- 1 Saw Swee Hock School of Public Health, National University of Singapore, Singapore
- 2 Singapore Immunology Network, Agency for Science Technology and Research, Singapore
Since the dawn of the post-genomic era a myriad of novel high-throughput technologies have been developed that are capable of measuring thousands of biological molecules at once, giving rise to various “omics” platforms. These advances offer the unique opportunity to study how individual parts of a biological system work together to produce emerging phenotypes. Today, many research laboratories are moving toward applying multiple omics platforms to analyze the same biological samples. In addition, network information of interacting molecules is being incorporated more and more into the analysis and interpretation of these multiple omics datasets, which provides novel ways to integrate multiple layers of heterogeneous biological information into a single coherent picture. Here, we provide a perspective on how such recent “integrative omics” efforts are likely going to shift biological paradigms once again, and what challenges lie ahead.
Introduction
The first generation of whole-genome sequencing projects have inspired the development of technologies aimed at comprehensively characterizing various types of biological molecules, opening up entirely new fields such as genomics, transcriptomics, proteomics, metabolomics, and so forth. Thanks to these technological advances, one can now routinely sequence the entire genome of an organism to scan for genetic polymorphisms, measure the abundance of genes and their products, map epigenetic modifications and transcriptional regulations, chart the global networks of genetic interactions or protein–protein interactions (PPI), and comprehensively measure sugars, lipids, and metabolites in virtually any biological specimen. The systems-level information provided by each omics platform offers a unique insight into the complexity of a biological system and, as a consequence, scientific discoveries and their clinical applications have immensely benefited from omics data over the past decade (Van de Vijver et al., 2002; Van ’t Veer et al., 2002; Hanash et al., 2008; Stratton et al., 2009; 1000_Genomes_Project_Consortium, 2010; Hudson et al., 2010; Meyerson et al., 2010; Pang et al., 2010; Solit and Mellinghoff, 2010).
Microarrays were among the first omics platforms to be developed, and already since their first appearance it became clear that microarray data would have to be integrated with other levels of biological information in order to allow researchers to see the “big picture” (Kohane et al., 2002). As experimental protocols evolve with declining costs, scientists are now starting to apply multiple omics platforms to analyze the same biological samples (Ideker et al., 2001; Joyce and Palsson, 2006; Zhang et al., 2010). This type of studies will be critically useful for biologists since they can measure molecular changes at multiple levels simultaneously and get one step closer to understanding how biological systems work as a whole, which is one of the primary goals of “systems biology” (Kitano, 2002; Ge et al., 2003; Fukushima et al., 2009). As such, combining multiple omics, or “integrative omics,” holds a great potential to revolutionize the systems-level analysis of complex biological phenomena and several efforts are already ongoing in various directions.
Given the enormous promise of integrative omics, questions regarding how to design experiments and jointly analyze the heterogeneous data are quickly becoming of interest. Indeed, these new technologies generate an unprecedentedly large amount of data and, ironically, the sheer volume makes it difficult to find a reasonable interpretation of the data. Thus the key to successful application will depend on properly designed experiments, statistically sound data analysis, and appropriate interpretation of the data. In this Perspective, we review both challenges and opportunities encountered by systems biologists, bioinformaticians, and statisticians undertaking the exciting and daunting task of integrating multiple heterogeneous omics datasets.
Opportunities of Integrative Omics
Biological Opportunities
Many problems in systems biology can be addressed only by integrating multiple layers of biological information. For example, numerous genetic studies using single-nucleotide polymorphism (SNP) microarrays or high-throughput sequencing often report hundreds of point mutations above the minimal allele frequency as potential disease markers (Carlson et al., 2004; Manolio et al., 2009). However, many of these markers lack the predictive power and fail to reproduce the results across different study populations (Altshuler et al., 2008). This implies that these candidate markers must be further prioritized with additional information such as transcriptional or translational regulation of the gene products affected by the mutations. Accordingly, recent genetics research frequently explores the “genetical genomics” approach (Li and Burmeister, 2005) to integrate population-wide SNP data and transcriptomics data, aiming to identify expression quantitative trait loci (eQTL; Cheung and Spielman, 2009; Cookson et al., 2009; Montgomery et al., 2011). The paired genotype and gene expression data reveals the impact of genetic mutations on transcriptional expression, which is the major mechanism to channel genetic abnormalities into phenotypes. On a similar front, many research articles have reported integration of copy number data and gene expression data to cancer or adaptive evolution studies (Pollack et al., 2002; Chin et al., 2006; Gresham et al., 2008; Rancati et al., 2008). The resulting data explains how various forms of copy number aberration, such as point amplification/deletion, segmental changes, and aneuploidy, induce gene expression changes (Bussey et al., 2006; Stranger et al., 2007). Besides the integration of genomic datasets, advances in tandem mass spectrometry have gradually allowed us to integrate transcriptomics data with quantitative proteomics data (Griffin et al., 2002; Cox et al., 2005; Lu et al., 2007; Fournier et al., 2010; Pavelka et al., 2010), where proteomics data provide direct information to assess the impact of transcriptional changes on the gene products.
So far we reviewed the opportunities when the same genes are profiled at different levels of the primary omics. However, there exists additional network information generated using other high-throughput technologies, where the correlation between interacting molecules can be explicitly modeled. These include various assays for screening PPI (Rual et al., 2005; Gingras et al., 2007; Costanzo et al., 2010), protein–DNA interaction data for mapping transcriptional regulation and epigenetics (Ren et al., 2000; Johnson et al., 2007), post-transcriptional regulation mediated by microRNAs (Bartel, 2009; Hafner et al., 2010), and so forth. Using this information, the association between different molecules, and the lack thereof, can be adjusted for other interacting molecules causally linked across available omics datasets. For instance, transcriptomics and metabolomics data were integrated to identify clusters of genes and metabolites that were coordinately modulated in response to specific nutritional stresses in the model plant Arabidopsis thaliana (Hirai et al., 2004). In addition, transcriptomics data were coupled with PPI networks to determine under which circumstances protein hubs are co-expressed with their respective interacting partners (Taylor et al., 2009) and to use joint expression levels of genes belonging to interaction subnetworks to establish more predictive breast cancer biomarkers (Chuang et al., 2007). Transcriptomics data were also combined with protein–DNA interaction data to infer gene regulatory networks (Lin et al., 2009; Ouyang et al., 2009).
Statistical Opportunities
Integrative omics also opens an opportunity for improved statistical analysis. For one, parallel omics datasets can help implement procedures to infer missing data. Many omics platforms are known to be subject to missing observations due to lagging depth, exemplified by the poor coverage of next-generation sequencing (NGS) in repeat-rich regions and the faltering peptide identification of tandem mass spectrometry in low-abundance proteins. Some transcriptomic platforms such as microarrays are also subject to the limitation that only a fixed form of transcripts can be measured while other isoforms present in the sample go undetected. By generating both transcriptomic and proteomic data, however, one can perform statistical inference on the missing observations in one platform using the observations in the other platform since the two data are expected to be correlated within the same biological sample. Recent endeavors to improve peptide sequencing in tandem mass spectrometry (MS/MS) using the parallel transcriptomic data are good examples of this kind (Ramakrishnan et al., 2009; Ning and Nesvizhskii, 2010), but a more sophisticated treatment of missing data using external sources is yet to be developed.
Another important problem in the omics data analysis is the control or estimation of false positives and false negatives, which are incurred when many statistical decisions are to be made simultaneously, i.e., the multiple testing problem. As simultaneous hypothesis testing typically leads to excessively many selections in omics data, currently existing multiple hypothesis testing methods are geared toward controlling the number of false positives, as evidenced by the development of false discovery rate estimation procedures (Benjamini and Hochberg, 1995; Efron et al., 2001). Although these procedures are applicable to the analysis of a single omics platform, the methods are easily generalizable to the multivariate cases for more sophisticated hypothesis testing when the data are available from more than a single omics platform. Suppose that differential expression is tested at the mRNA and protein level simultaneously. Then the hypothesis testing can be performed using bivariate statistics, which is expected to be more powerful than using two independent univariate statistics, since the correlation between the two dataset can be explicitly accounted for. In addition, the added complexity in the joint testing allows differentiation of the genes differentially expressed at both levels versus the genes regulated at either one of the two levels only, providing additional information to infer the underlying regulatory mechanism. Unfortunately, such routines using correlated statistics have rarely been implemented in the integrative omics data analysis so far, but we can envision that as the number of such integrated datasets will increase, so will the level of sophistication in the statistical analysis.
More importantly, the ultimate statistical opportunity in the integrative omics data is the possibility for systems-level probabilistic modeling of multiple data types. In practice, one may well perform a crude form of integrative analysis, i.e., analyze each type of molecular level separately and aggregate the results in a post hoc manner (Figure 1A). This approach, however, fails to capitalize on the power of the correlated data, especially for detecting weak yet consistent signals from multiple data sources (Ideker et al., 2011). Hence one can start using a slightly more sophisticated approach where the data measured at different molecular levels are modeled using multivariate probability models (Figure 1B). As the bivariate example showed above, incorporating data from multiple molecular levels can strengthen the statistical power, since the effects we aim to measure at one molecular level can be adjusted by the data at the other levels. Furthermore, the new threads of network-level information that is becoming increasingly available – such as transcriptional regulatory networks, genetic interaction networks, PPI networks, signal transduction pathways and metabolic networks – allows computational biologists to integrate omics datasets at the level of nodes and edges of biological networks (Figure 1C) and to move beyond the statistical analysis under the assumption of full independence among the different molecules. For instance, versatile statistical techniques such as graphical models can be used in conjunction with the experimentally validated networks, which provides the underlying backbone of the correlation structure. Such models give an efficient probabilistic representation of the complex, systems-wide molecular profiles and considerably improve the statistical power in the analysis.
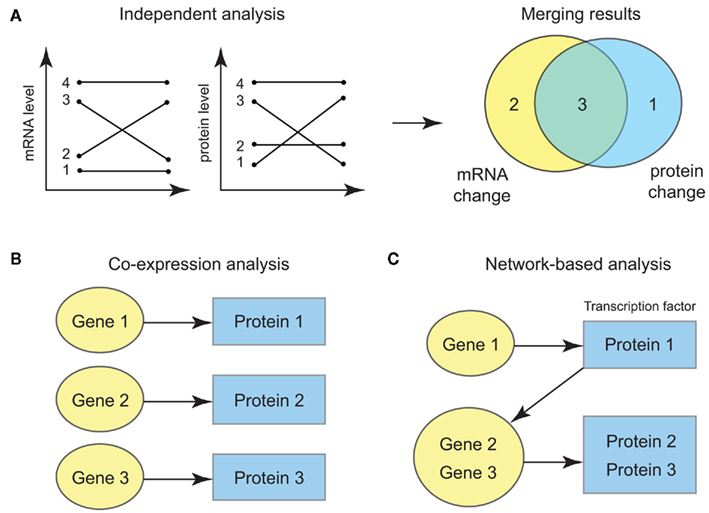
Figure 1. (A) Independent analysis of each data for each gene and protein. Significant findings are filtered at each data type and aggregated by looking at the overlap of the results. (B) Joint modeling of the bivariate distribution of transcript and protein level data for each gene. (C) Joint modeling incorporating the network information such as the interaction between transcription factors and their targets.
Challenges of Integrative Omics
Bioinformatics Challenges
The first problem bioinformaticians face when asked to integrate, for instance, a transcriptomics dataset and a proteomics dataset is how to map transcript identifiers to protein identifiers. If the one-gene-one-protein hypothesis still holds relatively well in prokaryotes and some lower eukaryotes, the same is certainly not true in higher organisms: genes often encode multiple transcripts by means of alternative splicing (Graveley, 2001) and transcripts can be translated into multiple protein isoforms by means of alternative translation initiation sites (Cavener and Ray, 1991) and post-translational modifications (Mann and Jensen, 2003). A partial solution to this problem is provided by genome-centric databases such as EnsEMBL (Hubbard et al., 2002), protein-centric databases such as UniProt (Apweiler et al., 2004) or more general-purposes web services such as Babelomics (Al-Shahrour et al., 2005), that provide coherent mappings between gene, transcript, and protein identifiers. The challenge becomes even more daunting when one starts to venture outside the central dogma of molecular biology and attempts to integrate a transcriptomics or proteomics dataset with a metabolomic, glycomic, or lipidomic dataset. Here, one could take advantage of the knowledge of metabolic networks to map enzymes involved in the synthesis or chemical conversion of metabolites (e.g., as provided by KEGG, Kanehisa and Goto, 2000, or Reactome, Joshi-Tope et al., 2005) to establish links between the two types of datasets (Antonov et al., 2010). To this end, the systems biology markup language (SBML) represents one of the first and most successful efforts in developing a unified language to represent complex models of interacting biological molecules (Hucka et al., 2003) and has been widely implemented by several software tools. However, only a fraction of the genes in a genome typically encode metabolic enzymes, the rest being structural, regulatory, or signal transduction proteins. Unfortunately, it is not immediately obvious how to close these gaps. It is thus expected that integrative omics data analysis methods will have to deal with the existence of “orphan” molecules that cannot be directly mapped between the two types of datasets.
Another bioinformatic issue is the existence of heterogeneous repositories of primary data sources. Due to the different nature of omics platforms, databases of microarray, NGS, proteomics, or metabolomics experiments have been designed according to different schemes. While it is true that each omics domain has developed its own standards (such as MIAME, Brazma et al., 2001, and MAGE-ML, Spellman et al., 2002, for microarray data, or mzXML, Pedrioli et al., 2004, and HUPO-PSI for proteomics data, Orchard et al., 2003), the lack of well-defined data standards and of standardized nomenclature across different data repositories makes the coherent retrieval and assembly of integrated datasets a non-trivial task. One way to address this issue is the development of so-called “data warehouses,” in which a significant effort is being put in by developers a priori to store and integrate heterogeneous primary databases into a coherent scheme by making use of intermediate abstraction layers between the raw data layer and the user access layer (Rhodes et al., 2004; Chen et al., 2010). An alternative promising approach to data integration in life sciences is offered by Semantic Web technologies (Splendiani et al., 2011). These technologies enable an immediate “connection” between data, which can be easily queried across different databases. At the same time they allow a precise characterization of the “semantics” of the data, i.e., which entities are represented, and which are their relations (Berners-Lee and Hendler, 2001). Such semantic characterization can then provide an integration of information across different databases, which can easily cope with a variety of rapidly evolving data sources and types (Cheung et al., 2005; Smith et al., 2007). How widely this technology will be adopted is likely tied to how well developers of primary omics databases will implement such data representation methods.
Statistical Challenges
In addition to the bioinformatics issues, there are important statistical challenges in the integrative omics analysis. As we build more complex models such as multivariate or inter-molecular models, we must revisit some limitations that had plagued the single-source omics data analysis. First, it is likely that the number of biological samples analyzed in a typical integrative study will remain limited, e.g., on the order of a few tens in case–control studies and at most several replicate experiments per comparative condition in the studies using cell lines. To address this limitation, one can utilize efficient statistical methods such as hierarchical models, which are capable of pooling statistical information across different molecular levels (Parmigiani et al., 2002; Sharpf et al., 2009; Ji and Liu, 2010). Second, as we consider modeling the correlations among an increasing number of molecules in the statistical model, the model parameter space will expand in a computationally intractable manner and the limiting sample size will likely lead to over-fitting of models even further. As such, although advanced statistical methods for model selection (e.g., regularization Tibshirani, 1996) may facilitate the choice of predictive models, it must be reminded that there exists a certain trade-off between the gain in power from the added complexity and the loss in specificity due to a poor model fit, where the latter is mainly determined by experimental design issues such as the sample size. Therefore, when complex models are employed, the interaction between model complexity and experimental design factors must be thoroughly evaluated in terms of strengthening sensitivity–specificity profile and reproducibility of results. In sum, it is necessary to find the right balance between complexity and model sparsity to deliver the most reproducible system-wide models from multi-layered omics data.
Conclusion
As it is becoming increasingly clear that integrating multiple omics dataset allows researchers to explore previously uncharted territories describing the functioning of biological systems, more advanced data analysis methods will be required to fully translate this enormous wave of information into biological knowledge. Will the field of bioinformatics and computational biology be able to keep the pace with the exponential development of omics technologies? While it is currently difficult to predict whether this gap will eventually be filled, we argue that if careful statistical considerations are taken into account already at the experimental design phase of a multi-omics project, then there is an opportunity to build rigorous systems-level statistical models that fully take advantage of the interdependent workings of biological molecules. Finally, to foster further advancement of the field, it will be critical to build integrated multi-omics statistical models that are both reusable and easily extendable by other researchers.
Conflict of Interest Statement
The authors declare that the research was conducted in the absence of any commercial or financial relationships that could be construed as a potential conflict of interest.
Acknowledgments
Authors are grateful to Andrea Splendiani for inputs on Semantic Web technologies and to Giulia Rancati for critical reading of the manuscript. Hyungwon Choi is supported in part by NUS YLLSOM grant. Norman Pavelka is funded by an A*STAR Investigatorship award.
References
1000_Genomes_Project_Consortium. (2010). A map of human genome variation from population-scale sequencing. Nature 467, 1061–1073.
Al-Shahrour, F., Minguez, P., Vaquerizas, J. M., Conde, L., and Dopazo, J. (2005). BABELOMICS: a suite of web tools for functional annotation and analysis of groups of genes in high-throughput experiments. Nucleic Acids Res. 33, W460–W464.
Altshuler, D., Daly, M. J., and Lander, E. S. (2008). Genetic mapping in human disease. Science 322, 881–888.
Antonov, A. V., Schmidt, E. E., Dietmann, S., Krestyaninova, M., and Hermjakob, H. (2010). R spider: a network-based analysis of gene lists by combining signaling and metabolic pathways from Reactome and KEGG databases. Nucleic Acids Res. 38, W78–W83.
Apweiler, R., Bairoch, A., Wu, C. H., Barker, W. C., Boeckmann, B., Ferro, S., Gasteiger, E., Huang, H., Lopez, R., Magrane, M., Martin, M. J., Natale, D. A., O’donovan, C., Redaschi, N., and Yeh, L. S. (2004). UniProt: the universal protein knowledgebase. Nucleic Acids Res. 32, D115–D119.
Benjamini, Y., and Hochberg, Y. (1995). Controlling the false discovery rate: a practical and powerful approach to multiple testing. J. R. Stat. Soc. B Stat. Methodol. 57, 289–300.
Brazma, A., Hingamp, P., Quackenbush, J., Sherlock, G., Spellman, P., Stoeckert, C., Aach, J., Ansorge, W., Ball, C. A., Causton, H. C., Gaasterland, T., Glenisson, P., Holstege, F. C., Kim, I. F., Markowitz, V., Matese, J. C., Parkinson, H., Robinson, A., Sarkans, U., Schulze-Kremer, S., Stewart, J., Taylor, R., Vilo, J., and Vingron, M. (2001). Minimum information about a microarray experiment (MIAME)-toward standards for microarray data. Nat. Genet. 29, 365–371.
Bussey, K. J., Chin, K., Lababidi, S., Reimers, M., Reinhold, W. C., Kuo, W. L., Gwadry, F., Kouros-Mehr, H., Fridlyand, J., Jain, A., Collins, C., Nishizuka, S., Tonon, G., Roschke, A., Gehlhaus, K., Kirsch, I., Scudiero, D. A., Gray, J. W., and Weinstein, J. N. (2006). Integrating data on DNA copy number with gene expression levels and drug sensitivities in the NCI-60 cell line panel. Mol. Cancer Ther. 5, 853–867.
Carlson, C. S., Eberle, M. A., Kruglyak, L., and Nickerson, D. A. (2004). Mapping complex disease loci in whole-genome association studies. Nature 429, 446–452.
Cavener, D. R., and Ray, S. C. (1991). Eukaryotic start and stop translation sites. Nucleic Acids Res. 19, 3185–3192.
Chen, C., Mcgarvey, P. B., Huang, H., and Wu, C. H. (2010). Protein bioinformatics infrastructure for the integration and analysis of multiple high-throughput “omics” data. Adv. Bioinformatics 423589. doi:10.1371/journal.pone.0006589
Cheung, K. H., Yip, K. Y., Smith, A., Deknikker, R., Masiar, A., and Gerstein, M. (2005). YeastHub: a semantic web use case for integrating data in the life sciences domain. Bioinformatics 21(Suppl. 1), i85–i96.
Cheung, V. G., and Spielman, R. S. (2009). Genetics of human gene expression: mapping DNA variants that influence gene expression. Nat. Rev. Genet. 10, 595–604.
Chin, K., Devries, S., Fridlyand, J., Spellman, P. T., Roydasgupta, R., Kuo, W. L., Lapuk, A., Neve, R. M., Qian, Z., Ryder, T., Chen, F., Feiler, H., Tokuyasu, T., Kingsley, C., Dairkee, S., Meng, Z., Chew, K., Pinkel, D., Jain, A., Ljung, B. M., Esserman, L., Albertson, D. G., Waldman, F. M., and Gray, J. W. (2006). Genomic and transcriptional aberrations linked to breast cancer pathophysiologies. Cancer Cell 10, 529–541.
Chuang, H. Y., Lee, E., Liu, Y. T., Lee, D., and Ideker, T. (2007). Network-based classification of breast cancer metastasis. Mol. Syst. Biol. 3, 140.
Cookson, W., Liang, L., Abecasis, G., Moffatt, M., and Lathrop, M. (2009). Mapping complex disease traits with global gene expression. Nat. Rev. Genet. 10, 184–194.
Costanzo, M., Baryshnikova, A., Bellay, J., Kim, Y., Spear, E. D., Sevier, C. S., Ding, H., Koh, J. L., Toufighi, K., Mostafavi, S., Prinz, J., St Onge, R. P., Vandersluis, B., Makhnevych, T., Vizeacoumar, F. J., Alizadeh, S., Bahr, S., Brost, R. L., Chen, Y., Cokol, M., Deshpande, R., Li, Z., Lin, Z. Y., Liang, W., Marback, M., Paw, J., San Luis, B. J., Shuteriqi, E., Tong, A. H., Van Dyk, N., Wallace, I. M., Whitney, J. A., Weirauch, M. T., Zhong, G., Zhu, H., Houry, W. A., Brudno, M., Ragibizadeh, S., Papp, B., Pal, C., Roth, F. P., Giaever, G., Nislow, C., Troyanskaya, O. G., Bussey, H., Bader, G. D., Gingras, A. C., Morris, Q. D., Kim, P. M., Kaiser, C. A., Myers, C. L., Andrews, B. J., and Boone, C. (2010). The genetic landscape of a cell. Science 327, 425–431.
Cox, B., Kislinger, T., and Emili, A. (2005). Integrating gene and protein expression data: pattern analysis and profile mining. Methods 35, 303–314.
Efron, B., Tibshirani, R., Storey, J., and Tusher, V. (2001). Empirical Bayes analysis of a microarray experiment. J. Am. Stat. Assoc. 96, 1151–1160.
Fournier, M. L., Paulson, A., Pavelka, N., Mosley, A. L., Gaudenz, K., Bradford, W. D., Glynn, E., Li, H., Sardiu, M. E., Fleharty, B., Seidel, C., Florens, L., and Washburn, M. P. (2010). Delayed correlation of mRNA and protein expression in rapamycin-treated cells and a role for Ggc1 in cellular sensitivity to rapamycin. Mol. Cell. Proteomics 9, 271–284.
Fukushima, A., Kusano, M., Redestig, H., Arita, M., and Saito, K. (2009). Integrated omics approaches in plant systems biology. Curr. Opin. Chem. Biol. 13, 532–538.
Ge, H., Walhout, A. J., and Vidal, M. (2003). Integrating “omic” information: a bridge between genomics and systems biology. Trends Genet. 19, 551–560.
Gingras, A. C., Gstaiger, M., Raught, B., and Aebersold, R. (2007). Analysis of protein complexes using mass spectrometry. Nat. Rev. Mol. Cell Biol. 8, 645–654.
Graveley, B. R. (2001). Alternative splicing: increasing diversity in the proteomic world. Trends Genet. 17, 100–107.
Gresham, D., Desai, M. M., Tucker, C. M., Jenq, H. T., Pai, D. A., Ward, A., Desevo, C. G., Botstein, D., and Dunham, M. J. (2008). The repertoire and dynamics of evolutionary adaptations to controlled nutrient-limited environments in yeast. PLoS Genet. 4, e1000303. doi:10.1371/journal.pgen.1000303
Griffin, T. J., Gygi, S. P., Ideker, T., Rist, B., Eng, J., Hood, L., and Aebersold, R. (2002). Complementary profiling of gene expression at the transcriptome and proteome levels in Saccharomyces cerevisiae. Mol. Cell. Proteomics 1, 323–333.
Hafner, M., Landthaler, M., Burger, L., Khorshid, M., Hausser, J., Berninger, P., Rothballer, A., Ascano, M. Jr., Jungkamp, A. C., Munschauer, M., Ulrich, A., Wardle, G. S., Dewell, S., Zavolan, M., and Tuschl, T. (2010). Transcriptome-wide identification of RNA-binding protein and microRNA target sites by PAR-CLIP. Cell 141, 129–141.
Hanash, S. M., Pitteri, S. J., and Faca, V. M. (2008). Mining the plasma proteome for cancer biomarkers. Nature 452, 571–579.
Hirai, M. Y., Yano, M., Goodenowe, D. B., Kanaya, S., Kimura, T., Awazuhara, M., Arita, M., Fujiwara, T., and Saito, K. (2004). Integration of transcriptomics and metabolomics for understanding of global responses to nutritional stresses in Arabidopsis thaliana. Proc. Natl. Acad. Sci. U.S.A. 101, 10205–10210.
Hubbard, T., Barker, D., Birney, E., Cameron, G., Chen, Y., Clark, L., Cox, T., Cuff, J., Curwen, V., Down, T., Durbin, R., Eyras, E., Gilbert, J., Hammond, M., Huminiecki, L., Kasprzyk, A., Lehvaslaiho, H., Lijnzaad, P., Melsopp, C., Mongin, E., Pettett, R., Pocock, M., Potter, S., Rust, A., Schmidt, E., Searle, S., Slater, G., Smith, J., Spooner, W., Stabenau, A., Stalker, J., Stupka, E., Ureta-Vidal, A., Vastrik, I., and Clamp, M. (2002). The Ensembl genome database project. Nucleic Acids Res. 30, 38–41.
Hucka, M., Finney, A., Sauro, H. M., Bolouri, H., Doyle, J. C., Kitano, H., Arkin, A. P., Bornstein, B. J., Bray, D., Cornish-Bowden, A., Cuellar, A. A., Dronov, S., Gilles, E. D., Ginkel, M., Gor, V., Goryanin, I. I., Hedley, W. J., Hodgman, T. C., Hofmeyr, J. H., Hunter, P. J., Juty, N. S., Kasberger, J. L., Kremling, A., Kummer, U., Le Novere, N., Loew, L. M., Lucio, D., Mendes, P., Minch, E., Mjolsness, E. D., Nakayama, Y., Nelson, M. R., Nielsen, P. F., Sakurada, T., Schaff, J. C., Shapiro, B. E., Shimizu, T. S., Spence, H. D., Stelling, J., Takahashi, K., Tomita, M., Wagner, J., and Wang, J. (2003). The systems biology markup language (SBML): a medium for representation and exchange of biochemical network models. Bioinformatics 19, 524–531.
Hudson, T. J., Anderson, W., Artez, A., Barker, A. D., Bell, C., Bernabe, R. R., Bhan, M. K., Calvo, F., Eerola, I., Gerhard, D. S., Guttmacher, A., Guyer, M., Hemsley, F. M., Jennings, J. L., Kerr, D., Klatt, P., Kolar, P., Kusada, J., Lane, D. P., Laplace, F., Youyong, L., Nettekoven, G., Ozenberger, B., Peterson, J., Rao, T. S., Remacle, J., Schafer, A. J., Shibata, T., Stratton, M. R., Vockley, J. G., Watanabe, K., Yang, H., Yuen, M. M., Knoppers, B. M., Bobrow, M., Cambon-Thomsen, A., Dressler, L. G., Dyke, S. O., Joly, Y., Kato, K., Kennedy, K. L., Nicolas, P., Parker, M. J., Rial-Sebbag, E., Romeo-Casabona, C. M., Shaw, K. M., Wallace, S., Wiesner, G. L., Zeps, N., Lichter, P., Biankin, A. V., Chabannon, C., Chin, L., Clement, B., De Alava, E., Degos, F., Ferguson, M. L., Geary, P., Hayes, D. N., Johns, A. L., Kasprzyk, A., Nakagawa, H., Penny, R., Piris, M. A., Sarin, R., Scarpa, A., Van De Vijver, M., Futreal, P. A., Aburatani, H., Bayes, M., Botwell, D. D., Campbell, P. J., Estivill, X., Grimmond, S. M., Gut, I., Hirst, M., Lopez-Otin, C., Majumder, P., Marra, M., Mcpherson, J. D., Ning, Z., Puente, X. S., Ruan, Y., Stunnenberg, H. G., Swerdlow, H., Velculescu, V. E., Wilson, R. K., Xue, H. H., Yang, L., Spellman, P. T., Bader, G. D., Boutros, P. C., Flicek, P., Getz, G., Guigo, R., Guo, G., Haussler, D., Heath, S., Hubbard, T. J., Jiang, T., Jones, S. M., Li, Q., López-Bigas, N., Luo, R., Muthuswamy, L., Ouellette, B. F., Pearson, J. V., Puente, X. S., Quesada, V., Raphael, B. J., Sander, C., Shibata, T., Speed, T. P., Stein, L. D., Stuart, J. M., Teague, J. W., Totoki, Y., Tsunoda, T., Valencia, A., Wheeler, D. A., Wu, H., Zhao, S., Zhou, G., Stein, L. D., Guigó, R., Hubbard, T. J., Joly, Y., Jones, S. M., Kasprzyk, A., Lathrop, M., López-Bigas, N., Ouellette, B. F., Spellman, P. T., Teague, J. W., Thomas, G., Valencia, A., Yoshida, T., Kennedy, K. L., Axton, M., Dyke, S. O., Futreal, P. A., Gerhard, D. S., Gunter, C., Guyer, M., Hudson, T. J., McPherson, J. D., Miller, L. J., Ozenberger, B., Shaw, K. M., Kasprzyk, A., Stein, L. D., Zhang, J., Haider, S. A., Wang, J., Yung, C. K., Cros, A., Liang, Y., Gnaneshan, S., Guberman, J., Hsu, J., Bobrow, M., Chalmers, D. R., Hasel, K. W., Joly, Y., Kaan, T. S., Kennedy, K. L., Knoppers, B. M., Lowrance, W. W., Masui, T., Nicolás, P., Rial-Sebbag, E., Rodriguez, L. L., Vergely, C., Yoshida, T., Grimmond, S. M., Biankin, A. V., Bowtell, D. D., Cloonan, N., DeFazio, A., Eshleman, J. R., Etemadmoghadam, D., Gardiner, B. B., Kench, J. G., Scarpa, A., Sutherland, R. L., Tempero, M. A., Waddell, N. J., Wilson, P. J., McPherson, J. D., Gallinger, S., Tsao, M. S., Shaw, P. A., Petersen, G. M., Mukhopadhyay, D., Chin, L., DePinho, R. A., Thayer, S., Muthuswamy, L., Shazand, K., Beck, T., Sam, M., Timms, L., Ballin, V., Lu, Y., Ji, J., Zhang, X., Chen, F., Hu, X., Zhou, G., Yang, Q., Tian, G., Zhang, L., Xing, X., Li, X., Zhu, Z., Yu, Y., Yu, J., Yang, H., Lathrop, M., Tost, J., Brennan, P., Holcatova, I., Zaridze, D., Brazma, A., Egevard, L., Prokhortchouk, E., Banks, R. E., Uhlén, M., Cambon-Thomsen, A., Viksna, J., Ponten, F., Skryabin, K., Stratton, M. R., Futreal, P. A., Birney, E., Borg, A., Børresen-Dale, A. L., Caldas, C., Foekens, J. A., Martin, S., Reis-Filho, J. S., Richardson, A. L., Sotiriou, C., Stunnenberg, H. G., Thoms, G., van de Vijver, M., van’t Veer, L., Calvo, F., Birnbaum, D., Blanche, H., Boucher, P., Boyault, S., Chabannon, C., Gut, I., Masson-Jacquemier, J. D., Lathrop, M., Pauporté, I., Pivot, X., Vincent-Salomon, A., Tabone, E., Theillet, C., Thomas, G., Tost, J., Treilleux, I., Calvo, F., Bioulac-Sage, P., Clément, B., Decaens, T., Degos, F., Franco, D., Gut, I., Gut, M., Heath, S., Lathrop, M., Samuel, D., Thomas, G., Zucman-Rossi, J., Lichter, P., Eils, R., Brors, B., Korbel, J. O., Korshunov, A., Landgraf, P., Lehrach, H., Pfister, S., Radlwimmer, B., Reifenberger, G., Taylor, M. D., von Kalle, C., Majumder, P. P., Sarin, R., Rao, T. S., Bhan, M. K., Scarpa, A., Pederzoli, P., Lawlor, R. A., Delledonne, M., Bardelli, A., Biankin, A. V., Grimmond, S. M., Gress, T., Klimstra, D., Zamboni, G., Shibata, T., Nakamura, Y., Nakagawa, H., Kusada, J., Tsunoda, T., Miyano, S., Aburatani, H., Kato, K., Fujimoto, A., Yoshida, T., Campo, E., López-Otín, C., Estivill, X., Guigó, R., de Sanjosé, S., Piris, M. A., Montserrat, E., González-Díaz, M., Puente, X. S., Jares, P., Valencia, A., Himmelbauer, H., Quesada, V., Bea, S., Stratton, M. R., Futreal, P. A., Campbell, P. J., Vincent-Salomon, A., Richardson, A. L., Reis-Filho, J. S., van de Vijver, M., Thomas, G., Masson-Jacquemier, J. D., Aparicio, S., Borg, A., Børresen-Dale, A. L., Caldas, C., Foekens, J. A., Stunnenberg, H. G., van’t Veer, L., Easton, D. F., Spellman, P. T., Martin, S., Barker, A. D., Chin, L., Collins, F. S., Compton, C. C., Ferguson, M. L., Gerhard, D. S., Getz, G., Gunter, C., Guttmacher, A., Guyer, M., Hayes, D. N., Lander, E. S., Ozenberger, B., Penny, R., Peterson, J., Sander, C., Shaw, K. M., Speed, T. P., Spellman, P. T., Vockley, J. G., Wheeler, D. A., Wilson, R. K., Hudson, T. J., Chin, L., Knoppers, B. M., Lander, E. S., Lichter, P., Stein, L. D., Stratton, M. R., Anderson, W., Barker, A. D., Bell, C., Bobrow, M., Burke, W., Collins, F. S., Compton, C. C., DePinho, R. A., Easton, D. F., Futreal, P. A., Gerhard, D. S., Green, A. R., Guyer, M., Hamilton, S. R., Hubbard, T. J., Kallioniemi, O. P., Kennedy, K. L., Ley, T. J., Liu, E. T., Lu, Y., Majumder, P., Marra, M., Ozenberger, B., Peterson, J., Schafer, A. J., Spellman, P. T., Stunnenberg, H. G., Wainwright, B. J., Wilson, R. K., and Yang, H. (2010). International network of cancer genome projects. Nature 464, 993–998.
Ideker, T., Dutkowski, J., and Hood, L. (2011). Boosting signal-to-noise in complex biology: prior knowledge is power. Cell 144, 860–863.
Ideker, T., Thorsson, V., Ranish, J. A., Christmas, R., Buhler, J., Eng, J. K., Bumgarner, R., Goodlett, D. R., Aebersold, R., and Hood, L. (2001). Integrated genomic and proteomic analyses of a systematically perturbed metabolic network. Science 292, 929–934.
Ji, H., and Liu, X. S. (2010). Analyzing omics data using hierarchical models. Nat. Biotechnol. 28, 337–340.
Johnson, D. S., Mortazavi, A., Myers, R. M., and Wold, B. (2007). Genome-wide mapping of in vivo protein-DNA interactions. Science 316, 1497–1502.
Joshi-Tope, G., Gillespie, M., Vastrik, I., D’eustachio, P., Schmidt, E., De Bono, B., Jassal, B., Gopinath, G. R., Wu, G. R., Matthews, L., Lewis, S., Birney, E., and Stein, L. (2005). Reactome: a knowledgebase of biological pathways. Nucleic Acids Res. 33, D428–D432.
Joyce, A. R., and Palsson, B. O. (2006). The model organism as a system: integrating “omics” data sets. Nat. Rev. Mol. Cell Biol. 7, 198–210.
Kanehisa, M., and Goto, S. (2000). KEGG: Kyoto encyclopedia of genes and genomes. Nucleic Acids Res. 28, 27–30.
Kohane, I. S., Kho, A., and Butte, A. J. (2002). Microarrays for an Integrative Genomics. Cambridge: MIT Press.
Li, J., and Burmeister, M. (2005). Genetical genomics: combining genetics with gene expression analysis. Hum. Mol. Genet. 2, R163–R169.
Lin, B., Wang, J., Hong, X., Yan, X., Hwang, D., Cho, J. H., Yi, D., Utleg, A. G., Fang, X., Schones, D. E., Zhao, K., Omenn, G. S., and Hood, L. (2009). Integrated expression profiling and ChIP-seq analyses of the growth inhibition response program of the androgen receptor. PLoS ONE 4, e6589.
Lu, P., Vogel, C., Wang, R., Yao, X., and Marcotte, E. M. (2007). Absolute protein expression profiling estimates the relative contributions of transcriptional and translational regulation. Nat. Biotechnol. 25, 117–124.
Mann, M., and Jensen, O. N. (2003). Proteomic analysis of post-translational modifications. Nat. Biotechnol. 21, 255–261.
Manolio, T. A., Collins, F. S., Cox, N. J., Goldstein, D. B., Hindorff, L. A., Hunter, D. J., Mccarthy, M. I., Ramos, E. M., Cardon, L. R., Chakravarti, A., Cho, J. H., Guttmacher, A. E., Kong, A., Kruglyak, L., Mardis, E., Rotimi, C. N., Slatkin, M., Valle, D., Whittemore, A. S., Boehnke, M., Clark, A. G., Eichler, E. E., Gibson, G., Haines, J. L., Mackay, T. F., Mccarroll, S. A., and Visscher, P. M. (2009). Finding the missing heritability of complex diseases. Nature 461, 747–753.
Meyerson, M., Gabriel, S., and Getz, G. (2010). Advances in understanding cancer genomes through second-generation sequencing. Nat. Rev. Genet. 11, 685–696.
Montgomery, S. B., Lappalainen, T., Gutierrez-Arcelus, M., and Dermitzakis, E. T. (2011). Rare and common regulatory variation in population-scale sequenced human genomes. PLoS Genet. 7, e1002144. doi:10.1371/journal.pgen.1002144
Ning, K., and Nesvizhskii, A. I. (2010). The utility of mass spectrometry-based proteomic data for validation of novel alternative splice forms reconstructed from RNA-Seq data: a preliminary assessment. BMC Bioinformatics 11(Suppl. 11), S14. doi:10.1186/1471-2105-11-S11-S14
Orchard, S., Hermjakob, H., and Apweiler, R. (2003). The proteomics standards initiative. Proteomics 3, 1374–1376.
Ouyang, Z., Zhou, Q., and Wong, W. H. (2009). ChIP-Seq of transcription factors predicts absolute and differential gene expression in embryonic stem cells. Proc. Natl. Acad. Sci. U.S.A. 106, 21521–21526.
Pang, A. W., Macdonald, J. R., Pinto, D., Wei, J., Rafiq, M. A., Conrad, D. F., Park, H., Hurles, M. E., Lee, C., Venter, J. C., Kirkness, E. F., Levy, S., Feuk, L., and Scherer, S. W. (2010). Towards a comprehensive structural variation map of an individual human genome. Genome Biol. 11, R52.
Parmigiani, G., Garrett, E. S., Anbazhaghan, R., and Gabrielson, E. (2002). A statistical framework for expression-based molecular classification in cancer. J. R. Stat. Soc. B Stat. Methodol. 64, 717–736.
Pavelka, N., Rancati, G., Zhu, J., Bradford, W. D., Saraf, A., Florens, L., Sanderson, B. W., Hattem, G. L., and Li, R. (2010). Aneuploidy confers quantitative proteome changes and phenotypic variation in budding yeast. Nature 468, 321–325.
Pedrioli, P. G., Eng, J. K., Hubley, R., Vogelzang, M., Deutsch, E. W., Raught, B., Pratt, B., Nilsson, E., Angeletti, R. H., Apweiler, R., Cheung, K., Costello, C. E., Hermjakob, H., Huang, S., Julian, R. K., Kapp, E., Mccomb, M. E., Oliver, S. G., Omenn, G., Paton, N. W., Simpson, R., Smith, R., Taylor, C. F., Zhu, W., and Aebersold, R. (2004). A common open representation of mass spectrometry data and its application to proteomics research. Nat. Biotechnol. 22, 1459–1466.
Pollack, J. R., Sorlie, T., Perou, C. M., Rees, C. A., Jeffrey, S. S., Lonning, P. E., Tibshirani, R., Botstein, D., Borresen-Dale, A. L., and Brown, P. O. (2002). Microarray analysis reveals a major direct role of DNA copy number alteration in the transcriptional program of human breast tumors. Proc. Natl. Acad. Sci. U.S.A. 99, 12963–12968.
Ramakrishnan, S. R., Vogel, C., Prince, J. T., Li, Z., Penalva, L. O., Myers, M., Marcotte, E. M., Miranker, D. P., and Wang, R. (2009). Integrating shotgun proteomics and mRNA expression data to improve protein identification. Bioinformatics 25, 1397–1403.
Rancati, G., Pavelka, N., Fleharty, B., Noll, A., Trimble, R., Walton, K., Perera, A., Staehling-Hampton, K., Seidel, C. W., and Li, R. (2008). Aneuploidy underlies rapid adaptive evolution of yeast cells deprived of a conserved cytokinesis motor. Cell 135, 879–893.
Ren, B., Robert, F., Wyrick, J. J., Aparicio, O., Jennings, E. G., Simon, I., Zeitlinger, J., Schreiber, J., Hannett, N., Kanin, E., Volkert, T. L., Wilson, C. J., Bell, S. P., and Young, R. A. (2000). Genome-wide location and function of DNA binding proteins. Science 290, 2306–2309.
Rhodes, D. R., Yu, J., Shanker, K., Deshpande, N., Varambally, R., Ghosh, D., Barrette, T., Pandey, A., and Chinnaiyan, A. M. (2004). ONCOMINE: a cancer microarray database and integrated data-mining platform. Neoplasia 6, 1–6.
Rual, J. F., Venkatesan, K., Hao, T., Hirozane-Kishikawa, T., Dricot, A., Li, N., Berriz, G. F., Gibbons, F. D., Dreze, M., Ayivi-Guedehoussou, N., Klitgord, N., Simon, C., Boxem, M., Milstein, S., Rosenberg, J., Goldberg, D. S., Zhang, L. V., Wong, S. L., Franklin, G., Li, S., Albala, J. S., Lim, J., Fraughton, C., Llamosas, E., Cevik, S., Bex, C., Lamesch, P., Sikorski, R. S., Vandenhaute, J., Zoghbi, H. Y., Smolyar, A., Bosak, S., Sequerra, R., Doucette-Stamm, L., Cusick, M. E., Hill, D. E., Roth, F. P., and Vidal, M. (2005). Towards a proteome-scale map of the human protein-protein interaction network. Nature 437, 1173–1178.
Sharpf, R. B., Tjelmeland, H., Parmigiani, G., and Nobel, A. B. (2009). A Bayesian model for cross-study differential gene expression. J. Am. Stat. Assoc. 104, 1295–1310.
Smith, A. K., Cheung, K. H., Yip, K. Y., Schultz, M., and Gerstein, M. K. (2007). LinkHub: a semantic web system that facilitates cross-database queries and information retrieval in proteomics. BMC Bioinformatics 8(Suppl. 3), S5. doi:10.1186/1471-2105-8-S3-S5
Solit, D. B., and Mellinghoff, I. K. (2010). Tracing cancer networks with phosphoproteomics. Nat. Biotechnol. 28, 1028–1029.
Spellman, P. T., Miller, M., Stewart, J., Troup, C., Sarkans, U., Chervitz, S., Bernhart, D., Sherlock, G., Ball, C., Lepage, M., Swiatek, M., Marks, W. L., Goncalves, J., Markel, S., Iordan, D., Shojatalab, M., Pizarro, A., White, J., Hubley, R., Deutsch, E., Senger, M., Aronow, B. J., Robinson, A., Bassett, D., Stoeckert, C. J. Jr., and Brazma, A. (2002). Design and implementation of microarray gene expression markup language (MAGE-ML). Genome Biol. 3, RESEARCH0046.
Splendiani, A., Burger, A., Paschke, A., Romano, P., and Marshall, M. S. (2011). Biomedical semantics in the semantic web. J. Biomed. Semantics 2(Suppl. 1), S1.
Stranger, B. E., Forrest, M. S., Dunning, M., Ingle, C. E., Beazley, C., Thorne, N., Redon, R., Bird, C. P., De Grassi, A., Lee, C., Tyler-Smith, C., Carter, N., Scherer, S. W., Tavare, S., Deloukas, P., Hurles, M. E., and Dermitzakis, E. T. (2007). Relative impact of nucleotide and copy number variation on gene expression phenotypes. Science 315, 848–853.
Stratton, M. R., Campbell, P. J., and Futreal, P. A. (2009). The cancer genome. Nature 458, 719–724.
Taylor, I. W., Linding, R., Warde-Farley, D., Liu, Y., Pesquita, C., Faria, D., Bull, S., Pawson, T., Morris, Q., and Wrana, J. L. (2009). Dynamic modularity in protein interaction networks predicts breast cancer outcome. Nat. Biotechnol. 27, 199–204.
Tibshirani, R. (1996). Regression shrinkage and selection via the lasso. J. R. Stat. Soc. B Stat. Methodol. 58, 267–288.
Van de Vijver, M. J., He, Y. D., Van’t Veer, L. J., Dai, H., Hart, A. A., Voskuil, D. W., Schreiber, G. J., Peterse, J. L., Roberts, C., Marton, M. J., Parrish, M., Atsma, D., Witteveen, A., Glas, A., Delahaye, L., Van Der Velde, T., Bartelink, H., Rodenhuis, S., Rutgers, E. T., Friend, S. H., and Bernards, R. (2002). A gene-expression signature as a predictor of survival in breast cancer. N. Engl. J. Med. 347, 1999–2009.
Van ’t Veer, L. J., Dai, H., Van De Vijver, M. J., He, Y. D., Hart, A. A., Mao, M., Peterse, H. L., Van Der Kooy, K., Marton, M. J., Witteveen, A. T., Schreiber, G. J., Kerkhoven, R. M., Roberts, C., Linsley, P. S., Bernards, R., and Friend, S. H. (2002). Gene expression profiling predicts clinical outcome of breast cancer. Nature 415, 530–536.
Keywords: data integration, omics, systems biology, statistical data analysis
Citation: Choi H and Pavelka N (2012) When one and one gives more than two: challenges and opportunities of integrative omics. Front. Gene. 2:105. doi: 10.3389/fgene.2011.00105
Received: 11 October 2011;
Paper pending published: 02 November 2011;
Accepted: 21 December 2011;
Published online: 06 January 2012.
Edited by:
Thiago Motta Venancio, Universidade Estadual do Norte Fluminense, BrazilReviewed by:
Helder I. Nakaya, Emory University, USARobson Francisco De Souza, National Institutes of Health, USA
Fabio Passetti, Instituto Nacional de Câncer, Brazil
Copyright: © 2012 Choi and Pavelka. This is an open-access article distributed under the terms of the Creative Commons Attribution Non Commercial License, which permits non-commercial use, distribution, and reproduction in other forums, provided the original authors and source are credited.
*Correspondence: Hyungwon Choi, Saw Swee Hock School of Public Health, National University of Singapore, MD3, 16 Medical Drive, Singapore 117597. e-mail: hyung_won_choi@nuhs.edu.sg; Norman Pavelka, Singapore Immunology Network, Agency of Science, Technology and Research, 8A Biomedical Grove, Level 3, Immunos, Singapore 138648. e-mail: norman_pavelka@immunol.a-star.edu.sg