- 1Human Factors and Applied Statistics Lab, Department of Mechanical and Industrial Engineering, University of Toronto, Toronto, ON, Canada
- 2The Hong Kong University of Science and Technology (Guangzhou), Guangzhou, China
Background: SAE Level 2 driving automation, the state-of-the-art in commercial vehicles, requires drivers to monitor the environment to resume vehicle control when automation limits are surpassed. However, this type of driving automation was found to increase distraction engagement. Although experienced drivers were shown to better regulate their visual engagement in distracting activities when driving with and without automation, the moderating effects of road demands (e.g., road alignment) have yet to be explored in detail, in particular, for driving with automation.
Objective: To better understand the combined effects of road alignment, driving automation, and driving experience, we investigated the effects of these factors on driver glances to a visual-manual distraction task.
Method: We present a secondary analysis, using quantile regression, of two previously reported driving simulator experiments. A total of 32 participants’ data, 16 from each experiment, were utilized. Half of these participants were novice and the other half were experienced drivers. The first experiment focused on non-automated driving, while the second focused on driving with adaptive cruise control and lane keeping assistance systems combined (i.e., SAE Level 2). The analysis reported here focuses on drivers’ visual distraction engagement in two highway drives that were identical across the two experiments.
Results: With driving automation, compared to experienced driver glances, the duration of novice driver glances to the distraction task was more variable, longer, and less sensitive to variations in road alignment.
Implications: These findings suggest that, with driving automation, novice drivers are more at risk of inappropriate engagement in distractions and do not adapt to road demands as well as experienced drivers, and thus should be supported accordingly.
Introduction
Driving automation technologies that are currently commercially available can relieve drivers from vehicle control tasks such as steering, accelerating, and braking. When these technologies are used together, such as when Adaptive Cruise Control (ACC) and Lane Keeping Assist (LKA) systems are combined, the driver does not have to provide control inputs to the vehicle for periods of time. However, given the limitations of these systems, the driver has to monitor the roadway at all times to take over control of the vehicle when system limits are exceeded. This type of driving automation is categorized as Level 2 by the Society of Automotive Engineers (SAE On-Road Automated Vehicle Standards Committee, 2018) and is the most advanced type of driving automation that is currently available commercially. For example, according to AAA Foundation for Traffic Safety, 13.9% of vehicles produced in 2018 are equipped with ACC, and 11.8% are equipped with LKA (American Automobile Association, 2019).
Both simulator and naturalistic studies suggest that drivers are more likely to engage in distracting activities and look less at the road when driving with Level 2 automation (Carsten et al., 2012; de Winter et al., 2014; Gaspar and Carney, 2019; He and Donmez, 2019; Dunn et al., 2021). This degraded attention to the roadway can impair drivers’ ability to respond to events that the automation cannot handle. Longer off-road glances (usually to non-driving or secondary tasks) can be especially distracting as they can divert drivers’ attention away from the driving task for a considerable period of time. In non-automated driving, it has been observed that off-road glances longer than 2 s can double the risk of safety-critical events (Klauer et al., 2006; Victor et al., 2015). Although a quantifiable relationship between off-road glance durations and crash risk is yet to be established in the context of driving automation, investigations of recent automated vehicle crashes have revealed that drivers were not able to respond when automation failed because they were engaging in distracting activities, which diverted their attention away from the roadway (e.g., National Transportation Safety Board, 2018a; National Transportation Safety Board, 2018b). Simulator studies also suggest that distraction engagement can divert drivers’ attention from safety-critical areas (e.g., cues indicating potential traffic conflicts) at inopportune moments when driving with ACC and LKA (He et al., 2021).
Various situational factors have been documented to moderate distraction engagement and visual attention in non-automated driving. For example, based on a subset of data from Second Strategic Highway Research Program 2, Risteska et al. (2021) investigated several environmental factors, in particular, the visual demand (based on weather, lighting, locality and traffic density) and the motor control difficulty of the road (based on surface condition and alignment), and found that higher visual demand and higher motor control difficulty are both associated with decreased likelihood of distraction engagement. Drivers were also found to have shorter off-road glances on curves (Tsimhoni and Green, 2001), where visual demand increases linearly as a function of increasing curvature (Tivesten and Dozza, 2014). With SAE Level 2 driving automation, drivers have been found to allocate more attention to the road center during lane change maneuvers (Goncalves et al., 2020), during night-time driving, and in the presence of a lead vehicle (Morando et al., 2018). The moderating effects of road demands on distraction engagement or off-road glance behaviour, however, have not yet been investigated in the context of driving automation systems like ACC and LKA, which have limited functionality in many aspects, particularly on curves. For example, the LKA function in Ford Co-Pilot360™ would stop working when “driving at high speeds on curves” (Ford Motor Company, 2021). Given the potential automation failures that might occur on curves, it is especially important to investigate whether drivers who use driving automation continue to adapt their visual distraction engagement based on roadway curvature. It is possible that they may not, given that they do not have to physically control the vehicle.
Driving experience may further moderate how road demands affect drivers’ visual distraction engagement when using automation. More experienced drivers have been observed to demonstrate better regulation of distraction engagement, particularly off-road glances, in both non-automated (Wikman et al., 1998) and automated vehicles (He and Donmez, 2019). Short and frequent off-road glances have been observed among more experienced drivers who, in general, have safer driving records (Mayhew et al., 2003). In non-automated driving, experienced drivers have been shown to adapt their visual attention based on the complexity of the roadway better than novices (Crundall and Underwood, 1998). Further, in both non-automated (Wikman et al., 1998) and automated driving (He and Donmez, 2019), experienced drivers have been found to exhibit safer (i.e., shorter) off-road glances when engaging in non-driving activities. How road demands and driving experience would interact to guide distraction engagement in automated driving is however not yet investigated.
The objective of this paper is to investigate the effects of road alignment (straight road segments versus curved segments), automation, and driving experience on driver glances to distracting activities. We conducted a secondary analysis of data from two previously reported driving simulator experiments. The first experiment, reported in He and Donmez (2020), focused on non-automated driving. The second experiment, reported in He et al. (2021), was comparable to the first in terms of driving scenarios and experimental conditions and investigated driving with ACC and LKA. Both experiments had both novice and experienced drivers (counterbalanced in number). Half of the drivers in each experiment were provided with a visual-manual secondary task (i.e., non-driving task or distraction); our analysis focused on these drivers.
The analysis in this paper extends the analysis we reported in He and Donmez (2019), in which we used data from both experiments to compare secondary task engagement between automated and non-automated driving, while considering the role of driving experience but not road alignment. Further, the analysis in He and Donmez (2019) focused only on limited glance metrics and more traditional analysis techniques that utilized mean glance durations, which did not provide insights on how the various factors affect the distribution of glance durations. Previous studies have used quantile regression to more fully investigate the distribution of off-road glance durations (Liu et al., 2018; Yang et al., 2020). For example, in a simulator study of non-automated driving, Liu et al. (2018) found that the modality of the secondary task (e.g., reading versus text entry) affected different quantiles of the off-road glance distribution differently, with a particular impact on the upper tail (85th percentile) of the distribution, i.e., longer glances. In an on-road study comparing SAE Level 2 automation to no automation, Yang et al. (2020) found that automation led to a longer-tailed distribution of off-road glance durations when drivers engaged in distractions (e.g., email sorting and music selection). Similar to these earlier studies, we also utilize a quantile regression methodology to investigate the distribution of off-road glance durations in our analysis, but we additionally focused on the effects of road alignment and driving experience, and their interactions with automation.
Methods
Dataset
The dataset analyzed in this paper comes from two experiments conducted in a fixed-base NADS MiniSim driving simulator (Figure 1A). A 10.6-inch Surface Pro 2 tablet was mounted to the right of the dashboard (Figure 1A) and displayed the secondary task for those in the secondary task conditions (Figure 1B). All participants wore a Dikablis head-mounted eye-tracker to record their eye movements. The first experiment focused on non-automated driving (He and Donmez, 2020), while the second investigated driving with automation, in particular, the combined use of ACC and LKA (He et al., 2021). In the sections below, we provide the relevant methodological details for these two experiments; further details can be found in the respective publications for each study.
Experimental Design and Participants. Each experiment had two between-subjects variables: driving experience (experienced vs. novice) and secondary task (yes vs. no). The analysis reported in the current paper focuses on the secondary task condition. A total of 32 participants completed the secondary task condition in the two simulator studies (Table 1), with 16 of them driving without automation in Experiment 1, and 16 of them driving with automation (ACC and LKA) in Experiment 2. In each experiment, half of these participants (n = 8) were novice drivers, and the other half were experienced drivers (n = 8). Participants were balanced for gender (4 female and 4 male) under each experimental condition.
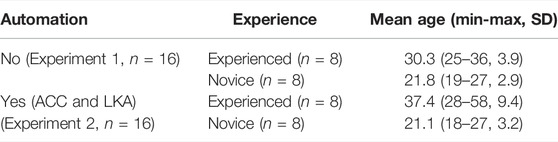
TABLE 1. Age information for the participants whose data were used in the current analyses, i.e., for participants who completed the secondary task condition. Automation and experience are both between-subjects variables; SD: standard deviation.
Following the criteria used in previous studies that identified significant differences between experienced and novice drivers (Stahl et al., 2014; Stahl et al., 2016), the novice drivers in our two studies were required to have had their G2 license in Ontario (or equivalent in Canada or the U.S.) for less than 3 years and less than 10,000 km driving mileage in the past 1 year; experienced drivers were required to have had a full license in Ontario (or equivalent in Canada or the U.S.) for more than 8 years and more than 20,000 km driving mileage in the past 1 year. All participants were recruited mainly through advertisements posted on the University of Toronto campus, in online forums, and nearby residential areas. Participants used in this analysis were comparable in age across the two experiments (p = 0.13), except novices were younger than experienced drivers as one would expect (mean difference: 12.4 years, F (1, 28) = 34.9, p < 0.0001). Only a few participants in Experiment 2 reported to have ever used ACC or LKA systems, thus, they were largely novices when it comes to using this type of driving automation.
Driving Task. In both experiments, the participants completed four experimental drives, each about 5 min long, in the order of a rural drive, a highway drive, another rural drive, and a final highway drive. The drives were the same across the two experiments. The analysis reported in this paper focused on the highway drives (as shown in Figure 2), as highway driving is the primary operational design domain for most ACC and LKA systems available in the market (e.g., Cadillac, 2021). Participants were instructed to drive at a speed limit of 60 mph during the highway drives. The 4-lane highway was separated by grass median strip. There was moderate ambient traffic (4–5 vehicles per minute) in the opposite lanes, several lead vehicles ahead, and 1 or 2 following vehicles in the ego-lane. There was one curve in each highway drive. The curves had moderate curvature: 2,100 feet and 2,950 feet radius for the first and the second highway drives, respectively. The LKA in Experiment 2 could navigate through these curves without drivers’ intervention.
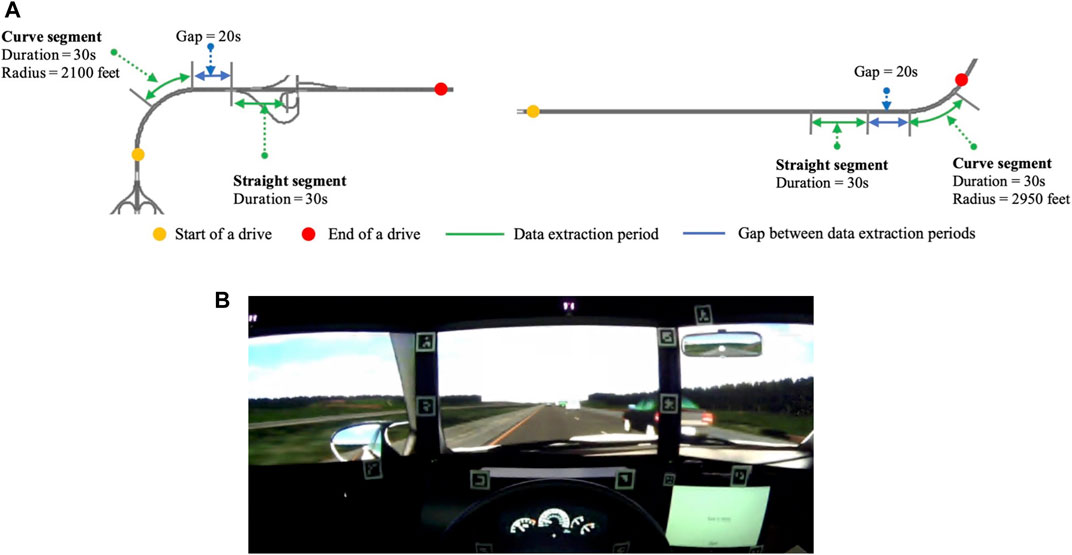
FIGURE 2. Data extraction period of highway drives (A), and picture of the simulated environment on highway (B).
In Experiment 1, participants drove the vehicle themselves. In Experiment 2, participants were required to use both the ACC and LKA systems when possible and only take over control of the vehicle when they deemed it necessary—participants utilized in our analysis, on average, engaged ACC 78.3% (standard deviation, SD: 10.9%) and LKA 88.5% (SD: 6.5%) of the total driving time. The participants were verbally informed about some limitations of the automation (i.e., ACC would not respond to stationary objects and may fail to stop the vehicle successfully in intensive braking events; and LKA may not work if the lane markings are faded or not visible). However, they were not informed specifically about any potential automation failures on curves, as the experiments were not designed for the purpose of investigating the effects of road alignment. The drivers practiced using the ACC and LKA in a route that was similar to the experimental drives in terms of the road alignment and traffic density, and experienced one failure in the practice drive that was designed to prime their anticipation of automation failures. However, there were no automation failures during the experimental drives that required driver intervention. In general, there was also training and practice in both experiments regarding the use of the driving simulator as well as how to perform the secondary task.
Secondary Task. A self-paced visual-manual secondary task developed by Donmez et al. (2007) was used in both experiments. In the secondary task conditions, the task was available throughout the whole drive and participants could choose when to engage in the task. The task mimicked interactions with in-vehicle infotainment systems and required participants to search through a list of 10 phrases in the presence of distractor words (e.g., “Predict”), for a phrase that had either “Discover” as the first word, “Project” as the second word, or “Missions” as the third word. This secondary task has been demonstrated to degrade driving performance in several stimulator studies (e.g., Merrikhpour and Donmez, 2017; Chen et al., 2018) and enabled the participants to control their distraction engagement given its self-paced nature.
Analysis
In addition to the between-subject factors of automation and driving experience (see Table 1), our analysis also investigated road alignment (straight vs. curved segments) as a within-subject factor. Gaze data was extracted from the two highway drives of each participant. In particular, a 30-second-long curved segment and a matching 30-second-long straight segment were chosen for data extraction for each drive, given that it took 37 s (in highway drive 1) and 35 s (in highway drive 2) to drive through the curves at the speed limit (60 mph). We imposed a 20-s gap between the two segments to avoid any overlapping effects of road geometry, and picked the straight segment to come after the curve in drive 1 and before the curve in drive 2 to help counterbalance potential order effects.
We conducted a quantile regression analysis to investigate the effects of road alignment, automation, driving experience, and their two-way and three-way interactions (i.e., predictor variables) on the distribution of glance durations toward the secondary task display. Unlike linear regression, which focuses on the mean of the dependent variable, quantile regression can directly estimate the effect of a predictor on the tails of the distributions of the dependent variable and makes no assumptions about the distribution of the dependent variable. In our study, the quantile regression was built in R 4.0.4 “brms” package, a package that supports the development of Bayesian generalized linear and nonlinear multivariate multilevel models using the probabilistic programming language Stan (Bürkner, 2017). We used uninformative priors and focused on the 15th, 50th, and 85th percentile glance duration on the secondary task display (i.e., dependent variables). These percentiles were selected following Liu et al. (2018), DinparastDjadid et al. (2019), and Yang et al. (2020). While the 15th and 50th percentile values capture the distributions of shorter glances and the central tendency, the 85th percentile captures the distribution of longer glances that are particularly important for safety considerations. As stated in the introduction, long off-road glances, in particular, glances longer than 2 s, are known to increase crash risk (Klauer et al., 2006). For all three models, participant was always included as a random effect (1|participant specified in the R model); in addition, for the 85th percentile model, the experience-by-automation interaction was further allowed to deviate from its single global estimation (i.e., “1+experience*automation|participant” is further specified in the R model). The choice of the models was based on the model fit, evaluated using the Posterior Predictive Checks function.
Based on earlier studies (e.g., He and Donmez, 2019; Yang et al., 2020), we expected that compared to no automation, automation would lead to longer glances to the secondary task, as indicated by increases in the three percentile values, with a larger increase in longer glances (85th percentile) as demonstrated by Yang et al. (2020). However, this hypothesized automation effect was expected to be moderated by road alignment, i.e., we expected that the effect would be smaller on curves compared to on straight road segments, similar to what has been observed in non-automated driving (Tsimhoni and Green, 2001), given that the automation we evaluated required drivers to monitor the road at all times. This hypothesized automation effect was also expected to be smaller for experienced drivers compared to novices, as experienced drivers were found to be better at self-regulating their off-road glances in non-automated driving (Wikman et al., 1998), exhibited fewer long off-road glances (> 2 s) when driving with automation, and had a smaller increase in average off-road glance duration when presented with automation (He and Donmez, 2019).
Results
Figure 3 visualizes the distribution of glance durations toward the secondary task display under different experimental conditions along with quantile regression estimates. Based on the plotted distributions, it appears that when using driving automation (ACC and LKA), the glance durations were more dispersed with longer off-road glances (i.e., the distribution was more skewed) than when not using automation; this difference was more noticeable for novice drivers compared to experienced drivers. Further, it is apparent that there is more variability across the glance distributions of different individuals, particularly novice drivers, when using automation. For non-automated driving, the glance durations appear to get shorter on curves than straight segments, with less variability among individuals. With driving automation (ACC and LKA), glance durations appear to get shorter on curves than on straight segments only for experienced drivers. Tables 2 and 3 present the results of the quantile regression analysis investigating these data trends statistically, which will be discussed further in the following sections.
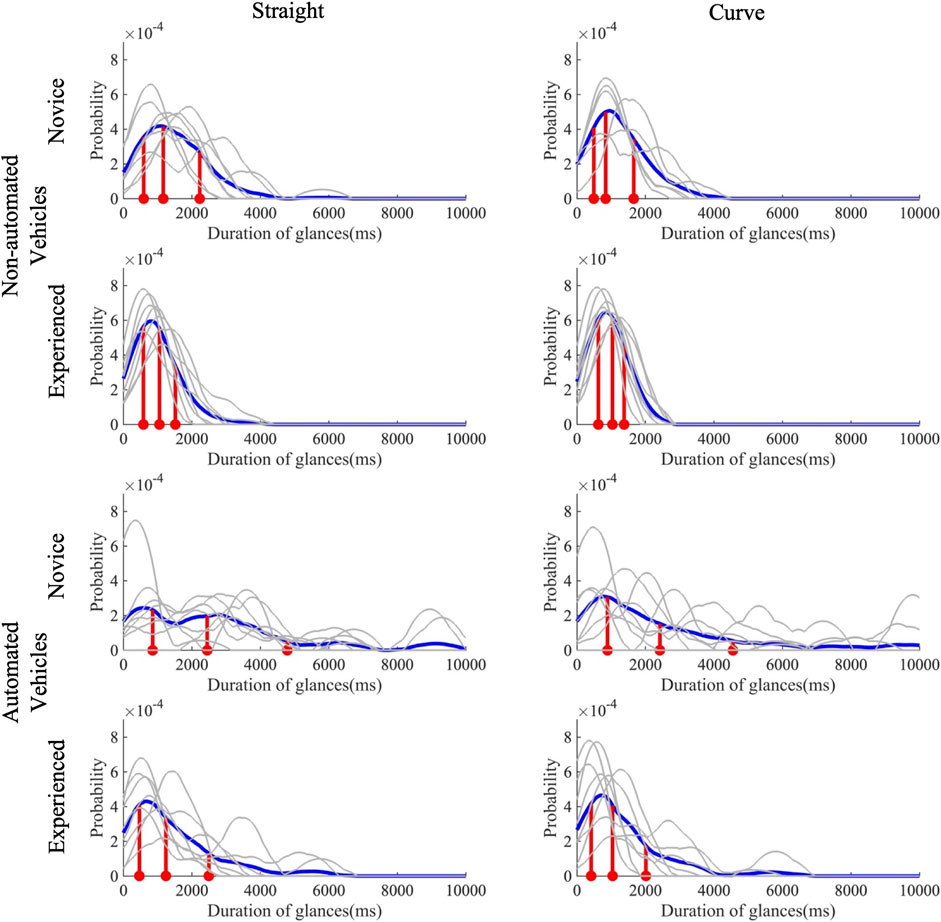
FIGURE 3. Distribution of glance durations toward the secondary task. The grey lines represent the distribution of glance durations for each participant and the blue lines represent the distribution of all glances from all participants within each experimental condition. From left to right, the vertical red lines mark 15th percentile, 50th percentile, and 85th percentile glance durations extracted from the quantile regression analysis.
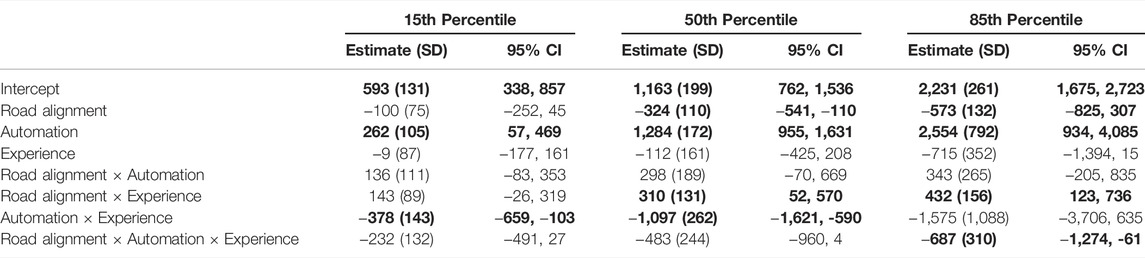
TABLE 2. Quantile regression results; significant (p < 0.05) findings are bolded; SD: standard deviation, CI: credible interval.
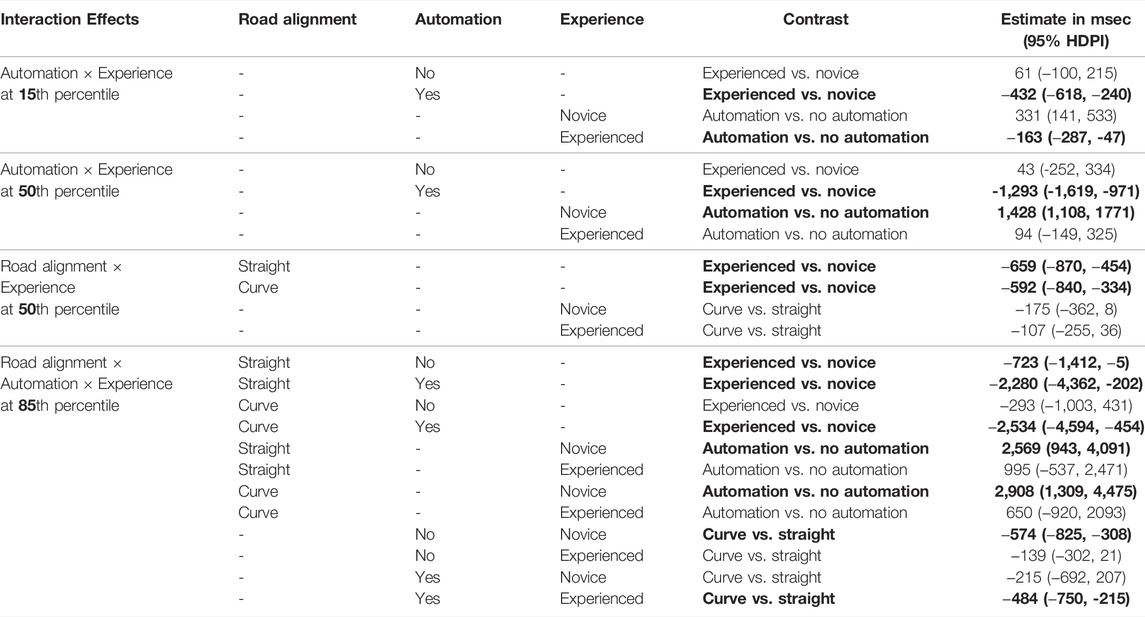
TABLE 3. Post-hoc contrasts for significant main and interaction effects; significant (p < 0.05) findings are bolded; HPDI: highest posterior density interval.
Overall, the quantile regression estimates presented in Figure 3 reveal that, particularly risky levels of off-road glance durations (i.e., > 2 s) are observed at much lower quantiles for automated driving than for non-automated driving. For non-automated driving, only the novice drivers on straight road segments had an 85th percentile estimate longer than 2 s, and at a value slightly above 2 s. For automated driving, the 85th percentile estimate for experienced drivers were at (curve) or slightly above 2 s (straight), whereas the 50th percentile estimate for novice drivers exceeded 2 s with 85th percentile values around 5 s, indicating an alarming trend.
As presented in Table 2, at the 15th percentile level, a significant two-way interaction between automation and driving experience was observed; at the 50th percentile level, two significant two-way interactions were observed, i.e., between driving experience and road alignment, and between automation and driving experience; at the 85th percentile level, a three-way interaction was observed. Follow-up contrasts were built to investigate these interaction significant interaction effects. Table 3 presents the results of the follow-up contrasts.
As supported by the significant contrasts presented in Table 3, our expectation that driving automation would result in longer glances overall, at all percentile levels, was confirmed, but only for novice drivers: the statistical results show that at all percentiles analyzed, driving automation increased glance durations among novice drivers—the increase was the largest at the 85th percentile, followed by the 50th, and then the 15th percentile. This effect of driving automation was not observed for experienced drivers, who did not exhibit any changes at the 50th and 85th percentile levels, but shorter glance durations at the 15th percentile level. As a result, with driving automation, experienced drivers exhibited shorter glances toward the secondary task compared to novice drivers at all percentile levels, with the largest difference observed at the 85th percentile, followed by the 50th percentile, and then the 15th percentile. Whereas, with no automation, experienced drivers exhibited shorter glances toward the secondary task compared to novice drivers only at the 85th percentile level and on straight roads. Thus, the differences between novice and experienced drivers were amplified going from no automation to automation.
As for the effect of road alignment, it was found to be moderated by the effect of driving experience and automation. With driving automation, experienced drivers exhibited shorter 85th percentile glance durations on curves compared to straight segments; this effect was not observed among novice drivers with driving automation. Novice drivers, however, had shorter 85th percentile glance durations on curves compared to straight segments in non-automated driving, potentially due to the increased difficulty in manually controlling the vehicle on curves.
Discussion
In this paper, we investigated the effects of road alignment, automation (in the form of ACC and LKA, or SAE Level 2 automation), and driving experience on driver glance durations toward a visual-manual distracting task. We utilized quantile regression in our analysis to examine glance distributions at the 15th, 50th, and 85th percentile levels, going beyond measures of central tendency.
Our results confirm our expectation that automation would be associated with longer glances to the distracting task, as was reported in earlier studies (e.g., He and Donmez, 2019; Yang et al., 2020), and that the effect of automation would be moderated by driving experience. In particular, novice drivers exhibited longer off-road glances with automation than without automation at all percentiles analyzed, with larger differences observed at higher percentiles, while automation did not lengthen experienced driver glances. The larger differences observed for novice drivers at higher percentiles are in line with Yang et al. (2020) who found automation to affect the 85th percentile glance durations the most. Overall, with automation, novice drivers had longer glance durations compared to experienced drivers at all percentiles—in fact, the 50th percentile glance duration estimates for novices exceeded 2 s, with the 85th percentile estimates around 5 s. This trend is alarming, as these extremely long off-road glances can impair novice drivers’ ability to perceive issues with automated control and resume vehicle control in a timely and safe manner.
Studies on automated driving have demonstrated that drivers can adapt their attention based on driving maneuvers and lighting conditions: e.g., drivers using automation allocate more attention to the road center during a lane change maneuver (Goncalves et al., 2020), during night-time driving, and in the presence of a lead vehicle (Morando et al., 2018). The analyses we present extend these earlier automation studies by focusing on the effects of road alignment while considering distraction engagement and driving experience. Our findings suggest that, like for non-automated driving (Tsimhoni and Green, 2001), experienced drivers are better able to adapt their visual distraction engagement based on road alignment also with Level 2 driving automation. In particular, at the 85th percentile level, we observed shorter glances toward the secondary task on curves compared to straight segments among experienced drivers but not among novice drivers. Novice drivers had shorter 85th percentile glance durations on curves compared to straight segments only in non-automated driving, potentially due to the increased difficulty in manually controlling the vehicle on curves.
As can be seen in Figure 3, the variability across the glance distributions of novice drivers was in general larger than it was across the glance distributions of experienced drivers; the contrast was more visible with automation than it was without automation. Off-road glance behaviors appear to become more uniform across individuals with the accumulation of driving experience, yet automation introduces some variability, e.g., due to attitudes towards or experience with automation (Hergeth et al., 2016; Roche et al., 2019). Automation however appears to have amplified the individual differences among novice drivers to a greater extent than it did among experienced drivers. Further research is needed to investigate the individual differences among these different demographic groups to develop personalized and more targeted countermeasures for using driving automation while distracted. Overall, our findings point toward a need for attention management training for novice drivers in the use of driving automation systems like ACC and LKA. Given the proliferation of these systems, novice drivers are now more likely to first start driving a vehicle with automation, and may not learn how to properly adapt their attention based on road demands if they do not get much experience in manual vehicle control. Although there has been research for novice driver training for non-automated driving (e.g., FOCAL in Pradhan et al., 2011), research is lacking with regards to the training of novice drivers in the use of driving automation.
Our use of only two driving scenarios—driving on curved and straight segments of a highway with light ambient traffic—is one of the limitations of this study. Most driving automation systems that are available in production vehicles can navigate through these scenarios safely as was the case for the SAE Level 2 system simulated in our experiment. However, a wider variety of scenarios and scenarios where the limits of automation are surpassed are needed to generalize our findings about road alignment in particular and road demands in general. Future work can also investigate the moderating effects of other environmental factors (e.g., weather and lighting) on drivers’ distraction engagement behaviors. Further, the experiments, which provided the data for the analysis presented here, were not originally designed to investigate road alignment. Although the order of the two drives were counterbalanced, the differences in curvature across the two drives may potentially bias the results. Additionally, the participants were not explicitly informed about the potential limitations of the automation on curves. It is unclear if and how the glance behaviors may differ across novice and experienced drivers if they are informed about curves being potentially problematic with driving automation. Future research should investigate to what extent informing the drivers about automation limitations factors into their scanning of the environment.
It should be noted that as experience and age are inherently confounded in the driving population, we did not strictly control for age when recruiting our participants within the different experience groups because we wanted to obtain practically relevant results. However, given that our experienced participants were older than novices, we cannot attribute experience-related findings solely to driving experience. Given the relatively small sample size, we also did not investigate the potential influence of other demographic factors (e.g., education level). Finally, although our analysis investigated some of the factors that are influential to off-road glances, it was based on driving simulator data and hence cannot provide insights about crash risk. Future research based on naturalistic driving studies conducted for Level 2 driving automation can address this research gap.
Conclusion
Through a driving simulator study, this paper investigated the effect of road alignment, driving experience and driving automation on the distribution of drivers’ off-road glance durations when they were provided with a self-paced non-driving task. Agreeing with previous research, our results indicate that drivers, especially novice drivers, increased their distraction engagement when driving with Level 2 driving automation. Further, experienced drivers were found to be able to adapt their visual distraction engagement based on road alignment with Level 2 driving automation, but such an adaptation was not observed among novice drivers. Lastly, automation has also been found to amplify individual differences in distraction engagement with Level 2 driving automation, especially among novice drivers. Given that being distracted can impair driver responses to roadway events, it is important to develop countermeasures for novice drivers’ distraction engagement with Level 2 driving automation, while considering roadway demands (e.g., alignment).
Data Availability Statement
The original contributions presented in the study are included in the article/supplementary material, further inquiries can be directed to the corresponding author.
Ethics Statement
The studies involving human participants were reviewed and approved by University of Toronto (U of T) Research Ethics Boards (REBs). The participants provided their written informed consent to participate in this research.
Author Contributions
DH: Conceptualization, Methodology, Software, Writing original draft, Investigation, Visualization. DK: Conceptualization, Methodology, Writing original draft. BD: Conceptualization, Methodology, Funding acquisition, Supervision, Writing-review and editing.
Funding
This research is supported by Natural Sciences and Engineering Research (NSERC) Discovery Accelerator Grant No. RGPIN-2016-05580.
Conflict of Interest
The authors declare that the research was conducted in the absence of any commercial or financial relationships that could be construed as a potential conflict of interest.
Publisher’s Note
All claims expressed in this article are solely those of the authors and do not necessarily represent those of their affiliated organizations, or those of the publisher, the editors and the reviewers. Any product that may be evaluated in this article, or claim that may be made by its manufacturer, is not guaranteed or endorsed by the publisher.
Acknowledgments
We gratefully acknowledge the members of the Human Factors and Applied Statistics Laboratory of the University of Toronto for their advice in manuscript preparation. We also acknowledge Wenyi Peng, Songhui Xu, Meining Ji, Tanishq Singh, Amir Ganiev, Shuran Tang, and Dahong Zhang for their help in data cleaning.
References
Bürkner, P. C. (2017). An R Package for Bayesian Multilevel Models Using Stan. J. Stat. Softw. 80, 1–28.
Cadillac (2021). Super Cruise - Hands Free Driving | Cadilac Ownership. Available at: https://www.cadillac.com/world-of-cadillac/innovation/super-cruise (Accessed June 9.
Carsten, O., Lai, F. C. H., Barnard, Y., Jamson, A. H., and Merat, N. (2012). Control Task Substitution in Semiautomated Driving. Hum. Factors 54 (5), 747–761. doi:10.1177/0018720812460246
Chen, H.-Y. W., Hoekstra-Atwood, L., and Donmez, B. (2018). Voluntary- and Involuntary-Distraction Engagement: An Exploratory Study of Individual Differences. Hum. Factors 60 (4), 575–588. doi:10.1177/0018720818761293
Crundall, D. E., and Underwood, G. (1998). Effects of Experience and Processing Demands on Visual Information Acquisition in Drivers. Ergonomics 41 (4), 448–458. doi:10.1080/001401398186937
de Winter, J. C. F., Happee, R., Martens, M. H., and Stanton, N. A. (2014). Effects of Adaptive Cruise Control and Highly Automated Driving on Workload and Situation Awareness: A Review of the Empirical Evidence. Transportation Res. F: Traffic Psychol. Behav. 27, 196–217. doi:10.1016/j.trf.2014.06.016
DinparastDjadid, A., Lee, J. D., Domeyer, J., Schwarz, C., Brown, T. L., and Gunaratne, P. (2019). Designing for the Extremes: Modeling Drivers’ Response Time to Take Back Control from Automation Using Bayesian Quantile Regression. Human Factors: The Journal of the Human Factors and Ergonomics Society, 0018720819893429.
Dunn, N. J., Dingus, T. A., Soccolich, S., and Horrey, W. J. (2021). Investigating the Impact of Driving Automation Systems on Distracted Driving Behaviors. Accid. Anal. Prev. 156, 106152. doi:10.1016/j.aap.2021.106152
Ford Motor Company (2021). Lane Keeping System | Vehicle Features | Official Ford Owner Site. Available at: https://owner.ford.com/support/how-tos/safety/driver-assist-technology/driving/how-to-use-lane-keeping-system.html (Accessed June 10.
Gaspar, J., and Carney, C. (2019). The Effect of Partial Automation on Driver Attention: A Naturalistic Driving Study. Hum. Factors 61 (8), 1261–1276. doi:10.1177/0018720819836310
Goncalves, R. C., Louw, T. L., Quaresma, M., Madigan, R., and Merat, N. (2020). The Effect of Motor Control Requirements on Drivers' Eye-Gaze Pattern during Automated Driving. Accid. Anal. Prev. 148, 105788. doi:10.1016/j.aap.2020.105788
He, D., DeGuzman, C. A., and Donmez, B. (2021). Anticipatory Driving in Automated Vehicles: The Effects of Driving Experience and Distraction. Human Factors: Journal of Human Factors and Ergonomics Society. In press. doi:10.1177/00187208211026133
He, D., and Donmez, B. (2019). Influence of Driving Experience on Distraction Engagement in Automated Vehicles. Transportation Res. Rec. 2673 (9), 142–151. doi:10.1177/0361198119843476
He, D., and Donmez, B. (2020). The Influence of Visual-Manual Distractions on Anticipatory Driving. Human Factors: The Journal of the Human Factors and Ergonomics Society. In Press. doi:10.1177/0018720820938893
Hergeth, S., Lorenz, L., Vilimek, R., and Krems, J. F. (2016). Keep Your Scanners Peeled. Hum. Factors 58, 509–519. doi:10.1177/0018720815625744
Klauer, S. G., Dingus, T. A., Neale, V. L., Sudweeks, J. D., and Ramsey, D. J. (2006). The Impact of Driver Inattention on Near-Crash/Crash Risk: An Analysis Using the 100-Car Naturalistic Driving Study Data. (DOT HS 810 594).
Liu, S.-Y., Lee, J. D., Lee, J. Y., and Venkatraman, V. (2018). “Glances that Matter: Applying Quantile Regression to Assess Driver Distraction from Off-Road Glances,” in Proceedings of the Human Factors and Ergonomics Society Annual Meeting.
Mayhew, D. R., Simpson, H. M., and Pak, A. (2003). Changes in Collision Rates Among Novice Drivers during the First Months of Driving. Accid. Anal. Prev. 35 (5), 683–691. doi:10.1177/1541931218621443
Merrikhpour, M., and Donmez, B. (2017). Designing Feedback to Mitigate Teen Distracted Driving: A Social Norms Approach. Accid. Anal. Prev. 104, 185–194. doi:10.1016/j.aap.2017.04.016
Morando, A., Victor, T., and Dozza, M. (2018). A Reference Model for Driver Attention in Automation: Glance Behavior Changes during Lateral and Longitudinal Assistance. IEEE Transactions on Intelligent Transportation Systems.
Pradhan, A. K., Divekar, G., Masserang, K., Romoser, M., Zafian, T., Blomberg, R. D., et al. (2011). The Effects of Focused Attention Training on the Duration of Novice Drivers' Glances inside the Vehicle. Ergonomics 54 (10), 917–931. doi:10.1080/00140139.2011.607245
Risteska, M., Kanaan, D., Donmez, B., and Winnie Chen, H.-Y. (2021). The Effect of Driving Demands on Distraction Engagement and Glance Behaviors: Results from Naturalistic Data. Saf. Sci. 136, 105123. doi:10.1016/j.ssci.2020.105123
Roche, F., Somieski, A., and Brandenburg, S. (2019). Behavioral Changes to Repeated Takeovers in Highly Automated Driving: Effects of the Takeover-Request Design and the Nondriving-Related Task Modality. Hum. Factors 61 (5), 839–849. doi:10.1177/0018720818814963
SAE On-Road Automated Vehicle Standards Committee (2018). Taxonomy and Definitions for Terms Related to Driving Automation Systems for On-Road Motor Vehicles, 1–16.J3016_202104
Stahl, P., Donmez, B., and Jamieson, G. A. (2014). Anticipation in Driving: The Role of Experience in the Efficacy of Pre-event Conflict Cues. IEEE Trans. Human-mach. Syst. 44 (5), 603–613. doi:10.1109/thms.2014.2325558
Stahl, P., Donmez, B., and Jamieson, G. A. (2016). Supporting Anticipation in Driving through Attentional and Interpretational In-Vehicle Displays. Accid. Anal. Prev. 91, 103–113. doi:10.1016/j.aap.2016.02.030
Tivesten, E., and Dozza, M. (2014). Driving Context and Visual-Manual Phone Tasks Influence Glance Behavior in Naturalistic Driving. Transportation Res. Part F: Traffic Psychol. Behav. 26, 258–272. doi:10.1016/j.trf.2014.08.004
Tsimhoni, O., and Green, P. (2001). “Visual Demand of Driving and the Execution of Display-Intensive In-Vehicle Tasks,” in Proceedings of the Human Factors and Ergonomics Society Annual Meeting.
Victor, T., Dozza, M., Bärgman, J., Boda, C.-N., Engström, J., Flannagan, C., et al. (2015). Analysis of Naturalistic Driving Study Data: Safer Glances, Driver Inattention, and Crash Risk. (Report S2-S08A-RW-1). doi:10.1177/154193120104502305
Wikman, A.-S., Nieminen, T., and Summala, H. (1998). Driving Experience and Time-Sharing during In-Car Tasks on Roads of Different Width. Ergonomics 41 (3), 358–372. doi:10.1080/001401398187080
Keywords: automated driving, visual behaviors, gaze, distraction, novice drivers, road curvature, vehicle automation
Citation: He D, Kanaan D and Donmez B (2022) Distracted when Using Driving Automation: A Quantile Regression Analysis of Driver Glances Considering the Effects of Road Alignment and Driving Experience. Front. Future Transp. 3:772910. doi: 10.3389/ffutr.2022.772910
Received: 08 September 2021; Accepted: 11 April 2022;
Published: 19 May 2022.
Edited by:
Xiaohua Zhao, Beijing University of Technology, ChinaReviewed by:
Ran Tu, Southeast University, ChinaWesley Kumfer, University of North Carolina at Chapel Hill, United States
Nengchao Lyu, Wuhan University of Technology, China
Copyright © 2022 He, Kanaan and Donmez. This is an open-access article distributed under the terms of the Creative Commons Attribution License (CC BY). The use, distribution or reproduction in other forums is permitted, provided the original author(s) and the copyright owner(s) are credited and that the original publication in this journal is cited, in accordance with accepted academic practice. No use, distribution or reproduction is permitted which does not comply with these terms.
*Correspondence: Birsen Donmez, ZG9ubWV6QG1pZS51dG9yb250by5jYQ==