- 1TS2-LESCOT, IFSTTAR, Univ Gustave Eiffel, Univ Lyon, Lyon, France
- 2Nantes Laboratory of Digital Sciences (LS2N), University of Nantes, Nantes, France
- 3Study of Cognitive Mechanisms Laboratory, Lyon Lumière University, Lyon, France
- 4TS2, IFSTTAR, Univ Gustave Eiffel, Univ Lyon, Lyon, France
Driving automation has become a trending topic over the past decade, as recent technical and technological improvements have created hope for a possible short-term release of partially automated vehicles. Several research teams have been exploring driver performance during control transitions performed under highly automated driving (i.e., while resuming manual driving, when facing a critical situation for instance). In this paper, we present a state of the art of studies dealing with control transitions as well as the concept of non-driving-related task (NDRT) engagement. More specifically, we aim to provide a global view on how task engagement is investigated in the literature. Two main utilisations of task engagement emerged from our literature review: its manipulation as independent variable to vary the driver’s engagement state before a control transition, and its measurement as dependent variable to compare its variation to driving behaviour variables during a control transition. Furthermore, we propose a new perspective on control transition, which was so far studied through a techno-centric approach; research works were indeed designed in function of the system state. Our article suggests a more cognitive-centred view by taking in account the evolution of engagement mechanisms along control transition stages. Finally, we provide a categorisation of engagement mechanisms’ variables involved during these different stages, with a view to facilitate future investigations on the driver’s engagement state during this crucial phase of highly automated driving.
Introduction
Recent technical and technological progresses have set the scene for the introduction of driving automation systems. Automated vehicles, i.e., vehicles without active drivers, may appear as the most critical challenge. As they have been studied since the advent of automation in aeronautics, the impacts of delegation of control, system supervision, and control transitions, are now investigated for car drivers’ behaviour (Bainbridge, 1987; Endsley and Kaber, 1999). In the near future, drivers will be able to act like passengers, i.e., to focus on a completely different activity than road management, leading to an evolution of the cognitive functions involved (e.g., allocation of attention). Considered in the past as distractions and the potential cause of numerous road accidents, activities realised on board during automation phases, called non-driving-related tasks (NDRTs), are now studied from a different point of view. Stanton and Young first advocated the benefits of staying stimulated when automation on board increases, as the amount of attentional resources generated for a given task is considered “available” for a potential new task to process (Stanton and Young, 1998). They later concluded that the phenomenon could be the same in the case of highly automated driving (Young and Stanton, 2002). From this point of view, NDRTs could be seen as a tool to maintain drivers in a cognitive state which would allow them to takeover control of the vehicle. However, other researchers do not share this opinion. In fact, several studies found that being involved in a NDRT significantly led to worse takeover quality (Neubauer et al., 2012; Naujoks et al., 2016; Zeeb et al., 2016; Eriksson and Stanton, 2017; Mok et al., 2017). Yet, other research works showed that most drivers decided to engage in a NDRT when they could under automated driving (Carsten et al., 2012; Llaneras et al., 2013). Assuming that some activities will become very popular on board, such as the use of electronic devices (Dingus et al., 2006), exploring their impact on drivers’ attentional state, and capacity to resume manual driving when necessary, has become very common over the last decade. The question “how much time does a driver need to safely take over?” has already been widely addressed. In their large and complete meta-analysis, Zhang et al. discussed the critical issues raised by automation-to-manual driving transition throughout time to regain control (Zhang et al., 2019). Significant decrease of performance was observed compared to control groups (Merat et al., 2012; Beukel and Voort, 2013). Other studies showed that, in situations of control transitions, experienced drivers were quicker to achieve road situation elements than novices (Wright et al., 2016). Several works concluded that simulator variables were robust measures to assess manual driving performance (Wester et al., 2008; Melcher et al., 2015; Gold et al., 2016). A few studies on general transitions from system to driver showed that the higher the level of automation, the longer the drivers’ reaction times (Gold et al., 2013; Strand, 2014). The strong interest of researchers for control transition between a driver and an automated driving system is legitimate. It indeed involves complex mechanisms (as we will see afterwards), taking place in complex situations. The legislation around highly automated vehicle is being discussed and will have to determine, among other issues, whether it is a good thing or not to do activities during the autonomous phases. Are these activities beneficial or harmful? Should they be prioritised? This ongoing challenge is addressed by several research teams from the field of highly automated vehicles, in different ways around the world. Our review puts together these approaches and offers a global view on how transition of control should be addressed by taking into account the human factor.
Takeover design, time to takeover, steering wheel angle, and standard deviation of lateral or longitudinal position have been widely used to study control transition. Their importance is indisputable in the process of understanding how automation modifies driving behaviour, yet human factors should be addressed with the same interest. NDRTs have been less investigated through their impact on drivers’ cognitive state. Indeed, they have often been defined as secondary tasks, or even conditions to induce different driver states prior to a takeover request. Even though task engagement mechanisms seem to be the most involved cognitive functions during on-board activities, only a few studies explored them for the driving context (Park et al., 2019), despite their major implication on drivers’ attentional state and availability to ensure safe driving. The few studies which examined the impact of NDRTs on driver performance have increasingly used the concept of task engagement. Whether it is considered as the result of the provision of attentional resources (Young, 2010; Lee, 2014), workload level (Ko and Ji, 2018), task difficulty (Pohl and Murray-Smith, 2013), or as a combination of key variables like energy arousal (Matthews et al., 2010), the study of task engagement seems essential to understand the mechanisms involved in the complex task of control transition. Control transition is indeed a complex situation, and contains several phases to be distinguished (Naujoks et al., 2018). Soon, drivers will be able to delegate the driving situation handling to a system and concentrate on other activities on board. They become “drivengers”, a term to evocate the switch between driver and passenger states (Reilhac et al., 2015). The “drivengers” can engage in NDRTs, from which they will then have to disengage while re-engaging in the driving situation. The importance of studying engagement mechanisms during transitions between tasks was previously highlighted by various researchers. The existence of an optimal state of engagement in a task before switching to another one has been theorised. In the case of an optimal task engagement state, a transition to another task would be easy and would not degrade performance (Pashler et al., 2001). Marberger et al. tackled this issue through the concept of “driver availability”, within the framework of control transition (Marberger et al., 2018). Indeed, their model attempted to discern the phases of transitions, notably by considering the driver’s cognitive state. A driver state transition is mentioned, distinguished from the system state and from the two surrounding phases (i.e., NDRT and manual driving). The need to differentiate the driver’s cognitive states as stages of a transition progress was also put forward by the multitasking model based on the threaded cognition theory (Salvucci and Taatgen, 2008; Salvucci et al., 2009). They defined the concepts of “interruption lag” and “resumption lag”, constituting the transient state of the driver between two tasks. It therefore appeared necessary to focus more precisely on these stages of the control transition. The objective of this state of the art is to provide a clearer view on how engagement in NDRT is manipulated and/or measured in studies on vehicle control transition. We present how NDRTs were used by research teams who aimed to observe their impact on the driver state during vehicle control transition, and how this effect was measured in terms of engagement in both NDRT and driving task. Furthermore, existing literature currently proposes techno-centric approaches on control transitions (Naujoks et al., 2018), whereas our article suggests a more cognitive-centred view. Finally, we provide a categorisation of engagement mechanisms’ variables involved during the different stages of control transition.
To this end, several articles were collected via Google Scholar searches and via a list of references from a previous literature review (Naujoks et al., 2018). The covered publication period was restricted to the last decade (i.e., between 2010 and 2020). The screening process was based on several inclusion criteria. First, attention, workload, engagement, or disengagement concepts had to be clearly mentioned as a variable. Selected studies also had to provide enough accurate information on the procedure dedicated to instructions and design for the NDRTs. Being centred on NDRT and providing cognitive explanation was also necessary for inclusion (e.g., studies focusing on NDRT and comparing different age groups were included). Finally, NDRTs had to be ecological (i.e., close to most likely chosen NDRTs in real life), to avoid the existing gap between standardised and ecological tasks (Stanton and Young, 2003; Shinar et al., 2005; Zeeb et al., 2016). Automated driving systems implemented and tested in these studies had to be of SAE level 3 or 4 (SAE On-Road Automated Vehicle Standards Committee, 2014). After a screening procedure and duplicate removal, twenty-three entries were thoroughly studied, resulting in the dismissal of seven entries due to incompatibility.
In this article, a specific approach of engagement mechanisms in the context of control transition under highly automated driving (HAD) was adopted. Indeed, after literature inspection, it appeared that engagement in NDRT was either considered as a manipulated variable in order to assess its consequences, or as a measured variable with a view to quantify, describe, and interpret the process of transition from NDRT to manual control in the advent of a takeover request. In the studied experimental protocols, engagement in NDRT was sometimes considered as an independent variable, or as a dependent variable. On the basis of this observation, this review examines the presented literature depending on how engagement was considered. The Manipulation of Engagement firstly considers articles that manipulated engagement with a task-driven approach, and then with a cognition-driven approach; in The Evaluation of Task Engagement are scrutinised articles measuring engagement through performance, and then through subjective assessments. Table 1 offers a global view of the repartition applied to the reviewed paper corpus. The literature is finally discussed in order to provide a synthetic view of the matter in Discussion, where a resulting more complete way of approaching the study of engagement in NDRT during automated driving is introduced.
The Manipulation of Engagement
This first section includes and details studies which declared a manipulation of task engagement or engagement factors through non-driving related tasks. To explain their methodological approach to vary drivers’ engagement in NDRTs, two main perspectives were observed in the reviewed papers. One was focused on the task, in which case, the research teams justified the creation of different drivers’ engagement levels as independent variables by the manipulation of the task. Trough the manipulation of the task properties, different levels of engagement were created. This task-driven approach is presented in Task-Driven Approach, which describes works where NDRTs were established in function of their type, or of an expected difficulty level. The other approach identified in the reviewed papers was defined on cognitive process manipulation. Indeed, cognitive theories on engagement mechanisms were used to create experimental designs varying drivers’ engagement in NDRTs. Cognition-Driven Approach deals with these studies. Figure 1 illustrates how the repartition of the studies was made. This tree-figure contains two main parts: the left branch presents the criteria used to organise Task-Driven Approach, describing the papers which used a task-driven approach, while the right branch illustrates the criteria related to Cognition-Driven Approach. Table 2 provides a detailed overview of the content extracted from the articles that manipulated engagement.
Task-Driven Approach
Miller et al., identified task engagement as the new cognitive mechanism to investigate in the context of highly automated driving, instead of distraction and secondary tasks in the case of manual driving (Miller et al., 2015). To do this, they designed a simulator study where participants had to experienced three different NDRTs during automated drives, i.e., reading a book extract on a tablet, watching a video on a tablet, and supervising the advanced driver-assistance system (ADAS). The NDRT type was chosen in function of their induced level of driver: the authors expected a low level of engagement when supervising the ADAS, associated with a high level of drowsiness; whereas they qualified the two other tasks as “stimulating”, and yielding more engagement but less drowsiness. After each automated drive, a takeover was necessary. A post-drive questionnaire questioning participants about their engagement in each NDRT was mentioned in the protocol description, yet no information was given in the results. Reaction times when a takeover request appeared (i.e., time to press a button, and time to initiate first manoeuvre), and the minimum distance to a leading object were also recorded.
Last year, Wu et al. released their study on the effects of NDRT engagement on drowsiness and takeover performance (Wu et al., 2020). Three age groups were invited to sit in a driving simulator and to engage in diverse tasks: automated driving without NDRT (i.e., control group), video watching, or alternating video watching with playing Tetris. They were asked to stay in their motorway lane. Suddenly, a stopped van appeared in front of the ego vehicle, and a takeover request was issued. Before and after each scenario, participants were asked to rate their drowsiness using the Karolinska sleepiness scale (Shahid et al., 2012). Their eye blink duration was recorded thanks to an electrooculogram, and the TimeSteer (i.e., time until steering) and sdSteer (i.e., standard deviation of the steering wheel angle) were collected. sdSteer allowed to measure manoeuvring smoothness. Differences between the ratings of drowsiness before and after each scenario was calculated; statistical analyses revealed a significantly smaller value for younger drivers between NDRT conditions and no NDRT. For middle-aged drivers, only the alternating task led to a smaller difference compared to the control condition. Finally, no significant difference was found between the tasks for older drivers. Eye blink durations generated similar results. TimeSteer values were not statistically significant among task conditions for younger drivers, however, the latter tended to react faster when engaged in a NDRT. Opposite results were found for the middle-aged group. For older drivers, there was no difference between the control and video-watching conditions, whereas alternating between watching and playing yielded slower reactions. Differences in sdSteer were only revealed for older drivers, with a poorer stability under the swap condition. In general, younger drivers showed faster reactions but less stable steering under NDRT conditions; middle-aged drivers had slower reactions under both NDRT conditions; and older drivers had long reaction times and poor stability under the demanding NDRT. The authors concluded that NDRT engagement had a positive influence for younger drivers.
Lately, Yoon and Ji examined the impact of three different NDRTs, i.e., interacting with an entertainment console by continuously searching for a radio station, interacting with a smartphone by playing “1–50”, and watching a video where they had to count the number of balls passed (Yoon and Ji, 2019). The participants’ inner spirit of competition was highly invoked as they had to obtain the best scores possible for the first two tasks, and to inform the experimenter about the number of passes. Workload was assessed using the NASA-TLX questionnaire (Hart and Staveland, 1988); and the gaze-on time (i.e., before first gaze through windshield after takeover request), fixation time (i.e., before first gaze on the road), hands-on time (i.e., before grasping steering wheel), and takeover time (i.e., to resume manual control) were calculated to quantify takeover performance. Visual performance data was collected using an eye-tracker. Each participant was required to complete three sessions for the experiment. Within each session, they were asked to perform takeover at three distinct points, for non-emergency situations: participants had to change lanes as the number of lanes changed from three to four, or from four to three. Data analysis was conducted in three steps, i.e., subjective evaluation, objective evaluation, and correlation between both. Statistical analyses showed that each task had a significant effect on the workload: interaction with the entertainment console was scored significantly lower than the two other tasks, notably in terms of mental demand and effort. As for objective measurements, an ANOVA demonstrated significant differences between tasks for the gaze-on, fixation, and takeover times; however, there was no impact on the hands-on time. A negative correlation was found between workload and takeover performance. As a summary, the different tasks had statistically significant effects on manual takeover.
Borojeni et al. presented their work, with two main research questions in mind: one linked to the type of automation, and one to the effects of engagement (Borojeni et al., 2018). The participants had to engage in a reading span task with unconnected sentences, and were required to determine which ones made sense. They were also asked to recall a word after each sentence, and later to type them in the correct serial order (three words for the low-level condition, five under the high-level one). Two takeover requests were studied: an information one, warning about an upcoming takeover, and a decision one, priming with appropriate manoeuvre. Requests were made to the participants for specific events, e.g., when the lead car suddenly braked. Depending on the traffic situation, drivers had to steer left, change lane and overtake, or to brake and overtake. Reaction time (i.e., between request and action), time to collision (i.e., between action and collision with lead car), number of collisions, of alternative manoeuvres (i.e., when participants steered instead of braking and vice versa), gaze behaviour (i.e., frequency and duration), and performance in NDRT were measured. Workload was rated thanks to the NASA task load index (NASA-TLX) questionnaire (Hart and Staveland, 1988). An analysis of variance (ANOVA) showed a significant effect of automation type (i.e., information vs. decision) on reaction time: takeover was performed faster under the decision condition. However, no significant effect of engagement level was found on reaction time. Similar results were observed for the time to collision. Statistical analyses of the subjective data demonstrated no effect of the automation type, but a significant impact of the NDRT engagement on the workload. Collected eye-tracking data showed no differences between experimental conditions. Borojeni et al. concluded their article by suggesting a few interesting points for the design of future takeover requests.
In a simulator study, Wandtner et al. investigated NDRTs’ engagement mechanisms, also using different task difficulty levels (Wandtner et al., 2018c). In addition, they studied the effects of a predictive human-machine interface (HMI) on participants’ behaviour during control transitions. Twenty participants drove on a simulated track, alternating sections of manual and automated driving, while a visual-manual texting task was offered to them on a tablet. More precisely, they had to transcribe a given text made with short or long sentences (i.e., thirty vs. ninety characters). One group was encouraged to achieve as many tasks as possible while the other had no particular instruction. An HMI was located on the centre console. It continually displayed the test progression in real-time gauges with more or less information, depending on the HMI condition (i.e., predictive vs. basic HMI). The predictive HMI gave much more precise information than the basic one about the progression of each section (i.e., automated or manual). The same takeover request was presented at the end of each automated phases when a sharp bend appeared to the left side of the road. To measure task engagement, the number of tasks accepted during both manual and automated driving was counted. Typical simulator aspects, like time-response variables or quality measures (i.e., time until hands were back at the steering wheel, time until system deactivation or variability of lateral position, lane exceedances, and maximum accelerations), were recorded and analysed. Results showed that, even in the case of imminent takeover, several participants started a new NDRT. NDRT engagement was correlated with significantly poorer performances during takeover. A phenomenon of task perseverance was observed during takeover in most cases. Under the predictive HMI condition, where self-regulation processes were encouraged, takeover performance was better. For instance, during regaining control of the vehicle, participants better controlled lateral parameters.
In 2017, Zeeb et al. studied the impact of NDRTs on critical takeover situations (Zeeb et al., 2017). Participants were asked to engage in two tasks i.e., reading (“easy task”), and proofreading (“difficult task”). One group of participants had the tablet mounted, whereas another group had to hold it to analyse the effects of manual task load. Finally, a control group (i.e., not engaged in any NDRT) was involved. Two distinct takeover situations were presented: a lateral one (wind gust), where participants had to react with a steering intervention; and a longitudinal one, where they had to brake to avoid a collision. After each situation, the participants were asked to score their alertness, and how mentally demanding were the minutes prior to the takeover. Time to eyes on (i.e., before first gaze on the road), time to hands on (i.e., before first contact with the wheel), and time before intervention were measured. Furthermore, takeover quality was recorded. Data analysis showed a significant effect of the task difficulty on alertness and mental load ratings: the control group rated both values lower than the other groups. No difference in alertness was found between easy and difficult tasks; however, difficult task yielded higher mental load. The tablet position had a significant influence on the reaction times, which were longer for the handheld group. Differences were also found in terms of takeover quality, with the handheld group having larger lane deviation and shorter time to lane crossing. The cognitive task load also had an influence on times to hands on and to first steering, but not on time to eyes on; yet there were no differences between easy and difficult tasks. To conclude, manual task load had effects on reaction times and takeover quality for both interventions, whereas cognitive task load had no influence on takeover performance.
Cognition-Driven Approach
In a first study, Wandtner et al. used Wickens’ MRT (Wickens, 2002) to analyse the influence of task modality and cognitive workload on takeover (Wandtner et al., 2018a). Fourteen participants experienced six automated driving scenarios, each followed by a critical takeover situation. During automation phases, participants conducted NDRTs on a handheld tablet; all of them experienced each task type, as described below. To explore the impact of NDRTs, the authors applied a mix of task modality (i.e., visual, auditory, and manual) and cognitive workload (i.e., medium vs. high level) factors. The visual-manual condition was equivalent to previous study (i.e., Wandtner et al., 2018b). The visual-manual with high workload condition had, in addition, the objective to “alphabetise” each word that contained errors (e.g., “deirv” had to become “drive”). The authors assumed that such variations could affect takeover performance. Classical metrics of drivers’ takeover performance, concerning reaction time and quality takeover, were recorded and analysed. Timing aspects were assessed using the time from takeover request until both hands were on the steering wheel, and from the brake-response time. Reaction times showed an influence of the NDRT type, with a significantly longer hands-on time for the visual-manual task than for the auditory-vocal task. A significant effect of a high workload task was observed on the time to brake.
Wandtner et al. focused on the influence of NDRTs on drivers’ engagement and takeover performance in another study (Wandtner et al., 2018b). Thirty participants were invited to experience five scenarios ending by a takeover. All takeover situations were considered critical as the participants had no possibility to anticipate (i.e., situations were hidden by scene objects). During automated phases, participants were asked to engage in NDRTs. Based on Wickens’ multiple resource theory (MRT) (Wickens, 2002), cognitive modalities were manipulated to create different demand levels. For one group, tasks were locked out when takeover requests appeared, whereas no such condition was applied to the other group, who could continue their task. The NDRTs were exercise-type, based on the same goal, i.e., to transcribe sentences. For the auditory-vocal condition, participants had to listen to sentences and repeat them aloud. Under the visual-vocal condition, participants had to repeat sentences presented on the tablet mounted on the centre console. For the visual-manual condition, they were requested to read sentences displayed on the tablet and to type them. This condition was split into two versions depending on where the tablet was located (i.e., on the centre console or in participants’ hands). Sentences followed each other without any break to ensure participants stayed engaged, and the latter were encouraged to complete as many tasks as possible with a monetary incentive. All participants experienced each of the four task types, as well as a control sequence (i.e., without NDRT). Classical dependent variables were used to evaluate takeover performance: reaction time was measured through hands-on and brake-response times (i.e., from takeover request until the brake pedal was sunk). A significant effect of task modality was found on hands-on time: when using hands to hold the tablet, the time to regain control was higher. No significant effect but a tendency on brake-response time was found. A significant effect of NDRT condition was found on the time to collision, with the visual condition associated with shorter times, whereas only a slight tendency was observed on the number of collisions.
Three years ago, Roche et al. explored the impact of NDRT cognitive modality on drivers’ behaviour (Roche et al., 2019). Six automated phases, which always ended by a takeover request, were tested in their experiment. Participants had to regain control before the beginning of a construction site that prevented the automated system from continuing. Two takeover request designs were tested on two distinct groups; one was an auditory alert, whereas the other one was combined with a visual message. During automated phases, participants were instructed to play a game consisting in a question-answer challenge, which was designed in two versions to vary involved cognitive dimensions. More specifically, the game was either offered via an auditory mode (i.e., the experimenter read questions aloud, and participants were asked to answer aloud), or via a visual mode (i.e., questions were presented on a tablet, and participants had to tap the answers). In this study, the NDRTs were presented as distractions for the drivers. It was expected that the multiplication of stimuli which came from the same cognitive modality, especially in visual cases will lead to poorer performance, in line with Wickens’ multiple resources theory (Wicken, 2002). Classical driving data were recorded from the simulator as the takeover time, some lateral and longitudinal variables (i.e., minimum acceleration, standard definition of lateral position (SDLP), and steering wheel angle), and time to collision. Fixation durations on the road were also recorded thanks to an eye-tracker. Results showed an effect of NDRT modality on SDLP and gaze behaviour: participants looked less at the road when NDRT required visual effort, compared to auditory NDRT. From the takeover request to its end, corresponding to the beginning of a construction site, SDLP appeared to be larger after a visual NDRT than an auditory NDRT.
Befelein et al. investigated eight takeover situations on the highway to evaluate the impact of NDRTs’ motivational aspects and interruption effort on takeover performance (Befelein et al., 2018). Emergency takeover requests were examined: the ego-vehicle was following a lead vehicle when, suddenly, a broken-down vehicle appeared. A visual and auditory takeover request was consequently issued to avoid collision. Each participant was required to complete two distinct blocks; one where the NDRT led to external incentive, and one where it was only a hobby. Each block was composed of two situations with high interruption effort, and two with low interruption effort, as further defined. The authors chose the video game Tetris as NDRT. For the high interruption effort, participants were asked to pause the game, put the tablet in a box, and place a lid on that box; whereas, for the low effort, they only had to pause and put the device aside. Reaction times, i.e., time between request and first driver reaction, were measured for every situation. Furthermore, participants were asked to rate several items on discrete Likert scales, including the situation criticality, their takeover performance, and their motivation. Subjective ratings showed significant differences between low and high interruption efforts: criticality was rated higher, and takeover performance was scored lower in situations with high interruption effort. No statistically significant differences were found between both motivation conditions (i.e., with or without external rewards), however, the performance condition was considered more dangerous than the hobby condition. As for objective measurements, participants reacted significantly slower in situations with high interruption effort. There were no significant differences between motivation conditions. Befelein et al. concluded by highlighting the fact that task interruption effort requires further attention, as it has a critical impact on takeover reaction times.
Simultaneously, Ko and Ji published their own work in two parts, aiming to analyse flow experience, mental workload, and reaction time under automated driving (Ko and Ji, 2018). Their first experiment is detailed in Subjective Ratings. In the second experiment, participants were asked to sit in a driving simulator and to perform two different NDRTs, i.e., watching a video and reading an article on a tablet, under three levels: a low-demand level (i.e., boredom condition), a mid-skill level (i.e., fit condition), and a high-demand level (i.e., anxiety condition). At given points, the participants were informed through an auditory sound to resume manual driving. Their gaze behaviour was recorded using eye-tracking glasses. Their reaction time, consisting of four variables, i.e., gaze-on time, road-fixation time, hands-on time, and takeover time, was registered. Participants were required to fill in two subjective questionnaires after completing the scenarios: a flow short scale to measure flow experience (Engeser and Rheinberg, 2008), and the NASA-TLX questionnaire (Hart and Staveland, 1988). Statistical analyses showed a significant difference in the perceived difficulty of each task, with video watching perceived easier. The anxiety condition required a higher demand level than the fit condition, which required a higher one than the boredom condition. Each task had a significant impact on the flow experience, with a difference between the fit condition and the other two conditions (i.e., boredom and anxiety). Mental workload was rated differently for each task, with the reading task rated higher than video watching. Objective measurements led to complementary results: significant effects of the experimental condition were found for all the aspects of the reaction times. More precisely, there was a significant difference between the fit condition and the other conditions, but no difference between boredom and anxiety conditions. To conclude, the authors claimed that flow experience, mental workload, and reaction time depended on the experimental condition.
Last year, Tanshi and Söffker conducted a driving simulator study to better understand human behaviours while resuming manual driving (Tanshi and Söffker, 2019). They defined four distinct scenarios; each scenario presenting two different complexity levels (i.e., easy vs. difficult, depending on the ego speed). The first scenario represented a fixed obstacle on the highway, not visible due to fog. For the second scenario, also taking place on the highway, a slow vehicle ahead had to be overtaken. For the third one, participants were required to exit the highway. Finally, the fourth scenario corresponded to a right turn on a four-way intersection on a country road. Before taking over from automated to manual driving, the participants were invited to perform three NDRTs: reading, proofreading, and proofreading out loud. After takeover, participants had to fill in the situation awareness rating technique (Taylor, 1990), and NASA-TLX (Hart and Staveland, 1988) questionnaires. Moreover, their takeover time was recorded. Analyses of the results showed that takeover time under difficult complexity level was higher for the first and third scenarios, but not for the second and fourth. This can be explained by the fact that dynamics were different. As far as situation awareness and workload are concerned, they appeared to be higher for the easy level for all scenarios but the second one. In general, takeover time for the reading task was higher than for proofreading and proofreading out loud; however, this was due to a learning effect, as reading was linked to the first scenario presented to the participants. Thanks to these results, Tanshi and Söffker developed a model defining the dependencies of a situation complexity on takeover time.
The Evaluation of Task Engagement
In this section, studies which measured task engagement as a dependent variable are described. Two main ways to measure NDRT engagement was observed. Task-Related Measurements represents works where engagement was measured through task management during automated driving and control transition., whereas Subjective Ratings concerns articles where task engagement measurement was made through participants’ subjective ratings. Figure 2 recapitulates this categorisation. The figure is composed of two parts: the left branch describes the criteria used to organise Task-Related Measurements; dealing with the papers which used task-related measurements to evaluate NDRT engagement, whereas the right branch represents Subjective Ratings which reviews the use of subjective ratings. Table 3 further details the articles’ content relating to the evaluation and measurement of task engagement.
Task-Related Measurements
To analyse some quality aspects to further investigate drivers’ behaviour, Wandtner et al. used multiple dependent variables in their simulator study described in Task-Driven Approach (Wandtner et al., 2018a). The number of collisions, maximum longitudinal decelerations, takeover criticality, and subjective workload were recorded. Workload was used to assess engagement, whereas disengagement was measured by observing how drivers dealt with NDRTs in takeover situations. In fact, NDRT disengagement was evaluated using a behaviour classification regarding the interruption strategy and more precisely the task manipulation from the takeover request until the end of the takeover situation. Behaviours varied depending on the task, more especially on the task modality and time-budget.
Then, to continue the exploration of engagement and disengagement measurements, Wandtner et al. defined new dependent variables (Wandtner et al., 2018b). In their second study, detailed in Cognition-Driven Approach, the authors observed how participants dealt with the tablet when a takeover request appeared. Only five participants dropped it off the passenger seat, whereas twelve put it on their thighs, and thirteen kept it in one hand while taking back control of the vehicle with the other hand. The authors also coded the behaviour series. They noted that more participants braked before putting their hands on the steering wheel under the visual–manual handheld condition than under the other conditions. The number of collisions, minimum time to collision, and maximum decelerations were investigated as quality measures. The way participants from the no-lockout group (i.e., when the task was not blocked when a takeover request appeared) dealt with the task was also examined. Three different behaviours were observed, i.e., an immediate interruption and no task resumption, an interruption with a resumption of the task after responding to the request, and a continuation of the task even during takeover and manual driving. Along with Wickens’ theory (Wickens, 2002), the more the task was demanding, the more it was immediately abandoned; auditory-vocal tasks were more often continued than visual-manual-handled ones. Subjective workload ratings indicated a higher level for visual-manual tasks than for different vocal tasks. Visual-vocal conditions were also rated significantly higher than auditory-vocal conditions.
Concerned by age differences in driving and on-board behaviours, Clark et al. explored this question through a simulator study (Clark et al., 2017). Two groups of participants (younger vs. older) drove on experienced three driving sessions, each with four transitions of control, on a simulated highway track. A takeover was requested when entering a construction zone. During automated driving, they were free to engage in any NDRT as they would do in real life. The NDRTs observed were: reading, reaching an object, grooming, using an electronic device, playing a horse videogame, talking to other people (i.e., experimenters), talking to self, listening to music, and looking at the road scene. The number of occurrences and the total duration of each NDRT recorded through an eye-tracker was used by the authors to distinguish participants: a “low activity” and a “high activity” groups were identified. The level of engagement in NDRT was associated with this classification. In fact, participants who had a high level of off-road gaze occurrences and duration was considered as highly engaged in the NDRT while the opposite was classified in the low engaged participants, corresponding to the “low activity” group. Regarding the activities observed, the most popular in all groups was to look at the road environment. Apart from this activity, younger participants principally used their electronic device or did some kind of grooming, whereas older drivers preferred to talk to the experimenters. Younger participants in the high activity group spent up five times more in doing a NDRT than those in the low activity group, and more than ten times more than older drivers. Driving data recorded were analysed in function of the different groups (i.e., younger vs. older, and low activity vs. high activity). Speed, steering wheel angle, response time from notification to driver’s first reaction, lateral lane position, throttle, and brake input were collected. The younger and high activity group had the highest speed after a takeover. No significant differences were found between the older high and low activity groups, except a tendency in braking. In fact, older drivers who engaged the most in NDRT tended to brake harder during a transition of control.
Five years ago, Mok et al. investigated the effects of distraction during transitions of control (Mok et al., 2015). They observed participants perform a regain of control after an automated driving session while they had to watch a video, presented as a passive distraction. Three levels of time were tested for transitions, i.e., two, five, and eight seconds. Each participant experienced one of these time conditions. During the automated drive, participants successively watched two videos on a tablet. They had no specific instructions about its manipulation during transition of control. To measure participant engagement in the task, unexpected items were placed on road sides (e.g., a giant gorilla). In a post-simulation questionnaire, participants were asked to recall what they saw. Their performance to identify the items was used to evaluate task engagement: a participant who missed all the items was considered as highly engaged in the task. Driving behavioural data were recorded, including standard deviation of road offset (i.e., distance from the centreline of the road), standard deviation of steering wheel position, and deviation from a lane in curve. The main results concerned the minimum time to correctly regain control after a phase of automation. The authors noted that their results were close to some from a previous research where participants were not given any distraction during automation (Mok et al., 2015). Focusing on the transition phase and the driver needs at this moment, Mok et al. did not neglect the importance of driver cognitive state and addressed it through the question of task engagement and disengagement (Mok et al., 2015).
In their experiment described in Task-Driven Approach, Wandtner et al. measured task engagement and disengagement through different variables (Wandtner et al., 2018c). NDRTs were offered one after another during manual and automated driving. The number of accepted tasks during each session was recorded to assess drivers’ engagement level in NDRT. The authors considered that participants who rejected tasks were more engaged in road supervision than in the NDRTs. Task interruption management was used to evaluate disengagement from the task when a takeover request appeared; disengagement was consequently considered higher when a task was cancelled. Such variables, related to task engagement and disengagement, were used to interpret takeover performance. Results showed a decrease of the performance under highly task engagement. Regarding disengagement, results showed a persistence in the task during takeover. The authors shaded this finding by the effect of money incentive and the simulated context that probably led to prioritise the task.
Miller et al. processed driver’s eye and face behaviours to analyse drowsiness which is, according to their approach, negatively correlated to task engagement level (Miller et al., 2015). In their simulator study described in Task-Driven Approach, they coded the number of yawning and eye-blinking duration, which were identified as markers of drowsiness. The results showed a significant difference of drowsiness markers between the supervising ADAS task and the two others (i.e., reading a book extract and watching a video on a tablet). Indeed, supervising ADAS led to much more eye closure and yawn, which was consistent with the preliminary hypothesis. No influence of the NDRT type was found in the case of critical control transition (i.e., when the takeover request appeared 5 s before the automated system deactivation) on reaction time or minimum headway distance.
In their experiment described in Cognition-Driven Approach, Roche et al. recorded fixation durations on the road thanks to an eye-tracker. This variable was considered a robust indicator to measure allocation of attention resources. Results showed that participants’ fixation durations decreased along a session. Visual NDRT was associated to fewer gazes on the road than the auditory NDRT. Results also showed that even after the TOR, there was still a difference on gaze behaviour in function of the NDRT modality. Indeed, after the TOR, when participants were engaged in a visual NDRT, their fixation duration to the road is still poorer than the auditory NDRT condition. Finally, the takeover request condition (auditory vs. visual-auditory) did not had an effect on fixation duration.
Subjective Ratings
Zeeb et al. investigated the effects of NDRTs on drivers’ distraction with four driving sessions ending by a takeover control after a phase of automation on a simulated highway (Zeeb et al., 2016). Participants were instructed that the system would manage the entire vehicle function as long as it could stay on the same road lane; meaning that, when a lane change was required, the system would request drivers to take over. Among the four sessions, two ended by a critical scenario, and two by a non-critical situation. Two different groups of participants were defined, i.e., one without NDRT (control group), and another with the instruction to perform predefined NDRTs. The latter were asked to write emails, read news, and watch videos. An extra NDRT was used to complete automation phases for two longer scenarios, i.e., listening to music. To assess drivers’ response, time from takeover request to first gaze at the road, to drivers’ hands on the steering wheel, and to deactivate the system were recorded. These time responses were considered as indicators of drivers’ distraction. No significant differences were found between distraction conditions on the times to hands on and to deactivate the system. Deviation from lane centre and lateral acceleration were used as takeover quality measures: video watching and news reading resulted in a larger deviation from lane centre, and news reading was also associated with larger lateral acceleration. Participants were invited to associate a subjective level of distraction (i.e., low, medium, high) for each NDRT. Results indicated that writing emails was the more distracting task, followed by reading news and video watching, which was the opposite of what was expected from the takeover quality results.
Before testing the impact of NDRTs on driving performance in their second experiment presented in Cognition-Driven Approach, Ko and Ji studied the differences between NDRT designs, especially their effects on attentional engagement factors (Ko and Ji, 2018). They asked participants to sit in a driving simulator and to perform different tasks without any driving simulation running. Their aim was to examine whether differences appeared between each task and each condition on perceived difficulty, rated workload, and flow experience. Participants performed two tasks, i.e., watching a video and reading an article, under three conditions (boredom, flow, and anxiety). A secondary two-back task was added to vary and control mental workload. NDRTs were performed on a tablet placed on the right side of the steering wheel. After each task, participants filled in the NASA-TLX questionnaire (Hart and Staveland, 1988), and the Flow Short Scale (FKS) which measures the perceived demand level of a task (Engeser and Rheinberg, 2008). Main results indicated that perceived difficulty was significantly different between each task and each condition. Reading task was rated higher than video watching, and the perceived demand level increased from boredom to anxiety. Flow experience provided a significant effect between experimental condition and task type, with the best flow experience under fit condition. Again, a significant effect was found on the mental workload for each task type and experimental condition. The watching task was rated lower significantly than the reading task, and it increased with the experimental condition from boredom to anxiety.
A relatively new study conducted by Lee et al. investigated the effects of the NDRT type on takeover quality after automated driving (Lee et al., 2020). During automated driving, the participants experienced nine NDRTs (i.e., conversing with a passenger, listening to music, talking to their phone (handheld), watching a video on the centre console, reading a book, texting on a smartphone, operating the in-vehicle information system, playing games on a phone, and holding and drinking a beverage). A categorisation of NDRTs (i.e., two physical attributes, two cognitive attributes, and one visual attribute) was determined to analyse the effects of the NDRT type. Physical attributes referred to the place where the NDRT was held and to the number of hands required. Cognitive attributes referred to the dimensions used to perform the NDRT and to the cognitive load declared. Finally, the visual attribute corresponded to the video-coded gaze position during NDRT. Cognitive load was used as a factor of NDRT differentiation. Classical takeover quality measures, like longitudinal and lateral data, were recorded after control transitions. The effects of each attribute were explored using aforementioned measures, as well as subjective ratings of cognitive load (in the case of cognitive attributes). A positive correlation was found between cognitive load level and the maximum longitudinal deceleration in the case of hazard avoidance. This means that participants who declared highest levels of cognitive load were also the ones who braked the most when they had to avoid a collision. The cognitive load also had an effect on distance and time to collision variables. By contrast, resource allocation had no effect on the takeover quality. The researchers concluded that the increase of cognitive load during NDRT was associated with a difficulty to perform lateral and longitudinal control during a takeover situation. They also deduced that physical and visual attributes were less important than cognitive ones regarding takeover control performance.
Subjective workload also brought interesting results in another research team’s study (Wandtner et al., 2018a). It was significantly different between each task, with lowest workload recorded under the auditory-vocal condition, and highest one under the visual-manual high workload condition. No effect of cognitive workload was found on the first response variables (e.g., hands-on time). On the contrary, an influence of both task modalities and mental workload was observed on time to brake. Indeed, participants braked stronger under visual-manual than auditory-visual conditions, and under visual-manual high workload than visual-manual task conditions. The authors specifically noted that high mental workload had no effect on first reactions, but on actions that needed higher cognitive processes, such as action selection and decision.
Wandtner et al, also used subjective ratings as dependent variables in their second experiment (Wandtner et al., 2018b). The authors used the common NASA-TLX (Hart and Staveland, 1988) to evaluate the workload level associated with the NDRTs. They also asked participants to rate their subjective perception of the NDRTs levels of safety, and to classify the NDRTs in function of their level of disruption in the case of a takeover situation. Results confirmed the association between workload and an increase of cognitive dimensions, as the auditory tasks were associated with smaller scores than visual-manual tasks. The perceived safety level of each NDRT increased with the number of cognitive dimensions needed to get the task done. Participants also declared the most complex (i.e., visual–manual handheld) NDRT as the most impairing under takeover situations.
Discussion
Task Engagement: Manipulation and Measurement
The objective of this work was to propose a literature review of the existing studies dealing with non-driving-related tasks engagement, as well as its impact on taking over control of highly automated vehicles. More precisely, we attempted to better understand and explain how task engagement was manipulated and analysed in the literature. The complexity firstly lied in grouping together studies with close, or at least similar, exploration goals (i.e., around task engagement, NDRTs, and automation), in spite of the heterogeneity of the terms used (e.g., task engagement, task demand, cognitive load, and effort), and of the theoretical approaches. Among the selected studies, we noted that some authors (i.e., The Manipulation of Engagement) designed specific experimental protocols to vary task engagement by the choice of activities to be carried out on board, with a view to analyse the effects of such variability. In this precise case, engagement was used as an independent variable. Other research teams (i.e., The Evaluation of Task Engagement), on the other hand, preferred to use measures of task engagement through recognised factors (e.g., resource allocation, cognitive effort, and mental workload). Engagement, or its associated factors, were then used as dependent variables. Both approaches aimed to study differences between daily life activities carried out on board, and to look for correlations with driving behaviour when regaining control.
Eight main tasks were identified within the articles studied, i.e., object manipulation, audio listening, video watching, gaming or video gaming, searching for information, typing, reading, and taking part in text exercises. Figure 3 illustrates the type and the occurrence of NDRTs used in the reviewed studies. We decided to distinguish classical reading activity to the reading activities with exercises because the cognitive dimensions involved were not the same, as demonstrated by Wickens’ MRT (Wickens, 2002). Three activities stood out within the selected articles, i.e., reading (simply or as an exercise), video watching, and game playing.
Two main approaches were observed regarding studies varying engagement through their task. The first one focuses on generating differences by choosing particular tasks. Most of the authors postulated that there were differences between chosen tasks based on previous research on the same topic (i.e., studies on takeover performance after a period of highly automated driving, e.g., Llaneras et al., 2013; Merat et al., 2012; Kim et al., 2015). Other studies (e.g., Zeeb et al., 2017; Borojeni et al., 2018; Wandtner et al., 2018b) relied on the notion of task difficulty to yield variations, sometimes within a single NDRT. Generally, instructions were then more complex for the difficult version of the task, ranging from a version without instruction (e.g., watching a video), to a version with a particular instruction requiring textual or audio-visual data processing. The second approach identified was the reliance on cognitive models defining cognitive dimensions which create different levels of difficulty. The predominantly used model, when a model was cited, is Wickens’ multiple resources theory (MRT) (Wickens, 2002). This model was used to justify a manipulation of the cognitive load/workload, and of the difficulty generated by the task. Thus, by combining several dimensions [e.g., motor and visual dimensions (Borojeni et al., 2018)], the research teams obtained different levels in the subjective evaluation of engagement factors (e.g., workload). The threaded cognition theory (Salvucci and Taatgen, 2008) appeared in one paper (i.e., Tanshi and Söffker, 2019) in order to justify an increase of the level of resources required between the different proposed versions of a reading task. It can be noted that the authors who used a cognitive theory (i.e., Wickens, 2002, or Salvucci and Taatgen, 2008) to choose their activities all ended up with reading tasks.
Among the studies which chose task engagement as variable to be measured (i.e., The Evaluation of Task Engagement), two main types of measurements were found. The first one corresponds to the way of managing the task with driving (i.e., autonomy supervision and/or transition), whereas the second one designates participants’ subjective assessment of their engagement, or of various factors of engagement (e.g., effort, estimated level of demand, distraction). Task performance was identified as another metric which we did not rank at the same level as the ones aforementioned, as its relevance in the cited research was only minor. Yet, task performance can be considered as an indicator of task engagement, even though it is not self-sufficient.
Task engagement is a state resulting from multiple factors, which are important to combine to best cover such a complex cognitive state. Control transition phases also comprise different stages which can be linked to sub-factors of task engagement, as shown in Figure 4. Figures 4, 5 illustrate our identification and distinction of these phases, by associating to each of them their corresponding measurements. Existing approaches lack Such distinction is often lacking in current approaches to control transition (Melcher et al., 2015; Walch et al., 2015; Dogan et al., 2017).
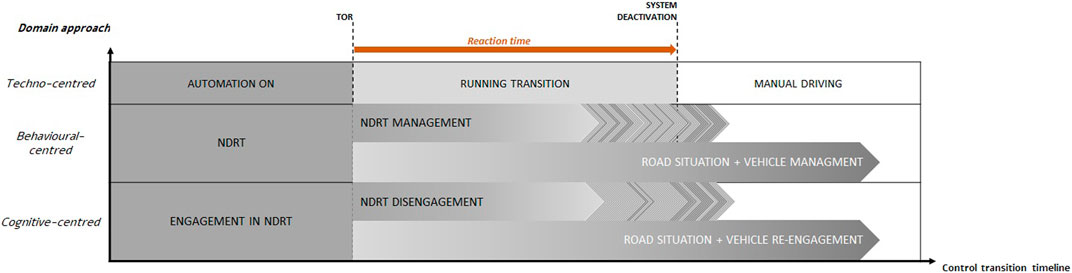
FIGURE 4. Illustration of different ways to investigate a control transition, from system to driver.
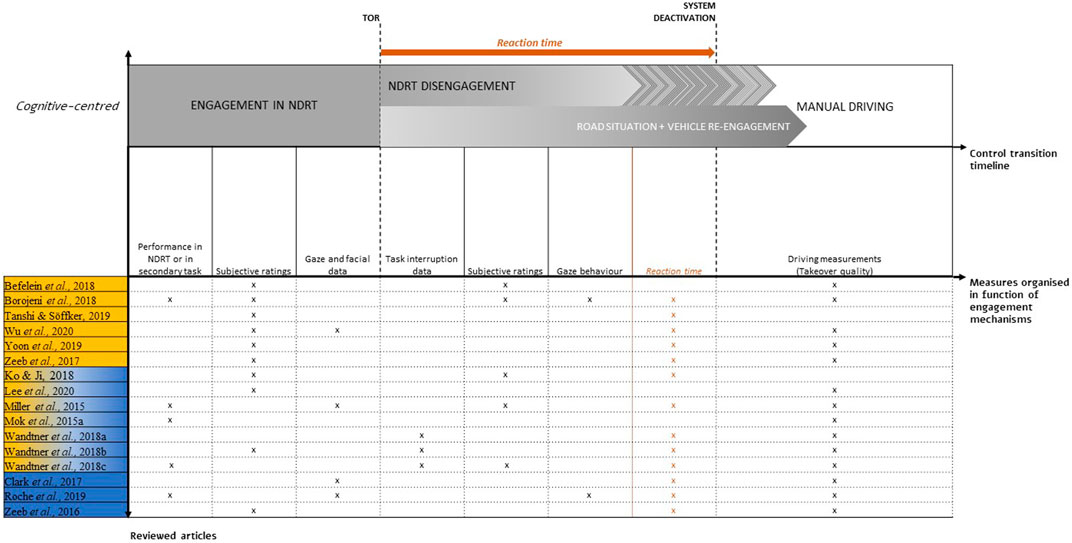
FIGURE 5. Illustration of the attribution of reviewed articles measurements to each control phase according to engagement mechanisms.
Task Engagement in the Course of Control Transition
Figure 4 exposes three principal ways to address takeover. It starts with a techno-centric approach, the most common one nowadays, which mainly describes takeover according to a system’s behaviour. Techno-centric studies clearly tend to separate delegation of control, and takeover alert. System deactivation then initiates the last phase, that is to say: manual driving. Starting from this techno-centric point of view, an approach by the driver’s behaviour can be proposed. During driving delegation, drivers usually engage in NDRTs (Pfleging et al., 2016; Large et al., 2018). From takeover request, a greater complexity can be observed for the behaviour-centred approach. In fact, phases are not sequential but layered. Stopping the NDRT does not necessarily precede regaining control of the vehicle; these can overlap, which creates the complexity and multifactorial nature of the takeover. From a safety-centred point of view, a quick and total abandonment of the NDRT in favour of regaining total control of the vehicle is expected. Nonetheless, the human factor with its wide variability has to be considered. Using a cognitive-centred approach, we attempted to identify the mechanisms of task engagement involved from these observable behaviours.
From such a cognitive-centred point of view, Figure 5 illustrates a repartition of measurements found in the reviewed articles that fit each phase of a control transition. A control transition phase, i.e., from automation to driver, can be considered both multidimensional and multiphasic. It is indeed multidimensional because it simultaneously calls on several functions, such as auditory perception (e.g., alarms), visual perception, motor reaction, and attention. Control transition can also be considered multiphasic by distinguishing three main phases, as follows. The first one corresponds to the pre-alarm stage, during which the NDRT is performed under automated driving conditions. This phase is important in the study of control transition as it appears necessary to know the driver’s state at the moment of a takeover request. Thanks to our literature review, we assigned three main measures to this phase. The first one includes subjective variables about the driver’s cognitive state, collected, or not, through standardised questionnaires [e.g., NASA-TLX (Hart and Staveland, 1988)], such as mental workload, interest in the task, and experienced difficulty. Gaze behaviour represents the second measure, as it was identified as a variable used to assess driver’s engagement in the NDRT, particularly using the fixations (i.e., number and time) distribution between road and task. Finally, even though it was not clearly identified as an engagement variable, task performance was sometimes used by the authors, which also appears as an interesting indicator. On the contrary, performance measurement of the driving task was a central component of takeover assessment. According to the techno-centric approach, we noticed a transition phase between takeover request and deactivation of the automated system. From a cognitive perspective, this phase is composed of two interlocking stages of task engagement, i.e., a disengagement of the activity and a re-engagement in the road situation and driving, lasting until the final phase. A mobilisation of several cognitive and motor skills thus takes place in a very short period of time. We consequently identified three main variables as well as an ancillary variable in the experiments studied. A first type of measurement groups together all the variables recording the way the NDRT is handled from the takeover request. Some authors (e.g., Wandtner et al., 2018a) chose to code the NDRT management differences, e.g., “completely abandoned”, “paused before stopping”, “continued in parallel”. The notion of interruptibility level of the task appears as an indicator of the driver’s disengagement from the NDRT when a takeover was requested. It can be measured in several ways, yet the goal always seems to be the same, i.e., to evaluate to what extent the driver decreases the use of their task resources to the NDRT. We also assigned several subjective evaluations to this phase, such as the difficulty experienced to perform the takeover, or the distraction generated by the NDRT, compared to the driving task. Note subjective evaluations could relate to both disengagement and re-engagement in the task. Gaze behaviour (i.e., fixation duration and number of glances to the mirrors) was also used to determine the driver’s strategy to regain control.
In the literature, reaction time is commonly used as an indicator of takeover performance. However, according to the multiplicity of involved mechanisms as we demonstrated in this article, this metric needs to be nuanced, as different types of reaction times can be identified. For instance, several information processing and decision-taking processes are involved behind considering the time to redirect gaze towards the road and the time to effectively act on the steering wheel. This could lead to attributing different meanings to similar values of these two types of reaction times. The time to redirect gaze towards the driving environment could be considered as a reference to measure the first instants of NDRT disengagement. On another side, the time to effectively act on the vehicle commands refers more to the first instants of driving task reengagement. Even with this subtlety, we argue that reaction time cannot be considered alone to provide a detailed insight on the ongoing task transition process. Thus, it should be at least combined with the other driving performance indicators. It seems that takeover quality indicators are considered as key information to assess re-engagement in driving. Even though they provide reliable and useful information to assess driving performance and fitness of behavior, such variable appears to be insufficient to provide detailed explanation in case of takeover failure. Consequently, considering the driver’s cognitive state should be central for a better understanding of the transition steps during the takeover process. As suggested in our approach, NDRT disengagement, road situation, and vehicle control re-engagement appear to be more complex and entangled than simple on/off transitions. As illustrated in several studies, it frequently happens that the driver returns back to the NDRT after at least partially regaining manual control of the vehicle (e.g., Feldhütter et al., 2017). Such phenomenon could be explained with the help of deeper assessment about cognitive processes and situation awareness rebuilding states. Therefore, a better consideration for temporal lability of resources allocation to the two considered tasks (i.e., NDRT and driving) through more extensive probing of driver cognition should become the new gold standard in takeover studies.
Conclusion
In this paper, we presented a literature review on existing research works studying the effects of non-driving related tasks (NDRTs) on task engagement under highly automated driving (i.e., SAE levels 3 and 4). More specifically, we analysed how engagement in NDRT was manipulated and/or measured in the specific context of control transition, i.e., from automated driving to manual takeover. Based on this work, we proposed a novel approach of the transition of control, more centred on engagement mechanisms, which better considers the cognitive aspects of such a complex action, compared to a more traditional techno-centric perspective. Indeed, our literature review revealed the lack of ability of the techno-centric approach to properly grasp the subtlety of the cognitive engagement-disengagement processes, especially the fact that disengagement from NDRT and reengagement in driving is more of a continuum than a simple on-off switch. Our cognitive-centred approach aims to compensate for this flaw. More particularly, it postulates that transition phases cannot only be considered through a timing aspect. We demonstrated that the time between two behaviours could not be used as the only measure of the cognitive processes in course. To this end, a better consideration of disengagement and reengagement processes is the clue to understand the driver ability to takeover. Consequently, we came up with a combination of the different cognitive states involved, and the many diverse measures of engagement observed in our review. We believe that this clarification of the stages of task engagement state and of its associated metrics allows for a more complete and accurate approach of control transition from automation to human. We further suggest that the cognitive engagement-centred view developed in this work serves as a methodological guide for future research about engagement in control transitions. This would allow for more accurate findings about the takeover inner dynamics.
Author Contributions
MJ and LL contributed to the design of the literature review. TB, HT, and CM-L supervised the research project. MJ wrote the first draft of the manuscript. LL and JD wrote sections of the manuscript. All the authors contributed to manuscript revision; read, and approved the submission.
Conflict of Interest
The authors declare that the research was conducted in the absence of any commercial or financial relationships that could be construed as a potential conflict of interest.
Publisher’s Note
All claims expressed in this article are solely those of the authors and do not necessarily represent those of their affiliated organizations, or those of the publisher, the editors and the reviewers. Any product that may be evaluated in this article, or claim that may be made by its manufacturer, is not guaranteed or endorsed by the publisher.
References
Bainbridge, L. (1987). Ironies of Automation. New Technol. Hum. Error 15, 271–283. doi:10.1016/S1474-6670(17)62897-0
Befelein, D., Boschet, J., and Neukum, A. (2018). Influence of Non-driving-related Tasks’ Motivational Aspects and Interruption Effort on Driver Take-Over Performance in Conditionally Automated Driving. 6th international conference on driver distraction and inattention, Gothenburg, Sweden, October, 2018. (Gothenburg, Sweden), 9. Retrieved from: http://ddi2018.org/wp-content/uploads/2018/10S
Beukel, A. P. v. d., and Voort, M. C. v. d. (2013). “The Influence of Time-Criticality on Situation Awareness when Retrieving Human Control after Automated Driving,” in 16th International IEEE Conference on Intelligent Transportation Systems (ITSC 2013), Netherlands, October, 2013 (IEEE). 2000–2005. doi:10.1109/ITSC.2013.6728523
Borojeni, S. S., Weber, L., Heuten, W., and Boll, S. (2018). “From reading to Driving,” in Proceedings of the 20th International Conference on Human-Computer Interaction with Mobile Devices and Services MobileHCI ’18, Barcelona, Spain, September, 2018 (New York, NY, USA, ACM14, 1–12. doi:10.1145/3229434.3229464
Carsten, O., Lai, F. C. H., Barnard, Y., Jamson, A. H., and Merat, N. (2012). Control Task Substitution in Semiautomated Driving. Hum. Factors 54, 747–761. doi:10.1177/0018720812460246
Clark, H., McLaughlin, A. C., Williams, B., and Feng, J. (2017). “Performance in Takeover and Characteristics of Non-driving Related Tasks during Highly Automated Driving in Younger and Older Drivers,” in Proceedings of the Human Factors and Ergonomics Society Annual Meeting, Sage, CA, September, 2017 (Los Angeles, CA: SAGE Publications) 61 (1), 37–41. doi:10.1177/1541931213601504
Dingus, T., Klauer, S., Lewis, V., Petersen, A., Lee, S., Sudweeks, J., et al. (2006). The 100-Car Naturalistic Driving Study: Phase II – Results of the 100-Car Field Experiment (No. DOT-HS-810-593). United States: National Highway Traffic Safety Administration.
Dogan, E., Rahal, M.-C., Deborne, R., Delhomme, P., Kemeny, A., and Perrin, J. (2017). Transition of Control in a Partially Automated Vehicle: Effects of Anticipation and Non-driving-related Task Involvement. Transportation Res. F: Traffic Psychol. Behav. 46, 205–215. doi:10.1016/j.trf.2017.01.012
Endsley, M. R., and Kaber, D. B. (1999). Level of Automation Effects on Performance, Situation Awareness and Workload in a Dynamic Control Task. Ergonomics 42, 462–492. doi:10.1080/001401399185595
Engeser, S., and Rheinberg, F. (2008). Flow, Performance and Moderators of challenge-skill Balance. Motiv. Emot. 32, 158–172. doi:10.1007/s11031-008-9102-4
Eriksson, A., and Stanton, N. A. (2017). Takeover Time in Highly Automated Vehicles: Noncritical Transitions to and from Manual Control. Hum. Factors 59, 689–705. doi:10.1177/0018720816685832
Feldhütter, A., Gold, C., Schneider, S., and Bengler, K. (2017). How the Duration of Automated Driving Influences Take-Over Performance and Gaze Behavior (Heidelberg: Springer), 309–318.
Gold, C., Körber, M., Lechner, D., and Bengler, K. (2016). Taking over Control from Highly Automated Vehicles in Complex Traffic Situations. Hum. Factors 58, 642–652. doi:10.1177/0018720816634226
Gold, C., Lorenz, L., Damböck, D., and Bengler, K. (2013). Partially Automated Driving as a Fallback Level of High Automation. Fahrerassistenz Weg Zum Autom. Fahr. 6 Tag.
Hart, S. G., and Staveland, L. E. (1988). “Development of NASA-TLX (Task Load Index): Results of Empirical and Theoretical Research,” in Advances in Psychology Human Mental Workload. Editors P. A. Hancock, and N. Meshkati (North-Holland)), 139–183. doi:10.1016/S0166-4115(08)62386-9
Kim, I.-H., Kim, J.-W., Haufe, S., and Lee, S.-W. (2015). Detection of Braking Intention in Diverse Situations during Simulated Driving Based on EEG Feature Combination. J. Neural Eng. 12, 016001. doi:10.1088/1741-2560/12/1/016001
Ko, S. M., and Ji, Y. G. (2018). How We Can Measure the Non-driving-task Engagement in Automated Driving: Comparing Flow Experience and Workload. Appl. Ergon. 67, 237–245. doi:10.1016/j.apergo.2017.10.009
Large, D. R., Burnett, G., Morris, A., Muthumani, A., and Matthias, R. (2018). “A Longitudinal Simulator Study to Explore Drivers' Behaviour during Highly-Automated Driving,” in Advances in Human Aspects of Transportation Advances in Intelligent Systems and Computing. Editor N. A. Stanton (Cham: Springer International Publishing), 583–594. doi:10.1007/978-3-319-60441-1_57
Lee, J. D. (2014). Dynamics of Driver Distraction: The Process of Engaging and Disengaging. Ann. Adv. Automot Med. 58, 24–32.
Lee, S. C., Yoon, S. H., and Ji, Y. G. (2020). Effects of Non-driving-related Task Attributes on Takeover Quality in Automated Vehicles. Int. J. Human-Computer Interaction 37, 211–219. doi:10.1080/10447318.2020.1815361
Llaneras, R., Salinger, J., and Green, C. (2013). Human Factors Issues Associated with Limited Ability Autonomous Driving Systems: Drivers’ Allocation of Visual Attention to the Forward Roadway. Proceedings of the Seventh International Driving Symposium on Human Factors in Driver Assessment, Training and Vehicle Design, Bolton Landing, NY, June 17–20, 2013 (Iowa City, IA: Public Policy Center, University of Iowa), 92–98.
Marberger, C., Mielenz, H., Naujoks, F., Radlmayr, J., Bengler, K., and Wandtner, B. (2018). “Understanding and Applying the Concept of "Driver Availability" in Automated Driving,” in Advances in Human Aspects of Transportation. Editor N. A. Stanton (Cham: Springer International Publishing), 595–605. doi:10.1007/978-3-319-60441-1_58
Matthews, G., Warm, J. S., Reinerman, L. E., Langheim, L. K., and Saxby, D. J. (2010). Task Engagement, Attention, and Executive Control. In Handbook of Individual Differences in Cognition: Attention, Memory, and Executive Control the Springer Series on Human Exceptionality. New York, NY, US: Springer Science + Business Media), 205–230. doi:10.1007/978-1-4419-1210-7_13
Melcher, V., Rauh, S., Diederichs, F., Widlroither, H., and Bauer, W. (2015). Take-Over Requests for Automated Driving. Proced. Manufacturing 3, 2867–2873. doi:10.1016/j.promfg.2015.07.788
Merat, N., Jamson, A. H., Lai, F. C. H., and Carsten, O. (2012). Highly Automated Driving, Secondary Task Performance, and Driver State. Hum. Factors 54, 762–771. doi:10.1177/0018720812442087
Miller, D., Sun, A., Johns, M., Ive, H., Sirkin, D., Aich, S., et al. (2015). Distraction Becomes Engagement in Automated Driving. Proc. Hum. Factors Ergon. Soc. Annu. Meet. 59, 1676–1680. doi:10.1177/1541931215591362
Mok, B., Johns, M., Miller, D., and Ju, W. (2017). “Tunneled in: Drivers with Active Secondary Tasks Need More Time to Transition from Automation,” in Proceedings of the 2017 CHI Conference on Human Factors in Computing Systems, Denver, CO, United States, May 6–11, 2017 (ACM), 2840–2844.
Mok, B. K.-J., Johns, M., Lee, K. J., Ive, H. P., Miller, D., and Ju, W. (2015). “Timing of Unstructured Transitions of Control in Automated Driving,” in 2015 IEEE Intelligent Vehicles Symposium, 1167–1172. doi:10.1109/IVS.2015.7225841
Naujoks, F., Befelein, D., Wiedemann, K., and Neukum, A. (2018). “A Review of Non-driving-related Tasks Used in Studies on Automated Driving,” in Advances in Human Aspects of Transportation Advances in Intelligent Systems and Computing. Editor N. A. Stanton (Springer International Publishing), 525–537. doi:10.1007/978-3-319-60441-1_52
Naujoks, F., Purucker, C., and Neukum, A. (2016). Secondary Task Engagement and Vehicle Automation - Comparing the Effects of Different Automation Levels in an On-Road experiment. Transportation Res. Part F: Traffic Psychol. Behav. 38, 67–82. doi:10.1016/j.trf.2016.01.011
Neubauer, C., Matthews, G., and Saxby, D. (2012). The Effects of Cell Phone Use and Automation on Driver Performance and Subjective State in Simulated Driving. Proc. Hum. Factors Ergon. Soc. Annu. Meet. 56, 1987–1991. doi:10.1177/1071181312561415
Park, J., Jung, E. S., and Im, Y. (2019). 2H1-6 the Effect of Driver Engagement in Autonomous Driving Based on Flow Experience. Jpn. J. Ergon. 55, 2H1-6. doi:10.5100/jje.55.2H1-6
Pashler, H., Johnston, J. C., and Ruthruff, E. (2001). Attention and Performance. Annu. Rev. Psychol. 52, 629–651. doi:10.1146/annurev.psych.52.1.629
Pfleging, B., Rang, M., and Broy, N. (2016). “Investigating User Needs for Non-driving-related Activities during Automated Driving,” in Proceedings of the 15th International Conference on Mobile and Ubiquitous Multimedia MUM ’16 (New York, NY, USA: ACM), 91–99. doi:10.1145/3012709.3012735
Pohl, H., and Murray-Smith, R. (2013). “Focused and Casual Interactions,” in Proceedings of the SIGCHI Conference on Human Factors in Computing Systems (New York, NY, USA: Association for Computing Machinery), 2223–2232. Available at: https://doi.org/10.1145/2470654.2481307 (Accessed January 29, 2021).
Reilhac, P., Millett, N., and Hottelart, K. (2015). Thinking intuitive driving automation, in Road Vehicle Automation 2. Cham: Springer, 61–70.
Roche, F., Somieski, A., and Brandenburg, S. (2019). Behavioral Changes to Repeated Takeovers in Highly Automated Driving: Effects of the Takeover-Request Design and the Nondriving-Related Task Modality. Hum. Factors 61, 839–849. doi:10.1177/0018720818814963
SAE On-Road Automated Vehicle Standards Committee (2014). Taxonomy and Definitions for Terms Related to On-Road Motor Vehicle Automated Driving Systems. SAE Std. J., 3016, 1–16.
Salvucci, D. D., Taatgen, N. A., and Borst, J. P. (2009). Toward a Unified Theory of the Multitasking Continuum: From Concurrent Performance to Task Switching, Interruption, and Resumption. Proceedings of the SIGCHI Conference on Human Factors in Computing Systems, Boston, MA, United States, April, 2009, 1819–1828.
Salvucci, D. D., and Taatgen, N. A. (2008). Threaded Cognition: An Integrated Theory of Concurrent Multitasking. Psychol. Rev. 115, 101–130. doi:10.1037/0033-295X.115.1.101
Shahid, A., Wilkinson, K., Marcu, S., and Shapiro, C. M. (2011). “Karolinska Sleepiness Scale (KSS),” in STOP, THAT and One Hundred Other Sleep Scales. Editors A. Shahid, K. Wilkinson, S. Marcu, and C. M. Shapiro (New York, NY: Springer), 209–210. doi:10.1007/978-1-4419-9893-4_47
Shinar, D., Tractinsky, N., and Compton, R. (2005). Effects of Practice, Age, and Task Demands, on Interference from a Phone Task while Driving. Accid. Anal. Prev. 37, 315–326. doi:10.1016/j.aap.2004.09.007
Stanton, N. A., and Young, M. S. (2003). Giving Ergonomics Away? the Application of Ergonomics Methods by Novices. Appl. Ergon. 34, 479–490. doi:10.1016/S0003-6870(03)00067-X
Stanton, N. A., and Young, M. S. (1998). Vehicle Automation and Driving Performance. Ergonomics 41, 1014–1028. doi:10.1080/001401398186568
Strand, N. (2014). Doktorsavhandlingar Vid Chalmers Tek. Högsk. Ny Ser. Available at: https://trid.trb.org/view/1367603 (Accessed January 29, 2021).
Tanshi, F., and Söffker, D. (2019). Modeling of Takeover Variables with Respect to Driver Situation Awareness and Workload for Intelligent Driver Assistance. 2019 IEEE Intelligent Vehicles Symposium, June, 2019 (IV), 1667–1672. doi:10.1109/IVS.2019.8814045
Taylor, R. M. (1989). Situational Awareness Rating Technique (SART): The Development of a Tool for Aircrew Systems Design. Proceeding of the AGARD AMP Symposium on Situational Awareness in Aerospace Operations, CP478, Seuilly-sur Seine (NATO AGARD).
Walch, M., Lange, K., Baumann, M., and Weber, M. (2015). “Autonomous Driving,” in Proceedings of the 7th International Conference on Automotive User Interfaces and Interactive Vehicular Applications AutomotiveUI ’15 (New York, NY, USA: ACM), 11–18. doi:10.1145/2799250.2799268
Wandtner, B., Schmidt, G., Schoemig, N., and Kunde, W. (2018a). “Non-driving Related Tasks in Highly Automated Driving - Effects of Task Modalities and Cognitive Workload on Take-Over Performance,” in AmE 2018 - Automotive meets Electronics; 9th GMM-Symposium, Dortmund, Germany, 1–6.
Wandtner, B., Schömig, N., and Schmidt, G. (2018b). Effects of Non-driving Related Task Modalities on Takeover Performance in Highly Automated Driving. Hum. Factors 60, 870–881. doi:10.1177/0018720818768199
Wandtner, B., Schömig, N., and Schmidt, G. (2018c). Secondary Task Engagement and Disengagement in the Context of Highly Automated Driving. Transportation Res. Part F: Traffic Psychol. Behav. 58, 253–263. doi:10.1016/j.trf.2018.06.001
Wester, A. E., Böcker, K. B. E., Volkerts, E. R., Verster, J. C., and Kenemans, J. L. (2008). Event-related Potentials and Secondary Task Performance during Simulated Driving. Accid. Anal. Prev. 40, 1–7. doi:10.1016/j.aap.2007.02.014
Wickens, C. D. (2002). Multiple Resources and Performance Prediction. Theor. Issues Ergon. Sci. 3, 159–177. doi:10.1080/14639220210123806
Wright, T. J., Samuel, S., Borowsky, A., Zilberstein, S., and Fisher, D. L. (2016). “Experienced Drivers are Quicker to Achieve Situation Awareness than Inexperienced Drivers in Situations of Transfer of Control Within a Level 3 Autonomous Environment,” in Proceedings of the Human Factors and Ergonomics Society Annual Meeting, 60 (1), 270–273. doi:10.1177/1541931213601062
Wu, Y., Kihara, K., Hasegawa, K., Takeda, Y., Sato, T., Akamatsu, M., et al. (2020). Age-related Differences in Effects of Non-driving Related Tasks on Takeover Performance in Automated Driving. J. Saf. Res. 72, 231–238. doi:10.1016/j.jsr.2019.12.019
Yoon, S. H., and Ji, Y. G. (2019). Non-driving-related Tasks, Workload, and Takeover Performance in Highly Automated Driving Contexts. Transportation Res. Part F: Traffic Psychol. Behav. 60, 620–631. doi:10.1016/j.trf.2018.11.015
Young, M. S. (2010). Human Factors of Visual and Cognitive Performance in Driving. Ergonomics 53, 444–445. doi:10.1080/00140130903494785
Young, M. S., and Stanton, N. A. (2002). Malleable Attentional Resources Theory: A New Explanation for the Effects of Mental Underload on Performance. Hum. Factors 44, 365–375. doi:10.1518/0018720024497709
Zeeb, K., Buchner, A., and Schrauf, M. (2016). Is Take-Over Time All that Matters? the Impact of Visual-Cognitive Load on Driver Take-Over Quality after Conditionally Automated Driving. Accid. Anal. Prev. 92, 230–239. doi:10.1016/j.aap.2016.04.002
Zeeb, K., Härtel, M., Buchner, A., and Schrauf, M. (2017). Why Is Steering Not the Same as Braking? the Impact of Non-driving Related Tasks on Lateral and Longitudinal Driver Interventions during Conditionally Automated Driving. Transportation Res. Part F: Traffic Psychol. Behav. 50, 65–79. doi:10.1016/j.trf.2017.07.008
Keywords: highly automated driving, non-driving-related task, task engagement, task disengagement, interruptibility, takeover
Citation: Jaussein M, Lévêque L, Deniel J, Bellet T, Tattegrain H and Marin-Lamellet C (2021) How Do Non-driving-related Tasks Affect Engagement Under Highly Automated Driving Situations? A Literature Review. Front. Future Transp. 2:687602. doi: 10.3389/ffutr.2021.687602
Received: 29 March 2021; Accepted: 22 July 2021;
Published: 30 July 2021.
Edited by:
Xiaohua Zhao, Beijing University of Technology, ChinaReviewed by:
Asad Waqr Malik, National University of Sciences and Technology, PakistanYiping Wu, Beijing University of Technology, China
Copyright © 2021 Jaussein, Lévêque, Deniel, Bellet, Tattegrain and Marin-Lamellet. This is an open-access article distributed under the terms of the Creative Commons Attribution License (CC BY). The use, distribution or reproduction in other forums is permitted, provided the original author(s) and the copyright owner(s) are credited and that the original publication in this journal is cited, in accordance with accepted academic practice. No use, distribution or reproduction is permitted which does not comply with these terms.
*Correspondence: Marie Jaussein, bWFyaWUuamF1c3NlaW5AdW5pdi1laWZmZWwuZnI=