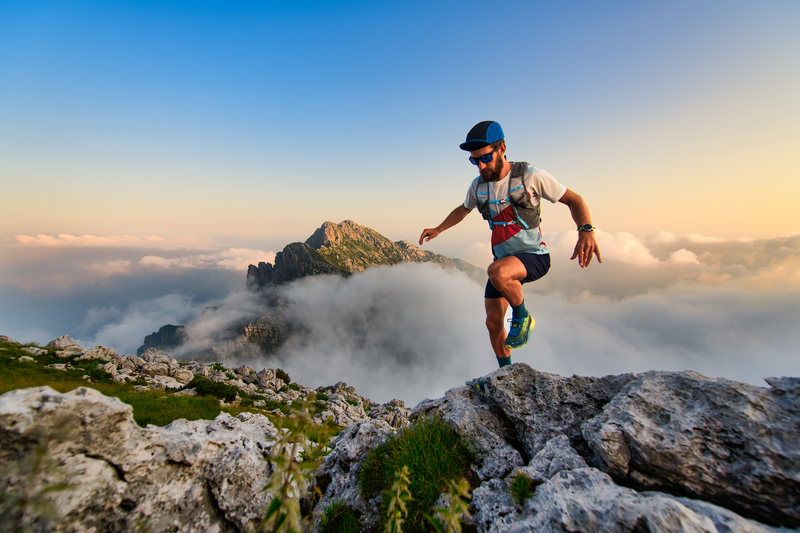
95% of researchers rate our articles as excellent or good
Learn more about the work of our research integrity team to safeguard the quality of each article we publish.
Find out more
REVIEW article
Front. Genet. , 27 December 2011
Sec. RNA
volume 2 - 2011 | https://doi.org/10.3389/fgene.2011.00096
This article is part of the Research Topic Genomic “dark matter”: implications for understanding human disease mechanisms, diagnostics, and cures. View all 12 articles
ncRNAs are key genes in many human diseases including cancer and viral infection, as well as providing critical functions in pathogenic organisms such as fungi, bacteria, viruses, and protists. Until now the identification and characterization of ncRNAs associated with disease has been slow or inaccurate requiring many years of testing to understand complicated RNA and protein gene relationships. High-throughput sequencing now offers the opportunity to characterize miRNAs, siRNAs, small nucleolar RNAs (snoRNAs), and long ncRNAs on a genomic scale, making it faster and easier to clarify how these ncRNAs contribute to the disease state. However, this technology is still relatively new, and ncRNA discovery is not an application of high priority for streamlined bioinformatics. Here we summarize background concepts and practical approaches for ncRNA analysis using high-throughput sequencing, and how it relates to understanding human disease. As a case study, we focus on the parasitic protists Giardia lamblia and Trichomonas vaginalis, where large evolutionary distance has meant difficulties in comparing ncRNAs with those from model eukaryotes. A combination of biological, computational, and sequencing approaches has enabled easier classification of ncRNA classes such as snoRNAs, but has also aided the identification of novel classes. It is hoped that a higher level of understanding of ncRNA expression and interaction may aid in the development of less harsh treatment for protist-based diseases.
The discovery and analysis of ncRNAs has become an important step in the understanding of the genomics behind human disease. Genomics in humans has in the past tended to concentrate on the small percentage (1–2%) of genomic space coding for proteins. Since the vast majority of human ncRNAs lie in non-coding regions including introns and intergenic spaces, there is a need for fast and flexible methods of ncRNA identification. ncRNA classes in general, and in particular microRNAs (miRNAs) and short interfering RNAs (siRNAs) are of great interest in disease studies. In some cases miRNAs have been implicated in various cancers with altered expression levels appearing to be associated with the genetic alterations seen in malignancies (Ryther et al., 2003). miRNAs and siRNAs to be important effectors in host–pathogen interaction networks between humans and their viruses (Aurrecoechea et al., 2009), most of which use RNA interference processes. RNA interference (RNAi) has also been raised as an option for medical treatment of human diseases including cancer (Garlapati et al., 2011), viruses (Khaliq et al., 2010; Haasnoot and Berkhout, 2011), and transplantation (Zhang et al., 2011b).
RNA interference in general, is a process where small RNAs (e.g., miRNAs and siRNAs) are used by a protein macromolecule, to target and then to cleave transcribed mRNAs, hence “interfering” with the expression of a targeted gene. There are a number of different pathways for this interference (Collins and Penny, 2009; Batista and Marques, 2011; Ketting, 2011), with three main proteins or their like, being typically required. These proteins are Dicer, Argonaute, and RNA-dependent RNA polymerase (RdRp). Finding homologs to these proteins in protist species is usually the first step in determining that RNAi exists in that species. However, as can be seen in species such as Giardia lamblia and Entamoeba histolytica, some of these proteins may not contain all the domains we expect to find (Macrae et al., 2006; Carlton et al., 2007; Batista and Marques, 2011). It is thus, very likely that protist RNAi pathways will differ from their well-studied multicellular counterparts, and that understanding these differences will enable a far more efficient use of RNA as a molecular tool.
RNA interference has been used to understand gene expression levels and the changes that occur at different stages of disease, different stages of life cycle or development, and differences in environmental conditions (typically with miRNA studies). Genes can also be specifically “knocked-out” using gene silencing studies to investigate the effects of particular parts of metabolic pathways. This has been done in some protists, in particular Kinetoplasts where RNAi has been used as a tool in genomic studies for Trypanosoma brucei (reviewed in Kolev et al., 2011), and to a lesser extent in Leishmania braziliensis subgenus Viannia (Lye et al., 2011). The difficulty in protist RNAi research is that the small RNAs that are used in RNAi (i.e., miRNAs and siRNAs), are not easily isolated and characterized.
Genomic-wide sequencing is also furthering studies on how a host species reacts to pathogens in immune and preventative responses. One non-protist example is where high-throughput sequencing was used to characterize miRNA levels and identify novel miRNAs involved in avian influenza virus (AIV) infection of chicken (Aurrecoechea et al., 2009). In this study, sequences were matched not only to genomic sequences but to mature miRNA sequences previously lodged in miRbase (Finn et al., 2006), allowing for insertions and deletions of 1–4 nt. Profiling analysis compared infected and non-infected tissue to identify miRNAs that changed expression upon infection. Mapping of the sequences also revealed that many miRNAs are grouped in clusters on the chicken chromosomes and up- or down-regulated together. Results from this study suggest that different miRNA regulation mechanisms may exist on host response to virus infection with some genes up regulated to aid host immune response and down regulation of targets to aid inhibition of virus replication. Different tissues may express different levels of miRNAs. For example in the Wang et al. study 377 miRNAs were identified from chicken lung tissue but only 149 miRNAs were identified from tracheae. Clearly this type of study will soon extend to the host response to protists. The techniques for analysis will be similar but will require a greater understanding of the typical features of the different miRNA classes in the protist of study.
There are many different classes of ncRNAs found in protists (Table 1), and only some of these such as miRNAs and siRNAs and sometimes small nucleolar RNAs (snoRNAs) are involved in RNAi. Other ncRNAs such as tRNAs and rRNAs are relatively easy to characterize because they look familiar to those already studied, but there are classes such as snoRNAs, that are harder to find and classify because either their sequence or their action, is novel. Previously, there were two main approaches to ncRNA identification (Figure 1). The first, the “traditional” approach, involved the isolation of expressed RNA in a designated size range, cloning, sequencing then finally, Northern blotting to confirm size and conformational isotopes from RNA modifications. This approach was costly both in laboratory expenditure and time, and was not very practical on a genomic scale. The other approach took a sequenced genome and computationally screened it for candidate ncRNAs, using mathematical models based on the sequence and structural characteristics of a class of ncRNA. This second approach, although it could be applied on a genomic scale, often produced masses of candidates that would then have to be experimentally tested by the first approach. Another issue with the computational approach is that only a single class could be searched at a time, and one had to know what that class looked like both in sequence and secondary structure in order to find it. Permitting more flexibility however, results in more false positives, a circumstance that can quickly overload the computer and its user. High-throughput sequencing permits the genome-wide sequencing of ncRNAs from expressed RNA (the power of the first approach), and for rapid comparison to known classes (the power of the second approach), as well as the characterization of novel ncRNAs (Figure 1). The disadvantage of this type of sequencing is that it demands a different type of computational analysis than previously used with ncRNAs (see later).
Figure 1. Genomic approaches to ncRNA identification. Both the traditional laboratory approach and the more recent high-throughput sequencing approach begin with the isolation of total RNA from culture, followed by size selection of the RNA by excising a given band from a polyacrylamide gel. Under the traditional approach the excised RNA is cloned then sequenced by Sanger Sequencing to obtain candidate miRNA sequences. With High-throughput sequencing the RNA is sequenced directly without cloning and bioinformatics is used to select the best candidates. Computational approaches do not begin with biological samples but instead use mathematical models based on known ncRNAs to search an already sequenced genome. Both the traditional and computational approaches require that candidate gene expression be confirmed by additional laboratory work. Key: Molecular biology stages are represented by the flask icon. All other stages use RNA genomic and bioinformatics procedures.
Short interfering or silencing RNAs (siRNAs) are similar to miRNAs but are produced from double stranded precursors instead of folded single-stranded precursors. What makes this mechanism of great interest is that the gene silencing is highly specific, and also highly potent in where only a few copies of an small RNA (22–25 nt long) can demonstrate wide ranging affects. In plants, they have been highly investigated for their role in virus response (Ridanpaa et al., 2003; Pantaleo, 2011), but in humans and mice they are under intense study for therapeutic medicine (recently reviewed in Burnette et al., 2005; Khaliq et al., 2010; Vaishnaw et al., 2011). In an example, a combination of host and viral genes has been used as a siRNA-based treatment for hepatitis C virus (HCV; Ashfaq et al., 2011). Developing RNAi based treatments for HCV are an important are of research, since there is currently no vaccine available for HCV due to a high degree of strain variation. Another factor is that the current drug treatment (with a pegylated interferon α/ribavirin combination) is costly, has significant side effects and is not always effective (Ashfaq et al., 2011). RNAi offers new and less harsher types of treatment especially for viral diseases, but there are still challenges in this area in systematic siRNA delivery and distribution to appropriate tissue (Vaishnaw et al., 2011).
Small nucleolar RNAs typically have roles in the modification of other ncRNAs such as rRNA, small nuclear RNAs (snRNAs), and possibly even mRNAs (Ridanpaa et al., 2003; Bompfunewerer et al., 2005; Gardner et al., 2010; Khanna and Stamm, 2010). snoRNAs are between 60 and 150 nt long and fall into two main classes based on conserved sequence motifs, H/ACA (sometimes called SNORAs), and C/D (sometimes called SNORDs). snoRNAs may have a high potential for use as markers for diseases either by having mutated sequences or differential expression. In one example some snoRNAs were discovered to have a higher expression in some lung cancer cells than in non-cancerous cells, and thus have a potential as markers for the early detection of this cancer (Liao et al., 2010).
The examples above focus on how ncRNAs from the host can be used to study gene expression from healthy, diseased, and sometimes treated tissue. A second type of study looks at the ncRNAs from the pathogen itself to understand where the pathogen could be vulnerable and hence open to new treatment options. This is where there is less work published since until now the discovery and characterization of ncRNAs in most pathogens was slow and laborious. Until very recently ncRNAs in prokaryotes (often called “small RNAs”) were not commonly thought of as being important in pathogenic studies. The characterization of the CRISPR system and small RNA pathways (e.g., Hfq-binding sRNAs) has made us more aware that an RNA-based backbone exists just as much in prokaryotes as in eukaryotes (for review see Biggs and Collins, 2011; Collins and Biggs, 2011). Eukaryotic pathogens (e.g., nematodes, yeast, and protists) have received a little more attention but lag behind our understanding of host (typically human and mice) ncRNAs (review by Batista and Marques, 2011).
RNA interference in the protist T. brucei (causative agent of sleeping sickness) was characterized early on as functional (Ngo et al., 1998), only months after it was demonstrated in the nematode Caenorhabditis elegans (Fire et al., 1998; reviewed in Kolev et al., 2011). However, RNAi mechanisms can be lost from a lineage, as demonstrated in some yeast (Drinnenberg et al., 2009, 2011) and some trypanosomatids (Lye et al., 2011). Thus, even though RNAi is considered to be an ancient system that was likely to be present in the last common ancestor of eukaryotes (Collins and Chen, 2009), it does not follow that it will be still be present. Further studies (reviewed in Kolev et al., 2011) have revealed that RNAi in trypanosomatids loss in multiple lineages are in most cases correlated with the lack of mobile elements (Kolev et al., 2011; Lye et al., 2011). The lack of RNAi but presence of mobile element-like sequences in one lineage of trypanosomatids T. cruzi falls against that trend (Kolev et al., 2011) indicating that there is much more to be learned about the evolution of RNAi mechanisms.
One of the issues contributing to the slow progress in understanding protist RNAi is that many of these pathogens (and especially the protists) do not yet have well annotated genomes. Others may have genomes sequenced (some fungi and nematodes) but genes are annotated based on sequence similarity to the few very well known genomes. ncRNA genes change rapidly and mis-annotation is common. Very small genes such as those for miRNAs and siRNAs can be extremely hard to characterize based on sequence similarity. Hence, the arrival of high-throughput sequencing has enabled these ncRNAs to be tackled in a slightly easier manner, but it has meant the incorporation of more bioinformatics into these projects. High-throughput sequencing of pathogens and especially protists in practice uses the power of computational biology combined with the expression from real RNA. With this technology we can look at miRNAs, siRNAs, snoRNA, and even longer ncRNAs from a pathogenic protist and potentially link some of them to host responses. However, first we have to characterize the ncRNA classes from our species of choice. Here we will use the two pathogenic protists, G. lamblia and Trichomonas vaginalis as examples to highlight the issues and possible solutions with high-throughput small RNA sequencing. It should be noted however, that these issues and solutions are not confined to protist genomics, but are also generally applicable to other species including prokaryotes.
Pathogenic protists are responsible for a host of human diseases that affect millions worldwide, but not surprisingly ncRNA research in these pathogens has lagged behind protein-based research. Over the last decade, there are two protist species, G. lamblia and T. vaginalis that are of interest in the characterization of their RNAs because of their evolutionary distance from other eukaryotes (Collins and Penny, 2005, 2009; Collins and Chen, 2009; Chen et al., 2011). G. lamblia is a Diplomonad anaerobic protist that infects humans and other mammals. When ingested, the cysts hatch into trophozoites in the small intestine causing diarrhea and growth hindrance in children. It is a significant pathogen for the immune-compromised and those in developing countries affected by malnutrition. The most common treatment for giardiasis is Metronidazole (Flagyl), which unfortunately has unpleasant side effects including nausea and dizziness, but more importantly has potential carcinogenic properties. Metronidazole is not approved by the FDA in the USA for treatment in human medicine for this reason.
Trichomonas vaginalis is an anaerobic Parabasalid protist that causes the sexually transmitted disease trichomoniasis in humans. Despite its name, infections are common in men but are typically asymptomatic. In women trichomoniasis is symptomatic as an STD, but it can also lead to adverse pregnancy outcomes and be associated with an increased risk of human immunodeficiency virus (HIV) transmission (Cudmore et al., 2004). Trichomonas differs from Giardia in that it does not have a cyst stage, so infection is directly by the trophozoites being transferred from patient to patient. Treatment of trichomoniasis also includes Metronidazole, but studies have shown at least 5% of cases are resistant to this drug (Cudmore et al., 2004).
Other pathogenic protists include Plasmodium falciparum (malaria), E. histolytica (amebic dysentery) and T. brucei (sleeping sickness) and T. cruzi (Chagas disease). Although drug treatments for all these diseases are available there is still a need for further development, especially for Giardia and Trichomonas where problems with treatment persist. Thus, the use of ncRNAs and RNAi is especially applicable to eukaryotic pathogens such as Giardia and Trichomonas as both a tool and a potential treatment option.
High-throughput sequencing has been used to assemble protist genomes. Giardia and Trichomonas were “completed” before this technology appeared meaning that their assembly was slow and most is still in the form of large pieces (supercontigs). This should not put any researcher off using such genomes since genomes like this are very usable for high-throughput small RNA studies. From a number of studies, all using these annotated genomes, we can see that Giardia and Trichomonas like their distant multicellular human host contain a rich collection of ncRNAs (Chen et al., 2007, 2008, 2009). These include RNase P (Piccinelli et al., 2005), RNase MRP (Chen et al., 2011) snoRNAs (Yang et al., 2005; Chen et al., 2007, 2011), spliceosomal snRNAs (Chen et al., 2008), miRNAs (Saraiya and Wang, 2008; Chen et al., 2009; Zhang et al., 2009), and antisense transcripts (Teodorovic et al., 2007). Studies on Trichomonas ncRNAs show that the currently known ncRNAs also exhibit typical features of eukaryotes (Piccinelli et al., 2005; Simoes-Barbosa et al., 2008; Lin et al., 2009; Smith and Johnson, 2011) including RNase P (Piccinelli et al., 2005), RNase MRP (Piccinelli et al., 2005), snRNAs (Simoes-Barbosa et al., 2008), and some snoRNAs (Chen et al., 2007, 2009, 2011). However, some classes of ncRNAs (especially the snoRNAs) contain some features that are not typical. It is high-throughput sequencing that offers the opportunity to investigate these novel classes of ncRNA.
High-throughput sequencing of small RNAs requires an RNA sample of high quality and reasonably high concentration. An issue with many protists is that they are not culturable so RNA is sometimes extracted from patient samples. Therefore, obtaining enough clean and uncontaminated RNA for genomic sequencing is sometimes not easy or possible unless from a culturable strain. Additionally, lab strains carry the risk that they may contain genetic differences from their clinical relatives. Advances in sequencing protocols have meant that the amount of RNA required for sequencing (genomic, transcriptomic, and to some extent small RNA) is reduced, and it is hoped that with further improvements in protocols, more protists will be sequenced. Once a sample of RNA is obtained, then the next stage is to decide which ncRNAs are going to be sequenced. A sample of total RNA contains some RNAs that can drown out any underrepresented ncRNAs in samples so mRNAs, rRNAs, and tRNAs must be removed as much as possible. A typical procedure is to run the sample on a polyacrylamide gel, then excise a band corresponding to the size for sequencing (e.g., miRNAs, siRNAs:19–30 nt, snoRNAs:50–200 nt; Chen et al., 2009). Gel isolation, although contributing to the reduction in the amount of RNA available for sequencing, offers flexibility and selectivity in the class of ncRNA being examined. Overall, small RNA sequencing experiments are individualistic with all the advantages and disadvantages that come with the use of developing technology.
The actual sequencing of small RNA samples is typically performed by the operator of the technology, whether at a service provider or in-house facility. There are currently choices of sequencing platforms for high-throughput sequencing, and highly likely that there will be even more choice in the near future. For small RNA sequencing the platform selected will depend on the length of the ncRNA to be sequenced. Some platforms such as the Roche 4541 produce long sequences (400–1000 nt) which is really only suitable if trying to sequence very long ncRNAs. Small RNAs can be done on this platform but it is not optimized for this type of work. Presently available platforms that generate short sequences are the Illumina systems (HiSeq, MiSeq, and Genome Analyzer2) and the SOLiD system3.Because they enable sequences of short length to be obtained (36–50 nt) these systems are ideal for sequencing small ncRNAs such as miRNAs and siRNAs and as you will see later, can also be used to sequence ncRNAs of longer lengths. For a review of these platforms and their use in small RNA studies in general please see McCormick et al. (2011). There are also different RNA-based protocols available, including those that combine mRNA and ncRNA sequencing (i.e., without need for the mRNA to have polyA tails; Yang et al., 2011). This is very useful for prokaryotic sequencing and also for combining mRNA and ncRNA data in a single run. However, “small RNA sequencing” is the typical protocol used for human ncRNA analysis. The field of high-throughput sequencing is very dynamic with platforms and protocols constantly being added and upgraded. It is therefore best to consult with the platform operator on what is available, prior to submitting samples.
If the idea is to compare miRNAs and their expression levels across different conditions (i.e., ncRNA gene expression) then there is one further consideration. There can be affects in running samples in different partitions of the sequencing apparatus (e.g., in different lanes or partitions on a “flowcell”). To avoid an excess of statistical analysis to take “lane-effect” into account, it is suggested that both samples are run in the same partition or partitions and barcoded to aid sorting after the sequencing. This is now common practice for digital gene expression analysis with mRNAs.
The analysis of data from high-throughput sequencing is quite unlike other genomic analysis and can be daunting to the newcomer. Unfortunately the analysis of ncRNA data is one of the lesser-described protocols in high-throughput sequencing meaning that there are fewer automated pipelines, and the software is primarily at a command-line level. Only now are guidelines to small RNA analysis being published (e.g., McCormick et al., 2011) and this is primarily for mammalian and plant research. What follows in this section is a general approach to the analysis of short (<25 nt) and medium/long (>50 nt) ncRNA sequencing data from high-throughput sequencing (Figure 2).
Figure 2. A general approach for using high-throughput sequencing data to search for small and larger ncRNAs. Total RNA can contain ncRNA sequences of different sizes. Small ncRNAs such as miRNAs and siRNAs will produce data containing the ncRNA sequence plus adaptor sequence. Once the adaptor is trimmed off, the sequence is ready for mapping and further genomics. When longer ncRNAs are sequenced, they are fragmented to a predetermined size by the sequencing process. After sequencing they can be assembled by mapping (see text) into their longer sequences. This approach was successfully used for the analysis of ncRNAs from Giardia lamblia (see text for details).
The output from high-throughput sequencing is typically a file of short sequences (often termed short-reads or reads) accompanied by a quality score for each nucleotide in each sequence. This is termed FASTQ format with four lines for each sequence (Cock et al., 2010). However, because of the high sensitivity of this type of sequencing, the “raw” data will also contain sequences, including sequencing primers and contaminants, which occur in all high-sequencing datasets. Data filtering is therefore the first step to analysis and for small RNA sequencing it can permit the separation of reads into those likely to be from small ncRNAs (miRNAs and siRNAs) and those from medium ncRNAs. Sequencing adaptors and sequencing primers will occur in small RNA sequencing datasets. Adaptors are the short sequences that are added to the ends of the RNA fragment during the sequencing process. Additionally, during RNA work (due to the fragments being very short) we can also get adaptor dimers forming and these will show up in the results. Adaptor sequence information is available from the sequencing vendors and can be removed from the data using text-mining or sequence manipulation software. One example is the FASTX-toolkit4 that contains scripts not only to remove adaptor sequences, but can also remove sequences containing too many N’s and those sequences of lower quality. Other quality assessment tools include many commercial software packages, and freeware such as FastQC5, which can help guide data-filtering requirements.
Biologically ncRNAs may be of different lengths in the cell, and this can cause numerous issues for downstream analysis where computer programs prefer the data length to be consistent. It is therefore particularly recommended that sequences are trimmed to a common nucleotide length before mapping. For work with protist small RNA data such as that from Giardia and Trichomonas (used in studies by Chen et al., 2007, 2008, 2009, 2011), this basic approach of filtering sequences prior to further analysis was used. It was found that by filtering those sequences with adaptors, and those without, into different datasets, ncRNAs of different length ranges (small and medium) could be characterized (Figure 3).
Figure 3. ncRNAs processes characterized in Giardia lamblia and their likely cellular locations in the trophozoite stage, and in relation to the major RNA processes of Transcription and Translation. Giardia has two nuclei which appear identical and replicate at the same time and all of the processes within Transcription, RNP Biogenesis, Transcriptional regulation, and Core RNA processing could be expected to be found in both nuclei. RNAi may also be important in viral defense since some siRNAs found in high-throughput sequencing do map to the stable Giardiavirus (unpublished results). It is not known yet whether Giardia has RNA storage granules but their presence in Trypanosoma does not preclude them here. A similar diagram can be visualized for Trichomonas except that it only has one nucleus.
Mapping (or matching) of the short-read sequences to a reference genome is the easiest approach for finding ncRNAs from protists. One approach is to map sequences to ncRNAs already characterized. Since there are still only a relatively few classes of ncRNAs characterized for many protists this approach can yield limited results but is worth doing to characterize medium length ncRNAs such as snoRNAs, snRNAs, RNase P, and RNase MRP. Smaller ncRNA classes such as miRNAs and siRNAs may have too many sequence differences over their short length to permit accurate mapping directly to known sequences, but mapping of miRNAs is possible to their longer pre-miRNA precursors, and siRNAs to potential target sequences. Mapping tools such as BWA (Li and Durbin, 2009), Bowtie (Langmead et al., 2009), and SOAP2 (Aurrecoechea et al., 2009), as well as commercial options are available for mapping small RNA short-read sequences. However, all of this software was designed for dealing with sequences longer than 22 nt, so software parameters must be changed for mapping short ncRNAs such as miRNAs and siRNAs. One of the key parameters to adjust is the “seed length” for the alignments, which is where the initial contact between the read and the reference genome is made. This should be set to about 17–18. Allowing more mismatches, e.g., up to n = 3 mismatches per seed (the standard is n = 2) may only be beneficial if the species being sequenced is different from the reference genome. However, this can cause spurious “false” mappings and typically n = 2 should suffice. Another useful parameter is in the reporting of the results. Often when a short-read maps to multiple places, only one place is reported and this is typically assigned by random. Since we commonly have miRNAs and siRNAs match both at their place in the genome and at their target position in the genome, multiple matches are normal, thus reporting settings should be adjusted for the reporting of all hits.
In practice, miRNAs have been characterized from protists using a mixture of traditional, computational, and high-throughput sequencing approaches. Some miRNAs from Giardia have been shown to be derived from snoRNAs (Saraiya and Wang, 2008; Kolev and Ullu, 2009), with two of these, miR2 and miR4 consequently shown to regulate the expression of variant surface proteins (VSPs), involved in resistance to host intestinal proteases (Prucca et al., 2008; Garlapati et al., 2011). A computational study of miRNAs from Giardia (Zhang et al., 2009) identified 50 mature miRNA candidates, some of which also targeted VSP genes. Using high-throughput sequencing these VSP targeting miRNA candidates were also found (Chen et al., 2007, 2009) as well as many other miRNAs conforming to the expected size range of 25–27 nt (Macrae et al., 2006; Chen et al., 2007).
In Trichomonas, miRNAs have been characterized using traditional (Lin et al., 2009), and high-throughput sequencing approaches (Chen et al., 2009). Here, the use of miRNA-based software such as miRanda6, aided in the selection of strong miRNA candidates. In practice, although high-throughput sequencing is sequencing biologically expressed RNAs (unlike computational analysis), there may also be some mRNA degradation products and contaminants that may slip through filtering. Studies in Giardia and Trichomonas have highlighted that confirmation procedures from another source (including miRNA-based software), is still required. Expression levels of some Trichomonas miRNAs have also been examined showing that one miRNA tva-miR-001 has a significantly lower expression level in the ameboid stage (Lin et al., 2009).
Most of the small RNA work to date in Giardia and Trichomonas has focused on miRNAs but siRNAs are also present (Chen et al., 2009). Some siRNAs appear to map in Giardia to a long-tandem repeat RNAs (Girep-1–5) which show a high degree of sequence similarity with a number of VSP genes (Chen et al., 2009) indicating yet another connection between RNAi and VSP gene selection. In addition, both Giardia and Trichomonas contain stable RNA viruses and high-throughput sequencing has resulted in some reads mapping to these viruses (unpublished results).
Small RNAs from other protists have also been characterized using traditional or computational methods. In T. cruzi, where there standard RNAi proteins have not been found, small RNAs derived from tRNAs have been characterized which are usually recruited to specific cytoplasmic granules (Garcia-Silva et al., 2010). T. brucei does contain standard RNAi pathways and computational studies have uncovered miRNAs that target another type of antigenic variation variant surface glycoproteins (VSGs; Rudra et al., 2007). Some miRNA candidates have also been characterized in E. histolytica using a computational study (De et al., 2006), but research here has focused on using dsRNA for gene silencing (reviewed by Kolev et al., 2011; Zhang et al., 2011a). Studies of protist miRNAs to date are showing that RNAi has an important role in antigenic variation and hence the parasite’s survival in its host. Learning more about this system could enable more effective strategies to prevent and treat a range of protist diseases.
To characterize medium length ncRNAs, such as snoRNAs, RNase P, and RNase MRP RNAs, it can be useful to generate “contigs” (overlapping consensus fragments) from the mapping data. De novo assembly tools such as Velvet (Zerbino and Birney, 2008) and Abyss (Simpson et al., 2009) which assemble fragments without any prior alignment to a reference genome, are again primarily designed for working with longer sequences, and in practice did not perform well with data from Giardia and Trichomonas data (Chen et al., 2007, 2008, 2009, 2011). However, with careful parameter choice and a bit of experimentation, it is not inconceivable that these tools could be useful in the assembly of long (>200 nt long) ncRNAs, such as those discovered to be crucial for human and mouse epigenetics. An easier way to generate consensus contigs can be to use the results from mapping and convert them to contig sequences using software such as the mpileup function of SAMtools (Li et al., 2009), or the now depreciated tools in Maq (Li et al., 2008). The use of a reference genome to guide the assembly of contigs means that areas separated by low coverage of reads can be joined for further analysis. This technique was used to find medium length ncRNAs such as RNase P and RNase MRP RNAs from Giardia (Chen et al., 2011). Although the RNase P RNA has been previously identified from Giardia, the closely related RNase MRP RNA was found by forming larger contigs from short-reads then using the INFERNAL RNA comparison software (Nawrocki et al., 2009) to compare these contigs to ncRNAs from Rfam. Using this method RNase P and MRP RNAs were either characterized, confirmed for had ambiguous genomic positions clarified (Chen et al., 2011). Comparative genomes has been used to characterize snoRNAs from trypanosomatids including Leishmania (Liang et al., 2007) and T. brucei (Uliel et al., 2004; Barth et al., 2008; Gupta et al., 2010).
Specialist software such as snoScan (Schattner et al., 2005) can be used to characterize snoRNAs from both classes C/D box and H/ACA. Often it can be necessary to change parameters within this software to permit changes in expected secondary structure. C/D box snoRNAs direct 2′-O methylation, and are relatively easy to identify based on conserved sequence elements (stem-loop features) and complementary binding to the target RNAs. H/ACA box snoRNAs direct pseudouridylation, and often exhibit more variable features due to their shorter length of conserved elements and discontinuous complementary target binding regions. A combined experimental and computation approach using high-throughput sequencing enabled both of these snoRNAs classes to be characterized in G. lamblia (Chen et al., 2007). Here, snoScan was modified to search for snoRNAs in the Giardia WB genome but these adjustments caused a loosening of parameters, and hence, an increase in false positives being reported. Positive hits were compared to high-throughput sequencing results to filter through the large number of false positives. When the sequencing results were combined with information from potential ribosomal pseudouridylation sites, other snoRNAs were characterized from both Giardia and Trichomonas (Chen et al., 2011).
There are many snoRNAs even from well characterized species (i.e., humans) that do not have identified targets. These are termed orphan snoRNAs (Bazeley et al., 2008) and it is likely that such snoRNAs will be found in protists, but perhaps not as closely associated with splicing (Giardia and Trichomonas have few introns). However, without potential binding sites to check results against, much more laboratory work will be required to characterize these orphan snoRNAs, even those potential candidates found by high-throughput sequencing.
High-throughput sequencing has opened the door on more research into the use of ncRNAs either as tools for investigating protist biology, markers for disease detection or progression, or as potential avenues for treatment. Although there is a long way to go to catch up with ncRNA analysis from host species (e.g., human and mouse), the genomic sequencing of many pathogenic protists is already permitting genome-wide screens of ncRNAs such as miRNAs and siRNAs. Studies of protist miRNAs to date are showing that RNAi has an important role in antigenic variation and hence the parasite’s survival in its host. Learning more about these systems could enable more effective strategies to prevent and treat a range of protist diseases. Other areas of active research are now looking at the integration of regulatory RNA (miRNA and siRNA) data with protein gene expression sequencing data (i.e., RNA-seq, or mRNAseq), to characterize how miRNAs control their targets, and are themselves controlled, in different environments. In effect, this is a combination of miRNA expression and target expression, all coming from the same sample.
The use of high-throughput sequencing to uncover and characterize ncRNAs has both the biological relevance of traditional laboratory approaches and the genome-wide scale of the computational approaches. However, it does require the understanding of both the biological and computational aspects of RNA analysis. Although software both for mapping, assembly and sequence manipulation was written for longer mRNAs and genomic sequencing, it can be applied to short ncRNAs such as miRNAs and siRNAs with careful parameter adjustment. It is likely that in the next few years we will see further development of the small RNA sequencing protocols that are available especially as they rise in importance in the medical research world. What is needed to meet this rise is a general upskilling of molecular researchers to deal with the increased bioinformatics that this new technology brings, and further development of software pipelines to make it easier to adapt RNA software to non-mammalian and non-plant species. Protist biology is very different and their ncRNA systems are delivering us many surprises (Collins and Penny, 2009). It is clear that genome biology of host and pathogens can no longer exclude the analysis of non-coding sequences.
The author declares that the research was conducted in the absence of any commercial or financial relationships that could be construed as a potential conflict of interest.
Many thanks go to David Penny for his support and critical reading of this manuscript. This work was supported by the New Zealand Marsden Fund.
Ashfaq, U. A., Yousaf, M. Z., Aslam, M., Ejaz, R., Jahan, S., and Ullah, O. (2011). siRNAs: potential therapeutic agents against hepatitis C virus. Virol. J. 8, 276.
Aurrecoechea, C., Brestelli, J., Brunk, B. P., Carlton, J. M., Dommer, J., Fischer, S., Gajria, B., Gao, X., Gingle, A., Grant, G., Harb, O. S., Heiges, M., Innamorato, F., Iodice, J., Kissinger, J. C., Kraemer, E., Li, W., Miller, J. A., Morrison, H. G., Nayak, V., Pennington, C., Pinney, D. F., Roos, D. S., Ross, C., Stoeckert, C. J. Jr., Sullivan, S., Treatman, C., and Wang, H. (2009). GiardiaDB and TrichDB: integrated genomic resources for the eukaryotic protist pathogens Giardia lamblia and Trichomonas vaginalis. Nucleic Acids Res. 37, D526–D530.
Barth, S., Shalem, B., Hury, A., Tkacz, I. D., Liang, X. H., Uliel, S., Myslyuk, I., Doniger, T., Salmon-Divon, M., Unger, R., and Michaeli, S. (2008). Elucidating the role of C/D snoRNA in rRNA processing and modification in Trypanosoma brucei. Eukaryot. Cell 7, 86–101.
Batista, T. M., and Marques, J. T. (2011). RNAi pathways in parasitic protists and worms. J. Proteomics 74, 1504–1514.
Baum, J., Papenfuss, A. T., Mair, G. R., Janse, C. J., Vlachou, D., Waters, A. P., Cowman, A. F., Crabb, B. S., and De Koning-Ward, T. F. (2009). Molecular genetics and comparative genomics reveal RNAi is not functional in malaria parasites. Nucleic Acids Res. 37, 3788–3798.
Bazeley, P. S., Shepelev, V., Talebizadeh, Z., Butler, M. G., Fedorova, L., Filatov, V., and Fedorov, A. (2008). snoTARGET shows that human orphan snoRNA targets locate close to alternative splice junctions. Gene 408, 172–179.
Biggs, P. J., and Collins, L. J. (2011). RNA networks in prokaryotes I: CRISPRs and riboswitches. Adv. Exp. Med. Biol. 722, 209–220.
Bompfunewerer, A. F., Flamm, C., Fried, C., Fritzsch, G., Hofacker, I. L., Lehmann, J., Missal, K., Mosig, A., Muller, B., Prohaska, S. J., Stadler, B. M. R., Stadler, P. F., Tanzer, A., Washietl, S., and Witwer, C. (2005). Evolutionary patterns of non-coding RNAs. Theory Biosci. 123, 301–369.
Burnette, J. M., Miyamoto-Sato, E., Schaub, M. A., Conklin, J., and Lopez, A. J. (2005). Subdivision of large introns in Drosophila by recursive splicing at non-exonic elements. Genetics 170, 661–674.
Carlton, J. M., Hirt, R. P., Silva, J. C., Delcher, A. L., Schatz, M., Zhao, Q., Wortman, J. R., Bidwell, S. L., Alsmark, U. C. M., Besteiro, S., Sicheritz-Ponten, T., Noel, C. J., Dacks, J. B., Foster, P. G., Simillion, C., Van De Peer, Y., Miranda-Saavedra, D., Barton, G. J., Westrop, G. D., Müller, S., Dessi, D., Fiori, P. L., Ren, Q., Paulsen, I., Zhang, H., Bastida-Corcuera, F. D., Simoes-Barbosa, A., Brown, M. T., Hayes, R. D., Mukherjee, M., Okumura, C. Y., Schneider, R., Smith, A. J., Vanacova, S., Villalvazo, M., Haas, B. J., Pertea, M., Feldblyum, T. V., Utterback, T. R., Shu, C.-L., Osoegawa, K., De Jong, P. J., Hrdy, I., Horvathova, L., Zubacova, Z., Dolezal, P., Malik, S.-B., Logsdon, J. M., Henze, K., Gupta, A., Wang, C. C., Dunne, R. L., Upcroft, J. A., Upcroft, P., White, O., Salzberg, S. L., Tang, P., Chiu, C.-H., Lee, Y.-S., Embley, T. M., Coombs, G. H., Mottram, J. C., Tachezy, J., Fraser-Liggett, C. M., and Johnson, P. J. (2007). Draft genome sequence of the sexually transmitted pathogen Trichomonas vaginalis. Science 315, 207–212.
Chen, X. S., Collins, L. J., Biggs, P. J., and Penny, D. (2009). High throughput genome-wide survey of small rnas from the parasitic protists Giardia intestinalis and Trichomonas vaginalis. Genome Biol. Evol. 2009, 165–175.
Chen, X. S., Penny, D., and Collins, L. J. (2011). Characterization of RNase MRP RNA and novel snoRNAs from Giardia intestinalis and Trichomonas vaginalis. BMC Genomics 12, 550. doi:10.1186/1471-2164-12-550
Chen, X. S., Rozhdestvensky, T. S., Collins, L. J., Schmitz, J., and Penny, D. (2007). Combined experimental and computational approach to identify non-protein-coding RNAs in the deep-branching eukaryote Giardia intestinalis. Nucleic Acids Res. 35, 4619–4628.
Chen, X. S., White, W. T., Collins, L. J., and Penny, D. (2008). Computational identification of four spliceosomal snRNAs from the deep-branching eukaryote Giardia intestinalis. PLoS ONE 3, e3106. doi:10.1371/journal.pone.0003106
Cock, P. J., Fields, C. J., Goto, N., Heuer, M. L., and Rice, P. M. (2010). The Sanger FASTQ file format for sequences with quality scores, and the Solexa/Illumina FASTQ variants. Nucleic Acids Res. 38, 1767–1771.
Collins, L., and Penny, D. (2005). Complex spliceosomal organization ancestral to extant eukaryotes. Mol. Biol. Evol. 22, 1053–1066.
Collins, L. J., and Biggs, P. J. (2011). RNA networks in prokaryotes II: tRNA processing and small RNAs. Adv. Exp. Med. Biol. 722, 221–230.
Collins, L. J., and Chen, X. S. (2009). Ancestral RNA: the RNA biology of the eukaryotic ancestor. RNA Biol. 6, 495–502.
Collins, L. J., and Penny, D. (2009). The RNA infrastructure: dark matter of the eukaryotic cell? Trends Genet. 25, 120–128.
Cudmore, S. L., Delgaty, K. L., Hayward-Mcclelland, S. F., Petrin, D. P., and Garber, G. E. (2004). Treatment of infections caused by metronidazole-resistant Trichomonas vaginalis. Clin. Microbiol. Rev. 17, 783–793.
De, S., Pal, D., and Ghosh, S. K. (2006). Entamoeba histolytica: computational identification of putative microRNA candidates. Exp. Parasitol. 113, 239–243.
Drinnenberg, I. A., Fink, G. R., and Bartel, D. P. (2011). Compatibility with killer explains the rise of RNAi-deficient fungi. Science 333, 1592.
Drinnenberg, I. A., Weinberg, D. E., Xie, K. T., Mower, J. P., Wolfe, K. H., Fink, G. R., and Bartel, D. P. (2009). RNAi in budding yeast. Science 326, 544–550.
Finn, R. D., Mistry, J., Schuster-Bockler, B., Griffiths-Jones, S., Hollich, V., Lassmann, T., Moxon, S., Marshall, M., Khanna, A., Durbin, R., Eddy, S. R., Sonnhammer, E. L., and Bateman, A. (2006). Pfam: clans, web tools and services. Nucleic Acids Res. 34, D247–D251.
Fire, A., Xu, S., Montgomery, M. K., Kostas, S. A., Driver, S. E., and Mello, C. C. (1998). Potent and specific genetic interference by double-stranded RNA in Caenorhabditis elegans. Nature 391, 806–811.
Garcia-Silva, M. R., Frugier, M., Tosar, J. P., Correa-Dominguez, A., Ronalte-Alves, L., Parodi-Talice, A., Rovira, C., Robello, C., Goldenberg, S., and Cayota, A. (2010). A population of tRNA-derived small RNAs is actively produced in Trypanosoma cruzi and recruited to specific cytoplasmic granules. Mol. Biochem. Parasitol. 171, 64–73.
Gardner, P. P., Bateman, A., and Poole, A. M. (2010). SnoPatrol: how many snoRNA genes are there? J. Biol. 9, 4.
Garlapati, S., Saraiya, A. A., and Wang, C. C. (2011). A la autoantigen homologue is required for the internal ribosome entry site mediated translation of giardiavirus. PLoS ONE 6, e18263. doi:10.1371/journal.pone.0018263
Gupta, S. K., Hury, A., Ziporen, Y., Shi, H., Ullu, E., and Michaeli, S. (2010). Small nucleolar RNA interference in Trypanosoma brucei: mechanism and utilization for elucidating the function of snoRNAs. Nucleic Acids Res. 38, 7236–7247.
Haasnoot, J., and Berkhout, B. (2011). RNAi and cellular miRNAs in infections by mammalian viruses. Methods Mol. Biol. 721, 23–41.
Khaliq, S., Khaliq, S. A., Zahur, M., Ijaz, B., Jahan, S., Ansar, M., Riazuddin, S., and Hassan, S. (2010). RNAi as a new therapeutic strategy against HCV. Biotechnol. Adv. 28, 27–34.
Khanna, A., and Stamm, S. (2010). Regulation of alternative splicing by short non-coding nuclear RNAs. RNA Biol. 7, 480–485.
Kolev, N. G., Tschudi, C., and Ullu, E. (2011). RNA interference in protozoan parasites: achievements and challenges. Eukaryot. Cell 10, 1156–1163.
Kolev, N. G., and Ullu, E. (2009). snoRNAs in Giardia lamblia: a novel role in RNA silencing? Trends Parasitol. 25, 348–350.
Langmead, B., Trapnell, C., Pop, M., and Salzberg, S. L. (2009). Ultrafast and memory-efficient alignment of short DNA sequences to the human genome. Genome Biol. 10, R25.
Li, H., and Durbin, R. (2009). Fast and accurate short read alignment with Burrows-Wheeler transform. Bioinformatics 25, 1754–1760.
Li, H., Handsaker, B., Wysoker, A., Fennell, T., Ruan, J., Homer, N., Marth, G., Abecasis, G., and Durbin, R. (2009). The Sequence Alignment/Map format and SAMtools. Bioinformatics 25, 2078–2079.
Li, H., Ruan, J., and Durbin, R. (2008). Mapping short DNA sequencing reads and calling variants using mapping quality scores. Genome Res. 18, 1851–1858.
Liang, X. H., Hury, A., Hoze, E., Uliel, S., Myslyuk, I., Apatoff, A., Unger, R., and Michaeli, S. (2007). Genome-wide analysis of C/D and H/ACA-like small nucleolar RNAs in Leishmania major indicates conservation among trypanosomatids in the repertoire and in their rRNA targets. Eukaryot. Cell 6, 361–377.
Liao, J., Yu, L., Mei, Y., Guarnera, M., Shen, J., Li, R., Liu, Z., and Jiang, F. (2010). Small nucleolar RNA signatures as biomarkers for non-small-cell lung cancer. Mol. Cancer 9, 198.
Lin, W. C., Li, S. C., Lin, W. C., Shin, J. W., Hu, S. N., Yu, X. M., Huang, T. Y., Chen, S. C., Chen, H. C., Chen, S. J., Huang, P. J., Gan, R. R., Chiu, C. H., and Tang, P. (2009). Identification of microRNA in the protist Trichomonas vaginalis. Genomics 93, 487–493.
Lye, L. F., Owens, K., Shi, H., Murta, S. M., Vieira, A. C., Turco, S. J., Tschudi, C., Ullu, E., and Beverley, S. M. (2011). Retention and loss of RNA interference pathways in trypanosomatid protozoans. PLoS Pathog. 6, e1001161. doi:10.1371/journal.ppat.1001161
Macrae, I. J., Zhou, K., Li, F., Repic, A., Brooks, A. N., Cande, W. Z., Adams, P. D., and Doudna, J. A. (2006). Structural basis for double-stranded RNA processing by Dicer. Science 311, 195–198.
McCormick, K. P., Willmann, M. R., and Meyers, B. C. (2011). Experimental design, preprocessing, normalization and differential expression analysis of small RNA sequencing experiments. Silence 2, 2.
Nawrocki, E. P., Kolbe, D. L., and Eddy, S. R. (2009). Infernal 1.0: inference of RNA alignments. Bioinformatics 25, 1335–1337.
Ngo, H., Tschudi, C., Gull, K., and Ullu, E. (1998). Double-stranded RNA induces mRNA degradation in Trypanosoma brucei. Proc. Natl. Acad. Sci. U.S.A. 95, 14687–14692.
Piccinelli, P., Rosenblad, M. A., and Samuelsson, T. (2005). Identification and analysis of ribonuclease P and MRP RNA in a broad range of eukaryotes. Nucleic Acids Res. 33, 4485–4495.
Prucca, C. G., Slavin, I., Quiroga, R., Elías, E. V., Rivero, F. D., Saura, A., Carranza, P. G., and Luján, H. D. (2008). Antigenic variation in Giardia lamblia is regulated by RNA interference. Nature 456, 750–754.
Ridanpaa, M., Ward, L. M., Rockas, S., Sarkioja, M., Makela, H., Susic, M., Glorieux, F. H., Cole, W. G., and Makitie, O. (2003). Genetic changes in the RNA components of RNase MRP and RNase P in Schmid metaphyseal chondrodysplasia. J. Med. Genet. 40, 741–746.
Rivero, M. R., Kulakova, L., and Touz, M. C. (2010). Long double-stranded RNA produces specific gene downregulation in Giardia lamblia. J. Parasitol. 96, 815–819.
Rudra, D., Mallick, J., Zhao, Y., and Warner, J. R. (2007). Potential interface between ribosomal protein production and pre-rRNA processing. Mol. Cell. Biol. 27, 4815–4824.
Ryther, R. C., Mcguinness, L. M., Phillips, J. A. III, Moseley, C. T., Magoulas, C. B., Robinson, I. C., and Patton, J. G. (2003). Disruption of exon definition produces a dominant-negative growth hormone isoform that causes somatotroph death and IGHD II. Hum. Genet. 113, 140–148.
Saraiya, A. A., and Wang, C. C. (2008). snoRNA, a novel precursor of microRNA in Giardia lamblia. PLoS Pathog. 4, e1000224. doi:10.1371/journal.ppat.1000224
Schattner, P., Brooks, A. N., and Lowe, T. M. (2005). The tRNAscan-SE, snoscan and snoGPS web servers for the detection of tRNAs and snoRNAs. Nucleic Acids Res. 33, W686–W689.
Simoes-Barbosa, A., Meloni, D., Wohlschlegel, J. A., Konarska, M. M., and Johnson, P. J. (2008). Spliceosomal snRNAs in the unicellular eukaryote Trichomonas vaginalis are structurally conserved but lack a 5′-cap structure. RNA 14, 1617–1631.
Simpson, J. T., Wong, K., Jackman, S. D., Schein, J. E., Jones, S. J., and Birol, I. (2009). ABySS: a parallel assembler for short read sequence data. Genome Res. 19, 1117–1123.
Smith, A., and Johnson, P. (2011). Gene expression in the unicellular eukaryote Trichomonas vaginalis. Res. Microbiol. 162, 646–654.
Teodorovic, S., Walls, C. D., and Elmendorf, H. G. (2007). Bidirectional transcription is an inherent feature of Giardia lamblia promoters and contributes to an abundance of sterile antisense transcripts throughout the genome. Nucleic Acids Res. 35, 2544–2553.
Uliel, S., Liang, X. H., Unger, R., and Michaeli, S. (2004). Small nucleolar RNAs that guide modification in trypanosomatids: repertoire, targets, genome organisation, and unique functions. Int. J. Parasitol. 34, 445–454.
Vaishnaw, A. K., Gollob, J., Gamba-Vitalo, C., Hutabarat, R., Sah, D., Meyers, R., De Fougerolles, T., and Maraganore, J. (2011). A status report on RNAi therapeutics. Silence 1, 14.
Yang, C. Y., Zhou, H., Luo, J., and Qu, L. H. (2005). Identification of 20 snoRNA-like RNAs from the primitive eukaryote, Giardia lamblia. Biochem. Biophys. Res. Commun. 328, 1224–1231.
Yang, L., Duff, M. O., Graveley, B. R., Carmichael, G. G., and Chen, L. L. (2011). Genomewide characterization of non-polyadenylated RNAs. Genome Biol. 12, R16.
Zerbino, D. R., and Birney, E. (2008). Velvet: algorithms for de novo short read assembly using de Bruijn graphs. Genome Res. 18, 821–829.
Zhang, H., Pompey, J. M., and Singh, U. (2011a). RNA interference in Entamoeba histolytica: implications for parasite biology and gene silencing. Future Microbiol. 6, 103–117.
Zhang, Z. X., Min, W. P., and Jevnikar, A. M. (2011b). Use of RNA interference to minimize ischemia reperfusion injury. Transplant. Rev. (Orlando). PMID: 22000663. [Epub ahead of print].
Keywords: ncRNA, high-throughput sequencing, miRNA, siRNA, snoRNA, Giardia, Trichomonas
Citation: Collins LJ (2011) Characterizing ncRNAs in human pathogenic protists using high-throughput sequencing technology. Front. Gene. 2:96. doi: 10.3389/fgene.2011.00096
Received: 05 November 2011;
Paper pending published: 28 November 2011;
Accepted: 07 December 2011;
Published online: 27 December 2011.
Edited by:
Philipp Kapranov, St. Laurent Institute, USAReviewed by:
Toshifumi Inada, Tohoku University, JapanCopyright: © 2011 Collins. This is an open-access article distributed under the terms of the Creative Commons Attribution Non Commercial License, which permits non-commercial use, distribution, and reproduction in other forums, provided the original authors and source are credited.
*Correspondence: Lesley Joan Collins, Massey University, Palmerston North, New Zealand. e-mail:bC5qLmNvbGxpbnNAbWFzc2V5LmFjLm56
Disclaimer: All claims expressed in this article are solely those of the authors and do not necessarily represent those of their affiliated organizations, or those of the publisher, the editors and the reviewers. Any product that may be evaluated in this article or claim that may be made by its manufacturer is not guaranteed or endorsed by the publisher.
Research integrity at Frontiers
Learn more about the work of our research integrity team to safeguard the quality of each article we publish.