multiple samples of a tumor. LICHeE first groups SNVs and identifies clusters of SNVs based on VAFs, and then uses a network to represent VAFs constraints imposed by the evolutionary process. It then identifies an evolutionary model by looking for the spanning tree that best supports the cluster VAF data. BitPhylogeny (Yuan et al., 2015) provides a probabilistic framework that allows the joint inference of the number and composition of clones in a tumor, as well as the most probable tree representing their evolutionary relationship. SPRUCE (El-Kebir et al., 2016) infers evolutionary trees jointly from SNVs and CNAs from multiple tumor samples, with CNAs that are modeled as multi-state alterations, in which alterations can only mutate to a given state at most once in the tree. SPRUCE starts from clusters of SNVs and copy number mixing proportions, and derives a compatibility graph describing the compatibility of state trees for pairs of clusters. The evolutionary trees compatible with the input data are derived by enumerating all spanning trees with appropriate constraints in a labeled multi-graph constructed starting from the compatibility graph. The application of SPRUCE on real data show that many evolutionary trees are compatible with data from multiple samples, cautioning on drawing strong conclusions on any single such tree (Hu and Curtis, 2016). Canopy (Jiang et al., 2016) is a related method to infer evolutionary trees using both CNAs and SNVs from one or more samples, but it starts from raw copy number ratios estimated from CNA segmentation programs. It uses a statistical model and a MCMC algorithm to sample from the space of evolutionary trees, providing a confidence assessment from the posterior distribution. Additional methods to infer clonal evolution are presented in Hajirasouliha et al. (2014), Donmez et al. (2016), Qiao et al. (2014), and El-Kebir et al. (2015).
While each method displays specific features addressing one or more of the challenges above, they are all based, in one form or the other, on the infinite-site assumption: the same site is not mutated twice during the evolutionary history of a tumor. Such assumption may be violated in tumors with high genomic instability, undermining the accuracy of the inferred evolutionary trees. However, without such assumption the inference problem becomes computationally intractable even assuming perfect knowledge of mutations in each clone.
While bulk sequencing provides some information to infer the evolutionary tree describing a tumor history, the best way to elucidate such history is from single-cell data, which provides direct measurements for some of the leaves of a tumor evolutionary tree. Single-cell sequencing technology has been improving in recent years and datasets with SNVs from >40 single-cells are now available (Hou et al., 2012; Xu et al., 2012; Wang et al., 2014). However, mutation calls from single-cell sequencing still suffer from high false positive and false negative rate and missing values, due to various technical reasons (e.g., allele dropout; Kuipers et al., 2017). In addition, while obtaining measurements from hundreds of single-cells is an incredible advance, such cells still represent an extremely small fraction of all cells in a tumor (>109 in advanced tumors). For these reasons, standard phylogenetic approaches cannot be used to infer evolutionary trees from single cell data.
Few methods have been designed to infer the evolutionary relationships among single cells. Youn and Simon (2011) develop a method to infer a mutation tree, in which each node corresponds to a mutation and the tree relations describe the relative order among the appearance of mutations in a sample. The mutation tree is reconstructed by using a pairwise test to define the order for pairs of mutations. While the restriction to pairs of genes makes the method efficient, it discards the information among high order relations among mutations. SCITE (Jahn et al., 2016) identifies evolutionary trees from noisy and incomplete mutation data from single-cell sequencing. SCITE uses a statistical model and an MCMC approach to sample trees, error rates, and placement of single cells in the tree. While providing interesting insights, the method is fairly expensive computationally, allowing proper inference only for the limited number of cells available in current datasets. OncoNEM (Ross and Markowetz, 2016) is a related method that uses a nested effects model for the data and employs a heuristic local search algorithm to explore possible tree topologies. While appropriate for current dataset sizes, for much larger dataset such a search algorithm may be too expensive.
A major goal in characterizing inter- and intra-tumor heterogeneity is to understand its impact on prognosis and therapy. In most case, clinical data has been used after the computational characterization of tumor heterogeneity, as a post-processing step testing whether heterogeneity-related features are associated to or predictive for some clinical variable, mostly survival time. For example: survival data or other clinical information are used to evaluate the results of patients stratification methods (Hofree et al., 2013); Andor et al. (2016) computationally assessed the clonal composition of >1,000 samples of various cancer types and then assessed the association between the number of clones in a sample with overall and progression-free survival; Chowdhury et al. (2014, 2015) designed and used a novel algorithm to reconstruct trees describing cancer evolution from single cell copy number data obtained by fluorescence in situ hybridization (FISH), and showed that improved prediction accuracy is obtained for classification tasks (e.g., distinguishing primary vs. metastases in the same patient) when features from the cancer evolutionary tree are considered.
The discovery of mutations or mutated groups associated with clinical data starting from genome-wide measurements poses several challenges, due to the peculiar characteristic of genomic data, including the relatively low frequency of individual mutations (Vandin et al., 2015). A standard analysis (Gross et al., 2014) is to first identify driver mutations or pathways and then assess the association of the mutated genes or group of genes with a clinical variable (e.g., survival time). While providing useful information on the clinical relevance of the driver genes and pathway identified by approaches above, such methods may not identify groups of genes with low mutation frequency whose mutations are collectively associated with survival. Few methods have been developed to directly leverage clinical information to identify gene sets associated with clinical data. Vandin et al. (2012a) use gene scores derived from the p-values for the association of individual gene mutations with survival as input to HotNet to identify subnetworks associated with survival, but do not provide a method to directly identify gene sets associated with survival. HyperModules (Reimand and Bader, 2013) looks for subnetworks of a large interaction network that are associated with survival using a local search algorithm that builds a subnetwork by starting from one seed vertex and then greedily adds neighbors (at distance at most 2) from the seed. Leung et al. (2014) used it to find subnetworks of a kinase-substrate interaction network with phosphorylation-associated mutations associated with survival. NoMAS (Hansen and Vandin, 2016) is an efficient method based on graph color-coding which identifies subnetworks with mutations associated with survival by looking for subnetworks maximizing the log-rank statistic of subnetworks (Figure 5). NoMAS identifies subnetworks with stronger association with survival compared to greedy procedures, and also reports valid permutational p-values. REVEALER (Kim J.W. et al., 2016) is a computational method to identify groups of mutually exclusive genes correlated with a functional phenotype, for example sensitivity to a drug treatment. REVEALER uses a gene set score derived from mutual information and employs a greedy strategy to find genes sets associated with the target functional phenotype.
Figure 5. Network of Mutations Associated with Survival (NoMAS). NoMAS combines mutations measured in many patients and the corresponding survival time with a large interaction network to identify subnetworks of genes with significant association to survival. NoMAS is based on an efficient graph color-coding algorithm, and uses a permutation test to correct for multiple hypothesis testing.
The methods above provide initial steps to discover gene sets driven by inter-tumor heterogeneity and associated with clinical features, but much more work is required to identify clinically relevant features from tumor heterogeneity.
This review described some of the challenges that arise in studying and characterizing cancer inter- and intra-tumor heterogeneity. We focused on some computational methods which characterize inter-tumor heterogeneity at the level of pathways, infer intra-tumor heterogeneity from bulk or single-cell sequencing, and identify pathways associated with clinical variables. These and other methods are increasingly used to characterize heterogeneity in large sequencing studies and for individual patients. Given its importance for therapeutic decisions, the fast and precise characterization of cancer heterogeneity is likely to remain a key step in precision medicine.
The methods we described have significantly advanced our understanding of cancer heterogeneity and its importance in patient prognosis and treatment, but there still challenges to be addressed. First, while recent studies have shown that intra-tumor heterogeneity has clinical implications (McGranahan and Swanton, 2015, 2017; Andor et al., 2016), it is still unclear which ones among its features are key determinants for therapeutic decisions. The development of more precise computational methods to infer intra-tumor clonal composition and evolution is a necessary step to properly assess the relevance of each aspect for therapy and inform effort for noninvasive monitoring of tumors (e.g., liquid biopsies; Diaz and Bardelli, 2014). Second, the extensive intra-tumor heterogeneity and the stochasticity of some of the processes shaping the evolution of a tumor may limit the ability to accurately predict the future behavior of an individual cancer. Studies (e.g., Jamal-Hanjani et al., 2014) that are collecting molecular and clinical measurements at different time points during treatment for a large number of patients will provide the data necessary to understand the extent of the diversity in the evolutionary paths explored by different tumors, but substantially different computational methods are needed to rigorously and effectively analyze such datasets. Third, current methods for inferring a tumor evolution from single-cell data are computationally intensive, and will not be able to analyze much larger datasets which may soon be available. Fourth, current methods for analyzing bulk sequencing and single-cell sequencing data are orthogonal, but the two technologies provide complementary information about the same tumor. ddClone (Salehi et al., 2017) is a recent method which combines data from the two technologies, but the development of additional methods may be crucial in fully exploiting the power of next-generation sequencing to characterize cancer heterogeneity. Fifth, methods for inter-patient heterogeneity focus mostly on coding variants, while noncoding variants are known to be recurrently mutated in cancer (Weinhold et al., 2014; Melton et al., 2015; Puente et al., 2015), with the mutation in the promoter region of the TERT gene in melanoma (Huang et al., 2013) and other cancer types (Fredriksson et al., 2014; Melton et al., 2015) being a prominent example. Finally, other data types, including RNA sequencing, methylation data, and chromatin modifications need to be considered to understand the genomic heterogeneity of cancer. While there are some methods that integrate some of these data types with mutation data (Vaske et al., 2010; McPherson et al., 2012; Paull et al., 2013), additional work is required to characterize cancer heterogeneity by the full integration of the various data types. All these challenges need to be addressed to reach true precision medicine, and computational methods will continue to play a key role in advancing our understanding of cancer heterogeneity.
The author confirms being the sole contributor of this work and approved it for publication.
This work is supported, in part, by MIUR of Italy under project AMANDA and by NSF grant IIS-1247581.
The author declares that the research was conducted in the absence of any commercial or financial relationships that could be construed as a potential conflict of interest.
Alexandrov, L. B., Jones, P. H., Wedge, D. C., Sale, J. E., Campbell, P. J., Nik-Zainal, S., et al. (2015). Clock-like mutational processes in human somatic cells. Nat. Genet. 47, 1402–1407. doi: 10.1038/ng.3441
Alexandrov, L. B., Ju, Y. S., Haase, K., Van Loo, P., Martincorena, I., Nik-Zainal, S., et al. (2016). Mutational signatures associated with tobacco smoking in human cancer. Science 354, 618–622. doi: 10.1126/science.aag0299
Alexandrov, L. B., Nik-Zainal, S., Wedge, D. C., Aparicio, S. A., Behjati, S., Biankin, A. V., et al. (2013a). Signatures of mutational processes in human cancer. Nature 500, 415–421. doi: 10.1038/nature12477
Alexandrov, L. B., Nik-Zainal, S., Wedge, D. C., Campbell, P. J., and Stratton, M. R. (2013b). Deciphering signatures of mutational processes operative in human cancer. Cell Rep. 3, 246–259. doi: 10.1016/j.celrep.2012.12.008
Anderson, K., Lutz, C., Van Delft, F. W., Bateman, C. M., Guo, Y., Colman, S. M., et al. (2011). Genetic variegation of clonal architecture and propagating cells in leukaemia. Nature 469, 356–361. doi: 10.1038/nature09650
Andor, N., Graham, T. A., Jansen, M., Xia, L. C., Aktipis, C. A., Petritsch, C., et al. (2016). Pan-cancer analysis of the extent and consequences of intratumor heterogeneity. Nat. Med. 22, 105–113. doi: 10.1038/nm.3984
Babur, Ö., Gönen, M., Aksoy, B. A., Schultz, N., Ciriello, G., Sander, C., et al. (2015). Systematic identification of cancer driving signaling pathways based on mutual exclusivity of genomic alterations. Genome Biol. 16, 45. doi: 10.1186/s13059-015-0612-6
Boca, S. M., Kinzler, K. W., Velculescu, V. E., Vogelstein, B., and Parmigiani, G. (2010). Patient-oriented gene set analysis for cancer mutation data. Genome Biol. 11:R112. doi: 10.1186/gb-2010-11-11-r112
Bolli, N., Avet-Loiseau, H., Wedge, D. C., Van Loo, P., Alexandrov, L. B., Martincorena, I., et al. (2014). Heterogeneity of genomic evolution and mutational profiles in multiple myeloma. Nat. Commun. 5:2997. doi: 10.1038/ncomms3997
Brastianos, P. K., Carter, S. L., Santagata, S., Cahill, D. P., Taylor-Weiner, A., Jones, R. T., et al. (2015). Genomic characterization of brain metastases reveals branched evolution and potential therapeutic targets. Cancer Discov. 5, 1164–1177. doi: 10.1158/2159-8290.CD-15-0369
Burrell, R. A., McGranahan, N., Bartek, J., and Swanton, C. (2013). The causes and consequences of genetic heterogeneity in cancer evolution. Nature 501, 338–345. doi: 10.1038/nature12625
Cerami, E., Demir, E., Schultz, N., Taylor, B. S., and Sander, C. (2010). Automated network analysis identifies core pathways in glioblastoma. PLoS ONE 5:e8918. doi: 10.1371/journal.pone.0008918
Chowdhury, S. A., Gertz, E. M., Wangsa, D., Heselmeyer-Haddad, K., Ried, T., Schäffer, A. A., et al. (2015). Inferring models of multiscale copy number evolution for single-tumor phylogenetics. Bioinformatics 31, i258–i267. doi: 10.1093/bioinformatics/btv233
Chowdhury, S. A., Shackney, S. E., Heselmeyer-Haddad, K., Ried, T., Schäffer, A. A., and Schwartz, R. (2014). Algorithms to model single gene, single chromosome, and whole genome copy number changes jointly in tumor phylogenetics. PLoS Comput. Biol. 10:e1003740. doi: 10.1371/journal.pcbi.1003740
Ciriello, G., Cerami, E., Sander, C., and Schultz, N. (2012). Mutual exclusivity analysis identifies oncogenic network modules. Genome Res. 22, 398–406. doi: 10.1101/gr.125567.111
Ciriello, G., Miller, M. L., Aksoy, B. A., Senbabaoglu, Y., Schultz, N., and Sander, C. (2013). Emerging landscape of oncogenic signatures across human cancers. Nat. Genet. 45, 1127–1133. doi: 10.1038/ng.2762
Constantinescu, S., Szczurek, E., Mohammadi, P., Rahnenführer, J., and Beerenwinkel, N. (2015). Timex: a waiting time model for mutually exclusive cancer alterations. Bioinformatics 32, 968–975. doi: 10.1093/bioinformatics/btv400
Creixell, P., Reimand, J., Haider, S., Wu, G., Shibata, T., Vazquez, M., et al. (2015). Pathway and network analysis of cancer genomes. Nat. Methods 12:615. doi: 10.1038/nmeth.3440
Cristea, S., Kuipers, J., and Beerenwinkel, N. (2016). pathtimex: joint inference of mutually exclusive cancer pathways and their progression dynamics. J. Comput. Biol. 24, 603–615. doi: 10.1089/cmb.2016.0171
D'Antonio, M., and Ciccarelli, F. D. (2013). Integrated analysis of recurrent properties of cancer genes to identify novel drivers. Genome Biol. 14:R52. doi: 10.1186/gb-2013-14-5-r52
Dees, N. D., Zhang, Q., Kandoth, C., Wendl, M. C., Schierding, W., Koboldt, D. C., et al. (2012). Music: identifying mutational significance in cancer genomes. Genome Res. 22, 1589–1598. doi: 10.1101/gr.134635.111
Deshwar, A. G., Vembu, S., Yung, C. K., Jang, G. H., Stein, L., and Morris, Q. (2015). Phylowgs: reconstructing subclonal composition and evolution from whole-genome sequencing of tumors. Genome Biol. 16, 35. doi: 10.1186/s13059-015-0602-8
Diaz, L. A., and Bardelli, A. (2014). Liquid biopsies: genotyping circulating tumor dna. J. Clin. Oncol. 32, 579–586. doi: 10.1200/JCO.2012.45.2011
Ding, L., Ley, T. J., Larson, D. E., Miller, C. A., Koboldt, D. C., Welch, J. S., et al. (2012). Clonal evolution in relapsed acute myeloid leukaemia revealed by whole-genome sequencing. Nature 481, 506–510. doi: 10.1038/nature10738
Donmez, N., Malikic, S., Wyatt, A. W., Gleave, M. E., Collins, C. C., and Sahinalp, S. C. (2016). “Clonality inference from single tumor samples using low coverage sequence data,” in International Conference on Research in Computational Molecular Biology (Santa Monica: Springer), 83–94.
El-Kebir, M., Oesper, L., Acheson-Field, H., and Raphael, B. J. (2015). Reconstruction of clonal trees and tumor composition from multi-sample sequencing data. Bioinformatics 31, i62–i70. doi: 10.1093/bioinformatics/btv261
El-Kebir, M., Satas, G., Oesper, L., and Raphael, B. J. (2016). Inferring the mutational history of a tumor using multi-state perfect phylogeny mixtures. Cell Syst. 3, 43–53. doi: 10.1016/j.cels.2016.07.004
Fischer, A., Vázquez-García, I., Illingworth, C. J., and Mustonen, V. (2014). High-definition reconstruction of clonal composition in cancer. Cell Rep. 7, 1740–1752. doi: 10.1016/j.celrep.2014.04.055
Fredriksson, N. J., Ny, L., Nilsson, J. A., and Larsson, E. (2014). Systematic analysis of noncoding somatic mutations and gene expression alterations across 14 tumor types. Nat. Genet. 46, 1258–1263. doi: 10.1038/ng.3141
Garraway, L. A., and Lander, E. S. (2013). Lessons from the cancer genome. Cell 153, 17–37. doi: 10.1016/j.cell.2013.03.002
Gerlinger, M., Horswell, S., Larkin, J., Rowan, A. J., Salm, M. P., Varela, I., et al. (2014). Genomic architecture and evolution of clear cell renal cell carcinomas defined by multiregion sequencing. Nat. Genet. 46, 225–233. doi: 10.1038/ng.2891
Gerlinger, M., Rowan, A. J., Horswell, S., Larkin, J., Endesfelder, D., Gronroos, E., et al. (2012). Intratumor heterogeneity and branched evolution revealed by multiregion sequencing. N. Engl. J. Med. 2012, 883–892. doi: 10.1056/NEJMoa1113205
Greaves, M., and Maley, C. C. (2012). Clonal evolution in cancer. Nature 481, 306–313. doi: 10.1038/nature10762
Gross, A. M., Orosco, R. K., Shen, J. P., Egloff, A. M., Carter, H., Hofree, M., et al. (2014). Multi-tiered genomic analysis of head and neck cancer ties tp53 mutation to 3p loss. Nat. Genet. 46, 939–943. doi: 10.1038/ng.3051
Gundem, G., Van Loo, P., Kremeyer, B., Alexandrov, L. B., Tubio, J. M., Papaemmanuil, E., et al. (2015). The evolutionary history of lethal metastatic prostate cancer. Nature 520, 353–357. doi: 10.1038/nature14347
Ha, G., Roth, A., Khattra, J., Ho, J., Yap, D., Prentice, L. M., et al. (2014). Titan: inference of copy number architectures in clonal cell populations from tumor whole-genome sequence data. Genome Res. 24, 1881–1893. doi: 10.1101/gr.180281.114
Hajirasouliha, I., Mahmoody, A., and Raphael, B. J. (2014). A combinatorial approach for analyzing intra-tumor heterogeneity from high-throughput sequencing data. Bioinformatics 30, i78–i86. doi: 10.1093/bioinformatics/btu284
Hanahan, D., and Weinberg, R. A. (2000). The hallmarks of cancer. Cell 100, 57–70. doi: 10.1016/S0092-8674(00)81683-9
Hanahan, D., and Weinberg, R. A. (2011). Hallmarks of cancer: the next generation. Cell 144, 646–674. doi: 10.1016/j.cell.2011.02.013
Hansen, T., and Vandin, F. (2016). “Finding mutated subnetworks associated with survival time in cancer,” in 20th Annual Conference on Research in Computational Molecular Biology. Santa Monica.
Hofree, M., Shen, J. P., Carter, H., Gross, A., and Ideker, T. (2013). Network-based stratification of tumor mutations. Nat. Methods 10, 1108–1115. doi: 10.1038/nmeth.2651
Hou, Y., Song, L., Zhu, P., Zhang, B., Tao, Y., Xu, X., et al. (2012). Single-cell exome sequencing and monoclonal evolution of a jak2-negative myeloproliferative neoplasm. Cell 148, 873–885. doi: 10.1016/j.cell.2012.02.028
Hu, Z., and Curtis, C. (2016). Inferring tumor phylogenies from multi-region sequencing. Cell Syst. 3, 12–14. doi: 10.1016/j.cels.2016.07.007
Huang, D. W., Sherman, B. T., and Lempicki, R. A. (2009). Systematic and integrative analysis of large gene lists using david bioinformatics resources. Nat. Protoc. 4, 44–57. doi: 10.1038/nprot.2008.211
Huang, F. W., Hodis, E., Xu, M. J., Kryukov, G. V., Chin, L., and Garraway, L. A. (2013). Highly recurrent tert promoter mutations in human melanoma. Science 339, 957–959. doi: 10.1126/science.1229259
Jahn, K., Kuipers, J., and Beerenwinkel, N. (2016). Tree inference for single-cell data. Genome Biol. 17:86. doi: 10.1186/s13059-016-0936-x
Jamal-Hanjani, M., Hackshaw, A., Ngai, Y., Shaw, J., Dive, C., Quezada, S., et al. (2014). Tracking genomic cancer evolution for precision medicine: the lung tracerx study. PLoS Biol. 12:e1001906. doi: 10.1371/journal.pbio.1001906
Jiang, Y., Qiu, Y., Minn, A. J., and Zhang, N. R. (2016). Assessing intratumor heterogeneity and tracking longitudinal and spatial clonal evolutionary history by next-generation sequencing. Proc. Natl. Acad. Sci. U.S.A. 113, E5528–E5537. doi: 10.1073/pnas.1522203113
Jiao, W., Vembu, S., Deshwar, A. G., Stein, L., and Morris, Q. (2014). Inferring clonal evolution of tumors from single nucleotide somatic mutations. BMC Bioinformatics 15:35. doi: 10.1186/1471-2105-15-35
Kandoth, C., McLellan, M. D., Vandin, F., Ye, K., Niu, B., Lu, C., et al. (2013). Mutational landscape and significance across 12 major cancer types. Nature 502, 333–339. doi: 10.1038/nature12634
Kanehisa, M., Furumichi, M., Tanabe, M., Sato, Y., and Morishima, K. (2017). Kegg: new perspectives on genomes, pathways, diseases and drugs. Nucleic Acids Res. 45, D353–D361. doi: 10.1093/nar/gkw1092
Kanehisa, M., and Goto, S. (2000). Kegg: kyoto encyclopedia of genes and genomes. Nucleic Acids Res. 28, 27–30. doi: 10.1093/nar/28.1.27
Kanehisa, M., Sato, Y., Kawashima, M., Furumichi, M., and Tanabe, M. (2015). Kegg as a reference resource for gene and protein annotation. Nucleic Acids Res. 44, D457–D462. doi: 10.1093/nar/gkv1070
Kim, J. W., Botvinnik, O. B., Abudayyeh, O., Birger, C., Rosenbluh, J., Shrestha, Y., et al. (2016). Characterizing genomic alterations in cancer by complementary functional associations. Nat. Biotechnol. 34, 539-546. doi: 10.1038/nbt.3527
Kim, Y.-A., Cho, D.-Y., Dao, P., and Przytycka, T. M. (2015). Memcover: integrated analysis of mutual exclusivity and functional network reveals dysregulated pathways across multiple cancer types. Bioinformatics 31, i284–i292. doi: 10.1093/bioinformatics/btv247
Kim, Y.-A., Madan, S., and Przytycka, T. M. (2016). Wesme: uncovering mutual exclusivity of cancer drivers and beyond. Bioinformatics 33, 814–821. doi: 10.1093/bioinformatics/btw242
Kuipers, J., Jahn, K., and Beerenwinkel, N. (2017). Advances in understanding tumour evolution through single-cell sequencing. Biochim. Biophys. Acta 1867, 127–138. doi: 10.1016/j.bbcan.2017.02.001
Lawrence, M. S., Stojanov, P., Mermel, C. H., Robinson, J. T., Garraway, L. A., Golub, T. R., et al. (2014). Discovery and saturation analysis of cancer genes across 21 tumour types. Nature 505, 495–501. doi: 10.1038/nature12912
Lawrence, M. S., Stojanov, P., Polak, P., Kryukov, G. V., Cibulskis, K., Sivachenko, A., et al. (2013). Mutational heterogeneity in cancer and the search for new cancer-associated genes. Nature 499, 214–218. doi: 10.1038/nature12213
Leiserson, M. D., Blokh, D., Sharan, R., and Raphael, B. J. (2013). Simultaneous identification of multiple driver pathways in cancer. PLoS Comput. Biol. 9:e1003054. doi: 10.1371/journal.pcbi.1003054
Leiserson, M. D., Vandin, F., Wu, H.-T., Dobson, J. R., Eldridge, J. V., Thomas, J. L., et al. (2015a). Pan-cancer network analysis identifies combinations of rare somatic mutations across pathways and protein complexes. Nat. Genet. 47, 106–114. doi: 10.1038/ng.3168
Leiserson, M. D., Wu, H.-T., Vandin, F., and Raphael, B. J. (2015b). Comet: a statistical approach to identify combinations of mutually exclusive alterations in cancer. Genome Biol. 16:160. doi: 10.1186/s13059-015-0700-7
Leung, A., Bader, G. D., and Reimand, J. (2014). Hypermodules: identifying clinically and phenotypically significant network modules with disease mutations for biomarker discovery. Bioinformatics 30, 2230–2232. doi: 10.1093/bioinformatics/btu172
Liberzon, A., Birger, C., Thorvaldsdóttir, H., Ghandi, M., Mesirov, J. P., and Tamayo, P. (2015). The molecular signatures database hallmark gene set collection. Cell Syst. 1, 417–425. doi: 10.1016/j.cels.2015.12.004
Lin, J., Gan, C. M., Zhang, X., Jones, S., Sjöblom, T., Wood, L. D., et al. (2007). A multidimensional analysis of genes mutated in breast and colorectal cancers. Genome Res. 17, 1304–1318. doi: 10.1101/gr.6431107
Ling, S., Hu, Z., Yang, Z., Yang, F., Li, Y., Lin, P., et al. (2015). Extremely high genetic diversity in a single tumor points to prevalence of non-darwinian cell evolution. Proc. Natl. Acad. Sci. U.S.A. 112, E6496–E6505. doi: 10.1073/pnas.1519556112
Lipinski, K. A., Barber, L. J., Davies, M. N., Ashenden, M., Sottoriva, A., and Gerlinger, M. (2016). Cancer evolution and the limits of predictability in precision cancer medicine. Trends Cancer 2, 49–63. doi: 10.1016/j.trecan.2015.11.003
Malikic, S., McPherson, A. W., Donmez, N., and Sahinalp, C. S. (2015). Clonality inference in multiple tumor samples using phylogeny. Bioinformatics 31, 1349–1356. doi: 10.1093/bioinformatics/btv003
Mardis, E. R. (2008). Next-generation dna sequencing methods. Annu. Rev. Genomics Hum. Genet. 9, 387–402. doi: 10.1146/annurev.genom.9.081307.164359
McCormick, F. (1999). Signalling networks that cause cancer. Trends Biochem. Sci. 24, M53–M56. doi: 10.1016/S0968-0004(99)01480-2
McGranahan, N., and Swanton, C. (2015). Biological and therapeutic impact of intratumor heterogeneity in cancer evolution. Cancer Cell 27, 15–26. doi: 10.1016/j.ccell.2014.12.001
McGranahan, N., and Swanton, C. (2017). Clonal heterogeneity and tumor evolution: past, present, and the future. Cell 168, 613–628. doi: 10.1016/j.cell.2017.01.018
McPherson, A., Wu, C., Wyatt, A. W., Shah, S., Collins, C., and Sahinalp, S. C. (2012). nFuse: discovery of complex genomic rearrangements in cancer using high-throughput sequencing. Genome Res. 22, 2250–2261. doi: 10.1101/gr.136572.111
Melton, C., Reuter, J. A., Spacek, D. V., and Snyder, M. (2015). Recurrent somatic mutations in regulatory regions of human cancer genomes. Nat. Genet. 47, 710–716. doi: 10.1038/ng.3332
Meyerson, M., Gabriel, S., and Getz, G. (2010). Advances in understanding cancer genomes through second-generation sequencing. Nat. Rev. Genet. 11, 685–696. doi: 10.1038/nrg2841
Miller, C. A., Settle, S. H., Sulman, E. P., Aldape, K. D., and Milosavljevic, A. (2011). Discovering functional modules by identifying recurrent and mutually exclusive mutational patterns in tumors. BMC Med. Genomics 4:34. doi: 10.1186/1755-8794-4-34
Miller, C. A., White, B. S., Dees, N. D., Griffith, M., Welch, J. S., Griffith, O. L., et al. (2014). Sciclone: inferring clonal architecture and tracking the spatial and temporal patterns of tumor evolution. PLoS Comput. Biol. 10:e1003665. doi: 10.1371/journal.pcbi.1003665
Navin, N. E. (2015a). Delineating cancer evolution with single-cell sequencing. Sci. Transl. Med. 7, 296fs29. doi: 10.1126/scitranslmed.aac8319
Navin, N. E. (2015b). The first five years of single-cell cancer genomics and beyond. Genome Res. 25, 1499–1507. doi: 10.1101/gr.191098.115
Newburger, D. E., Kashef-Haghighi, D., Weng, Z., Salari, R., Sweeney, R. T., Brunner, A. L., et al. (2013). Genome evolution during progression to breast cancer. Genome Res. 23, 1097–1108. doi: 10.1101/gr.151670.112
Nik-Zainal, S., Alexandrov, L. B., Wedge, D. C., Van Loo, P., Greenman, C. D., Raine, K., et al. (2012a). Mutational processes molding the genomes of 21 breast cancers. Cell 149, 979–93. doi: 10.1016/j.cell.2012.04.024
Nik-Zainal, S., Davies, H., Staaf, J., Ramakrishna, M., Glodzik, D., Zou, X., et al. (2016). Landscape of somatic mutations in 560 breast cancer whole-genome sequences. Nature 534, 47–54. doi: 10.1038/nature17676
Nik-Zainal, S., Van Loo, P., Wedge, D. C., Alexandrov, L. B., Greenman, C. D., Lau, K. W., et al. (2012b). The life history of 21 breast cancers. Cell 149, 994–1007. doi: 10.1016/j.cell.2012.04.023
Nowell, P. C. (1976). The clonal evolution of tumor cell populations. Science 194, 23–28. doi: 10.1126/science.959840
Oesper, L., Mahmoody, A., and Raphael, B. J. (2013). Theta: inferring intra-tumor heterogeneity from high-throughput dna sequencing data. Genome Biol. 14:R80. doi: 10.1186/gb-2013-14-7-r80
Oesper, L., Satas, G., and Raphael, B. J. (2014). Quantifying tumor heterogeneity in whole-genome and whole-exome sequencing data. Bioinformatics 30, 3532–3540. doi: 10.1093/bioinformatics/btu651
Ortmann, C. A., Kent, D. G., Nangalia, J., Silber, Y., Wedge, D. C., Grinfeld, J., et al. (2015). Effect of mutation order on myeloproliferative neoplasms. N. Engl. J. Med. 372, 601–612. doi: 10.1056/NEJMoa1412098
Paull, E. O., Carlin, D. E., Niepel, M., Sorger, P. K., Haussler, D., and Stuart, J. M. (2013). Discovering causal pathways linking genomic events to transcriptional states using tied diffusion through interacting events (tiedie). Bioinformatics 29, 2757–2764. doi: 10.1093/bioinformatics/btt471
Petljak, M., and Alexandrov, L. B. (2016). Understanding mutagenesis through delineation of mutational signatures in human cancer. Carcinogenesis 37, 531–540. doi: 10.1093/carcin/bgw055
Popic, V., Salari, R., Hajirasouliha, I., Kashef-Haghighi, D., West, R. B., and Batzoglou, S. (2015). Fast and scalable inference of multi-sample cancer lineages. Genome Biol. 16:91. doi: 10.1186/s13059-015-0647-8
Puente, X. S., Beà, S., Valdés-Mas, R., Villamor, N., Gutiérrez-Abril, J., Martín-Subero, J. I., et al. (2015). Non-coding recurrent mutations in chronic lymphocytic leukaemia. Nature 526:519–524. doi: 10.1038/nature14666
Qiao, Y., Quinlan, A. R., Jazaeri, A. A., Verhaak, R. G., Wheeler, D. A., and Marth, G. T. (2014). Subcloneseeker: a computational framework for reconstructing tumor clone structure for cancer variant interpretation and prioritization. Genome Biol. 15:443. doi: 10.1186/s13059-014-0443-x
Raphael, B. J., Dobson, J. R., Oesper, L., and Vandin, F. (2014). Identifying driver mutations in sequenced cancer genomes: computational approaches to enable precision medicine. Genome Med. 6:5. doi: 10.1186/gm524
Raphael, B. J., and Vandin, F. (2015). Simultaneous inference of cancer pathways and tumor progression from cross-sectional mutation data. J. Comput. Biol. 22, 510–527. doi: 10.1089/cmb.2014.0161
Reimand, J., Arak, T., Adler, P., Kolberg, L., Reisberg, S., Peterson, H., et al. (2016). g: Profiler—a web server for functional interpretation of gene lists (2016 update). Nucleic Acids Res. 44, W83–W89. doi: 10.1093/nar/gkw199
Reimand, J., and Bader, G. D. (2013). Systematic analysis of somatic mutations in phosphorylation signaling predicts novel cancer drivers. Mol. Syst. Biol. 9:637. doi: 10.1038/msb.2012.68
Ross, E. M., and Markowetz, F. (2016). Onconem: inferring tumor evolution from single-cell sequencing data. Genome Biol. 17:69. doi: 10.1186/s13059-016-0929-9
Roth, A., Khattra, J., Yap, D., Wan, A., Laks, E., Biele, J., et al. (2014). Pyclone: statistical inference of clonal population structure in cancer. Nat. Methods 11, 396–398. doi: 10.1038/nmeth.2883
Salehi, S., Steif, A., Roth, A., Aparicio, S., Bouchard-Côté, A., and Shah, S. P. (2017). ddclone: joint statistical inference of clonal populations from single cell and bulk tumour sequencing data. Genome Biol. 18:44. doi: 10.1186/s13059-017-1169-3
Schuh, A., Becq, J., Humphray, S., Alexa, A., Burns, A., Clifford, R., et al. (2012). Monitoring chronic lymphocytic leukemia progression by whole genome sequencing reveals heterogeneous clonal evolution patterns. Blood 120, 4191–4196. doi: 10.1182/blood-2012-05-433540
Schwartz, R., and Schäffer, A. A. (2017). The evolution of tumour phylogenetics: principles and practice. Nat. Rev. Genet. 18, 213–229. doi: 10.1038/nrg.2016.170
Sengupta, S., Wang, J., Lee, J., Müller, P., Gulukota, K., Banerjee, A., et al. (2015). “Bayclone: Bayesian nonparametric inference of tumor subclones using ngs data,” in Pacific Symposium on Biocomputing (Big Island), Vol. 20:467.
Shrestha, R., Hodzic, E., Yeung, J., Wang, K., Sauerwald, T., Dao, P., et al. (2014). “Hit'ndrive: multi-driver gene prioritization based on hitting time,” in International Conference on Research in Computational Molecular Biology (Pittsburgh, PA: Springer), 293–306.
Sjöblom, T., Jones, S., Wood, L. D., Parsons, D. W., Lin, J., Barber, T. D., et al. (2006). The consensus coding sequences of human breast and colorectal cancers. Science 314, 268–274. doi: 10.1126/science.1133427
Sottoriva, A., Kang, H., Ma, Z., Graham, T. A., Salomon, M. P., Zhao, J., et al. (2015). A big bang model of human colorectal tumor growth. Nat. Genet. 47, 209–216. doi: 10.1038/ng.3214
Stratton, M. R., Campbell, P. J., and Futreal, P. A. (2009). The cancer genome. Nature 458, 719–724. doi: 10.1038/nature07943
Strino, F., Parisi, F., Micsinai, M., and Kluger, Y. (2013). Trap: a tree approach for fingerprinting subclonal tumor composition. Nucleic Acids Res. 41:e165. doi: 10.1093/nar/gkt641
Subramanian, A., Tamayo, P., Mootha, V. K., Mukherjee, S., Ebert, B. L., Gillette, M. A., et al. (2005). Gene set enrichment analysis: a knowledge-based approach for interpreting genome-wide expression profiles. Proc. Natl. Acad. Sci. U.S.A. 102, 15545–15550. doi: 10.1073/pnas.0506580102
Swanton, C. (2012). Intratumor heterogeneity: evolution through space and time. Cancer Res. 72, 4875–4882. doi: 10.1158/0008-5472.CAN-12-2217
Swanton, C. (2016). Tumor evolutionary principles: how intratumor heterogeneity influences cancer treatment and outcome. Am. Soc. Clin. Oncol. 35, e141–e149. doi: 10.14694/EDBK_158930
Tamborero, D., Gonzalez-Perez, A., Perez-Llamas, C., Deu-Pons, J., Kandoth, C., Reimand, J., et al. (2013). Comprehensive identification of mutational cancer driver genes across 12 tumor types. Sci. Rep. 3:2650. doi: 10.1038/srep02650
The Cancer Genome Atlas Research Network (2017a). Integrated genomic and molecular characterization of cervical cancer. Nature 543, 378–384. doi: 10.1038/nature21386
The Cancer Genome Atlas Research Network, (2017b). Integrated genomic characterization of oesophageal carcinoma. Nature 541, 169–175. doi: 10.1038/nature20805
Vandin, F., Clay, P., Upfal, E., and Raphael, B. J. (2012a). Discovery of mutated subnetworks associated with clinical data in cancer. Pac. Symp. Biocomput. 2012, 55–66. doi: 10.1142/9789814366496_0006
Vandin, F., Papoutsaki, A., Raphael, B. J., and Upfal, E. (2015). Accurate computation of survival statistics in genome-wide studies. PLoS Comput. Biol. 11:e1004071. doi: 10.1371/journal.pcbi.1004071
Vandin, F., Raphael, B. J., and Upfal, E. (2016). On the sample complexity of cancer pathways identification. J. Comput. Biol. 23, 30–41. doi: 10.1089/cmb.2015.0100
Vandin, F., Upfal, E., and Raphael, B. J. (2011). Algorithms for detecting significantly mutated pathways in cancer. J. Comput. Biol. 18, 507–522.
Vandin, F., Upfal, E., and Raphael, B. J. (2012b). De novo discovery of mutated driver pathways in cancer. Genome Res. 22, 375–385. doi: 10.1101/gr.120477.111
Vandin, F., Upfal, E., and Raphael, B. J. (2012c). Finding driver pathways in cancer: models and algorithms. Algorithms Mol. Biol. 7:23. doi: 10.1186/1748-7188-7-23
Vanunu, O., Magger, O., Ruppin, E., Shlomi, T., and Sharan, R. (2010). Associating genes and protein complexes with disease via network propagation. PLoS Comput. Biol. 6:e1000641. doi: 10.1371/journal.pcbi.1000641
Vaske, C. J., Benz, S. C., Sanborn, J. Z., Earl, D., Szeto, C., Zhu, J., et al. (2010). Inference of patient-specific pathway activities from multi-dimensional cancer genomics data using paradigm. Bioinformatics 26, i237–i245. doi: 10.1093/bioinformatics/btq182
Vogelstein, B., and Kinzler, K. W. (2004). Cancer genes and the pathways they control. Nat. Med. 10, 789–799. doi: 10.1038/nm1087
Vogelstein, B., Papadopoulos, N., Velculescu, V. E., Zhou, S., Diaz, L. A., and Kinzler, K. W. (2013). Cancer genome landscapes. Science 339, 1546–1558. doi: 10.1126/science.1235122
Wang, Y., Waters, J., Leung, M. L., Unruh, A., Roh, W., Shi, X., et al. (2014). Clonal evolution in breast cancer revealed by single nucleus genome sequencing. Nature 512, 155–160. doi: 10.1038/nature13600
Weinhold, N., Jacobsen, A., Schultz, N., Sander, C., and Lee, W. (2014). Genome-wide analysis of noncoding regulatory mutations in cancer. Nat. Genet. 46, 1160–1165. doi: 10.1038/ng.3101
Wendl, M. C., Wallis, J. W., Lin, L., Kandoth, C., Mardis, E. R., Wilson, R. K., et al. (2011). Pathscan: a tool for discerning mutational significance in groups of putative cancer genes. Bioinformatics 27, 1595–1602. doi: 10.1093/bioinformatics/btr193
Xu, X., Hou, Y., Yin, X., Bao, L., Tang, A., Song, L., et al. (2012). Single-cell exome sequencing reveals single-nucleotide mutation characteristics of a kidney tumor. Cell 148, 886–895. doi: 10.1016/j.cell.2012.02.025
Yachida, S., Jones, S., Bozic, I., Antal, T., Leary, R., Fu, B., et al. (2010). Distant metastasis occurs late during the genetic evolution of pancreatic cancer. Nature 467, 1114–1117. doi: 10.1038/nature09515
Yates, L. R., and Campbell, P. J. (2012). Evolution of the cancer genome. Nat. Rev. Genet. 13, 795–806. doi: 10.1038/nrg3317
Yeang, C.-H., McCormick, F., and Levine, A. (2008). Combinatorial patterns of somatic gene mutations in cancer. FASEB J. 22, 2605–2622. doi: 10.1096/fj.08-108985
Youn, A., and Simon, R. (2011). Identifying cancer driver genes in tumor genome sequencing studies. Bioinformatics 27, 175–181. doi: 10.1093/bioinformatics/btq630
Yuan, K., Sakoparnig, T., Markowetz, F., and Beerenwinkel, N. (2015). Bitphylogeny: a probabilistic framework for reconstructing intra-tumor phylogenies. Genome Biol. 16, 36. doi: 10.1186/s13059-015-0592-6
Keywords: cancer heterogeneity, mutations, cancer pathways, mutual exclusivity, clinical association
Citation: Vandin F (2017) Computational Methods for Characterizing Cancer Mutational Heterogeneity. Front. Genet. 8:83. doi: 10.3389/fgene.2017.00083
Received: 07 February 2017; Accepted: 30 May 2017;
Published: 14 June 2017.
Edited by:
Rosalba Giugno, University of Verona, ItalyReviewed by:
Luciano Cascione, Institute of Oncology Research, SwitzerlandCopyright © 2017 Vandin. This is an open-access article distributed under the terms of the Creative Commons Attribution License (CC BY). The use, distribution or reproduction in other forums is permitted, provided the original author(s) or licensor are credited and that the original publication in this journal is cited, in accordance with accepted academic practice. No use, distribution or reproduction is permitted which does not comply with these terms.
*Correspondence: Fabio Vandin, ZmFiaW8udmFuZGluQHVuaXBkLml0
Disclaimer: All claims expressed in this article are solely those of the authors and do not necessarily represent those of their affiliated organizations, or those of the publisher, the editors and the reviewers. Any product that may be evaluated in this article or claim that may be made by its manufacturer is not guaranteed or endorsed by the publisher.
Research integrity at Frontiers
Learn more about the work of our research integrity team to safeguard the quality of each article we publish.