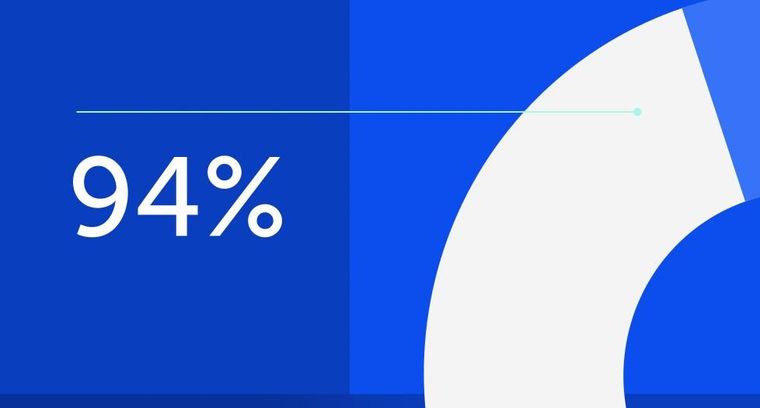
94% of researchers rate our articles as excellent or good
Learn more about the work of our research integrity team to safeguard the quality of each article we publish.
Find out more
REVIEW article
Front. Genet., 30 September 2014
Sec. Systems Biology Archive
Volume 5 - 2014 | https://doi.org/10.3389/fgene.2014.00315
This article is part of the Research TopicProtein Phosphorylation in Health and DiseaseView all 13 articles
Phosphate plays a chemically unique role in shaping cellular signaling of all current living systems, especially eukaryotes. Protein phosphorylation has been studied at several levels, from the near-site context, both in sequence and structure, to the crowded cellular environment, and ultimately to the systems-level perspective. Despite the tremendous advances in mass spectrometry and efforts dedicated to the development of ad hoc highly sophisticated methods, phosphorylation site inference and associated kinase identification are still unresolved problems in kinome biology. The sequence and structure of the substrate near-site context are not sufficient alone to model the in vivo phosphorylation rules, and they should be integrated with orthogonal information in all possible applications. Here we provide an overview of the different contexts that contribute to protein phosphorylation, discussing their potential impact in phosphorylation site annotation and in predicting kinase-substrate specificity.
Phosphorylation, the enzymatic reaction resulting in the addition of a phosphate group to several types of residues, which in eukaryotes are mainly serines, threonines, or tyrosines, generates de facto a new side chain whose physico-chemical properties are different from those of the unmodified residues. This mechanism of Post-Translational Modification (PTM) is strikingly common throughout evolution and in particular for eukaryotes where it is involved in a myriad of cellular processes (Manning et al., 2002a,b, 2008, 2011; Caenepeel et al., 2004; Bradham et al., 2006).
The chemical properties of phosphate make this group a perfect candidate for protein modification, and allow its broad use as a molecular switch within the cell (Hunter, 2012). Indeed the hydrolytic stability of phosphate esters (for instance phosphoserine, phosphotyrosine, phosphothreonine, etc.) in aqueous solutions at pH7 allows the cell to minimize the noise in signal transduction due to non-enzymatically catalyzed hydrolysations. In addition, phosphate monoesters act as sensors, as their electric charge can be influenced by the chemical environment. Lastly, phosphate is a largely available molecule, as it is abundant on Earth and particularly within the cell, where it is included in a fundamental energy storage molecule, i.e., ATP. Differently from other types of PTMs, only one group can be enzymatically added to one residue, underlining the peculiar binary nature of this protein modification. The modified residue can undergo inter- or intra-molecular interactions, causing changes to the protein structure or interfering with its function, probably the most famous and complex example being the allosteric regulation of glycogen phosphorylase (Barford et al., 1991). Additional mechanisms for phosphorylation-mediated modulation have also been reported, such as for instance the inhibition of a binding site (Hurley et al., 1990). A beautiful electrostatic-based tuning of protein function mediated by phosphorylation has been described in yeast cell-cycle regulation, where the membrane localization of the MAPKs scaffold protein Ste5 is disrupted by phosphorylation of a cluster of sites flanking a basic membrane binding motif (Strickfaden et al., 2007).
However, the reason for the success of this type of PTM during evolution, at least in eukaryotes, has to be found largely in its ability to be edited and recognized selectively by specific protein domains, thus providing an efficient tool for transient molecular recognition in the context of signal transduction networks (Lim and Pawson, 2010).
With PTM-based proteomics, phosphorylation sites, as well as other PTMs, are identified and stored in large-scale datasets (Olsen and Mann, 2013). As a consequence of this explosion of data, there is great demand for functional annotation studies that largely exceeds what current technology offers. Furthermore, some observations question the functionality of a substantial fraction of these sites (Landry et al., 2009; Moses and Landry, 2010; Levy et al., 2012; Tan and Bader, 2012).
Given the difficulties in the experimental annotation of the kinase responsible for the phosphorylation, many attempts have been made to computationally model cellular signaling events. Some of the published reviews examine the field of kinase specificity from a more biological perspective, discussing the protein kinase specificity rules in sequence and in structure, while some others compare the different tools, and the techniques used to model kinase-substrate interaction and in general those used to build phosphorylation site predictors (Zhu et al., 2005; Ubersax and Ferrell, 2007; Miller and Blom, 2009; Xue et al., 2010; Trost and Kusalik, 2011; Via et al., 2011). Here we will focus on kinase-substrate interaction at the kinase domain and the substrate-peptide level, and then we will summarize the contextual information that could help to better understand the molecular determinants of kinase specificity, contributing also to boost the performances of phosphorylation site predictors.
While recent advancements in phosphoproteomics allow the identification of phosphosites from entire proteomes with ever increasing reliability and higher coverage, no high-throughput method is able to pinpoint which kinases are responsible for phosphorylating which protein substrates. Therefore, in a high-throughput context, only in silico approaches can effectively help in reconstructing molecular signaling circuits.
All the methods can be grouped according to different criteria, but arguably the main differences are between motif- or PSSM-based and machine learning-based methods and in the use of evolutionary information. We select seven major aspects, as exemplars of different methodologies that have been developed, namely: motif-based identification of phosphorylation sites, structural information integration, integration of phosphorylation site structural context, phospho-clusters modeling, integration of Protein-Protein Interaction Network (PPIN) information and multi-organisms prediction. For a complete list of currently available methods, see Table 1.
The first method to predict the specific kinases that are responsible for the phosphorylations is Scansite (Yaffe et al., 2001), developed by Yaffe and colleagues, using Position Specific Scoring Matrices (PSSMs) for 62 different kinase phosphorylation motifs. Following an extensive analysis of the PKA motifs, PkaPS (Neuberger et al., 2007) was developed, exclusively suited for the prediction of protein kinase A-specific phosphorylation sites. Taking advantage of the structural information, Kobe and his collaborators developed PrediKin (Brinkworth et al., 2003; Ellis and Kobe, 2011), which is based on the analysis of the contact positions between kinases and substrates in proteins of known structure. The authors were able to associate the identification of specific kinase residues with a corresponding preference in the sequence of the substrate. PrediKin outperformed other three predictors in the DREAM4 challenge, whose goal was to predict peptide recognition domain specificity in protein kinases. In another work the information about the 3d-context of phosphorylation sites has been directly integrated in kinase-specific predictions, defining 3d-signatures motifs, even if the improvement with respect to sequence information is small (Durek et al., 2009). Conservation-based methods for predicting kinase-substrates usually assume that phosphorylation sites should be positionally conserved in Multiple Sequence Alignments (MSA) of orthologs (Budovskaya et al., 2005; Gnad et al., 2011). However, it was observed that phospho-motifs may also be found in different positions of the same local regions of orthologous proteins (Moses et al., 2007). In these cases only the local density of phosphorylation sites, but not their exact position, is conserved across orthologs. Lai et al. designed a method, ConDens, which computes the probability of observing a number of matches to a kinase motif in a MSA, under a null evolutionary model (Lai et al., 2012).
The most complete and updated collection of kinase classifiers is NetPhorest (Miller et al., 2008), currently covering 222 kinases and other fundamental signaling domains(Horn et al., 2014). Another milestone in the classification of the kinases responsible for the phosphorylations is NetworKIN (Linding et al., 2007, 2008; Horn et al., 2014), which combines the NetPhorest score with a score that considers the network context of kinases and phosphoproteins, derived from STRING (Franceschini et al., 2013) and based on genomic context, primary experimental evidence, manually curated pathway databases, and automatic literature mining.
Thanks to recent genome sequencing initiatives and phosphoproteomic efforts in several eukaryotes, organism-specific predictors have been developed (Ingrell et al., 2007; Miller et al., 2009; Gao et al., 2010). These methods aim at increasing the prediction accuracy by training on phosphopeptides derived from single organisms. The rationale for these organism-based approaches is that phosphopeptides observed in mass spectrometry experiments performed in these organisms should better represent kinome-specific phosphorylation motifs preferences (Palmeri et al., 2011).
The choice of the predictor is dramatically dependent on user needs, in terms of sensitivity and tolerance to false positives. Some predictors offer to set specific thresholds for specificity and sensitivity (Gao et al., 2010; Xue et al., 2010). Motif-based methods, depending on the motif length and distribution in the proteome, are likely to produce false positives, which can be pruned out by adding more contextual or evolutionary information. Currently, to the best of our knowledge, there is no method that takes advantage of all the aspects here reviewed. Performances will greatly vary from kinase family to family. From Src family to CDK, there are several families whose members share the same motif, and only by deploying contextual information it is possible to distinguish between those members.
Different predictors are often benchmarked using different datasets, at different redundancy levels, with different criteria, and reporting different performance measures (Table 1). Therefore, it is quite impractical to rank all the available predictors precisely, only considering the reported accuracies, and establishing the state-of-the-art is unfeasible. Initiatives, like predictors competitions such as DREAM, could be valuable opportunities to set the standards and offer more reliable evaluations.
Given the relatively high frequency of Ser, Thr and Tyr residues in proteomes (in human 8.5, 5.1, and 2.5% respectively), biological systems have evolved efficient strategies to increase the signal to noise ratio and more importantly to minimize those off target phosphorylations leading to detrimental consequences.
The mechanisms of kinase-substrate specificity can be explored at several levels. A major separation is usually operated between peptide specificity and recruitment. Peptide specificity arises from the interactions between the catalytic kinase domain and the substrate peptide, while recruitment is based on interactions between kinase and substrate that do not involve surfaces localized at the catalytic center. During the phosphorylation reaction, the substrate is located together with the ATP in the structural region between the two kinase domain lobes, so that the gamma phosphate of the ATP can be transferred to the substrate site. The binding site differs between Ser/Thr and Tyr kinases, allowing the enzymes to discriminate between the three residues. In general each kinase shows a preference for one of these residues. Not only the site, but also its surrounding sequence provides information that is used by kinases to recognize their target sites (Figure 1A). The geometrical and electrostatic properties of the substrate binding sites across the kinases have a substantial impact on substrate specificity. Also different kinases show different electrostatic distributions over their entire surfaces that can influence substrate binding.
Figure 1. Specificity levels in Protein Phosphorylation. (A) Peptide specificity in a tyrosine kinase, Insulin Receptor (IR), a proline-directed kinase, Cyclin-dependent kinase 2 (CDK2), and a serine threonine kinase, cAMP-dependent protein kinase catalytic subunit alpha (PKA). Peptide preferences for each kinase are represented as sequence logos (top). The binding pockets of the three kinases have been visualized with UCSF Chimera, and the surfaces colored according to their electrostatic potential: red, positive; blue, negative; white, neutral (bottom). The structures from left to right show IR in complex with a peptide (pdb 1IR3), CDK2 in complex with a substrate peptide and cyclin A (pdb 1QMZ), which contributes to peptide specificity with a negative charged surface shown in the upper right of the figure, and PKA in complex with a peptide inhibitor (pdb 3FJQ). (B) Substrate recruitment. The kinase-substrate complexes concentration can be locally increased with docking motifs, protein interaction domains, and scaffold proteins. As an example of a docking motif, MAPK p38 bound to the docking site on its nuclear substrate MEF2A is shown on the left, colored in purple (pdb 1LEW). The protein interaction domains SH3 and SH2 domains in Src are fundamental for Src activation (inactive Src: pdb 2SRC), as shown in the cartoon in the middle (Xu et al., 1999). MAPK Fus3 in complex with a Ste5 peptide (pdb 2F49) is shown on the right.
Usually, screenings for kinase peptide specificity are performed with Oriented Peptide Libraries (OPL) (Hutti et al., 2004). This approach revolutionized the determination of kinase specificity, using a mix of solution-phase and solid-support strategies, making kinase specificity screenings both scalable and accurate (Yaffe, 2004). It consists in the quantification of the phosphorylation frequency in degenerate peptide libraries, composed of peptides with a fixed central phosphoacceptor residue and a fixed amino acid in any one of the positions flanking the phosphoacceptor, while the remaining positions are usually drawn from a uniform amino acids distribution. The phosphorylation reaction is performed incubating the kinase with radio-labeled ATP in solution-phase, and after, thanks to a C-terminal biotin tag that is present in all libraries, the peptides are fixed to avidin-coated membranes (Songyang et al., 1994; Hutti et al., 2004; Turk, 2008). The kinase preferences for certain amino acids in fixed peptide positions can then be encoded in consensus sequences, in Position Specific Scoring Matrices or more complex classifiers (Miller et al., 2008). From these data, it emerges that peptide specificities of distinct protein kinases are highly variable (Ubersax and Ferrell, 2007; Turk, 2008).
It is generally assumed that the specificity between kinases and substrates is mostly driven by the substrate-binding pocket residues (Ellis and Kobe, 2011), even if also residues localized far from the kinase binding cleft may contribute to shape the peptide specificity.
Durek and collegues attempted to characterize 3d-signature phosphorylation site motifs and evaluated their contribution to phosphorylation site prediction performance (Durek et al., 2009). They studied the spatial distribution of amino acids from 2 to 10 Angstrom around each phosphosite, and defined family-specific 3d-profiles. They reported a modest improvement in predicting the kinase families that phosphorylate serine phosphosites, due to the inclusion of structural information. Despite the small discriminatory power of 3d motifs, structural information, like disorder and secondary structure predictions can more efficiently be deployed to improve phosphorylation site predictors performances (Iakoucheva et al., 2004; Durek et al., 2009).
In 2012 Joughin et al. explored the inter-positional dependence on substrates of ATM/ATR, Cdk1/Cyclin B and CK2 kinases (Joughin et al., 2012). They found only a few significant substrate sequence position pairs that show deviations from position-wise independence. They also tested the ability of first and second order models to correctly separate between the true kinase substrates and mock substrates. Firstly they just used shuffled negative controls (i.e., they shuffled the substrate peptide positions, drawing from the distribution of the true substrates in each position), and they uncovered that mock substrates were similar in quality to the true ones. Then, by using proteomically derived mock substrates, they uncovered that second order were either equal to first order models, or due to over-fitting in training, even worse. Therefore, they concluded that higher-order interdependences in peptides do not seem to give a significant contribution to predictive performances.
This work has interesting implications for the evolution of signaling networks. There are several examples in the literature showing that the molecular recognition of substrates and phosphopeptide-binding domains is subjected to inter-positional dependences. If also other kinases not included in this study turn out not to show marked second or higher-order preferences on substrate sequences, this would mean that there is a fundamental difference in the way these two components behave in the evolution of signaling networks. As the authors point out, the fitness landscape might look smooth for kinase peptide specificity, and the fitness of the kinase substrate could be boosted after sequential mutations on the peptide, while the fitness landscape for phosphopeptide-binding domain substrate may contain energetic barriers. From a phosphosite predictor perspective, this means that greater efforts should be centered around the development of context-dependent methodologies.
Although modeling kinase-peptide specificity is fundamental for understanding kinases preferences for their substrates, to study signal propagation in biological systems all phosphorylation events need also to be placed in time and space. As Alexander and colleagues clearly demonstrated in a paper published in 2011, the in vivo specificity of mitotic kinases arises from both subcellular localization and preferences for phosphorylation motifs (Alexander et al., 2011). For the first time they described an evolutionary conserved mechanism based on a combination of negative and positive phosphorylation motifs selection and spatial localization, to secure proper signal propagation during mitosis. Thus, even if two kinases can share a phosphorylation motif or can localize in the same place, none of the mitotic kinases shares similar preferences in phosphorylation motifs and is also co-localized with any other mitotic kinase.
Protein kinases are highly flexible molecules, and this intrinsic flexibility has likely favored the engineering of complex regulatory and specificity mechanisms throughout eukaryotic evolution. Several mechanisms of recruitment are peculiar to some kinase families, and they can generally be grouped into: scaffold interactions, docking sites, and domain-domain interactions (Reményi et al., 2006; Miller et al., 2008) (Figure 1B).
Scaffold proteins can contribute to specificity increasing the local concentration of the kinase and the substrate, thus enhancing phosphorylation. Probably the best known are MAPK and PKA scaffolds (Wong and Scott, 2004; Strickfaden et al., 2007).
Docking motifs are distant from the phosphosite and facilitate the kinase-substrate recognition (Biondi and Nebreda, 2003). They can be discovered using experimental screening of focused or randomized peptide libraries (Reményi et al., 2006). In the case of Tyr kinases, the motif is usually found in domains that are different from the ones that catalyze the phosphorylation reaction. The motif can also be induced, as in the case of conditional docking sites, where the kinase is recruited only after a phosphorylation event takes place in the motif (Elia et al., 2003). This could also be a way used by the cell to implement logic gates and keep the timing of phosphorylation. Protein interaction domains, like SH2, SH3, PTB, 14-3-3 can also promote the association between kinases and substrates. Src activation, for instance, is mediated by its SH2 domain (Xu et al., 1999) (see Figure 1B). Domain-peptide interactions can be studied experimentally with peptide binding assays, while domain-domain interactions can be modeled using data collected in several databases (Luo et al., 2011; Yellaboina et al., 2011; Kim et al., 2012; Mosca et al., 2014), from high-throughput experiments, like yeast two hybrid, or extracted from the literature (see Figure 2 for an overview of the major experimental techniques used to identify phosphorylation sites and kinase-substrates interactions).
Figure 2. Methodologies for the experimental identification of phosphorylation sites. In the inner circle the techniques for the detection of phosphorylation sites are reported, while the outer circle displays the major techniques for dissecting kinase-substrates interactions, both at the level of direct determination of kinase-substrate interaction (kinase activity assays, OPL, MS with ATP analogs, structural data, western blot, optogenetics) and at the contextual information generation level, i.e., the methodologies that allow the identification of interacting domain preferences, domain-peptide interactions, etc. (Y2H, Y3H, phage display, structural data, western blot, optogenetics). In the field of PTM identification, future advancements in MS will allow the monitoring of multiple PTMs co-modulation, while for kinase-substrate interactions, the use of ATP analogs coupled with MS/MS is currently the most promising high-throughput technique to link kinases to their substrates in vivo. Y2H/Y3H: yeast 2/3 hybrid system (Y3H could be deployed for the study of scaffold proteins-mediated interactions).
In 2011 Won and colleagues described the contribution of recruitment interactions to the kinase specificity of Ste7, a MAPKK involved in mating signal flow in S. cerevisiae (Won et al., 2011). Ste7 has an interaction with the scaffold protein Ste5 and two docking interactions that allow it to bind to the MAPK Fus3. They uncovered that two out of the three other MAPKK encoded in S. cerevisiae genome can functionally replace the MAPKK Ste7, grafting recruitment interactions in their kinase domain. Notably, grafting only the scaffold, or only the docking interactions is not enough to restore the mating signal. This underlines the critical importance of recruitment mechanisms acting concertedly. Scaffold proteins mediating interactions may in theory be discovered using a yeast three hybrid approach, where the kinase and the substrate are fused as bait and pray, and their indirect interaction could be tested expressing the scaffold.
The cellular context has a fundamental role in the determination of the substrate specificity. For instance, kinase localization is important for proper CDKs function. A number of cyclins activate and localize CDKs to different compartments. Overexpression of cyclin B1 causes chromosome condensation, reorganization of the microtubules, and disassembly of the nuclear lamina and of the Golgi apparatus, while overexpression of cyclin B2 only causes the disassembly of Golgi apparatus. Changing the localization motifs, and swapping the two cyclins localizations reverses their phenotypes (Draviam et al., 2001).
Several mechanisms of specificity have recently been explored also for protein phosphatases (Tiganis and Bennett, 2007; Roy and Cyert, 2009). Despite these enzymes lack strong preferences for substrate sequences, higher specificity is obtained with recruitment via domains and short linear motifs-mediated interactions and subcellular localization (Sacco et al., 2012).
Currently tens of thousands of phosphorylation sites can be identified by MS-based proteomics in a single experiment (Olsen and Mann, 2013). These large-scale datasets challenged the view of PTMs gained from low-throughput experiments, where a few highly important sites are studied, questioning the functionality of all these PTMs. Several evolutionary studies on phosphorylation sites have confirmed that sites known to be associated to a function are significantly more conserved than non-phosphorylated residues (Gnad et al., 2007; Malik et al., 2008; Landry et al., 2009; Tan et al., 2009a; Moses and Landry, 2010). However, a large fraction of phosphorylation sites identified in high-throughput experiments does not show strong evolutionary conservation.
The high evolutionary turnover of phosphoproteomes may be due either to non-functional phosphorylation sites or to species-specific regulation. Recently, a model has been proposed that could explain observations about phosphorylation enrichment in abundant proteins combined with the low stoichiometry of these phosphorylation sites (Levy et al., 2012; Tan and Bader, 2012). According to this model, random encounters between a kinase and proteins in the same subcellular location could end up in a-specific phosphorylations, that will more likely affect highly abundant proteins. This model implies also that only a minimal fraction of an abundant protein population should host these off-target sites. Not all unintended phosphorylations are necessarily damaging the cell, otherwise they would have been removed during evolution. Therefore, a fraction of all phosphorylation events could be neutral from an evolutionary perspective. In this scenario, the upper bound to the accumulation in the proteome of such sites during evolution is the signaling networks tolerance to noise levels. A nice example of noise minimization has been observed in metazoan lineage evolution, where it has been hypothesized that the signaling networks may have eliminated detrimental phosphorylation sites and limited the noise in the system as tyrosine kinases expanded, by tyrosine-removing mutations (Tan et al., 2009b). In shorter evolutionary distances, the signaling networks properties will more tightly be coupled with the mutational properties of the codons encoding the different phosphorylatable residues. Amongst all residues, serine is considered a mutational hub, as it is very close in mutational space to most residues (Creixell et al., 2012). Indeed it is the only amino acid whose codons are distributed in two groups that are at least two mutations away from each other.
Another explanation for the low conservation of some phosphorylation sites could reside in compensatory mechanisms. In a pioneering work Bodenmiller and colleagues performed single deletions of all yeast kinases and phosphatases, surprisingly observing only a small amount of regulation in the phosphoproteome (Bodenmiller et al., 2010). Even more strikingly, the indirect effects are predominant on effects on the direct targets of the deleted kinases, without strong phenotype alterations, in agreement with the view of signaling networks as systems that are robust to perturbations. Crucially this highlights that a similar cellular state can be the result of different systems regulations. From an evolutionary perspective, different signaling solutions, independently evolved, could be analogous implementations of the same function.
A consistent fraction of eukaryotic phosphoproteomes may represent an evolutionary reservoir that the different organisms could exploit to evolve specific regulation. Estimating more precisely the magnitude of these non-functional phosphorylations will contribute in the near future to improve our understanding of post-translational regulatory networks and their properties.
In the case of phosphosites involved in modulating protein-protein interactions, the site may not necessarily be positionally conserved (Tan et al., 2010). Phosphosites in different organisms at the same interface tend also to be phosphorylated by kinases of similar specificity. Therefore, the same protein interface may be modulated by functionally redundant sites that are weakly conserved in sequence (Tan et al., 2010; Palmeri et al., 2014).
Many domains in the human proteome seem to have peculiar preferences for being targets of phosphorylation. Some domains, like the kinase domain, tend to be significantly enriched, while other ones tend to be depleted in phosphorylation. Within the same domain, phospho-hot spots can also be identified, i.e., regions that are highly enriched in phosphorylation, suggesting modulation of the domain function via these segments, as in the kinase activation loop, or the C-terminus of HSP90 domain (Beltrao et al., 2012). The domain context of a phosphosite can then be used to functionally characterize the site, and also to improve phosphosite predictor performances (Palmeri et al., 2014).
Several low-throughput experiments offer nice examples of how the cell uses PTMs combinations to reach highly sophisticated levels of control (Lo et al., 2001; Choudhary et al., 2009; Wang et al., 2011; Zheng et al., 2011). Difficulties in high-throughput determination of co-modulation between different PTMs currently limit the scale at which analysis of cross-regulation can be conducted. However, in a recent work, Swaney and colleagues studied the cross-talk between phosphorylation and ubiquitylation after proteasome inhibition, thus identifying potential phosphodegrons, analyzing the pairs of phosphorylation sites and ubiquitylation sites that increased in abundance after proteasome inhibition (Swaney et al., 2013). Computational works have already started exploring this relatively novel territory. For instance, Woodsmith and collaborators suggested that PTMs clusters may represent signal integration platforms (Woodsmith et al., 2013). In another work, Minguez and colleagues from Bork's group, used the concept of correlated evolution to discover new types of co-regulation within different PTMs (Minguez et al., 2012). Greater efforts in the developments of experimental methods for large scale monitoring of co-modulated PTMs will enormously help in understanding how the signaling networks respond to and integrate different inputs from a systems level perspective. But also new computational models will have to be developed and may benefit from coordinated modeling of the different PTMs.
Modeling kinase specificity for substrates is one of the most challenging bioinformatics contributions to cellular signaling. Mass Spectrometry is able to generate large amount of data, but there is currently no high-throughput experimental way to identify the candidate responsible for the phosphorylation.
The two main challenges in developing computational approaches to kinase-substrate specificity are: modeling kinase-peptide specificity and substrate recruitment preferences. Bioinformatics solutions have extensively explored the motif-context, and as pointed out by Joughin et al. the construction of higher order mathematical models might have limited, if any, advantage, at the high cost of overfitted models (Joughin et al., 2012). In silico modeling efforts should be centered around a more effective integration of different levels of contextual information, placing the kinases and the substrates in correct space and time, but also considering the interactions outside the kinase domain (domain-domain, scaffolds and docking sites interactions), that can increase locally the concentration of kinases and substrates. Data is obviously critical to all this, therefore more OPL screenings on kinases with unknown specificity, combined with more comprehensive studies to explore scaffold-mediated kinase-substrate interactions and also more efforts dedicated to assess the impact of mutations in domain-domain interactions involved in signaling could contribute to the development of more refined models of kinase-substrate specificity. Probably the most difficult challenge is to convert results from in vitro studies to approaches that make reliable in vivo predictions. Different kinases vary in their dependence on contextual information. Holistic, i.e., highly integrative, approaches, that allow the modeling of many contexts at the same time, will be able in the future to dissect for each kinase (or kinase group) the different contributions that shape the logic of the signaling system, like for instance in the remarkable case of mitotic kinases, studied by Yaffe's group (Alexander et al., 2011). High-throughput identification of the kinases responsible for the phosphorylation events is critical to achieve this. MS coupled with ATP analogs is currently the most promising approach in this field (Lopez et al., 2013).
Function-dependent classifiers, like those considering the identity of the domain where the phosphorylation is located, can be an alternative way to boost performances in phosphorylation prediction and they might be considered also for biomedical applications. Lastly, from the computational integration of different PTMs models (Creixell and Linding, 2012; Minguez et al., 2012), it may be possible, in the future, to infer and monitor system-level regulatory centers, whose function might be impaired in complex diseases.
The authors declare that the research was conducted in the absence of any commercial or financial relationships that could be construed as a potential conflict of interest.
This work was supported by the EPIGEN flagship project MIUR-CNR to Manuela Helmer-Citterich.
Alexander, J., Lim, D., Joughin, B. A., Hegemann, B., Hutchins, J. R. A., Ehrenberger, T., et al. (2011). Spatial exclusivity combined with positive and negative selection of phosphorylation motifs is the basis for context-dependent mitotic signaling. Sci. Signal. 4:ra42. doi: 10.1126/scisignal.2001796
Pubmed Abstract | Pubmed Full Text | CrossRef Full Text | Google Scholar
Barford, D., Hu, S. H., and Johnson, L. N. (1991). Structural mechanism for glycogen phosphorylase control by phosphorylation and AMP. J. Mol. Biol. 218, 233–260. doi: 10.1016/0022-2836(91)90887-C
Pubmed Abstract | Pubmed Full Text | CrossRef Full Text | Google Scholar
Biondi, R. M., and Nebreda, A. R. (2003). Signalling specificity of Ser/Thr protein kinases through docking-site-mediated interactions. Biochem. J. 372, 1–13. doi: 10.1042/BJ20021641
Pubmed Abstract | Pubmed Full Text | CrossRef Full Text | Google Scholar
Beltrao, P., Albanèse, V., Kenner, L. R., Swaney, D. L., Burlingame, A., Villén, J., et al. (2012). Systematic functional prioritization of protein posttranslational modifications. Cell 150, 413–425. doi: 10.1016/j.cell.2012.05.036
Pubmed Abstract | Pubmed Full Text | CrossRef Full Text | Google Scholar
Blom, N., Sicheritz-Pontén, T., Gupta, R., Gammeltoft, S., and Brunak, S. (2004). Prediction of post-translational glycosylation and phosphorylation of proteins from the amino acid sequence. Proteomics 4, 1633–1649. doi: 10.1002/pmic.200300771
Pubmed Abstract | Pubmed Full Text | CrossRef Full Text | Google Scholar
Bodenmiller, B., Wanka, S., Kraft, C., Urban, J., Campbell, D., Pedrioli, P. G., et al. (2010). Phosphoproteomic analysis reveals interconnected system-wide responses to perturbations of kinases and phosphatases in yeast. Sci. Signal. 3:rs4. doi: 10.1126/scisignal.2001182
Pubmed Abstract | Pubmed Full Text | CrossRef Full Text | Google Scholar
Bradham, C. A., Foltz, K. R., Beane, W. S., Arnone, M. I., Rizzo, F., Coffman, J. A., et al. (2006). The sea urchin kinome: a first look. Dev. Biol. 300, 180–193. doi: 10.1016/j.ydbio.2006.08.074
Pubmed Abstract | Pubmed Full Text | CrossRef Full Text | Google Scholar
Brinkworth, R. I., Breinl, R. A., and Kobe, B. (2003). Structural basis and prediction of substrate specificity in protein serine/threonine kinases. Proc. Natl. Acad. Sci. U.S.A. 100, 74–79. doi: 10.1073/pnas.0134224100
Pubmed Abstract | Pubmed Full Text | CrossRef Full Text | Google Scholar
Budovskaya, Y. V., Stephan, J. S., Deminoff, S. J., and Herman, P. K. (2005). An evolutionary proteomics approach identifies substrates of the cAMP-dependent protein kinase. Proc. Natl. Acad. Sci. U.S.A. 102, 13933–13938. doi: 10.1073/pnas.0501046102
Pubmed Abstract | Pubmed Full Text | CrossRef Full Text | Google Scholar
Caenepeel, S., Charydczak, G., Sudarsanam, S., Hunter, T., and Manning, G. (2004). The mouse kinome: discovery and comparative genomics of all mouse protein kinases. Proc. Natl. Acad. Sci. U.S.A. 101, 11707–11712. doi: 10.1073/pnas.0306880101
Pubmed Abstract | Pubmed Full Text | CrossRef Full Text | Google Scholar
Choudhary, C., Kumar, C., Gnad, F., Nielsen, M. L., Rehman, M., Walther, T. C., et al. (2009). Lysine acetylation targets protein complexes and co-regulates major cellular functions. Science 325, 834–840. doi: 10.1126/science.1175371
Pubmed Abstract | Pubmed Full Text | CrossRef Full Text | Google Scholar
Creixell, P., and Linding, R. (2012). Cells, shared memory and breaking the PTM code. Mol. Syst. Biol. 8, 598. doi: 10.1038/msb.2012.33
Pubmed Abstract | Pubmed Full Text | CrossRef Full Text | Google Scholar
Creixell, P., Schoof, E. M., Tan, C. S. H., and Linding, R. (2012). Mutational properties of amino acid residues: implications for evolvability of phosphorylatable residues. Philos. Trans. R. Soc. Lond. B Biol. Sci. 367, 2584–2593. doi: 10.1098/rstb.2012.0076
Pubmed Abstract | Pubmed Full Text | CrossRef Full Text | Google Scholar
Dang, T. H., Van Leemput, K., Verschoren, A., and Laukens, K. (2008). Prediction of kinase-specific phosphorylation sites using conditional random fields. Bioinformatics 24, 2857–2864. doi: 10.1093/bioinformatics/btn546
Pubmed Abstract | Pubmed Full Text | CrossRef Full Text | Google Scholar
Draviam, V. M., Orrechia, S., Lowe, M., Pardi, R., and Pines, J. (2001). The localization of human cyclins B1 and B2 determines Cdk1 substrate specificity and neither enzyme requires MEK to disassemble the Golgi apparatus. J. Cell Biol. 152, 945–958. doi: 10.1083/jcb.152.5.945
Pubmed Abstract | Pubmed Full Text | CrossRef Full Text | Google Scholar
Durek, P., Schudoma, C., Weckwerth, W., Selbig, J., and Walther, D. (2009). Detection and characterization of 3D-signature phosphorylation site motifs and their contribution towards improved phosphorylation site prediction in proteins. BMC Bioinformatics 10:117. doi: 10.1186/1471-2105-10-117
Pubmed Abstract | Pubmed Full Text | CrossRef Full Text | Google Scholar
Elia, A. E. H., Cantley, L. C., and Yaffe, M. B. (2003). Proteomic screen finds pSer/pThr-binding domain localizing Plk1 to mitotic substrates. Science 299, 1228–1231. doi: 10.1126/science.1079079
Pubmed Abstract | Pubmed Full Text | CrossRef Full Text | Google Scholar
Ellis, J. J., and Kobe, B. (2011). Predicting protein kinase specificity: predikin update and performance in the DREAM4 challenge. PLoS ONE 6:e21169. doi: 10.1371/journal.pone.0021169
Pubmed Abstract | Pubmed Full Text | CrossRef Full Text | Google Scholar
Fan, W., Xu, X., Shen, Y., Feng, H., Li, A., and Wang, M. (2014). Prediction of protein kinase-specific phosphorylation sites in hierarchical structure using functional information and random forest. Amino Acids 46, 1069–1078. doi: 10.1007/s00726-014-1669-3
Pubmed Abstract | Pubmed Full Text | CrossRef Full Text | Google Scholar
Franceschini, A., Szklarczyk, D., Frankild, S., Kuhn, M., Simonovic, M., Roth, A., et al. (2013). STRING v9.1: protein-protein interaction networks, with increased coverage and integration. Nucleic Acids Res. 41, D808–D815. doi: 10.1093/nar/gks1094
Pubmed Abstract | Pubmed Full Text | CrossRef Full Text | Google Scholar
Gao, J., Thelen, J. J., Dunker, A. K., and Xu, D. (2010). Musite, a tool for global prediction of general and kinase-specific phosphorylation sites. Mol. Cell. Proteomics 9, 2586–2600. doi: 10.1074/mcp.M110.001388
Pubmed Abstract | Pubmed Full Text | CrossRef Full Text | Google Scholar
Gnad, F., Gunawardena, J., and Mann, M. (2011). PHOSIDA 2011: the posttranslational modification database. Nucleic Acids Res. 39, D253–D260. doi: 10.1093/nar/gkq1159
Pubmed Abstract | Pubmed Full Text | CrossRef Full Text | Google Scholar
Gnad, F., Ren, S., Cox, J., Olsen, J. V., Macek, B., Oroshi, M., et al. (2007). PHOSIDA (phosphorylation site database): management, structural and evolutionary investigation, and prediction of phosphosites. Genome Biol. 8:R250. doi: 10.1186/gb-2007-8-11-r250
Pubmed Abstract | Pubmed Full Text | CrossRef Full Text | Google Scholar
Horn, H., Schoof, E. M., Kim, J., Robin, X., Miller, M. L., Diella, F., et al. (2014). KinomeXplorer: an integrated platform for kinome biology studies. Nat. Methods 11, 603–604. doi: 10.1038/nmeth.2968
Pubmed Abstract | Pubmed Full Text | CrossRef Full Text | Google Scholar
Huang, H.-D., Lee, T.-Y., Tzeng, S.-W., and Horng, J.-T. (2005). KinasePhos: a web tool for identifying protein kinase-specific phosphorylation sites. Nucleic Acids Res. 33, W226–W229. doi: 10.1093/nar/gki471
Pubmed Abstract | Pubmed Full Text | CrossRef Full Text | Google Scholar
Huang, K.-Y., Wu, H.-Y., Chen, Y.-J., Lu, C.-T., Su, M.-G., Hsieh, Y.-C., et al. (2014). RegPhos 2.0: an updated resource to explore protein kinase-substrate phosphorylation networks in mammals. Database 2014:bau034. doi: 10.1093/database/bau034
Pubmed Abstract | Pubmed Full Text | CrossRef Full Text | Google Scholar
Hunter, T. (2012). Why nature chose phosphate to modify proteins. Philos. Trans. R. Soc. Lond. B. Biol. Sci. 367, 2513–2516. doi: 10.1098/rstb.2012.0013
Pubmed Abstract | Pubmed Full Text | CrossRef Full Text | Google Scholar
Hurley, J. H., Dean, A. M., Sohl, J. L., Koshland, D. E., and Stroud, R. M. (1990). Regulation of an enzyme by phosphorylation at the active site. Science 249, 1012–1016. doi: 10.1126/science.2204109
Pubmed Abstract | Pubmed Full Text | CrossRef Full Text | Google Scholar
Hutti, J. E., Jarrell, E. T., Chang, J. D., Abbott, D. W., Storz, P., Toker, A., et al. (2004). A rapid method for determining protein kinase phosphorylation specificity. Nat. Methods 1, 27–29. doi: 10.1038/nmeth708
Pubmed Abstract | Pubmed Full Text | CrossRef Full Text | Google Scholar
Iakoucheva, L. M., Radivojac, P., Brown, C. J., O'Connor, T. R., Sikes, J. G., and Obradovic, Z. (2004). The importance of intrinsic disorder for protein phosphorylation. Nucleic Acids Res. 32, 1037–1049. doi: 10.1093/nar/gkh253
Pubmed Abstract | Pubmed Full Text | CrossRef Full Text | Google Scholar
Ingrell, C. R., Miller, M. L., Jensen, O. N., and Blom, N. (2007). NetPhosYeast: prediction of protein phosphorylation sites in yeast. Bioinformatics 23, 895–897. doi: 10.1093/bioinformatics/btm020
Pubmed Abstract | Pubmed Full Text | CrossRef Full Text | Google Scholar
Joughin, B. A., Liu, C., Lauffenburger, D. A., Hogue, C. W. V., and Yaffe, M. B. (2012). Protein kinases display minimal interpositional dependence on substrate sequence: potential implications for the evolution of signalling networks. Philos. Trans. R. Soc. Lond. B Biol. Sci. 367, 2574–2583. doi: 10.1098/rstb.2012.0010
Pubmed Abstract | Pubmed Full Text | CrossRef Full Text | Google Scholar
Kim, Y., Min, B., and Yi, G.-S. (2012). IDDI: integrated domain-domain interaction and protein interaction analysis system. Proteome Sci. 10(Suppl. 1):S9. doi: 10.1186/1477-5956-10-S1-S9
Pubmed Abstract | Pubmed Full Text | CrossRef Full Text | Google Scholar
Lai, A. C. W., Nguyen Ba, A. N., and Moses, A. M. (2012). Predicting kinase substrates using conservation of local motif density. Bioinformatics 28, 962–969. doi: 10.1093/bioinformatics/bts060
Pubmed Abstract | Pubmed Full Text | CrossRef Full Text | Google Scholar
Landry, C. R., Levy, E. D., and Michnick, S. W. (2009). Weak functional constraints on phosphoproteomes. Trends Genet. 25, 193–197. doi: 10.1016/j.tig.2009.03.003
Pubmed Abstract | Pubmed Full Text | CrossRef Full Text | Google Scholar
Lee, T.-Y., Bo-Kai Hsu, J., Chang, W.-C., and Huang, H.-D. (2011). RegPhos: a system to explore the protein kinase-substrate phosphorylation network in humans. Nucleic Acids Res. 39, D777–D787. doi: 10.1093/nar/gkq970
Pubmed Abstract | Pubmed Full Text | CrossRef Full Text | Google Scholar
Levy, E. D., Michnick, S. W., and Landry, C. R. (2012). Protein abundance is key to distinguish promiscuous from functional phosphorylation based on evolutionary information. Philos. Trans. R. Soc. Lond. B Biol. Sci. 367, 2594–2606. doi: 10.1098/rstb.2012.0078
Pubmed Abstract | Pubmed Full Text | CrossRef Full Text | Google Scholar
Li, T., Li, F., and Zhang, X. (2008). Prediction of kinase-specific phosphorylation sites with sequence features by a log-odds ratio approach. Proteins 70, 404–414. doi: 10.1002/prot.21563
Pubmed Abstract | Pubmed Full Text | CrossRef Full Text | Google Scholar
Lim, W. A., and Pawson, T. (2010). Phosphotyrosine signaling: evolving a new cellular communication system. Cell 142, 661–667. doi: 10.1016/j.cell.2010.08.023
Pubmed Abstract | Pubmed Full Text | CrossRef Full Text | Google Scholar
Linding, R., Jensen, L. J., Ostheimer, G. J., van Vugt, M. A., Jørgensen, C., Miron, I. M., et al. (2007). Systematic discovery of in vivo phosphorylation networks. Cell 129, 1415–1426. doi: 10.1016/j.cell.2007.05.052
Pubmed Abstract | Pubmed Full Text | CrossRef Full Text | Google Scholar
Linding, R., Jensen, L. J., Pasculescu, A., Olhovsky, M., Colwill, K., Bork, P., et al. (2008). NetworKIN: a resource for exploring cellular phosphorylation networks. Nucleic Acids Res. 36, D695–D699. doi: 10.1093/nar/gkm902
Pubmed Abstract | Pubmed Full Text | CrossRef Full Text | Google Scholar
Lo, W. S., Duggan, L., Emre, N. C., Belotserkovskya, R., Lane, W. S., Shiekhattar, R., et al. (2001). Snf1–a histone kinase that works in concert with the histone acetyltransferase Gcn5 to regulate transcription. Science 293, 1142–1146. doi: 10.1126/science.1062322
Pubmed Abstract | Pubmed Full Text | CrossRef Full Text | Google Scholar
Lopez, M. S., Choy, J. W., Peters, U., Sos, M. L., Morgan, D. O., and Shokat, K. M. (2013). Staurosporine-derived inhibitors broaden the scope of analog-sensitive kinase technology. J. Am. Chem. Soc. 135, 18153–18159. doi: 10.1021/ja408704u
Pubmed Abstract | Pubmed Full Text | CrossRef Full Text | Google Scholar
Luo, Q., Pagel, P., Vilne, B., and Frishman, D. (2011). DIMA 3.0: Domain interaction map. Nucleic Acids Res. 39, D724–D729. doi: 10.1093/nar/gkq1200
Pubmed Abstract | Pubmed Full Text | CrossRef Full Text | Google Scholar
Malik, R., Nigg, E. A., and Körner, R. (2008). Comparative conservation analysis of the human mitotic phosphoproteome. Bioinformatics 24, 1426–1432. doi: 10.1093/bioinformatics/btn197
Pubmed Abstract | Pubmed Full Text | CrossRef Full Text | Google Scholar
Manning, G., Plowman, G. D., Hunter, T., and Sudarsanam, S. (2002a). Evolution of protein kinase signaling from yeast to man. Trends Biochem. Sci. 27, 514–520. doi: 10.1016/S0968-0004(02)02179-5
Pubmed Abstract | Pubmed Full Text | CrossRef Full Text | Google Scholar
Manning, G., Reiner, D. S., Lauwaet, T., Dacre, M., Smith, A., Zhai, Y., et al. (2011). The minimal kinome of Giardia lamblia illuminates early kinase evolution and unique parasite biology. Genome Biol. 12:R66. doi: 10.1186/gb-2011-12-7-r66
Pubmed Abstract | Pubmed Full Text | CrossRef Full Text | Google Scholar
Manning, G., Whyte, D. B., Martinez, R., Hunter, T., and Sudarsanam, S. (2002b). The protein kinase complement of the human genome. Science 298, 1912. doi: 10.1126/science.1075762
Pubmed Abstract | Pubmed Full Text | CrossRef Full Text | Google Scholar
Manning, G., Young, S. L., Miller, W. T., and Zhai, Y. (2008). The protist, monosiga brevicollis, has a tyrosine kinase signaling network more elaborate and diverse than found in any known metazoan. Proc. Natl. Acad. Sci. U.S.A. 105, 9674–9679. doi: 10.1073/pnas.0801314105
Pubmed Abstract | Pubmed Full Text | CrossRef Full Text | Google Scholar
Miller, M., Jensen, L., Diella, F., Jorgensen, C., Tinti, M., Li, L., et al. (2008). Linear motif atlas for phosphorylation-dependent signaling. Sci. Signal. 1:ra2. doi: 10.1126/scisignal.1159433
Pubmed Abstract | Pubmed Full Text | CrossRef Full Text | Google Scholar
Miller, M. L., and Blom, N. (2009). Kinase-specific prediction of protein phosphorylation sites. Methods Mol. Biol. 527, 299–310. doi: 10.1007/978-1-60327-834-8_22
Pubmed Abstract | Pubmed Full Text | CrossRef Full Text | Google Scholar
Miller, M. L., Soufi, B., Jers, C., Blom, N., Macek, B., and Mijakovic, I. (2009). NetPhosBac - a predictor for Ser/Thr phosphorylation sites in bacterial proteins. Proteomics 9, 116–125. doi: 10.1002/pmic.200800285
Pubmed Abstract | Pubmed Full Text | CrossRef Full Text | Google Scholar
Minguez, P., Parca, L., Diella, F., Mende, D. R., Kumar, R., Helmer-Citterich, M., et al. (2012). Deciphering a global network of functionally associated post-translational modifications. Mol. Syst. Biol. 8, 599. doi: 10.1038/msb.2012.31
Pubmed Abstract | Pubmed Full Text | CrossRef Full Text | Google Scholar
Mosca, R., Céol, A., Stein, A., Olivella, R., and Aloy, P. (2014). 3did: a catalog of domain-based interactions of known three-dimensional structure. Nucleic Acids Res. 42, D374–D379. doi: 10.1093/nar/gkt887
Pubmed Abstract | Pubmed Full Text | CrossRef Full Text | Google Scholar
Moses, A. M., Hériché, J.-K., and Durbin, R. (2007). Clustering of phosphorylation site recognition motifs can be exploited to predict the targets of cyclin-dependent kinase. Genome Biol. 8:R23. doi: 10.1186/gb-2007-8-2-r23
Pubmed Abstract | Pubmed Full Text | CrossRef Full Text | Google Scholar
Moses, A. M., and Landry, C. R. (2010). Moving from transcriptional to phospho-evolution: generalizing regulatory evolution? Trends Genet. 26, 462–467. doi: 10.1016/j.tig.2010.08.002
Pubmed Abstract | Pubmed Full Text | CrossRef Full Text | Google Scholar
Neuberger, G., Schneider, G., and Eisenhaber, F. (2007). pkaPS: prediction of protein kinase a phosphorylation sites with the simplified kinase-substrate binding model. Biol. Direct 2:1. doi: 10.1186/1745-6150-2-1
Pubmed Abstract | Pubmed Full Text | CrossRef Full Text | Google Scholar
Obenauer, J. C., Cantley, L. C., and Yaffe, M. B. (2003). Scansite 2.0: proteome-wide prediction of cell signaling interactions using short sequence motifs. Nucleic Acids Res. 31, 3635–3641. doi: 10.1093/nar/gkg584
Pubmed Abstract | Pubmed Full Text | CrossRef Full Text | Google Scholar
Olsen, J. V., and Mann, M. (2013). Status of large-scale analysis of post-translational modifications by mass spectrometry. Mol. Cell. Proteomics 12, 3444–3452. doi: 10.1074/mcp.O113.034181
Pubmed Abstract | Pubmed Full Text | CrossRef Full Text | Google Scholar
Palmeri, A., Ausiello, G., Ferre, F., Helmer-Citterich, M., and Gherardini, P. F. (2014). A proteome-wide domain-centric perspective on protein phosphorylation. Mol. Cell. Proteomics 13, 2198–2212. doi: 10.1074/mcp.M114.039990
Pubmed Abstract | Pubmed Full Text | CrossRef Full Text | Google Scholar
Palmeri, A., Gherardini, P. F., Tsigankov, P., Ausiello, G., Späth, G. F., Zilberstein, D., et al. (2011). PhosTryp: a phosphorylation site predictor specific for parasitic protozoa of the family trypanosomatidae. BMC Genomics 12:614. doi: 10.1186/1471-2164-12-614
Pubmed Abstract | Pubmed Full Text | CrossRef Full Text | Google Scholar
Reményi, A., Good, M. C., and Lim, W. A. (2006). Docking interactions in protein kinase and phosphatase networks. Curr. Opin. Struct. Biol. 16, 676–685. doi: 10.1016/j.sbi.2006.10.008
Pubmed Abstract | Pubmed Full Text | CrossRef Full Text | Google Scholar
Roy, J., and Cyert, M. S. (2009). Cracking the phosphatase code: docking interactions determine substrate specificity. Sci. Signal. 2:re9. doi: 10.1126/scisignal.2100re9
Pubmed Abstract | Pubmed Full Text | CrossRef Full Text | Google Scholar
Sacco, F., Perfetto, L., Castagnoli, L., and Cesareni, G. (2012). The human phosphatase interactome: an intricate family portrait. FEBS Lett. 586, 2732–2739. doi: 10.1016/j.febslet.2012.05.008
Pubmed Abstract | Pubmed Full Text | CrossRef Full Text | Google Scholar
Saunders, N. F. W., Brinkworth, R. I., Huber, T., Kemp, B. E., and Kobe, B. (2008). Predikin and PredikinDB: a computational framework for the prediction of protein kinase peptide specificity and an associated database of phosphorylation sites. BMC Bioinformatics 9:245. doi: 10.1186/1471-2105-9-245
Pubmed Abstract | Pubmed Full Text | CrossRef Full Text | Google Scholar
Songyang, Z., Blechner, S., Hoagland, N., Hoekstra, M. F., Piwnica-Worms, H., and Cantley, L. C. (1994). Use of an oriented peptide library to determine the optimal substrates of protein kinases. Curr. Biol. 4, 973–982. doi: 10.1016/S0960-9822(00)00221-9
Pubmed Abstract | Pubmed Full Text | CrossRef Full Text | Google Scholar
Strickfaden, S. C., Winters, M. J., Ben-Ari, G., Lamson, R. E., Tyers, M., and Pryciak, P. M. (2007). A mechanism for cell-cycle regulation of MAP kinase signaling in a yeast differentiation pathway. Cell 128, 519–531. doi: 10.1016/j.cell.2006.12.032
Pubmed Abstract | Pubmed Full Text | CrossRef Full Text | Google Scholar
Swaney, D. L., Beltrao, P., Starita, L., Guo, A., Rush, J., Fields, S., et al. (2013). Global analysis of phosphorylation and ubiquitylation cross-talk in protein degradation. Nat. Methods 10, 676–682. doi: 10.1038/nmeth.2519
Pubmed Abstract | Pubmed Full Text | CrossRef Full Text | Google Scholar
Tan, C. S. H., and Bader, G. D. (2012). Phosphorylation sites of higher stoichiometry are more conserved. Nat. Methods 9, 317; author reply: 318. doi: 10.1038/nmeth.1941
Pubmed Abstract | Pubmed Full Text | CrossRef Full Text | Google Scholar
Tan, C. S. H., Bodenmiller, B., Pasculescu, A., Jovanovic, M., Hengartner, M. O., Jørgensen, C., et al. (2009a). Comparative analysis reveals conserved protein phosphorylation networks implicated in multiple diseases. Sci. Signal. 2:ra39. doi: 10.1126/scisignal.2000316
Pubmed Abstract | Pubmed Full Text | CrossRef Full Text | Google Scholar
Tan, C. S. H., Jørgensen, C., and Linding, R. (2010). Roles of “junk phosphorylation” in modulating biomolecular association of phosphorylated proteins? Cell cycle Georg. Tex 9, 1276–1280. doi: 10.4161/cc.9.7.11066
Tan, C. S. H., Pasculescu, A., Lim, W. A., Pawson, T., Bader, G. D., and Linding, R. (2009b). Positive selection of tyrosine loss in metazoan evolution. Science 325, 1686–1688. doi: 10.1126/science.1174301
Pubmed Abstract | Pubmed Full Text | CrossRef Full Text | Google Scholar
Tiganis, T., and Bennett, A. M. (2007). Protein tyrosine phosphatase function: the substrate perspective. Biochem. J. 402, 1–15. doi: 10.1042/BJ20061548
Pubmed Abstract | Pubmed Full Text | CrossRef Full Text | Google Scholar
Trost, B., and Kusalik, A. (2011). Computational prediction of eukaryotic phosphorylation sites. Bioinformatics 27, 2927–2935. doi: 10.1093/bioinformatics/btr525
Pubmed Abstract | Pubmed Full Text | CrossRef Full Text | Google Scholar
Turk, B. E. (2008). Understanding and exploiting substrate recognition by protein kinases. Curr. Opin. Chem. Biol. 12, 4–10. doi: 10.1016/j.cbpa.2008.01.018
Pubmed Abstract | Pubmed Full Text | CrossRef Full Text | Google Scholar
Ubersax, J. A., and Ferrell, J. E. (2007). Mechanisms of specificity in protein phosphorylation. Nat. Rev. Mol. Cell Biol. 8, 530–541. doi: 10.1038/nrm2203
Pubmed Abstract | Pubmed Full Text | CrossRef Full Text | Google Scholar
Via, A., Diella, F., Gibson, T. J., and Helmer-Citterich, M. (2011). From sequence to structural analysis in protein phosphorylation motifs. Front. Biosci. 16, 1261–1275. doi: 10.2741/3787
Pubmed Abstract | Pubmed Full Text | CrossRef Full Text | Google Scholar
Wan, J., Kang, S., Tang, C., Yan, J., Ren, Y., Liu, J., et al. (2008). Meta-prediction of phosphorylation sites with weighted voting and restricted grid search parameter selection. Nucleic Acids Res. 36, e22. doi: 10.1093/nar/gkm848
Pubmed Abstract | Pubmed Full Text | CrossRef Full Text | Google Scholar
Wang, Z. A., Singh, D., van der Wel, H., and West, C. M. (2011). Prolyl hydroxylation- and glycosylation-dependent functions of Skp1 in O2-regulated development of Dictyostelium. Dev. Biol. 349, 283–295. doi: 10.1016/j.ydbio.2010.10.013
Pubmed Abstract | Pubmed Full Text | CrossRef Full Text | Google Scholar
Won, A. P., Garbarino, J. E., and Lim, W. A. (2011). Recruitment interactions can override catalytic interactions in determining the functional identity of a protein kinase. Proc. Natl. Acad. Sci. U.S.A. 108, 9809–9814. doi: 10.1073/pnas.1016337108
Pubmed Abstract | Pubmed Full Text | CrossRef Full Text | Google Scholar
Wong, W., and Scott, J. D. (2004). AKAP signalling complexes: focal points in space and time. Nat. Rev. Mol. Cell Biol. 5, 959–970. doi: 10.1038/nrm1527
Pubmed Abstract | Pubmed Full Text | CrossRef Full Text | Google Scholar
Wong, Y.-H., Lee, T.-Y., Liang, H.-K., Huang, C.-M., Wang, T.-Y., Yang, Y.-H., et al. (2007). KinasePhos 2.0: a web server for identifying protein kinase-specific phosphorylation sites based on sequences and coupling patterns. Nucleic Acids Res. 35, W588–W594. doi: 10.1093/nar/gkm322
Pubmed Abstract | Pubmed Full Text | CrossRef Full Text | Google Scholar
Woodsmith, J., Kamburov, A., and Stelzl, U. (2013). Dual coordination of post translational modifications in human protein networks. PLoS Comput. Biol. 9:e1002933. doi: 10.1371/journal.pcbi.1002933
Pubmed Abstract | Pubmed Full Text | CrossRef Full Text | Google Scholar
Xu, W., Doshi, A., Lei, M., Eck, M. J., and Harrison, S. C. (1999). Crystal structures of c-Src reveal features of its autoinhibitory mechanism. Mol. Cell 3, 629–638. doi: 10.1016/S1097-2765(00)80356-1
Pubmed Abstract | Pubmed Full Text | CrossRef Full Text | Google Scholar
Xu, X., Li, A., Zou, L., Shen, Y., Fan, W., and Wang, M. (2014). Improving the performance of protein kinase identification via high dimensional protein-protein interactions and substrate structure data. Mol. Biosyst. 10, 694–702. doi: 10.1039/c3mb70462a
Pubmed Abstract | Pubmed Full Text | CrossRef Full Text | Google Scholar
Xue, Y., Gao, X., Cao, J., Liu, Z., Jin, C., Wen, L., et al. (2010). A summary of computational resources for protein phosphorylation. Curr. Protein Pept. Sci. 11, 485–96. doi: 10.2174/138920310791824138
Pubmed Abstract | Pubmed Full Text | CrossRef Full Text | Google Scholar
Xue, Y., Li, A., Wang, L., Feng, H., and Yao, X. (2006). PPSP: prediction of PK-specific phosphorylation site with Bayesian decision theory. BMC Bioinformatics 7:163. doi: 10.1186/1471-2105-7-163
Pubmed Abstract | Pubmed Full Text | CrossRef Full Text | Google Scholar
Xue, Y., Liu, Z., Cao, J., Ma, Q., Gao, X., Wang, Q., et al. (2011). GPS 2.1: enhanced prediction of kinase-specific phosphorylation sites with an algorithm of motif length selection. Protein Eng. Des. Sel. 24, 255–260. doi: 10.1093/protein/gzq094
Pubmed Abstract | Pubmed Full Text | CrossRef Full Text | Google Scholar
Xue, Y., Ren, J., Gao, X., Jin, C., Wen, L., and Yao, X. (2008). GPS 2.0, a tool to predict kinase-specific phosphorylation sites in hierarchy. Mol. Cell. Proteomics 7, 1598–1608. doi: 10.1074/mcp.M700574-MCP200
Pubmed Abstract | Pubmed Full Text | CrossRef Full Text | Google Scholar
Yaffe, M. B. (2004). Novel at the library. Nat. Methods 1, 13–14. doi: 10.1038/nmeth1004-13
Pubmed Abstract | Pubmed Full Text | CrossRef Full Text | Google Scholar
Yaffe, M. B., Leparc, G. G., Lai, J., Obata, T., Volinia, S., and Cantley, L. C. (2001). A motif-based profile scanning approach for genome-wide prediction of signaling pathways. Nat. Biotechnol. 19, 348–353. doi: 10.1038/86737
Pubmed Abstract | Pubmed Full Text | CrossRef Full Text | Google Scholar
Yellaboina, S., Tasneem, A., Zaykin, D. V., Raghavachari, B., and Jothi, R. (2011). DOMINE: a comprehensive collection of known and predicted domain-domain interactions. Nucleic Acids Res. 39, D730–D735. doi: 10.1093/nar/gkq1229
Pubmed Abstract | Pubmed Full Text | CrossRef Full Text | Google Scholar
Zheng, B., Han, M., Shu, Y.-N., Li, Y.-J., Miao, S.-B., Zhang, X.-H., et al. (2011). HDAC2 phosphorylation-dependent Klf5 deacetylation and RARα acetylation induced by RAR agonist switch the transcription regulatory programs of p21 in VSMCs. Cell Res. 21, 1487–1508. doi: 10.1038/cr.2011.34
Pubmed Abstract | Pubmed Full Text | CrossRef Full Text | Google Scholar
Zhou, F.-F., Xue, Y., Chen, G.-L., and Yao, X. (2004). GPS: a novel group-based phosphorylation predicting and scoring method. Biochem. Biophys. Res. Commun. 325, 1443–1448. doi: 10.1016/j.bbrc.2004.11.001
Pubmed Abstract | Pubmed Full Text | CrossRef Full Text | Google Scholar
Zhu, G., Liu, Y., and Shaw, S. (2005). Protein kinase specificity. a strategic collaboration between kinase peptide specificity and substrate recruitment. Cell Cycle 4, 52–56. doi: 10.4161/cc.4.1.1353
Pubmed Abstract | Pubmed Full Text | CrossRef Full Text | Google Scholar
Zou, L., Wang, M., Shen, Y., Liao, J., Li, A., and Wang, M. (2013). PKIS: computational identification of protein kinases for experimentally discovered protein phosphorylation sites. BMC Bioinformatics 14:247. doi: 10.1186/1471-2105-14-247
Pubmed Abstract | Pubmed Full Text | CrossRef Full Text | Google Scholar
Keywords: kinase-substrate specificity, phosphorylation context, phosphorylation prediction, cellular signaling, kinase-peptide specificity, substrate recruitment, signaling networks
Citation: Palmeri A, Ferrè F and Helmer-Citterich M (2014) Exploiting holistic approaches to model specificity in protein phosphorylation. Front. Genet. 5:315. doi: 10.3389/fgene.2014.00315
Received: 06 June 2014; Accepted: 21 August 2014;
Published online: 30 September 2014.
Copyright © 2014 Palmeri, Ferrè and Helmer-Citterich. This is an open-access article distributed under the terms of the Creative Commons Attribution License (CC BY). The use, distribution or reproduction in other forums is permitted, provided the original author(s) or licensor are credited and that the original publication in this journal is cited, in accordance with accepted academic practice. No use, distribution or reproduction is permitted which does not comply with these terms.
*Correspondence: Antonio Palmeri, Department of Biology, Centre for Molecular Bioinformatics, University of Rome Tor Vergata, Via della Ricerca Scientifica snc, 00133 Rome, Italy e-mail:YW50b25pby5wYWxtZXJpQHVuaXJvbWEyLml0
Disclaimer: All claims expressed in this article are solely those of the authors and do not necessarily represent those of their affiliated organizations, or those of the publisher, the editors and the reviewers. Any product that may be evaluated in this article or claim that may be made by its manufacturer is not guaranteed or endorsed by the publisher.
Research integrity at Frontiers
Learn more about the work of our research integrity team to safeguard the quality of each article we publish.