- 1Department of Enterprise Engineering, University of Rome Tor Vergata, Rome, Italy
- 2Dipartimento di ingegneria dell’Informazione, delle Infrastrutture e dell’Energia Sostenibile, Mediterranea University of Reggio Calabria, Reggio Calabria, Italy
Recently, the evolution of emerging information and communication technologies (e-ICTs) has opened the road for developing and implementing new integrated and dynamic city logistics solutions and subsequently for identifying new frontiers of intelligent transport systems (ITSs). The study evolves pointing out the different city logistics groups of actors and their reciprocal interactions in relation to the considered new technologies (i.e., internet of things, block chain, big data, and artificial intelligence), which allow actors to increase their utility exploiting the advancement of current intelligent transport systems. The changes in the generalized path costs supported by each stakeholder are explored, and the modifications guided by e-ICTs are analyzed. The learning process due to the update for within-day and day-to-day dynamics is detailed and formalized. In this way, the current formulation of the classical transport problem (vehicle routing and scheduling) and the new one (pushed from the growth of ecommerce, i.e., courier routing) are explored under the light of the new knowledge derived from the use of e-ICTs. Novelty of the study mainly comprises the analysis of each emerging ICT group and of the formalization, in the classical transport system models (TSM), of the new knowledge for each actor group.
Introduction
Recently, the evolution of emerging information and communication (e-ICT)–based technologies has opened the road for developing and implementing new integrated and dynamic city logistics solutions (Atzori et al., 2010; Taniguchi et al., 2018; Schroten et al., 2020; Russo and Comi, 2021). The e-ICTs optimized to develop transport systems are, in fact, the real advanced intelligent transport systems (ITSs), which are useful for establishing a new generation of city logistics systems for supporting goods operations. Then, these new technologies allow different actors (as discussed in Section 2) to participate directly in city logistics:
1. to optimize, at a very high level, their own operations (e.g., delivery time);
2. to integrate different platforms used for managing and controlling urban freight transport systems, aiming to optimize and integrate dynamically the operations of all involved actors.
As largely investigated (Russo and Comi, 2020), city logistics issues in terms of sustainability cross problems between
• environment/society and economy (sustainable development, as pointed out by Sustainable Development Goal 11—make cities and human settlements inclusive, safe, resilient, and sustainable; UN, 2015) and
• static solution today and main next steps to introduce/integrate dynamics (smart development; Ang et al., 2022).
Therefore, the possibility given by the e-ICTs can move from fleet tracing (Comi and Polimeni, 2021), which allow companies to monitor and find out the gaps in statutory and regulatory requirements as well as to process real-time information on weather conditions, roadblocks, traffic congestion, or to plan a fuel-saving route. Therefore, ITSs, and more in general ICTs, include opportunity for each private (i.e., transport and logistics operators, retailers, and end consumers) or public actor (i.e., public administrations) to increase their own utility.
It should be noted that the considered emerging technologies, in the ICT field, have been developed and used, in the last 10 years, in many sectors. These technologies are then mature; however, they could be considered emerging, or more appropriately as new, just for city logistics due to their current limited use (He and Haasis, 2019). Thus, the emerging ICTs belong to the ITSs. In addition, it has to be stated that there is an increase, in the last 10 years, with an exponential increase in the new technologies in many industrial areas. In the study, the use of new or emerging technologies is, as discussed as follows, strictly related to the field of city logistics, while the technologies that mainly refer to the characteristics of vehicles, such as electric propulsion and automation (e.g., warehouse management), are out of the scope of this study. However, these technologies are particularly important for increasing safety (e.g., crash sensors) and reducing environmental (e.g., diesel particulate filter) impacts, considering that the vehicle stands alone.
It should be noted that there is a large number of e-ICTs (Schroten et al., 2020). Therefore, below macro-aggregation of them will be considered, and four groups of technologies are identified because they are useful for establishing smart city logistics (Nikitas et al., 2020; Russo and Comi, 2021).
These emerging technologies (e-ICTs) include the following: internet of things (IoT), block chain (BC), big data (BD), and artificial intelligence (AI). They are directly inserted in the owners’ platform of each stakeholder to increase the performance and more, in general as said, to increase their specific utility. These technologies are, at today, mature and are ICT, but the current evolution toward the transportation system produce, or will produce, a significant advancement in the specific area of ITS.
As it can be seen, in the following, each technology can be considered stand-alone and can be used by single actor, and the impact on the city logistics could be valid. However, today, telematics is evolving offering to city logistics actors the opportunity to use them jointly. Thus, a new level of results germinates both from the viewpoint of transport and logistics operators, retailers and couriers (e.g., operation cost reduction), and collectivity (e.g., reduction of externalities).
In addition, these emerging technologies can make a significant advancement to city logistics in all cities within all countries. They give win-win results when the same device systems are used in a cooperative glance among different stakeholders. Their function includes, for example, collecting data, storing data, and analyzing data for improving existing urban freight transport systems. Subsequently, the open questions to which the study contribute are the following:
• How to aggregate actors and how to identify and aggregate/integrate the emerging ICTs? (Section 2)
• Given the main generalized path problems, where does the emerging technologies impact on classical problem formulation? (Section 3)
Therefore, the study aimed to give macro answers to questions relative to the improvement of city logistics actors’ utilities through the dynamic integration of different parts of the owners’ platforms (for each actor) and different actors’ platforms of the same group (e.g., set of transport and logistics operators) or of different groups of actors (e.g., set of transport and logistics operators vs. set of retailers). Subsequently, the first objective of the study is to investigate and formalize the different city logistics groups of actors to explore their interactions (Section 2). Below, actors/stakeholders will be used as synonymous, but, in general, actor is preferred when, inside the process, all the groups have the same decisional power, otherwise the stakeholder is chosen when the group of public administrations makes the rule without an explicit sharing with the other involved groups.
In addition, nowadays, collaboration in city logistics operations can play a key role for every actor’s process because it can contribute to improve the sustainable development of the city (Gómez-Marín et al., 2020). Therefore, another objective of the research is to study the opportunity for city logistics to integrate the different actors through the definition of new functionalities supplied by new technologies, while fostering collaboration among practitioners and scholars. It will be considered in detail, for the strong impacts on the city sustainable development, the first two-actor class: enterprise (namely, freight transport and logistics operators) and public administration. Then, recalling studies carried out by Russo and Comi (2021) and Russo and Comi (2022) in integrating opportunities offered by e-ICTs into urban freight transport modeling, the study evolves pointing out the different technologies able to support enterprise freight management. For each class, then, the stand-alone use is considered, and the integrated dynamic use is pointed out. Then, the study proposes a unified formulation, within the theory of transport system modeling (TSM), of the problems related to transport management systems, with the use of e-ICT for the doubly dynamic learning process of path costs.
The study is structured as follows. Section 2 deals with respect to the first objective by reviewing city logistics actors’ aims and needs, pointing out their relations and identifying which functions should be foreseen in order to improve/support their activities presenting the useful e-ICTs. In Section 3, the problems relative to main on-road operations are recalled, and the emerging technologies used by enterprise implemented mainly in own account are collected and presented, linking each theoretical problem with the specific emerging technology. Finally, conclusions and the road ahead are drawn in Section 4, pointing out the benefits deriving for planners (in optimizing urban space and times for meeting the city users’ needs) and researchers (in developing tools able to increase the utility of each actor involved in urban goods movements).
Aggregate Actors and Emerging Technologies Involved in City Logistics
City Logistics Actors
The emerging ICTs, and more in general ITSs (consolidated and advanced), include opportunity for each private or public actor of city logistics to increase the own utility. The single actors, even if a small town is considered, are in hundreds. It is possible to consider some aggregations deriving from the homogeneous use of the emerging technologies, and subsequently four classes with similar potential use can be identified:
• transport and logistics operators (transport enterprises) could optimize their choice including vehicle load, routing, and scheduling, as well as the delivery travel time in terms of last mile operations, i.e., at-customer deliveries, and their part of reverse logistics (Kin et al., 2017; Ruiz-Meza et al., 2021);
• public administration, in its different levels and branches, could optimize the sustainability/liveability of the city in terms of better use of urban public space (both those destined to drive and those destined to park) with respect to all the different demand components (i.e., passengers and freight) using different mode-services (Calabrò et al., 2019; Hadavi et al., 2019; Trecozzi et al., 2022); it can be noted that the role of public administration is twofold, and from one side, it has the commitment to organize public spaces for freight vehicles driving and parking (in a strategic view and then in an off-line design); on the other side, it can have the role of supply information on park and path in real time and to manage reservation (in operational view and then in on-line management and control); therefore, the main role of emerging ICTs is linked to the dynamic real-time connection and all the new options coming from there;
• retailers could optimize their restocking process and integrate the freight receiving operations within their selling activity, considering also the payments; they could also optimize their part of reverse logistics reducing their estate costs and minimizing (or nulling) the inventory cost (Gonzalez-Feliu and Peris-Pla, 2017; Rai et al., 2019);
• end consumers which can benefit, from one side, as citizens for the reduction of traffic due to city logistics optimizations and for the increase of liveability due to the improvement of the safety and the reduction of pollution emissions; on the other hand, as consumers, for the availability of new delivery services (e.g., instant deliveries; Dablanc et al., 2017).
Each actor class uses the ICTs and mainly the emerging ones, obtaining a reduction of own cost and then a modification of cost at fixed flow. Then, there is a first great earning for each actor just improving the utility for a single actor inside its class (i.e., individual utility) and subsequentially for all the actors belonging to each class (i.e., shared utility).
Today, and even more tomorrow, the opportunity offered by the emerging ICTs allows among the other to collect, store, and share data and then to use advanced real-time or off-line procedures to analyze data for improving existing urban freight transport systems. In this way, a new generation of utility is born. The utility derives from use of the interaction between the different classes of technologies. Some interactions at a very rough level are tested today in some city, but the new technology will explain its potentiality in the next years. The main earning will be, at a first time, the static integration of the platforms and at the highest level with the dynamic integration of the platforms.
Just to introduce the great opportunity that derives from the dynamic integration of platforms, it is possible to consider that:
• transport and logistics operators (enterprises) optimize their operations using real-time routing and scheduling actualized by information coming from public management and private retailer; they optimize the travel time according to the given time windows and loading/unloading times using area for freight operations (delivery bays) and the at-receiver (e.g., retailer) actualized information, e.g., info on their availability to receive goods;
• public administrations optimize freight vehicle paths and parks. On the time other than in the space, shared services for supporting integrated dynamic platform to manage and control city logistics system are provided; they can introduce local or global intelligence as it will be recalled as follows;
• retailers optimize their daily schedule for restocking, avoiding interferences between selling and freight receiving activities; they optimize their part of reverse logistics considering the dynamic actualization of loading space availability;
• end consumers who can benefit, as consumers (freight receivers), of the optimization of at-home deliveries and can have real-time information about the home delivery.
Nowadays, the opportunity offered by telematics in fostering collaboration in logistics is becoming a key factor to every business process. It allows competitiveness of firms in the global markets (Banco Mundial, 2008) to be increased. In city logistics, this is not an exception, and the tendency to use electronic collaboration is increasing day by day. Therefore, the future research directions should be devoted toward the development of an integrated platform for innovative city logistics, creating unified space for all the stakeholders with the emerging technologies like the ones built in the port communities with the Port Community System (PCS; Moros-Daza et al., 2020; Russo and Musolino, 2021). The city logistics community system could be defined as an interorganizational system used for enabling commercial services and providing information exchange between the freight receivers (e.g., end consumers and retailers) and a variety of actors, such as transport and logistics operators (e.g., carriers and couriers), among others, under the rules defined by public administrations. Therefore, such a system could become a reference in developing city logistics and contributes to consolidate the innovation coming from logistics as a service (LaaS) as happened in the passenger mobility with the introduction of mobility as service (MaaS). For example, transport and logistics operators to accomplish the requirements coming from receivers (e.g., retailers) when delivering, such as preferred time for picking up and delivering, can benefit from the emerging technologies in planning their arrivals at customers. On the other hand, also the relationship by such enterprises with end consumers can benefit. For examples, the growing share of e-commerce cause (Campisi et al., 2021) relevant problems with home deliveries, which can be mitigated by the introduction of tracing and tracking applications as well as by lockers/parcel points (Dablanc et al., 2017; Mommens et al., 2021).
Emerging Technologies Impacting on City Logistics
The application field of the emerging technologies becomes every day larger and more popular. It is needed to define the edges with respect to the studied topics of city logistics. It is also needed to identify some classes of new technologies that present homogeneity with respect to the investigated topics.
In this way, the investigation of the technologies that involve the transport, but that refer to the characteristics of vehicles is out of the scope of this study, as briefly discussed earlier. In this field, there are many considerable new technologies on active and passive safety. For example, safety passive devices are the airbag systems, or the adaptive braking systems (ABS), traction control system (TCS), and electronic stability control (ESP). All these devices impact directly on transport safety and only indirectly on city logistics, as shown in Russo and Comi (2017). In the same way, it is possible to consider the new technologies that involve only logistics. In this field, there are different technologies that support the management of warehouses toward the full automation, e.g., in the warehouse, the augmented-reality that can be used in different forms with different devices. In this case, the direct impacts of these devices are only on logistics and only indirectly on city logistics (Cirulis and Ginters, 2013).
Restricting the field to the technologies that impact directly on city transport and logistics, there are still a large number of elements. It is possible to consider aggregation of elements that define homogeneous class of ICTs useful for city logistics. Just considering the emerging technologies, which, although existing, are currently under further development for city logistics, they are used as stand-alone or crossing other technologies, generating hybrid tools, sometimes mix of software and hardware.
Finally, the automotive industry is pushing to develop autonomous vehicles for urban freight transport. In fact, autonomous vehicles can guide the definition of new delivery services to city inhabitants (Nuzzolo et al., 2019), as shown by some tests performed by autonomous trucks or autonomous robots for urban deliveries (Haas and Friedrich, 2017; Baum et al., 2019; Bakach et al., 2022). They would allow us to reduce delivering costs and to mitigate the negative impacts by transport. Although they will be the future evolution of last-mile operations, at today, they are prototypes, and subsequently, they are not further pointed out in this study (Kim et al., 2020; Vermesan et al., 2021; Büyüközkan and Ilıcak, 2022).
With all these warnings and analyzing the scientific and technological literature, it is possible to identify four main classes of technologies that impact directly on city logistics toward a smart city logistics. These emerging technologies considered below include internet of things (IoT), block chain (BC), big data (BD), and artificial intelligence (AI). These technologies can contribute to develop an integrated platform for managing dynamically freight transport systems in urban areas.
In the consolidated approach, each emerging technology can be considered stand-alone, and the research develops each technology specifically for each class of actors. From a general point of view, it can be considered that the basis is IoT, when data refer to “value” that is immaterial it passes to block chain (or Internet of Value—IoV). IoT and BC, every day, generate a large amount of data (BD) that consolidated algorithms cannot treat, and then the opportunity offered by BD is derived. If from data (normal or big), a set of decision will derive, with the hierarchization from the best to the worst sub different criteria, the AI can be used.
Internet of Things
The internet of things (IoT) describes the set of two meta-elements: physical objects—“things”—that are embedded with sensors, software, and other technologies for the purpose of exchanging data, and a telematics network qualified to link these objectives with other devices and systems.
For IoT, it is difficult to find a shared definition, but it is possible to mark the emerging and realized systems. First, let the last-mile deliveries be considered. It is highly dependent on labor, and as consumer demand becomes more sophisticated and delivery points continue to multiply, logistics providers face new challenges (Atzori et al., 2010; DHL, 2015). They need to find new solutions for this important stage in the supply chain, e.g., cost-effective solutions that provide value for the end customer and operational efficiency for the logistics provider. In the last mile, IoT connects the enterprises with receivers (e.g., end consumers and retailers) supporting the dynamic implementation of new business models (e.g., the same principle could be applied to the parcel box, to accommodate the e-commerce purchases). In addition, automatic restocking or anticipatory shipping solutions can be developed: retailer’s stock is detected when it is low, and automatically the restocking process places at the nearest distribution center. It allows lead time to be optimized as well as stock-outs (subsequent possible missed sales) to be avoided.
Further cases of IoT derive from the spreading of smart device and home products: flexible delivery address, monetization, and optimization of return trips. Currently, receivers are asked to provide the delivery address, but parcels equipped with IoT-enabled solutions can provide real time info and allow to know when delivery is approaching or change of address is required because, for example, at work and not at home. On the other hand, innovative start-ups such as Shyp (DHL, 2015) are proposing new shipping solutions, with the opportunity to add further services through tagging. In fact, the single products can be monitored through near-field communication (NFC) smart label that incorporates sensors to monitor temperature and humidity (e.g., radio frequency identification—RFID—or other sensory tags). If such a detailed piece of information becomes available for each parcel and for each product, enterprises could obtain more transparency on the contents of parcels and receivers’ acceptance (e.g., possibility to know if the temperature was maintained within the suggested thresholds or if the contents are particularly fragile). Of course, the complexity of delivering raises; however, the enterprises have the opportunity to increase the services offered. Not as the least, the diffusion of traffic sensors and vehicle detection sensors allows to update real time traffic conditions and to produce information for users.
Block Chain
The e-ICT for the exchange of values is referred to block chain. Many times, the value refers to sensible data, i.e., both personal or/and commercial ones. Block chain technology has been viewed as a disruptive technology for its strong impacts on different economic fields, such as healthcare, manufacturing, logistics, transport, and data management (DHL, 2018a; Tan et al., 2020). It can be defined as a chain of blocks, in which each block contains value data that are shared and validated. It belongs to the larger class of distributed ledger and can be also recalled Internet of Value. Several projects are implementing block chain for improving transparency as well as for monitoring supply chains. For example, it allows to manage data on how goods are made, where they come from, and how they are managed. The data become permanent and easily shared, giving supply chain providers more comprehensive track-and-trace capabilities. Companies can use this information to provide proof of legitimacy or authenticity, and consumers can benefit knowing whether a product has been ethically sourced, is an original item, and it was preserved in the correct conditions. In addition, very relevant is the contribution on contracts. Smart contracts in block chain are an important function that is designed to automatically facilitate, verify, and enforce the negotiation. Therefore, the implementation of digital contracts without central authorities can be performed. Furthermore, block chain is adopted to support the implementation of the IoT (Buccafurri et al., 2017). In fact, although the IoT can connect smart devices to collect data for real-time decision-making, IoT technology is being impeded by some issues, such as data privacy and security issues (Panarello et al., 2018; Lax et al., 2021). A direct connection between buyers and sellers eliminates many intermediaries like brokers involved in these processes. Through block chain, this is possible because there are no more trust and reputation problems.
Big Data
It is difficult to find a shared definition of big data or a predefined size from which it can refer as big data. In general, it is possible to speak on big data when the data set is so large and complex that it requires the definition of new tools and methodologies to extrapolate, manage, and process information within a reasonable time. The set of data and the new tools are defined big data (Zhu et al., 2019). A different specification, properly, BD refer to a big set of data, and data mining refers to the engine for searching finalized data (e.g. by specific queries). It can be considered that BD is “what” and data mining is “how.” With the integration of tools for subsequent data extrapolation, the repository is defined data warehouse. In this study, BD includes all the aforementioned elements.
Precise data of the movements of freight vehicles and goods using new technologies (e.g., vehicles sensors) can be collected in a very large amount. For example, GPS (global position systems) historical data can be saved creating a very large data set, tendentially big (Antoniou et al., 2018; Comi and Polimeni, 2021; Nigro et al., 2022). Mehmood et al. (2017) demonstrated how big data can be used to improve transport efficiency and lower externalities. One of the important points of big data applications in city logistics is the collaborative operation of urban freight transport in sharing the capacity of vehicles, distribution centers and information systems in the sharing economy. However, the capacity of data is usually owned by private companies which miss to share for privacy and competitiveness matters. But their integration, at least, with the public ones (e.g., traffic and infrastructure data) should be favored for enhancing the operations of urban freight transport. Cleophas et al. (2019) discussed collaborative urban freight systems, identifying two key types of collaboration, vertical and horizontal. Comi et al. (2020) pointed out the contributions offered by these big data in supporting urban freight transport planning.
Artificial Intelligence
The expression artificial intelligence synthetically indicates the algorithms, which analyze a set of (normal or big) data, take (or address) a decision. For example, the algorithms can support, in the choice of path for going from warehouse to customers, the definition of possible/feasible paths among which to choose the best ones or to identify the set of first best ones as well as the worst ones to avoid driving. In the literature, a large and diversified classification of AI exists. It can be recalled the one that classifies the decisional method on the basis of the previous information deriving from a single field of observation reproducing logical decision or the other one that, on the basis of information coming from different fields, produces divergent decision. Advancing the current definition, the former can be considered “machine learning” while the latter as “deep learning” (Veres and Moussa, 2020).
Online shopping experiences have much AI in the background (DHL, 2018b; Abduljabbar et al., 2020; Bakach et al., 2022). In an increasingly complex and competitive business world, companies that operate global supply chains are under unprecedented pressure to deliver higher service levels at flat or even lower costs. At the same time, internal functions of global corporations such as accounting, finance, human resources, legal, and information technology are plagued by large amounts of detail-oriented, repetitive tasks. Here, AI presents a significant opportunity to save time, reduce costs, and increase productivity and accuracy with cognitive automation. In addition, AI can assist address management for operators, ensuring successful delivery, or in predicting spikes in demand for specific goods.
Freight Transport Management Systems
Shared Formulation
In this section, the freight transport management systems are analyzed. The main objective is to identify all components of urban freight transport, seeking to link user needs and features of the tools. The issue of modification of knowledge for the user is considered, analyzing the learning process for the generic user (belonging to the enterprise) with the new information that can arrive from his/her experience, from previous and actual information given by public administration. Then, path choice, vehicle routing, scheduling, and courier routes are investigated. For each problem, the known formulation is recalled (problem definition), pointing out the elements that can be modified by the emerging technologies (the role of emerging technologies).
It should be noted that the utility/disutility of each class of actors can evolve through a within-day or day-to-day dynamics according to the use of different knowledge of the attributes, which in turn depend on the used technologies. In the following, the learning processes as modified by the new technologies are pointed out, and the main classical problems are updated at the light of the emerging technologies earlier recalled.
In the following part of the article, referring to the shared notation used in the literature, the path is defined as a sequence of links between two nodes relevant for freight management (i.e., origin node and destination node), while a tour/trip chain is a sequence of more than one path using the same mode (e.g., road or walking) with last destination coinciding with the first origin. If different modes are used within a tour, the route is born. In addition, for sake of notations, the ownership of the technology will be recalled only in Section 4, when all the involved stages are discussed.
Problem Definition
As said, the focus as follows is on vehicle paths, vehicle routing and scheduling, and on courier routes. Subsequently, the models developed for providing three levels of integration are analyzed:
• proactive and tailored-user path advices (smart routing–navigation systems),
• support in defining tour/trip chain for sustainable urban delivery (computerized vehicle routing and scheduling),
• support to couriers in defining routes within inner areas.
Therefore, the main innovation consists on the learning process of path costs enhanced by e-ICTs which allows to integrate it within the literature formulations, where
The aforementioned modeling tasks are solved in the literature using the deterministic or stochastic models (Daganzo, 1991; Ghiani et al., 2004). However, they do not consider explicitly the evolution and perception (i.e., learning process) of travel costs that can be obtained by experimenting with different network configurations. Thus, there is a need to consider explicitly the learning process of path costs. Therefore, this section deals with the problem of using the e-ICTs within the path choices, traveling salesman problem (TSP), and couriers’ routing, which allows more knowledge on the real past and current evolutions of the network to be acquired. In fact, e-ICTs can modify various elements of the real system, with the information that derives from them, and this study deals with the modifications that must be included in the simulation models for considering the presence of the different e-ICTs in the real system.
Within the framework defined by each of the recalled research tasks (path, traveling salesman problem, and couriers’ route), the e-ICTs intervene in the knowledge of the generalized travel cost of the network link which, for this intervention, modifies the nature, evolving from static to dynamic. The modifications are many in relation to the multiplicity of devices (e.g., minute by minute, hour by hour, and day by day) of the various types of e-ICTs available on the market. The various e-ICTs are then recalled in the aggregate form in relation to the functions in which they intervene. Therefore, the modifications concern the transformation of the generalized travel cost from static to dynamic.
In the dynamic area, the main changes concern
• within-day changes that are achieved with the use of IoT;
• day-to-day changes that are obtained with the use of BD.
Role of Emerging Technologies
Given the geographical area, containing the transport system where the vehicle drives, it may be expressed by means of a graph G = (N, L), where N is the set of nodes and L the set of pairs of nodes belonging to N, called links. Then, a transport network is obtained assigning to each graph link (or one or more nodes) a quantity expressed by a numerical value, which in the case of transport network, is represented by generalized transport cost (disutility or “utility”).
Let cij be the generalized travel link cost on the link identified by the ordered node pair (i, j) and c the link cost vector of dimension (nL × 1).
Let C be the vector of path costs for users of O-D pair od, consisting of elements Ck, k ∈ Kod (path choice set on O-D pair od), while X be the vector of path attributes for users of O-D pair od, consisting of elements Xk (e.g., travel time on minimum path k on O-D pair od, monetary cost on minimum path k on O-D pair od), and k ∈ Kod (path choice set on O-D pair od).
Path costs on time τ of day t, C[τ, t] can be expressed as a function of path attributes depending on time τ of day t:
where X[τ, t] indicates path cost attributes relative to all paths k on time τ of day t. Such attributes can be estimated by users according to a learning process. The formulation can be extended to freight vehicles choice just for the possibilities given by the emerging technologies, leaving from the private/transit models (Cantarella, 2013; Cascetta, 2013).
Users can estimate such attributes according to a learning process synthesized in Figure 1 (Russo and Comi, 2021). Generally, learning points out both the evolution of τ and the evolution of t (Cascetta, 2013). In addition, for some attributes, the value experienced (tested) in previous periods X[t-1], X[t-2] and for other attributes, the value updated by the user at each time τ in day t, can be considered through the availability of real-time information.
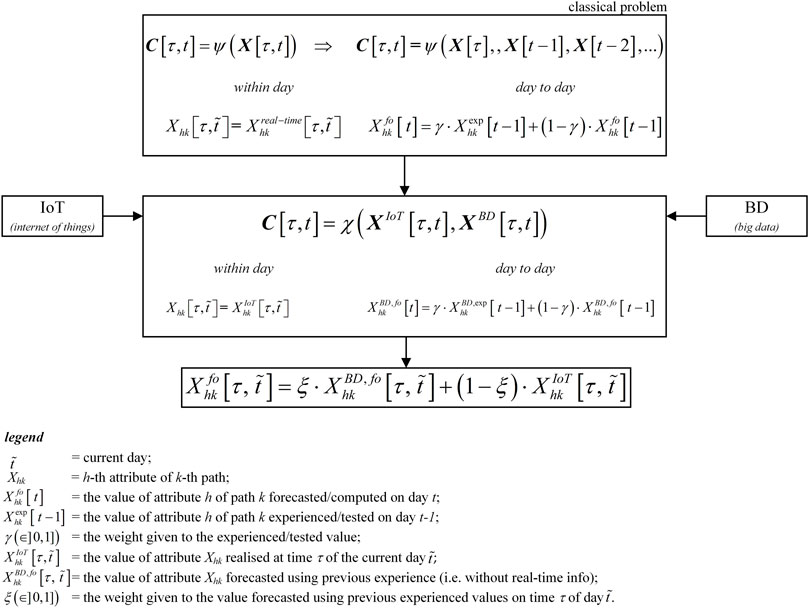
FIGURE 1. Learning process of path attributes enhanced by emerging technologies, IoT and BD (Russo and Comi, 2021).
Pointing out the role of emerging technologies (i.e., IoT for obtaining real-time configuration of the network–intra-period evolution, and BD for highlighting the inter-period evolution) in forecasting path attributes, Eq. 3.1 can be updated as follows:
where
•
•
•
Navigation Systems
In this subsection, a definition of the problem and the innovation coming from the use of e-ICTs are pointed out. Therefore, the modeling advancement is formalized.
Problem Definition
Navigation systems were developed to provide specific route guidance exploiting the information about traffic regulations (e.g., road works and lane directions). Navigation systems are able to determine the best paths among destinations (customers to serve) according to the average configuration of the road network (e.g., average link travel time according to time of days (DfT, 2010; De Moraes Ramos et al., 2020; Kessler et al., 2021).
The aforementioned tools exploit the potentiality offered by minimum path algorithm on a network. In fact, given a road network, the most used algorithms for the search of the minimum cost paths (or of the ordered list of the shortest paths) among all the pairs of nodes of the network allow us to calculate the minimum cost trees (or the ordered cost trees) rooted in each origin (or destination). From these, it is possible to derive the minimum path (or the ordered list of shortest paths) that connects each destination node to the root node. In the following, the subgraph “tree of least cost with root the node o” is indicated with T*(o) and any other tree with the same root with T(o). For more details on ordered cost tree, refer to Russo and Vitetta (2006).
All the algorithms for the computation of T*(o) are iterative type, i.e., at each iteration there is a tree T(o) which is modified until it “coincides” with T*(o). The theoretical basis of these algorithms is Bellmann’s theorem; it demonstrates that called Co,i, and Co,j the costs necessary to reach the nodes i and j from the root or with the only path allowed by a generic root tree o, a necessary and sufficient condition for a tree T(o) to be the least cost tree, i.e., T(o) ≡ T*(o), is that for each pair of nodes i, j connected by a link belonging to the examined graph; the triangular condition occurs as follows:
The shortest path problem has been intensively investigated over years due to its extensive applications in graph theory, computer network, and design of transportation systems. The classic Dijkstra’s algorithm (Dijkstra, 1959) was designed to solve the single-source shortest path problem for a static graph. Then, guided by the needs to find the shortest path in the stochastic and congested network, several advancements were performed (Zhan and Noon, 1998; Cascetta et al., 2002; Zhang et al., 2010; Bast et al., 2015).
The real network of a city can be better simulated by means of a stochastic network, i.e., the actual link travel times may differ from historical or forecasted one. Therefore, in a stochastic network, an optimal path from node i (origin) to node j (destination), found at the origin (a priori routing) on the basis of historical or forecasted times and suggested to a user, may include a link that could prove to be non-optimal when the user arrives at those links caused, as earlier introduced, by within-day and day-to-day dynamics.
Because of stochasticity, the outcome of any decision depends partly on the user’s decisions and partly on randomness. Therefore, currently, the new and more advanced navigation systems provide not a complete single origin–destination (O-D) path, but they suggest rather one optimal travel strategy based on the learning process of the user augmented by the emerging technologies at disposal, adopting the optimal strategy as suggested for transit services (Spiess and Florian, 1989; Nuzzolo and Comi, 2018).
For example, referring to a simple network, where the user is asked to go from point A (i.e., depot/warehouse) to B (i.e., customer 1), different paths can be available and some changes could occur according to the within-day or day-to-day dynamic. Then, the use of the past stored information on the network working can suggest the best path, allowing time savings and operational cost reduction, and on the other hand to reduce the vehicle-kilometers with benefit for city sustainability and liveability.
Role of Emerging Technologies
The complete specification of the systemic costs, by which users consider their choice at time τ of period/day t, requires explicit treatment of the learning mechanism of path cost (disutility or “utility”) attributes (X) or, in other words, how experience and information about attributes of path costs on previous days influence the forecast of the current ones (Nuzzolo et al., 2001, 2003).
Therefore, actualizing Eq. 3.2 for path advices (Russo and Comi, 2021), it should be noted that the learning occurs both with the evolution of τ and the evolution of t. In addition, it can be considered, for some attributes, the value experienced (tested) in previous periods X[t-1], X[t-2] and for other attributes updating which users perform for each time τ in day t. Therefore, as summarized in Figure 1, by means of algorithms that support decision (AI) which allow past (BD) and real-time info (IoT) to be merged, the value of attribute Xhk at time τ of the current day
where
•
with
o
o
o
•
•
Advanced Navigation Systems
The new opportunity offered by research on optimal path finder can be applied to better use the new technologies, specifically in the most advanced situation: the IoT updates current information, BD provide day-to-day advices, and AI allows decision on better choice based on attribute evolution and forecasting to be taken. The new challenge is the generation of a set of paths more than the shortest one, i.e., not only the first best, but also the second best, …, the g best until to obtain a set of specific paths (Russo and Vitetta, 2006). This class of models has been, at today, used only to define the path choice set for the user in a static approach. User’s choice can be updated modifying the path costs as described below. For example, when a path k is chosen, and during the travel, the user is informed that another path can become more attractive both for events which are endogenous to traffic and for events that are exogenous (e.g., along the path k there is a road accident or a specific event and the traffic is delayed), the user can reconsider in an intelligent en route way to continue on path k if the cost of path k is given by
which is less than the cost of each path k’ belonging to his/her choice set; K[τ, t], which is obtained by new information, is given by
that is
As said, the value of Ck [τ, t] depends, in general, on IoT and BD:
where
Therefore, the costs of paths evolve during time τ of the current day
Computerized Vehicle Routing and Scheduling
Problem Definition
Freight distribution concerns the pickup and delivery of freight using a fleet of trucks. As a rule, vehicles are based on a single depot (warehouse), and the vehicle tours are performed in a single work shift and may include several pickup and delivery points. The optimization process of assigning customers (pickup and delivery points) to trucks and determining the visiting order of customers and routes refer to vehicle routing and scheduling problems (Taniguchi et al., 2001; Ghiani et al., 2003; Russo et al., 2010; Thompson and Zhang, 2018; Danchuk et al., 2019). The basic information needed for the vehicle routing problem (VRP) is the location of customers, road network conditions, travel times, traffic regulations, etc. In addition to this basic information, other specific information for each customer (including the daily request for carrying out goods, the designed time windows, the identified driver) is given to identify the optimal visiting order and the route for each vehicle.
Travelling salesman problems (TSPs) are the basic problem for VRP, which can be described as follows (Lawyer et al., 1992; Musolino et al., 2016; Cattaruzza et al., 2017). There are n customers and the cost Cij to travel from customer i to customer j is given. A salesman (vehicle) starts from the depot (warehouse) to visit each customer exactly once and return to the depot. The problem is to find the optimal route (visiting order of customers) that has the minimum total travel cost. TSP is hence formulated as follows:
subject to
•
•
•
•
The problem formulated has different variants. In its basic version, it has been shown to be NP hard. Removing constraints 3.13, it can be obtained a good lower-bound to start the optimal solution.
Starting from the presented formulation, the main variants are descriptively (given that it is out of the main scope of the study) recalled, sending to the literature for the formulation of specific constraints that must be added to the equations before reported (Ghiani et al., 2004; Farahani et al., 2011):
• the symmetric travelling salesman problem, where each edge (road link) has the same cost in the two directions.
• the node routing problem with capacity and length constraints (DC-VRP), then, considering a capacity dimension for the vehicle and a maximum operating time (e.g., electric vehicles).
• the node routing and scheduling problem with time windows (VRP-TW), it happens when one or more customers need to be served in specific temporal windows.
• the edge routing problems, commonly known as the Chinese postman problem, where it needs to travel along all edges and all nodes of a defined list.
• the multiple vehicles for routing problem, where the formulation (3.10) and (3.13) can be evolved to include the number of identical vehicles at disposal.
• the vehicle routing problem with reverse for backhaul (VRP-B), when some customers require to pick up parcels to backhaul to the depot.
• the vehicle routing problem with pickup and delivery (VRP-PD), as in the previous one, but each customer can ask for delivery and pickups in the same time.
Other variants of VRP exist with similar basic formulation and heuristic solutions (Ghiani et al., 2004; Farahani et al., 2011; Kim et al., 2015).
Role of Emerging Technologies
The emerging technologies modify the considered approach both for daily dynamic and weekly planning. In the planning, there is the possibility to use BD in the off-line analytics and decision-making, regarding the main variables to be forecasted in a given temporal period. In the daily dynamics, the use of IoT becomes crucial to update the model, again following the learning process of the generalized path costs introduced in previous section (Eq. 3.2):
As introduced earlier, some attributes can depend on the values experimented (tested) in the previous days, which can be computed as Eq. 3.4 describes. Therefore, merging past (BD) and real-time information (IoT), the value of attribute Xhk at time τ of the current day
Then, the model of Eq. 3.9 can be updated. Also on this case, the support of AI can optimize the results, giving the better solution for each time of each day.
Figure 2 briefly plots the process of support provided by such a system. In the example, a user has to serve five customers (destinations) and different tours (sequence of customers to be served) are available with different costs:
• T1: Origin – Destination 1 – Destination 2 – Destination 3 – Destination 4 – Destination 5 – Depot;
• T2: Origin – Destination 1 – Destination 4 – Destination 3 – Destination 2 – Destination 5 – Depot;
• T3: Origin – Destination 1 – Destination 2 – Destination 5 – Destination 4 – Destination 3 – Depot;
• T4: Origin – Destination 5 – Destination 4 – Destination 3 – Destination 2 – Destination 1 – Depot.
The routing system allows user to select the best tour (i.e., T3) for performing delivery service taking into account all information that can be obtained from the network (e.g., travel time forecasts) and can benefit from the integration with navigation systems (see the earlier section). On the other hand, if each customer also provides some time constraints, as well as time spent for serving at destination, the routing and scheduling system allows such needs (included load factor), as represented in Figure 3, to be considered.
For each customer (destination), different times (BD) could be required for serving (vertical continuous line in Figure 3) as well as time slice availability (vertical dotted line in Figure 3) could be differently distributed during the working day. In this respect, the system can help user in defining the optimal sequence of customer to serve providing time savings as well as cost reduction. In the reported example (Figure 3), a possible solution is to serve as the first customer 3, then customer 5, customer 1, customer 2, and finally customer 4.
Advanced Vehicle Routing and Scheduling
Vehicle routing and scheduling have attracted considerable attention (see, for example, Eksioglu, 2009; Erdoĝan and Miller-Hooks, 2012; Dullaerta and Zamparini, 2013; Musolino et al., 2016; Kim et al., 2016; Cattaruzza et al., 2017; and references quoted therein), but only recently the research moved forward to include in the problem definition the possibility of allowing info on real-time network status (Sánchez-Díaz et al., 2015). Such models are usually described as the rich vehicle routing problem (RVRP; Hoff et al., 2010). Recent studies often involve routing whereby real-time data are included. In these cases, vehicle routes are built in an on-going fashion as vehicle locations, travel times and customer requests are revealed over the planning horizon (Ghiani et al., 2003; Ruand et al., 2012). Even if RVRP and the real-time vehicle routing problem (RTVRP) are better at describing real distribution processes, they need to consider many constraints and objectives, and often cannot be optimally solved or are NP-hard. Thus, feasible solutions often need to be found for real world application. Recalling the formulation given by Eq. 3.9, following Ghiani et al. (2004), the real-time vehicle routing and dispatching can be formulated and solved with stand-alone or parallel hardware. This process is performed by computerized vehicle routing and scheduling (CVRS) systems (DfT, 2007). CVRS are thus used to generate and optimize routes and schedules for transport operations and journeys (AI). In general, these tools allow users to hold information concerning a digital map of the road network integrated with further info, such as
• customer locations, delivery, and collection windows,
• quantities and types of goods to be delivered or collected,
• vehicle availability and capacities, and
• driver shift patterns.
Customer orders and time constraints are input into the system, which then generates the optimum set of routes that meets the delivery need. The subsequent advancement is linked to the subsequent insertion of IoT for real-time data providing, of BD for data saving and analysis, of AI for taking decisions.
Systems can be used for dynamic daily or weekly planning, as well as for strategic analysis. The powerful capabilities of the model include the ability to
• reduce operational costs by minimizing resource requirements, i.e., the number of vehicles and drivers for a given workload;
• determine the most appropriate depot from which to schedule specific calls/services;
• observe all customer access constraints e.g. closed days, vehicle size and type restrictions, delivery time windows, and pre-booked times;
• reduce loaded and empty mileage;
• accommodate collections and deliveries en route without exceeding payload tonnes, available load space or drivers’ hours limits.
Courier Route Problem
Problem Definition
Couriers, often, when delivering goods to customers in central business districts (CBDs), have difficulty in finding suitable locations for delivering goods. Searching for available on-street delivery bays can cause a significant amount of additional time as well as can increase traffic impacts (e.g., congestion and pollutant emission). The e-ICTs can help to book delivery bays. Therefore, the new challenges for couriers is to have tools that allow them to identify the best delivery bay (in the inner areas) which minimizes the delivery route (generalized travel costs), taking into consideration that couriers often serve multiple customers (e.g. retailers and end consumers–home deliveries) from the same delivery bays. Thus, optimizing CBD distribution routes needs to incorporate both vehicle/truck and walking tours (Thompson and Zhang, 2018).
Following Thompson and Zhang (2018), problem can be formulated and solved as a multi-objective two-layer programming optimization model. In the upper layer, the choice and order of delivery zones to be used are produced, and at the lower layer, the pedestrian paths are generated. The objective function consists of the distances traveled by the vehicle and walking combined with their relative weights. Constraints relating to maximum parking durations are also incorporated. To solve the model, a genetic algorithm—based search method or other heuristics can be used.
For exemplificative scope, without losing of generality, the problem to minimize sum of driving and walking time (cost) can be formulated according to Thompson and Zhang (2018) and foreseen its evolution in order to introduce constraints on parcels, where the first part of equation recalls the previously introduced VRP problem:
subject to
•
•
•
•
•
•
•
•
•
•
•
•
where
• α is the weight parameter of walking with respect to driving;
• B is the set of delivery bays (b), while S is the set of customer s;
• E is the set of entrance/exit point e of the CBD;
• Cij is the travel cost from i to j points (i.e., delivery bays or customer or entrance/exit);
• D and W are the driving route as well as the walking route;
• xij and z′ij are binary variables equal to 1 if path from i to j is part of D or W, respectively, 0 otherwise.
Role of Emerging Technologies
The e-ICTs modify the considered approach both for daily dynamics and weekly planning. In the planning, as happens in CVRS, BD offers opportunity to forecast the path costs and to identify preferred delivery bays and subsequently the best path to reach customers from the chosen delivery bay. In daily dynamics, IoT plays a key role in updating the model as specified through Eq. 3.4. Furthermore, the BC could allow us to manage exchanges of values. Therefore Eq. 3.14 can be computed updating the path costs, according to Eq. 3.2, where the path attributes are forecasted through Eq. 3.4 (Figure 1).
Advanced Courier Routing
The model formulated at Eq. 3.14 can benefit from the e-ICTs offering the enterprise the opportunity to identify the set of preferred delivery zones (IoT provides info on their availability), as well as to estimate route costs (disutility/utility; BD), and to identify the optimal driving and walking route to the customers using estimates and forecasts of the route costs. Therefore, the e-ICTs becomes crucial for designing advanced guidance advisors for couriers integrated with delivery bay systems (Comi et al., 2018a; Comi et al., 2018b). In addition, the optimization problem can be extended incorporating a further stage for locating delivery bays within CBD to reduce the operations costs by couriers according to the demand forecasted through info obtained from BD. Such a formulation can benefit from the research results coming from two or multiple echelon problem (Lan et al., 2020).
Discussion and CONCLUSIONS
To gain insight into the opportunities offered by emerging ICT, or advanced ITS, and its impact on generalized path costs analyzed in the earlier sections, a synthesis is proposed in Table 1.
As discussed, the navigation systems can benefit from GPS (global positioning systems) and other telecommunication technologies which allow us to obtain location data of freight vehicles and goods at every instant. In addition, the sensors or other mobile devices (IoT) available on the infrastructure (IoTPA, i.e., owned by public administration) allow data on traffic to be collected (big data, BDPA). If dynamic reorganization of the traffic lights is operative then the machine learning techniques (AITLO, i.e., owned by enterprise) can be useful to foresee the network configuration. Then, path searching formulation (Section 3.1) can be modified including the opportunity to consider time and then to provide more effective suggestions on path to follow for reaching destination. In this way, a considerable time saving can be obtained with a subsequent cost decrease.
Routing and scheduling (TSP) can benefit from the aforementioned recalled technologies, i.e., IoT for daily decision making and BD for weekly decision making. As carried out by WalMart for food delivering, smart devices (IoTTLO) can be securely tied to or embedded in the physical product to autonomously record and transmit data about item condition including temperature variation, to ensure product integrity, as well as any evidence of product damage (BCTLO). Of course, big data (BDPA and BDTLO) on the transport network and on the service provided allow operators to identify eventually critical stages in their services with classical methods or through advanced machine learning techniques and to optimize their services (AITLO). Then, the costs for moving from costumer i to j (Eq. 3.1) can be significantly reduced, owing to these technologies.
Using BC, transport and logistics operators can share data on their business with PA (BCPA). In addition, block chain allows to manage the contracts and to monitor the service provided in terms of delivery time, and the goods have been preserved in the correct conditions (BCTLO). In the classical way, BC gives the possibility to make a transaction in the value between the retailer (BCRE) and enterprise (BCTLO), as well as between the retailer (BCRE) and end consumer (BCEC). Similarly, this happens for the in-cab communication system which thanks BC company planners and drivers could exchange information (BCTLO), as well as sensors (IoTTLO) to have permanent control on the vehicle status as well as to assist drivers in decision making (AITLO).
Courier routing problem exploiting the emerging technologies allows optimizing the costs supported for driving as well as for walking efficiently using the data (BDPA and BDTLO) acquired by sensors (IoTPA and IoTTLO) choosing the best generalized path (AITLO).
Concluding, it can be summarized how this study focuses on the opportunity offered by e-ICTs for improving city logistics as well as on the identification of their application field in relation to city logistics. Therefore, the different city logistics groups of actors and their interactions were pointed out in relation to internet of things, block chain, big data and artificial intelligence, considering explicitly who is the owner of each emerging technologies.
In particular, the evolution in relation to the impacts of these emerging technologies on generalized path costs have been pointed out showing how the current structure of costs can be in depth modified by the technology. Then, the learning process due to the updating for within-day and day-to-day dynamics were formalized. At an advanced level, the opportunity to design a multilevel delivery path (tour) providing nodes (points) where walking for reaching the final customers (e.g., retailers or end consumers’ homes) were discussed.
It has been pointed out that the classical algorithms interest the freight operators, the collaboration with the public administration evolves the information on specific attributes defining the advanced model. It is the public administration, owner of the intelligent objects, that can organize a IoTPA with operators in collecting the data and can load with a BCPA process. As said before, if there are modifications in the network in terms of daily traffic lights or delivery bay windows, AITLO can reorganize decisions. The presence of change on values can else be considered if the stakeholders are only public and private operators. The presence of transaction on values (BCTLO, BCRE, BCEC) becomes important, if in the process, participate the retailers. Finally, if the end consumers are directly involved all the new technologies are involved.
Novelty of the study mainly consists of the analysis of each emerging ICT regarding city logistics and of the formalization, in the transport system models of the new knowledge for each group of actors. Based on a set of concrete evolution to implement, a broad set of advancements of current ITSs was focused, and a global view on dynamic freight management in city logistics was given. The impacts of new technologies on city logistics and the relevant knowledge of ITS were, thus, presented. In particular, the framework of conducting evolution in current ITS was discussed showing that the new technologies could have profound impacts on the design of advanced intelligent transportation system, and make it safer, more efficient, and profitable. However, the presented research does not point out the modifications on user’s choice mechanism and how updating/learning of users’ utility can be included in such a modeling task. Then, the further opened research questions are related to revise the choice models also looking at sequential choice formulation (Manski and Sherman, 1980), updating model of the choice.
From the analysis of the opportunities offered by emerging technologies used in city logistics for improving the utility and the subsequent modifications caused in the structure of path costs, the new challenges emerge, i.e., the changes that could occur in the generalized loads through the implementations of new freight nodes where transshipment can be performed in terms of modification of vehicles and/or quantities. In addition, the active role of public administrations has become so relevant due to the opportunity to plan and govern the freight network with its loads. Therefore, the advancement of this study will be mainly addressed to investigate the generalized load similarly as performed for the generalized path, as well as to explore how the administrations can govern the evolution of city logistics where the new technologies can play a key role to design a multilevel supply network in terms of single and multi-echelon allowing load and vehicles to be adapted to the current area requirements (e.g., small vehicles, trolley).
From the synoptic overview, it emerges the role of the shared emerging technologies. The further scientific works and the technical advancement must address the possibility to implement a unified platform to share some of technologies looking at building the logistics community system–LCS. A further advancement regards the possibility to organize an integrated software and hardware system, digital twin (Marcucci et al., 2020), where the methods for planning and those for assessing the general product as a service business models will be tested for city logistics. The digital twin will be the virtual twin of the real LCS. The first level of real and virtual environments can be carried out by sharing IoT among EC, RE, TLO and PA. Then, a further level could allow data between customer (i.e., EC and RE) and TLO to be shared (retailer or end consumer, BCR and BCEC; i.e., owned by retailer or end consumer, respectively). Thus, the supplied service can be promptly adapted and operated. BD can evolve saving the historical IoT and again each actor can use her AI to operate. BC could support the exchanges in value and the monitoring/control of parcel features. In addition, how happened in ports with the introduction of port community system (PCS), similarly city logistics could be impacted by an inter-organizational system (logistics community system–LCS) used for enabling commercial services and providing information exchange between the transport and logistics operators (enterprises)/retailers and their customers, and/or a variety of actors, such as end consumers, local administrations, among the others. The concepts encompass joint delivery systems with shared use of pickup and delivery trucks, urban consolidation centers, and transhipment points based on big data analytics. These systems could involve public-private partnerships among shippers, freight carriers, UCC (urban consolidation center) operators, municipalities, and regional planning organizations since the location of UCC and TP (transhipment points) are highly related to urban land use plans and infrastructure provision as well as urban traffic management. ICT based communication systems for actors could thus also become essential for the efficient management of integrated platforms for both real-time and long-term operations. Integrated platforms could provide benefits such as improving the efficiency of urban deliveries increasing safety and reducing CO2 footprints. Therefore, each of the recalled actors has their own specific objectives and tend to behave in a different manner. LCSs need to recognize these factors and the subsequent relationships. For example, freight transport and logistics operators are enterprises which use urban spaces on the time and then interact with the public administration which manages and governs these spaces. Therefore, telematics systems such as freight transport management systems (e.g., fleet management systems and tracking and tracing systems) or (freight) traffic control and info systems (e.g., access control systems, traffic and information systems) or city logistics support for management and planning can help them optimize their costs and to maximize their level of service. Therefore, the road ahead could meet, more in depth, the needs of the different actors/stakeholders and to favor the transit toward more sustainable, liveable, and smart cities, as promoted by the EU directives.
Data Availability Statement
The original contributions presented in the study are included in the article, further inquiries can be directed to the corresponding author.
Author Contributions
AC and FR wrote sections of the manuscript. All authors contributed to manuscript revision, read, and approved the submitted version.
Funding
The waiver will be charged by editorial team after submission.
Conflict of Interest
The authors declare that the research was conducted in the absence of any commercial or financial relationships that could be construed as a potential conflict of interest.
Publisher’s Note
All claims expressed in this article are solely those of the authors and do not necessarily represent those of their affiliated organizations, or those of the publisher, the editors, and the reviewers. Any product that may be evaluated in this article, or claim that may be made by its manufacturer, is not guaranteed or endorsed by the publisher.
Acknowledgments
Authors wish to thank the editor and reviewers for their contribution, which were considerably useful in improving the study.
References
Abduljabbar, R., Dia, H., Liyanage, S., and Bagloee, S. A. (2020). Applications of Artificial Intelligence in Transport: An Overview. Sustainability 11, 189. doi:10.3390/su11010189
Ang, K. L.-M., Seng, J. K. P., Ngharamike, E., and Ijemaru, G. K. (2022). Emerging Technologies for Smart Cities’ Transportation: Geo-Information, Data Analytics and Machine Learning Approaches. ISPRS Int. J. Geo-Inf. 11 (2), 85. doi:10.3390/ijgi11020085
Antoniou, C., Dimitriou, L., and Pereira, F. (2018). Mobility Patterns, Big Data and Transport Analytics: Tools and Applications for Modeling. Amsterdam, Netherlands: Elsevier, 1–432.
Atzori, L., Iera, A., and Morabito, G. (2010). The Internet of Things: A Survey. Comput. Netw. 54, 2787–2805. doi:10.1016/j.comnet.2010.05.010
Bakach, I., Campbell, A. M., and Ehmke, J. F. (2022). Robot-Based Last-Mile Deliveries with Pedestrian Zones. Front. Future Transp. 2. doi:10.3389/ffutr.2021.773240
Banco Mundial (2008). Doing Business en Colombia, Government document – report. NW Washington, D.C.: The International Bank for Reconstruction and Development/The World Bank.
Bast, H., Delling, D., Goldberg, A., Müller-Hannemann, M., Pajor, T., Sanders, P., et al. (2015). Route Planning in Transportation Networks. Available at: https://arxiv.org/pdf/1504.05140.pdf (February 2022).
Baum, L., Assmann, T., and Strubelt, H. (2019). State of the Art - Automated Micro-vehicles for Urban Logistics. IFAC-PapersOnLine 52 (13), 2455–2462. doi:10.1016/j.ifacol.2019.11.575
Büyüközkan, G., and Ilıcak, Ö. (2022). Smart Urban Logistics: Literature Review and Future Directions. Socio-Econ. Plan. Sci. 81, 101197. doi:10.1016/j.seps.2021.101197
Buccafurri, F., Lax, G., Nicolazzo, S., and Nocera, A. (2017). Overcoming Limits of Blockchain for IoT Applications. ACM Int. Conf. Proc. Ser. Part F130521, a26. doi:10.1145/3098954.3098983
Calabrò, T., Iiritano, G., and Trecozzi, M. R. (2019). Activities Training on City Logistics: Case of Calabria Region. WIT Trans. Ecol. Environ. 238. doi:10.2495/SC190151
Campisi, T., Russo, A., Tesoriere, G., Bouhouras, E., and Basbas, S. (2021). “COVID-19’s Effects over E-Commerce: A Preliminary Statistical Assessment for Some European Countries,” in Lecture Notes in Computer Science (Including Subseries Lecture Notes in Artificial Intelligence and Lecture Notes in Bioinformatics), 370–385. 12954 LNCS. doi:10.1007/978-3-030-86979-3_27
Cantarella, G. E. (2013). Day-to-day Dynamic Models for Intelligent Transportation Systems Design and Appraisal. Transp. Res. Part C Emerg. Technol. 29, 117–130. doi:10.1016/j.trc.2012.03.005
Cascetta, E., Russo, F., Viola, F., and Vitetta, A. (2002). A Model of Route Perception in Urban Road Networks. Transp. Res. Part B Methodol. 36 (7), 577–592. doi:10.1016/s0191-2615(00)00041-2
Cascetta, E. (2013). Transportation Systems Engineering: Theory and Methods, 49. Dordrecht: Springer Science & Business Media.
Cattaruzza, D., Absi, N., Feillet, D., and González-Feliu, J. (2017). Vehicle Routing Problems for City Logistics. EURO J. Transp. Logist., 6, 51–79. Springer. doi:10.1007/s13676-014-0074-0
Cirulis, A., and Ginters, E. (2013). Augmented Reality in Logistics. Proc. Comput. Sci. 26, 14–20. doi:10.1016/j.procs.2013.12.003
Cleophas, C., Cottrill, C., Jan Fabian Ehmke, J. F., and Tierney, K. (2019). Collaborative Urban Transportation: Recent Advances in Theory and Practice. Eur. J. Oper. Res. 273, 801–816. doi:10.1016/j.ejor.2018.04.037
Comi, A., and Polimeni, A. (2021). Forecasting Delivery Pattern through Floating Car Data: Empirical Evidence. Future Transp. 2021 (1), 707–719. doi:10.3390/futuretransp1030038
Comi, A., Buttarazzi, B., and Schiraldi, M. (2018a). Smart Urban Freight Transport: Tools for Planning and Optimising Delivery Operations. Simul. Model. Pract. Theory, 88, 48–61. Elsevier. doi:10.1016/j.simpat.2018.08.006
Comi, A., Buttarazzi, B., Schiraldi, M., Innarella, R., Varisco, M., and Traini, P. (2018b). An Advanced Planner for Urban Freight Delivering. Archives Transp. 48 (4), 27–40. doi:10.5604/01.3001.0012.8363
Comi, A., Nuzzolo, A., and Polimeni, A. (2020). Urban Freight Vehicle Flows: an Analysis of Freight Delivery Patterns through Floating Car Data. Transp. Res. Proc. 47, 409–416. doi:10.1016/j.trpro.2020.03.116
Dablanc, L., Morganti, E., Arvidsson, N., Woxenius, J., and Browne, M. (2017). The Rise of On-Demand ‘instant Deliveries’ in European Cities. Suppl. chain forum Int. J. 18, 203–217. Taylor & Francis. doi:10.1080/16258312.2017.1375375
Danchuk, V., Bakulich, O., and Svatko, V. (2019). Building Optimal Routes for Cargo Delivery in Megacities. Transp. Telecommun. J. 20 (Issue 2), 142–152. doi:10.2478/ttj-2019-0013
De Moraes Ramos, G., Mai, T., Daamen, W., Frejinger, E., and Hoogendoorn, S. P. (2020). Route Choice Behaviour and Travel Information in a Congested Network: Static and Dynamic Recursive Models. Transp. Res. Part C 114, 681–693. doi:10.1016/j.trc.2020.02.014
DfT (2007). Computerised Vehicle Routing and Scheduling (CVRS) for Efficient Logistics – Guide. United Kingdom: Department for Transport. Available at: www.freightbestpractice.org.uk.
DfT (2010). Planning and Managing Effective Customer Deliveries. United Kingdom: Department for Transport. Available at: www.freightbestpractice.org.uk.
DHL (2018a). Blockchain in Logistics - Perspectives on the Upcoming Impact of Blockchain Technology and Use Cases for the Logistics Industry. Troisdorf, Germany: DHL Trend Research.
Dijkstra, E. W. (1959). A Note Two Problems in Connection with Graphs. Numer. Math. 1, 269–271. doi:10.1007/bf01386390
Dullaerta, W., and Zamparini, L. (2013). The Impact of Lead Time Reliability in Freight Transport: A Logistics Assessment of Transport Economics Findings. Transp. Res. Part E Logist. Transp. Rev. Articl. 49 (1), 190–200. doi:10.1016/j.tre.2012.08.005
Eksioglu, B. V. (2009). The Vehicle Routing Problem: a Taxonomic Review. Comput. Indust. Eng. 57 (4), 1472–1483. doi:10.1016/j.cie.2009.05.009
Erdoĝan, S., and Miller-Hooks, E. (2012). A Green Vehicle Routing Problem. Transp. Res. Part E Logist. Transp. Rev. Articles 48 (1), 100–114. doi:10.1016/j.tre.2011.08.001
Farahani, R. Z., Rezapour, S., and Kardar, L. (2011). Logistics Operations and Management. London: Elsevier Insights.
Ghiani, G., Guerriero, F., Laporte, G., and Musmanno, R. (2003). Real-time Vehicle Routing: Solution Concepts, Algorithms and Parallel Computing Strategies. Eur. J. Oper. Res. 151 (1), 1–11. doi:10.1016/s0377-2217(02)00915-3
Ghiani, G., La Porte, G., and Musmanno, R. (2004). Introducing to Logistics Systems Planning and Control. Chichester: Wiley.
Gómez-Marín, C. G., Serna-Urán, C. A., Arango-Serna, M. D., and Comi, A. (2020). Microsimulation-based Collaboration Model for Urban Freight Transport. IEEE Access, 8 182853–182867. doi:10.1109/ACCESS.2020.3028564
Gonzalez-Feliu, J., and Peris-Pla, C. (2017). Impacts of Retailing Attractiveness on Freight and Shopping Trip Attraction Rates. Res. Transp. Bus. Manag. 24, 49–58. doi:10.1016/j.rtbm.2017.07.004
Haas, I., and Friedrich, B. (2017). Developing a Micro-simulation Tool for Autonomous Connected Vehicle Platoons Used in City Logistics. Transp. Res. Proc. 27, 1203–1210. doi:10.1016/j.trpro.2017.12.084
Hadavi, S., Verlinde, S., Verbeke, W., Macharis, C., and Guns, T. (2019). Monitoring Urban-Freight Transport Based on GPS Trajectories of Heavy-Goods Vehicles. IEEE Trans. Intell. Transp. Syst. 20 (10), 3747–3758. doi:10.1109/TITS.2018.2880949
He, Z., and Haasis, H. D. (2019). Integration of Urban Freight Innovations: Sustainable Inner-Urban Intermodal Transportation in the Retail/Postal Industry. Sustainability 11 (6), 1749. doi:10.3390/su11061749
Hoff, A. A., Andersson, H., Christiansen, M., Hasle, G., and Løkketangen, A. (2010). Industrial Aspects and Literature Survey: Fleet Composition and Routing. Comput. Operations Res. 37 (12), 2041–2061. doi:10.1016/j.cor.2010.03.015
Kessler, L., Rempe, F., and Bogenberger, K. (2021). Multi-Sensor Data Fusion for Accurate Traffic Speed and Travel Time Reconstruction. Front. Future Transp. 2, 766951. doi:10.3389/ffutr.2021.766951
Kim, G., Ong, Y., Heng, C. K., Tan, P. S., and Zhang, N. A. (2015). City Vehicle Routing Problem (City VRP): A Review. IEEE Trans. Intelligent Transp. Syst. 16 (4), 1654–1666. doi:10.1109/TITS.2015.2395536
Kim, G., Ong, Y. S., Cheong, T., and Tan, P. S. (2016). Solving the Dynamic Vehicle Routing Problem under Traffic Congestion. IEEE Trans. Intell. Transp. Syst. 17 (8), 2367–2380. doi:10.1109/TITS.2016.2521779
Kim, J., Moon, H., and Jung, H. (2020). Drone-based Parcel Delivery Using the Rooftops of City Buildings: Model and Solution. Appl. Sci. Switz. 10 (12), 4362. doi:10.3390/app10124362
Kin, B., Verlinde, S., Mommens, K., and Macharis, C. (2017). A Stakeholder-Based Methodology to Enhance the Success of Urban Freight Transport Measures in a Multi-Level Governance Context. Res. Transp. Econ. 65, 10–23. doi:10.1016/j.retrec.2017.08.003
Lan, Y., Liu, F., Huang, Z., Ng, W. W. Y., and Zhong, J. (2020). Two-Echelon Dispatching Problem with Mobile Satellites in City Logistics. IEEE Trans. Intelligent Transp. Syst 23 (1). doi:10.1109/TITS.2020.3003598
Lawyer, E. L., Lentra, A. H., Rinnooy, H. G., and Shmys, D. B. (1992). The Travelling Salesman Problem. Chichester: Wiley.
Lax, G., Russo, A., and Fascì, L. S. (2021). A Blockchain-Based Approach for Matching Desired and Real Privacy Settings of Social Network Users. Inf. Sci. 557, 220–235. doi:10.1016/j.ins.2021.01.004
Manski, C. F., and Sherman, L. (1980). An Empirical Analysis of Household Choice Among Motor Vehicles. Transp. Res. 14A, 349–366. doi:10.1016/0191-2607(80)90054-0
Marcucci, E., Gatta, V., Le Pira, M., Hansson, L., and Bråthen, S. (2020). Digital Twins: A Critical Discussion on Their Potential for Supporting Policy-Making and Planning in Urban Logistics. Sustainability 12, 10623. doi:10.3390/su122410623
Mehmood, R., Meriton, R., Graham, G., Hennelly, P., and Kumar, M. (2017). Exploring the Influence of Big Data on City Transport Operations: a Markovian Approach. Int. J. Oper. Prod. Manag. 37 (1), 75–104. doi:10.1108/ijopm-03-2015-0179
Mommens, K., Buldeo Rai, H., van Lier, T., and Macharis, C. (2021). Delivery to Homes or Collection Points? A Sustainability Analysis for Urban, Urbanised and Rural Areas in Belgium. J. Transp. Geogr. 94, 103095. doi:10.1016/j.jtrangeo.2021.103095
Moros-Daza, A., Amaya-Miera, R., and Paternina-Arboleda, C. (2020). Port Community Systems: A Structured Literature Review. Transp. Res. Part A 133, 27–46. doi:10.1016/j.tra.2019.12.021
Musolino, G., Polimeni, A., and Vitetta, A. (2016). Freight Vehicle Routing with Reliable Link Travel Times: a Method Based on Network Fundamental Diagram. Transp. Lett. 14, 1–13. doi:10.1080/19427867.2016.1241040
Nikitas, A., Michalakopoulou, K., Njoya, E. T., and Karampatzakis, D. (2020). Artificial Intelligence, Transport and the Smart City: Definitions and Dimensions of a New Mobility Era. Sustainability 12, 2789. doi:10.3390/su12072789
Nigro, M., Castiglione, M., Colasanti, F. M., De Vincentis, R., Valenti, G., Liberto, C., et al. (2022). Exploiting Floating Car Data to Derive the Shifting Potential to Electric Micromobility. Transp. Res. Part A Policy Pract. 157, 78–93. doi:10.1016/j.tra.2022.01.008
Nuzzolo, A., and Comi, A. (2018). A Subjective Optimal Strategy for Transit Simulation Models. J. Adv. Transp. 2018, 1–10. Article ID 8797328. doi:10.1155/2018/8797328
Nuzzolo, A., Russo, F., and Crisalli, U. (2001). A Doubly Dynamic Schedule-Based Assignment Model for Transit Networks. Transp. Sci. 35 (3), 268–285. doi:10.1287/trsc.35.3.268.10149
Nuzzolo, A., Russo, F., and Crisalli, U. (2003). Transit Network Modelling. The Schedule-Based Dynamic Approach. Milan (Italy): Franco Angeli.
Nuzzolo, A., Persia, L., Comi, A., and Polimeni, A. (2019). “Shared Autonomous Electrical Vehicles and Urban Mobility: A Vision for Rome in 2035,” in Data Analytics: Paving the Way to Sustainable Urban Mobility. Advances in Intelligent Systems and Computing. Editors E. Nathanail, and I. Karakikes (Cham: Springer), 879, 772–779. CSUM 2018. doi:10.1007/978-3-030-02305-8_93
Panarello, A., Tapas, N., Merlino, G., Longo, F., and Puliafito, A. (2018). Blockchain and IoT Integration: A Systematic Survey. Sensors 2018 (18), 2575. doi:10.3390/s18082575
Rai, H. B., Mommens, K., Verlinde, S., and Macharis, C. (2019). How Does Consumers' Omnichannel Shopping Behaviour Translate into Travel and Transport Impacts? Case-Study of a Footwear Retailer in Belgium. Sustain. Switz. 11 (9), 2534. doi:10.3390/su11092534
Ruand, M., Lina, J., and Kawamura, K. (2012). Modeling Urban Commercial Vehicle Daily Tour Chaining. Transp. Res. Part E Logist. Transp. Rev. 48 (6), 1169–1184. doi:10.1016/j.tre.2012.06.003
Ruiz-Meza, J., Meza-Peralta, K., Montoya-Torres, J. R., and Gonzalez-Feliu, J. (2021). Location of Urban Logistics Spaces (ULS) for Two-Echelon Distribution Systems. Axioms 10 (3), 214. doi:10.3390/axioms10030214
Russo, F., and Comi, A. (2017). From the Analysis of European Accident Data to Safety Assessment for Planning: the Role of Good Vehicles in Urban Area. Eur. Transp. Res. Rev. 9. Springer Berlin Heidelberg. doi:10.1007/s12544-017-0225-09
Russo, F., and Comi, A. (2020). Investigating the Effects of City Logistics Measures on the Economy of the City. Sustainability 12 (4), 1439. doi:10.3390/su12041439
Russo, F., and Comi, A. (2021). Sustainable Urban Delivery: The Learning Process of Path Costs Enhanced by Information and Communication Technologies. Sustainability 13, 13103. doi:10.3390/su132313103
Russo, F., and Comi, A. (2022). Providing Dynamic Route Advice for Urban Goods Vehicles: the Learning Process Enhanced by the Emerging Technologies. Transp. Res. Proc. 62, 632–639. Elsevier Ltd. doi:10.1016/j.trpro.2022.02.078
Russo, F., and Musolino, G. (2021). The Role of Emerging ICT in the Ports: Increasing Utilities According to Shared Decisions. Front. Future Transp. 2, 722812. doi:10.3389/ffutr.2021.722812
Russo, F., and Vitetta, A. (2006). La ricerca di percorsi in una rete. Algoritmi di minimo costo ed estensioni. Milan: Franco Angeli.
Russo, F., Vitetta, A., and Polimeni, A. (2010). From Single Path to Vehicle Routing: The Retailer Delivery Approach. Proc. - Soc. Behav. Sci. 2 (3), 6378–6386. doi:10.1016/j.sbspro.2010.04.046
Sánchez-Díaz, I., Holguín-Veras, J., and Ban, X. J. (2015). A Time-dependent Freight Tour Synthesis Model. Transp. Res. Part B Methodol. 78, 144–168.
Schroten, A., Van Grinsven, A., Tol, E., Leestemaker, L., Schackmann, P. P., Vonk-Noordegraaf, D., et al. (2020). Research for TRAN Committee – The Impact of Emerging Technologies on the Transport System. Brussels: European Parliament, Policy Department for Structural and Cohesion Policies.
Spiess, H., and Florian, M. (1989). Optimal Strategies: a New Assignment Model for Transit Networks. Transp. Res. Part B Methodol. 23 (2), 83–102. doi:10.1016/0191-2615(89)90034-9
Tan, B. Q., Wang, F., Liu, J., Kang, K., and Costa, F. (2020). A Blockchain-Based Framework for Green Logistics in Supply Chains. Sustainability 12, 4656. doi:10.3390/su12114656
Taniguchi, E., Thompson, R. G., Yamada, T., and van Duin, R. (2001). City Logistics – Network Modelling and Intelligent Transport Systems. Kidlington, Oxford: Pergamon.
Taniguchi, E., Dupas, R., Deschanmps, J-C., and Qureshi, A. G. (2018). “Concepts of an Integrated Platform for Innovative City Logistics with Urban Consolidation Centers and Transshipment Points,” in City Logistics 3: Towards Sustainable and Liveable Cities. Editors E. Taniguchi, and R. G. Thompson (London: ISTE), 129–146. doi:10.1002/9781119425472.ch7
Thompson, R. G., and Zhang, L. (2018). Optimising Courier Routes in Central City Areas. Transp. Res. Part C 93, 1–12. doi:10.1016/j.trc.2018.05.016
Trecozzi, M. R., Iiritano, G., and Petrungaro, G. (2022). Liveability and Freight Transport in Urban Areas: the Example of the Calabria Region for City Logistics. Transp. Res. Proc. 60, 116–123. doi:10.1016/j.trpro.2021.12.016
UN (2015). Transforming Our World: The 2030 Agenda for Sustainable Development. United Nations. Available at: https://sdgs.un.org/2030agenda.
Veres, M., and Moussa, M. (2020). Deep Learning for Intelligent Transportation Systems: A Survey of Emerging Trends. IEEE Trans. Intell. Transp. Syst. 21 (8), 3152–3168. doi:10.1109/TITS.2019.2929020
Vermesan, O., John, R., Pype, P., Daalderop, G., Kriegel, K., Mitic, G., et al. (2021). Automotive Intelligence Embedded in Electric Connected Autonomous and Shared Vehicles Technology for Sustainable Green Mobility. Front. Future Transp. 2, 688482. doi:10.3389/ffutr.2021.688482
Zhan, F. B., and Noon, C. E. (1998). Shortest Path Algorithms: An Evaluation Using Real Road Networks. Transp. Sci. 32, 65–73. doi:10.1287/trsc.32.1.65
Zhang, Z., Jigang, W., and Duan, X. (2010). “Practical Algorithm for Shortest Path on Transportation Network,” .in 2010 International Conference on Computer and Information Application (Tianjin), 48–51. doi:10.1109/ICCIA.2010.6141534
Keywords: city logistics, intelligent transport systems, information and communication technologies, navigation system, vehicle routing and scheduling, courier route, dynamic freight management, smart city
Citation: Comi A and Russo F (2022) Emerging Information and Communication Technologies: the Challenges for the Dynamic Freight Management in City Logistics. Front. Future Transp. 3:887307. doi: 10.3389/ffutr.2022.887307
Received: 01 March 2022; Accepted: 24 May 2022;
Published: 22 July 2022.
Edited by:
Luigi Dell'Olio, University of Cantabria, SpainReviewed by:
Edoardo Marcucci, Roma Tre University, ItalyJose Luis Moura, University of Cantabria, Spain
Copyright © 2022 Comi and Russo. This is an open-access article distributed under the terms of the Creative Commons Attribution License (CC BY). The use, distribution or reproduction in other forums is permitted, provided the original author(s) and the copyright owner(s) are credited and that the original publication in this journal is cited, in accordance with accepted academic practice. No use, distribution or reproduction is permitted which does not comply with these terms.
*Correspondence: Antonio Comi, comi@ing.uniroma2.it