- 1European Centre for Environment and Human Health, University of Exeter Medical School, Cornwall, United Kingdom
- 2Environment and Sustainability Institute, University of Exeter Medical School, Cornwall, United Kingdom
- 3Medical Research Council Centre for Medical Mycology, University of Exeter, Exeter, United Kingdom
- 4Chief Scientist’s Group, Environment Agency, Horizon House, Bristol, England, United Kingdom
This scoping review aims to summarise the current understanding of selection for antifungal resistance (AFR) and to compare and contrast this with selection for antibacterial resistance, which has received more research attention. AFR is an emerging global threat to human health, associated with high mortality rates, absence of effective surveillance systems and with few alternative treatment options available. Clinical AFR is well documented, with additional settings increasingly being recognised to play a role in the evolution and spread of AFR. The environment, for example, harbours diverse fungal communities that are regularly exposed to antifungal micropollutants, potentially increasing AFR selection risk. The direct application of effect concentrations of azole fungicides to agricultural crops and the incomplete removal of pharmaceutical antifungals in wastewater treatment systems are of particular concern. Currently, environmental risk assessment (ERA) guidelines do not require assessment of antifungal agents in terms of their ability to drive AFR development, and there are no established experimental tools to determine antifungal selective concentrations. Without data to interpret the selective risk of antifungals, our ability to effectively inform safe environmental thresholds is severely limited. In this review, potential methods to generate antifungal selective concentration data are proposed, informed by approaches used to determine antibacterial minimal selective concentrations. Such data can be considered in the development of regulatory guidelines that aim to reduce selection for AFR.
Introduction
Antimicrobials, including antibacterial, antiviral, antifungal and antiparasitic agents, underpin modern medical practice and extensive agriculture (O’Neill, 2015; O'Neill, 2016; Fisher et al., 2018; Murray et al., 2018). However, the efficacy of antimicrobial drug therapy is increasingly challenged by the emergence and spread of antimicrobial resistance (AMR). AMR microorganisms, including bacteria, viruses, fungi, and parasites have the ‘ability to multiply or persist in the presence of an increased level of an antimicrobial agent’ (Ashbolt et al., 2013). Owing to drug-resistant infections of clinical importance, AMR research is well represented in the literature with respect to antibacterial resistance (ABR). In comparison, antifungal resistance (AFR) has been less well studied.
Fungi are a diverse group of eukaryotes, ranging from single-celled yeasts to complex, multicellular moulds (More et al., 2010). Numerous fungal species are responsible for invasive infections, with Candida spp. (yeasts) and Aspergillus spp. (moulds) being the major human fungal pathogens (Hill et al., 2015; Gow and Yadav, 2017). Collectively, invasive fungal infections cause more annual deaths than malaria or tuberculosis (Barnes et al., 2014; Van Dijck et al., 2018). Importantly, fungal communities are adaptable and may evolve resistance following antifungal exposure (Odds et al., 2003; Anderson, 2005; Perlin et al., 2017; Ksiezopolska and Gabaldon, 2018; Revie et al., 2018), making infections more difficult to treat.
As a consequence of the current COVID-19 pandemic, the urgency to tackle and mitigate AFR has increased due to critically ill COVID-19 patients suffering drug-resistant, invasive fungal infections. For instance, COVID-19 patients in India are reported to have suffered multidrug-resistant Candida auris infections, with high case-fatality rates (Chowdhary et al., 2020). Cases of COVID-19 associated aspergillosis and, the less common but serious infection, mucormycosis have also been reported (Singh et al., 2021). This exemplifies the importance of monitoring AFR and improving our understanding of the mechanisms behind the evolution of AFR across all ‘One Health’ sectors. The One Health approach to tackling AMR is inclusive of human, environment and animal health (Chowdhary and Meis, 2018). Previous research (Brown et al., 2012) highlights the threat of AFR in the clinic and the risk of AFR evolution due to the agricultural use of azoles, but the wider environmental dimension of AFR has comparatively been neglected.
AFR in the Environment
Uses of antifungal compounds vary, including therapeutic use in human and/or veterinary medicine; use in personal care products, such as antidandruff shampoos (Richter et al., 2013); and the application of fungicides as plant protection products (PPPs) in agricultural settings. The three primary classes of antifungal agents used in the clinic are echinocandins, azoles and polyenes (Ksiezopolska and Gabaldon, 2018). The azoles are used in both human/veterinary medicine and in agriculture (Fisher et al., 2018). Given the varied applications of antifungals, there are a number of pathways for antifungals to enter the environment (Figure 1).
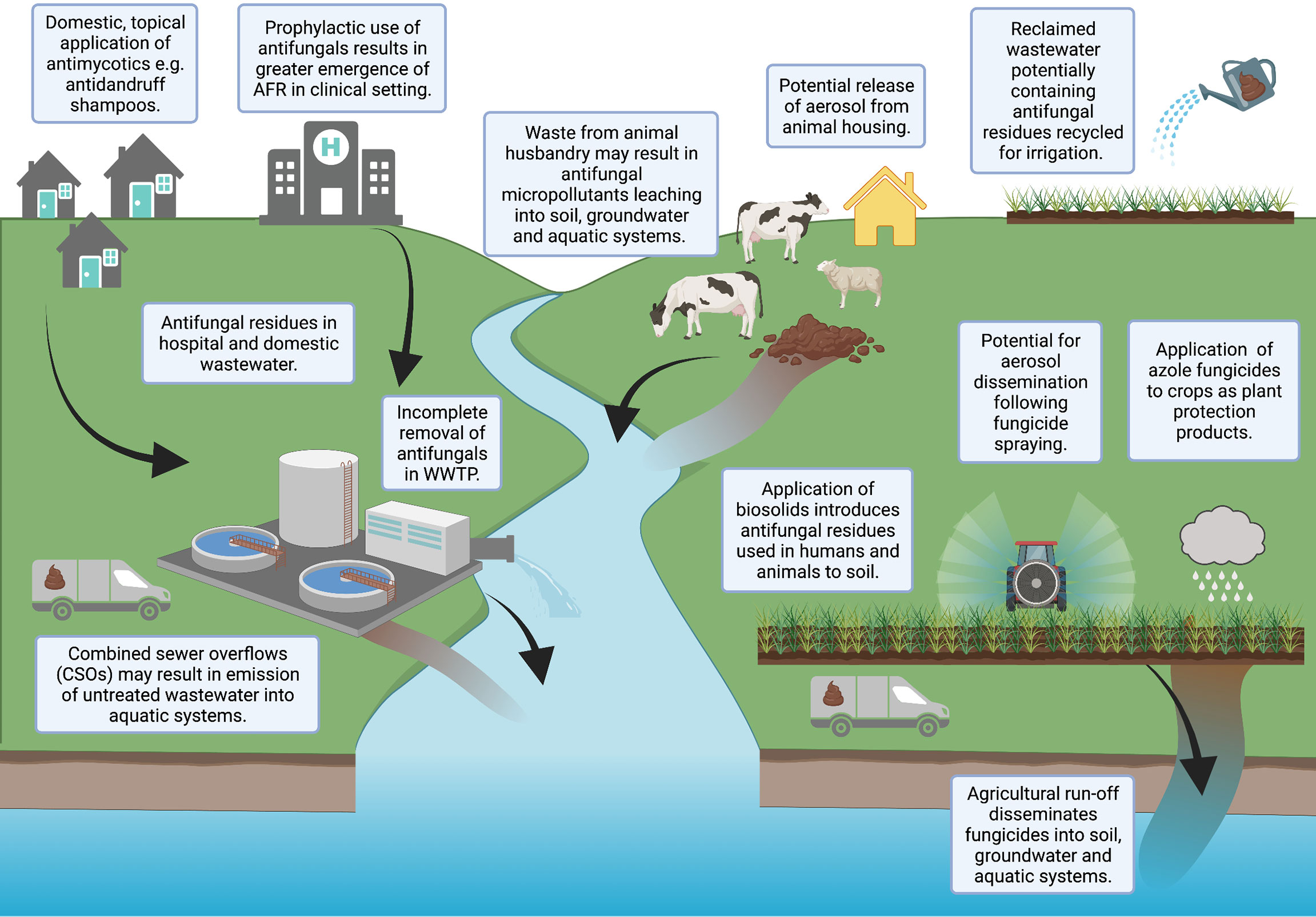
Figure 1 Potential pathways for antifungals to enter the environment. Created with BioRender.com.
Wastewater, both domestic and clinical, has been reported to contain antifungal residues (Lindberg et al., 2010; Escher et al., 2011; Chen and Ying, 2015). Antifungals are commonly applied topically to the human body, resulting in high emissions of active ingredients (90-95%) into wastewater (Letzel et al., 2009; Peng et al., 2012; Richter et al., 2013; Assress et al., 2019; Assress et al., 2020; Assress et al., 2021b). Wastewater treatments are unable to effectively remove such compounds, resulting in antifungal contamination of sewage sludge, biosolid amended soil, wastewater effluent and receiving surface waters (Kahle et al., 2008; Peng et al., 2012; Chen et al., 2014; Assress et al., 2019; Assress et al., 2020; Assress et al., 2021b). Furthermore, reclaimed wastewater is also increasingly recycled for irrigation purposes, potentially increasing the contamination of agricultural land with antifungals not efficiently removed during treatment (Calderon-Preciado et al., 2011; Chen et al., 2011). Effluent from pharmaceutical factories (Liu and Wong, 2013; Assress et al., 2021a) and waste from industrial applications [e.g., anti-fouling paints (Andersson Trojer et al., 2013)] have also been highlighted as environmental sources of antifungals.
A further pathway for antifungals to enter the environment is the direct application of effect concentrations of azole fungicides to crops as PPPs, for instance metconazole or tebuconazole. Though most agricultural azoles are approximately 10-100 times less intrinsically active than their therapeutic counterparts (Gisi, 2014), the spraying of fungicides occurs regularly (Lago et al., 2014), with pesticide usage data indicating 1.3 million kg of triazole fungicides were applied to crops in the UK in 2016 (Garthwaite et al., 2018). Once present in natural environments, some antifungals may accumulate and persist for long periods of time (Bhagat et al., 2021).
AFR in environmental fungal communities has been documented and suggested to increase human exposure risk to AFR pathogens (Bader et al., 2015; Chen and Ying, 2015; Chowdhary and Meis, 2018; Assress et al., 2020; Jeanvoine et al., 2020; Fisher et al., 2022; Rhodes et al., 2022). However, little research has investigated the selective potential of antifungals for AFR at environmentally relevant concentrations. Without empirical data to interpret the selective risk of measured environmental concentrations (MECs) of antifungals, our ability to effectively inform safe environmental thresholds is severely limited.
Antifungal Monitoring, Risk Assessments and Selective Concentrations
The ‘traditional selective window’ hypothesis proposes that selection for resistance will only take place at antimicrobial concentrations above the minimum inhibitory concentration (MIC) of susceptible strains, and below the MIC of resistant strains (Gullberg et al., 2011; Murray et al., 2018). However, data for ABR have challenged this assumption, revealing selection at very low, sub-clinical concentrations in both single species and complex microbial communities (Gullberg et al., 2011; Gullberg et al., 2014; Liu et al., 2015; Le Page et al., 2017; Kraupner et al., 2018; Murray et al., 2018; Murray, 2020; Murray et al., 2020; Stanton et al., 2020). Hence, it is possible that AFR selection may also take place at concentrations below the MIC of susceptible fungi, but still at environmentally relevant concentrations.
European regulations dictate that an environmental risk assessment (ERA) for a pharmaceutical is required where the predicted environmental concentration (PEC) exceeds 10ng/L (Huschek et al., 2004; Le Page et al., 2017). Traditionally, an ERA will be based on ecotoxicological tests (e.g., reproductive toxicity (Bhagat et al., 2021)) that generate a No Observed Effect Concentration (NOEC). Predicted No Effect Concentrations (PNECs) can then be generated by dividing the NOEC by an assessment factor (Murray et al., 2021).
Ecotoxicological risks have been used to inform the European Water Framework Directive’s ‘Watch List’, where compounds of concern are included if the PEC or MEC frequently exceeds the PNEC (Cortes et al., 2020). Until recently, AMR endpoints have not been considered in such assessment frameworks, although antimicrobial selective concentrations are now considered in terms of ABR selection risk. Though there are ten antifungals on the most recent iteration of the Watch List (Cortes et al., 2020) (three clinical compounds: clotrimazole, fluconazole & miconazole; and seven agricultural compounds: enilconazole, ipconazole, metconazole, penconazole, prochloraz, tebuconazole & tetraconazole), AFR selection is not an endpoint that is currently considered within antifungal ERAs (e.g., for pesticides), and it is unclear whether existing ecotoxicological assays are protective of AFR selection at environmentally relevant concentrations (Le Page et al., 2017). Importantly, previous research on antibacterial drugs, often referred to as antibiotics, has shown that conventional ecotoxicological test data using traditional endpoints [e.g., neurotoxicity and developmental toxicity (Bhagat et al., 2021)] are not always protective of ABR selection (Bengtsson-Palme and Larsson, 2016; Le Page et al., 2017; Tell et al., 2019; Murray et al., 2020), raising concerns this may also be the case for AFR.
The threshold at which AFR or ABR strains are enriched in relation to susceptible strains is defined as the minimal selective concentration (‘MSC’). Gullberg et al. (2011) first conceptualised the experimental determination of the MSC for antibacterials, using a competition-based, single-species evolution assay. This identified that the MSC for antibacterials can be > 200 times lower than the MIC of the susceptible strain (Murray et al., 2021). There have been a number of noteworthy publications since this work, which were recently reviewed by Murray et al. (2021). However, there are no previous attempts to experimentally determine MSC data for antifungals, nor is there an established definition for an antifungal MSC. This review recommends the following definition: the lowest concentration of an antifungal at which positive selection for AFR occurs.
Though there are no experimental MSC data currently available for antifungals, PNECs for resistance (PNECRs) have been theoretically estimated for a number of key antifungal agents (Bengtsson-Palme and Larsson, 2016). These PNECR values were derived by dividing the lowest 1% of observed MICs obtained from the European Committee on Antimicrobial Susceptibility Testing (EUCAST) database by an assessment factor of 10, therefore accounting for the hypothesised differences between MICs and MSCs (Bengtsson-Palme and Larsson, 2016). This data has aided initial developments in assessing antifungal selective risk. Assress et al. (2021b) performed an elaborate monitoring assessment on eight azole antifungals in wastewater and surface waters in South Africa. Based on risk quotient [RQ; generated by dividing the MEC or PEC by the PNEC (Murray et al., 2021)] calculations, this work found that the MECs of antifungals did not pose a high risk to aquatic organisms (algae, daphnia and fish) using traditional ecotoxicity endpoints. However, using the PNECRs generated by Bengtsson-Palme and Larsson (2016), concentrations of fluconazole and itraconazole were found ‘to pose moderate to high risk for development of AFR’ (Assress et al., 2021b). This supports the concern that ecotoxicologically derived endpoints may not always be protective of resistance selection, and thus reinforces the importance of developing experimental tools to determine empirical MSC data for antifungals.
Commonalities and Contrasts Between Antifungal and Antibacterial Resistance
Whilst there are clear differences between bacterial and eukaryotic genetics and physiology, some elements of our understanding of ABR are likely to be transferable to AFR. Where possible, this review will compare and contrast AFR and ABR to establish experimental tools for the study of AFR, informed by methods that have previously been used to generate antibacterial MSC data.
Mode of Action
The mode of action (MoA) across the primary antifungal classes is similar (Figure 2) in consistently impairing cell structure and rigidity by interacting with either cell wall or cell membrane constituents. Azoles target the ergosterol biosynthesis pathway by inhibiting the enzyme lanosterol demethylase (LD), which is encoded by the erg11 gene in yeasts and the cyp51 gene in moulds (Morschhäuser et al., 2007; Chowdhary et al., 2014; Sagatova et al., 2015; Perlin et al., 2017). The LD enzyme is responsible for the synthesis of ergosterol, a key sterol constituent of fungal cell membranes (Odds et al., 2003; Chen and Ying, 2015; Moye-Rowley, 2020), meaning azole exposure results in ergosterol depletion (Hof, 2008). Polyenes act by binding to ergosterol, creating channels in the fungal cell membrane and killing cells by ‘allowing ions and other cellular components to escape’ (Perlin et al., 2017). Finally, echinocandin compounds inhibit the enzyme β-1-3-glucan synthase, which results in a depletion of glucans: an essential fungal cell wall component (Chaabane et al., 2019).
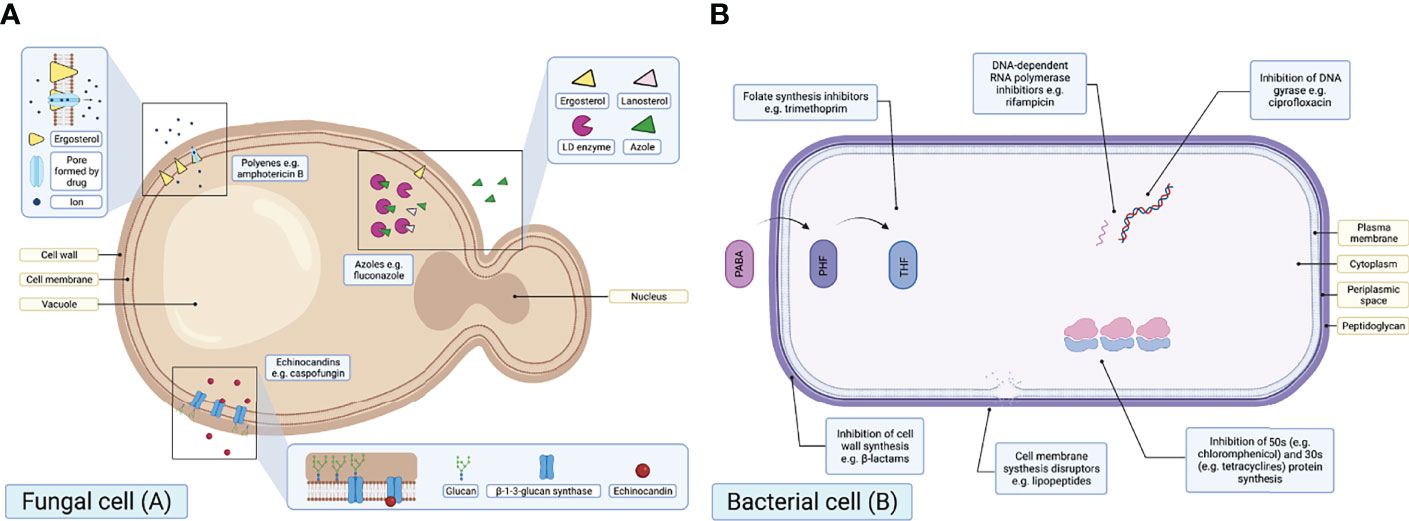
Figure 2 Schematic diagrams illustrating the MoAs of the primary antifungal drug classes in a fungal cell (A) and primary antibacterial drug classes in a bacterial cell (B). PABA, para-aminobenzoic; DHF, dihydrofolic acid; THF, tetrahydrofolic acid [adapted from Sanseverino et al. (2018)]. Created with BioRender.com.
Similar to antifungals, some antibacterials target the bacterial cell wall or membrane. However, unlike antifungals, there are additional antibacterial intracellular targets (Figure 2). One concern relating to AFR is that there are few therapeutic antifungal alternative drug targets, in comparison to the broad range of antibacterial drug targets available. As a result, resistance to one or more classes of antifungals drastically reduces viable treatment options for potentially life-threatening infections.
Mechanisms of Resistance
When considering mechanisms of resistance, both AFR and ABR may be either intrinsic or acquired (Ben-Ami et al., 2017; Perlin et al., 2017). Intrinsic resistance includes inherent resistance to antimicrobial drugs. For instance, Aspergillus spp. are intrinsically resistant to fluconazole (Azevedo et al., 2015). On the other hand, acquired resistance typically follows exposure to antimicrobial selective pressures, and may be caused by mutations, genome rearrangements and/or overexpression of resistance gene products (Lockhart et al., 2017; Chaabane et al., 2019; Berman and Krysan, 2020) (Figure 3).
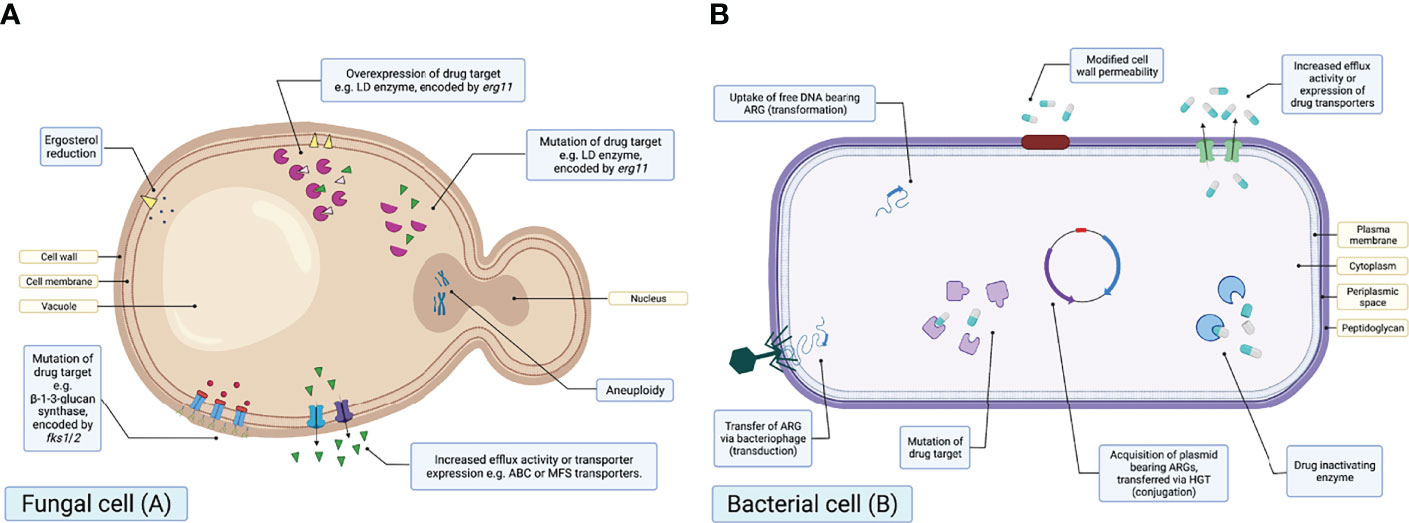
Figure 3 Schematic diagrams illustrating the known mechanisms of antifungal drug resistance in a fungal cell (A) and antibacterial resistance in a bacterial cell (B). Created with BioRender.com.
Many of the resistance mechanisms observed in fungi have also been documented in bacteria. For example, both AFR and ABR may be due to drug efflux, upregulation of resistance genes or mutations. Efflux pumps are transmembrane proteins able to actively transport drugs outside of the cell, thus reducing intracellular drug concentrations (Perlin et al., 2017; Chaabane et al., 2019; Jeanvoine et al., 2020). Overexpression involves the upregulation of genes, including erg11/cyp51A and genes encoding efflux transporters, resulting in overexpression of the proteins encoded by those genes. Finally, mutations in the amino acid sequence of drug targets can also result in resistance.
On the other hand, there are resistance mechanisms that are specific to either ABR or AFR. Mechanisms unique to AFR include changes in ergosterol synthesis and aneuploidy. For example, reduction in ergosterol synthesis via altered expression or mutation has been identified to result in resistance to polyenes, including amphotericin B (Young et al., 2003). Aneuploidy or aneuploids refer to cells exhibiting a chromosome number greater or less than the ‘normal’ number (Kwon-Chung and Chang, 2012), which has recently been associated with azole AFR (Sionov et al., 2010).
ABR mechanisms in bacteria may be described as either selfish or producing of ‘public goods’; referring to those that offer a community wide benefit. One of the most notably documented ABR mechanisms that produces public goods is the production of enzymes capable of degrading or inactivating antibacterials. This results in a rapid reduction in extracellular drug concentrations, allowing susceptible strains to persist in the presence of an antibacterial (Yurtsev et al., 2013; Bottery et al., 2016; Murray et al., 2018). This mechanism of ABR is widely documented, with extended spectrum beta-lactamase (ESBL) (Medaney et al., 2016) or carbapenemase producers shown to allow the growth of susceptible bacterial strains above their MIC following enzymatic degradation of antibacterial compounds (Murray et al., 2018). Fungal enzymes able to degrade antifungals have not yet been identified (Hof, 2008), therefore it could be argued that AFR mechanisms are less likely to produce ‘public goods’. Indeed, given that drug efflux is one of the predominant mechanisms of AFR and the efflux of antifungal compounds may increase extracellular drug concentrations, AFR mechanisms are more likely to be selfish than ABR mechanisms. It could also be suggested that, in comparison to bacterial communities harbouring ESBL producers, increased extracelluar antifungal concentrations resulting from drug efflux may drive further AFR evolution in fungal communities, particularly owing to the prolonged half-life of some antifungal agents (Hill et al., 2015).
Microbial communities may also become resistant to antimicrobial therapy due to phenotypic mechanisms, such as biofilm formation. As is observed with bacterial biofilm-forming phenotypes (Perlin et al., 2017), fungal biofilm communities have also been found to show increased levels of antifungal resistance. For example, Candida species may attach to various surfaces to form a community of sessile cells encapsuled in an extraceullar matrix (Berman and Krysan, 2020). This complex, glucan-rich matrix is able to sequester drugs e.g., fluconazole or reduce penetration of antifungal compounds, thus reducing antifungal exposure. In contrast to fungal biofilms, however, bacterial biofilms have been found to promote the horizontal gene transfer (HGT) of antibacterial resistance genes (ARGs), due to the greater proximity of individuals in the biofilm (Arias-Andres et al., 2018).
It is key to note that conjugation, transduction and transformation are not mechanisms of resistance, but rather the three key pathways for the HGT of mobile genetic elements (MGEs) bearing ARGs (Figure 3). These are also unique to bacteria and there is currently no evidence to suggest this can occur within fungal communities.
Evolution of Resistance
The longstanding assumption of AMR is that the evolutionary selective pressure i.e., antibacterial or antifungal concentration must be sufficient to offset any incurred fitness cost of resistance (Melnyk et al., 2015), and the maintenance of resistance in a population is dependent upon fitness cost in the absence of the drug (Anderson, 2005; Gagneux et al., 2006; MacLean et al., 2010; Gullberg et al., 2014; Hill et al., 2015). This implies that resistance always has a fitness cost. Generally, ABR is associated with a fitness cost (Andersson and Hughes, 2010). For example, the alteration of an antibacterial target may impair its function (Enne et al., 2005). However, fitness costs may be negated by subsequent compensatory mutations (Baquero, 2001; Enne et al., 2005; Andersson and Hughes, 2012), and resistance genes that confer a fitness advantage have also been identified (Michon et al., 2011).
Comparatively less is known about the fitness costs of AFR in fungi, though there is evidence that, as with ABR, further evolution may ameliorate incurred fitness costs via compensatory mutations (Cowen et al., 2002; Barker et al., 2004; Anderson, 2005; Morschhäuser et al., 2007; Hof, 2008; Sharma et al., 2015; Morschhauser, 2016; Verweij et al., 2016). In rare cases, overexpression of resistance determinants, such as efflux transporters, have led to a gain in fitness in the presence and absence of a drug (Guo et al., 2017). This is because many drug transporters are not specific to antifungals and so intracellular concentrations of other toxic compounds may also be reduced (Hof, 2008). Similarities may be drawn from this to co-selection of ABR in bacteria. Co-selection involves the indirect selection for ABR through the presence of multiple ARGs on a MGE (co-resistance) or where a single gene confers for resistance to multiple compounds (cross-resistance), for example, drug efflux. Finally, it could also be argued that fungal ploidy may have an effect on the fitness cost or maintenance of AFR in fungi. For example, a mutation on one chromosome may decrease susceptibility, whilst an unmutated gene on another chromosome may reduce associated fitness costs by retaining the original function of the gene.
There are clear differences in the evolution of resistance between fungi and bacteria (Figure 3). One of the most obvious differences is the absence of fungal capacity to readily take up or horizontally transfer exogenous DNA, such as plasmids (Hof, 2008; Azevedo et al., 2015). This suggests resistance development in fungal communities may be more gradual, as opposed to the ‘explosive expansion of resistance’ observed in bacteria, which may acquire multiple resistance mechanisms in one evolutionary step (Hof, 2008). Nevertheless, fungi have been described as ‘evolvable’ (Cowen et al., 2002), owing to the large number of genes encoding for resistance mechanisms (Berman and Krysan, 2020). For example, there are 30 known genes for ATP binding cassette (ABC) transporters present in the Saccharomyces cerevisiae genome, increasing the probability for resistance mutations to arise (Cowen, 2001; Hof, 2001). Moreover, fungal genomes (e.g., S. cerevisiae: 12,068kb) are significantly larger than bacterial genomes (e.g., Escherichia coli: 4,640kb), a factor that has been suggested to increase the likelihood of genetic mutations arising that confer AFR (Cowen et al., 2002).
Proposed Methods to Determine AFR Selective Concentrations
Recent work by Murray et al. (2021) synthesised and critically appraised the methodologies previously described to determine antibacterial MSC data. From this, benefits and weaknesses in such assays were highlighted, all previous MSC data for antibacterials were collated and a novel AMR ERA framework able to identify compounds posing the greatest selection risk was proposed. Using the novel ERA framework outlined by Murray et al. (2021), antibacterial classes of high priority (in terms of generating PNECR data and assessing selection risk) were identified; namely, quinolones, cephalosporins, beta-lactams and sulphonamides. This work exemplifies the importance of having both validated methods to determine MSC data, and a streamlined framework to incorporate selection risk posed by antimicrobial compounds. To determine selection risk of environmentally relevant antifungal concentrations, experimental methods specific for antifungals must be designed and is the key focus of this section. All proposed methods are summarised in Figure 4.
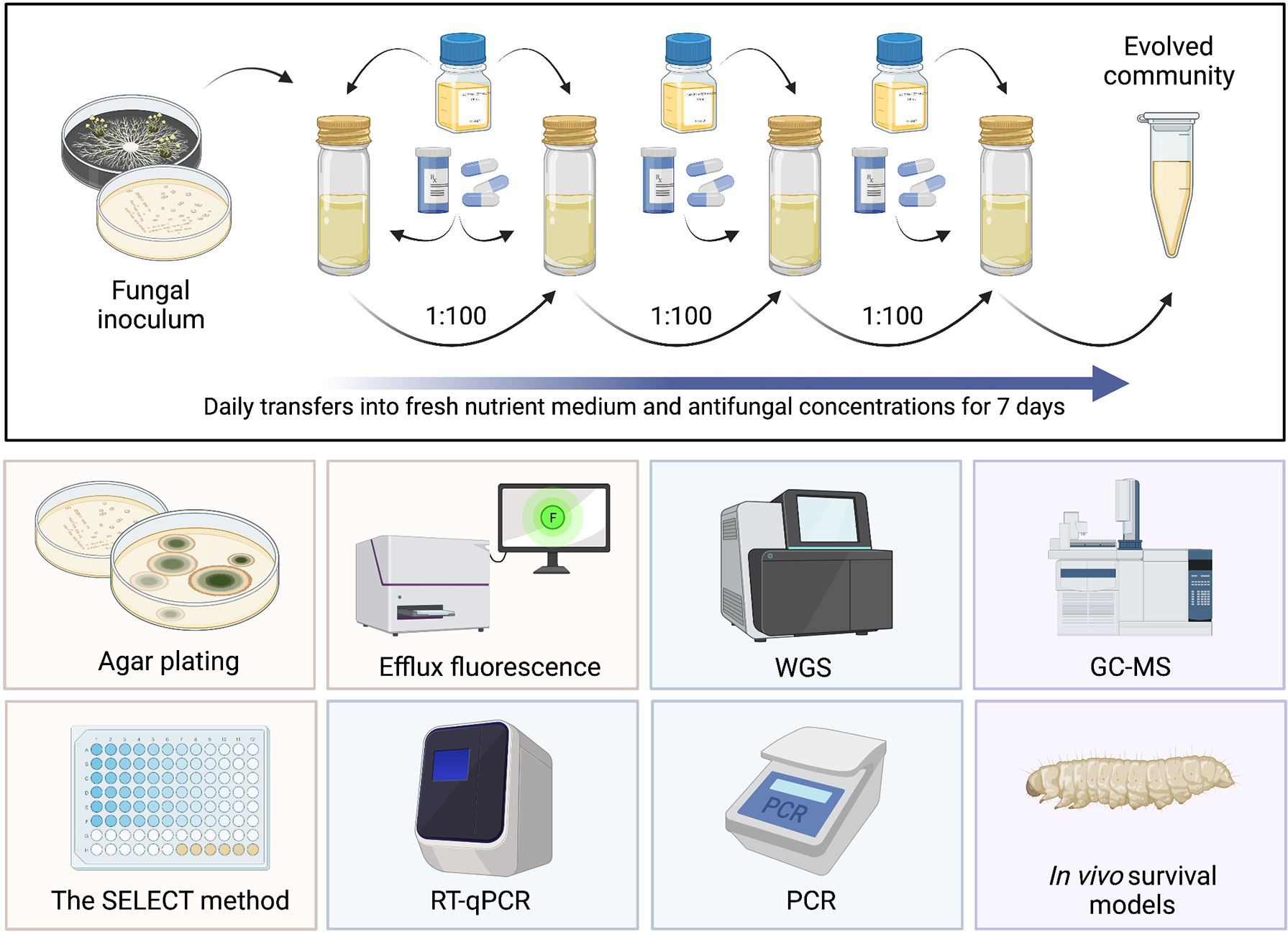
Figure 4 Summary of proposed assays to generate antifungal MSC data. Top: serial culture of a fungal community exposed to different antifungal concentrations for a period of 7 days, with daily transfers into fresh nutrient medium and antifungal concentrations. Yellow: phenotypic methods, blue: genotypic methods, purple: methods requiring further investigation. RT-qPCR, reverse transcription quantitative polymerase chain reaction; WGS, whole genome sequencing; PCR, polymerase chain reaction; GC-MS, gas chromatography mass spectroscopy. Created with BioRender.com.
Phenotypic Assays
Phenotypic, culture-based assays used to determine antibacterial MSCs could potentially be modified and applied to antifungal agents. For example, Kraupner et al. (2018) established selective concentrations for ciprofloxacin (CIP) resistance in a sewage-effluent bacterial community. In summary, E. coli inoculated into low-nutrient medium were supplemented with and without incremental concentrations of CIP for 24 hours. Cultures were then diluted and re-inoculated with fresh medium and CIP at the same concentration after 24 hours. At 0, 24 and 48 hours aliquots of each culture were plated on chromogenic agar, supplemented with or without CIP, and colony forming units (cfu) were enumerated following incubation. Selective concentrations were defined where relative resistance (calculated by comparing cfu counts of control and CIP supplemented plates) was significantly different from the no antibacterial evolved control (Kraupner et al., 2018).
To enable application for antifungals, this method requires clear modifications, such as adoption of a fungal selective agar and specific nutrient medium (Assress et al., 2021b). In comparison with single-celled bacteria, fungal growth and morphology is more complex, particularly in moulds. It is important to note that there are key differences in the growth, structure and function of yeast cells in comparison to moulds. These differences impact the organism’s ability to evolve and maintain AFR, and therefore impact our ability to quantify resistance selection. Similarities between yeast and bacteria may be drawn upon, as they are both unicellular, undergo asexual reproduction and have typically short doubling times. This means the application of methods [including those described by Kraupner et al. (2018)] may be suited to testing antifungal selective effects against yeast cultures. Though, previous work has successfully exposed conidial suspensions of filamentous moulds, such as A. fumigatus, to antifungals at different concentrations before plating on selective agar (Assress et al., 2021b). This suggests there may be scope to test antifungal effects against both yeasts and moulds. Necessary modifications for moulds may include increasing agar plate incubation time, which would significantly increase the duration of the assay. For example, Assress et al. (2021b) suggest a plate incubation time of 7-10 days.
There may be opportunity to detect cross-resistance to multiple antifungal agents via this method. For example, 4-well plates containing minimum inhibitory breakpoint concentrations of itraconazole, posaconazole and voriconazole with an additional drug-free control have recently been developed and validated for A. fumigatus susceptibility testing [VIPcheck, Nijmegen, Netherlands (Arendrup et al., 2016)]. Resistance to compounds is confirmed where growth is detected in drug-containing wells. This would increase the number of compounds that could be tested and would reveal more environmentally relevant data, as environmental fungal communities are exposed to a diverse mixture of compounds in wastewater and aquatic systems. As stated, this method is currently only validated for A. fumigatus, however, application to yeasts may also be possible, provided appropriate antifungal breakpoints and selective media are adopted.
The SELECT Method
A novel assay has recently been published that enables the rapid and cost-effective determination of antibacterial MSCs: the ‘SELection Endpoints in Communities of baCTeria’ (SELECT) method (Murray et al., 2020). In brief, the SELECT method exposes wastewater-derived complex communities of bacteria to a gradient of antibacterial concentrations (Figure 5). This generates selective concentrations that can be used to derive PNECRs, using a significant reduction in bacterial community growth as a proxy for selection for AMR (Murray et al., 2020).
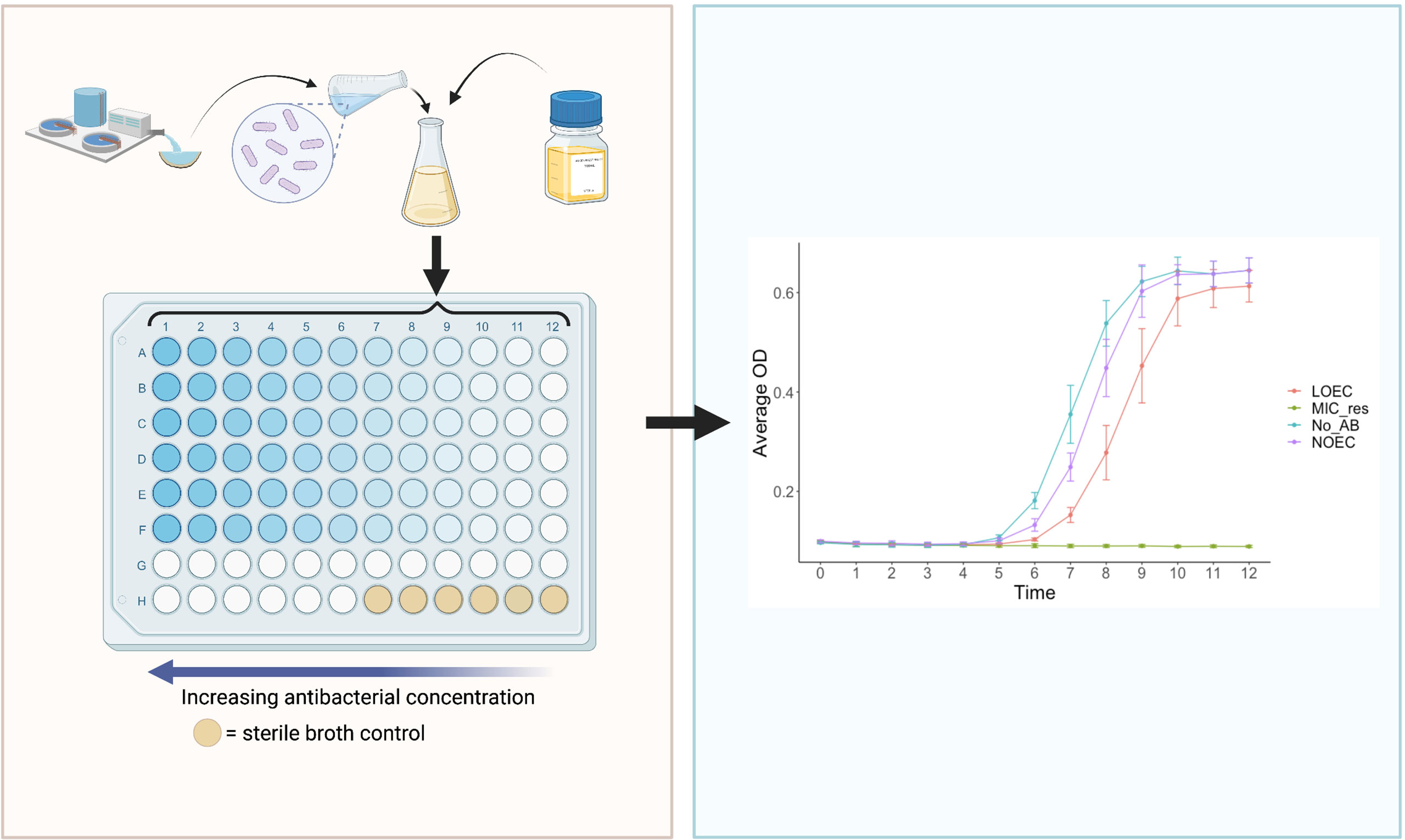
Figure 5 The ‘SELection End points in Communities of bacTeria’ (SELECT) Method (Murray et al., 2020). Left: a schematic overview of the SELECT assay; a 96-well-plate containing Iso-Sensitest broth inoculated with a complex sewage community, exposed to two-fold dilutions of an antibacterial. Right: an expected graphical output of the SELECT method, showing complex bacterial community growth curves in response to different concentrations of antibacterials. The LOEC is identified where the growth of the community is significantly lower than the no-antibacterial control, at the time point which exhibits the strongest dose-response relationship. LOEC, Lowest Observed Effect Concentration; NOEC, No Observed Effect Concentration; MIC_res, minimum inhibitory concentration of resistant strains, No_AB, No Antibacterial control. Created with BioRender.com.
The hypothesis that community growth may be used as a proxy for ABR selection in bacteria was first proposed in the initial antibacterial MSC work from Gullberg et al. (2011). The findings from this study indicated that the MSC can be derived using selection coefficient data points to identify where the ‘numbers of resistant bacteria are expected to increase over time, relative to non-resistant bacteria’ (Murray et al., 2021). In addition, a recent mathematical model identified that a loss in net bacterial growth, especially of susceptible strains, was found to be the most sensitive for MSC predictions (Greenfield et al., 2018).
The evidence to support if reduced growth can be used as a proxy for AFR in fungi is sparse, due to a lack of experimental research. However, broth microdilution antifungal susceptibility testing (AFST) is common, and these methods follow a similar protocol to the SELECT method. For example, susceptibility profiles were previously determined across a range of C. albicans growth rates, measured by taking optical density (OD) measurements (Baillie and Douglas, 1998). Given the lack of further evidence, it is crucial to consider accompanying or alternative assays to empirically determine if observed growth reduction in a SELECT-type assay is associated with selection for known resistance determinants.
Initial considerations to enable use of antifungals using the SELECT method include changes to experimental inoculum and conditions. Use of a sewage derived inoculum in the original SELECT method is advantageous as the sewage microbiome is ‘representative of both the human gut, hospital effluent and WWTP influent’ (Murray, 2017). Importantly, this provides a diverse community of bacteria and resistance mechanisms for selection to act upon, including de novo mutations and MGEs that can be transferred between bacteria via HGT. For fungi, this may be less important as HGT does not occur and most AFR will be conferred by mutation or upregulation of resistance genes. Therefore, though evidence suggests fungal communities are an important component of the sewage microbiome (Whaley et al., 2016; Niu et al., 2017; Zhang et al., 2018; Chaabane et al., 2019; Bhattacharya et al., 2020), initial modifications of the SELECT method should consider focussing on a single-species yeast inoculum e.g., Candida spp. A weakness in restricting experimental inocula to a single-species is that this significantly reduces environmental realism (Bengtsson-Palme and Larsson, 2016; Le Page et al., 2017; Murray et al., 2020; Murray et al., 2021), as microorganisms predominantly live in complex natural communities (Van Dijck et al., 2018; Assress et al., 2019). At the same time, there are strengths in single species assays as they are less complex, allowing greater control over test organisms [e.g., ploidy (Cowen et al., 2002)] and may generate data with lower variance. One solution to improving environmental realism of a single species assay is to use strains of the sample species with variable susceptibility profiles, such as the artificial mix of E. coli strains previously utilised by Kraupner et al. (2021). This will account for competition between different strains and is therefore likely more representative of ‘real world scenarios’.
Genotypic Assays
Whilst phenotypic assays are often more cost effective and provide a community-wide representation of resistance, genotypic methods have been previously employed to generate MSC data for both single species and community based bacterial assays by targeting well characterised resistance determinants. For example, the MSCs determined by the SELECT method were initially validated against a previously published, longer-term genotypic assay (Murray et al., 2018; Murray et al., 2020; Stanton et al., 2020) using quantitative polymerase chain reaction (qPCR) to quantify prevalence of key resistance genes. This method serially cultured sewage bacterial communities for a total of 7 days in the presence of different concentrations of a test antibacterial, and effect concentrations were determined where target gene prevalence was significantly greater than the no-antibacterial control. Exposing mixed bacterial communities to different antibacterials and using genotypic assays to target known resistance determinants provides an established experimental framework that may be modified to target known AFR determinants in fungi.
The two primary resistance mechanisms observed in AFR include the inhibition of target access e.g., overexpression of efflux pumps and target modification e.g., mutations (Figure 3).
Overexpression
Upregulation of genes will result in overexpression of the proteins encoded by those genes e.g., efflux pumps (Figure 3). Selective effect concentrations of antifungals could be determined where expression levels are significantly different to basal control levels.
Methods to profile expression (including reverse transcription qPCR (RT-qPCR)), using messenger ribonucleic acid (mRNA), are extremely sensitive and have previously been used to quantify AFR determinant expression levels. For example, using RT-qPCR, Flowers et al. (2012) investigated erg11 regulation in fluconazole-resistant versus susceptible C. albicans isolates, identifying that in 75% of test fluconazole-resistant isolates, erg11 was upregulated by at least two-fold compared to unrelated susceptible strains. Efflux protein expression has also been quantified using RT-qPCR, with one study finding efflux expression levels were consistent among susceptible isolates of Nakaseomyces glabrata, but with a three-fold increase in fluconazole resistant isolates (Gygax et al., 2008). These methods are significantly more expensive and time consuming relative to phenotypic assays, and variable results between laboratories may be generated due to the multiple RT-qPCR enzymes and oligonucleotides commercially available (Valasek and Repa, 2005). In addition, the reliability of RT-qPCR-based measurements are dependent on normalisation, typically using an internal-control housekeeping or reference gene [reviewed in Paul et al. (2021)].
As an accompanying validation or alternative assay, there are less costly phenotypic assays available to quantify efflux pump activity. The general principle behind such assays involves the addition of fluorescent dyes to a cell suspension, allowing the kinetics of efflux to be measured. An increase in efflux pump activity is indicated where an increase in fluorescence is observed. Bhattacharya et al. (2020) evaluated C. albicans efflux pump activity in association with ABC and major facilitator superfamily (MFS) transporter overexpression, using the straightforward and cost-effective fluorescence assays: alanine-β-naphthylamide (Ala-Nap) and rhodamine 6G (R6G). A. fumigatus transporter activity can also be determined using the same protocol outlined for R6G (Nakamura et al., 2001). Following the batch microcosm experimental guidelines, MSCs can be determined where efflux activity, conferred by increased fluorescence, is significantly greater than no antifungal controls.
Mutation
Genetic mutations play an important role in AFR and recent advancements in sequencing technologies have allowed exploration of fungal genomes, enabling the identification of important AFR genes and target site mutations (Ball et al., 2020). Vincent et al. (2013) used whole genome sequencing (WGS) to identify mutations conferring amphotericin B resistance in C. albicans isolates. Though more time-consuming and expensive than many of the other methods discussed, sequencing remains the ‘gold standard’ for mutation detection (Zhao et al., 2016; Paul et al., 2021) and may be used to investigate AFR selection by comparing sequencing data of control strains and evolved isogenic strains following exposure to a range of antifungal concentrations. There are also other more specific methods available to detect mutations conferring AFR. For instance, in addition to upregulation of erg11, mutations in this gene can confer azole resistance in yeasts. Tetra-primer-amplification refractory mutation system-PCR (T-ARMS-PCR), restriction site mutation (RSM), and high-resolution melt (HRM) analysis methods are some of the molecular tools available to determine resistance caused by erg11 polymorphisms. Briefly, T-ARMS-PCR and HRM are used for single-nucleotide polymorphism (SNP) genotyping (Etlik et al., 2011; Caban et al., 2016). The RSM assay detects mutations using restriction enzymes and has only been adopted once to detect erg11 mutations (Steingrimsdottir et al., 1996; Paul et al., 2021). In a recent review (Paul et al., 2021) comparing these assays, the T-ARMS-PCR and RSM approaches were deemed marginally more sensitive in discriminating resistant and susceptible isolates than HRM analysis.
Mutations to the cyp51A gene are the most commonly reported mechanism of azole resistance in A. fumigatus (Perlin et al., 2017; Ren et al., 2017), present in over 90% of clinical azole-resistant isolates (Verweij et al., 2009). This is thought to have been driven by the widespread application of azole fungicides in agriculture (Bader et al., 2015). Specifically, tandem repeats (TRs) in the cyp51A promoter region are frequently observed in environmental azole resistant strains (Arai et al., 2020). The most common of these include a TR sequence of 34 base pairs with L98H mutations (TR34/L98H) and a TR sequence of 46 base pairs with Y121F/T289A mutations (TR46/Y121F/T289A) (Bader et al., 2015; Arai et al., 2020). Bader et al. (2015) PCR-amplified and sequenced the cyp51A gene of 55 resistant A. fumigatus isolates and the majority of resistant isolates (80%) harboured the TR34/L98H allele, with TR46/Y121F/T289A variants the second most frequently observed. Given that these are known mutation variants, qPCR primers and probes can be used to quantify the prevalence of such variants in a sample. Again, by following similar experimental guidelines as those outlined by Murray et al. (2020), selective concentrations may be determined where mutation variant prevalence is significantly greater than no antifungal controls.
Areas for Further Testing
The adaptation of existing experimental tools to generate antifungal MSC data is promising, however, there may also be additional assays that are able to achieve this that have not yet been tested, including the use of reduced ergosterol content or virulence as a proxy for AFR. It should be noted that these suggestions are hypothetical and would require additional testing before application.
Ergosterol Content Quantification
Resistance to azole compounds frequently occurs due to the upregulation of erg11 and cyp51A genes, resulting in changes to ergosterol concentration. Evidence recently provided by Ballard et al. (2019) suggested that azole resistance is associated with decreased ergosterol content in A. fumigatus fungal membranes, as measured by gas chromatography-mass spectrometry. If changes in ergosterol content can be used as a proxy for resistance selection, ergosterol quantification assays could be modified to detect selective endpoints of antifungals. Following the experimental evolution of fungal strains in the presence of difference antifungal concentrations, AFR selection may be observed at the antifungal concentration where ergosterol in the fungal cell membrane decreases significantly below the control (i.e., no antifungal present) - analogous to that conferred by a reduction in growth in the SELECT method.
Virulence
It is postulated that acquired AFR may result in reduced virulence (Hill et al., 2015; Ben-Ami et al., 2017), although, this is still under investigation. To previously investigate this, the invertebrate host model Galleria mellonella was employed and a correlation between MIC and virulence (conferred by fatal outcome) was calculated (Borghi et al., 2014). This work concluded that, owing to a similar rate of killing across resistant isolates with varying susceptibility patterns of N. glabrata, increased resistance did not influence virulence. However, opposing evidence suggests AFR may impose an associated virulence cost (Hill et al., 2015; Ben-Ami et al., 2017).
If reduced virulence can be used as a proxy for AFR, the G. mellonella model could be modified to enable detection of antifungal selection endpoints. Fungal cell suspensions exposed to different antifungal concentrations could be injected into the G. mellonella haemocoel, and by using mortality as a measure of virulence and reduced virulence as a proxy for resistance, AFR selection could be identified where rate of killing is significantly lower than the no-antifungal control. Mini-host model assays, including G. mellonella, have advantages such as their cost and simplicity allowing greater replication of larvae injection (Borghi et al., 2014). In addition, this model invertebrate has been utilised for a variety of Candida species, including C. albicans (Cotter et al., 2000; Brennan et al., 2002), C. parapsilosis (Gago et al., 2014), C. orthopsilosis (Gago et al., 2014), C. metapsilosis (Gago et al., 2014), C. tropicalis (Mesa-Arango et al., 2013) and C. krusei (Scorzoni et al., 2013).
Discussion: Comparison of Proposed Methods
The methods outlined here have the potential to address the scarcity of antifungal MSC data, but there are strengths, weaknesses, opportunities and threats specific to the different methods, which are outlined in Table 1. There are also advantages and disadvantages that are shared across these methods, which will be outlined in this section.
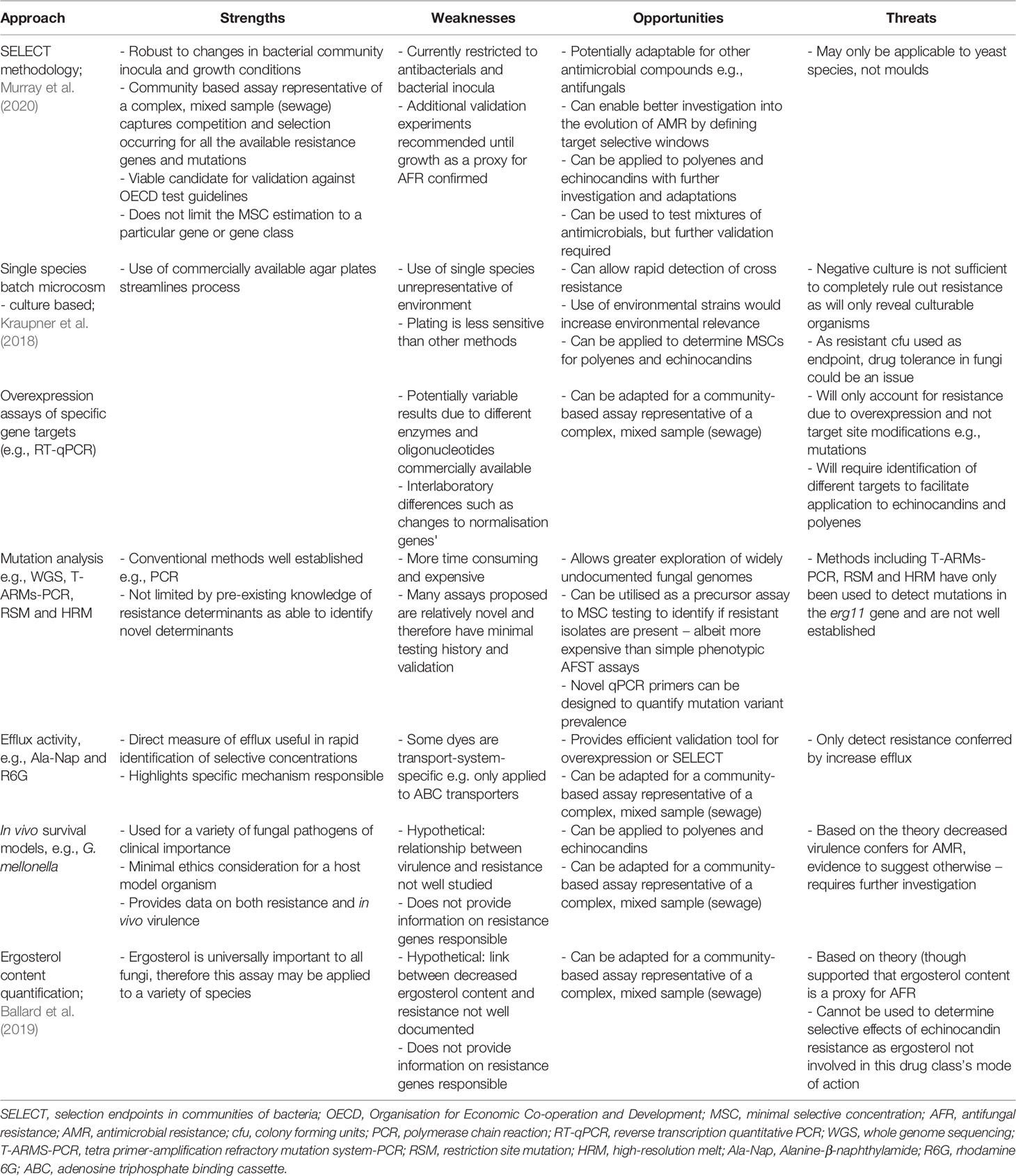
Table 1 Strengths, weaknesses, opportunities and threats (S.W.O.T) analysis of proposed assays [adapted from Murray et al. (2021)].
Phenotypic assays are inexpensive, allowing the rapid generation of highly replicable data. Moreover, phenotypic methods do not require a priori knowledge of resistance mechanisms present and can encompass all responsible resistance determinants in the test culture, therefore providing a population-wide representation of resistance selection.
On the other hand, genotypic assays are extremely sensitive and offer accurate measures of specific resistance mechanisms. Though, genotypic assays can usually only analyse one target, with each additional target adding further time and expense, which could compromise data quality.
An overall benefit is that all of the proposed methods can be used for both yeast and mould species, except the SELECT method which would require additional testing and modifications. A key concern for all methods described, however, is that they are limited to laboratory culturable microorganisms, with evolution experiments currently conducted under optimum laboratory conditions (e.g., high temperatures and nutrients), which are not representative of the natural environment and may lead to the possible under or overestimation of MSCs. Importantly though, nutrient and temperature conditions can be modified to improve environmental realism. Further to this, there is concern of high intrinsic resistance in many fungal species, so drug-bug combinations used with these assays must be specific. Finally, the testing of single antimicrobials does not account for complex antimicrobial mixtures and co-selection, which has been found to be of importance in environmental settings.
Conclusion
The extent of antifungal exposure in the environment and its impact on AFR is understudied, and ERA guidelines do not require assessment of antifungals in terms of their ability to drive AFR development. Due to the incomplete removal or inactivation of antifungals during wastewater treatment and the direct application of effect antifungal concentrations to agricultural crops, the environmental dimension of AFR warrants greater attention. With fungal diseases predicted to become more common due to climate change and increasing human populations, with no effective alternatives to azole fungicides currently available, it is critical to monitor and limit these impacts to secure future food security, healthcare and the global economy. Without considering resistance selection alongside traditional ecotoxicity endpoints, the risk of AFR emergence is not currently effectively assessed and novel assays are required to generate such data. Likewise, without the ability to generate and interpret MSC data for antifungals of concern, our understanding of AFR evolution, in both the clinic and the environment, is limited.
This review describes methods that could prove valuable in addressing the lack of antifungal MSC data. Following a detailed comparison of such methods, the SELECT method is highlighted as a unique and valuable tool to determine MSC values for antibacterials and is the most cost-effective and least labour-intensive experimental option to generate antifungal MSC data, should the proposed modifications allow antifungal application. An important, initial modification proposed is the adoption of a single species system, consisting of strains with varied susceptibility profiles, rather than the mixed sewage community used in the original antibacterial SELECT method. This, in combination with the other adaptations proposed, should simplify antifungal application, account for the marked differences between the evolution of antimicrobial resistance in bacteria and fungi, and considers the key differences in eubacterial genetics and growth versus eukaryotic physiology and competition. In addition, this method provides a promising tool for future adaptations, including the use of mixed compounds or other antifungal classes besides azoles. The remaining experimental assays outlined in this review offer valuable alternatives to aid the generation of novel antifungal MSC data or may also be used as validation tools to support the application of the SELECT method for antifungals and fungi. The proposed SELECT method could also be used in combination with the other methods described, whereby the SELECT method may indicate if resistance selection has taken place, accounting for all resistance mechanisms (i.e. not limited to a particular gene or gene class), then further methods could be used to investigate specific mechanisms where relevant or necessary. This work and the generation of antifungal MSC data will significantly improve our ability to inform release limits and risk assessment of antifungal use in our wider environment.
Data Availability Statement
The original contributions presented in the study are included in the article/supplementary material. Further inquiries can be directed to the corresponding author.
Author Contributions
ES wrote the main manuscript text with AM. ES and JU prepared the figures, and all authors reviewed them. All authors conceptualised the project, reviewed the manuscript and performed the literature validation. WG, HW and AM were responsible for project administration. WG, AH and AM led the funding acquisition. AM supervised the project. All authors contributed to the article and approved the submitted version.
Funding
ES and AM were supported by a NERC Industrial Innovation Fellowship (NE/R01372X/1). WG was supported by NERC Knowledge Exchange Fellowships (NE/S006257/1 and NE/V019279/1). NG and AW are supported by the MRC Centre for Medical Mycology (grant MR/N006364/2). JU is funded by a BBSRC Discovery Fellowship BB/W009625/1. Funding for this work was provided by the Environment Agency through project SC200011. NG also acknowledges support from the Wellcome Trust [Senior Investigator (101873/Z/13/Z), Collaborative (200208/A/15/Z and 215599/Z/19/Z) awards.
Conflict of Interest
The authors declare that the research was conducted in the absence of any commercial or financial relationships that could be construed as a potential conflict of interest.
Publisher’s Note
All claims expressed in this article are solely those of the authors and do not necessarily represent those of their affiliated organizations, or those of the publisher, the editors and the reviewers. Any product that may be evaluated in this article, or claim that may be made by its manufacturer, is not guaranteed or endorsed by the publisher.
Acknowledgments
Thank you to the University of Exeter’s AMR network (http://www.exeter.ac.uk/amr/) for support, scientific guidance and advice throughout the project. Thank you to Emma Lamb for thorough proofreading and reviewing drafts. For the purpose of open access, the authors have applied a ‘Creative Commons Attribution (CC BY) to any Author Accepted Manuscript version arising.
This study did not generate any new data.
References
Anderson J. B. (2005). Evolution of Antifungal-Drug Resistance: Mechanisms and Pathogen Fitness. Nat. Rev. Microbiol. 3 (7), 547–556. doi: 10.1038/nrmicro1179
Andersson D. I., Hughes D. (2010). Antibiotic Resistance and Its Cost: Is it Possible to Reverse Resistance? Nat. Rev. Microbiol. 8 (4), 260–271. doi: 10.1038/nrmicro2319
Andersson D. I., Hughes D. (2012). Evolution of Antibiotic Resistance at Non-Lethal Drug Concentrations. Drug Resist. Update 15 (3), 162–172. doi: 10.1016/j.drup.2012.03.005
Arai T., Majima H., Watanabe A., Kamei K. (2020). A Simple Method To Detect Point Mutations in Aspergillus Fumigatus Cyp51a Gene Using a Surveyor Nuclease Assay. Antimicrob. Agents Chemother. 64 (4), e02271–19. doi: 10.1128/AAC.02271-19
Arendrup M. C., Meletiadis J., Mouton J. W., Guinea J., Cuenca-Estrella M., Lagrou K., et al. (2016). EUCAST Technical Note on Isavuconazole Breakpoints for Aspergillus, Itraconazole Breakpoints for Candida and Updates for the Antifungal Susceptibility Testing Method Documents. Clin. Microbiol. Infect. 22 (6), 571 e571–574. doi: 10.1016/j.cmi.2016.01.017
Arias-Andres M., Klümper U., Rojas-Jimenez K., Grossart H.-P. (2018). Microplastic Pollution Increases Gene Exchange in Aquatic Ecosystems. Environ. pollut. 237, 253–261. doi: 10.1016/j.envpol.2018.02.058
Ashbolt N. J., Amezquita A., Backhaus T., Borriello P., Brandt K. K., Collignon P., et al. (2013). Human Health Risk Assessment (HHRA) for Environmental Development and Transfer of Antibiotic Resistance. Environ. Health Perspect. 121 (9), 993–1001. doi: 10.1289/ehp.1206316
Assress H. A., Nyoni H., Mamba B. B., Msagati T. A. M. (2020). Occurrence and Risk Assessment of Azole Antifungal Drugs in Water and Wastewater. Ecotoxicol. Environ. Saf. 187, 109868. doi: 10.1016/j.ecoenv.2019.109868
Assress H. A., Selvarajan R., Nyoni H., Mamba B. B., Msagati T. A. (2021a). “Antifungal Azoles and Azole Resistance in the Environment: Current Status and Future Perspectives—a Review,” in Reviews in Environmental Science and Bio/Technology. 20 (4), 1011–1041. doi: 10.1007/s11157-021-09594-w
Assress H. A., Selvarajan R., Nyoni H., Ntushelo K., Mamba B. B., Msagati T. A. M. (2019). Diversity, Co-Occurrence and Implications of Fungal Communities in Wastewater Treatment Plants. Sci. Rep. 9 (1), 14056. doi: 10.1038/s41598-019-50624-z
Assress H. A., Selvarajan R., Nyoni H., Ogola H. J. O., Mamba B. B., Msagati T. A. M. (2021b). Azole Antifungal Resistance in Fungal Isolates From Wastewater Treatment Plant Effluents. Environ. Sci. Pollut. Res. Int. 28 (3), 3217–3229. doi: 10.1007/s11356-020-10688-1
Azevedo M. M., Faria-Ramos I., Cruz L. C., Pina-Vaz C., Rodrigues A. G. (2015). ). Genesis of Azole Antifungal Resistance From Agriculture to Clinical Settings. J. Agric. Food Chem. 63 (34), 7463–7468. doi: 10.1021/acs.jafc.5b02728
Bader O., Tunnermann J., Dudakova A., Tangwattanachuleeporn M., Weig M., Gross U. (2015). Environmental Isolates of Azole-Resistant Aspergillus Fumigatus in Germany. Antimicrob. Agents Chemother. 59 (7), 4356–4359. doi: 10.1128/AAC.00100-15
Baillie G. S., Douglas L. J. (1998). Effect of Growth Rate on Resistance of Candida Albicans Biofilms to Antifungal Agents. Antimicrob. Agents Chemother. 42 (8), 1900–1905. doi: 10.1128/AAC.42.8.1900
Ballard E., Weber J., Melchers W. J. G., Tammireddy S., Whitfield P. D., Brakhage A. A., et al. (2019). Recreation of In-Host Acquired Single Nucleotide Polymorphisms by CRISPR-Cas9 Reveals an Uncharacterised Gene Playing a Role in Aspergillus Fumigatus Azole Resistance via a Non-Cyp51a Mediated Resistance Mechanism. Fungal Genet. Biol. 130, 98–106. doi: 10.1016/j.fgb.2019.05.005
Ball B., Langille M., Geddes-McAlister J. (2020). Fun(gi)omics: Advanced and Diverse Technologies to Explore Emerging Fungal Pathogens and Define Mechanisms of Antifungal Resistance. mBio 11 (5), e01020–20. doi: 10.1128/mBio.01020-20
Baquero F. (2001). Low-Level Antibacterial Resistance: A Gateway to Clinical Resistance. Drug Resist. Update 4 (2), 93–105. doi: 10.1054/drup.2001.0196
Barker K. S., Crisp S., Wiederhold N., Lewis R. E., Bareither B., Eckstein J., et al. (2004). Genome-Wide Expression Profiling Reveals Genes Associated With Amphotericin B and Fluconazole Resistance in Experimentally Induced Antifungal Resistant Isolates of Candida Albicans. J. Antimicrob. Chemother. 54 (2), 376–385. doi: 10.1093/jac/dkh336
Barnes R. A., Gow N. A., Denning D. W., May R. C., Haynes K., British Society of Medical M. (2014). Antifungal Resistance: More Research Needed. Lancet 384, 1427. doi: 10.1016/S0140-6736(14)61861-4
Ben-Ami R., Berman J., Novikov A., Bash E., Shachor-Meyouhas Y., Zakin S., et al. (2017). Multidrug-Resistant Candida Haemulonii and C. Auris, Tel Aviv, Israel. Emerg. Infect. Dis. 23 (2), 195. doi: 10.3201/eid2302.161486
Bengtsson-Palme J., Larsson D. G. (2016). Concentrations of Antibiotics Predicted to Select for Resistant Bacteria: Proposed Limits for Environmental Regulation. Environ. Int. 86, 140–149. doi: 10.1016/j.envint.2015.10.015
Berman J., Krysan D. J. (2020). Drug Resistance and Tolerance in Fungi. Nat. Rev. Microbiol. 18 (6), 319–331. doi: 10.1038/s41579-019-0322-2
Bhagat J., Singh N., Nishimura N., Shimada Y. (2021). A Comprehensive Review on Environmental Toxicity of Azole Compounds to Fish. Chemosphere 262, 128335. doi: 10.1016/j.chemosphere.2020.128335
Bhattacharya S., Sae-Tia S., Fries B. C. (2020). Candidiasis and Mechanisms of Antifungal Resistance. Antibio. (Basel) 9 (6), 312. doi: 10.3390/antibiotics9060312
Borghi E., Andreoni S., Cirasola D., Ricucci V., Sciota R., Morace G. (2014). Antifungal Resistance Does Not Necessarily Affect Candida Glabrata Fitness. J. Chemother. 26 (1), 32–36. doi: 10.1179/1973947813Y.0000000100
Bottery M. J., Wood A. J., Brockhurst M. A. (2016). Selective Conditions for a Multidrug Resistance Plasmid Depend on the Sociality of Antibiotic Resistance. Antimicrob. Agents Chemother. 60 (4), 2524–2527. doi: 10.1128/AAC.02441-15
Brennan M., Thomas D. Y., Whiteway M., Kavanagh K. (2002). Correlation Between Virulence of Candida Albicans Mutants in Mice and Galleria Mellonella Larvae. FEMS Immunol. Med. Microbiol. 34 (2), 153–157. doi: 10.1111/j.1574-695X.2002.tb00617.x
Brown G. D., Denning D. W., Gow N. A., Levitz S. M., Netea M. G., White T. C. (2012). Hidden Killers: Human Fungal Infections. Sci. Trans. Med. 4 (165), 165rv113. doi: 10.1126/scitranslmed.3004404
Caban M., Strapagiel D., Dziadek J., Korycka-Machala M., Grzelak A. (2016). Principles of a New Protocol for Prediction of Azole Resistance in Candida Albicans Infections on the Basis of ERG11 Polymorphisms. Curr. Microbiol. 73 (2), 172–182. doi: 10.1007/s00284-016-1039-3
Calderon-Preciado D., Jimenez-Cartagena C., Matamoros V., Bayona J. M. (2011). Screening of 47 Organic Microcontaminants in Agricultural Irrigation Waters and Their Soil Loading. Water Res. 45 (1), 221–231. doi: 10.1016/j.watres.2010.07.050
Chaabane F., Graf A., Jequier L., Coste A. T. (2019). Review on Antifungal Resistance Mechanisms in the Emerging Pathogen Candida Auris. Front. Microbiol. 10. doi: 10.3389/fmicb.2019.02788
Chen Z. F., Ying G. G. (2015). Occurrence, Fate and Ecological Risk of Five Typical Azole Fungicides as Therapeutic and Personal Care Products in the Environment: A Review. Environ. Int. 84, 142–153. doi: 10.1016/j.envint.2015.07.022
Chen F., Ying G. G., Kong L. X., Wang L., Zhao J. L., Zhou L. J., et al. (2011). Distribution and Accumulation of Endocrine-Disrupting Chemicals and Pharmaceuticals in Wastewater Irrigated Soils in Hebei, China. Environ. Pollut. 159 (6), 1490–1498. doi: 10.1016/j.envpol.2011.03.016
Chen Z. F., Ying G. G., Liu Y. S., Zhang Q. Q., Zhao J. L., Liu S. S., et al. (2014). Triclosan as a Surrogate for Household Biocides: An Investigation Into Biocides in Aquatic Environments of a Highly Urbanized Region. Water Res. 58, 269–279. doi: 10.1016/j.watres.2014.03.072
Chowdhary A., Meis J. F. (2018). Emergence of Azole Resistant Aspergillus Fumigatus and One Health: Time to Implement Environmental Stewardship. Environ. Microbiol. 20 (4), 1299–1301. doi: 10.1111/1462-2920.14055
Chowdhary A., Sharma C., Hagen F., Meis J. F. (2014). Exploring Azole Antifungal Drug Resistance in Aspergillus Fumigatus With Special Reference to Resistance Mechanisms. Future Microbiol. 9 (5), 697–711. doi: 10.2217/fmb.14.27
Chowdhary A., Tarai B., Singh A., Sharma A. (2020). Multidrug-Resistant Candida Auris Infections in Critically Ill Coronavirus Disease Patients, India, April–July 2020. Emerg. Infect. Dis. 26 (11), 2694. doi: 10.3201/eid2611.203504
Cortes L. G., Marinov D., Sanseverino I., Cuenca A. N., Niegowska M., Rodriguez E., et al. (2020). Selection of Substances for the 3rd Watch List Under the Water Framework Directive (Luxembourg: Office of the European Union).
Cotter G., Doyle S., Kavanagh K. (2000). Development of an Insect Model for the In Vivo Pathogenicity Testing of Yeasts. FEMS Immunol. Med. Microbiol. 27 (2), 163–169. doi: 10.1111/j.1574-695X.2000.tb01427.x
Cowen L. E. (2001). Predicting the Emergence of Resistance to Antifungal Drugs. FEMS Microbiol. Lett. 204 (1), 1–7. doi: 10.1111/j.1574-6968.2001.tb10853.x
Cowen L. E., Anderson J. B., Kohn L. M. (2002). Evolution of Drug Resistance in Candida Albicans. Annu. Rev. Microbiol. 56, 139–165. doi: 10.1146/annurev.micro.56.012302.160907
Enne V. I., Delsol A. A., Davis G. R., Hayward S. L., Roe J. M., Bennett P. M. (2005). Assessment of the Fitness Impacts on Escherichia Coli of Acquisition of Antibiotic Resistance Genes Encoded by Different Types of Genetic Element. J. Antimicrob. Chemother. 56 (3), 544–551. doi: 10.1093/jac/dki255
Escher B. I., Baumgartner R., Koller M., Treyer K., Lienert J., McArdell C. S. (2011). Environmental Toxicology and Risk Assessment of Pharmaceuticals From Hospital Wastewater. Water Res. 45 (1), 75–92. doi: 10.1016/j.watres.2010.08.019
Etlik O., Koksal V., Arican-Baris S. T., Baris I. (2011). Development and Validation of a Cost-Effective in-House Method, Tetra-Primer ARMS PCR Assay, in Genotyping of Seven Clinically Important Point Mutations. Mol. Cell Probes 25 (4), 177–181. doi: 10.1016/j.mcp.2011.04.005
Fisher M. C., Alastruey-Izquierdo A., Berman J., Bicanic T., Bignell E. M., Bowyer P., et al. (2022). Tackling the Emerging Threat of Antifungal Resistance to Human Health. Nat. Rev. Microbiol. 1–15. doi: 10.1038/s41579-022-00720-1
Fisher M. C., Hawkins N. J., Sanglard D., Gurr S. J. (2018). Worldwide Emergence of Resistance to Antifungal Drugs Challenges Human Health and Food Security. Science 360 (6390), 739–742. doi: 10.1126/science.aap7999
Flowers S. A., Barker K. S., Berkow E. L., Toner G., Chadwick S. G., Gygax S. E., et al. (2012). Gain-Of-Function Mutations in UPC2 are a Frequent Cause of ERG11 Upregulation in Azole-Resistant Clinical Isolates of Candida Albicans. Eukaryot Cell 11 (10), 1289–1299. doi: 10.1128/EC.00215-12
Gagneux S., Long C. D., Small P. M., Van T., Schoolnik G. K., Bohannan B. J. (2006). The Competitive Cost of Antibiotic Resistance in Mycobacterium Tuberculosis. Science 312, 1944–1946. doi: 10.1126/science.1124410
Gago S., Garcia-Rodas R., Cuesta I., Mellado E., Alastruey-Izquierdo A. (2014). Candida Parapsilosis, Candida Orthopsilosis, and Candida Metapsilosis Virulence in the Non-Conventional Host Galleria Mellonella. Virulence 5 (2), 278–285. doi: 10.4161/viru.26973
Garthwaite D., Barker I., Ridley L., Mace A., Parrish G., Macarthur R., et al. (2018). “Amenity Pesticide Usage In The United Kingdom 2016.” in Pesticide Usage Survey Report 278.
Gisi U. (2014). Assessment of Selection and Resistance Risk for Demethylation Inhibitor Fungicides in Aspergillus Fumigatus in Agriculture and Medicine: A Critical Review. Pest Manag. Sci. 70 (3), 352–364. doi: 10.1002/ps.3664
Gow N. A. R., Yadav B. (2017). Microbe Profile: Candida Albicans: A Shape-Changing, Opportunistic Pathogenic Fungus of Humans. Microbiol. (Reading) 163 (8), 1145–1147. doi: 10.1099/mic.0.000499
Greenfield B. K., Shaked S., Marrs C. F., Nelson P., Raxter I., Xi C., et al. (2018). Modeling the Emergence of Antibiotic Resistance in the Environment: An Analytical Solution for the Minimum Selection Concentration. Antimicrob. Agents Chemother. 62 (3), e01686–e01617. doi: 10.1128/AAC.01686-17
Gullberg E., Albrecht L. M., Karlsson C., Sandegren L., Andersson D. I. (2014). Selection of a Multidrug Resistance Plasmid by Sublethal Levels of Antibiotics and Heavy Metals. mBio 5 (5), e01918–e01914. doi: 10.1128/mBio.01918-14
Gullberg E., Cao S., Berg O. G., Ilback C., Sandegren L., Hughes D., et al. (2011). Selection of Resistant Bacteria at Very Low Antibiotic Concentrations. PloS Pathog. 7 (7), e1002158. doi: 10.1371/journal.ppat.1002158
Guo H., Xie S. M., Li S. X., Song Y. J., Zhong X. Y., Zhang H. (2017). Involvement of Mitochondrial Aerobic Respiratory Activity in Efflux-Mediated Resistance of C. Albicans to Fluconazole. J. Mycol. Med. 27 (3), 339–344. doi: 10.1016/j.mycmed.2017.04.004
Gygax S. E., Vermitsky J. P., Chadwick S. G., Self M. J., Zimmerman J. A., Mordechai E., et al. (2008). Antifungal Resistance of Candida Glabrata Vaginal Isolates and Development of a Quantitative Reverse Transcription-PCR-Based Azole Susceptibility Assay. Antimicrob. Agents Chemother. 52 (9), 3424–3426. doi: 10.1128/AAC.00462-08
Hill J. A., O'Meara T. R., Cowen L. E. (2015). Fitness Trade-Offs Associated With the Evolution of Resistance to Antifungal Drug Combinations. Cell Rep. 10 (5), 809–819. doi: 10.1016/j.celrep.2015.01.009
Hof H. (2001). Critical Annotations to the Use of Azole Antifungals for Plant Protection. Antimicrob. Agents Chemother. 45 (11), 2987–2990. doi: 10.1128/AAC.45.11.2987-2990.2001
Hof H. (2008). Is There a Serious Risk of Resistance Development to Azoles Among Fungi Due to the Widespread Use and Long-Term Application of Azole Antifungals in Medicine? Drug Resist. Update 11 (1-2), 25–31. doi: 10.1016/j.drup.2008.01.001
Huschek G., Hansen P. D., Maurer H. H., Krengel D., Kayser A. (2004). Environmental Risk Assessment of Medicinal Products for Human Use According to European Commission Recommendations. Environ. Toxicol. An Inter J. 19 (3), 226–240. doi: 10.1002/tox.20015
Jeanvoine A., Rocchi S., Bellanger A. P., Reboux G., Millon L. (2020). Azole-Resistant Aspergillus Fumigatus: A Global Phenomenon Originating in the Environment? Med. Mal. Infect. 50 (5), 389–395. doi: 10.1016/j.medmal.2019.07.014
Kahle M., Buerge I. J., Hauser A., Muller M. D., Poiger T. (2008). Azole Fungicides: Occurrence and Fate in Wastewater and Surface Waters. Environ. Sci. Technol. 42 (19), 7193–7200. doi: 10.1021/es8009309
Kraupner N., Ebmeyer S., Bengtsson-Palme J., Fick J., Kristiansson E., Flach C. F., et al. (2018). Selective Concentration for Ciprofloxacin Resistance in Escherichia Coli Grown in Complex Aquatic Bacterial Biofilms. Environ. Int. 116, 255–268. doi: 10.1016/j.envint.2018.04.029
Kraupner N., Hutinel M., Schumacher K., Gray D. A., Genheden M., Fick J., et al. (2021). Evidence for Selection of Multi-Resistant E. Coli by Hospital Effluent. Environ. Int. 150, 106436. doi: 10.1016/j.envint.2021.106436
Ksiezopolska E., Gabaldon T. (2018). Evolutionary Emergence of Drug Resistance in Candida Opportunistic Pathogens. Genes (Basel) 9 (9), 461. doi: 10.3390/genes9090461
Kwon-Chung K. J., Chang Y. C. (2012). Aneuploidy and Drug Resistance in Pathogenic Fungi. PloS Pathog. 8 (11), e1003022. doi: 10.1371/journal.ppat.1003022
Lago M., Aguiar A., Natario A., Fernandes C., Faria M., Pinto E. (2014). Does Fungicide Application in Vineyards Induce Resistance to Medical Azoles in Aspergillus Species? Environ. Monit. Assess. 186 (9), 5581–5593. doi: 10.1007/s10661-014-3804-8
Le Page G., Gunnarsson L., Snape J., Tyler C. R. (2017). Integrating Human and Environmental Health in Antibiotic Risk Assessment: A Critical Analysis of Protection Goals, Species Sensitivity and Antimicrobial Resistance. Environ. Int. 109, 155–169. doi: 10.1016/j.envint.2017.09.013
Letzel M., Metzner G., Letzel T. (2009). Exposure Assessment of the Pharmaceutical Diclofenac Based on Long-Term Measurements of the Aquatic Input. Environ. Int. 35 (2), 363–368. doi: 10.1016/j.envint.2008.09.002
Lindberg R. H., Fick J., Tysklind M. (2010). Screening of Antimycotics in Swedish Sewage Treatment Plants–Waters and Sludge. Water Res. 44 (2), 649–657. doi: 10.1016/j.watres.2009.10.034
Liu J., Lu G., Xie Z., Zhang Z., Li S., Yan Z. (2015). Occurrence, Bioaccumulation and Risk Assessment of Lipophilic Pharmaceutically Active Compounds in the Downstream Rivers of Sewage Treatment Plants. Sci. Total Environ. 511, 54–62. doi: 10.1016/j.scitotenv.2014.12.033
Liu J.-L., Wong M.-H. (2013). Pharmaceuticals and Personal Care Products (PPCPs): A Review on Environmental Contamination in China. Environ. Int. 59, 208–224. doi: 10.1016/j.envint.2013.06.012
Lockhart S. R., Etienne K. A., Vallabhaneni S., Farooqi J., Chowdhary A., Govender N. P., et al. (2017). Simultaneous Emergence of Multidrug-Resistant Candida Auris on 3 Continents Confirmed by Whole-Genome Sequencing and Epidemiological Analyses. Clin. Infect. Dis. 64 (2), 134–140. doi: 10.1093/cid/ciw691
MacLean R. C., Hall A. R., Perron G. G., Buckling A. (2010). The Population Genetics of Antibiotic Resistance: Integrating Molecular Mechanisms and Treatment Contexts. Nat. Rev. Genet. 11 (6), 405–414. doi: 10.1038/nrg2778
Medaney F., Dimitriu T., Ellis R. J., Raymond B. (2016). Live to Cheat Another Day: Bacterial Dormancy Facilitates the Social Exploitation of β-Lactamases. ISME J. 10 (3), 778–787. doi: 10.1038/ismej.2015.154
Melnyk A. H., Wong A., Kassen R. (2015). The Fitness Costs of Antibiotic Resistance Mutations. Evol. Appl. 8 (3), 273–283. doi: 10.1111/eva.12196
Mesa-Arango A. C., Forastiero A., Bernal-Martínez L., Cuenca-Estrella M., Mellado E., Zaragoza O. (2013). The Non-Mammalian Host Galleria Mellonella can be Used to Study the Virulence of the Fungal Pathogen Candida Tropicalis and the Efficacy of Antifungal Drugs During Infection by This Pathogenic Yeast. Med. Mycol. 51 (5), 461–472. doi: 10.3109/13693786.2012.737031
Michon A., Allou N., Chau F., Podglajen I., Fantin B., Cambau E. (2011). Plasmidic Qnra3 Enhances Escherichia Coli Fitness in Absence of Antibiotic Exposure. PloS One 6 (9), e24552. doi: 10.1371/journal.pone.0024552
More T. T., Yan S., Tyagi R. D., Surampalli R. Y. (2010). Potential Use of Filamentous Fungi for Wastewater Sludge Treatment. Bioresour. Technol. 101 (20), 7691–7700. doi: 10.1016/j.biortech.2010.05.033
Morschhauser J. (2016). The Development of Fluconazole Resistance in Candida Albicans - an Example of Microevolution of a Fungal Pathogen. J. Microbiol. 54 (3), 192–201. doi: 10.1007/s12275-016-5628-4
Morschhäuser J., Barker K. S., Liu T. T., Blaß-Warmuth J., Homayouni R., Rogers P. D. (2007). The Transcription Factor Mrr1p Controls Expression of the MDR1 Efflux Pump and Mediates Multidrug Resistance in Candida Albicans. PloS Pathog. 3 (11), e164. doi: 10.1371/journal.ppat.0030164
Moye-Rowley W. S. (2020). Linkage Between Genes Involved in Azole Resistance and Ergosterol Biosynthesis. PloS Pathog. 16 (9), e1008819. doi: 10.1371/journal.ppat.1008819
Murray A. K. (2017). Selection for Antibiotic Resistance in the Aquatic Environment: Novel Assays to Detect Effect Concentrations of Micropollutants. (Doctoral dissertation, University of Exeter).
Murray A. K. (2020). The Novel Coronavirus COVID-19 Outbreak: Global Implications for Antimicrobial Resistance. Front. Microbiol. 11. doi: 10.3389/fmicb.2020.01020
Murray A. K., Stanton I., Gaze W. H., Snape J. (2021). Dawning of a New ERA: Environmental Risk Assessment of Antibiotics and Their Potential to Select for Antimicrobial Resistance. Water Res. 200, 117233. doi: 10.1016/j.watres.2021.117233
Murray A. K., Stanton I. C., Wright J., Zhang L., Snape J., Gaze W. H. (2020). The 'Selection End Points in Communities of Bacteria' (SELECT) Method: A Novel Experimental Assay to Facilitate Risk Assessment of Selection for Antimicrobial Resistance in the Environment. Environ. Health Perspect. 128 (10), 107007. doi: 10.1289/EHP6635
Murray A. K., Zhang L., Yin X., Zhang T., Buckling A., Snape J., et al. (2018). Novel Insights Into Selection for Antibiotic Resistance in Complex Microbial Communities. mBio 9 (4), e00969–18. doi: 10.1128/mBio.00969-18
Nakamura K., Niimi M., Niimi K., Holmes A. R., Yates J. E., Decottignies A., et al. (2001). Functional Expression of Candida Albicans Drug Efflux Pump Cdr1p in a Saccharomyces Cerevisiae Strain Deficient in Membrane Transporters. Antimicrob. Agents Chemother. 45 (12), 3366–3374. doi: 10.1128/AAC.45.12.3366-3374.2001
Niu L., Li Y., Xu L., Wang P., Zhang W., Wang C., et al. (2017). Ignored Fungal Community in Activated Sludge Wastewater Treatment Plants: Diversity and Altitudinal Characteristics. Environ. Sci. Pollut. Res. Int. 24 (4), 4185–4193. doi: 10.1007/s11356-016-8137-4
O'Neill J. (2016). “Review on Antimicrobial Resistance: Tackling Drug-Resistant Infections Globally: Final Report and Recommendations. Review on Antimicrobial Resistance,” in Tackling Drug-Resistant Infections Globally: Final Report and Recommendations. (London: Wellcome Trust and HM Government), Available online at: www.amr-review.org
Odds F. C., Brown A. J., Gow N. A. (2003). Antifungal Agents: Mechanisms of Action. Trends Microbiol. 11 (6), 272–279. doi: 10.1016/s0966-842x(03)00117-3
O’Neill J. (2015). Antimicrobials in Agriculture and the Environment: Reducing Unnecessary Use and Waste (The Review on Antimicrobial Resistance), 40. London: Wellcome Trust. Available online at: www.amr-review.org
Paul S., Dadwal R., Singh S., Shaw D., Chakrabarti A., Rudramurthy S. M., et al. (2021). Rapid Detection of ERG11 Polymorphism Associated Azole Resistance in Candida Tropicalis. PloS One 16 (1), e0245160. doi: 10.1371/journal.pone.0245160
Peng X., Huang Q., Zhang K., Yu Y., Wang Z., Wang C. (2012). Distribution, Behavior and Fate of Azole Antifungals During Mechanical, Biological, and Chemical Treatments in Sewage Treatment Plants in China. Sci. Total Environ. 426, 311–317. doi: 10.1016/j.scitotenv.2012.03.067
Perlin D. S., Rautemaa-Richardson R., Alastruey-Izquierdo A. (2017). The Global Problem of Antifungal Resistance: Prevalence, Mechanisms, and Management. Lancet Infect. Dis. 17 (12), e383–e392. doi: 10.1016/S1473-3099(17)30316-X
Ren J., Jin X., Zhang Q., Zheng Y., Lin D., Yu Y. (2017). Fungicides Induced Triazole-Resistance in Aspergillus Fumigatus Associated With Mutations of TR46/Y121F/T289A and its Appearance in Agricultural Fields. J. Hazard. Mater. 326, 54–60. doi: 10.1016/j.jhazmat.2016.12.013
Revie N. M., Iyer K. R., Robbins N., Cowen L. E. (2018). Antifungal Drug Resistance: Evolution, Mechanisms and Impact. Curr. Opin. Microbiol. 45, 70–76. doi: 10.1016/j.mib.2018.02.005
Rhodes J., Abdolrasouli A., Dunne K., Sewell T. R., Zhang Y., Ballard E., et al. (2022). Population Genomics Confirms Acquisition of Drug-Resistant Aspergillus Fumigatus Infection by Humans From the Environment. Nat. Microbiol. 7, 663–674. doi: 10.1038/s41564-022-01091-2
Richter E., Wick A., Ternes T. A., Coors A. (2013). Ecotoxicity of Climbazole, a Fungicide Contained in Antidandruff Shampoo. Environ. Toxicol. Chem. 32 (12), 2816–2825. doi: 10.1002/etc.2367
Sagatova A. A., Keniya M. V., Wilson R. K., Monk B. C., Tyndall J. D. (2015). Structural Insights Into Binding of the Antifungal Drug Fluconazole to Saccharomyces Cerevisiae Lanosterol 14α-Demethylase. Antimicrob. Agents Chemother. 59 (8), 4982–4989. doi: 10.1128/AAC.00925-15
Sanseverino I., Navarro Cuenca A., Loos R., Marinov D., Lettieri T. (2018). State of the Art on the Contribution of Water to Antimicrobial Resistance (Brussels: European Union).
Scorzoni L., de Lucas M. P., Mesa-Arango A. C., Fusco-Almeida A. M., Lozano E., Cuenca-Estrella M., et al. (2013). Antifungal Efficacy During Candida Krusei Infection in non-Conventional Models Correlates With the Yeast In Vitro Susceptibility Profile. PloS One 8 (3), e60047. doi: 10.1371/journal.pone.0060047
Sharma C., Hagen F., Moroti R., Meis J. F., Chowdhary A. (2015). Triazole-Resistant Aspergillus Fumigatus Harbouring G54 Mutation: Is it De Novo or Environmentally Acquired? J. Glob. Antimicrob. Resist. 3 (2), 69–74. doi: 10.1016/j.jgar.2015.01.005
Singh A. K., Singh R., Joshi S. R., Misra A. (2021). Mucormycosis in COVID-19: A Systematic Review of Cases Reported Worldwide and in India. Diabetes Metab. Syndrome 15 (4), 102146. doi: 10.1016/j.dsx.2021.05.019
Sionov E., Lee H., Chang Y. C., Kwon-Chung K. J. (2010). Cryptococcus Neoformans Overcomes Stress of Azole Drugs by Formation of Disomy in Specific Multiple Chromosomes. PloS Pathog. 6 (4), e1000848. doi: 10.1371/journal.ppat.1000848
Stanton I. C., Murray A. K., Zhang L., Snape J., Gaze W. H. (2020). Evolution of Antibiotic Resistance at Low Antibiotic Concentrations Including Selection Below the Minimal Selective Concentration. Commun. Biol. 3 (1), 467. doi: 10.1038/s42003-020-01176-w
Steingrimsdottir H., Beare D., Cole J., Leal J. F., Kostic T., Lopez-Barea J., et al. (1996). Development of New Molecular Procedures for the Detection of Genetic Alterations in Man. Mutat. Res. 353 (1-2), 109–121. doi: 10.1016/0027-5107(95)00244-8
Tell J., Caldwell D. J., Häner A., Hellstern J., Hoeger B., Journel R., et al. (2019). Science-Based Targets for Antibiotics in Receiving Waters From Pharmaceutical Manufacturing Operations. Integrated Environ. Assess. Manage. 15 (3), 312–319. doi: 10.1002/ieam.4141
Trojer M. A., Movahedi A., Blanck H., Nydén M. (2013). Imidazole and Triazole Coordination Chemistry for Antifouling Coatings. J. Chem. 10, 11. doi: 10.1155/2013/946739
Valasek M. A., Repa J. J. (2005). The Power of Real-Time PCR. Adv. Physiol. Educ. 29 (3), 151–159. doi: 10.1152/advan.00019.2005
Van Dijck P., Sjollema J., Cammue B. P., Lagrou K., Berman J., d'Enfert C., et al. (2018). Methodologies for In Vitro and In Vivo Evaluation of Efficacy of Antifungal and Antibiofilm Agents and Surface Coatings Against Fungal Biofilms. Microb. Cell 5 (7), 300–326. doi: 10.15698/mic2018.07.638
Verweij P. E., Snelders E., Kema G. H., Mellado E., Melchers W. J. (2009). Azole Resistance in Aspergillus Fumigatus: A Side-Effect of Environmental Fungicide Use? Lancet Infect. Dis. 9 (12), 789–795. doi: 10.1016/S1473-3099(09)70265-8
Verweij P. E., Zhang J., Debets A. J. M., Meis J. F., van de Veerdonk F. L., Schoustra S. E., et al. (2016). In-Host Adaptation and Acquired Triazole Resistance in Aspergillus Fumigatus: A Dilemma for Clinical Management. Lancet Infect. Dis. 16 (11), e251–e260. doi: 10.1016/S1473-3099(16)30138-4
Vincent B. M., Lancaster A. K., Scherz-Shouval R., Whitesell L., Lindquist S. (2013). Fitness Trade-Offs Restrict the Evolution of Resistance to Amphotericin B. PloS Biol. 11 (10), e1001692. doi: 10.1371/journal.pbio.1001692
Whaley S. G., Berkow E. L., Rybak J. M., Nishimoto A. T., Barker K. S., Rogers P. D. (2016). Azole Antifungal Resistance in Candida Albicans and Emerging Non-Albicans Candida Species. Front. Microbiol. 7. doi: 10.3389/fmicb.2016.02173
Young L. Y., Hull C. M., Heitman J. (2003). Disruption of Ergosterol Biosynthesis Confers Resistance to Amphotericin B in Candida Lusitaniae. Antimicrob. Agents Chemother. 47 (9), 2717–2724. doi: 10.1128/AAC.47.9.2717-2724.2003
Yurtsev E. A., Chao H. X., Datta M. S., Artemova T., Gore J. (2013). Bacterial Cheating Drives the Population Dynamics of Cooperative Antibiotic Resistance Plasmids. Mol. Syst. Biol. 9 (1), 683. doi: 10.1038/msb.2013.39
Zhang H., Feng J., Chen S., Li B., Sekar R., Zhao Z., et al. (2018). Disentangling the Drivers of Diversity and Distribution of Fungal Community Composition in Wastewater Treatment Plants Across Spatial Scales. Front. Microbiol. 9. doi: 10.3389/fmicb.2018.01291
Keywords: antifungal resistance, antifungals, antimicrobial resistance, experimental evolution, selection, minimal selective concentration, fungi
Citation: Stevenson EM, Gaze WH, Gow NAR, Hart A, Schmidt W, Usher J, Warris A, Wilkinson H and Murray AK (2022) Antifungal Exposure and Resistance Development: Defining Minimal Selective Antifungal Concentrations and Testing Methodologies. Front. Fungal Biol. 3:918717. doi: 10.3389/ffunb.2022.918717
Received: 12 April 2022; Accepted: 16 May 2022;
Published: 13 June 2022.
Edited by:
Robert T. Wheeler, University of Maine, United StatesReviewed by:
Renátó Kovács, University of Debrecen, HungaryJoão Brandão, Instituto Nacional de Saúde Doutor Ricardo Jorge (INSA), Portugal
Copyright © 2022 Stevenson, Gaze, Gow, Hart, Schmidt, Usher, Warris, Wilkinson and Murray. This is an open-access article distributed under the terms of the Creative Commons Attribution License (CC BY). The use, distribution or reproduction in other forums is permitted, provided the original author(s) and the copyright owner(s) are credited and that the original publication in this journal is cited, in accordance with accepted academic practice. No use, distribution or reproduction is permitted which does not comply with these terms.
*Correspondence: Aimee K. Murray, YS5rLm11cnJheUBleGV0ZXIuYWMudWs=