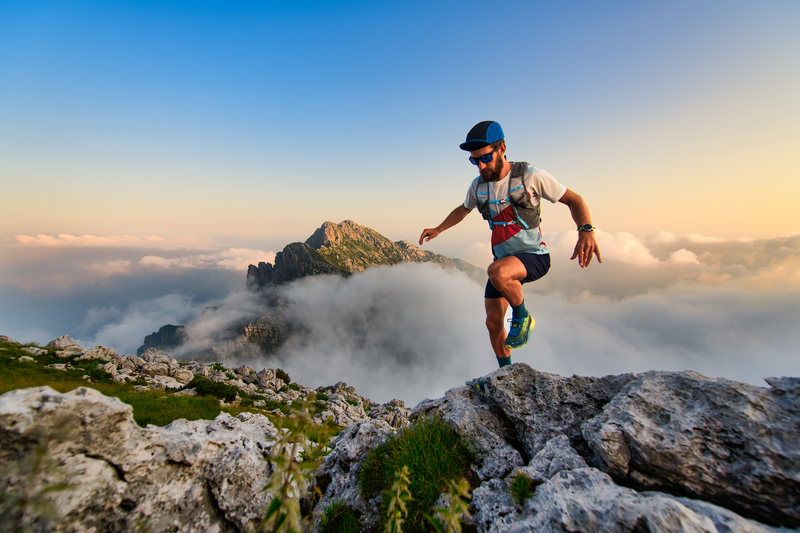
94% of researchers rate our articles as excellent or good
Learn more about the work of our research integrity team to safeguard the quality of each article we publish.
Find out more
ORIGINAL RESEARCH article
Front. Future Transp. , 27 August 2021
Sec. Freight Transport and Logistics
Volume 2 - 2021 | https://doi.org/10.3389/ffutr.2021.722812
This article is part of the Research Topic Frontiers in Maritime Transport Chains View all 7 articles
Geographical location, infrastructures, and services are the main consolidated pillars of a port in terms of its capacity to compete and cooperate with other ports. In the last years, a new pillar was identified: emerging technologies. Ports’ issues were initially solved with individual ICT solutions adopted by each decision-maker, which generated efficiencies in the three main port flows: cargo, information, and financial. However, new benefits and challenges are connected with the introduction of shared emerging ICT among decision-makers inside ports. The crucial issue concerns the fact that several decision-makers could share a decision about a single-port operation. Therefore, the effectiveness and efficiency of ports depend on how the interactions between the decision-makers are solved. Port operations are associated with movements (cargo) and transactions (information and financial) in a synchronic graph, which allows highlighting the role of emerging technologies in the modification of port operation generalized cost, considering the different decision-makers. The focal point concerns the building of a theoretical model using the formal equations of Transport System Models (TSMs) for the estimation of the cost for a Unit of Load (UL), e.g., a container traveling along a path, composed of a sequence of port operations, inside a port with and without emerging technologies. The proposed theoretical model provides the possibility of estimating ex ante the reduction of cost (port time of UL) given by introducing new technologies and a Port Community System (PCS). Different scenarios, considering some cases, ranging from the absence of ICT to the presence of a PCS, are compared, considering the different situations from a non-congested port to a congested one. The main results of the study and its novelty concern, on the one hand, the extension of TSMs to port systems, highlighting the problem of a non-single decision-maker (two or more) in some port operations and, on the other hand, the possibility of reducing the generalized cost (e.g., time) in the same operations in which there are concurrent decision-makers, through the use of an advanced PCS. The reported numerical example confirms the theoretical results. The work can be useful for researchers for port planners (e.g., port authorities) because it permits evaluating the utility for introducing shared emerging technologies using advanced PCS in a unified view.
From ancient times, ports are the interface of freight transport between land and sea. The first ports were built in the proximity of the cities, and they were the gate for the exchange of freight among cities. The port-city model worked for centuries and these ports are classified as first-generation ports.
During the middle of the XX century, some ports were built “ex novo” in the proximity of great industrial areas, offering industrial or commercial services to industrial plants. This port model belongs to the class of second-generation ports.
In the last decade of the XX century, third-generation ports emerged, principally due to the worldwide large-scale containerization, combined with the growing requirements of international trade. Ports became integrated transport centers and logistic platforms. From the economic point of view, ports increase the added value of the goods that transit through them due to not only the traditional advantage of position but also to the manipulations that are placed in the goods (UNCTAD, 1994; UNCTAD, 1999; Russo and Musolino, 2020).
At the beginning of the XXI century, growing ships’ dimensions, alliances in the shipping market, and shared hinterland access among ports in proximity determined pressure on individual ports to invest in the development of new facilities. Several ports cannot sustain this competitive game, which requires an appropriate amount of funds (Inoue, 2018). In any case, the increment or duplication of physical facilities is highly inefficient in ports of the same region if they have unused capacity. Therefore, closer ports started to cooperate, leaving the traditional attitude to compete (Martín-Alcalde et al., 2016). The four-generation ports were born by the adoption of different types of alliance-merging.
The introduction of emerging technologies in ports showed that ICT (Information and Communication Technology) is a further pillar of port competitiveness next to a geographical location near the city or industrial plants and generated added values, other than the provided infrastructure and services (Heaver et al., 2010; Carlan et al., 2016). Ports are entering a new generation: the fifth.
Issues connected to financial and information transactions are supported by the adoption of stand-alone ICT. They increase the efficiency of the individual decision-maker but do not solve the interactions between different decision-makers. The interactions are relevant in the three main flows present in the port: cargo, information (clearance authorization and permit (Gattuso et al., 2005)), and financial (Carlan et al., 2020).
In terms of physical flow, cargo management implies data exchange to optimize ship and port facilities (e.g., quays and yards) capacities. Moving from one great quantity of freight on one dedicated ship on only one origin-destination couple to thousands of containers on thousands of origin-destination couples for each ship, the complexity increases exponentially, and the traditional approach to management cannot support the challenge determined by these quantities, generating low utilization of the available ship and port facilities (e.g., quays and yards) capacities.
Regarding information/documents related to the authorization flows, old paper solutions represent such a strong constraint at a higher level that international banks define a grade of inefficiencies for each state in the Logistic Performance Index, especially in the ports (The World Bank, 2018).
Financial flows are the third class of movement in the ports. Due to the nature of international goods, containers are subject to financial transactions among different countries with different bank regulations determining delays to compare the funds and release the validation of the payments.
This article will consider mainly the technologies relative to the ICT field, not the advancement in the automation technologies that could determine the reduction in (handling) times of a Unit of Load (UL). In fact, as it will be shown in the following, the automation technologies impact only decision-makers who are in charge of the physical movements of the goods. For example, the passage from gantry cranes to autonomous transtainers could reduce the handling time; let us say from 60 to 45 s (this reduction may be simulated with the supply model, as shown in the following sections).
The full deployment of the potentialities offered by emerging ICT is possible due to the functionalities of the Port Community Systems (PCS) (Caldeirinha et al., 2020; Moros Diaz et al., 2020). They aim to increase the cooperation among all the decision-makers of a port and, therefore, their competitiveness as a community. The cooperation is possible by integrating the communication procedures, by sharing information and documents between decision-makers.
In the last 10 years, mature technologies on ICT have been introduced in the ports, firstly in the stand-alone form of DSSs (Decision Support Systems) to support cargo flow and, then, in the cooperative form of PCSs. Today, the emerging technologies define a new frontier for the ports.
The issue that arises in the ports concerns the presence of more than one decision-maker in managing port operations, considering the importance that the information and financial processes are assumed in the ports. It is often more time-consuming to solve administrative and financial problems than to execute the physical movement of goods. A decisive role in cutting down times connected to administrative and financial operations is given today by the emerging ICT, allowing different interacting decision-makers to optimize the solution of problems.
The above issue is solved by building a theoretical model using the Transport System Models (TSMs) equations. The Basic Models: Port Operations and Decision-Makers section examines the formulation of the supply model, representative of the individual port operations, the identification of the different decision-makers, references for demand, and some of the main types of choice that arise in a port. Then, the emerging technologies are recalled, which allow decisions to be shared, reducing generalized cost (time).
Each port decision-maker has developed its own stand-alone ICT system. It should be noted that the more proprietary these ICT systems are, the less they can be shared with other decision-makers. The proprietary approach allows full integration of emerging technologies within the company, ensuring high security in data control but makes it difficult (or impossible) to interact with other ICT systems. At the same time, the different flows of goods, information, and monetary value are governed by different decision-makers, which are often in opposite roles. The Port Operation Modeling section presents a modeling structure, which identifies the links between port operations, flows (of the three segments), and decision-makers. This structure highlights the role of emerging ICTs in cost functions and, therefore, builds a theoretical model to study and evaluate different ICT scenarios with different levels of shared decision among decision-makers.
The theoretical model, built based on TSM, allows studying the introduction of emerging ICT. The Deterministic User Equilibrium Model section presents a schematic example of a port system in two scenarios: with and without shared ICT. The results of the example are interesting and encourage developing the research towards the calibration, in real cases, of the cost functions with different levels of sharing decisions, supported by the ICT, among the decision-makers.
The novelty of the article lies in the possibility of studying and evaluating the benefits of ICT systems inside the ports using the TSM equations. The increases in utilities that the shared emerging ICTs can provide allow us to identify and develop a new generation of ports.
This section presents the theoretical background of the TSM, highlighting the elements that differentiate the real cases of the urban transport systems, for which the TSM was developed, from those specific of the port systems. To this end, the basic elements of the TSM are first recalled as unified in the literature of the sector.
The supply model is discussed, presenting the peculiar characteristic of the system, given by components of travel times of the links not dependent on physical movements of UL.
The demand model is then discussed, presenting, also in this case, the peculiar characteristic given by the presence of several decision-makers on which the advancement of the cargo along a link depends, unlike urban systems in which there is always only one decision-maker (in the case of passenger mobility).
The role that emerging ICT can assume with respect to the peculiar characteristics mentioned above is therefore defined. These technologies allow decision-makers to interact directly and reduce the generalized costs (times) with respect to the feasibility of a port operation, which, as shown in the supply model, is simulated with the travel time of the link.
TSMs simulate a system in which travel demand and transport supply interact. The three components of the TSM (Ortuzar and Willumsen, 2001; Cascetta, 2009) are the transport supply model, the travel demand model, and the supply-demand interaction model.
This section analyzes the basic elements for applying TSM to ports. The main operations that take place inside the ports could be analyzed and schematized using a supply (network) model. The supply model simulates performance and flows derived from choices of users and physical transport (and digital) infrastructures and services. The most common modeling approach is a network model, with links, nodes, and cost functions (e.g., time-flow relationship); in the case of ports, it is necessary to associate each link with a single operation performed.
Then, the demand component is examined by analyzing the decision-makers. TSMs traditionally consider only one decision-maker, as far as concerning the movement of a UL inside the port. In this work, the presence of administrative or financial transactions implies that there could be several decision-makers that allow and determine the movement of a UL and several emerging ICT that allow reducing the port operations times. The travel demand model simulates the choices of users derived from the performance of the port infrastructures and services. Travel demand models can be non-behavioral or behavioral. In the behavioral approach (Ben-Akiva and Lerman, 1984), travel demand patterns can be stochastic if the (dis)utility associated with each user’s choice is a random variable or deterministic if the (dis)utility associated with each user choice is a deterministic variable. In the case of ports, the demand models should evolve considering the multiplicity of decision-makers present. Therefore, it is necessary to introduce various decision-makers in the basic model.
The supply-demand interaction model simulates the interaction between the choices of users and the infrastructure and service performance.
Port operations may be associated with cargo movements in the classical synchronic graph representation (Nuzzolo and Russo 1997; Russo 2005), for the case of a container port (Galletta et al., 1999). As previously recalled, cargo flow is the reference variable given by the physical flow of containers that are “traveling” on each link. The base unit considered in this article for cargo flow is the UL (e.g., TEU for a container) (Russo, 2001). Considering also other container dimensions as FEU (Forty-Foot Equivalent Unit) can be possible, using equivalent coefficients as the ones considered in the road traffic flow to convert trucks into cars.
Disutility due to authorization and payment can be associated with the possibility of moving the UL along a specific link inside the port, that is, the same link of cargo flow in the synchronic graph.
Then, the disutility of each link represents the one associated with cargo movement and the one associated with (administrative and financial) transactions.
The individual port operations of each UL are analyzed, which are composed of physical paths that the UL must go through in the port area.
The study area considered for a port is the one that goes from the bay on the seaside to the closest national freight railway station and to the port truck terminal on the landside. The area is discretized into nodes and links and is simulated by a synchronic graph.
The main paths are as follows:
• From ship to ship
• From ship to train (and vice versa)
• From ship to truck (and vice versa)
• From train to truck (and vice versa)
In general terms, specific nodes and links representing logistics operations should be considered in the above paths, considering a port industrial park (or a Special Economic Zone), which lies inside or near the port boundary.
The main paths mentioned above are physical paths undertaken by the UL. In most of the links, the paths are governed by a single decision-maker who is in charge of the cargo's' physical movement. On the other hand, there are some links in which the cargo physical movement occurs after decisions are shared between several decision-makers. It is also worth noting that it is necessary to continuously track UL inside the ports for security purposes.
The role of the PCS is to support the interaction between multiple decision-makers to solve transactions (administrative and financial). Therefore, the PCS is fundamental to reduce the generalized port costs (times) along the above-defined paths that contain different links representative of transaction operations.
The PCS operates in four main work areas (Carlan et al., 2016) by connecting multiple decision-makers along the cargo physical flow:
• Logistics and transport, with the management of the logistics/transport chain from the hinterland to the connection with maritime transport (and vice versa); this area can be divided into logistics (agri-food, automotive, …); transport by truck, over long or short distances; transport by train.
• Navigation, to ensure regular and safe traffic and optimal planning of ship arrivals and departures (this function usually includes services, use of the port infrastructure and administrative management).
• Custom, import, and export control procedures and administrative control processes (this function has an impact on the transit times of the unit of load).
• Dangerous goods, providing the data flow for the declaration of dangerous goods and related obligations.
Schematically, the first two work areas require interaction between many decision-makers, but all of them are in charge of cargo physical movement. In comparison, the other two work areas imply interactions between decision-makers in charge of administrative and financial transactions, and then it is necessary that the information and monetary value flows are managed to minimize the transaction time. Each of the last two work areas can be further segmented into administrative and payment. By considering, for example, custom and dangerous goods, custom payment and administration and dangerous good payment and administration should be taken into account.
This definition of paths allows directly calculating some components of the Logistics Performance Index (The World Bank, 2018) and quantifying the efficiency improvements provided by the single emerging technology.
The main decision-maker in charge of physical cargo (UL) movements is the MTO (Multimodal Transport Operator). The MTO, in the typical scheme of an intercontinental route from the production region (origin of the trip) to the consumption region (destination of the trip), interacts vertically with other decision-makers, operating in the work area of transport and logistics, along a supply chain where the MTO itself always has the final decision. There are links inside the port that could comprehend an industrial park (or a Special Economic Zone), for which the decision about cargo physical movement depends on shared decisions of two or more decision-makers, interacting horizontally. These decision-makers are part of the port community, which is composed of several decision-makers, other than the MTO, that interact with each other often in a horizontal way between them and with the external environment (both on the seaside and on the landside).
The decisions involve different decision-makers, even if frequently the final path disutility is in charge of MTO. Decision-makers (also called “stakeholders” in the literature) belonging to the port community can be grouped into five categories:
• State authorities: port authority, customs, finance police, maritime police, and fire brigade
• Port companies and terminal companies
• Agencies: line agencies, maritime agencies, and port agencies
• Port service managers: maritime warning, pilots, tugs, and moorers
• Sanitary facilities: port chemist, phytopathological presidium, and maritime health
The external environments interacting with decision-makers of the port community outside the port area are as follows:
• Seaside: ship owners, shipping lines, customers, and other ports communities
• Landside: land transport operators (rail and truck operators at different distances)
The roles of decision-makers belonging to the port community have been investigated in the literature. A synthesis has been reported in Carlan et al. (2016), specific roles of decision-makers involved in agri-food logistics have been presented in Castelein et al. (2019), general roles in pursuing sustainability goals have been presented in Durán et al. (2021).
Emerging ICT involved in horizontal interactions between different decision-makers could reduce the generalized costs of port operations (e.g., times). ICT (as the name implies) modifies horizontal interactions through a more advanced level of “communication.”
A large number of emerging technologies are aggregated into five groups in this work (Atzori et al., 2017; Carlan et al., 2020; Moros Daza et al., 2020):
• Internet of Things (IoT) that allows exchanging information (e.g., authority-sanitary) about the position, temperature, and weight of goods
• Big Data (BD) that allow reconstructing the history of each container for security purposes (e.g., finance police and maritime police—shipowner)
• Blockchain (BC) also called the internet of value that allows carrying out monetary transactions between decision-makers (e.g., customs agencies)
• Artificial Intelligence (AI) that can allow allocating containers in an optimal position in yards (e.g., shipping line terminal operator)
• Digital Twin (DT) that allows simulating all the modifications of the interactions (e.g., all-all)
The 5G (or 6G) communication network is not considered because it is transversal to all other technologies.
The above technologies are mature and are generally part of the stand-alone digital platform of every decision-maker: mainly the MTO, given its global dimension. It is worth noting that the impact of stand-alone platforms on port performances is marginal because emerging ICTs is generally installed but not shared among port decision-makers. An example is given by a network of sensors or by the RFID codes associated with containers.
The new-generation PCSs offer the opportunity to incorporate emerging technologies and share them: the expected benefits increase significantly as the number of decision-makers sharing them grows.
The section presents the specification of TSM for the port operation.
Firstly, a method is presented that allows estimating the disutility of a UL for the execution of the port operations. For simplicity, the disutility (generalized cost) related to the port operation is expressed in terms of time.
The method allows estimating the port time (disutility) associated with an “origin-destination” couple along one of the above-defined paths, tod, as the combination of the time derived from a single operation, associated with a link of the synchronic network. For example, in the path from ship to train, the origin is associated with a node located where the ship arrives in the bay and is at anchor, while the destination is associated with a node located where the container is on-board the train, which is ready to leave from the railway station of primary level.
Let us define the set of port operations as An, with n being the number of port operations.
Considering the generic work area connecting several decision-makers, defined in previous sections, the generic port operation, a ∈ An, belongs to one of the following two sub-sets:
• a ∈ Amov, which includes port operations connected to cargo physicals movement, e.g., container movement
• a ∈ Atra, which includes port operations connected to a transaction (financial, or information)
Port operations generally include physical movement and transactions. Therefore, the following relationships hold:
The set An is represented by a synchronic graph, composed of links and nodes, and by cost functions.
The graph is composed of links, a = (i,j), identified with the couple (i,j) of the initial and final nodes associated with each port operation a.
The cost function associated with each link a is defined as the time associated with port operation a, ta:
where τ() is the port operation time model; Pm is defined as the set of port work areas, with p∈Pm being the generic port work area.
According to the above segmentation, the port operation time, ta, depends on two components:
where τmov() is the port operation time model associated with connected to cargo physicals movement a ∈ Amov; τtra() is the port operation time model associated with a transaction a ∈ Atra; Pmov is the subsets of Pm associated with movement p ∈ Pmov; Ptra is the subsets of Pm associated with transaction p ∈ Ptra.
The operation time ta of Eq. 3.2 may be calculated as follows by introducing some hypotheses:
• Sum of two components, assume that the transactions are always made before the physical movement (sequential execution):
• Maximum among the two components, assume that the transactions are made during the physical movement (parallel execution):
At this stage, we can introduce the following definitions:
t[n x 1] = […. ta, …..]T is the vector of link port operation times (costs) with n being the number of links (port operations).
B[n x m] = […, ta,p, ….] is the matrix of port operation times vs. work areas with ta,p, time of port operation a associated withto work area p.
According to matrix B, Eq. 3.2 can be generalized and specified as follows:
where pmov is the vector of physical movement work area; α is the vector of parameters.
where ptra is the vector of transaction work area; β is the vector of parameters.
The two components, tamov and tatra, of port operation time can be linked to different decision-makers, as described in the following:
• The component, tamov, is the time connected to cargo physical movement (handling); then, it depends on transport/logistic decision-makers.
• The component, tatra, is the time not only directly connected to cargo physical movement but also connected to financial and/or information transactions that allow cargo to be handled. Therefore, it depends on one or more decision-makers, which do not belong to the transport/logistic work area (e.g., custom) and interact with transport/logistic decision-makers.
Sq of decision-makers belonging to the port community can be introduced set, with q being the number of decision-makers and s ∈ Sq the generic decision-maker.
In order to identify the decision-makers associated with each port operation, let us define the incidence matrix of decision-makers vs. work areas:
where
By considering that:
two cases can be obtained
• ep = 1, if there are no interactions among the decision-makers in work area p.
• ep > 1, if there are interactions among the decision-makers in work area p.
According to Eq. 3.6, the two components, tamov and tatra, may be estimated as follows:
where emov is the individual decision-maker associated with cargo physical movement (the time associated with physical movement on link a, in the simplest case, is associated with one decision-maker); γ is the vector of parameters.
where etra is the vector of decision-makers associated with transaction a (the transaction time on link a is generally associated with more than one decision-maker); ϕ() is the interaction function describing the costs associated with the interaction between the decision-makers, etra is involved in the transaction associated with port operation a; δ is the vector of parameters.
The impact that each emerging technology has on port operation a, belonging to the transaction (maintaining the hypothesis that for physical movement work areas, there is only one decision-maker), could be estimated by means of function ϕa(etra). It is possible to consider different scenarios.
The time associated with port operation a, ta_A, depends on the performance of each decision-maker and the interactions among the decision-makers, as defined in matrix E.
If two decision-makers are considered, acting on a transaction work area, the interaction function becomes
Eq. 3.2, in case the condition (Eq. 3.3) holds, is specified as follows:
The time associated with port operation a, ta_B, still depends (as in scenario A) on the performance of each decision-maker and the interactions among the decision-makers, as defined in matrix E. However, it is expected that the times associated with the interactions between the decision-makers reduce. By considering the case of a DSS shared between two decision-makers (Eq. 3.8), it is expected that the following relationships hold:
DSS is the generic DSS shared by a couple of stakeholders, q and r.
The time associated with port operation a, ta_C, depends on the performance of the decision-makers, the interactions among the decision-makers, as defined in matrix E, and the emerging ICT. It is expected that times reduce for the transversal effect of the emerging ICT on the different decision-makers and work areas.
It is worth noting that the emerging ICTs can unfold their full potentialities in the presence of an electronic platform shared among decision-makers of the port community, which is a PCS:
By comparing the three above scenarios, the introduction of DSS and, then, the emerging ICT operating inside a PCS determine the following general condition for the port operation time ta:
This section presents the demand-supply interaction model that simulates the mutual interaction between port decision-makers and the infrastructure and service performance, using the TSM equations. According to the limited capacity (or not) of the available infrastructures and services, then congestion arises (or not) and costs (e.g., times) increase (or not).
Demand-supply interaction models may be classified into the following (Cascetta, 2009; Cantarella et al., 2019): static, if they simulate a transport system in stationary conditions, when travel demand, path choices, and transport network are consistent in a reference time; or dynamic, removing stationary conditions. The static models can be segmented into the free-flow approach, such as Network Loading (NL), and equilibrium approach, such as User Equilibrium (UE) (Cantarella and Cascetta, 1995). The UE model will be formulated for the port system problem, allowing the estimation of cargo flows in order to let decision-makers minimize their individual costs (Wardrop, 1952). It is worth noting that the free-flow approach can be solved directly if formalizing the cost function is possible without dependency on the flow modification.
The section presents a method that allows estimating the time of a UL that travels along a path, k, associated with an origin-destination couple, tk,od. The time associated with the path undertaken by the UL is composed of a combination (sum) of times associated with each port operation (link).
The method refers to the DUE equilibrium model by assuming that ports are congested. A user (e.g., MTO) chooses deterministically the path of UL between the origin o and the destination d, which allows minimizing his individual cost. The choice is referred to as the UL.
The path cost (time) specified in this work, tk (omitting the subscript od for simplicity), expresses the total time spent in a port (with one terminal) of a UL. The case of a port with several terminals may be obtained by replicating the same calculation. The following equation does not consider the apex UL for simplicity:
where tk(fk) [h] is the total port time along path k, which depends on the UL flow fk, (the identification between path flow and link flow holds); t0,k [h] is the total port time without congestion; Cp [UL/hour] the port capacity, defined as the maximum number of UL that may be handled in the port in an hour; μ, θ are the cost function parameters.
Without reducing the generality, it can be assumed that Eq. 4.1 can be a specification of Eq. 3.2 (in the form of Eq. 3.3) assuming that the interactions between the decision-makers, as defined using matrix E, determine the modification of the parameters μ and θ.
The travel demand model simulates the mutual interaction between port decision-makers and the infrastructure and service performance. Travel demand models can be non-behavioral, based on gravitational-entropic theory, or behavioral, based on random utility, fuzzy, or quantum utility theories (Ben-Akiva and Lerman, 1984).
The demand-supply interaction (assignment) model simulates the mutual interaction between users’ choices and infrastructure and service performance. If the capacity of the available infrastructures and services is limited, costs (e.g., times) increase and congestion arises.
Several demand-supply interaction models existing in literature are based on an equilibrium approach, such as the Deterministic User Equilibrium (DUE) (see Cantarella and Cascetta (1995)). The DUE model estimates flows in order to let users minimize their individual costs (First Wardrop principle) (Wardrop, 1952).
The DUE equilibrium is formulated as follows:
Where ζ (f) = Σp ∫0fk ck(xk) dxk is the objective function to minimize, defined as the integral cost (integral of marginal costs of each UL), depending on the vector, f, of flows; f opt,DUE is the optimum vector of flows, or DUE equilibrium vector of flows, for which costs associated with the choice of alternative ports are equal (it is the minimum value of function ζ(f)); ck(xk) is the marginal cost function of the individual UL on path/port k; fk is the flow at path/port k.
This section reports an application of the DUE equilibrium model for the test case of two ports (each of one having one terminal), equipped with different levels of technology (“tech” scenarios). Two “tech” scenarios are considered and compared:
• The first one assumes that the two ports operate with their own technologies.
• The second one assumes that there is a shared PCS operating in the two ports.
The total time in the two scenarios is estimated using the cost function of Eq. 3.12. The values of parameters are presented in Table 1. The values proposed in the table for the cost functions and the demand values are all assumed by the authors, made based on orders of magnitude present in the existing ports.
TABLE 1. Parameters of the supply model (Eq. 4.1) in the two “tech” scenarios.
The demand model of the port (the choice set is assumed to be composed of two ports: 1, 2), considered in this work, is deterministic:
The total demand is assumed to be the following:
The value of capacity for both ports is C = 500 [UL/h] and the values of the port time without congestion are different: t0,1 = 30 [h] and t0,2 = 60 [h], to emphasize the differences between the two ports.
The two “tech” scenarios are represented by different values of parameter μ, while parameter θ remains fixed. In the test case proposed, it is assumed that the port cost function (Eq. 4.1) halves the value of the variable component in the presence of PCS. It is worth noting that the proposed test case is simple, but the values used are in line with the experimental ones present in the literature.
The introduction of PCS has led to a 90% reduction in time in some fields (Carlan et al., 2016). Considering the cost reduction obtained with the use of electronic documents (compared to traditional paper documents), there is a value of about 40% going from $4.52 (electronic document with PCS) to $7.39 (paper document) (Čišić et al., 2009; Carlan et al., 2016). Starting from these values, a saving of 50% is assumed. To this end, the parameter μ is set to 2 (system without PCS) and halved and therefore equals 1 (system with PCS).
The cost functions of Eq. 4.1, with the specification of Table 1 for the two scenarios, are depicted in Figure 1, which reports the plots of the port time for each of the two ports.
FIGURE 1. Cost functions of ports 1 and 2 with and without PCS (Eq. 4.1).
The two curves for each scenario intersect at the point where the port times are equal, representing the DUE equilibrium flows: t1(f opt,UE1) = t2(f opt,UE2).
Scenario 1, represented with red curves, shows that equilibrium is reached for a greater value of port time than the value of port time related to Scenario 2, represented with green curves.
The integral cost function in Eq. 4.2 is specified for the two ports as follows:
is plotted in Figure 2 considering the two “tech” scenarios.
FIGURE 2. Integral cost function (ζ) of ports 1 and 2 with and without PCS (Eq. 4.2).
The minimum value of the function ζ() corresponds to the vector of optimal flows (or DUE equilibrium flows):
The curve of Scenario 2 always has lower values than those of the curve of Scenario 1, showing that the introduction of a shared PCS among two ports determines benefits in terms of port operation time reduction in every condition.
This article considers the role of emerging ICT in the ports, focusing on the next-generation PCSs. After a brief introduction in which the main pillars of ports are recalled, as historically determined, the new challenges related to introducing a new pillar are recalled: emerging ICT integrated inside PCSs.
The article introduces a specific element present inside the ports and not in other transport systems: the existence of work areas in which the decision is shared among different decision-makers. The problem of shared decisions is crucial in ports nowadays because the effectiveness and efficiency of ports are derived from its level of solution, which can be estimated with international indicators (The World Bank, 2018). The article presents the different types of work areas with directly affected decision-makers. The emerging ICTs technologies that can further increase the efficiency and effectiveness of the PCSs are as follows: IoT, BD, BC, AI, and DT. The individual technologies are not explored in detail, which is beyond the objectives of the article.
After introducing the different work areas, the decision-makers, and the PCSs with the emerging ICT, a unifying framework is presented, where the three elements are integrated, by introducing the TSMs equations. The simulation of supply is proposed through a synchronic graph, where all port operations are represented. For each link, the possibility is considered that the disutility derives from a single decision-maker or from more than one. This is formalized by considering the physical movements and transactions for each link. This approach allows the simulation in each link of all the work areas that insist on it.
This formulation reveals the role of PCSs (updated by emerging ICT) in the increase of utilities (or in the reduction of times). These hypotheses make it possible to simulate different scenarios, ranging from the absence of emerging ICT to the presence of PCS that supports shared decisions. The PCS can be considered a neutral system that allows the introduction of different waves of technologies without creating specific economic advantage for any decision-maker.
The formalization introduced gives the possibility of building a theoretical model using the formal equation of TSMs, quantifying the cost of a UL traveling inside the port in the presence of a PCS. The proposed approach provides the possibility of defining a new port generation formally, as introduced by UNCTAD in a descriptive way.
The approach proposed in the article provides a framework that, starting from the results of the specific study on hypothetical ports, will lead to the calibration of the (dis)utility functions for real ports.
The study presents the proposed theoretical formulation of the problem and a test case, built with parameters that reflect the time reduction in real observed cases. The limit of the study, and at the same time the development perspective, lies in the experimentation of real cases. More specifically, the theoretical results and general conclusions need to be validated in different port contexts, with different types of PCS. It is recalled that the trends of the functions presented in the Deterministic User Equilibrium Model section, even if they do not refer to a specific real case, are based on values of generalized cost reduction measured experimentally.
Future work will focus on the single transaction in an analytic form and as an individual contribution to estimating the whole port operation time. This approach offers the possibility of ex ante assessing the reduction of cost generated by the introduction of emerging ICT technologies and a new wave of PCS.
Future studies will be concerned with calibrating the generalized cost functions specified in relation to the decision-makers involved and the specific ICT technology (IoT, BC, ...) incorporated into the PCS.
The calibrated values could support the estimation of the Logistics Performance Index, measured at the international level to evaluate the efficiency and effectiveness of a port.
This work can be useful for researchers that can introduce the developed formalization of TSM in the port: from the stochastic equilibrium to the most advanced dynamic models (Cantarella et al., 2019); and it can be interesting for the planners and analysts, mainly port authorities, because it allows evaluating, in a unified view, the utility associated with the introduction of emerging ICT to be shared among port decision-makers. In a complementary way, it can be useful for the producers of technologies and the designers of PCSs, as it allows them to better finalize their production.
The raw data supporting the conclusions of this article will be made available by the authors without undue reservation.
FR was responsible for the conceptualization; GM performed the formal analysis; FR and GM contributed to the methodology, supervised the study, conducted the data curation and the investigation, validated the results, and wrote, reviewed, and edited the manuscript.
The authors declare that the research was conducted in the absence of any commercial or financial relationships that could be construed as a potential conflict of interest.
All claims expressed in this article are solely those of the authors and do not necessarily represent those of their affiliated organizations, or those of the publisher, the editors and the reviewers. Any product that may be evaluated in this article, or claim that may be made by its manufacturer, is not guaranteed or endorsed by the publisher.
Atzori, L., Iera, A., and Morabito, G. (2017). Understanding the Internet of Things: Definition, Potentials, and Societal Role of a Fast Evolving Paradigmfinition, Potentials, and Societal Role of a Fast Evolving Paradigm. Ad Hoc Networks. 56, 122–140. doi:10.1016/j.adhoc.2016.12.004
Ben-Akiva, M., and Lerman, S. R. (1984). Discrete Choice Analysis. Theory and Application to Travel Demand. Cambridge: MIT Press.
Caldeirinha, V., Felício, J. A., Salvador, A. S., Nabais, J., and Pinho, T. (2020). The Impact of Port Community Systems (PCS) Characteristics on Performance. Res. Transportation Econ. 80. doi:10.1016/j.retrec.2020.100818
Cantarella, G. E., and Cascetta, E. (1995). Dynamic Processes and Equilibrium in Transportation Networks: Towards a Unifying Theory. Transportation Sci. 29, 305–329. doi:10.1287/trsc.29.4.305
Cantarella, G. E., Watling, D., de Luca, S., and Di Pace, R. (2019). Dynamics and Stochasticity in Transportation Systems, Tools for Transportation Network Modelling. 1st Edition. Amsterdam, Netherlands: Elsevier.
Carlan, V., Coppens, F., Sys, C., Vanelslander, T., and Van Gastel, G. (2020). Blockchain Technology as Key Contributor to the Integration of Maritime Supply Chain? Maritime Supply Chains., 229–259. doi:10.1016/b978-0-12-818421-9.00012-4
Carlan, V., Sys, C., and Vanelslander, T. (2016). How Port Community Systems Can Contribute to Port Competitiveness: Developing a Cost-Benefit Framework. Res. Transportation Business Management. 19, 51–64. doi:10.1016/j.rtbm.2016.03.009
Cascetta, E. (2009). Transportation Systems Analysis. Models and Applications. New York: Springer-Verlag.
Castelein, B., van Duin, R., and Geerlings, H. (2019). Identifying Dominant Stakeholder Perspectives on Sustainability Issues in Reefer Transportation. A Q-Method Study in the Port of Rotterdam. Sustainability. 11 (12), 3425. doi:10.3390/su11123425
Čišić, D., Hadžić, A. P., and Tijan, E. (2009). The Economic Impact of E-Business in Seaport Systems. MIPRO 2009, 32nd International Convention on Information and Communication Technology, Electronic and Microelectronics, Proceeding Vol. V. Retrieved from: http://bib.irb.hr/prikazi-rad?rad=395814 (Accessed April 20, 2020).
Durán, C., Palominos, F., Carrasco, R., and Carrillo, E. (2021). Influence of Strategic Interrelationships and Decision-Making in Chilean Port Networks on Their Degree of Sustainability. Sustainability. 13 (7), 3959. doi:10.3390/su13073959
Galletta, A., Gattuso, D., and Musolino, G. (1999). Il porto di Gioia Tauro nel contesto Euro Mediterraneo. Analisi di scenario con il supporto di modelli di offerta di trasporto merci intermodale. Atti della XX Conferenza Italiana di Scienze Regionali. Piacenza, 5-7 October 1999. Piacenza, Italy: Università Cattolica.
Gattuso, D., Musolino, G., and Perri, V. (2005). Flussi informativi nel porto di Gioia Tauro. In Progetto REPORTS e progetto SESTANTE. D. Gattuso (a cura di). Milano: Collana Trasporti, FrancoAngeli, 167–184.
Heaver, T., Meersman, H., and Van De Voorde, E. (2010). Co-operation and Competition in International Container Transport: Strategies for Ports. Maritime Pol. Management. 28 (3), 293–305. doi:10.1080/03088830110055693
Inoue, S. (2018). Realities and Challenges of Port alliance in Japan — Ports of Kobe and Osaka. Res. Transportation Business Management. 26, 45–55. doi:10.1016/j.rtbm.2018.02.004
Martín-Alcalde, E., Saurí, S., and Ng, A. K. Y. (2016). Port-Focal Logistics and the Evolution of Port Regions in a Globalized World, in: Dynamic Shipping and Port Development in the Globalized Economy Volume 2: Emerging Trends in Ports, P. Tae-Woo Lee, and K. Cullinane (eds.). Mcmillan, Palmgrave, pp 102–127. doi:10.1057/9781137514295_5
Moros-Daza, A., Amaya-Mier, R., and Paternina-Arboleda, C. (2020). Port Community Systems: A Structured Literature Review. Transportation Res. A. 133, 27. doi:10.1016/j.tra.2019.12.021
Nuzzolo, A., and Russo, F. (1997). Modelli per l'analisi e la simulazione dei sistemi di trasporto collettivo. Milano, Italia: Editore FrancoAngeli.
Russo, F. (2001). “Trasporto Intermodale Delle Merci,” in Introduzione alla Tecnica dei Trasporti e del Traffico con Elementi di Economia dei Trasporti. Editor G. E. Cantarella (Torino (Italy): UTET).
Ortuzar, J., and Willumsen, L. G. (2001). Modelling Transport. 3rd Edition. Chichester, United Kingdom: Wiley.
Russo, F. (2005). Sistemi di trasporto merci. Approcci quantitativi per il supporto alle decisioni di pianificazione strategica tattica ed operativa a scala nazionale. FrancoAngeli.
Russo, F., and Musolino, G. (2020). Quantitative Characteristics for Port Generations: the Italian Case Study. Int. J. TDI. 4 (2), 103–112. doi:10.2495/tdi-v4-n2-103-112
The World Bank (2018). Available at: https://lpi.worldbank.org/international/global?sort=asc&order=LPI%20Rank#datatable.
UNCTAD (1999). Fourth-generation Port: Technical Note. Ports Newsletter N. 19, Prepared by UNCTAD D Secretariat. Geneva, Switzerland.
UNCTAD (1994). Port Marketing and the challenge of the Third Generation Port. Geneva, Switzerland: Trade and Development Board Committee on Shipping ad hoc Inter-government Group of Port Experts.
Keywords: port operations, emerging ICT, transportation system models, equilibrium assignment, port community systems
Citation: Russo F and Musolino G (2021) The Role of Emerging ICT in the Ports: Increasing Utilities According to Shared Decisions. Front. Future Transp. 2:722812. doi: 10.3389/ffutr.2021.722812
Received: 09 June 2021; Accepted: 29 July 2021;
Published: 27 August 2021.
Edited by:
Edwin Van Hassel, University of Antwerp, BelgiumReviewed by:
Valentin Carlan, University of Antwerp, BelgiumCopyright © 2021 Russo and Musolino. This is an open-access article distributed under the terms of the Creative Commons Attribution License (CC BY). The use, distribution or reproduction in other forums is permitted, provided the original author(s) and the copyright owner(s) are credited and that the original publication in this journal is cited, in accordance with accepted academic practice. No use, distribution or reproduction is permitted which does not comply with these terms.
*Correspondence: Giuseppe Musolino, Z2l1c2VwcGUubXVzb2xpbm9AdW5pcmMuaXQ=
Disclaimer: All claims expressed in this article are solely those of the authors and do not necessarily represent those of their affiliated organizations, or those of the publisher, the editors and the reviewers. Any product that may be evaluated in this article or claim that may be made by its manufacturer is not guaranteed or endorsed by the publisher.
Research integrity at Frontiers
Learn more about the work of our research integrity team to safeguard the quality of each article we publish.