- 1Faculty of Veterinary and Animal Sciences, University of Chile, Santiago, Chile
- 2Aquainnovo, Puerto Montt, Chile
- 3The Roslin Institute and Royal (Dick) School of Veterinary Studies, University of Edinburgh, Midlothian, UK
- 4Genus plc, Hendersonville, TN, USA
Infectious and parasitic diseases generate large economic losses in salmon farming. A feasible and sustainable alternative to prevent disease outbreaks may be represented by genetic improvement for disease resistance. To include disease resistance into the breeding goal, prior knowledge of the levels of genetic variation for these traits is required. Furthermore, the information from the genetic architecture and molecular factors involved in resistance against diseases may be used to accelerate the genetic progress for these traits. In this regard, marker assisted selection and genomic selection are approaches which incorporate molecular information to increase the accuracy when predicting the genetic merit of selection candidates. In this article we review and discuss key aspects related to disease resistance in salmonid species, from both a genetic and genomic perspective, with emphasis in the applicability of disease resistance traits into breeding programs in salmonids.
Introduction
Farming of salmonid species is one of the largest aquaculture industries, with a worldwide production of approximately 1.9 million tons of high value product in 2010 (Food and Agriculture Organization of the United Nations [FAO], 2012). As in other animal production systems, the success and sustainability of salmonid aquaculture largely depends on the control of diseases. A clear example of the negative impact of infectious diseases in salmon farming is the unprecedented economic loss caused by outbreaks of the viral disease infectious salmon anemia (ISA) between 2007 and 2009 in Chile (Asche et al., 2010).
Genetic improvement programs are focused on increasing economic return of aquaculture systems via selective breeding (Gjedrem, 2012). In this regard, all heritable and economically relevant traits should be included in the breeding objective. Thus, in salmonid species, traits such as growth rate, flesh color, and resistance to viral, bacterial, and parasitic diseases should be included (Gjedrem, 2000, 2012). Selective breeding can utilize trait information recorded on selection candidates themselves or, particularly in the case of disease or invasive traits, on relatives. Until now, salmon breeding programs have typically included disease resistance based only on information from relatives, which affects the degree of genetic progress achievable on each generation. This is because of the lower accuracy of estimated breeding values (EBVs) when using only sib information compared to the accuracy obtained when using information of the selection candidates themselves (Falconer and Mackay, 1996).
Recent advances in molecular biology techniques, such as next generation sequencing and high throughput genotyping methods, have helped identify genetic variants influencing phenotypic variation for different traits in a wide range of organisms (Goddard and Hayes, 2009). Molecular markers can be used for a variety of applications in livestock and aquaculture species, such as strain and hybrid identification, genetic variability and genetic diversity evaluation, parentage analyses, quantitative trait loci (QTL) mapping, marker assisted selection (MAS), and genomic selection (GS; Liu and Cordes, 2004; Goddard and Hayes, 2009). Information from a few molecular markers linked to QTL (i.e., genomic regions harboring genes with a significant effect on the trait) might be implemented in breeding schemes through MAS, if they explain a high proportion of genetic variation in the trait. Additionally, the information of 1000s of markers might be simultaneously incorporated into genetic evaluation to estimate genomic breeding values (GEBVs; Meuwissen et al., 2001). These marker-based methods may be particularly useful for the improvement of traits that are complicated or impossible to measure directly on selection candidates, as is the case of resistance to disease (Sonesson and Meuwissen, 2009; Villanueva et al., 2011; Taylor, 2014). A typical first step to implement MAS or GS is to quantify the level of genetic variation in the trait by dissecting its genetic architecture. In salmonids, there is limited information on the genetic architecture of disease resistance. Nevertheless, it is expected that more knowledge on the QTL or genes affecting disease resistance traits will be revealed in the near future, facilitated by the increasing availability of genomic resources and better understanding of the biology of immune response in these species.
This paper reviews aspects of conventional breeding to improve disease resistance in salmonids and the application of molecular tools for the identification of genetic factors involved in these traits. Additionally, the incorporation of molecular information into breeding schemes to improve disease resistance is discussed.
Importance of Disease Control in Salmon Farming
The health status of farmed fish is one of the main factors affecting the economic return in the salmon industry. Despite scientific, professional, and technical strategies aimed at improving health management, many novel pathological conditions have emerged in salmonid fish species worldwide in recent decades. A detailed description of each disease affecting culture of salmonid species would greatly exceed the purpose of this review. However, some particular examples are discussed to demonstrate the large economic impact diseases can cause in salmon production.
One of the most striking cases affecting salmon farming was the economic crisis triggered by ISA virus outbreaks since mid-2007 in Chile. The production of Chilean Atlantic salmon suffered a dramatic decrease due to increasingly frequent outbreaks between 2007 and 2009. In fact, total production of Atlantic salmon between 2005 and 2010 decreased by more than 60% in volume (Asche et al., 2010). Currently, ISA virus outbreaks appear to be controlled to a low number of events per year in Europe and North and South America. However, the prevalence and emergence of other viral diseases is still of concern. In Northern European countries including Norway and Scotland, ISA outbreaks have been rare in recent years. Nevertheless, infectious pancreatic necrosis (IPN), caused by an aquatic birnavirus, has caused large levels of mortality in Europe, particularly during the window of susceptibility following transfer from freshwater to seawater (Roberts and Pearson, 2005). Other viral diseases have also emerged and pose serious threats to salmon aquaculture, such as skeletal muscle inflammation (HSMI) – a piscine reovirus – and pancreas disease (PD) – an alphavirus, which has shown an increase in recent years (Biering et al., 2012). These viruses cause direct economic losses through mortality and indirect losses through reduced growth rate and treatment costs.
Among bacterial diseases with a negative impact on salmon farming, salmon rickettsial syndrome (SRS) caused by the Gram-negative bacterium Piscirickettsia salmonis, is one of the main sanitary challenges in Chilean salmon industry. This disease affects different salmonid species, including Atlantic salmon (Salmo salar), coho salmon (Oncorhynchus kisutch), and rainbow trout (O. mykiss; Fryer and Hedrick, 2003) and can generate economic losses equivalent to 25% of total profit in salmon exports in Chile (Rozas and Enríquez, 2014). Other bacterial diseases, such as those caused by Aeromonas salmonicida, Vibrio anguillarum, and Vibrio/Aliivibrio salminonicida, are recognized to be efficiently controlled by vaccination and do not currently represent a major economic threat for salmon (Biering et al., 2012).
In terms of parasitic diseases, two different species of sea lice, Lepeophtheirus salmonis and Caligus rogercresseyi, are most detrimental parasites for salmon farming at a worldwide level. In this regard, it has been estimated that on average, the economic impact of sea lice infestation is about 6% of the total value produced by the world salmon industry (Costello, 2009). An emerging threat to salmon production worldwide is Amoebic Gill Disease (AGD), which has been the major disease of farmed salmonid production in Tasmania for several decades (Mitchell and Rodger, 2011) and has appeared relatively recently in most major salmon-producing countries (Ruane and Jones, 2013). The free-living amoebic protozoan Neoparamoeba pemaquidensis is the primary causative agent for the disease that can cause serious morbidity and reduced growth, in addition to increasing susceptibility to other pathogens (Mitchell and Rodger, 2011).
The measures used for prevention and treatment (vaccinations, antibiotics, and antiparasitic drugs, biosecurity measures) of some of the diseases presented above have typically been only partially effective in field conditions (Bravo et al., 2013; Jones et al., 2013; Rozas and Enríquez, 2014). Where effective vaccines do exist, administration typically requires individual handling and treatment of all production fish, which can be expensive and impractical in a large-scale production environment. Due to the fact that improvement in economic efficiency of salmon farming is dependent on disease prevention and control, (Asche and Roll, 2013) it is imperative to develop alternative effective and sustainable strategies. Genetic improvement of disease resistance represents a feasible solution to increase the sanitary status in animal production (Stear et al., 2001; Bishop, 2010). In this regard, there is increasing scientific literature aiming at both quantifying levels of host genetic variation for resistance against different diseases and identifying the specific genetic factors that influence these traits in salmonid species, as discussed below.
Conventional Breeding for Disease Resistance in Salmonids
Resistance to diseases can be defined as the ability of the host to limit infection by reducing pathogen replication (Råberg et al., 2007; Doeschl-Wilson et al., 2012). Selecting animals with increased resistance to specific diseases is a feasible method to improve productivity and animal welfare and offers advantages over other control methods against infection, such as the cumulative and permanent benefits of the improved resistance (Stear et al., 2001; Bishop, 2010). Disease resistance has been a target trait for the salmon breeding industry for at least 20 years, with a Norwegian salmon breeding program including resistance to bacterial and viral diseases into its breeding goal since 1993 (Gjøen and Bentsen, 1997). However, the study of disease resistance and its incorporation into breeding programs can be hindered by the difficulty in determining and measuring accurate and appropriate phenotypes (Bishop and Woolliams, 2014). This in turn influences the accuracy of disease resistance EBVs that can be achieved. Another limiting step is that disease information is typically only available from relatives of the selection candidates and not directly from the candidates themselves. In the following, we review the main aspects of breeding for resistance to infectious diseases in salmonids and discuss current status and future directions of research in this area.
Challenge Against Pathogens
Host resistance to viral and bacterial pathogens can often be measured, in practical terms, as survival (and/or mortality) of individuals during an outbreak (Ødegård et al., 2011). Data and samples from field outbreaks can be used opportunistically to make inference about genetic resistance to infectious diseases. For this purpose, it is necessary that the pedigree of the population be accurately determined, often using genetic markers or electronic tagging of the fish (Guy et al., 2006). However, using the information from field outbreaks has some disadvantages, such as difficulty to identify the exact cause of death because the factors that influence survival under these conditions are likely to be diverse. Furthermore, the availability of information depends on the occurrence of high-mortality outbreaks, which are usually prevented or controlled to avoid serious economic loss. Moreover, the inference of pedigree using molecular markers can be expensive and laborious. Therefore, survival data are often obtained from experimental challenges, which can readily be standardized to control other variables and potentially allow a clearer interpretation of the results. In this case, it is necessary that a high genetic correlation between the trait measured in experimental and field conditions exists. High genetic correlations (rg ≥ 0.95) between field trials and experimental challenges to furunculosis in Atlantic salmon have been reported (Gjøen et al., 1997; Ødegård et al., 2006), suggesting that results from experimental challenges are likely to be directly applicable to commercial production systems. Therefore, challenge tests will often be more accurate and reliable than field outbreaks, due to decreased environmental variability and higher practical feasibility. In fact, challenge testing is currently used to select for resistance to viral, bacterial, and parasitic diseases in breeding programs for Atlantic salmon and rainbow trout (Gjøen and Bentsen, 1997; Leeds et al., 2010; Yáñez and Martínez, 2010; Ødegård et al., 2011; Gjedrem, 2012; Wiens et al., 2013a).
Immunological and Physiological Variables as Indirect Measures of Resistance
Direct genetic selection for improved disease resistance based on challenge testing can be costly and time consuming, and has negative animal welfare implications. Furthermore, selection decisions using this strategy can only be carried out using information from relatives and not the candidates themselves. Indirect selection based on the measurement of other characteristics that are genetically correlated with disease resistance, would simplify the data collection and allow the incorporation of individual information. Some studies have aimed at determining the genetic variation of physiological and immunological variables, and the correlation between them and survival in challenge tests in salmon. Examples of variables that have been studied to date are hemolytic activity of serum and lysozyme activity (Røed et al., 1993; Lund et al., 1995), plasma levels of cortisol (Fevolden et al., 1993; Weber et al., 2008), and levels of IgM and antibody titer (Lund et al., 1995), serum α2-antiplasmin (Salte et al., 1993), bactericidal and complement activity (Hollebecq et al., 1995). However, even when some studies show significant correlations between resistance and immune parameters, the proportion of the total variation in survival that could be explained by immune variables has been considered too low to be useful as a selection criterion. Hence, the prediction of breeding values for survival based on these variables may not be practically useful (Gjøen and Bentsen, 1997). This may be due in part to the complexity of the mechanisms involved in the immune response and the large number of factors that may be involved in disease resistance, which results in a great difficulty when trying to use the information from a single parameter for the genetic evaluation of disease resistance.
Genetic Variation in Resistance to Infectious Diseases
A requirement to improve a trait by means of artificial selection is that sufficient genetic variation for this trait exists in the population. Heritability is the proportion of the total phenotypic variance that is attributable to additive genetic variation (Falconer and Mackay, 1996). For disease resistance traits, heritability estimates can vary due to differences in trait definitions and the statistical models used in the analysis (Yáñez and Martínez, 2010; Ødegård et al., 2011). For example, some studies consider the binary trait of survival or mortality as a measure of resistance, whereas others consider the survival time following challenge. These require different statistical approaches to analysis, and are likely to inform on different components of the host response to infection. Nonetheless, there have been several studies aimed at determining levels of additive genetic variation for resistance to different diseases affecting salmonid species (see Table 1). The results from these studies show the feasibility of improving disease resistance through genetic improvement and the potential of this approach for helping in the control of disease problems in salmonids.
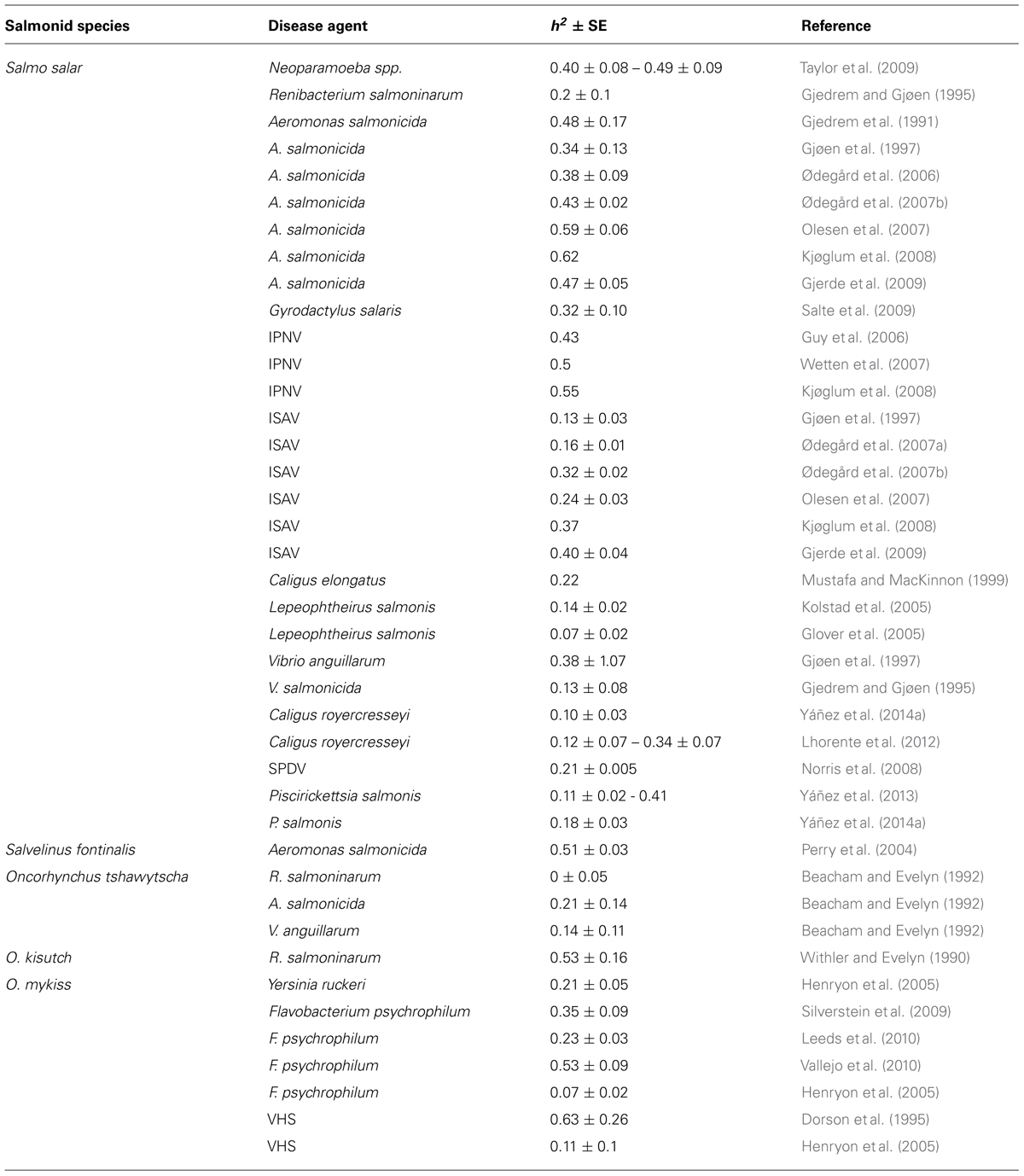
TABLE 1. Heritabilty values (h2) and their SE for resistance to different infectious and parasitic diseases in salmonid species.
Genetic Correlations between Disease Resistance and Other Traits
The potential to simultaneously improve resistance to different diseases and other economically important traits is partly dependent on the genetic correlations between the traits. Few studies to date have aimed to determine the genetic correlations for disease resistance traits in salmon. Some studies in Atlantic salmon have indicated positive genetic correlations between resistance to bacterial diseases such as furunculosis, BKD, and cold water Vibriosis (Gjedrem and Gjøen, 1995; Gjøen et al., 1997). Weak negative genetic correlations between resistance against ISA and bacterial diseases such as furunculosis, vibriosis, and cold-water vibriosis have been reported (Gjøen et al., 1997). However, positive genetic correlations between resistance against ISA virus and furunculosis have also been reported (Ødegård et al., 2007b). In rainbow trout, weak genetic correlations between viral hemorrhagic septicemia (VHS) and bacterial diseases such as enteric redmouth disease (ERM) and rainbow trout fry syndrome have been found (Henryon et al., 2005). Kjøglum et al. (2008) reported only weak genetic correlations when they estimated genetic correlations between resistance to IPN, ISA, and furunculosis. Verrier et al. (2013b) also failed to detect any genetic correlation between host resistance to two rhabdoviral pathogens (VHS and Infectious Hematopoietic Virus). Additionally, weak genetic correlations have also been calculated between resistance against SRS and C. rogercresseyi in Atlantic salmon (Yáñez et al., 2014a). In general, these results suggest no clear-cut relationship between genetic resistance to one pathogen and genetic resistance to another pathogen.
Moreover, it is important to know the genetic correlations between disease resistance and other economically important traits in salmon production, especially for selection index development. Previous reports of correlations between disease resistance and production traits have ranged from zero (Silverstein et al., 2009), low to moderately negative (Henryon et al., 2002), inconsistent (Beacham and Evelyn, 1992; Henryon et al., 2002), and low to moderately positive (Gjedrem et al., 1991; Perry et al., 2004; Yáñez et al., 2014a). Thus, it is important to evaluate these parameters for each particular breeding population to maximize the impact of selecting for improved disease resistance. Additionally, the relationship between ploidy levels and disease resistance is of interest because all female triploid rainbow trout are advantageous for production. Weber et al. (2013) showed that triploid fish are generally slightly more susceptible to Bacterial Coldwater Disease than diploids, but that selection for improved resistance in diploids is also effective for triploid production.
Resistance Versus Tolerance
If resistance is defined as an individual’s ability to block the reproduction of a pathogen, then disease tolerance can be defined as the ability to limit the impact of infection on a host (Råberg et al., 2007; Doeschl-Wilson et al., 2012). Information relating to the genetic basis of disease tolerance has been sparse in animal genetics studies to date (Doeschl-Wilson et al., 2012). However, it is important to disentangle resistance from tolerance because the appropriate trait to select for may differ depending on the disease, host species, and environment. It is also possible that the two traits will be antagonistic, which could result in inadvertent undesirable outcomes of selection for disease resistance (Doeschl-Wilson et al., 2012). The implications of selection for resistance or tolerance on the host–pathogen interaction and pathogen evolution have also been considered. For example, selection for resistance per se may lead to selection pressure for higher virulence in the pathogen, whereas selection for tolerance could result in co-existence of pathogen and host with minimal impact on the performance of the host population. Therefore, in future disease studies and in selective breeding programs, it will be important to carefully consider the optimal disease trait to target for maximum benefit at the population level, but also the analytic issues of resistance versus tolerance.
Strategies for Genetic Dissection of Disease Resistance Traits
Major advances in nucleotide sequencing, ever-improving bioinformatics pipelines, and high-throughput genotyping tools have helped to identify genes associated with complex traits in various species of vertebrates. In salmonids, this is reflected in the substantial increase in genomic resources for these species during recent years and, for example, in the formation of an international collaboration to sequence the Atlantic salmon genome (Davidson et al., 2010). The salmon genome has undergone a relatively recent duplication and has a very high content of long repeat elements. This has hampered the sequencing and assembly of a reference genome for salmonids. However, a high-quality reference sequence has been published for rainbow trout (Berthelot et al., 2014) and is available for Atlantic salmon (Davidson et al., 2010). Further, high density SNP genotyping arrays were recently developed for Atlantic salmon (Houston et al., 2014; Yáñez et al., 2014b), and a lower density platform (Lien et al., 2011) has previously been used for QTL mapping and population genetics. Currently, these genomic resources are increasingly being used for the identification of the genetic factors involved in the resistance to different diseases in salmonids. The strategies used in the study of the genetic architecture of disease resistance can be classified as: (i) candidate gene approaches, (ii) QTL mapping, and (iii) gene expression studies.
Candidate Gene Approach
Candidate gene theory states that a significant proportion of phenotypic variance of one trait in a population is determined by the presence of polymorphisms within genes known to be involved in the physiological regulation of that trait (Rothschild and Soller, 1997). This approach requires previous knowledge on the biology of the species, biochemical pathways, and especially gene sequences, to study the variation within specific candidate genes. In aquaculture species, the availability of annotated gene sequences of known function is typically low, but is likely to increase in the short term with the use of high-throughput sequencing and ongoing genome sequencing projects.
In vertebrates, the major histocompatibility complex (MHC) has attracted much attention in studies of association between genetic variants and disease resistance. However, other genes are likely to play an important role in the mechanisms of disease resistance in production animals, model organisms, and humans (Hill, 1999; Qureshi et al., 1999). To our knowledge, there are no studies aimed at establishing association between candidate genes, other than the MHC, and resistance to infectious diseases in salmonid species.
Major histocompatibility complex
The MHC is a multigene family that acts at the interface between the immune system and infectious pathogens. The MHC gene family comprises two subfamilies: class I and II. Both classes are membrane glycoproteins involved in the processing and removal of pathogens (Thorgaard et al., 2002). MHC genes have been identified, cloned, and characterized in Atlantic salmon, rainbow trout, and other salmonids (Grimholt et al., 1993; Hordvik et al., 1993; Hansen et al., 1996; Shum et al., 1999, 2002). Furthermore, it has been shown that these genes are highly polymorphic in these species (Grimholt et al., 1994, 2002; Miller and Withler, 1996; Hansen et al., 1999; Garrigan and Hedrick, 2001; Aoyagi et al., 2002). As in other vertebrates there are two types of class I genes; the UAA which are highly divergent, non-polymorphic and expressed at low levels, and the UBA which are polymorphic, expressed at high levels in spleen, and with structural features similar to those of class Ia molecules (classical) which present antigen to T lymphocytes (Shum et al., 1999). The class II genes are divided into Class II A (DAA) and II B (DAB), depending on whether encoding α or β chain of the molecule, respectively (Grimholt et al., 2000; Stet et al., 2002). Both loci (DAA and DAB) co-segregate as haplotypes, suggesting a close physical linkage between them in Atlantic salmon (Stet et al., 2002).
The association between a polymorphism linked to MHC class II genes and resistance to virus infectious hematopoietic necrosis (IHN) in backcrosses of rainbow trout and cutthroat trout (O. clarki) has been found, but was relatively weak and dependent on the family analyzed (Palti et al., 2001). Suggestive associations between rainbow trout MHC class IB alleles and bacterial cold water disease have also been shown (Johnson et al., 2008). In Atlantic salmon, the association between MH class IIB alleles and resistance against A. salmonicida has been reported (Langefors et al., 2001; Lohm et al., 2002). In the same species, MHC class I and class II variants have been associated with susceptibility to IHN (Miller et al., 2004) and resistance to furunculosis and ISA (Grimholt et al., 2003; Kjøglum et al., 2006). Although associations between MH gene variants and disease resistance have been established, the MHC is most likely not the only factor influencing genetic variation in disease resistance. For instance, in Atlantic salmon, a non-MHC effect for resistance to IPN, furunculosis and ISA has been detected (Kjøglum et al., 2005). Because disease resistance traits will typically be polygenic in nature, it is important to consider variants in a genome-wide context and possible interactions that can occur between genes (epistasis), which hinders the candidate gene approach as a comprehensive strategy for incorporating molecular information into the genetic evaluation of these traits.
QTL Mapping
Quantitative trait loci mapping is a strategy providing information on the location and effect of the gene variants influencing complex quantitative traits, but without prior hypotheses. The QTL detection methodologies are based on the use of (typically anonymous) DNA markers dispersed throughout the genome to identify genomic regions involved in the genetic variation of a particular trait, by means of statistical analyses utilizing the co-segregation between markers and the (unknown) causative variants.
DNA markers
The development of DNA markers has had a major impact on studies of genetic variation in animals and fish. The categories of DNA markers widely used historically include Restriction Fragment Length Polymorphisms (RFLP), Random Amplified Polymorphic DNA (RAPD), Amplified Fragment Length Polymorphism (AFLP), microsatellites, and single nucleotide polymorphisms (SNPs). More recently, SNP markers have become the predominant marker due to their abundance, ease of discovery and low cost of genotyping per locus (Houston et al., 2014). However, each of these marker types vary from each other in their mode of inheritance (i.e., dominant or codominant), identification and detection methods, number of spanning loci and polymorphic information content (Liu and Cordes, 2004).
Microsatellites are tandem repeat nucleotide sequences that generally span between one to six base pairs. Different alleles are generated due to the variation in the number of repeats. Their main useful features for genetic studies include high variability, codominant inheritance, abundance, and wide distribution across the genome. One of the major advantages of microsatellites is their high degree of polymorphism, with 10s of alleles often observed at a single locus in an outbred population. Additionally, their genotyping is typically based on a simple DNA amplification by means of polymerase chain reaction (PCR). Thus, genotyping can be relatively rapid, cheap, and the amount of DNA required is minimal (nanograms). However, the scoring of microsatellites often requires optimization and manual input, which reduces their scalability for large genetic studies. There are a considerable number of microsatellites identified for different salmonid species, available for use in genetic studies (e.g., Cairney et al., 2000; Gilbey et al., 2004; Phillips et al., 2009).
Historically, AFLPs (which typically score SNPs) had the advantage of being generated more easily and cheaply than SNPs and microsatellites, because previous knowledge on genomic sequence is not needed for their generation. However, their mode of inheritance, similar to RAPDs, is dominant, i.e., it is not possible to distinguish between heterozygous and one category of homozygous genotypes without the use of special equipment and software (Piepho and Koch, 2000). This reduces the amount of information provided by these kinds of markers.
Single nucleotide polymorphismss have several key advantages for genetic studies, which have led to a rapid increase in their popularity over recent years. Some of these include high abundance, amenability to automated scoring in large numbers, simultaneous assays of thousands of markers (e.g., using SNP arrays) and presence in both coding and non-coding regions. Therefore, SNPs have been applied recently for construction of dense genetic maps, which can be used for fine mapping of QTLs and facilitate the identification of causative genes involved in the genetic variation of specific characters (e.g., Lien et al., 2011; Gonen et al., 2014). Recently, there has been a rapid increase in available SNPs for salmonid species, mainly for Atlantic salmon and rainbow trout (Hayes et al., 2007; Sanchez et al., 2009; Everett et al., 2011, 2012; Hohenlohe et al., 2011; Lien et al., 2011; Houston et al., 2012, 2014; Salem et al., 2012). Additionally, SNP arrays are available for simultaneous genotyping of 10s to 100s of 1000s of markers in rainbow trout (Palti et al., 2014b) and Atlantic salmon (Houston et al., 2014; Yáñez et al., 2014b). These SNP resources are likely to be increasingly applied to high-resolution mapping of disease resistance genes in salmonid species.
In recent years, the same sequencing technology that has led to the increased ease of high-density SNP discovery described above has enabled direct genotyping of individual fish based on sequence information alone. Such techniques are collectively termed ‘genotyping by sequencing’ (GBS). Although GBS techniques encompass a diverse range of laboratory and bioinformatic pipelines, they are all based on the principle of using nucleotide barcodes ligated to the fragmented genomic DNA of individual fish and high-throughput sequencing in multiplexed pools (Davey et al., 2013). Partly due to the lack of established genomics resources for many farmed fish species, the aquaculture genetics community has been early adopters and developers of these techniques. In particular, RAD sequencing has been utilized in both Atlantic salmon (e.g., Houston et al., 2012) and rainbow trout (Palti et al., 2014a) as a conduit to incorporating genomic information into aquaculture breeding programs. While the application of GBS has huge potential for applied research in salmonids, there are also some challenges in managing and interpreting these datasets. For example, discovery of ‘false’ SNPs using sequencing can occur, particularly with the recent whole genome duplication of the salmonid species. Therefore, species-tailored bioinformatics pipelines are typically required to minimize these issues.
Detection of QTL affecting disease resistance in salmonid species
Salmon may be more likely than terrestrial farmed species to have QTL of major effect because they have had fewer generations of selection in the farmed environment and therefore the standing genetic variation for traits of economic importance may still be very large. The development of a genetic map based on linkage between genetic markers is the first step towards the identification of QTL. To date, several linkage maps have been constructed using different marker types for rainbow trout (e.g., Young et al., 1998; Sakamoto et al., 2000; Nichols et al., 2003; Guyomard et al., 2006; Rexroad et al., 2008; Palti et al., 2011, 2012; Guyomard et al., 2012); Atlantic salmon (Gilbey et al., 2004; Moen et al., 2004b; Lien et al., 2011; Gonen et al., 2014); coho salmon (McClelland and Naish, 2008); brown trout (Salmo trutta; Gharbi et al., 2006); Arctic Trout (Salvelinus alpinus; Woram et al., 2004); and sockeye salmon (Salmo nerka; Everett et al., 2012).
Using microsatellite markers, two QTL with major effects on resistance to IPN in rainbow trout has been detected. These loci explained a large proportion (27 and 34%) of the phenotypic variation in a family from a backcross between a strain susceptible to IPN (YK-RT101) and a resistant one (YN-RT201; Ozaki et al., 2001). Using AFLP and microsatellite markers, QTLs for IHN resistance have been identified in three different linkage groups of the same species (Rodriguez et al., 2004). For the same disease, RFLP markers were associated with resistant and susceptible families in backcrosses of rainbow trout and cutthroat trout (Palti et al., 1999). Extensive research into the genetic architecture of resistance to Bacterial Coldwater Disease has also been undertaken, with evidence for QTL of major effect (Wiens et al., 2013b; Vallejo et al., 2014a,b). Additionally, major QTL have been detected for resistance to whirling disease caused by the myxosporean parasite Myxobolus cerebralis (Baerwald et al., 2010) and VHS (Verrier et al., 2013a).
In Atlantic salmon, using AFLP markers, two QTL associated with resistance to ISA have been detected in two full sib families (Moen et al., 2004a). One of these QTLs has been validated using microsatellite markers from a higher number of genotyped fish. This QTL explained 6% of the phenotypic variation for resistance to ISA and has been mapped to linkage group VIII of the Atlantic salmon genome (following SALMAP notation; Moen et al., 2007). In the same species, a major QTL for resistance to IPN has been identified using data collected from a ‘field’ seawater outbreak of the disease (Houston et al., 2008a). The QTL detection strategy involved utilizing the low male recombination rate observed in salmonids by using just two to three microsatellites per chromosome and male segregation to determine linkage groups with a significant effect, and secondly, a higher number of markers per linkage group. Female segregation was used to confirm the previously detected QTL and position it within the linkage group (Houston et al., 2008a). The major QTL, mapped to linkage group 21, was subsequently confirmed by analyzing nine additional families and a higher saturation of markers (Houston et al., 2008b). The same QTL was then confirmed and fine mapped in an independent population, and haplotypes of markers that could predict the genotype at the QTL were identified based on the linked microsatellites (Moen et al., 2009). Using a restriction-site associated DNA sequencing approach (RAD sequencing), several additional SNP markers linked to the QTL were identified and two SNP markers showed a significant population-level association with resistance in two year classes from an Atlantic salmon breeding population (Houston et al., 2012). The high proportion of the total variance for IPN resistance that this QTL explains has allowed the incorporation of markers linked to it into MAS schemes for the genetic improvement of this trait in Atlantic salmon in both Norway (Moen et al., 2009) and Scotland (Houston et al., 2010).
In general, confidence intervals for mapped QTLs mapped are large. This issue has two consequences: first, widespread confidence intervals might contain a large number of genes (1000s) and, therefore, identification of the causative polymorphism is challenging. Second, the use of markers linked to QTLs in MAS programs is complicated, since the linkage phase between the marker and the QTL throughout the population may be different from family to family. An alternative for QTL fine mapping is to use information from linkage analysis in conjunction with information from linkage disequilibrium (LD) across the population (Meuwissen et al., 2002). Through simulation studies, power and accuracy of this combined approach has been successfully tested for QTL fine mapping, accounting for the structure of commercial salmon populations (Hayes et al., 2006). Additionally, the availability of both high-density SNPs marker panels and high-resolution genetic maps will contribute to detect association between markers and QTLs with higher precision by means of using across-population LD mapping (Goddard and Hayes, 2009). These strategies along with a reference sequence and a consolidated physical map of the genome, will facilitate the identification of causative mutations affecting disease resistance traits through positional studies in salmonid species.
Global Gene Expression
Functional genomics, defined as the application of experimental methods of genomic or systemic coverage to assess gene function using data from structural genomics (mapping and sequencing), has been recognized as an area of primary interest in disease studies (Hiendleder et al., 2005). These methodologies broaden the spectrum of biological research to study, simultaneously, the expression of thousands of genes at the transcriptional level. Currently, genomic resources and new sequencing technologies have helped to assess differential gene expression levels in the response against diseases in salmonid species. These data can help to pinpoint functional genetic variation underlying disease resistance.
An early example, using suppression subtractive hybridization (SSH) and liver samples from individuals injected with a V. Anguillarum bacterium and normal individuals, more than 25 genes important in the immune response in rainbow trout were identified, including sequences of proteins of acute phase of inflammation, complement, and coagulation system (Bayne et al., 2001). Using the same technique, genes involved in signal transduction and innate immunity (among others) have been identified as relevant factors in response to a challenge against A. salmonicida in Atlantic salmon (Tsoi et al., 2004).
The availability of ESTs (expression sequence tags) and cDNA libraries have allowed the development of DNA microarrays which can be used to study the differential expression patterns of a large number of genes simultaneously in salmonids (Rise et al., 2004b; Ewart et al., 2005; von Schalburg et al., 2005). Using a microarray of human cDNAs, differentially expressed transcripts against a challenge with A. Salmonicida have been identified in Atlantic salmon. However, due to species divergence, only 6% of the sequences of the microarray showed detectable hybridization against salmon liver cDNA (Tsoi et al., 2003). Rise et al. (2004a) conducted the first study using a microarray constructed from salmon cDNA libraries. Following this, differential gene expression has been assessed in between macrophages infected and uninfected with P. salmonis and hematopoietic kidney from Atlantic salmon individuals challenged or not against the same pathogen. Differentially expressed genes were proposed to be relevant in immune response and as potential biomarkers of infection with P. salmonis (Rise et al., 2004a). Additionally, using an Atlantic salmon cDNA microarray including more than 4,000 genes extracted from liver, spleen and hematopoietic kidney, several differentially expressed genes in response to infection by A. salmonicida have been found (Ewart et al., 2005). Furthermore, the transcriptomic response against vaccination with an A. salmonicida bacterium has been assessed in Atlantic salmon, revealing temporal and tissue differences in terms of expression levels, which may be relevant in establishing protection (Martin et al., 2006). In addition, gene expression profiles in response to a DNA vaccine for the IHN virus has been studied in rainbow trout, identifying 910 genes modulated in the injection site, and also determining the over-expression of genes of the type I Interferon system (IFN-1) in other tissues, suggesting that this system forms the basis of early antiviral immunity (Purcell et al., 2006). In the studies presented above, certain transcripts that showed variation in their expression levels during infection have low levels of homology with well-characterized genes available in public databases and, thus, do not have a known function (Rise et al., 2004a; Ewart et al., 2005; Martin et al., 2006; Purcell et al., 2006). Therefore, studies based on sequences of the encoded proteins aimed at decrypting their role in the immune response against infection are still needed.
To date, few studies have focused on the analysis of differential expression between resistant and susceptible fish for a particular disease. For example, Sutherland et al. (2014) analyzed gene expression profile differences between chum and pink salmon during infections with sea lice (L. salmonis) to gain insight into the functional mechanisms underlying the divergent resistance to lice observed in these two species. Zhang et al. (2011) took a similar approach to examine the differences between resistant and susceptible salmon families to the pathogen A. salmonicida to highlight the importance of several innate immune response genes. Cofre et al. (2014) also examined differential expression of several candidate genes in families with divergent resistance to IPNV. Finally, Langevin et al. (2012) compared the transcriptional response of resistant and susceptible clonal lines for Bacterial Coldwater Disease. The information provided by this type of analysis might be useful in the discovery of new sets of genes, with or without an assigned function, which may be associated with disease resistance (Walsh and Henderson, 2004). Another possibility is to consider the differential expression levels as a quantitative trait. This may allow identification of QTLs associated with differences in gene expression patterns between resistant and susceptible individuals (eQTL; Pomp et al., 2004; de Koning et al., 2005). However, it remains unclear how the information given by expression analysis can be used in breeding programs (Walsh and Henderson, 2004).
Molecular Marker-Assisted Selection (MAS) and Genomic Selection (GS)
While selective breeding based on performance information from the selection candidate and its relatives is a highly successful means of genetic improvement in the trait of interest, utilizing genetic markers can provide an improvement in both genetic gain and selection accuracy (Goddard and Hayes, 2009). Historically, the use of genetic markers required mapping of QTL and therefore a key parameter for subsequent application in MAS programs is the level of LD between markers and causative mutations at a population level (Goddard and Meuwissen, 2005).
One factor reducing the level of LD each generation is recombination. When the LD between the QTL and the marker only exists within families and not across families, recombination can break the association between marker alleles and the QTL between families. Therefore, the linkage phase between the marker and the QTL should be determined in each generation and separately for each family if it is to be utilized in selection (Wientjes et al., 2013). To determine if the marker and QTL are in LD within each family phenotypic records and genotypes are needed on each generation. This makes unattractive the implementation of MAS exploiting only within-family LD (i.e., linkage) between the QTL and markers (Dekkers and Van der Werf, 2007). In the case of disease resistance traits, this means that all families comprising the breeding nucleus would need to be challenged each generation.
Marker assisted selection schemes are likely to be optimal when the markers explain a large proportion of the total variance of the trait, as in the case of the QTL for resistance to IPN in Atlantic salmon (Houston et al., 2008a, 2012; Moen et al., 2009). The information from markers linked to this QTL is being applied in MAS programs in different breeding populations of Atlantic salmon. Although SNP variants associated with IPN resistance in two different year classes of a breeding nucleus have been discovered (Houston et al., 2012), there is still a need to validate if these alleles are associated with resistance in independent Atlantic salmon populations. This will depend on the LD relationship between the markers and the causative mutation in the population(s) of interest. As such, testing the association between markers and IPNV mortality in these populations should be considered a prerequisite for effective commercial application.
Another alternative to exploit the LD at the population level is using information from high density panels of markers to predict GEBVs of selection candidates (Meuwissen et al., 2001). This approach effectively takes into account all markers when estimating the breeding value of the candidate, without the need to surpass a significance threshold for association with a particular trait of interest. The effectiveness of this strategy will also depend on the magnitude of the effects associated with the markers. When the effects of the markers across the entire genome are estimated, these effects can be used to select individuals lacking phenotypes (Meuwissen et al., 2001). As such, in the case of disease resistance, there would be fewer requirements for challenge testing of siblings for diseases of economic importance. Recombination results in decay of LD on each generation and the magnitude of this reduction depend on several population features (Porto-Neto et al., 2014). This means that in practice, it is necessary to corroborate the accuracy in estimating the GEBVs and response selection in each generation.
Best linear unbiased prediction (BLUP) combines information from pedigree and phenotypes to predict breeding values (EBVs) of individuals. Molecular markers provide a new source of information, which will give higher accuracy when predicting EBVs. Thus, selection response will be potentially higher in traits in which the accuracy is low, i.e., traits with low heritability or traits that cannot be measured in the selection candidate, such as disease resistance (Sonesson and Meuwissen, 2009; Villanueva et al., 2011; Taylor, 2014). The relative increase in accuracy depends on the amount of variation explained by the markers. It is also possible to combine breeding values for disease resistance and other performance traits into a selection index with specified weightings based on their economic importance for multi-trait genetic improvement.
In livestock species the effects of QTL have been shown to exhibit a moderate leptokurtic gamma distribution, suggesting a small number of loci of large effect and high number of loci of small effect (Hayes and Goddard, 2001), which is likely to be expected in aquaculture species for most economically relevant traits. Therefore, it is expected that more than one marker is necessary to efficiently assist breeding programs using molecular information. The availability of high throughput SNP genotyping platforms will allow the use of these markers in selective breeding for aquaculture species by means of the implementation of GS schemes (Goddard and Hayes, 2009; Sonesson and Meuwissen, 2009; Taylor, 2014). There are currently at least three independent initiatives to generate high density SNP arrays for Atlantic salmon, run by groups from three of the leading producers of this species: Chile, Scotland and Norway. The first of these to be published is that of Houston et al. (2014) whereby approximately 132 K SNP markers were identified and verified in several populations of farmed and wild Atlantic salmon. The Chilean group validated almost 160 K SNP markers from a 200 K SNP platform for Atlantic salmon, genotyping fish representing wild and farmed populations from Europe, North America, and Chile (Yáñez et al., 2014b). Additionally, a 57 K SNP chip is available for rainbow trout (Palti et al., 2014b). Therefore, it can be expected that programs using a high number of markers in population LD will be implemented in the near future. However, it is necessary to determine the economic benefit of using high-density panels, versus the use of low-density panels supported by imputation methodologies and establish a strategy for GS taking into account technical and economic feasibility.
Conclusion
There is increasing information related to the determination of the genetic basis for disease resistance in salmonid species. The rapid development of genomic resources in these species will provide new tools for genetic dissection of these traits. The use of these tools will be of great help in identifying loci involved in the genetic variation of disease resistance. This information will increase our understanding of the underlying biology of resistance to disease. Further, it will be crucial for the implementation of MAS or GS programs that include disease resistance within the breeding objective. These methods will increase the accuracy of selection candidates, thereby improving the selection response. However, the economic feasibility and profitability of the implementation of these new strategies and its comparison with conventional selection schemes must be studied for each particular breeding program in salmonids. It will also be necessary to assess the long-term impact of these strategies on the control of each specific disease, in an epidemiological context.
Conflict of Interest Statement
The authors declare that the research was conducted in the absence of any commercial or financial relationships that could be construed as a potential conflict of interest.
Acknowledgments
This work was partially funded by grants from CORFO (11IEI-12843 and 12PIE-17669), Government of Chile and from Programa U-Inicia, Vicerrectoría de Investigación y Desarrollo, Universidad de Chile. José M. Yáñez would like to thank María E. López and Liane Bassini for their help in revising the manuscript.
References
Aoyagi, K., Dijkstra, J. M., Xia, C., Denda, I., Ototake, M., Hashimoto, K.,et al. (2002). Classical MHC class I genes composed of highly divergent sequence lineages share a single locus in rainbow trout (Oncorhynchus mykiss). J. Immunol. 168, 260–273. doi: 10.4049/jimmunol.168.1.260
Pubmed Abstract | Pubmed Full Text | CrossRef Full Text | Google Scholar
Asche, F., Hansen, H., Tveteras, R., and Tveteras, S. (2010). The salmon disease crisis in Chile. Marine Resour. Econ. 24, 405–411. doi: 10.5950/0738-1360-24.4.405
Pubmed Abstract | Pubmed Full Text | CrossRef Full Text | Google Scholar
Asche, F., and Roll, K. H. (2013). Determinants of inefficiency in Norwegian salmon aquaculture. Aquac. Econ. Manag. 17, 300–321. doi: 10.1080/13657305.2013.812154
Baerwald, M. R., Petersen, J. L., Hedrick, R. P., Schisler, G. J., and May, B. (2010). A major effect quantitative trait locus for whirling disease resistance identified in rainbow trout (Oncorhynchus mykiss). Heredity (Edinb.) 106, 920–926. doi: 10.1038/hdy.2010.137
Pubmed Abstract | Pubmed Full Text | CrossRef Full Text | Google Scholar
Bayne, C. J., Gerwick, L., Fujiki, K., Nakao, M., and Yano, T. (2001). Immune relevant (including acute phase) genes identified in the livers of rainbow trout, Oncorhynchus mykiss, by means of suppression subtractive hybridization. Dev. Comp. Immunol. 25, 205–217. doi: 10.1016/S0145-305X(00)00057-4
Pubmed Abstract | Pubmed Full Text | CrossRef Full Text | Google Scholar
Beacham, T. D., and Evelyn, T. P. T. (1992). Genetic variation in disease resistance and growth and growth of chinook, coho, and chum salmon with respect to vibriosis, furunculosis, and bacterial kidney disease. Trans. Am. Fish Soc. 121, 456–485. doi: 10.1577/1548-8659(1992)121<0456:GVIDRA>2.3.CO;2
Berthelot, C., Brunet, F., Chalopin, D., Juanchich, A., Bernard, M., Noël, B.,et al. (2014). The rainbow trout genome provides novel insights into evolution after whole-genome duplication in vertebrates. Nat. Commun. 5, 3657. doi: 10.1038/ncomms4657
Pubmed Abstract | Pubmed Full Text | CrossRef Full Text | Google Scholar
Biering, E., Madhun, A., Isachsen, C. H., Omdal, L. M., Einen, A. C. B., Garseth, Å. H.,et al. (2012). Annual Report on Health Monitoring of Wild Anadromous Salmonids in Norway. Annual Report 2012, No 6-2013. Bergen: Institute of Marine Research.
Bishop, S. C. (2010). “Disease resistance: genetics,” in Encyclopedia of Animal Science, eds W. G. Pond and A. W. Bell (New York, NY: Marcel Dekker, Inc.) 288–290.
Bishop, S. C., and Woolliams, J. A. (2014). Genomics and disease resistance studies in livestock. Livest. Sci. 166, 190–198. doi: 10.1016/j.livsci.2014.04.034
Bravo, S., Nunez, M., and Silva, M. T. (2013). Efficacy of the treatments used for the control of Caligus rogercresseyi infecting Atlantic salmon, Salmo salar L., in a new fish-farming location in Region XI, Chile.w J. Fish Dis. 36, 221–228. doi: 10.1111/jfd.12023
Pubmed Abstract | Pubmed Full Text | CrossRef Full Text | Google Scholar
Cairney, M., Taggart, J. B., and Høyheim, B. (2000). Characterization of microsatellite and minisatellite loci in Atlantic salmon (Salmo salar L.) and cross-species amplification in other salmonids. Mol. Ecol. 9, 2175–2178. doi: 10.1046/j.1365-294X.2000.105312.x
Pubmed Abstract | Pubmed Full Text | CrossRef Full Text | Google Scholar
Cofre, C., Gonzalez, R., Moya, J., and Vidal, R. (2014). Phenotype gene expression differences between resistant and susceptible salmon families to IPNV. Fish Physiol. Biochem. 40, 887–896. doi: 10.1007/s10695-013-9894-3
Pubmed Abstract | Pubmed Full Text | CrossRef Full Text | Google Scholar
Costello, M. J. (2009). The global economic cost of sea lice to the salmonid farming industry. J. Fish Dis. 32, 115–118. doi: 10.1111/j.1365-2761.2008.01011.x
Pubmed Abstract | Pubmed Full Text | CrossRef Full Text | Google Scholar
Davey, J. W., Cezard, T., Fuentes-Utrilla, P., Eland, C., Gharbi, K., and Blaxter, M. L. (2013). Special features of RAD Sequencing data: implications for genotyping. Mol. Ecol. 22, 3151–3164. doi: 10.1111/mec.12084
Pubmed Abstract | Pubmed Full Text | CrossRef Full Text | Google Scholar
Davidson, W., Koop, B., Jones, S., Iturra, P., Vidal, R., Maass, A.,et al. (2010). Sequencing the genome of the Atlantic salmon (Salmo salar). Genome Biol. 11, 403. doi: 10.1186/gb-2010-11-9-403
Pubmed Abstract | Pubmed Full Text | CrossRef Full Text | Google Scholar
de Koning, D. J., Carlborg, O., and Haley, C. S. (2005). The genetic dissection of immune response using gene-expression studies and genome mapping. Vet. Immunol. Immunopathol. 105, 343–352. doi: 10.1016/j.vetimm.2005.02.007
Pubmed Abstract | Pubmed Full Text | CrossRef Full Text | Google Scholar
Dekkers, J., and Van der Werf, J. (2007). “Strategies, limitations and opportunities for marker-assisted selection in livestock,” in Marker-Assisted Selection – Current Status and Future Perspectives in Crops, Livestock, Forestry and Fish, eds E. P. Guimarães, J. Ruane, B. D. Scherf, A. Sonnino, and J. D. Dargie (Rome: FAO), 167–184.
Doeschl-Wilson, A. B., Bishop, S. C., Kyriazakis, I., and Villanueva, B. (2012). Novel methods for quantifying individual host response to infectious pathogens for genetic analyses. Front. Genet. 3:266. doi: 10.3389/fgene.2012.00266
Pubmed Abstract | Pubmed Full Text | CrossRef Full Text | Google Scholar
Dorson, M., Quillet, E., Hollebecq, M., Torhy, C., and Chevassus, B. (1995). Selection of rainbow trout resistant to viral haemorrhagic septicaemia virus and transmission of resistance by gynogenesis. Vet. Res. 26, 361–368.
Everett, M., Grau, E., and Seeb, J. (2011). Short reads and nonmodel species: exploring the complexities of next-generation sequence assembly and SNP discovery in the absence of a reference genome. Mol. Ecol. Resour. 11, 93–108. doi: 10.1111/j.1755-0998.2010.02969.x
Pubmed Abstract | Pubmed Full Text | CrossRef Full Text | Google Scholar
Everett, M. V., Miller, M. R., and Seeb, J. E. (2012). Meiotic maps of sockeye salmon derived from massively parallel DNA sequencing. BMC Genomics 13:521. doi: 10.1186/1471-2164-13-521
Pubmed Abstract | Pubmed Full Text | CrossRef Full Text | Google Scholar
Ewart, K., Belanger, J., Williams, J., Karakach, T., Penny, S., Tsoi, S.,et al. (2005). Identification of genes differentially expressed in Atlantic salmon (S. salar) in response to infection by Aeromonas salmonicida using cDNA microarray technology. Dev. Comp. Immunol. 29, 333–347. doi: 10.1016/j.dci.2004.08.004
Falconer, D. S., and Mackay, T. F. C. (1996). Introduction to Quantitative Genetics, 4 Edn. Essex: Longman.
Fevolden, S. E., Nordmo, R., Refstie, T., and Røed, K. H. (1993). Disease resistance in Atlantic salmon (Salmo salar) selected for high or low responses to stress. Aquaculture 109, 215–224. doi: 10.1016/0044-8486(93)90164-T
Food and Agriculture Organization of the United Nations [FAO]. (2012). The State of World Fisheries and Aquaculture. Italy: FAO.
Fryer, J., and Hedrick, R. (2003). Piscirickettsia salmonis: a Gram-negative intracellular bacterial pathogen of fish. J. Fish Dis. 26, 251–262. doi: 10.1046/j.1365-2761.2003.00460.x
Garrigan, D., and Hedrick, P. W. (2001). Class I MHC polymorphism and evolution in endangered California Chinook and other Pacific salmon. Immunogenetics 53, 483–489. doi: 10.1007/s002510100352
Pubmed Abstract | Pubmed Full Text | CrossRef Full Text | Google Scholar
Gharbi, K., Gautier, A., Danzmann, R. G., Gharbi, S., Sakamoto, T., Hoyheim, B.,et al. (2006). A linkage map for brown trout (Salmo trutta): chromosome homeologies and comparative genome organization with other salmonid fish. Genetics 172, 2405–2419. doi: 10.1534/genetics.105.048330
Pubmed Abstract | Pubmed Full Text | CrossRef Full Text | Google Scholar
Gilbey, J., Verspoor, E., Mclay, A., and Houlihan, D. (2004). A microsatellite linkage map for Atlantic salmon (Salmo salar). Anim. Genet. 35, 98–105. doi: 10.1111/j.1365-2052.2004.01091.x
Pubmed Abstract | Pubmed Full Text | CrossRef Full Text | Google Scholar
Gjedrem, T. (2000). Genetic improvement of cold-water fish species. Aquac. Res. 31, 25–33. doi: 10.1046/j.1365-2109.2000.00389.x
Gjedrem, T. (2012). Genetic improvement for the development of efficient global aquaculture: a personal opinion review. Aquaculture 344, 12–22. doi: 10.1016/j.aquaculture.2012.03.003
Gjedrem, T., and Gjøen, H. (1995). Genetic variation in susceptibility of Atlantic salmon, Salmo salar L., to furunculosis, BKD and cold water vibriosis. Aquac. Res. 26, 129–134. doi: 10.1111/j.1365-2109.1995.tb00892.x
Gjedrem, T., Salte, R., and Gjøen, H. M. (1991). Genetic variation in susceptibility of Atlantic salmon to furunculosis. Aquaculture 97, 1–6. doi: 10.1016/0044-8486(91)90274-B
Gjerde, B., Evensen,Ø., Bentsen, H. B., and Storset, A. (2009). Genetic (co) variation of vaccine injuries and innate resistance to furunculosis (Aeromonas salmonicida) and infectious salmon anaemia (ISA) in Atlantic salmon (Salmo salar). Aquaculture 287, 52–58. doi: 10.1016/j.aquaculture.2008.10.028
Gjøen, H., and Bentsen, H. (1997). Past, present, and future of genetic improvement in salmon aquaculture. ICES J. Marine Sci. 54, 1009–1014. doi: 10.1016/S1054-3139(97)80005-7
Gjøen, H. M., Refstie, T., Ulla, O., and Gjerde, B. (1997). Genetic correlations between survival of Atlantic salmon in challenge and field tests. Aquaculture 158, 277–288. doi: 10.1016/S0044-8486(97)00203-2
Pubmed Abstract | Pubmed Full Text | CrossRef Full Text | Google Scholar
Glover, K., Aasmundstad, T., Nilsen, F., Storset, A., and Skaala, Ø. (2005). Variation of Atlantic salmon families (Salmo salar L.) in susceptibility to the sea lice Lepeophtheirus salmonis and Caligus elongatus. Aquaculture 245, 19–30. doi: 10.1016/j.aquaculture.2004.11.047
Goddard, M. E., and Hayes, B. J. (2009). Mapping genes for complex traits in domestic animals and their use in breeding programmes. Nat. Rev. Genet. 10, 381–391. doi: 10.1038/nrg2575
Pubmed Abstract | Pubmed Full Text | CrossRef Full Text | Google Scholar
Goddard, M. E., and Meuwissen, T. H. E. (2005). The use of linkage disequilibrium to map quantitative trait loci. Aust. J. Exp. Agric. 45, 837–845.
Gonen, S., Lowe, N. R., Cezard, T., Gharbi, K., Bishop, S. C., and Houston, R. D. (2014). Linkage maps of the Atlantic salmon (Salmo salar) genome derived from RAD sequencing. BMC Genomics 15:166. doi: 10.1186/1471-2164-15-166
Pubmed Abstract | Pubmed Full Text | CrossRef Full Text | Google Scholar
Grimholt, U., Drablos, F., Jorgensen, S. M., Hoyheim, B., and Stet, R. J. (2002). The major histocompatibility class I locus in Atlantic salmon (Salmo salar L.): polymorphism, linkage analysis and protein modelling. Immunogenetics 54, 570–581. doi: 10.1007/s00251-002-0499-8
Pubmed Abstract | Pubmed Full Text | CrossRef Full Text | Google Scholar
Grimholt, U., Getahun, A., Hermsen, T., and Stet, R. J. (2000). The major histocompatibility class II alpha chain in salmonid fishes. Dev. Comp. Immunol. 24, 751–763. doi: 10.1016/S0145-305X(00)00034-3
Grimholt, U., Hordvik, I., Fosse, V. M., Olsaker, I., Endresen, C., and Lie, Ø. (1993). Molecular cloning of major histocompatibility complex class I cDNAs from Atlantic salmon (Salmo salar). Immunogenetics 37, 469–473. doi: 10.1007/BF00222473
Pubmed Abstract | Pubmed Full Text | CrossRef Full Text | Google Scholar
Grimholt, U., Larsen, S., Nordmo, R., Midtlyng, P., Kjoeglum, S., Storset, A.,et al. (2003). MHC polymorphism and disease resistance in Atlantic salmon (Salmo salar); facing pathogens with single expressed major histocompatibility class I and class II loci. Immunogenetics 55, 210–219. doi: 10.1007/s00251-003-0567-8
Pubmed Abstract | Pubmed Full Text | CrossRef Full Text | Google Scholar
Grimholt, U., Olsaker, I., Lindstrøm, C., and Lie, Ø. (1994). A study of variability in the MHC class II β1 and class I α2 domain exons of Atlantic salmon, Salmo salar L. Anim. Genet. 25, 147–153. doi: 10.1111/j.1365-2052.1994.tb00103.x
Guy, D. R., Bishop, S. C., Brotherstone, S., Hamilton, A., Roberts, R. J., Mcandrew, B.,et al. (2006). Analysis of the incidence of infectious pancreatic necrosis mortality in pedigreed Atlantic salmon, Salmo salar L., populations. J. Fish Dis. 29, 637–647. doi: 10.1111/j.1365-2761.2006.00758.x
Pubmed Abstract | Pubmed Full Text | CrossRef Full Text | Google Scholar
Guyomard, R., Boussaha, M., Krieg, F., Hervet, C., and Quillet, E. (2012). A synthetic rainbow trout linkage map provides new insights into the salmonid whole genome duplication and the conservation of synteny among teleosts. BMC Genet. 13:15. doi: 10.1186/1471-2156-13-15
Pubmed Abstract | Pubmed Full Text | CrossRef Full Text | Google Scholar
Guyomard, R., Mauger, S., Tabet-Canale, K., Martineau, S., Genet, C., Krieg, F.,et al. (2006). A type I and type II microsatellite linkage map of rainbow trout (Oncorhynchus mykiss) with presumptive coverage of all chromosome arms. BMC Genomics 7:302. doi: 10.1186/1471-2164-7-302
Pubmed Abstract | Pubmed Full Text | CrossRef Full Text | Google Scholar
Hansen, J. D., Strassburger, P., and Du Pasquier, L. (1996). Conservation of an alpha 2 domain within the teleostean world, mhc class i from the rainbow trout Oncorhynchus mykiss. Dev. Comp. Immunol. 20, 417–425. doi: 10.1016/S0145-305X(96)00030-4
Pubmed Abstract | Pubmed Full Text | CrossRef Full Text | Google Scholar
Hansen, J. D., Strassburger, P., Thorgaard, G. H., Young, W. P., and Du Pasquier, L. (1999). Expression, linkage, and polymorphism of MHC-related genes in rainbow trout, Oncorhynchus mykiss. J. Immunol. 163, 774–786.
Hayes, B., and Goddard, M. E. (2001). The distribution of the effects of genes affecting quantitative traits in livestock. Genet. Select. Evol. 33, 209–230. doi: 10.1186/1297-9686-33-3-209
Pubmed Abstract | Pubmed Full Text | CrossRef Full Text | Google Scholar
Hayes, B., Laerdahl, J., Lien, S., Moen, T., Berg, P., Hindar, K.,et al. (2007). An extensive resource of single nucleotide polymorphism markers associated with Atlantic salmon (Salmo salar) expressed sequences. Aquaculture 265, 82–90. doi: 10.1016/j.aquaculture.2007.01.037
Hayes, B. J., Gjuvsland, A., and Omholt, S. (2006). Power of QTL mapping experiments in commercial Atlantic salmon populations, exploiting linkage and linkage disequilibrium and effect of limited recombination in males. Heredity (Edinb.) 97, 19–26. doi: 10.1038/sj.hdy.6800827
Pubmed Abstract | Pubmed Full Text | CrossRef Full Text | Google Scholar
Henryon, M., Berg, P., Olesen, N. J., Kjær, T. E., Slierendrecht, W. J., Jokumsen, A.,et al. (2005). Selective breeding provides an approach to increase resistance of rainbow trout (Oncorhynchus mykiss) to the diseases, enteric redmouth disease, rainbow trout fry syndrome, and viral haemorrhagic septicaemia. Aquaculture 250, 621–636. doi: 10.1016/j.aquaculture.2004.12.022
Henryon, M., Jokumsen, A., Berg, P., Lund, I., Pedersen, P. B., Olesen, N. J.,et al. (2002). Genetic variation for growth rate, feed conversion efficiency, and disease resistance exists within a farmed population of rainbow trout. Aquaculture 209, 59–76. doi: 10.1016/S0044-8486(01)00729-3
Hiendleder, S., Bauersachs, S., Boulesteix, A., Blum, H., Arnold, G., Frohlich, T.,et al. (2005). Functional genomics: tools for improving farm animal health and welfare. Rev. Sci. Tech. 24, 355. doi: 10.1186/1476-4598-13-88
Pubmed Abstract | Pubmed Full Text | CrossRef Full Text | Google Scholar
Hill, A. V. (1999). Genetics and genomics of infectious disease susceptibility. Br. Med. Bull. 55, 401–213. doi: 10.1258/0007142991902457
Hohenlohe, P. A., Amish, S. J., Catchen, J. M., Allendorf, F. W., and Luikart, G. (2011). Next-generation RAD sequencing identifies thousands of SNPs for assessing hybridization between rainbow and westslope cutthroat trout. Mol. Ecol. Resour. 11(Suppl. 1), 117–122. doi: 10.1111/j.1755-0998.2010.02967.x
Pubmed Abstract | Pubmed Full Text | CrossRef Full Text | Google Scholar
Hollebecq, M.-G., Faivre, B., Bourmaud, C., and Michel, C. (1995). Spontaneous bactericidal and complement activities in serum of rainbow trout (Oncorhynchus mykiss) genetically selected for resistance or susceptibility to furunculosis. Fish Shellfish Immunol. 5, 407–426. doi: 10.1006/fsim.1995.0040
Hordvik, I., Grimholt, U., Fosse, V. M., Lie,Ø., and Endresen, C. (1993). Cloning and sequence analysis of cDNAs encoding the MHC class II β chain in Atlantic salmon (Salmo salar). Immunogenetics 37, 437–441. doi: 10.1007/BF00222467
Houston, R. D., Davey, J. W., Bishop, S. C., Lowe, N. R., Mota-Velasco, J. C., Hamilton, A.,et al. (2012). Characterisation of QTL-linked and genome-wide restriction site-associated DNA (RAD) markers in farmed Atlantic salmon. BMC Genomics 13:244. doi: 10.1186/1471-2164-13-244
Pubmed Abstract | Pubmed Full Text | CrossRef Full Text | Google Scholar
Houston, R. D., Haley, C. S., Hamilton, A., Guy, D. R., Mota-Velasco, J. C., Gheyas, A. A.,et al. (2010). The susceptibility of Atlantic salmon fry to freshwater infectious pancreatic necrosis is largely explained by a major QTL. Heredity (Edinb.) 105, 318–327. doi: 10.1038/hdy.2009.171
Pubmed Abstract | Pubmed Full Text | CrossRef Full Text | Google Scholar
Houston, R. D., Haley, C. S., Hamilton, A., Guy, D. R., Tinch, A. E., Taggart, J. B.,et al. (2008a). Major quantitative trait loci affect resistance to infectious pancreatic necrosis in Atlantic salmon (Salmo salar). Genetics 178, 1109–1115. doi: 10.1534/genetics.107.082974
Pubmed Abstract | Pubmed Full Text | CrossRef Full Text | Google Scholar
Houston, R. D., Gheyas, A., Hamilton, A., Guy, D. R., Tinch, A. E., Taggart, J. B.,et al. (2008b). Detection and confirmation of a major QTL affecting resistance to infectious pancreatic necrosis (IPN) in Atlantic salmon (Salmo salar). Dev. Biol. (Basel) 132, 199–204. doi: 10.1159/000317160
Pubmed Abstract | Pubmed Full Text | CrossRef Full Text | Google Scholar
Houston, R. D., Taggart, J. B., Cézard, T., Bekaert, M., Lowe, N. R., Downing, A.,et al. (2014). Development and validation of a high density SNP genotyping array for Atlantic salmon (Salmo salar). BMC Genomics 15:90. doi: 10.1186/1471-2164-15-90
Pubmed Abstract | Pubmed Full Text | CrossRef Full Text | Google Scholar
Johnson, N. A., Vallejo, R. L., Silverstein, J. T., Welch, T. J., Wiens, G. D., Hallerman, E. M.,et al. (2008). Suggestive association of major histocompatibility IB genetic markers with resistance to bacterial cold water disease in rainbow trout (Oncorhynchus mykiss). Mar. Biotechnol. 10, 429–437. doi: 10.1007/s10126-007-9080-7
Pubmed Abstract | Pubmed Full Text | CrossRef Full Text | Google Scholar
Jones, P. G., Hammell, K. L., Gettinby, G., and Revie, C. W. (2013). Detection of emamectin benzoate tolerance emergence in different life stages of sea lice, Lepeophtheirus salmonis, on farmed Atlantic salmon, Salmo salar L. J. Fish Dis. 36, 209–220. doi: 10.1111/jfd.12022
Pubmed Abstract | Pubmed Full Text | CrossRef Full Text | Google Scholar
Kjøglum, S., Grimholt, U., and Larsen, S. (2005). Non-MHC genetic and tank effects influence disease challenge tests in Atlantic salmon (Salmo salar). Aquaculture 250, 102–109. doi: 10.1016/j.aquaculture.2005.03.021
Kjøglum, S., Henryon, M., Aasmundstad, T., and Korsgaard, I. (2008). Selective breeding can increase resistance of Atlantic salmon to furunculosis, infectious salmon anaemia and infectious pancreatic necrosis. Aquac. Res. 39, 498–505. doi: 10.1111/j.1365-2109.2008.01904.x
Kjøglum, S., Larsen, S., Bakke, H. G., and Grimholt, U. (2006). How specific MHC class I and class II combinations affect disease resistance against infectious salmon anaemia in Atlantic salmon (Salmo salar). Fish Shellfish Immunol. 21, 431–441. doi: 10.1016/j.fsi.2006.02.001
Pubmed Abstract | Pubmed Full Text | CrossRef Full Text | Google Scholar
Kolstad, K., Heuch, P. A., Gjerde, B., Gjedrem, T., and Salte, R. (2005). Genetic variation in resistance of Atlantic salmon (Salmo salar) to the salmon louse Lepeophtheirus salmonis. Aquaculture 247, 145–151. doi: 10.1016/j.aquaculture.2005.02.009
Langefors,Å., Lohm, J., Grahn, M., Andersen, Ø., and Von Schantz, T. (2001). Association between major histocompatibility complex class IIB alleles and resistance to Aeromonas salmonicida in Atlantic salmon. Proc. Biol. Sci. 268, 479–485. doi: 10.1098/rspb.2000.1378
Pubmed Abstract | Pubmed Full Text | CrossRef Full Text | Google Scholar
Langevin, C., Blanco, M., Martin, S. A. M., Jouneau, L., Bernardet, J.-F., Houel, A.,et al. (2012). Transcriptional responses of resistant and susceptible fish clones to the bacterial pathogen Flavobacterium psychrophilum. PLoS ONE 7:e39126. doi: 10.1371/journal.pone.0039126
Pubmed Abstract | Pubmed Full Text | CrossRef Full Text | Google Scholar
Leeds, T. D., Silverstein, J. T., Weber, G. M., Vallejo, R. L., Palti, Y., Rexroad, C. E.,et al. (2010). Response to selection for bacterial cold water disease resistance in rainbow trout. J. Anim. Sci. 88, 1936–1946. doi: 10.2527/jas.2009-2538
Pubmed Abstract | Pubmed Full Text | CrossRef Full Text | Google Scholar
Lhorente, J. P., Gallardo, J. A., Villanueva, B., Araya, A. M., Torrealba, D. A., Toledo, X. E.,et al. (2012). Quantitative genetic basis for resistance to Caligus rogercresseyi sea lice in a breeding population of Atlantic salmon (Salmo salar). Aquaculture 324, 55–59. doi: 10.1016/j.aquaculture.2011.10.046
Lien, S., Gidskehaug, L., Moen, T., Hayes, B. J., Berg, P. R., Davidson, W. S.,et al. (2011). A dense SNP-based linkage map for Atlantic salmon (Salmo salar) reveals extended chromosome homeologies and striking differences in sex-specific recombination patterns. BMC Genomics 12:615. doi: 10.1186/1471-2164-12-615
Pubmed Abstract | Pubmed Full Text | CrossRef Full Text | Google Scholar
Liu, Z., and Cordes, J. (2004). DNA marker technologies and their applications in aquaculture genetics. Aquaculture 238, 1–37. doi: 10.1016/j.aquaculture.2004.05.027
Lohm, J., Grahn, M., Langefors,Å., Andersen, Ø., Storset, A., and Von Schantz, T. (2002). Experimental evidence for major histocompatibility complex–allele–specific resistance to a bacterial infection. Proc. Biol. Sci. 269, 2029–2033. doi: 10.1098/rspb.2002.2114
Pubmed Abstract | Pubmed Full Text | CrossRef Full Text | Google Scholar
Lund, T., Gjedrem, T., Bentsen, H., Eide, D., Larsen, H., and Røed, K. (1995). Genetic variation in immune parameters and associations to survival in Atlantic salmon. J. Fish Biol. 46, 748–758. doi: 10.1111/j.1095-8649.1995.tb01598.x
Martin, S. A., Blaney, S. C., Houlihan, D. F., and Secombes, C. J. (2006). Transcriptome response following administration of a live bacterial vaccine in Atlantic salmon (Salmo salar). Mol. Immunol. 43, 1900–1911. doi: 10.1016/j.molimm.2005.10.007
Pubmed Abstract | Pubmed Full Text | CrossRef Full Text | Google Scholar
McClelland, E. K., and Naish, K. A. (2008). A genetic linkage map for coho salmon (Oncorhynchus kisutch). Anim. Genet. 39, 169–179. doi: 10.1111/j.1365-2052.2008.01699.x
Pubmed Abstract | Pubmed Full Text | CrossRef Full Text | Google Scholar
Meuwissen, T. H. E., Hayes, B., and Goddard, M. (2001). Prediction of total genetic value using genomewide dense marker maps. Genetics 157, 1819–1829.
Meuwissen, T. H., Karlsen, A., Lien, S., Olsaker, I., and Goddard, M. E. (2002). Fine mapping of a quantitative trait locus for twinning rate using combined linkage and linkage disequilibrium mapping. Genetics 161, 373–379.
Miller, K. M., Winton, J. R., Schulze, A. D., Purcell, M. K., and Ming, T. J. (2004). Major histocompatibility complex loci are associated with susceptibility of Atlantic salmon to infectious hematopoietic necrosis virus. Environ. Biol. Fishes 69, 307–316. doi: 10.1023/B:EBFI.0000022874.48341.0f
Miller, K. M., and Withler, R. E. (1996). Sequence analysis of a polymorphic Mhc class II gene in Pacific salmon. Immunogenetics 43, 337–351. doi: 10.1007/BF02199802
Mitchell, S., and Rodger, H. (2011). A review of infectious gill disease in marine salmonid fish. J. Fish Dis. 34, 411–432. doi: 10.1111/j.1365-2761.2011.01251.x
Pubmed Abstract | Pubmed Full Text | CrossRef Full Text | Google Scholar
Moen, T., Baranski, M., Sonesson, A. K., and Kjøglum, S. (2009). Confirmation and fine-mapping of a major QTL for resistance to infectious pancreatic necrosis in Atlantic salmon (Salmo salar): population-level associations between markers and trait. BMC Genomics 10:368. doi: 10.1186/1471-2164-10-368
Pubmed Abstract | Pubmed Full Text | CrossRef Full Text | Google Scholar
Moen, T., Fjalestad, K. T., Munck, H., and Gomez-Raya, L. (2004a). A multistage testing strategy for detection of quantitative trait Loci affecting disease resistance in Atlantic salmon. Genetics 167, 851–858. doi: 10.1534/genetics.103.013227.
Pubmed Abstract | Pubmed Full Text | CrossRef Full Text | Google Scholar
Moen, T., Hoyheim, B., Munck, H., and Gomez-Raya, L. (2004b). A linkage map of Atlantic salmon (Salmo salar) reveals an uncommonly large difference in recombination rate between the sexes. Anim. Genet. 35, 81–92. doi: 10.1111/j.1365-2052.2004.01097.x
Pubmed Abstract | Pubmed Full Text | CrossRef Full Text | Google Scholar
Moen, T., Sonesson, A. K., Hayes, B., Lien, S., Munck, H., and Meuwissen, T. H. (2007). Mapping of a quantitative trait locus for resistance against infectious salmon anaemia in Atlantic salmon (Salmo salar): comparing survival analysis with analysis on affected/resistant data. BMC Genet. 8:53. doi: 10.1186/1471-2156-8-53
Pubmed Abstract | Pubmed Full Text | CrossRef Full Text | Google Scholar
Mustafa, A., and MacKinnon, B. M. (1999). Genetic variation in susceptibility of Atlantic salmon to the sea louse Caligus elongatus Nordmann, 1832. Can. J. Zool. 77, 1332–1335. doi: 10.1139/cjz-77-8-1332
Nichols, K. M., Young, W. P., Danzmann, R. G., Robison, B. D., Rexroad, C., Noakes, M.,et al. (2003). A consolidated linkage map for rainbow trout (Oncorhynchus mykiss). Anim. Genet. 34, 102–115. doi: 10.1046/j.1365-2052.2003.00957.x
Norris, A., Foyle, L., and Ratcliff, J. (2008). Heritability of mortality in response to a natural pancreas disease (SPDV) challenge in Atlantic salmon, Salmo salar L., post-smolts on a West of Ireland sea site. J. Fish Dis. 31, 913–920. doi: 10.1111/j.1365-2761.2008.00982.x
Pubmed Abstract | Pubmed Full Text | CrossRef Full Text | Google Scholar
Ødegård, J., Baranski, M., Gjerde, B., and Gjedrem, T. (2011). Methodology for genetic evaluation of disease resistance in aquaculture species: challenges and future prospects. Aquac. Res. 42, 103-114. doi: 10.1111/j.1365-2109.2010.02669.x.
Ødegård, J., Olesen, I., Gjerde, B., and Klemetsdal, G. (2006). Evaluation of statistical models for genetic analysis of challenge test data on furunculosis resistance in Atlantic salmon (Salmo salar): prediction of field survival. Aquaculture 259, 116–123. doi: 10.1016/j.aquaculture.2006.05.034
Ødegård, J., Olesen, I., Gjerde, B., and Klemetsdal, G. (2007a). Evaluation of statistical models for genetic analysis of challenge-test data on ISA resistance in Atlantic salmon (Salmo salar): prediction of progeny survival. Aquaculture 266, 70–76. doi: 10.1016/j.aquaculture.2007.02.012
Ødegård, J., Olesen, I., Gjerde, B., and Klemetsdal, G. (2007b). Positive genetic correlation between resistance to bacterial (furunculosis) and viral (infectious salmon anaemia) diseases in farmed Atlantic salmon (Salmo salar). Aquaculture 271, 173–177. doi: 10.1016/j.aquaculture.2007.06.006
Olesen, I., Hung, D., and Ødegård, J. (2007). Genetic analysis of survival in challenge tests of furunculosis and ISA in Atlantic salmon. Genetic parameter estimates and model comparisons. Aquaculture 272(Suppl. 1), S297–S298. doi: 10.1016/j.aquaculture.2007.07.155
Ozaki, A., Sakamoto, T., Khoo, S., Nakamura, K., Coimbra, M. R., Akutsu, T.,et al. (2001). Quantitative trait loci (QTLs) associated with resistance/susceptibility to infectious pancreatic necrosis virus (IPNV) in rainbow trout (Oncorhynchus mykiss). Mol. Genet. Genomics 265, 23–31. doi: 10.1007/s004380000392
Pubmed Abstract | Pubmed Full Text | CrossRef Full Text | Google Scholar
Palti, Y., Gao, G., Miller, M. R., Vallejo, R. L., Wheeler, P. A., Quillet, E.,et al. (2014a). A resource of single-nucleotide polymorphisms for rainbow trout generated by restriction-site associated DNA sequencing of doubled haploids. Mol. Ecol. Res. 14, 588–596. doi: 10.1111/1755-0998.12204
Pubmed Abstract | Pubmed Full Text | CrossRef Full Text | Google Scholar
Palti, Y., Gao, G., Liu, S., Kent, M. P., Lien, S., Miller, M. R.,et al. (2014b). The development and characterization of a 57K SNP array for rainbow trout. Mol. Ecol. Res. doi: 10.1111/1755-0998.12337 [Epub ahead of print].
Pubmed Abstract | Pubmed Full Text | CrossRef Full Text | Google Scholar
Palti, Y., Genet, C., Gao, G., Hu, Y., You, F., Boussaha, M.,et al. (2012). A second generation integrated map of the rainbow trout (Oncorhynchus mykiss) genome: analysis of conserved synteny with model fish genomes. Mar. Biotechnol. 14, 343–357. doi: 10.1007/s10126-011-9418-z
Pubmed Abstract | Pubmed Full Text | CrossRef Full Text | Google Scholar
Palti, Y., Genet, C., Luo, M.-C., Charlet, A., Gao, G., Hu, Y.,et al. (2011). A first generation integrated map of the rainbow trout genome. BMC Genomics 12:180. doi: 10.1186/1471-2164-12-180
Pubmed Abstract | Pubmed Full Text | CrossRef Full Text | Google Scholar
Palti, Y., Nichols, K. M., Waller, K. I., Parsons, J. E., and Thorgaard, G. H. (2001). Association between DNA polymorphisms tightly linked to MHC class II genes and IHN virus resistance in backcrosses of rainbow and cutthroat trout. Aquaculture 194, 283–289. doi: 10.1016/S0044-8486(00)00526-3
Palti, Y., Parsons, J. E., and Thorgaard, G. H. (1999). Identification of candidate DNA markers associated with IHN virus resistance in backcrosses of rainbow (Oncorhynchus mykiss) and cutthroat trout (O. clarki). Aquaculture 173, 81–94. doi: 10.1016/S0044-8486(98)00471-2
Perry, G. M. L., Tarte, P., Croisetière, S., Belhumeur, P., and Bernatchez, L. (2004). Genetic variance and covariance for 0+ brook charr (Salvelinus fontinalis) weight and survival time of furunculosis (Aeromonas salmonicida) exposure. Aquaculture 235, 263–271. doi: 10.1016/j.aquaculture.2004.03.002
Phillips, R., Keatley, K., Morasch, M., Ventura, A., Lubieniecki, K., Koop, B.,et al. (2009). Assignment of Atlantic salmon (Salmo salar) linkage groups to specific chromosomes: conservation of large syntenic blocks corresponding to whole chromosome arms in rainbow trout (Oncorhynchus mykiss). BMC Genet. 10:46. doi: 10.1186/1471-2156-10-46.
Pubmed Abstract | Pubmed Full Text | CrossRef Full Text | Google Scholar
Piepho, H.-P., and Koch, G. (2000). Codominant analysis of banding data from a dominant marker system by normal mixtures. Genetics 155, 1459–1468.
Pomp, D., Allan, M. F., and Wesolowski, S. R. (2004). Quantitative genomics: exploring the genetic architecture of complex trait predisposition. J. Anim. Sci. 82, E300–E312.
Porto-Neto, L., Kijas, J., and Reverter, A. (2014). The extent of linkage disequilibrium in beef cattle breeds using high-density SNP genotypes. Genet. Select. Evol. 46, 22. doi: 10.1186/1297-9686-46-22
Pubmed Abstract | Pubmed Full Text | CrossRef Full Text | Google Scholar
Purcell, M. K., Nichols, K. M., Winton, J. R., Kurath, G., Thorgaard, G. H., Wheeler, P.,et al. (2006). Comprehensive gene expression profiling following DNA vaccination of rainbow trout against infectious hematopoietic necrosis virus. Mol. Immunol. 43, 2089–2106. doi: 10.1016/j.molimm.2005.12.005
Pubmed Abstract | Pubmed Full Text | CrossRef Full Text | Google Scholar
Qureshi, S. T., Skamene, E., and Malo, D. (1999). Comparative genomics and host resistance against infectious diseases. Emerging Infect. Dis. 5, 36–47. doi: 10.3201/eid0501.990105
Pubmed Abstract | Pubmed Full Text | CrossRef Full Text | Google Scholar
Råberg, L., Sim, D., and Read, A. F. (2007). Disentangling genetic variation for resistance and tolerance to infectious diseases in animals. Science 318, 812–814. doi: 10.1126/science.1148526
Pubmed Abstract | Pubmed Full Text | CrossRef Full Text | Google Scholar
Rexroad, C. E. III, Palti, Y., Gahr, S. A., and Vallejo, R. L. (2008). A second generation genetic map for rainbow trout (Oncorhynchus mykiss). BMC Genet. 9:74. doi: 10.1186/1471-2156-9-74
Pubmed Abstract | Pubmed Full Text | CrossRef Full Text | Google Scholar
Rise, M. L., Jones, S. R. M., Brown, G. D., Von Schalburg, K. R., Davidson, W. S., and Koop, B. F. (2004a). Microarray analyses identify molecular biomarkers of Atlantic salmon macrophage and hematopoietic kidney response to Piscirickettsia salmonis infection. Physiol. Genomics 15, 21–35. doi: 10.1152/physiolgenomics.00036.2004
Pubmed Abstract | Pubmed Full Text | CrossRef Full Text | Google Scholar
Rise, M. L., Von Schalburg, K. R., Brown, G. D., Mawer, M. A., Devlin, R. H., Kuipers, N.,et al. (2004b). Development and application of a salmonid EST database and cDNA microarray: data mining and interspecific hybridization characteristics. Genome Res. 14, 478–490. doi: 10.1101/gr.1687304
Pubmed Abstract | Pubmed Full Text | CrossRef Full Text | Google Scholar
Roberts, R. J., and Pearson, M. D. (2005). Infectious pancreatic necrosis in Atlantic salmon, Salmo salar L. J. Fish Dis. 28, 383–390. doi: 10.1111/j.1365-2761.2005.00642.x
Pubmed Abstract | Pubmed Full Text | CrossRef Full Text | Google Scholar
Rodriguez, M. F., Lapatra, S., Williams, S., Famula, T., and May, B. (2004). Genetic markers associated with resistance to infectious hematopoietic necrosis in rainbow and steelhead trout (Oncorhynchus mykiss) backcrosses. Aquaculture 241, 93–115. doi: 10.1016/j.aquaculture.2004.08.003
Røed, K. H., Fjalestad, K. T., and Strømsheim, A. (1993). Genetic variation in lysozyme activity and spontaneous haemolytic activity in Atlantic salmon (Salmo salar). Aquaculture 114, 19–31. doi: 10.1016/0044-8486(93)90247-V
Rothschild, M. F., and Soller, M. (1997). Candidate gene analysis to detect traits of economic importance in domestic livestock. Probe 8, 13–22.
Rozas, M., and Enríquez, R. (2014). Piscirickettsiosis and Piscirickettsia salmonis in fish: a review. J. Fish Dis. 37, 163–188. doi: 10.1111/jfd.12211
Pubmed Abstract | Pubmed Full Text | CrossRef Full Text | Google Scholar
Ruane, N. M., and Jones, S. R. M. (2013). “Amoebic Gill Disease (AGD) of farmed Atlantic salmon (Salmo salar L.),” in Proceedings of the ICES Identification Leaflets for Diseases and Parasites of Fish and Shellfish. Leaflet No. 60 (Copenhagen: International Council for the Exploration), 6.
Sakamoto, T., Danzmann, R. G., Gharbi, K., Howard, P., Ozaki, A., Khoo, S. K.,et al. (2000). A microsatellite linkage map of rainbow trout (Oncorhynchus mykiss) characterized by large sex-specific differences in recombination rates. Genetics 155, 1331–1345.
Salem, M., Vallejo, R. L., Leeds, T. D., Palti, Y., Liu, S., Sabbagh, A.,et al. (2012). RNA-Seq identifies SNP markers for growth traits in rainbow trout. PLoS ONE 7:e36264. doi: 10.1371/journal.pone.0036264
Pubmed Abstract | Pubmed Full Text | CrossRef Full Text | Google Scholar
Salte, R., Bentsen, H. B., Moen, T., Tripathy, S., Bakke, T. A., Ødegård, J.,et al. (2009). Prospects for a genetic management strategy to control Gyrodactylus salaris infection in wild Atlantic salmon (Salmo salar) stocks. Can. J. Fish. Aquat. Sci. 67, 121–129. doi: 10.1139/F09-168
Salte, R., Gjøen, H. M., Norberg, K., and Gjedrem, T. (1993). Plasma protein levels as potential marker traits for resistance to furunculosis. J. Fish Dis. 16, 561–568. doi: 10.1111/j.1365-2761.1993.tb00892.x
Sanchez, C., Smith, T., Wiedmann, R., Vallejo, R., Salem, M., Yao, J.,et al. (2009). Single nucleotide polymorphism discovery in rainbow trout by deep sequencing of a reduced representation library. BMC Genomics 10:559. doi: 10.1186/1471-2164-10-559
Pubmed Abstract | Pubmed Full Text | CrossRef Full Text | Google Scholar
Silverstein, J. T., Vallejo, R. L., Palti, Y., Leeds, T. D., Rexroad, C. E., Welch, T. J.,et al. (2009). Rainbow trout resistance to bacterial cold-water disease is moderately heritable and is not adversely correlated with growth. J. Anim. Sci. 87, 860–867. doi: 10.2527/jas.2008-1157
Pubmed Abstract | Pubmed Full Text | CrossRef Full Text | Google Scholar
Shum, B., Mason, P., Magor, K., Flodin, L., Stet, R., and Parham, P. (2002). Structures of two major histocompatibility complex class I genes of the rainbow trout (Oncorhynchus mykiss). Immunogenetics 54, 193–199. doi: 10.1007/s00251-002-0450-z
Pubmed Abstract | Pubmed Full Text | CrossRef Full Text | Google Scholar
Shum, B. P., Rajalingam, R., Magor, K. E., Azumi, K., Carr, W. H., Dixon, B.,et al. (1999). A divergent non-classical class I gene conserved in salmonids. Immunogenetics 49, 479–490. doi: 10.1007/s002510050524
Pubmed Abstract | Pubmed Full Text | CrossRef Full Text | Google Scholar
Sonesson, A., and Meuwissen, T. (2009). Testing strategies for genomic selection in aquaculture breeding programs. Genet. Select. Evol. 41, 37. doi: 10.1186/1297-9686-41-37
Pubmed Abstract | Pubmed Full Text | CrossRef Full Text | Google Scholar
Stear, M. J., Bishop, S. C., Mallard, B. A., and Raadsma, H. (2001). The sustainability, feasibility and desirability of breeding livestock for disease resistance. Res. Vet. Sci. 71, 1–7. doi: 10.1053/rvsc.2001.0496
Pubmed Abstract | Pubmed Full Text | CrossRef Full Text | Google Scholar
Stet, R., De Vries, B., Mudde, K., Hermsen, T., Van Heerwaarden, J., Shum, B.,et al. (2002). Unique haplotypes of co-segregating major histocompatibility class II A and class II B alleles in Atlantic salmon (Salmo salar) give rise to diverse class II genotypes. Immunogenetics 54, 320–331. doi: 10.1007/s00251-002-0477-1
Pubmed Abstract | Pubmed Full Text | CrossRef Full Text | Google Scholar
Sutherland, B., Koczka, K., Yasuike, M., Jantzen, S., Yazawa, R., Koop, B.,et al. (2014). Comparative transcriptomics of Atlantic Salmo salar, chum Oncorhynchus keta and pink salmon O. gorbuscha during infections with salmon lice Lepeophtheirus salmonis. BMC Genomics 15:200. doi: 10.1186/1471-2164-15-200
Pubmed Abstract | Pubmed Full Text | CrossRef Full Text | Google Scholar
Taylor, J. F. (2014). Implementation and accuracy of genomic selection. Aquaculture 420–421(Suppl. 1), S8–S14. doi: 10.1016/j.aquaculture.2013.02.017
Taylor, R. S., Kube, P. D., Muller, W. J., and Elliott, N. G. (2009). Genetic variation of gross gill pathology and survival of Atlantic salmon (Salmo salar L.) during natural amoebic gill disease challenge. Aquaculture 294, 172–179. doi: 10.1016/j.aquaculture.2009.06.007
Thorgaard, G. H., Bailey, G. S., Williams, D., Buhler, D. R., Kaattari, S. L., Ristow, S. S.,et al. (2002). Status and opportunities for genomics research with rainbow trout. Comp. Biochem. Physiol. B Biochem. Mol. Biol. 133, 609–646. doi: 10.1016/S1096-4959(02)00167-7
Pubmed Abstract | Pubmed Full Text | CrossRef Full Text | Google Scholar
Tsoi, S. M., Cale, J., Bird, I., Ewart, V., Brown, L., and Douglas, S. (2003). Use of human cDNA microarrays for identification of differentially expressed genes in Atlantic salmon liver during Aeromonas salmonicida infection. Mar. Biotechnol. 5, 545–554. doi: 10.1007/s10126-002-0112-z
Pubmed Abstract | Pubmed Full Text | CrossRef Full Text | Google Scholar
Tsoi, S. C. M., Ewart, K. V., Penny, S., Melville, K., Liebscher, R. S., Brown, L. L.,et al. (2004). Identification of immune-relevant genes from Atlantic salmon using suppression subtractive hybridization. Mar. Biotechnol. 6, 199–214. doi: 10.1007/s10126-002-0101-2
Pubmed Abstract | Pubmed Full Text | CrossRef Full Text | Google Scholar
Vallejo, R., Palti, Y., Liu, S., Evenhuis, J., Gao, G., Rexroad, C. III,et al. (2014a). Detection of QTL in rainbow trout affecting survival when challenged with Flavobacterium psychrophilum. Mar. Biotechnol. 16, 349–360. doi: 10.1007/s10126-013-9553-9
Pubmed Abstract | Pubmed Full Text | CrossRef Full Text | Google Scholar
Vallejo, R. L., Palti, Y., Liu, S., Marancik, D. P., and Wiens, G. D. (2014b). Validation of linked QTL for bacterial cold water disease resistance and spleen size on rainbow trout chromosome Omy19. Aquaculture 432, 139–143. doi: 10.1016/j.aquaculture.2014.05.003
Vallejo, R. L., Wiens, G. D., Rexroad, C. E. III, Welch, T. J., Evenhuis, J. P., Leeds, T. D.,et al. (2010). Evidence of major genes affecting resistance to bacterial cold water disease in rainbow trout using Bayesian methods of segregation analysis. J. Anim. Sci. 88, 3814–3832. doi: 10.2527/jas.2010-2951
Pubmed Abstract | Pubmed Full Text | CrossRef Full Text | Google Scholar
Verrier, E. R., Dorson, M., Mauger, S., Torhy, C., Ciobotaru, C., Hervet, C.,et al. (2013a). Resistance to a Rhabdovirus (VHSV) in rainbow trout: identification of a major QTL related to innate mechanisms. PLoS ONE 8:e55302. doi: 10.1371/journal.pone.0055302
Pubmed Abstract | Pubmed Full Text | CrossRef Full Text | Google Scholar
Verrier, E. R., Ehanno, A., Biacchesi, S., Le Guillou, S., Dechamp, N., Boudinot, P.,et al. (2013b). Lack of correlation between the resistances to two rhabdovirus infections in rainbow trout. Fish Shellfish Immunol. 35, 9–17. doi: 10.1016/j.fsi.2013.03.369
Pubmed Abstract | Pubmed Full Text | CrossRef Full Text | Google Scholar
Villanueva, B., Fernández, J., García-Cortés, L. A., Varona, L., Daetwyler, H. D., and Toro, M. A. (2011). Accuracy of genome-wide evaluation for disease resistance in aquaculture breeding programs. J. Anim. Sci. 89, 3433–3442. doi: 10.2527/jas.2010-3814
Pubmed Abstract | Pubmed Full Text | CrossRef Full Text | Google Scholar
von Schalburg, K., Rise, M., Cooper, G., Brown, G., Gibbs, A. R., Nelson, C.,et al. (2005). Fish and chips: various methodologies demonstrate utility of a 16,006-gene salmonid microarray. BMC Genomics 6:126. doi: 10.1186/1471-2164-6-126
Pubmed Abstract | Pubmed Full Text | CrossRef Full Text | Google Scholar
Walsh, B., and Henderson, D. (2004). Microarrays and beyond: what potential do current and future genomics tools have for breeders? J. Animal Sci. 82, E292–E299.
Weber, G. M., Vallejo, R. L., Lankford, S. E., Silverstein, J. T., and Welch, T. J. (2008). Cortisol response to a crowding stress: heritability and association with disease resistance to Yersinia ruckeri in rainbow trout. N. Am. J. Aquac. 70, 425–433. doi: 10.1577/A07-059.1
Weber, G. M., Wiens, G. D., Welch, T. J., Hostuttler, M. A., and Leeds, T. D. (2013). Comparison of disease resistance between diploid, induced-triploid, and intercross-triploid rainbow trout including trout selected for resistance to Flavobacterium psychrophilum. Aquaculture 410–411, 66–71. doi: 10.1016/j.aquaculture.2013.06.014
Wetten, M., Aasmundstad, T., Kjøglum, S., and Storset, A. (2007). Genetic analysis of resistance to infectious pancreatic necrosis in Atlantic salmon (Salmo salar L.). Aquaculture 272, 111–117. doi: 10.1016/j.aquaculture.2007.08.046
Wiens, G. D., Lapatra, S. E., Welch, T. J., Evenhuis, J. P., Rexroad, C. E. III, and Leeds, T. D. (2013a). On-farm performance of rainbow trout (Oncorhynchus mykiss) selectively bred for resistance to bacterial cold water disease: effect of rearing environment on survival phenotype. Aquaculture 388–391, 128–136. doi: 10.1016/j.aquaculture.2013.01.018
Wiens, G. D., Vallejo, R. L., Leeds, T. D., Palti, Y., Hadidi, S., Liu, S.,et al. (2013b). Assessment of genetic correlation between bacterial cold water disease resistance and spleen index in a domesticated population of rainbow trout: identification of QTL on chromosome Omy19. PLoS ONE 8: e75749. doi: 10.1371/journal.pone.0075749
Pubmed Abstract | Pubmed Full Text | CrossRef Full Text | Google Scholar
Wientjes, Y. C. J., Veerkamp, R. F., and Calus, M. P. L. (2013). The effect of linkage disequilibrium and family relationships on the reliability of genomic prediction. Genetics 193, 621–631. doi: 10.1534/genetics.112.146290
Pubmed Abstract | Pubmed Full Text | CrossRef Full Text | Google Scholar
Withler, R. E., and Evelyn, T. P. T. (1990). Genetic variation in resistance to bacterial kidney disease within and between two strains of coho salmon from British Columbia. Trans. Am. Fish. Soc. 119, 1003–1009. doi: 10.1577/1548-8659(1990)119<1003:GVIRTB>2.3.CO;2
Woram, R. A., Mcgowan, C., Stout, J. A., Gharbi, K., Ferguson, M. M., Hoyheim, B.,et al. (2004). A genetic linkage map for Arctic charr (Salvelinus alpinus): evidence for higher recombination rates and segregation distortion in hybrid versus pure strain mapping parents. Genome 47, 304–315. doi: 10.1139/g03-127
Pubmed Abstract | Pubmed Full Text | CrossRef Full Text | Google Scholar
Yáñez, J. M., Bangera, R., Lhorente, J. P., Oyarzún, M., and Neira, R. (2013). Quantitative genetic variation of resistance against Piscirickettsia salmonis in Atlantic salmon (Salmo salar). Aquaculture 414–415, 155–159. doi: 10.1016/j.aquaculture.2013.08.009
Yáñez, J. M., Lhorente, J. P., Bassini, L. N., Oyarzún, M., Neira, R., and Newman, S. (2014a). Genetic co-variation between resistance against both Caligus rogercresseyi and Piscirickettsia salmonis, and body weight in Atlantic salmon (Salmo salar). Aquaculture 433, 295–298. doi: 10.1016/j.aquaculture.2014.06.026
Yáñez, J. M., Naswa, S., López, M. E., Bassini, L., Cabrejos, M. E., Gilbey, J.,et al. (2014b). “Development of a 200K SNP array for Atlantic Salmon: exploiting across continents genetic variation,” in Proceedings of the 10th World Congress on Genetics Applied to Livestock Production, Vancouver.
Yáñez, J. M., and Martínez, V. (2010). Factores genéticos que inciden en la resistencia a enfermedades infecciosas en salmónidos y su aplicación en programas de mejoramiento. Arch. Med. Vet. 42, 1–13.
Young, W. P., Wheeler, P. A., Coryell, V. H., and Keim, P. (1998). A detailed linkage map of rainbow trout produced using doubled haploids. Genetics 148, 839–850.
Zhang, Z., Niu, C., Storset, A., Bøgwald, J., and Dalmo, R. A. (2011). Comparison of Aeromonas salmonicida resistant and susceptible salmon families: a high immune response is beneficial for the survival against Aeromonas salmonicida challenge. Fish Shellfish Immunol. 31, 1–9. doi: 10.1016/j.fsi.2010.12.019
Pubmed Abstract | Pubmed Full Text | CrossRef Full Text | Google Scholar
Keywords: salmon, disease resistance, breeding programs, QTL, genomic selection
Citation: Yáñez JM, Houston RD and Newman S (2014) Genetics and genomics of disease resistance in salmonid species. Front. Genet. 5:415. doi: 10.3389/fgene.2014.00415
Received: 26 September 2014; Accepted: 06 November 2014;
Published online: 26 November 2014.
Edited by:
Peng Xu, Chinese Academy of Fishery Sciences, ChinaReviewed by:
Zhi-Liang Hu, Iowa State University, USAYniv Palti, United States Department of Agriculture, USA
Copyright © 2014 Yáñez, Houston and Newman. This is an open-access article distributed under the terms of the Creative Commons Attribution License (CC BY). The use, distribution or reproduction in other forums is permitted, provided the original author(s) or licensor are credited and that the original publication in this journal is cited, in accordance with accepted academic practice. No use, distribution or reproduction is permitted which does not comply with these terms.
*Correspondence: José M. Yáñez, Faculty of Veterinary and Animal Sciences, University of Chile, Avenue Santa Rosa 11735, P.O. Box 8820808, La Pintana, Santiago, Chile e-mail: jmayanez@uchile.cl