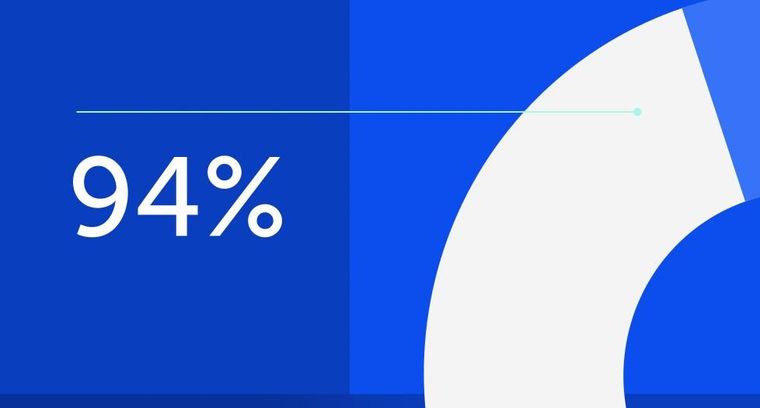
94% of researchers rate our articles as excellent or good
Learn more about the work of our research integrity team to safeguard the quality of each article we publish.
Find out more
ORIGINAL RESEARCH article
Front. Future Transp., 14 August 2023
Sec. Connected Mobility and Automation
Volume 4 - 2023 | https://doi.org/10.3389/ffutr.2023.1196629
Driving is a stressful activity because of the mental workload required to maneuver a vehicle in certain travel contexts, such as congested traffic, multi-modal networks requiring complex interaction with surrounding vehicles, and aggressive driving. Autonomous vehicles (AVs), on the other hand, can reduce the mental workload by performing most of the driving tasks and providing users with a comfortable ride. This study develops a pathway model to relate different health determinants, including travel reliability, safety, driving comfort, and value of time, to Autonomous vehicles driving and studies their impact on the value of driving stress. A case study example of Autonomous vehicles simulation is used to determine the impact of these health determinants. The value of driving stress in Autonomous vehicles is estimated as a function of the value of these individual health determinants. The results show that the perception of safe or unsafe driving in Autonomous vehicles is the most important factor in changing the perception of driving stress in Autonomous vehicles. Similarly, perceptions of comfortable driving in Autonomous vehicles and reduced workload with a higher value of time also reduce driving stress in Autonomous vehicles. These results allow Autonomous vehicles adoption models to explicitly consider driving stress reduction as a benefit and can improve understanding of Autonomous vehicles adoption, which may require quantitative analysis of underlying motivating benefits, including driving stress reduction.
The emergence of vehicle and communication technologies is giving rise to increasing market penetration of automated vehicle (AV) technologies such as advanced driving assistance systems (ADAS). However, it is uncertain how these technologies are perceived and valued by the users, which represents a knowledge gap in the analysis of the cost-effectiveness and adoption of AVs. These technological advancements are expected to bring about a paradigm shift in operations, traffic management, and travel patterns in cities. AVs will benefit transportation systems by improving vehicle throughput, safety, energy, and efficiency. However, in order to assess AV adoption and inform policy or industry decisions, understanding human perceptions of AV driving and their perceived benefits is necessary but challenging.
AVs could replace human-driven vehicles (HVs) and perform most driving tasks with little or no human feedback. According to resource theory (Welford, 1978; Funke et al., 2007; Funke et al., 2011, p. 1305)”, “As task demand increases, more effort is required to perform a task and as a result workload increases [sic]”. Limiting workload can reduce driving stress, which in turn can improve driving comfort and lead to fewer collisions. Similarly, reduced workload in critical driving conditions can also lead to lower stress levels. A reduced workload in AVs will also provide time for additional on-board activities that may not be possible in a human-driven vehicle. However, it is uncertain how increased or decreased workload with transition of controls in AVs may affect driving performance and how changes in workload may affect driving stress in AVs. AVs are expected to have an impact on different mental health determinants, such as travel reliability, safety, driving comfort, and value of time. A positive influence on mental health determinants changes the perception of stress. Previous studies (Funke et al., 2007; Heikoop et al., 2019) have analyzed driving stress as a function of heart rate and other surrogate measures of skin response and eye gaze using instrumented simulators. However, AV driving may be associated with different health determinants, and the link between these AV health determinants and their influence on driving stress is unclear. Moreover, it is uncertain how travelers value driving stress in AVs. This study would fill the existing gaps regarding the relationship between health determinants and driving stress in AVs and answer the following questions:
1) What are the mental health determinants of driving stress in AVs?
2) How do AVs impact driving stress with the reduced workload?
3) What is the value of driving stress in AVs, and how sensitive is driving stress to mental health determinants?
Several previous studies have used driving simulators, surveys, and field experiments to investigate driving behaviors and stress in AVs and electric vehicles. Jamson et al. (2020) used a driving simulator to investigate the ability of drivers to follow the eco-feedback advisories and their propensity to prioritize it over safe driving. They observed improved performance with eco-driving advice. Heikoop et al. (2019) studied driver workload and stress in partially automated vehicles in the real world using heart rate and respiratory measures and observed that the Tesla Model S was associated with a low workload that decreased over time. Arakawa et al. (2018) studied user reliance on the control of autonomous systems and observed that driver mental workload was lower in AVs They also observed distraction from eye gaze during the control transition Funke et al. (2007) used a driving simulator to analyze the effects of driving stress and automation. Consistent with resource theory, they observed that automation had moderate facilitative effects based on reduced stress due to reduced workload.
There have been studies on the value of vehicle automation or technologies and processes that generally reduce mental workload. Bansal et al. (2016) assessed consumers’ willingness to pay (WTP) for AVs using survey data and observed that tech-savvy male respondents who had experienced more collisions in the past had a higher WTP for AVs. Another study (Maness and Lin, 2019) analyzed the effect of free charging on consumers’ likelihood to adopt electric vehicles due to the reduced mental workload of free charging settings. They observed that short-term free public charging increases sales of plug-in electric vehicles. Lin (2012) measured the cost of range anxiety as a function of the value of time, vehicle ownership, charging infrastructure coverage, and driving patterns that contribute to the actual cost and mental workload of dealing with insufficient driving range for known travel needs. They found that reducing driving intensity, increasing vehicle range, and providing better charging infrastructure were effective in reducing range anxiety. Khattak et al. (2018a) studied driver stress in response to traveling on corridors with coordinated and non-coordinated traffic control. They observed that coordinated traffic signals provided a significant improvement in driving stress. Merat et al. (2014) used a driving simulator to study the transition from automated to manual driving. They showed that drivers were able to focus on the center of the road within 15–20 s for the fixed interval transition.
Harb et al. (2018) designed a naturalistic experiment by projecting people into a self-driving world using a free 60-h chauffeur service for 7 days. They observed a significant increase in vehicle miles traveled and the number of trips. Cottrell and Barton (2013) studied the effects of automation on driving stress by considering the personality and environmental factors of individuals and theorized that automation reduces mental workload and stress. Rojas-Rueda et al. (2020) reviewed the public health benefits of AVs, including impacts on traffic safety, physical activity, stress, congestion, urban design, and clean energy. They posited that AVs could improve health. Reduce fatalities from collisions and improve stress. Dean et al. (2019) reviewed the existing literature on AVs with thematic views on road safety, social equity, environment, lifestyle, and built environment for the health implications of AVs. The research provided a conceptual relationship between AV use and health outcomes.
Pudāne et al. (2019) used qualitative data from a focus group survey to study the expectations of travel and on-board activities with AVs. They identified the differences between travelers’ satisfaction with AVs and their potential for travel as an important aspect for modeling. Steck et al. (2018) analyzed the effect of AV driving on the value of time using data from a survey questionnaire that examined travel in privately owned and shared AVs. Time was considered a primary stress factor, and they observed that relieving users from driving and allowing time for additional activities in AVs is considered meaningful. Evans and Wener (2006) assessed how commuting to work by rail affects health and wellbeing in terms of stress. They found that as commute times increased, so did perceived stress levels compared to baseline values. Maier and Wilken (2014) studied the impact of stress on consumer purchasing behavior and discovered that stressed consumers perceive low- and high-level product features differently, which affects their willingness to pay. Bartel et al. (2019) conducted a qualitative assessment of the health risks of rideshare drivers using focus group interviews. They noted that the stressful nature of rideshare work impose stress on drivers, both mentally and physically.
In conclusion, previous studies have mostly relied on small samples from driving simulators to test driver responses to AV driving. These studies have primarily used heart rate sensors and perceived stress scales from surveys to assess driving stress. A few studies have analyzed stress using survey questionnaires, while others have investigated the implications of autonomous driving on travel behavior and conducted qualitative reviews of previous studies on driving stress. However, it is uncertain how travelers value their driving stress in AVs. There is a lack of guidance on how to quantify the value of driving stress (or its reduction) in AVs. Furthermore, the literature lacks a comprehensive assessment of the impact of autonomous driving on driving stress and the link between mental health determinants of AVs (such as congestion, safety, reliability, and value of time) and driving stress The present study develops a framework for linking mental health determinants of AVs to driving stress. The study proposes four mental health determinants, namely, congestion, safety, driving comfort, and value of time, and assesses the impact of AV driving on these mental health determinants. The value of driving stress is quantified as a function of these health determinants using AV simulation case studies.
A pathway model (Kim, 2018), which shows the cause-and-effect relationship in Figure 1, was used to identify the health determinants of AVs that lead to driving stress. The model links different pathways (factors such as congestion, safety, operating costs, reliability, collisions, and driving comfort) that contribute to the outcome of driving stress. AVs will directly reduce congestion and improve travel time reliability. These factors were selected because the literature (Rojas-rueda, 2017; Dean et al., 2019; Rojas-rueda et al., 2020) provided evidence of the relationship between these health determinants and driving stress. Additional factors, such as emergencies and bad weather, may also contribute to driving stress. The role of emergencies has been captured to some extent through incident modeling. However, it was difficult to measure a driver’s own level of fatigue since it can vary from person to person. While driving at night may have some influence on the driver’s ability to maneuver the vehicle, it may also vary from person to person. Since human subject testing was not involved in this study, these factors were not directly considered. Future investigations with human subjects could also incorporate these factors. Congestion lead to longer travel times and more stop-and-go waves in traffic, which increased driving stress (Khattak et al., 2018b). AVs improved travel time reliability, and drivers or travelers were able to reach their destinations on time, thus requiring less buffer time and reducing driving stress. Similarly, safety is another important health determinant that is a leading indicator of driving stress. AVs improved safety and driving comfort by reducing collisions and variability in driving profiles. Since 94% of collisions today (Khattak Z et al., 2020) are caused by human error, AVs are expected to improve safety by replacing the human factors element with automated control. While riding in AVs, travelers experienced a safe and comfortable ride that directly impacted their driving stress and led to reduced driving stress. Similarly, the value of time in AVs (Pudāne et al., 2019) was observed to be different compared to HVs since travelers were able to perform additional activities such as checking emails, watching movies, preparing for work, or other activities while traveling in AVs. Thus, having additional time that could be utilized for valuable activities that would otherwise not have been possible and being relieved from driving itself, also improved the value of time, which in turn impacts driving stress in AVs. A case study example was used to simulate AVs driving on a calibrated network under dynamic conditions. The performance measures from the simulation case study were utilized to estimate the mental health determinants. Furthermore, the mental health determinants were assigned a value in dollars, which was used to calculate the value of stress as a function of these mental health determinants.
Travel time reliability (Bhouri et al., 2016) is a good indicator of network-wide congestion, which is important to both policymakers and road users. Travelers are more concerned about unexpected delays due to congestion because of their greater consequences compared to daily delays Reliability measures capture the variance in travel time and provide an estimate of the time buffers that users need to include in their plans to arrive at their destination on time. The planning time index and buffer index were used to estimate how reliable time is when traveling in AVs because they account for both expected and unexpected delays. According to the Federal Highway Administration (FHWA, 2017a; Khattak et al., 2019, p.8), the planning time index (PTI) can be defined as the ratio of the 95th percentile to free-flow travel time. PTI describes the delay that people are willing to accept, for instance, if they have a tolerance for being late on one weekday per month, how early they need to leave for work PTI compares free-flow traffic time to near-worst-case travel time The PTI equation is:
Similarly, the extra time cushion when planning trips to arrive on time is represented by the buffer index. The time cushion accounts for unexpected delays.
The value of reliability is calculated as a function of the cost of travel, assumed to be the cost of tolls, and the difference in time savings in AVs and HVs.
Conflicts are defined as “A situation with risk of collision between two or more vehicles as they approach each other with [sic] constant position in space and time” (Amundsen, 1977; Chin and Quek, 1997, p.172). The premise for using time to collision (TTC) is its frequent and comparable nature to collisions, thus proactively predicting safety risks. The risk of collisions affects the driver’s perception of stress. While there are several surrogate measures of safety (Gettman and Head, 2003; Cunto and Saccomanno, 2008), TTC is widely used because it reflects the potential for a collision and is easy to calculate. Equation 3 provides the time to collision for successive vehicles.
where the TTC value for the
A rear end conflict is identified by an angle below 30°, while a lane change conflict is identified by an angle below 30–85°. The conflicts represent the likelihood of a collision. TTC thresholds of 1–2s were used based on guidance from previous studies (Papadoulis et al., 2019).
The driving comfort of a vehicle improves the traveling experience and has a direct impact on the driver’s perception of stress. A jerky and bumpy ride with an unpleasant experience is expected to negatively influence the driver’s perception of stress. Thus, driving comfort is estimated from measures of volatility, which express the variation in driving regimes and serve as a leading indicator of driving comfort by identifying critical vehicle maneuvers. Vehicular jerk and Bollinger Bands are used as measures of driving comfort.
Vehicular jerk better represents instantaneous driving volatility by indicating the marginal rate of change in acceleration or deceleration, as shown in Equation 5:
where
Bollinger Bands (Lento et al., 2007) are used to relate the relative moving average of velocities and accelerations to driving volatility. The standard deviation value above or below the mean indicates volatility, while the width and standard deviation indicate the level of volatility. Thus, the upper band (
where
Value of time indicates how much consumers value and are willing to pay for a technology or product. AVs take over most human controls and perform driving tasks in an automated manner. Automated controls in AVs fundamentally change the way people travel, freeing up time for additional activities. Travelers would value their time more in AVs due to the time available for on-board activities. The reduced workload for AVs reduces their perception of driving stress.
A simulation framework, shown in Figure 2, was utilized to model a case study site with AVs simulated through a calibrated network. The AV trajectory, travel time, and conflict data were used to assess the mental health determinants for their influence on AV driving stress. The VISSIM external driver behavior API was used to model the behavior of AVs in C++, and the simulation package was invoked through C#. Multiple factors, including several platoon sizes (3, 5, 7, 10 vehicles), headways of 0.6, 1.2, and 1.8 s, CACC speeds of 60, 65, and 70 mph, deceleration for platooning in the range of -3 ft/s2, 3.5 ft/s2, and 4 ft/s2, and roads with collitions or clear conditions, were used to test the sensitivity of the results as a function of these values. These parameters required multiple simulation runs in order to capture the true stochasticity. Thus, the Latin hypercube sampling approach was used to partition the design space into a small set and limit the simulation replication with multiple parameters to 15. These random seed-based replications capture the stochasticity and were selected based on the minimum sample size. In addition, both HVs and AVs were simulated on the calibrated I-66 network within the microscopic simulator VISSIM to generate the output for analysis.
The AV platoons traveling on a four-lane road network were modeled for the case study. The platooning model consists of both adaptive and cooperative adaptive cruise control (ACC and CACC). To mimic the conditions of congested traffic that could result in driving stress, incident data were also collected from the study site and modeled on the network. The disruption of traffic flow caused by incidents created stop-and-go waves that induced driving stress similar to that of urban roadways. In addition, the human driver behavior model parameters were calibrated for both highway and urban driving. Figure 3 provides an example of AV platoons operating on the network. A 12-mile directional section of I-66 in Northern Virginia was used as the testbed network. The route, located in Washington, D.C., is heavily congested and consists of one high-occupancy lane and three general-purpose lanes. The route has a speed limit of 65mph. A set of traffic and roadway data collected from I-66 in Northern Virginia was used to calibrate the model for HVs. Three days of detector data were collected to calibrate the simulation model in order to capture day-to-day variation in traffic conditions and to have confidence in generalizing the simulation results. Based on these calibration results in Figure 4, the simulation model was well calibrated with field data and thus serves as a good representation of real-world driving conditions at the study site. Different sets of traffic and driving conditions were used to represent the impact of AVs on driving performance compared to HVs, leading to conditions that resulted in the phenomenon of driving stress production.
Figure 5 shows that the AV host scanned the surrounding network for preceding and following vehicles. Speed control with ACC was activated when the distance exceeded the specified threshold in the absence of a preceding vehicle. Conversely, the host became a CACC follower in the presence of a preceding vehicle within the communication range. The time gap determined the specific selection of the gap regulation mode. Furthermore, the host became the platoon leader when the platoon length exceeded a specified maximum length. Otherwise, the AV host used the gap regulation-based logic modes from the previous time.
Platoon AV operation consists of modes including speed control, ACC control, and gap control mode for the CACC vehicles. The platooning algorithm identified the subject vehicle within the platoon during each update interval. The CACC gap regulation mode (Khattak Z H et al., 2020; Khattak et al., 2022) was utilized for a gap less than 2s when the host vehicle was identified within the platoon. The speed and acceleration for the longitudinal motion of the CACC were estimated with Eqs (9-10:
where
The
where
where
The CACC vehicle searched for equipped vehicles when the CACC vehicle itself was not in a platoon. The time lead was 2s and the vehicle was downgraded to ACC (Khattak Z H et al., 2020) in Equation 16 during this search period.
where,
The error (absolute) of the real speeds from the field tests and the simulated speeds in this study were used to calibrate the speed and position gains since the behavior observed from the traceable profiles was string-stable. The final model used values of 0.24
When there was no preceding vehicle and the vehicle was not within the range of the on-board sensors, the speed control mode for ACC (Khattak Z H et al., 2020) was activated, which maintained the vehicle cruising at the desired speed. This mode is given by Equation 17:
where
Furthermore, the model (car following) from the previous time step was used through a hysteresis loop when the clearance distance was between 90 and 130 m. This procedure achieved a smooth transition.
This section shows the experimental results involving the four health determinants and how they relate to driving stress in AVs. A scenario analysis is also presented to analyze the effect of an increase or decrease in each of the health determinants on the value of driving stress.
The value of stress is theoretically related to the mental health determinants discussed in the previous section, as they influence the perception of driving stress. The mental health determinants have been theoretically observed in the literature to influence the perception of driving stress (Rojas-Rueda, 2017; Dean et al., 2019; Rojas-Rueda et al., 2020). The health determinants have associated costs, which provides a framework to formulate the value of driving stress in AVs as a function of travel time reliability, safety, driving comfort, and value of time/cost of operation. The value of the driving stress function, which is a summation of all health determinants, is given in Equation 18:
The terms (a-d) represent the coefficients of these factors, indicating the varying degree to which each factor can contribute to the value of the driving stress. The value of driving stress is calculated considering the value of each individual measure estimated in the later section. The value of driving stress is a function of all the individual estimated values of the health determinants based on the assumptions specified in Sections 5.1–5.6. This value is approximately $18,071 over the annual cycle per mile. The highest percentage corresponds to safety improvement, followed by driving comfort and travel reliability, while the value of time has a nominal impact.
The travel time comparisons for both AV and HV operations are provided in this section. Figure 6 indicates a reduction in travel time with AVs for multiple scenarios involving low, medium, and heavy flows of 500 veh/hr, 2500 veh/hr, and 3500 veh/hr. An average improvement of 27.3% in travel time was observed with AVs as opposed to HVs. It was expected that AVs would improve travel time through platooning, allowing vehicles to move through the network in an efficient manner. Furthermore, travel time reliability indices, consisting of PTI and buffer index, were also estimated to account for both expected and unexpected delays (due to recurring and non-recurring congestion). This is helpful in making intuitive travel decisions, and the improvement in reliability contributed positively to driving stress, i.e., higher reliability led to a reduction in driving stress due to travelers’ confidence in AV to get to their destination on time. An improvement in reliability of 40.9% and 21.1% for PTI and buffer index, respectively, was observed for AVs compared to HVs. This was expected since AVs control driving functions with little to no human feedback and respond to traffic variations in real-time. Thus, improvements in reliability can be attributed to better flow conditions produced by tight platoons. Better flow conditions would change drivers’ perceptions of stress, as they would feel more relaxed if they were confident that they would arrive at their destination on time.
To estimate the reliability value, link flow capacities were assumed to be independent stochastic variables. This assumption simplifies the dependence of stochastic travel time on link flow. We also introduced the average toll cost on the I-66 study section to represent the cost of travel. Considering the average toll cost of $20 and the average reliability, the value of travel time reliability was calculated as a function of cost and average travel time on the links.
where R is the reliability value for a single trip, with a value of $5.87. Considering the total number of trips per day, the conversion to annual value is given by:
where
Driving safety is another important health determinant and has a major impact on the perception of driving stress (Rojas-Rueda, 2017; Dean et al., 2019; Rojas-Rueda et al., 2020). Approximately 30% of collisions (Desmond and Matthews, 2009) have been attributed to driving stress, making it a key contributor to stress. Thus, travelers who perceive AVs as safe would be relaxed, and their driving stress would be lower. The improvement in safety while driving AVs was assessed through a surrogate safety assessment model (Papadoulis et al., 2019). For the sake of brevity, a 2s TTC threshold was used. Figure 7 shows the conflicts for both AV driving and HVs. Conflicts with AVs were negligible compared to conflicts with HVs. An increase or decrease in the probability of conflicts indicates dangerous or safe driving, which affects the perception of driving stress. Travelers who perceived their driving as safe would be less stressed and more relaxed, and an average 60% decrease in conflicts was observed with AV driving. This decrease in conflicts revealed safer driving with AVs and a potential reduction in perceived driving stress. The improvement was expected due to less disruption of traffic flow in tight platoons and the real-time response of AVs to traffic conditions Lane change conflicts were observed to be lower compared to rear-end conflicts. This indicates that rear end conflicts are more critical than lane change conflicts. The conflicts indicate the probability of a collision.
According to the National Highway Traffic Safety Administration (Blincoe et al., 2015), the economic toll and societal harm of motor vehicle crashes in 2010 were $242 billion in economic costs. Since the analysis was conducted in 2020, the Consumer Price Index (CPI) approximated the total cost of a crash in 2020 dollars to be $47,021. It should be noted that the cost of decreased quality of life due to injuries was not included in these values to be conservative in the estimates. The probability of a collision occurring is given by Equation 21, which includes the 2s TTC crashes. This value is utilized in Eq. 22 to estimate the annual cost of crashes.
where
The value is slightly higher than the 0.007% observed in the simulation environment. The calibrated crash rates were also compared to the literature data in Table 1. Crash rates for local and freeway roads in Virginia and other states were collected from the literature and compared to the current estimates. The literature revealed estimates of a 0.0069 collision probability with two lane closures for Virginia freeways estimated from VATraffic (Lan et al., 2021). Furthermore, local and freeway average crash rates from Massachusetts (Mass.gov, 2022) were observed to be 0.0078 and 0.0080, respectively. The 3-year average local and freeway road crash rates for California (Caltrans, 2019) from 2016 to 2019 were observed to be 0.0089 and 0.0083, while the average freeway crash rate for Wisconsin (Szymkowski, 2019) from 2016 to 2020 was observed to be 0.81%. Thus, the estimated value was adjusted accordingly to account for variations in traffic and roadway conditions on both freeways and local roads in multiple states. The difference between the value based on real data and estimates from multiple states was observed to be approximately 5.1%. Thus, the simulation value was adjusted by a 5% increase, giving a value of 0.00735 The value of C per mile was approximately $16,605.
Driving comfort plays a significant role in the perception of stressful or calm driving. Thus, volatility was used to analyze acceleration and deceleration (hard braking) variations. This concept identifies erratic maneuvers and abrupt variations in the driving regime, which are indicative of ride comfort and jerks that are associated with driving stress. These were estimated for AVs driving along with HVs for the case study scenario Figure 8 shows the driving volatility from the perspective of Bollinger Bands. The results show the mean volatility with upper and lower band volatility over time. It was observed that AV driving provided a more comfortable ride with less variation compared to HVs. Low or high volatilities in acceleration and deceleration indicate a smooth or jerky flow of traffic, which creates an uncomfortable ride with serious safety concerns. Deceleration variations are more dangerous due to the consequences of negative jerks. When AV is operating at a constant speed in a platoon, jerks are minimal or zero with a constant acceleration-deceleration profile. These measures of volatility revealed that AV driving is less volatile, with low variations that are relatively constant compared to HV driving. Such low variations and jerks led to a comfortable ride, which is expected to reduce driving stress and make drivers more relaxed. With a comfortable ride, the traveler’s blood pressure would be lower, resulting in reduced driving stress.
FIGURE 8. Volatility in driving regimes for AVs and HVs (“B.B.” stands for Bollinger Bands). (A) Volatility for HVs, (B) Volatility for AVs.
Previous research (Wali et al., 2018) has shown that a 1% increase in volatility led to a 1.25% increase in crash frequency. Therefore, driving comfort, quantified by volatility in this research, was related to crashes in order to estimate the monetary value of driving comfort. An average 15% decrease in volatility was observed with AVs, corresponding to an 18.75% reduction in crashes. Considering the 38 crashes in AVs, the 18.75% decrease in crashes gives a value of 30.87. The value of driving comfort is given in Equation 24.
where
Automated vehicles (AVs) will fundamentally change the way people travel, freeing up time for additional activities as they replace human control and perform most driving actions. The concept that time spent in AVs can be used for a range of additional activities increases the value of time. This additional time provided by AVs benefits working people, those requiring assistance and those without license by utilizing time for work, shopping or to satisfy basic human needs such as sleeping, talking, eating, and drinking. This additional time granted by AVs reduces the overall workload, leading to less driving stress. While different AV users may value time differently, a recent study (Becker et al., 2018) analyzed the value of time for AV users from three different countries from a sample of 2500 participants. The study captured a broad spectrum of demographics, population, and travel time. The researchers observed a higher level of acceptance of the concept with time and familiarity with AVs. AV users from Germany were observed to value time in AVs as $20, Japanese were willing to pay $17 while Americans were willing to pay $16 for additional hour in AVs. Their value depended on their use of time in AVs for communication and entertainment related activities. Since our case study example consists of 1 h of AV driving, this translates to an average value of time of $16 for additional activities.
where
The value of driving stress for both AVs and HVs was also analyzed with individual health determinants. Figure 9 shows lower values for the health determinants, including travel reliability, safety, comfort, and value of time, for AVs compared to HVs. This contributed to an average 35.2% lower value for driving stress in AVs The findings are intuitive since AVs improve the driving experience and relieve travelers from exhausting driving tasks. In addition, the influence of increasing traffic flow ranging from low flow (1600 veh/hr) to medium flow (2400 veh/hr) and heavy flow (4500 veh/hr) on the value of driving stress was also analyzed, as shown in Figure 10. Overall, an increasing trend in the value of driving stress was observed, moving from lower flow to higher flow. However, only a marginal increase in driving stress was observed at higher traffic flows. This finding can be attributed to the ability of AVs to smooth the traffic flow and maneuver most driving tasks with automated controls in an efficient manner.
This section provides an assessment of driving stress in relation to the four health determinants of AV driving. A scenario analysis was conducted with a 20% and 50% increase and decrease in the value of the health determinants, and the effect of these changes on driving stress in AVs was observed
Travel time reliability indicates how reliable AV travel is and whether travelers can reach their destination on time. This, however, depends on the travel route and traffic congestion; AVs can smooth out traffic waves and improve reliability. The impact of travel reliability on the value of driving stress was tested with 20% and 50% more or less reliable travel. The improvement in reliability is indicated by lower values, showing less time required for planning travel. For instance, reducing the reliability values by 50%. Figure 11 shows that as travel time reliability improves, the value of driving stress decreases, while the value of driving stress increases as the value of travel time reliability increases. Improved reliability with AVs indicates lower congestion and improved flow conditions, resulting in lower driving stress.
The perception of safe driving plays a critical role in the traveler’s perception of driving stress. The improvement in safety conditions in turn improves vehicle maneuvering and perception reaction time. Since AVs can respond more efficiently to changing road and traffic conditions compared to HVs, the risk of involvement in safety-critical events is lower. This scenario tested the impact of crash risk involvement on driving stress by varying crash risks from positive to negative at 20% and 50%. Compared to the base case, a 50% increase in crash risk resulted in the highest increase in driving stress, as shown in Figure 12 This indicates that improved safety perceptions could increase AV adoption and consumer confidence in using AVs by reducing perceived driving stress. Furthermore, a 50% reduction in crash risk led to a net reduction in the value of driving stress of almost 45%. This was expected due to the direct relationship between stress and safety.
In this scenario, the driving stress response was tested with respect to driving comfort by increasing or decreasing its value by 20% and 50%, respectively. A more comfortable drive with fewer jerks and stop-and-go waves is expected to lower blood pressure and reduce driving stress (Khattak et al., 2018a). Figure 13 shows that reducing the value of driving comfort (indicated by higher volatility) increased the value of driving stress, while improving driving comfort reduced the value of driving stress. This is due to the fact that AVs offer a comfortable ride with constant acceleration and deceleration.
This scenario assesses the influence of the value of time on driving stress. The value of time is related to income level, and higher-income individuals are expected to have a higher value of time. Thus, AV users are expected to have a higher value of time, and increasing the value of time in Figure 14 shows a decrease in the value of driving stress in AVs. This was expected because travelers were comfortable performing additional activities in AVs that would otherwise not have been possible and due to the reduced workload in AVs. Similarly, a reduction in the value of time increased the value of driving stress. An average reduction of 6.94% was observed in the value of driving stress, with an increase in the value of time.
Driving is a stressful activity due to the mental workload required to maneuver a vehicle in congested traffic and the complex interaction with surrounding vehicles. Autonomous vehicles, on the other hand, can reduce the mental workload by performing most of the driving tasks and providing a comfortable ride to users. This study develops a pathway model to relate different mental health determinants, including travel reliability, safety, driving comfort, and value of time, to AV driving and investigates their impact on reducing driving stress. A case study example of AV simulation is used to determine the impact of these health determinants. The value of driving stress in AVs is estimated as a function of these health determinants.
The scenario analysis of driving stress in AVs revealed that safety and driving comfort are the health determinants that significantly contributed to the traveler’s perception of driving stress. This is intuitive since AVs can respond more efficiently to changing road and traffic conditions compared to HVs, reducing the risk of involvement in safety-critical events. AVs also operate at a constant speed in a platoon, with minimal jerks and a constant acceleration-deceleration profile. Therefore, drivers feel safer and more comfortable in AVs compared to HVs. As a result, AVs have the potential to reduce driving stress and make travel safer and more comfortable. These results have the potential to improve AV behavioral models in terms of accounting for driving stress and to improve AV adoption by demonstrating the benefits of AVs in terms of relieving commute stress.
The original contributions presented in the study are included in the article/supplementary material, further inquiries can be directed to the corresponding author.
All authors listed have made a substantial, direct, and intellectual contribution to the work and approved it for publication.
The research was supported through Vehicle Technologies Office of the U.S. Department of Energy.
This research was supported by the DOE Office of Energy Efficiency and Renewable Energy, Vehicle Technologies Office, and used resources at the National Transportation Research Center at Oak Ridge National Laboratory, a user facility of the DOE Office of Energy Efficiency and Renewable Energy. The authors are solely responsible for the views expressed in this study.
The authors declare that the research was conducted in the absence of any commercial or financial relationships that could be construed as a potential conflict of interest.
All claims expressed in this article are solely those of the authors and do not necessarily represent those of their affiliated organizations, or those of the publisher, the editors and the reviewers. Any product that may be evaluated in this article, or claim that may be made by its manufacturer, is not guaranteed or endorsed by the publisher.
Amundsen, H. (1977). “Traffic conflicts,” in Proceedings of the first workshop on traffic conflicts (Oslo: Institute of Transport Economics).
Arakawa, T., Hibi, R., and Fujishiro, T. (2018). Psychophysical assessment of a driver ’ s mental state in autonomous vehicles. Transp. Res. Part A 124, 587–610. doi:10.1016/j.tra.2018.05.003
Bansal, P., Kockelman, K. M., and Singh, A. (2016). Assessing public opinions of and interest in new vehicle technologies: an Austin perspective. Transp. Res. Part C 67, 1–14. doi:10.1016/j.trc.2016.01.019
Bartel, E., Maceachen, E., Reid-musson, E., Meyer, S. B., Saunders, R., Bigelow, P., et al. (2019). Stressful by design: exploring health risks of ride-share work. J. Transp. Health 14, 100571. doi:10.1016/j.jth.2019.100571
Becker, T., Herrman, F., Duwe, D., Stegmueler, S., Rockle, F., and Unger, N. (2018). Enabling the value of time: implications for the interior design of autonomous vehicles. Fraunhefer, Cordence Horvath.
Bhouri, N., Aron, M., and Scemama, G. (2016). Travel time reliability with and without the dynamic use of hard shoulder: field assessment from a French motorway. J. Traffic Transp. Eng. Engl. Ed. 3, 520–530. doi:10.1016/j.jtte.2016.01.008
Blincoe, L., Miller, T. R., Zaloshnja, E., and Lawrence, B. A. (2015). The economic and societal impact of motor vehicle crashes. USA: National Highway Traffic Safety Administration. Available at: https://crashstats.nhtsa.dot.gov/Api/Public/ViewPublication/812013.
Caltrans (2019). Crash data on California state highways. California: California Department of Transportation and US Department of Transportation.
Chin, H.-C., and Quek, S.-T. (1997). Measurement of traffic conflicts. Saf. Sci. 26, 169–185. doi:10.1016/S0925-7535(97)00041-6
Cottrell, N. D., and Barton, B. K. (2013). The role of automation in reducing stress and negative affect while driving. Theor. Issues Ergonomics Sci. 14, 53–68. doi:10.1080/1464536X.2011.573011
Cunto, F., and Saccomanno, F. F. (2008). Calibration and Validation of simulated vehicle safety performance at signalized intersections. Accid. Analysis Prev. 40, 1171–1179. doi:10.1016/j.aap.2008.01.003
Dean, J., Wray, A. J., Braun, L., Casello, J. M., Mccallum, L., and Gower, S. (2019). Holding the keys to health? A scoping study of the population health impacts of automated vehicles. BCM Public Health 19, 1258–1310. doi:10.1186/s12889-019-7580-9
Desmond, P. A., and Matthews, G. (2009). Individual differences in stress and fatigue in two field studies of driving. Transp. Res. Part F Traffic Psychol. Behav. 12, 265–276. doi:10.1016/j.trf.2008.12.006
Evans, G. W., and Wener, R. E. (2006). Rail commuting duration and passenger stress. Health Psychol. 25, 408–412. doi:10.1037/0278-6133.25.3.408
FHWA (2017b). National Household travel survey [WWW document]. Federal highway administration. Available at: https://nhts.ornl.gov/(accessed 5 1, 18).
FHWA (2017a). Travel time reliability: making it there on time, all the time. USA: US Department of Transportation. Available at: https://ops.fhwa.dot.gov/publications/tt_reliability/ttr_report.htm.
Funke, G. J., Knott, B. A., Salas, E., Pavlas, D., and Strang, A. J. (2011). Conceptualization and measurement of team workload. A Crit. Need 54, 36–51. doi:10.1177/0018720811427901
Funke, G., Matthews, G., Warm, J. S., and Emo, A. K. (2007). Vehicle automation: A remedy for driver stress ? Vehicle automation: a remedy for driver stress. Ergonomics 50, 1302–1323. doi:10.1080/00140130701318830
Gettman, D., and Head, L. (2003). Surrogate safety measures from traffic simulations models. New York: Federal Highway Administration.
Harb, M., Xiao, Y., Circella, G., Mokhtarian, P. L., and Walker, J. L. (2018). Projecting travelers into a world of self - driving vehicles: estimating travel behavior implications via a naturalistic experiment. Transportation 45, 1671–1685. doi:10.1007/s11116-018-9937-9
Heikoop, D. D., Winter, J. C. F. De, Arem, B. V., and Stanton, N. A. (2019). Acclimatizing to automation: driver workload and stress during partially automated car following in real traffic. Transp. Res. Part F 65, 503–517. doi:10.1016/j.trf.2019.07.024
Jamson, S. L., Hibberd, D. L., and Jamson, A. H. (2020). Drivers ’ ability to learn eco-driving skills; effects on fuel efficient and safe driving behaviour. Transp. Res. Part C 44, 657–668. doi:10.1016/j.trc.2015.02.004
Khattak Z H, Z. H., Fontaine, M. D., and Smith, B. L. (2020). Exploratory investigation of disengagements and crashes in autonomous vehicles under mixed traffic: an endogenous switching regime framework. IEEE Trans. Intelligent Transp. Syst. 22, 7485–7495. doi:10.1109/TITS.2020.3003527
Khattak Z, Z., Smith, B. L., Park, H., and Fontaine, M. D. (2020). Cooperative lane control application for fully connected and automated vehicles at multilane freeways. Transp. Res. Part C 111, 294–317. doi:10.1016/j.trc.2019.11.007
Khattak, Z. H., Fontaine, M. D., and Boateng, R. A. (2018b). Evaluating the impact of adaptive signal control technology on driver stress and behavior. Transp. Res. Board 97th Annu. Meet. 58, 133. doi:10.1016/j.trf.2018.06.006
Khattak, Z. H., Fontaine, M. D., and Boateng, R. A. (2018a). Evaluating the impact of adaptive signal control technology on driver stress and behavior using real-world experimental data. Transp. Res. Part F 58, 133–144. doi:10.1016/j.trf.2018.06.006
Khattak, Z. H., Smith, B. L., Fontaine, M. D., Ma, J., and Khattak, A. J. (2022). Active lane management and control using connected and automated vehicles in a mixed traffic environment. Transp. Res. Part C 139, 103648. doi:10.1016/j.trc.2022.103648
Khattak, Z., Magalotti, M., and Fontaine, M. (2019). Operational performance evaluation of adaptive traffic control systems: a bayesian modeling approach using real-world gps and private sector probe data. J. Intelligent Transp. Syst. 24, 156–170. doi:10.1080/15472450.2019.1614445
Kim, D. H. (2018). Development of a psychological pathway model linking lighting quality to well-being in indoor café environments. Indoor Built Environ. 27, 390–401. doi:10.1177/1420326X17753512
Lan, C.-L., Zhao, M., Goodall, N., and Gillespie, J. (2021). Cost of congestion due to incidents on freeways. Report. Richmond, VA: Virginia Department of Transportation.
Lento, C., Gradojevic, N., and Wright, C. S. (2007). Investment information content in bollinger bands? Appl. Financ. Econ. Lett. 3, 263–267. doi:10.1080/17446540701206576
Lin, Z. (2012). Measuring range anxiety: the substitution - emergency - detour (SED) method. World Electr. Veh. J. 5, 8–13. doi:10.3390/wevj5010008
Maier, E., and Wilken, R. (2014). The impact of stress on consumers ’ willingness to pay. Psychol. Mark. 31, 774–785. doi:10.1002/mar.20733
Maness, M., and Lin, Z. (2019). Free charging: exploratory study of its impact on electric vehicle sales and energy. Transp. Res. Rec. 2673, 590–601. doi:10.1177/0361198119844966
Mass.gov (2022). Intersection and roadway crash rate data for analysis Massachusetts Department of Transportation. Available at: https://www.mass.gov/service-details/intersection-and-roadway-crash-rate-data-for-analysis.
Merat, N., Jamson, A. H., Lai, F. C. H., Daly, M., and Carsten, O. M. J. (2014). Transition to manual: driver behaviour when resuming control from a highly automated vehicle. Transp. Res. Part F Psychol. Behav. 27, 274–282. doi:10.1016/j.trf.2014.09.005
Papadoulis, A., Quddus, M., and Imprialou, M. (2019). Evaluating the safety impact of connected and autonomous vehicles on motorways. Accid. Analysis Prev. 124, 12–22. doi:10.1016/j.aap.2018.12.019
Pudāne, B., Rataj, M., Molin, E. J. E., Mouter, N., Cranenburgh, S. V., and Chorus, C. G. (2019). How will automated vehicles shape users ’ daily activities? Insights from focus groups with commuters in The Netherlands. Transp. Res. Part D 71, 222–235. doi:10.1016/j.trd.2018.11.014
Rojas-Rueda, D. (2017). Autonomous vehicles and mental health. J. Urban Des. Ment. Health 41, 329. doi:10.1146/annurev-publhealth-040119-094035
Rojas-rueda, D., Nieuwenhuijsen, M. J., Khreis, H., and Frumkin, H. (2020). Autonomous vehicles and public health. Annual Reviews of Public Health.
Steck, F., Kolarova, V., Bahamonde-Birker, F., Trommer, S., and Lenz, B. (2018). How Autonomous Driving may affect the value of travel time savings for commuting. Transp. Res. Rec. 2672, 11–20. doi:10.1177/0361198118757980
Szymkowski, R. (2019). Statewide average crash rates and upper control limits. USA: Wisconsin Department of Transportation.
Wali, B., Khattak, A. J., Bozdogan, H., and Kamrani, M. (2018). How is driving volatility related to intersection safety ? A bayesian heterogeneity-based analysis of instrumented vehicles data. Transp. Res. Part C 92, 504–524. doi:10.1016/j.trc.2018.05.017
Keywords: driving stress, volatility, automated vehicles (AV), travel reliability, value of time, connected vehicles (CV)
Citation: Khattak ZH and Lin Z (2023) Quantifying automated vehicle benefits in reducing driving stress: a simulation experiment approach. Front. Future Transp. 4:1196629. doi: 10.3389/ffutr.2023.1196629
Received: 30 March 2023; Accepted: 11 May 2023;
Published: 14 August 2023.
Edited by:
Min Yang, Southeast University, ChinaCopyright © 2023 Khattak and Lin. This is an open-access article distributed under the terms of the Creative Commons Attribution License (CC BY). The use, distribution or reproduction in other forums is permitted, provided the original author(s) and the copyright owner(s) are credited and that the original publication in this journal is cited, in accordance with accepted academic practice. No use, distribution or reproduction is permitted which does not comply with these terms.
*Correspondence: Zulqarnain H. Khattak, emtoYXR0YWtAY211LmVkdQ==, ems2Y3FAdmlyZ2luaWEuZWR1
Disclaimer: All claims expressed in this article are solely those of the authors and do not necessarily represent those of their affiliated organizations, or those of the publisher, the editors and the reviewers. Any product that may be evaluated in this article or claim that may be made by its manufacturer is not guaranteed or endorsed by the publisher.
Research integrity at Frontiers
Learn more about the work of our research integrity team to safeguard the quality of each article we publish.