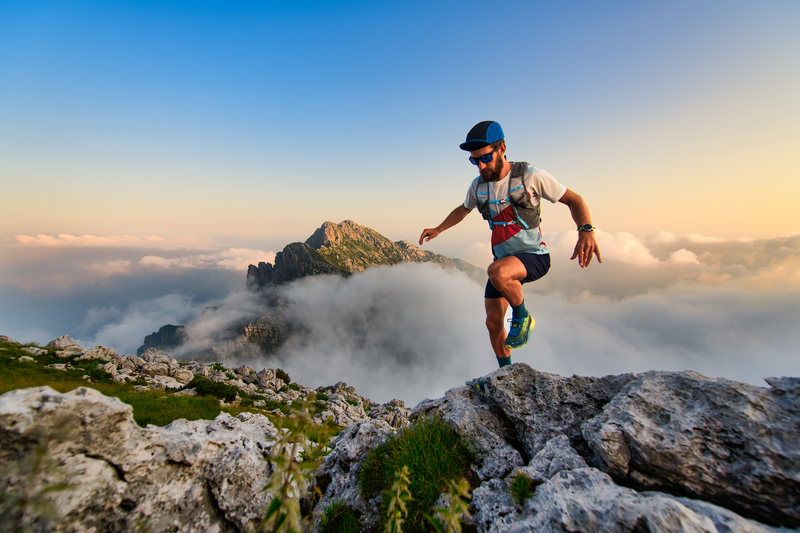
95% of researchers rate our articles as excellent or good
Learn more about the work of our research integrity team to safeguard the quality of each article we publish.
Find out more
ORIGINAL RESEARCH article
Front. Genet. , 17 May 2013
Sec. Behavioral and Psychiatric Genetics
Volume 4 - 2013 | https://doi.org/10.3389/fgene.2013.00087
This article is part of the Research Topic Ion Channels and Mental Illness: Exploring etiology and pathophysiology in major psychiatric disorders View all 9 articles
Genome-wide association studies (GWAS) have implicated ANK3 as a susceptibility gene for bipolar disorder (BP). We examined whether epistasis with ANK3 may contribute to the “missing heritability” in BP. We first identified via the STRING database 14 genes encoding proteins with prior biological evidence that they interact molecularly with ANK3. We then tested for statistical evidence of interactions between SNPs in these genes in association with BP in a discovery GWAS dataset and two replication GWAS datasets. The most significant interaction in the discovery GWAS was between SNPs in ANK3 and KCNQ2 (p = 3.18 × 10−8). A total of 31 pair-wise interactions involving combinations between two SNPs from KCNQ2 and 16 different SNPs in ANK3 were significant after permutation. Of these, 28 pair-wise interactions were significant in the first replication GWAS. None were significant in the second replication GWAS, but the two SNPs from KCNQ2 were found to significantly interact with five other SNPs in ANK3, suggesting possible allelic heterogeneity. KCNQ2 forms homo- and hetero-meric complexes with KCNQ3 that constitute voltage-gated potassium channels in neurons. ANK3 is an adaptor protein that, through its interaction with KCNQ2 and KCNQ3, directs the localization of this channel in the axon initial segment (AIS). At the AIS, the KCNQ2/3 complex gives rise to the M-current, which stabilizes the neuronal resting potential and inhibits repetitive firing of action potentials. Thus, these channels act as “dampening” components and prevent neuronal hyperactivity. The interactions between ANK3 and KCNQ2 merit further investigation, and if confirmed, may motivate a new line of research into a novel therapeutic target for BP.
Genome-wide association studies (GWAS) offer an unbiased, high-throughput approach for searching the entire genome to identify disease-causing variants. This approach has generated much enthusiasm in the study of complex disorders such as bipolar disorder (BP) that have been more difficult than Mendelian diseases to genetically map with linkage and candidate gene association approaches. However, there was little agreement in the findings from the initial GWAS of BP (Craddock and Sklar, 2009). These initial studies were likely under-powered to identify susceptibility loci for BP. A mega-analysis (Ferreira et al., 2008) addressed the issue of power by combining several GWAS, and reported the strongest evidence of an association with ANK3 (rs10994336, p = 9.1 × 10−9). A subsequent community-wide effort to combine GWAS from existing studies of BP, referred to as the Psychiatric GWAS Consortium (PGC), identified several additional susceptibility loci and provided further evidence for the association with ANK3 (rs10994397, p = 7.1 × 10−9) (Psychiatric GWAS Consortium Bipolar Disorder Working Group, 2011). Although promising, the loci identified thus far from these efforts likely account for only a small proportion of variation in susceptibility. Consequently, much of the genetic architecture of this disorder remains to be elucidated.
The identification of loci that only account for a small proportion of susceptibility to complex disorders appears to be a typical feature of GWAS of complex disorders. This has led investigators to question where the missing heritability is (Manolio et al., 2009). Epistasis, or gene–gene interaction, is one possible explanation for the so-called missing heritability. Epistasis can be conceptualized biologically or statistically (Moore and Williams, 2005). Biologically, it results from physical interactions between DNA, RNA, or proteins such that the resulting phenotype depends on the action of multiple genes. Statistically, it can be defined as a departure from the additive effects of alleles from different loci with respect to their contribution to variation in the resulting phenotype. Searching only for main effects of loci and ignoring possible epistasis may reduce power to detect potentially causal variants (Cordell, 2002). In fact, some researchers have argued that the lack of replication across studies may be a hallmark of epistasis (Wade, 2001; Hirschhorn et al., 2002; Moore and Williams, 2002, 2005). Moreover, the detection of epistasis may help reveal potentially meaningful biological mechanisms (Risch, 1990), which is a central goal of translational research.
The availability of dense SNP maps in GWAS presents the opportunity to investigate potential epistasis across the entire genome. However, this is limited by the computational challenge of testing all possible interactions between SNPs, and the enormous statistical penalty incurred by the multiple testing (Pattin and Moore, 2008). One strategy for overcoming these limitations is to condition the search for epistasis on known loci that have been previously associated with disease. Although this strategy runs the risk of missing purely epistatic models in which the genes involved have little or no main effect on disease susceptibility, it greatly reduces the possible search space, rendering the analyses more efficient, while still exploiting the vast amount of data available from the GWAS. A complementary approach to further reduce the search space involves limiting the analysis to genes whose products are expected to interact based on biological knowledge, such as protein interaction databases (Emily et al., 2009).
We therefore decided to search for evidence of epistasis in BP by focusing on ANK3. We chose this gene based on the prior evidence of association with BP, as well as the biological role of its protein product, which lends itself to this type of analysis. ANK3 belongs to a family of multifunctional membrane adapter proteins that target structurally diverse proteins to specialized membrane domains by linking them to the spectrin-based membrane skeleton (Bennett and Chen, 2001). We used a novel strategy that combined bioinformatics and statistical genetics approaches to test for relevant interactions with ANK3. First, we searched a well-curated protein–protein interaction database to identify genes encoding proteins that biological evidence suggests interact molecularly with ANK3. Then, we tested for congruent statistical evidence of interactions between ANK3 and these genes using GWAS data. By placing statistical findings in the context of human biology, we sought to strengthen the validity of any conclusions and facilitate the translation of results into potential benefits aimed at prevention and treatment of the disorder (Pattin and Moore, 2008).
We tested for statistical evidence of interactions with ANK3 using a GWAS dataset that consisted of two samples collected by the NIMH Genetics Initiative Bipolar Disorder Consortium, the Genetic Association Information Network Bipolar Disorder (GAIN) sample, and the Translational Genomics Research Institute Bipolar Disorder (TGEN) sample. The methods for collecting, diagnosing, and genotyping these samples have been described elsewhere (Dick et al., 2003; Kassem et al., 2006; Smith et al., 2009). Cases were ascertained from twelve clinical sites across the United States. They were assessed with the Diagnostic Interview for Genetic Studies (DIGS) (Nurnberger et al., 1994), and family informant data and medical records were obtained. Diagnoses were assigned following a best-estimate procedure according to DSM-III-R or DSM-IV criteria. The cases were all Caucasian and had a diagnosis of BP. Controls were ascertained through the efforts of the Molecular Genetics of Schizophrenia II (MGS-2) Collaboration (Sanders et al., 2008). Control subjects completed a brief psychiatric questionnaire and were excluded if they endorsed a history of BP, psychosis, or major depression. Cases and controls were matched on ethnicity, age, and sex. Appropriate IRB approval was obtained at each collaborating institution, and all subjects provided informed consent.
The cases and controls from both the GAIN and TGEN samples were genotyped on the Affymetrix 6.0 array. Quality control in both samples consisted of dropping subjects with ≥5% missing data, and dropping SNPs with ≥5% missing data, <1% minor allele frequency, or HWE p-value <10−6 among controls. We then imputed SNPs in each sample and combined them into one dataset. We used phased haplotype data from HapMap I and II release 241 as the reference panel. We used the program BEAGLE to flip orientation to the positive strand and impute estimated allelic dosages for autosomal SNPs (Browning and Yu, 2009). We excluded any SNPs with an imputation r2 < 0.3. The final GAIN-TGEN GWAS imputed dataset included 2,191 cases and 1,434 controls with data on 3,849,034 total SNPs.
We used two additional GWAS datasets for replication of the top findings. These datasets included the Wellcome Trust Case-Control Consortium (WTCCC) bipolar disorder sample and a German bipolar disorder sample. Methods for collecting, diagnosing, and genotyping both these samples have been described elsewhere (Fangerau et al., 2004; Wellcome Trust Case Control Consortium, 2007; McMahon et al., 2010). Appropriate IRB approval was obtained and all subjects provided informed consent. In the WTCCC sample, 2,000 cases were ascertained from sites across the United Kingdom, and assessed with semi-structured lifetime diagnostic psychiatric interviews (usually the Schedule for Clinical Assessment in Neuropsychiatry). A diagnosis of BP was assigned according to Research Diagnostic Criteria. Three thousand controls were obtained from the 1958 British Cohort study and UK blood donors. All cases and controls were Caucasian. Genotyping was done on the Affymetrix 500K Mapping Array. Quality control consisted of dropping subjects with ≥5% missing data, and dropping SNPs with ≥5% missing data rate, <1% minor allele frequency, <90% quality score, or HWE p-value <10−6 among controls. After imputation using procedures identical to those used with the GAIN-TGEN dataset as described above, there was a total of 1,868 BP cases and 2,996 controls with data on 3,849,034 SNPs in the final imputed WTCCC dataset.
Bipolar disorder cases for the German sample were recruited through hospital admissions and assessed with a structured interview. Diagnoses were assigned according to a best-estimate procedure using DSM-IV criteria. Population-based control subjects were obtained from the PopGen2, KORA3, and Heinz Nixdorf Recall Study4 cohorts. Genotyping was done on the Illumina HumanHap550 array. Quality control for this dataset included dropping subjects with >5% missing data, and dropping SNPs with >2% missing data, minor allele frequency <2% or HWE p-value <0.0001. After imputation using procedures identical to those used with the GAIN-TGEN dataset as described above, there was a total of 645 BP cases and 1,310 controls with data on 3,849,034 SNPs in the final imputed German dataset.
We used the STRING database version 9.05 to identify proteins with biological evidence for interaction with ANK3 (Jensen et al., 2009). STRING is a meta-resource of protein–protein interaction databases, based on the union of known physical protein interactions and curated data from biological pathways. This comprehensive tool extracts evidence from MINT, HPRD, BIND, DIP, BioGRID, KEGG, Reactome, IntAct, EcoCyc, NCI-Nature Pathway Interaction Database and Gene Ontology (GO) protein complexes. It also collects evidence of protein interactions via text mining (from SGD, OMIM, The Interactive Fly, and all abstracts on PubMed) and interaction transfers between organisms, whereby a pair of interacting proteins found in one organism is predicted to occur in another organism if the conservation between the two organisms implies that such a move is justified. Additionally, STRING supplements these known interactions with a computational prediction algorithm. All interactions are given a confidence score according to the joint probabilities from the different lines of evidence, correcting for randomly observed interactions. These scores represent an approximation of how likely each association describes a functional relationship between the two proteins that is at least as specific as that between any given pair of proteins that are annotated in the same KEGG pathway (Szklarczyk et al., 2011).
We adopted an analytic strategy that utilized both bioinformatics and statistical methods to identify and validate significant gene–gene interactions with ANK3 in BP cases. First, we queried the STRING protein–protein interaction database using ANK3 as the index to identify all proteins that had biological evidence of interaction with it with at least a high confidence score (defined by STRING to be 0.7000 or greater). Then, we analyzed the GAIN-TGEN dataset for statistical evidence of gene–gene interactions associated with BP between ANK3 and genes encoding the proteins identified by STRING. We used logistic regression to test all possible pair-wise interactions between SNPs in ANK3 and SNPs in each of the STRING-identified genes, with the gene boundaries defined according to the most inclusive RefSeq transcript (hg18) ±10 kb to include potential regulatory regions. The logistic regression models included terms for each SNP entered as allelic dosages representing the estimated number of copies of the minor allele and an interaction term between the two SNPs. The models also included terms to control for study site and the first two principal components (PCs) from a principal components analysis (PCA) using Eigenstrat (Price et al., 2006) with the genome-wide SNP data to assess for population structure among the samples in the dataset. We obtained the Wald statistic for the interaction terms, and then used permutation procedures to evaluate the significance of the interactions. We randomly permuted the case-control labels in the discovery sample to generate 1,000 replicates. We re-ran the SNP–SNP interaction tests in each replicate, and counted how many of the replicates contained SNP–SNP interactions between ANK3 and the STRING-identified genes that reached a higher level of significance than what was observed in the original dataset. The total number of replicates out of 1,000 provided an empirical estimate of the significance of the identified interactions while accounting for multiple testing.
We attempted to replicate any SNP pairs that passed the permuted p-value threshold, based on the 50th most significant of the 1,000 permuted p-values (permuted p-value of 0.05). We used the same logistic regression approach in the analysis of the replication samples as was used in the discovery sample.
A query of the STRING bioinformatics database yielded a total of 16 known proteins that had strong biological evidence (confidence score > 0.7000) for interacting with ANK3. Two of these genes (L1CAM and DMD) are located on chromosome X and were therefore excluded from the analysis, leaving 14 genes of interest (shown in Table 1). We then analyzed the GAIN-TGEN dataset to assess the statistical evidence for interactions between ANK3 and genes encoding each of the 14 identified proteins in association with BP (Table 2). KCNQ2 was the most significantly interacting gene, with a minimum p-value of 3.18 × 10−8, making it the only gene to remain significant after the permutations (permuted p-value = 0.005). In total, 31 SNP pairs between KCNQ2 and ANK3 were significant after permutation.
Table 2. Gene-level minimum p-values for interactions with ANK3: results from discovery analysis of GAIN-TGEN sample.
Interestingly, the most significant SNP pairs clustered within a distinct peak (Figure 1), and were driven by two SNPs in KCNQ2: rs2282150 (an intronic SNP) and rs2297385 (a synonymous coding SNP), both of which are in perfect LD (r2 = D′ = 1.0) with each other. These two SNPs interacted with 16 unique ANK3 SNPs that were all intronic (rs1459730, rs16914687, rs898329, rs11814450, rs12413770, rs7911285, rs17233373, rs7910984, rs7093272, rs16914683, rs12413099, rs16914663, rs16914670, rs12416179, rs16914968, and rs10994334). However, a cluster of these ANK3 SNPs fell within the boundaries of ankyrin repeat domains of the protein, which are known to mediate protein–protein interactions.
Figure 1. 3D plots of the most significant p-values (−log10 p > 3) in the GAIN-TGEN discovery sample. Of these, the 31 SNP pairs which were significant after permutation correction are shown in red and orange dots. RefSeq gene models representing ANK3 and KCNQ2 are shown on the X and Z axis, respectively, and the −log10 (p-value) of the interaction tests are shown on the Y axis.
Motivated by these results, we sought to replicate the interactions between the 31 SNP pairs in two additional GWAS datasets. Of these 31 significant SNP pairs, 28 were significant (p = 0.015–0.048) in the WTCCC sample. The remaining three were suggestive (p = 0.052–0.078). None of these SNP pairs were significant in the German dataset. However, interestingly, the two driving SNPs from KCNQ2 were both involved in significant interactions with five other SNPs in ANK3 (p = 0.033–0.039), suggesting the possibility of allelic heterogeneity. One of these ANK3 SNPs (rs10994406) is just over 10 kb away from the ANK3 SNP (rs10994397) implicated in the recently reported PGC mega-analysis of BP (Psychiatric GWAS Consortium Bipolar Disorder Working Group, 2011), and the other four (rs10994180, rs10740006, rs10821668, and rs10994181) are tightly clustered in a highly conserved region stretching over 9.5 kb (chr10:61,827,709–61,837,348) with an average phyloP of 1.37 and encompassing an unusually large exon (7,811 bp) in one of the ANK3 transcripts (refseq: NM_020987.3). Two of the SNPs are coding (rs10821668 is a mis-sense and rs10740006 is a synonymous SNP) and the four closely straddle a DNAse hypersensitive site that harbors a transcription factor binding region.
Several GWAS of BP have been reported, but conclusive findings have been elusive. Among the most compelling findings to emerge thus far have implicated the gene ANK3 (Ferreira et al., 2008; Kelsoe, 2009; Sklar et al., 2011). However, given the observed effect size on risk of this gene, it likely accounts for only a small portion of BP heritability. Because of its known role in interacting with other membrane bound proteins, we examined whether epistasis with ANK3 might further contribute to susceptibility for BP. We found compelling biological evidence for interactions between ANK3 and KCNQ2 based on a bioinformatics search using STRING, and observed congruent statistical evidence of interactions between these genes in association with BP using GWAS data.
Prior research has demonstrated an intriguing biological relationship between ANK3 and KCNQ2. ANK3 is a member of the ankyrin family of proteins that link integral membrane proteins to the underlying spectrin-actin cytoskeleton. It is expressed in the central and peripheral nervous system, and helps to regulate the distribution of voltage-gated ion channels (Garrido et al., 2003). In line with this role, ANK3 interacts directly with KCNQ2, which is expressed in the brain and forms homomeric and heteromeric voltage-gated potassium channels with another member of the KCNQ family, KCNQ3. These channels are referred to as Kv7.2/Kv7.3. Through this interaction, ANK3 directs the proper localization of the Kv7.2/Kv7.3 channels to the axonal initial segment (AIS) of neurons and nodes of Ranvier (Figure 2). Pan et al. (2006) demonstrated that the concentration of Kv7.2/Kv7.3 at the AIS was abolished in ANK3 knock-out mice, and that a short motif in the C-terminal, common to both Kv7.2 and Kv7.3, was found to be responsible for mediating in vivo interactions with ANK3 and retention of the subunits at the AIS. At the AIS, Kv7.2/Kv7.3 gives rise to the M-current, which serves to stabilize the neuronal resting potential and inhibit repetitive firing of action potentials. By doing so, these channels act as “dampening” components and prevent neuronal hyperactivity (Delmas and Brown, 2005). Interestingly, mutations in KCNQ2 have been shown to cause benign familial neonatal convulsions (BFNC), a rare autosomal dominant inherited form of epilepsy (Singh et al., 1998).
Figure 2. KCNQ2/3 gene products (Kv7.2 and Kv7.3) form a heterotetramer channel at the axon initial segments (AISs) and nodes of Ranvier of neurons. Ankyrin-G (ANK3 gene product) links Kv7.2 and Kv7.3 to the underlying actin-βIV spectrin cortical cytoskeleton and mediates their co-localization via ankyrin-binding motifs at their C-terminal regions.
Several converging lines of evidence suggest that Kv7.2/Kv7.3 channels and their interactions with ANK3 may play a role in the etio-pathogenesis of BP. Increased excitability of neurons, which is suppressed by the Kv7.2/Kv7.3 channel M-currents, may be characteristic of manic and/or hypomanic periods of BP (Xie and Hagan, 1998). In line with this, one study showed that the suppression of the M-current by KCNQ2 dominant-negative mutations in a transgenic mouse model led to hyperexcitability of neurons and morphological changes of the hippocampus, and mediated a notable decline in cognitive function, and behavioral changes corresponding to hyperactivity (Peters et al., 2005). Lithium, the leading mood stabilizer, is thought to work via two mechanisms of action that may also influence the activity of Kv7.2/Kv7.3 channels. Lithium inhibits several enzymes involved in inositol recycling, a crucial step in the synthesis of PIP2, a membrane phospholipid that is essential for Kv7.2 activity and for its regulation by acetylcholine (Brown and Passmore, 2009). It also inhibits GSK3β which is responsible for the phosphorylation of Kv7.2/Kv7.3, and phosphorylation of these channels suppresses the M-currents to promote neuronal hyperexcitability (Borsotto et al., 2007). Interestingly, ezogabine, which is a recently approved antiepileptic drug (AED) that targets Kv7.2/Kv7.3 channels, has been shown to have mood stabilizing properties in animal models (Dencker et al., 2008; Redrobe and Nielsen, 2009; Dencker and Husum, 2010; Kristensen et al., 2012) and in a small pilot study in humans (Amann et al., 2006). Finally, a previous genetic study found evidence of an association between variants in KCNQ2 and BP (Borsotto et al., 2007), and our group has reported genome-wide significant linkage of BP with chromosome 8q24 in a region that harbors KCNQ3 (Avramopoulos et al., 2004).
Despite the biological and statistical evidence reported here in support of a role for interactions between ANK3 and KCNQ2 in the etio-pathogenesis of BP, there are several limitations of the study that merit consideration. Foremost among these is the fact that the same interacting SNP pairs in ANK3 and KCNQ2 were not implicated in the German replication dataset. It is important to remember that the SNPs included in these tests are likely not causal and may only partially tag the actual causal variants responsible for disrupting the gene–gene interactions. As a result, different tagging SNPs may be implicated in the interaction tests in different datasets. Moreover, it is quite possible that there is allelic heterogeneity and different causal variants may contribute to the disruption of the gene–gene interactions in different samples. To this point, it is interesting to note that of the ANK3 SNPs implicated in the German dataset, one was located near the SNP that was most strongly implicated in the PGC mega-analysis of BP, and the other four clustered in another highly interesting region of ANK3 that may also have important functional relevance that needs to be further elucidated.
Another limitation is that we tested for interactions between SNPs in KCNQ2 and the top SNPs in ANK3 implicated in the GWAS by Ferreira et al. (rs10994336) (Ferreira et al., 2008) and in the mega-analysis by the PGC (rs10994397) (Psychiatric GWAS Consortium Bipolar Disorder Working Group, 2011), but none of these were notably significant (data not shown). However, as noted above, a SNP located near the PGC SNP was implicated in one of our replication datasets. It is also likely that the previously identified SNPs in ANK3 merely tag the true causally related variants and are imperfect proxies for them. The causally related variants in these genes need to be identified before we can more accurately characterize the nature of their interaction in susceptibility to BP. Toward this end, we are currently participating in a sequencing study of BP and plan to examine the data from this study in order to further examine these interactions. By identifying the causally related variants, will be able to examine in in vivo and in vitro models the mechanisms by which they disrupt the interactions between ANK3 and KCNQ2 and contribute to the molecular underpinnings of BP.
As far as we are aware, this is the first comprehensive effort to search for evidence of gene–gene interactions with ANK3 using genome-wide association data coupled with bioinformatics tools. We have found intriguing evidence that interactions of ANK3 with KCNQ2 may contribute to susceptibility for BP. These findings warrant further investigation to identify the causally related variants in these genes and characterize how interactions between them might contribute to the etiology of BP. This may be approached through various avenues, including further genetics studies (interaction analyses using sequencing or linkage data), expression studies (transcriptome or proteomics analyses), or epigenetic studies (methylation or histone modification analyses). These results may be further probed experimentally via animal models. If the interactions of ANK3 with KCNQ2 can be confirmed to play a role in the etio-pathogenesis of BP, it would motivate a new avenue of research into a novel therapeutic target for this disease. This would be advantageous because KCNQ2/KCNQ3 channels have been the focus of extensive pharmacologic research in relation to epilepsy, and several compounds that act either as activators or inhibitors of the complex have already been identified and are in various stages of clinical investigation (Miceli et al., 2008).
The authors declare that the research was conducted in the absence of any commercial or financial relationships that could be construed as a potential conflict of interest.
The authors express their profound appreciation to the families who participated in this project, and to the many clinicians who facilitated the referral of participants to the study. Data and biomaterials for the NIMH samples were collected as part of 10 projects that participated in the NIMH Bipolar Disorder Genetics Initiative. From 1991 to 1998, the principal investigators and co-investigators were: Indiana University, Indianapolis, IN, USA U01 MH46282 – John Nurnberger, M.D., Ph.D., Marvin J. Miller, M.D., and Elizabeth S. Bowman, M.D.; Washington University, St. Louis, MO, USA U01 MH46280 – Theodore Reich, M.D., Allison Goate, Ph.D., and John Rice, Ph.D.; Johns Hopkins University, Baltimore, MD, USA U01 MH46274 – J. Raymond DePaulo, Jr., M.D., Sylvia Simpson, M.D., M.P.H., and Colin Stine, Ph.D.; NIMH Intramural Research Program, Clinical Neurogenetics Branch, Bethesda, MD, USA – Elliot Gershon, M.D., Diane Kazuba, B.A., and Elizabeth Maxwell, M. S. W. From 1999 to 2003, the Principal Investigators and Co-Investigators were: Indiana University, Indianapolis, IN, USA R01 MH59545 – John Nurnberger, M.D., Ph.D., Marvin J. Miller, M.D., Elizabeth S. Bowman, M.D., N. Leela Rau, M.D., P. Ryan Moe, M.D., Nalini Samavedy, M.D., Rif El-Mallakh, M.D. (at University of Louisville), Husseini Manji, M.D. (at Wayne State University), Debra A. Glitz, M.D. (at Wayne State University), Eric T. Meyer, M.S., Carrie Smiley, R.N., Tatiana Foroud, Ph.D., Leah Flury, M.S., Danielle M. Dick, Ph.D., and Howard J. Edenberg, Ph.D.; Washington University, St. Louis, MO, USA R01 MH059534, John Rice, Ph.D., Theodore Reich, M.D., Allison Goate, Ph.D., and Laura Bierut, M.D.; Johns Hopkins University, Baltimore, MD, USA R01 MH59533 – Melvin G. McInnis, M.D., J. Raymond DePaulo, Jr., M.D., Dean F. MacKinnon, M.D., Francis M. Mondimore, M.D., James B. Potash, M.D., Peter P. Zandi, Ph.D., Dimitrios Avramopoulos, and Jennifer Payne; University of Pennsylvania, PA, USA R01 MH59553 – Wade Berrettini, M.D., Ph.D.; University of California at Irvine, CA, USA R01 MH60068 – William Byerley, M.D., and Mark Vawter, M.D.; University of Iowa, IA, USA R01 MH059548 – William Coryell, M.D., and Raymond Crowe, M.D.; University of Chicago, Chicago, IL, USA R01 MH59535 – Elliot Gershon, M.D., Judith A. Badner, Ph.D., Francis J. McMahon, M.D., Chunyu Liu, Ph.D., Alan R. Sanders, M.D., Maria Caserta, Stephen Dinwiddie, M.D., Tu Nguyen, Donna Harakal; University of California at San Diego, CA, USA R01 MH59567 – John R. Kelsoe, M.D., Rebecca McKinney, B.A.; Rush University, IL, USA R01 MH059556 – William A. Scheftner, M.D., Howard M. Kravitz, D.O., M.P.H., Diana Marta, B.A., Annette Vaughn-Brown, M.S.N., R.N., and Laurie Bederow, M.A.; NIMH Intramural Research Program, Bethesda, M.D., 1Z01MH002810-01, Francis J. McMahon, M.D., Layla Kassem, PsyD, Sevilla Detera-Wadleigh, Ph.D., Lisa Austin, Ph.D., Dennis L. Murphy, M.D. From 2003 to 2007, the Principal Investigators and Co-Investigators were: Indiana University, Indianapolis, IN, USA R01 MH59545, John Nurnberger, M.D., Ph.D., Marvin J. Miller, M.D., Elizabeth S. Bowman, M.D., N. Leela Rau, M.D., P. Ryan Moe, M.D., Nalini Samavedy, M.D., Rif El-Mallakh, M.D. (at University of Louisville), Husseini Manji, M.D. (at Johnson and Johnson), Debra A. Glitz, M.D. (at Wayne State University), Eric T. Meyer, Ph.D., M.S. (at Oxford University, UK), Carrie Smiley, R.N., Tatiana Foroud, Ph.D., Leah Flury, M.S., Danielle M. Dick, Ph.D (at Virginia Commonwealth University), Howard J. Edenberg, Ph.D.; Washington University, St. Louis, MO, USA R01 MH059534, John Rice, Ph.D., Theodore Reich, M.D., Allison Goate, Ph.D., Laura Bierut, M.D. K02 DA21237; Johns Hopkins University, Baltimore, MD, USA R01 MH59533, Melvin G. McInnis, M.D., J. Raymond DePaulo, Jr., M.D., Dean F. MacKinnon, M.D., Francis M. Mondimore, M.D., James B. Potash, M.D., Peter P. Zandi, Ph.D., Dimitrios Avramopoulos, and Jennifer Payne; University of Pennsylvania, PA, USA R01 MH59553, Wade Berrettini, M.D., Ph.D.; University of California at San Francisco, CA, USA R01 MH60068, William Byerley, M.D., and Sophia Vinogradov, M.D.; University of Iowa, IA, USA R01 MH059548, William Coryell, M.D., and Raymond Crowe, M.D.; University of Chicago, IL, USA R01 MH59535, Elliot Gershon, M.D., Judith A. Badner, Ph.D., Francis J. McMahon, M.D., Chunyu Liu, Ph.D., Alan R. Sanders, M.D., Maria Caserta, Stephen Dinwiddie, M.D., Tu Nguyen, Donna Harakal; University of California at San Diego, CA, USA R01 MH59567, John R. Kelsoe, M.D., Rebecca McKinney, B.A.; Rush University, IL, USA R01 MH059556, William A. Scheftner, M.D., Howard M. Kravitz, D.O., M.P.H., Diana Marta, B.S., Annette Vaughn-Brown, M.S.N., R.N., and Laurie Bederow, M.A.; NIMH Intramural Research Program, Bethesda, MD, USA 1Z01MH002810-01, Francis J. McMahon, M.D., Layla Kassem, Psy.D., Sevilla Detera-Wadleigh, Ph.D., Lisa Austin, Ph.D., Dennis L. Murphy, M.D.; Howard University, William B. Lawson, M.D., Ph.D., Evaristus A. Nwulia, M.D., and Maria Hipolito, M.D. Control subjects from the National Institute of Mental Health Schizophrenia Genetics Initiative (NIMH-GI), data and biomaterials are being collected by the “Molecular Genetics of Schizophrenia II” (MGS-2) collaboration. The investigators and co-investigators are: ENH/Northwestern University, Evanston, IL, USA MH059571, Pablo V. Gejman, M.D. (Collaboration Coordinator; PI), Alan R. Sanders, M.D.; Emory University School of Medicine, Atlanta, GA, USA MH59587, Farooq Amin, M.D. (PI); Louisiana State University Health Sciences Center, New Orleans, LA, USA MH067257, Nancy Buccola APRN, BC, MSN (PI); University of California-Irvine, Irvine, CA, USA MH60870, William Byerley, M.D. (PI); Washington University, St. Louis, MO, USA U01, MH060879, C. Robert Cloninger, M.D. (PI); University of Iowa, Iowa, IA, USA MH59566, Raymond Crowe, M.D. (PI), Donald Black, M.D.; University of Colorado, Denver, CO, USA MH059565, Robert Freedman, M.D. (PI); University of Pennsylvania, Philadelphia, PA, USA MH061675, Douglas Levinson M.D. (PI); University of Queensland, Brisbane, QLD, Australia, MH059588, Bryan Mowry, M.D. (PI); Mt. Sinai School of Medicine, New York, NY, USA MH59586, Jeremy Silverman, Ph.D. (PI). Genome-wide SNP genotyping of the NIMH samples was performed through the Genetic Association Information Network under the direction of the Bipolar Genetics Studies Collaboration. The Principal Investigators and Co-Investigators were: University of California San Diego, La Jolla, CA, USA John R. Kelsoe, M.D. (PI), Tiffany A. Greenwood, Ph.D., Paul D. Shilling, Ph.D., Caroline M. Nievergelt, Ph.D.; Scripps Research Institute, La Jolla, CA, USA: Nicholas Schork, Ph.D. (PI), Erin N. Smith, Ph.D., Cinnamon Bloss, Ph.D.; Indiana University, Bloomington, IN, USA John Nurnberger, M.D. (PI), Howard J. Edenberg, Ph.D., Tatiana Foroud, Ph.D.; University of Chicago, Chicago, IL, USA Elliot Gershon, M.D. (PI), Chunyu Liu, Ph.D., Judith A. Badner, Ph.D.; Rush University Medical Center, Chicago, IL, USA William A. Scheftner, M.D.; Howard University, Washington, DC, USA William B. Lawson, M.D. (PI), Evaristus A. Nwulia, M.D., Maria Hipolito, M.D.; University of Iowa, Iowa City, IA, USA William Coryell, M.D. (PI); Washington University, St. Louis, MO, USA John Rice, Ph.D. (PI); University of California San Francisco, San Francisco, CA, USA William Byerley, M.D. (PI); National Institute of Mental Health, Bethesda, MD, USA Francis J. McMahon, M.D. (PI), Thomas G. Schulze, M.D.; University of Pennsylvania, Philadelphia, PA, USA Wade Berrettini, M.D., Ph.D. (PI); Johns Hopkins University, Baltimore, MD, USA James B. Potash, M.D. (PI), Peter P. Zandi, Ph.D., Pamela Belmonte Mahon, Ph.D.; University of Michigan, Ann Arbor, MI, USA Melvin G. McInnis, M.D. (PI), Sebastian Zöllner, Ph.D.; Translation Genomic Research Institute, Phoenix, AZ, USA David Craig, Ph.D. (PI), Szabolics Szelinger. Data and biomaterials for the subjects in the Wellcome Trust Case-Control Consortium were collected by: University of Aberdeen, Foresterhill, Aberdeen, UK, Gerome Breen, David St. Clair; Birmingham University, Birmingham, UK, Sian Caesar, Katherine Gordon-Smith, Lisa Jones; Cardiff University, Cardiff, UK, Christine Fraser, Elaine K. Green, Detelina Grozeva, Marian L. Hamshere, Peter A. Holmans, Ian R. Jones, George Kirov, Valentina Moskvina, Ivan Nikolov, Michael C. O’Donovan, Michael J. Owen, Nick Craddock; The Institute of Psychiatry, King’s College, London, UK, David A. Collier, Amanda Elkin, Anne Farmer, Richard Williamson, Peter McGuffin; Royal Victoria Infirmary, Newcastle upon Tyne, UK, Allan H. Young, I. Nicol Ferrier; Supported in part by R01 MH079799 (Smoller). The collection of patients (Thomas G. Schulze, Marcella Rietschel) and genotyping (Markus Noethen, Sven Cichon) for the German dataset were supported by the German Federal Ministry of Education and Research within the context of the National Genome Research Network plus (NGFNplus), the MooDS-Net (grant 01GS08144 to Sven Cichon and Markus M. Noethen, grant 01GS08147 to Marcella Rietschel). Work on this study was supported by grants from the NIMH for Jennifer Toolan Judy (T32-MH14592) and Peter P. Zandi (K01-MH072866).
Amann, B., Sterr, A., Vieta, E., Stampfer, R., Walden, J., and Grunze, H. (2006). An exploratory open trial on safety and efficacy of the anticonvulsant retigabine in acute manic patients. J. Clin. Psychopharmacol. 26, 534–536.
Avramopoulos, D., Willour, V. L., Zandi, P. P., Huo, Y., MacKinnon, D. F., Potash, J. B., et al. (2004). Linkage of bipolar affective disorder on chromosome 8q24: follow-up and parametric analysis. Mol. Psychiatry 9, 191–196.
Bennett, V., and Chen, L. (2001). Ankyrins and cellular targeting of diverse membrane proteins to physiological sites. Curr. Opin. Cell Biol. 13, 61–67.
Borsotto, M., Cavarec, L., Bouillot, M., Romey, G., Macciardi, F., Delaye, A., et al. (2007). PP2A-bgamma subunit and KCNQ2 K+ channels in bipolar disorder. Pharmacogenomics J. 7, 123–132.
Brown, D. A., and Passmore, G. M. (2009). Neural KCNQ (Kv7) channels. Br. J. Pharmacol. 156, 1185–1195.
Browning, B. L., and Yu, Z. (2009). Simultaneous genotype calling and haplotype phasing improves genotype accuracy and reduces false-positive associations for genome-wide association studies. Am. J. Hum. Genet. 85, 847–861.
Cordell, H. J. (2002). Epistasis: what it means, what it doesn’t mean, and statistical methods to detect it in humans. Hum. Mol. Genet. 11, 2463–2468.
Craddock, N., and Sklar, P. (2009). Genetics of bipolar disorder: successful start to a long journey. Trends Genet. 25, 99–105.
Delmas, P., and Brown, D. A. (2005). Pathways modulating neural KCNQ/M (Kv7) potassium channels. Nat. Rev. Neurosci 6, 850–862.
Dencker, D., Dias, R., Pedersen, M. L., and Husum, H. (2008). Effect of the new antiepileptic drug retigabine in a rodent model of mania. Epilepsy Behav. 12, 49–53.
Dencker, D., and Husum, H. (2010). Antimanic efficacy of retigabine in a proposed mouse model of bipolar disorder. Behav. Brain Res. 207, 78–83.
Dick, D. M., Foroud, T., Flury, L., Bowman, E. S., Miller, M. J., Rau, N. L., et al. (2003). Genomewide linkage analyses of bipolar disorder: a new sample of 250 pedigrees from the National Institute of Mental Health Genetics Initiative. Am. J. Hum. Genet. 73, 107–114.
Emily, M., Mailund, T., Hein, J., Schauser, L., and Schierup, M. H. (2009). Using biological networks to search for interacting loci in genome-wide association studies. Eur. J. Hum. Genet. 17, 1231–1240.
Fangerau, H., Ohlraun, S., Granath, R. O., Nothen, M. M., Rietschel, M., and Schulze, T. G. (2004). Computer-assisted phenotype characterization for genetic research in psychiatry. Hum. Hered. 58, 122–130.
Ferreira, M. A., O’Donovan, M. C., Meng, Y. A. I., Jones, R., Ruderfer, D. M., Jones, L., et al. (2008). Collaborative genome-wide association analysis supports a role for ANK3 and CACNA1C in bipolar disorder. Nat. Genet. 40, 1056–1058.
Garrido, J. J., Giraud, P., Carlier, E., Fernandes, F., Moussif, A., Fache, M. P., et al. (2003). A targeting motif involved in sodium channel clustering at the axonal initial segment. Science 300, 2091–2094.
Hirschhorn, J. N., Lohmueller, K., Byrne, E., and Hirschhorn, K. (2002). A comprehensive review of genetic association studies. Genet. Med. 4, 45–61.
Jensen, L. J., Kuhn, M., Stark, M., Chaffron, S., Creevey, C., Muller, J., et al. (2009). STRING 8 – a global view on proteins and their functional interactions in 630 organisms. Nucleic Acids Res. 37, D412–6.
Kassem, L., Lopez, V., Hedeker, D., Steele, J., Zandi, P., Bipolar Disorder Consortium NIMH Genetics Initiative, et al. (2006). Familiality of polarity at illness onset in bipolar affective disorder. Am. J. Psychiatry 163, 1754–1759.
Kelsoe, J. (2009). The psychiatric GWAS consortium for bipolar disorder: significant association for multiple genes. Paper Presented at the XVII World Congress of Psychiatric Genetics, San Diego, CA.
Kristensen, L. V., Sandager-Nielsen, K., and Hansen, H. H. (2012). K(V) 7 (KCNQ) channel openers normalize central 2-deoxyglucose uptake in a mouse model of mania and increase prefrontal cortical and hippocampal serine-9 phosphorylation levels of GSK3beta. J. Neurochem. 121, 373–382.
Manolio, T. A., Collins, F. S., Cox, N. J., Goldstein, D. B., Hindorff, L. A., Hunter, D. J., et al. (2009). Finding the missing heritability of complex diseases. Nature 461, 747–753.
McMahon, F. J., Akula, N., Schulze, T. G., Muglia, P., Tozzi, F., Detera-Wadleigh, S. D., et al. (2010). Meta-analysis of genome-wide association data identifies a risk locus for major mood disorders on 3p21.1. Nat. Genet. 42, 128–131.
Miceli, F., Soldovieri, M. V., Martire, M., and Taglialatela, M. (2008). Molecular pharmacology and therapeutic potential of neuronal Kv7-modulating drugs. Curr. Opin. Pharmacol. 8, 65–74.
Moore, J. H., and Williams, S. M. (2002). New strategies for identifying gene-gene interactions in hypertension. Ann. Med. 34, 88–95.
Moore, J. H., and Williams, S. M. (2005). Traversing the conceptual divide between biological and statistical epistasis: systems biology and a more modern synthesis. Bioessays 27, 637–646.
Nurnberger, J. I. Jr., Blehar, M. C., Kaufmann, C. A., York-Cooler, C., Simpson, S. G., Harkavy-Friedman, J., et al. (1994). Diagnostic interview for genetic studies. rationale, unique features, and training. NIMH Genetics Initiative. Arch. Gen. Psychiatry 51, 849–859. discussion 863–864.
Pan, Z., Kao, T., Horvath, Z., Lemos, J., Sul, J. Y., Cranstoun, S. D., et al. (2006). A common ankyrin-G-based mechanism retains KCNQ and NaV channels at electrically active domains of the axon. J. Neurosci. 26, 2599–2613.
Pattin, K. A., and Moore, J. H. (2008). Exploiting the proteome to improve the genome-wide genetic analysis of epistasis in common human diseases. Hum. Genet. 124, 19–29.
Peters, H. C., Hu, H., Pongs, O., Storm, J. F., and Isbrandt, D. (2005). Conditional transgenic suppression of M channels in mouse brain reveals functions in neuronal excitability, resonance and behavior. Nat. Neurosci. 8, 51–60.
Price, A. L., Patterson, N. J., Plenge, R. M., Weinblatt, M. E., Shadick, N. A., and Reich, D. (2006). Principal components analysis corrects for stratification in genome-wide association studies. Nat. Genet. 38, 904–909.
Psychiatric GWAS Consortium Bipolar Disorder Working Group. (2011). Large-scale genome-wide association analysis of bipolar disorder identifies a new susceptibility locus near ODZ4. Nat. Genet. 43, 977–983.
Redrobe, J. P., and Nielsen, A. N. (2009). Effects of neuronal Kv7 potassium channel activators on hyperactivity in a rodent model of mania. Behav. Brain Res. 198, 481–485.
Risch, N. (1990). Linkage strategies for genetically complex traits. I. Multilocus models. Am. J. Hum. Genet. 46, 222–228.
Sanders, A. R., Duan, J., Levinson, D. F., Shi, J., He, D., Hou, C., et al. (2008). No significant association of 14 candidate genes with schizophrenia in a large European ancestry sample: implications for psychiatric genetics. Am. J. Psychiatry 165, 497–506.
Singh, N. A., Charlier, C., Stauffer, D., DuPont, B. R., Leach, R. J., Melis, R., et al. (1998). A novel potassium channel gene, KCNQ2, is mutated in an inherited epilepsy of newborns. Nat. Genet. 18, 25–29.
Sklar, P., Ripke, S., Scott, L. J., Andreassen, O. A., Cichon, S., Craddock, N., et al. (2011). Large-scale genome-wide association analysis of bipolar disorder identifies a new susceptibility locus near ODZ4. Nat. Genet. 43, 977–983.
Smith, E. N., Bloss, C. S., Badner, J. A., Barrett, T., Belmonte, P. L., Berrettini, W., et al. (2009). Genome-wide association study of bipolar disorder in European American and African American individuals. Mol. Psychiatry 14, 755–763.
Szklarczyk, D., Franceschini, A., Kuhn, M., Simonovic, M., Roth, A., Minguez, P., et al. (2011). The STRING database in 2011: functional interaction networks of proteins, globally integrated and scored. Nucleic Acids Res. 39, D561–D568.
Wellcome Trust Case Control Consortium. (2007). Genome-wide association study of 14,000 cases of seven common diseases and 3,000 shared controls. Nature 447, 661–678.
Xie, X., and Hagan, R. M. (1998). Cellular and molecular actions of lamotrigine: possible mechanisms of efficacy in bipolar disorder. Neuropsychobiology 38, 119–130.
Keywords: epistasis, interaction, bipolar disorder, ANK3, KCNQ2, channelopathy, ion channel
Citation: Judy JT, Seifuddin F, Pirooznia M, Mahon PB, The Bipolar Genome Study Consortium, Jancic D, Goes FS, Schulze T, Cichon S, Noethen M, Rietschel M, DePaulo JR, Potash JB and Zandi PP (2013) Converging evidence for epistasis between ANK3 and potassium channel gene KCNQ2 in bipolar disorder. Front. Genet. 4:87. doi: 10.3389/fgene.2013.00087
Received: 28 February 2013; Paper pending published: 22 March 2013;
Accepted: 25 April 2013; Published online: 17 May 2013.
Edited by:
Kathleen D. Askland, Brown University, USAReviewed by:
Radka Kaneva, Medical University – Sofia, BulgariaCopyright: © 2013 Judy, Seifuddin, Pirooznia, Mahon, The Bipolar Genome Study Consortium, Jancic, Goes, Schulze, Cichon, Noethen, Rietschel, DePaulo, Potash and Zandi. This is an open-access article distributed under the terms of the Creative Commons Attribution License, which permits use, distribution and reproduction in other forums, provided the original authors and source are credited and subject to any copyright notices concerning any third-party graphics etc.
*Correspondence: Peter P. Zandi, Department of Mental Health, Bloomberg School of Public Health, Johns Hopkins University, Hampton House, Room 857, 624 North Broadway, Baltimore, MD 21205, USA. e-mail:cHphbmRpQGpoc3BoLmVkdQ==
Disclaimer: All claims expressed in this article are solely those of the authors and do not necessarily represent those of their affiliated organizations, or those of the publisher, the editors and the reviewers. Any product that may be evaluated in this article or claim that may be made by its manufacturer is not guaranteed or endorsed by the publisher.
Research integrity at Frontiers
Learn more about the work of our research integrity team to safeguard the quality of each article we publish.